Notes
Article history
The research reported in this issue of the journal was funded by the HS&DR programme or one of its preceding programmes as project number 12/136/105. The contractual start date was in September 2013. The final report began editorial review in October 2017 and was accepted for publication in July 2018. The authors have been wholly responsible for all data collection, analysis and interpretation, and for writing up their work. The HS&DR editors and production house have tried to ensure the accuracy of the authors’ report and would like to thank the reviewers for their constructive comments on the final report document. However, they do not accept liability for damages or losses arising from material published in this report.
Declared competing interests of authors
John Marsden acknowledges research grants from the Department of Health and Social Care, the National Institute for Health Research (NIHR) and the NIHR Biomedical Research Centre for Mental Health at South London and Maudsley NHS Mental Health Foundation Trust, and part-time employment as Senior Academic Advisor for the Alcohol, Drugs and Tobacco Division, Health and Wellbeing Directorate, Public Health England. He declares investigator-led educational grant funding from Indivior PLC (administered by Action on Addiction) for a study of adjunctive, personalised psychosocial intervention for non-response to opioid agonist treatment (ARC Trial), and support from NIHR (Health Technology Assessment) for a trial of extended-release naltrexone. He has received honoraria from Merck Serono (Darmstadt, Germany; 2015; clinical oncology medicine); Martindale Pharma (Brentwood, UK; 2017; treatment for opioid use disorder); and Indivior PLC (via PCM Scientific) as co-chairperson and chairperson (2015–18) for the conference on Improving Outcomes in Treatment of Opioid Dependence. Tim Millar has received research funding from the UK National Treatment Agency for Substance Misuse, Public Health England and the Home Office. He has been a member of the organising committee for conferences supported by unrestricted educational grants from Reckitt Benckiser Group plc (Slough, UK), Lundbeck Ltd (Milton Keynes, UK), Martindale Pharma and Britannia Pharmaceuticals Ltd (Reading, UK), for which he received no personal remuneration. He is a member of the UK Advisory Council on the Misuse of Drugs. John Strang is a clinician and researcher and has worked extensively with agencies in the addiction treatment fields and addiction-related charities and with government departments and has contributed to clinical guidelines on treatment types and provision. John Strang’s employer (King’s College London) has received, connected to his work, project grant support and/or honoraria and/or consultancy payments from the Department of Health and Social Care, the National Treatment Agency, Public Health England, the Home Office, the National Institute for Health and Care Excellence and the European Monitoring Centre for Drugs and Drug Addiction (EMCDDA), as well as research grants from (2016–18) NIHR, the Medical Research Council and the Pilgrim Trust. He has also worked with the World Health Organization, the United Nations Office on Drugs and Crime, EMCDDA, the US Food and Drug Administration and the US National Institute on Drug Abuse, as well as other international government agencies. John Strang’s employer (King’s College London) has also received, connected to his work, research grant support and/or payment of honoraria, consultancy payments and expenses from pharmaceutical companies [including, for 2016–18, Martindale, Indivior PLC, Mundipharma (Cambridge, UK) and Braeburn/Camurus (Lund, Sweden)] and trial medication supply from iGen and Braeburn. John Strang’s employer (King’s College London) has registered intellectual property on an innovative buccal naloxone with which John Strang has been named in a patent registration for concentrated naloxone nasal spray by Euro-Celtique SA on behalf of Mundipharma Research Limited. For updated information see www.kcl.ac.uk/ioppn/depts/addictions/people/hod.aspx (accessed 2 October 2018). Matthew Hickman has received unrelated unrestricted honoraria from Gilead Sciences, Inc. (Cambridge, UK), AbbVie (Maidenhead, UK), Janssen Pharmaceuticals (High Wycombe, UK) and Merck Serono. Matthew Hickman is a member of the Public Health Research Research Funding Board.
Permissions
Copyright statement
© Queen’s Printer and Controller of HMSO 2019. This work was produced by Steer et al. under the terms of a commissioning contract issued by the Secretary of State for Health and Social Care. This issue may be freely reproduced for the purposes of private research and study and extracts (or indeed, the full report) may be included in professional journals provided that suitable acknowledgement is made and the reproduction is not associated with any form of advertising. Applications for commercial reproduction should be addressed to: NIHR Journals Library, National Institute for Health Research, Evaluation, Trials and Studies Coordinating Centre, Alpha House, University of Southampton Science Park, Southampton SO16 7NS, UK.
2019 Queen’s Printer and Controller of HMSO
Chapter 1 Context
Background
Opiate substitution treatment (OST) is the key treatment for heroin dependence and has been shown to have multiple benefits, including reducing drug-related crime and blood-borne virus transmission and improving social functioning, as well as reducing drug-related deaths. 1–5 Prescribed OST in the community includes primarily methadone but also buprenorphine and occasionally dihydrocodeine. 1,6 Clinical guidance advises that the choice of drug should take account of a number of factors, including retention and treatment compliance, the patient’s preference and the clinician’s experience with prescribing these drugs. 7,8 When the choice is unclear, methadone should be prescribed as the first choice. 8 In England there are approximately 350,000 opioid-dependent people and 150,000 people who inject drugs, although these estimates are uncertain as they can vary substantially by method of derivation and by source of information. 9–12 Of these people, about half are exposed to drug treatment annually, mainly OST (75%) but also non-pharmacological treatments delivered in specialist drug agencies and residential units. 9 OST is effective because it reduces illicit opioid drug consumption, in particular drugs used through injections, and is cost-effective because of the subsequent reductions in drug-related crime and health harms. 13 Observational studies14–18 have shown that the risk of mortality is reduced during OST. Buprenorphine, hypothetically, is less likely than methadone19 to cause fatal overdose, and in some studies it has been shown to be as good as methadone at treatment retention,20 although in others the dropout rates were higher. 16,21–23 In France, ecological analyses suggest that trends in overdose deaths are negatively associated with increases in buprenorphine prescription. 24 However, there is little direct comparative evidence on the risk of death during buprenorphine versus methadone treatment, and none in the UK.
Several recent studies15–17,25 have highlighted that there is a period of very high mortality risk in the first few months immediately after treatment cessation, which is at least eight times higher than the mortality risk during treatment. In the UK, analyses of primary care information have suggested that the risk of death in those who receive OST is twice as high among men as among women, is raised at the beginning and end of treatment, and may be higher in those co-prescribed benzodiazepines. 15,26 In Australia, the risk of death at treatment onset was greater than in the UK, with some evidence to suggest that the mortality risk at treatment onset was lower among those initiated on to buprenorphine than those initiated on to methadone, but these benefits may be offset by a shorter duration of treatment for those on buprenorphine than for those on methadone. 16 The evidence base for other drug treatments (residential rehabilitation, detoxification, and psychological treatments) is more limited, but studies also suggest that the risk of mortality is reduced during treatment compared with out of treatment, and is elevated within the first 30 days compared with > 30 days after treatment ceases. 17,27
The effect of prescribing other medications to OST patients has also been studied. Research to date has focused on benzodiazepines, z-drugs (zolpidem, zopiclone and zaleplon) and gabapentinoids (gabapentin and pregabalin). Here, studies26,28–33 have suggested that the prescription of these medications may increase the risk of mortality. Despite this, and against treatment guidelines,34,35 many OST patients are prescribed these medications. 28,29
Other aspects of treatment may also be important, such as additional psychological support, supervised consumption, titration up and tapering down of OST doses at the beginning and end of treatment, and take-home naloxone. 35 Clinical guidance recommends that, at the initiation of OST, patients are started on low levels of methadone or buprenorphine and then steadily increased to achieve an optimum level of prescription while minimising the risk of overdose. 7,36 The planned discharge of patients should involve gradually tapering doses to low levels while minimising withdrawal symptoms. Supervised consumption, at least initially, also is recommended to ensure compliance and reduce diversion.
In the UK, OST has expanded fivefold in the past 10 years to > 1800 kg per year (≈33.3 million doses per year). 37 The rate of methadone deaths per gram of methadone prescribed has fallen, coinciding with and attributed to the issuing and implementation of clinical guidelines recommending greater supervised consumption;38 however, the overall number of opiate-related deaths has not declined. 39,40
Need for further research
The management and expectations of drug treatment are evolving, with the current drug strategy and treatment guidance focusing on steps to improve ‘recovery’. 41 Evidence, however, on the impact of different ways of delivering treatment on drug-related mortality is limited,40 and this is essential for current and future policy. It is not the intention of current treatment guidelines to reduce patients’ access to, or limit the duration of, OST. Indeed, the latest guidance emphasises that ‘it is not acceptable to leave people on OST without actively supporting their recovery and regularly reviewing the benefits of their treatment . . . Nor is it acceptable to impose time limits on their treatment that take no account of individual history, needs and circumstances, or the benefits of continued treatment’. 41 Many of the sentiments expressed in this document are echoed in the latest Home Office guidelines. 42 Nonetheless, user groups do fear that a reduction in treatment duration may be a consequence of the promotion of recovery in some local areas. Clinical guidance also has been reissued since this study was completed, emphasising the importance of adjunct therapies (psychosocial support) to retain people in OST, prevent chaotic dropout and achieve multiple benefits of OST. 43 In addition, the guidance supports ‘a more explicit focus on individually defined recovery journeys with an enhanced focus on keyworking and care planning that integrates support for pharmacological and psychosocial interventions, and peer engagement and mutual aid’. OST is primarily delivered in the community through primary care, often in shared care arrangements with drug agencies or solely in the care of community drug agencies. 30,44 Earlier studies have examined mortality risk in and out of treatment utilising routine data collected from community drug agencies [National Drug Treatment Monitoring System (NDTMS)]. 30 However, NDTMS data may not record accurately the start and end of OST and historically NDTMS does not collect data on the dose or type of OST. In this study, therefore, we used Clinical Practice Research Datalink (CPRD) data to examine the mortality risk of patients with an opioid disorder in primary care.
Large-scale observational studies, combined with mathematical modelling, are the most feasible approach by which to address questions about mortality risk. Published trials of OST are underpowered and rarely measure mortality risk, making synthesis of their findings unlikely to be informative. 5 There are few large-scale existing observational cohorts that reliably and adequately measure the relevant outcomes and exposures18 and no large-scale ‘head-to-head’ comparisons of buprenorphine versus methadone treatment. In previous studies in the UK, there was insufficient power to detect differences in the risk of death for patients on methadone or buprenorphine, and information on specific causes of death was unavailable, prohibiting the investigation of drug-related deaths. 15,26 In addition, the exposure (and the effect) of the co-prescription of benzodiazepines or other drugs was examined in only one study in Scotland. 26 Careful consideration of confounders in the analysis of observational cohorts, however, will be important. One of the key analyses is to compare mortality risk between people prescribed buprenorphine or methadone (i.e. OST modality), and it is likely that the characteristics of opiate users prescribed buprenorphine or methadone will differ, which may be lost in simple comparisons of the risk of death by OST modality exposure. 45 Propensity score methods may be helpful in providing a more robust comparison of modality than simple covariate adjustment. 46,47 More recently, self-controlled case series (SCCS) methods have been developed, which may also be helpful in addressing these issues of residual confounding. 48–50
Thus, previous analyses need to be expanded to assess the effect of OST on drug-related poisoning (DRP) (as well as overall mortality), to assess how changing the delivery of treatment may influence the risk of death, and to use different methods to test and address issues of confounding.
Chapter 2 Aims and objectives
Our overarching hypotheses are that the impact of OST in reducing deaths from DRP is influenced by modifiable treatment-related factors (such as treatment duration, co-prescription of other drugs, treatment modality and dose, and dispensing arrangements). In addition, the benefits of OST in reducing drug-related deaths in the population may be outweighed or balanced out by other factors that increase the risk of mortality for subgroups of opioid users or for other aspects of treatment.
The aim of the study, therefore, is to add to the body of robust evidence concerning treatment-related factors to inform and improve treatment guidelines that can underpin the effective reduction of population drug-related deaths through primary-care-based intervention. This project will focus on the analysis of OST delivered in primary care using the analysis data from the CPRD.
Our main research questions and goals to be addressed include:
-
Is there evidence that the delivery of OST in primary care has changed over time – specifically in terms of changes in average dose and percentages of patients receiving optimal OST dose; OST modality; number and percentages of patients undergoing a planned discharged over time; number and percentages of patients receiving co-prescription of benzodiazepines; and number and percentages of patients with evidence of supervised consumption? [work package (WP) 1]
-
We shall determine the risk of overdose and death by treatment exposure and modality, relate these effects to the number of overdose deaths and coverage and duration of drug treatment in the community, and project what factors could reduce the number of drug-related deaths in the population. (WP 2)
-
Does any difference in the risk of mortality between prescribed methadone or buprenorphine change with the period of treatment exposure? (WP 2)
-
Is the risk of mortality increased in people who are co-prescribed benzodiazepines, z-drugs or gabapentinoids (pregabalin and gabapentin)? (WP 3)
-
Is the risk of death at treatment onset and cessation reduced in patients with evidence of supervised consumption and planned discharge? (WP 4)
-
Adapt the SCCS methodology, originally developed for assessing vaccinations on health outcomes, for use in medication-based treatment. (WP 5)
Work packages 1–5 are reported in Chapters 4–8, respectively.
Chapter 3 Methodology
This chapter describes all aspects of study design, data collection and data analysis.
Conceptual framework of study
Natural experiments or observational studies provide opportunities to study interventions for which ethical considerations may prevent a controlled experiment such as a randomised controlled trial. 45 Publicly accessible databases, such as CPRD,51 provide a cost-effective strategy for investigating interventions in a UK-representative sample. However, the lack of control in the study design can introduce bias into the results, and care is needed when analysing these data. 45
Clinical Practice Research Datalink
The CPRD is a large database of anonymised patient records from 674 general practices and > 11 million patients in UK, covering 7% of the UK population at the time of this study. It is broadly representative of the UK in terms of sociodemographic characteristics and has good validity and replicability in relation to chronic illness. 52
The Clinical Practice Research Datalink can provide information on the patient (gender, year of birth, registration and transfer out dates with the primary care practice, and date of death if applicable), their history of prescriptions (including daily dose and duration), entries in the clinical notes (including information on comorbid conditions and psychosocial adversity), the prescribing general practitioner (GP) (via anonymous identifier) and the primary care practice (region, date after which data are considered up to standard, and last data collection date by CPRD). 51
Study design
This study was a prospective observation study using CPRD. Data were extracted on patients prescribed methadone or buprenorphine in primary care between 1 January 1998 and 31 July 2014. These data were expanded to include patients prescribed dihydrocodeine when the clinical notes indicated evidence of substance abuse. Patients were eligible for inclusion in the study if they were aged 15–64 years at entry. Patients were followed up from the latest of the study start date, the patient registration date or the up-to-standard date. Follow-up ended at the earliest of the study end date, the last data collection date, the transfer out date, the date of death or 1 year after the last OST ended. OST patients were defined as having been prescribed a daily dose of at least 20 mg of methadone, 4 mg of buprenorphine or 480 mg of dihydrocodeine.
Participants
The data extracted consisted of all patients prescribed methadone or buprenorphine between the study dates with additional patients prescribed dihydrocodeine when there was some evidence of drug abuse from the clinical notes. Thus, the initial data set consisted of 50,151 patients, of whom 49,729 were prescribed methadone or buprenorphine at some time during the study. It was recognised that a large proportion of these were receiving treatment for pain relief. To remedy this, patient histories excluded any prescriptions on which the prescription text contained ‘pain’ (mainly affecting dihydrocodeine), where the form of medication was patches (exclusively affecting buprenorphine) or when the prescription preceded the start of OST (exclusively affecting dihydrocodeine). This led to 53% of the patients being excluded (Figure 1). With 20% excluded on dose criteria, the final number of valid patients was 13,005 (26%).
FIGURE 1.
Flow chart of patients, episodes and deaths included in this study. This figure shows the numbers of episodes, patients and deaths for the all-cause, drug-related and other, non-drug-related, data sets. Prescriptions for pain relief were identified based on prescription text, medication in the form of patches or episodes of dihydrocodeine prior to starting OST. The follow-up period varied by patient and reflected a combination of the study period (January 1998 to July 2014), the patient registration period with the primary care practice, the CPRD usable data date and 1 year after the last treatment ended.
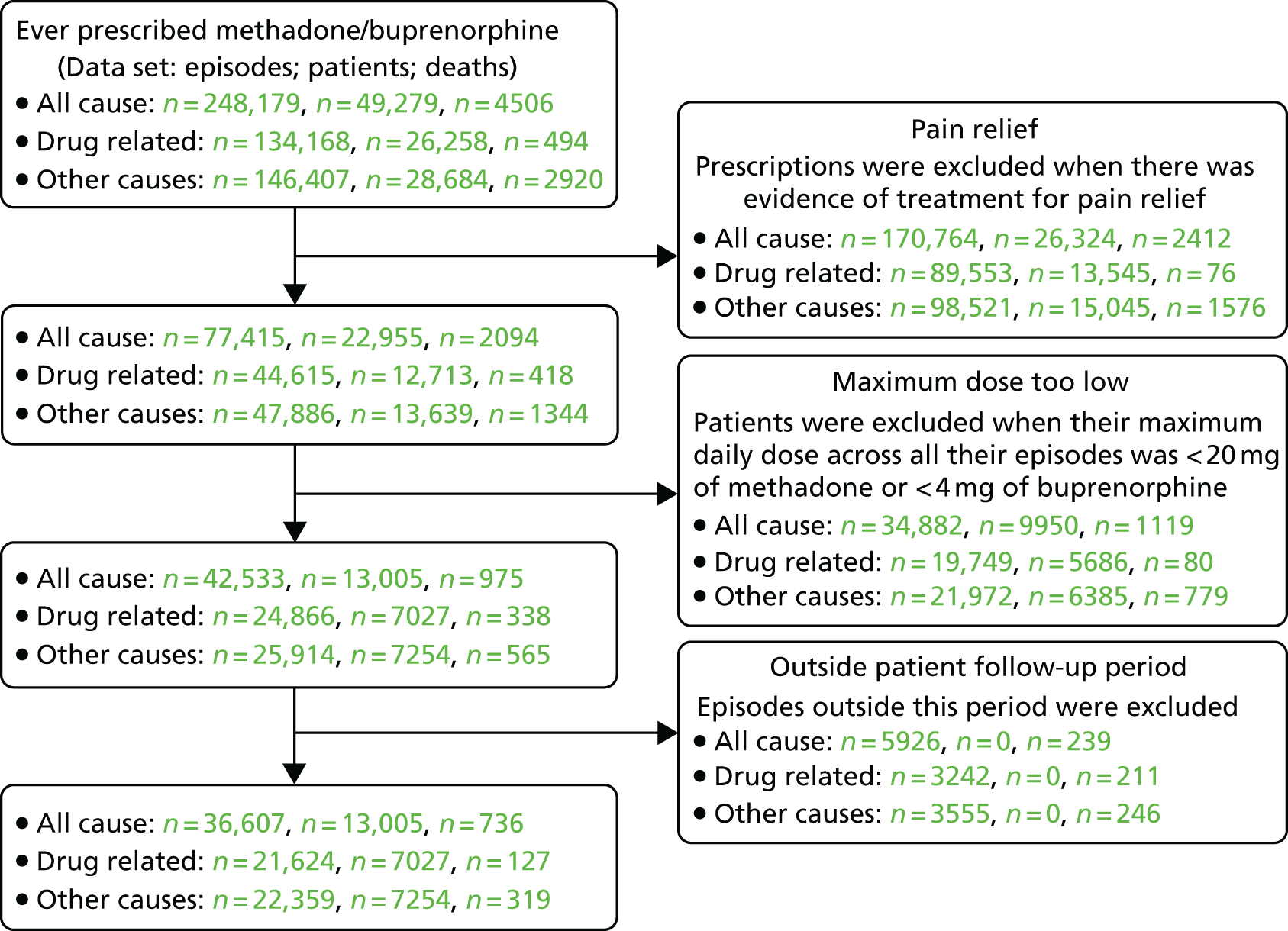
The number of patients varied between the WPs. The various exclusion criteria and the data relevant to each WP are shown in Figure 2.
FIGURE 2.
Relationship between the WPs in this study. B, buprenorphine; M, methadone; n/a, not applicable. WP 1 comprised the most data of all WPs, primarily because of the inclusion of dihydrocodeine episodes. Mortality was not analysed in this WP. The numbers quoted reflect minima used in the analyses of optimal doses. Other outcomes, such as the prevalence of OST, used the full OST data set. Other WPs restricted data to methadone and buprenorphine only, with WP 3 using the most data (any periods relating to these medications) and WP 2 using the fewest data (episodes of only one medication). The exclusion of periods > 1 year after the cessation of treatment for each episode (immortal time bias) affected the person-years at risk but not the number of deaths. The numbers for WP 4 reflect the combined total of all analyses, although in practice initiation and cessation used different data sets. Deaths relating to other non-drug-related causes were analysed in WP 3 only.
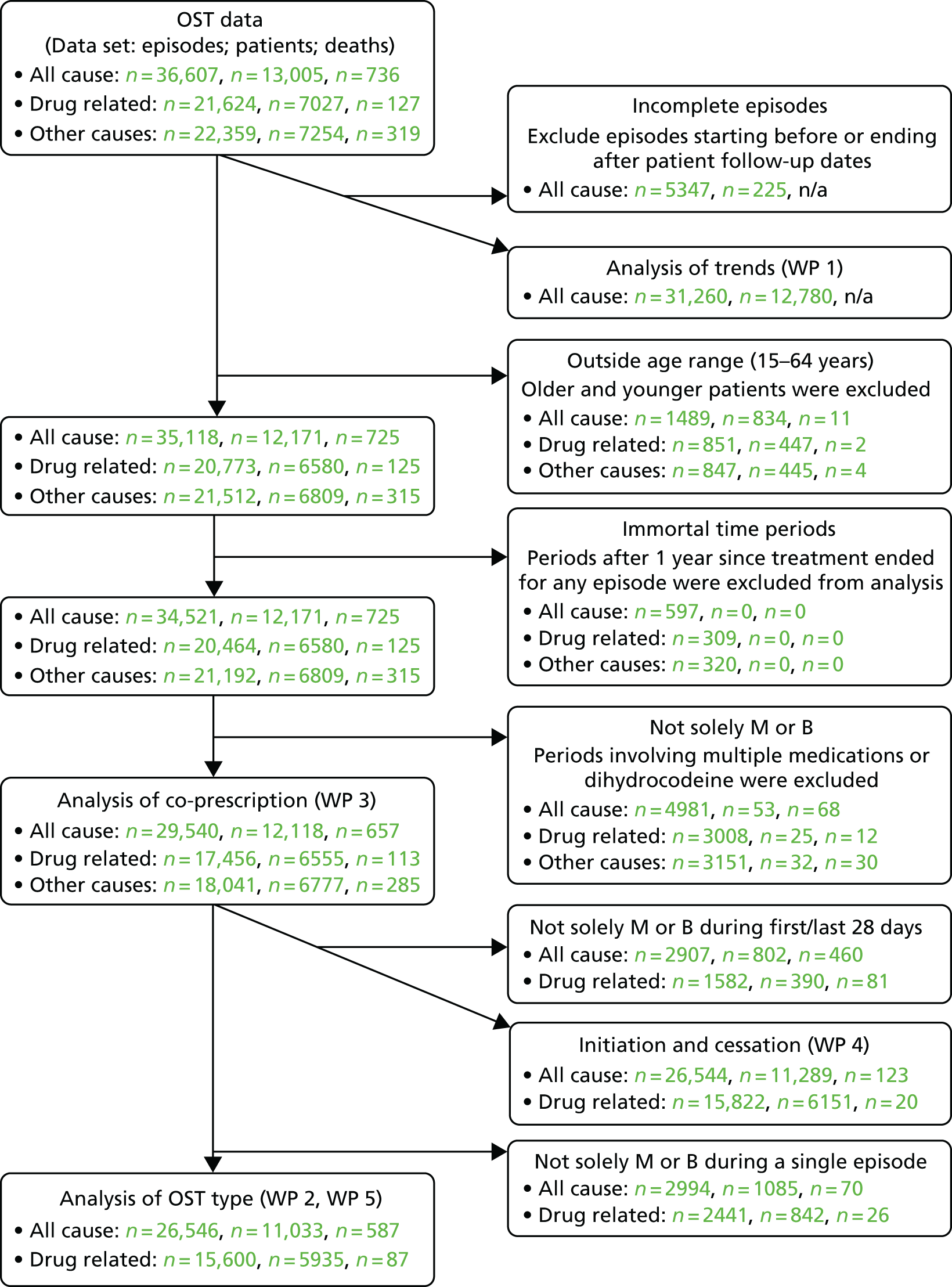
Prescription daily doses, prescription duration and treatment episodes
Information on total quantity was present for 99.9% of prescriptions, reducing to 28% for daily doses and 9.1% for duration. Overall, complete information was available for only 2.2% of prescriptions, a further 32% having two items from which the third could be derived. To estimate the missing information for the remaining 66%, prescription patterns (such as other proximal prescriptions of the same quantity or trends in dosage history) were used to estimate daily dose, or prescription intervals were used to estimate prescription duration.
Opiate substitution treatment episodes were derived from patient histories when a gap of > 28 days existed between the expected completion of one prescription and the start of the next (all WPs). The CPRD sequence number, intended to indicate repeat prescriptions within a treatment episode, was set for only 50% of prescriptions in these data. Having derived treatment episodes, OST periods could be defined for each patient history. Four categories, as used in other studies,15,53 were defined: the first 4 weeks of treatment, the remainder of any time on treatment, the first 4 weeks following cessation of treatment and any remainder of time off treatment.
Episodes for benzodiazepines and z-drugs were generated in a similar fashion, but the gap between treatments was reduced to > 14 days to reflect the shorter expected treatment duration for these medications (WPs 1 and 3). Episodes for gabapentinoids were generated using > 28 days.
Main outcomes
The main outcome was date of death. For all-cause mortality (ACM), the date of death was obtained from CPRD. This was, to some extent, a derived variable extracted from various sources with varying degrees of accuracy. The most accurate was the death administration database, followed by statements of death as recorded in the clinical notes and, finally, the least accurate was the transfer out date with the reason given as death. CPRD has developed an algorithm for reconciling these sources of information.
Cause-specific mortality could be identified only for those patients linked to death certificate data supplied by the Office for National Statistics. Deaths from DRP were identified from the International Classification of Diseases, Ninth Edition (ICD-9) and International Classification of Diseases, Tenth Edition (ICD-10) codes. 54 These codes were supplemented to include more general, non-specific causes to reflect the potential under-reporting of DRP. The list of relevant codes is given in Appendix 1. The remainder of the deaths were classified as other, non-drug related deaths. No information on cause of death was available for patients from Scotland, Wales or Northern Ireland.
Main predictors
Opiate substitution treatment medication, particularly methadone and buprenorphine, and OST period were studied in WP 2. The period was defined to cover both periods of treatment (the first 4 weeks and the remainder of the time until cessation of treatment) and subsequent periods following the cessation of treatment (the first 4 weeks and the remainder of time until the next episode). This data set was also used in WP 5, where the modified SCCS methods were applied. The co-prescriptions of benzodiazepines, z-drugs and gabapentinoids were the main predictors in WP 3. Initiation and cessation doses were studied in WP 4, while year (1998 to 2014) was the main predictor in WP 1. Further details can be found in the specific chapters relating to each WP.
In WP 1, the main predictors and patient characteristics used in other WPs became outcomes in this WP as their trends across time were explored.
Main confounders
The main adjustment variables were age, gender, calendar year, comorbidity score and UK region. 15,16,26,55 Other potential confounders such as social class,26,55 last treatment dose15,55 or episode number15 were omitted because of lack of any consistent evidence of an effect from these studies.
The comorbidity score was derived from 17 chronic illnesses. 56 A list of 3156 Read codes had been linked to these illnesses. These were translated to the current CPRD medcodes. This was possible for 2856 codes. A time-varying covariate was calculated based on information on the earliest onset of these illnesses and the weight given to each. The comorbidity score was derived by accumulating the weights across time for each patient. Although the score had a range of 0–11, in analyses, this variable was recoded to 0, 1 or ≥ 2.
In WP 3, OST type and period were used as additional adjustment variables.
Propensity scores
In WPs 2 and 3, propensity scores were generated for prescribed medications using logistic regression on four groups of variables.
-
Other prescribed medications. Propensity scores were generated for buprenorphine compared with methadone, and benzodiazepines, z-drugs and gabapentinoids compared with non-prescription. For each medication’s propensity score, the other three medications were used as predictors. As buprenorphine was an alternative to methadone, its propensity score could be generated for each episode. For other medications, propensity scores reflected whether or not they were ever exposed during each patient’s history of prescriptions.
-
Practice characteristics. General practices were described by the number of OST patients receiving treatment during each year of the study, the size of the practice and the UK region. The size of practice was defined by the number of GPs writing prescriptions, OST or other, in any one year, excluding those identified as locums. UK region was used in all analyses, and the other two measures were used solely in propensity score derivation.
-
Psychosocial adversity. Five measures of adversity were derived from the recording of relevant CPRD medcodes in the clinical notes (see Appendix 2). These data were used to generate non-time-varying binary variables reflecting any recoded event of self-harm, having ever taken an overdose, having ever had alcohol problems, having ever been in prison or having ever been homeless.
-
Main confounders. The five main confounders noted above were also included in the model.
These scores were used in inverse probability weighted (IPW) or propensity score matching (PSM) analyses.
Public and patient involvement
Interviews in small groups were conducted by two facilitators from the Bristol Drugs Project during 2015–16. These occurred in two geographical sites and involved multiple services (see Appendix 3). Focus groups with staff and service users explored their views and preferences around substitution therapy with methadone compared with buprenorphine and around considerations influencing their concurrent use of benzodiazepines, z-drugs and gabapentinoids. Service users were also asked for their views on the research questions we considered in this project in terms of the relevance of the questions, the priority they would attach to them and any additional questions that they felt were important. Where feasible, we used these views to inform our analysis plan.
Statistical analyses
Mortality data were analysed using Poisson regression (WPs 2 and 3). In WP 5, fixed-effect Poisson regression clustering on patients was used to analyse mortality data as part of SCCS analyses. The results of these analyses are reported as incidence rate ratios (IRRs). Mortality was also analysed using survival analysis (WPs 2–4). For these analyses, the risk of mortality was reported as hazard ratios (HRs). In unadjusted analyses for WPs 2–4, mortality rates are also reported. For WP 1, linear and logistic regression were utilised depending on the outcome. Further details can be found in Chapter 4.
A number of techniques were utilised in an attempt to provide estimates less susceptible to bias from residual confounding than those from standard adjusted Poisson analyses. The primary technique was IPW used in WPs 2 and 3. Simply, this method aims to emulate a randomised trial by utilising weights reflecting the inverse probability of receiving the observed treatment. 47 This has the effect of evenly distributing confounders between the exposure groups. PSM also attempts to balance confounders between the exposure groups, but here the balancing is achieved more directly by choosing observations from the two groups with similar propensity scores. In WP 2, PSM was applied to episodes of either methadone or buprenorphine. Matching was achieved if the two episodes had their logits of the propensity score within 0.25 standard deviation (SD). 46 The third method was instrumental variables (IVs). 57 A valid IV is causally related to the exposure, related to the outcome only via the exposure and unrelated to confounders. Previous studies have utilised physicians’ prescribing preference as an IV. 58,59 In WP 2, the prescribing GP’s previous OST prescription was explored as an IV.
In WP 4, initiation and cessation dose characteristics were estimated for the first/last 28 days of each episode. Because death may have occurred during these periods, linear growth models were used to estimate the latent trajectories. 60
On a technical point, it is worth commenting on the relationship between unadjusted mortality rates, IRRs and HRs. For standard Poisson regression, IRRs are identical to the equivalent mortality rate ratio, but for other analyses, this equality does not hold. Hence, Poisson regression clustering on patient will always adjust for patient differences. Similarly, in survival analysis, effects are always estimated relative to the survival function, which is usually related to age. Hence, although we refer to unadjusted analyses, models involving a single factor or covariate, it is important to recognise the implicit adjustment being made in some of these analyses.
Effect of opiate substitution treatment on drug-related poisoning mortality in the population
In WP 2, we estimated the probability that OST reduces DRP in the population by calculating weighted mortality risk ratios of DRP deaths. These mortality ratios compare the observed mortality risk in patients undergoing OST with the assumed mortality risk of opioid-dependent patients who do not enter OST (accounting for fluctuating mortality risk in different periods on and off OST, and for variation in the duration of current treatment). We also estimated the minimum duration of methadone and buprenorphine required to reduce DRP deaths in the population (for more details see web appendix 2 in that WP’s main report61).
Research ethics approval
Ethics approval for this research project was obtained from Independent Scientific Advisory Committee, Medicines and Healthcare products Regulatory Agency (protocol 14–0732R2Mn2).
Reporting guidelines
This report follows RECORD (REporting of studies Conducted using Observational Routinely-collected health Data) guidelines for the reporting of observational studies. 62
Chapter 4 Trends in opiate substitution treatment, patient characteristics and prescribing practice
Deaths from DRP were 50% higher in 2014 than in 1998. 54 This may suggest that there were major changes in the characteristics of those who abuse drugs and their treatment during this period. In this chapter on WP 1, we explore some of these changes.
Aims and objectives
In this WP, we aim to investigate how OST delivery has changed over time, in terms of:
-
number of OST patients and episodes by OST type
-
patient characteristics such as age, gender and medication prescribed
-
episode characteristics such as mean and maximum dose and duration.
Details of the starting and ending doses are considered in Chapter 7.
Data set
The data used in these analyses were restricted to episodes in which some part of treatment occurred during the study period. To obtain a more accurate picture of the trends in these data, we considered all episodes including those involving dihydrocodeine or more than one medication and all patients ignoring the age restriction used in other WPs (see Figure 2). This led to the data for 12,780 patients and 34,427 episodes being analysed.
Prevalence of opiate substitution treatment
Crude UK prevalence estimates were derived from valid OST patients within each year and the total number of patients within CPRD. To obtain adjusted prevalence estimates, it was necessary to estimate the number of OST patients for the UK. This was achieved by combining numbers of patients for each country, taking into account the different coverages for each country by CPRD.
Patient characteristics
These outcomes include age and gender demographics and the medications prescribed. Medications included not only the three OST medications but also benzodiazepines, z-drugs and gabapentinoids. The last three medications were considered to be prescribed only during valid periods for each patient.
Episode characteristics
Mean and maximum doses were considered as outcomes. These are reported by year for episodes involving only methadone or buprenorphine. Average doses were calculated daily for the parts of episodes within any given year. Maximum doses were reported as a percentage of episodes with ≥ 60 mg for methadone or ≥ 12 mg for buprenorphine. The last episode for each patient was excluded if treatment was ongoing at the time follow-up ceased. This reduced the episode count to 31,260. For this outcome, episodes were valid only for the year associated with the maximum dose.
In addition, on- and off-treatment duration were investigated. Episode treatment was considered to cease when there was a gap of > 28 days between the end of one prescription and the start of the next. Last episodes were right-censored by the death of the patient, the last CPRD data collection date or the end of the study. First episodes were potentially left-censored by the practice up-to-standard date, the start of patient follow-up (registration with a CPRD practice) or the study start date.
Statistical analysis
A number of different analyses were used to assess trends depending on the particular outcomes. The prevalence of OST was analysed using Poisson regression. Binary outcomes (gender, medications and dose criteria) were analysed using logistic regression. Trends in duration were analysed using survival analysis to take account of censoring. Different parametric distributions were compared to find the best fit (Table 1). These analyses suggested that the log-normal distribution was superior, although other distributions gave similar results. Other outcomes, namely age and mean dose, were analysed using linear regression.
Function | Duration model log-likelihood | |
---|---|---|
On treatment | Off treatment | |
Gompertz | –52,482 | –34,247 |
Log-logistic | –49,384 | –33,199 |
Generalised gamma | –48,827 | a |
Exponential | –57,672 | –35,245 |
Weibull | –50,558 | –34,372 |
Log-normal | –48,900 | –32,415 |
Primary analyses involved unadjusted year effects. Trends were assessed in two ways. First, a linear year effect was fitted to the data. Second, a deviation statistic was calculated. This reflected twice the difference in log-likelihoods between a model treating year as a factor and the linear model. The derived statistic [15 degrees of freedom (df)] provided evidence of any non-linearity. Where the analysis involved linear regression, the trend is additive from one year to the next. For other analyses, there is an implicit log transformation making the trend a multiplicative effect from one year to the next when back transformed.
Trends in prevalence of opiate substitution treatment
Most countries in the UK showed similar trends in that OST prevalence rates and number of patients were increasing at the start of the study and declining by the end (Table 2 and Figure 3), although for Northern Ireland, where the use of primary care to treat problem drug use is more limited, these data were underpowered to detect the possible inverted U-shaped trend. The observed maxima varied by country and ranged between 2008 and 2011 for three nations, with Scotland showing an earlier peak, in 2003. Overall, the UK showed a maximum in 2008. Perhaps as expected, the adjusted UK estimates were similar to the unadjusted estimates because of the major contribution of England to the UK total.
Year | CPRD coverage by country (%) | Prevalence by countrya | UK adjusted | |||||||||
---|---|---|---|---|---|---|---|---|---|---|---|---|
England | Wales | Scotland | Northern Ireland | UK | England | Wales | Scotland | Northern Ireland | UK | Prevalencea | n b | |
1998 | 4.61 | 6.36 | 3.19 | 4.71 | 4.57 | 4.04 | 1.94 | 12.34 | 0.96 | 4.32 | 4.58 | 28 |
1999 | 5.70 | 8.35 | 3.87 | 5.57 | 5.66 | 4.72 | 3.17 | 11.96 | 1.32 | 4.94 | 5.18 | 32 |
2000 | 6.40 | 10.01 | 4.52 | 6.97 | 6.43 | 4.86 | 3.32 | 12.75 | 1.13 | 5.11 | 5.36 | 33 |
2001 | 7.00 | 11.41 | 5.85 | 7.87 | 7.15 | 4.89 | 3.23 | 14.53 | 1.22 | 5.33 | 5.54 | 34 |
2002 | 7.45 | 11.63 | 7.13 | 8.82 | 7.66 | 5.24 | 3.96 | 15.60 | 1.15 | 5.84 | 5.96 | 37 |
2003 | 7.45 | 12.48 | 9.05 | 8.85 | 7.87 | 5.61 | 4.93 | 17.11 | 1.01 | 6.53 | 6.42 | 40 |
2004 | 7.68 | 13.06 | 9.46 | 9.21 | 8.14 | 6.05 | 5.36 | 16.87 | 1.15 | 6.91 | 6.80 | 43 |
2005 | 7.75 | 13.34 | 9.54 | 9.24 | 8.22 | 6.65 | 5.94 | 17.02 | 1.45 | 7.46 | 7.35 | 46 |
2006 | 7.87 | 13.33 | 9.64 | 9.21 | 8.32 | 6.96 | 5.87 | 13.99 | 1.62 | 7.40 | 7.35 | 47 |
2007 | 7.94 | 13.96 | 9.65 | 9.12 | 8.42 | 7.35 | 6.57 | 12.87 | 1.61 | 7.65 | 7.62 | 48 |
2008 | 7.79 | 13.99 | 9.63 | 8.43 | 8.26 | 7.64 | 7.44 | 12.90 | 1.41 | 7.97 | 7.90 | 51 |
2009 | 7.65 | 13.98 | 9.62 | 8.37 | 8.15 | 7.51 | 7.23 | 13.08 | 1.28 | 7.86 | 7.79 | 50 |
2010 | 7.51 | 14.00 | 9.61 | 8.36 | 8.02 | 7.40 | 7.57 | 14.86 | 1.27 | 7.98 | 7.86 | 52 |
2011 | 7.22 | 14.02 | 9.69 | 8.33 | 7.78 | 6.82 | 7.89 | 14.51 | 1.46 | 7.55 | 7.36 | 49 |
2012 | 6.92 | 14.11 | 9.68 | 8.34 | 7.54 | 6.67 | 7.59 | 13.02 | 1.38 | 7.26 | 7.09 | 47 |
2013 | 6.76 | 14.64 | 9.68 | 8.34 | 7.43 | 6.18 | 6.55 | 12.78 | 1.31 | 6.78 | 6.61 | 44 |
2014 | 5.93 | 14.37 | 9.74 | 8.34 | 6.72 | 5.16 | 5.05 | 12.31 | 1.05 | 5.87 | 5.64 | 38 |
Trendc | 1.023 | 1.050 | 0.989 | 1.009 | 1.022 | 1.020 | 1.026 | |||||
SE (trend)c | 1.18 | 4.12 | 2.20 | 12.32 | 1.01 | 0.26 | 0.26 | |||||
p-value | < 0.0001 | < 0.0001 | < 0.0001 | 0.4786 | < 0.0001 | < 0.0001 | < 0.0001 | |||||
Deviation | < 0.0001 | < 0.0001 | < 0.0001 | 0.9747 | < 0.0001 | < 0.0001 | < 0.0001 |
FIGURE 3.
Prevalence of OST by country and year. All countries except Northern Ireland showed non-linear trends in the prevalence of OST by year. The non-linearity reflected an increasing trend in the earlier years followed by a decline in the prevalence. The timing of the decline varied by country and may have started earliest in Scotland, followed by England, with Wales showing the latest downturn. Northern Ireland showed a constant prevalence of OST for each year studied.
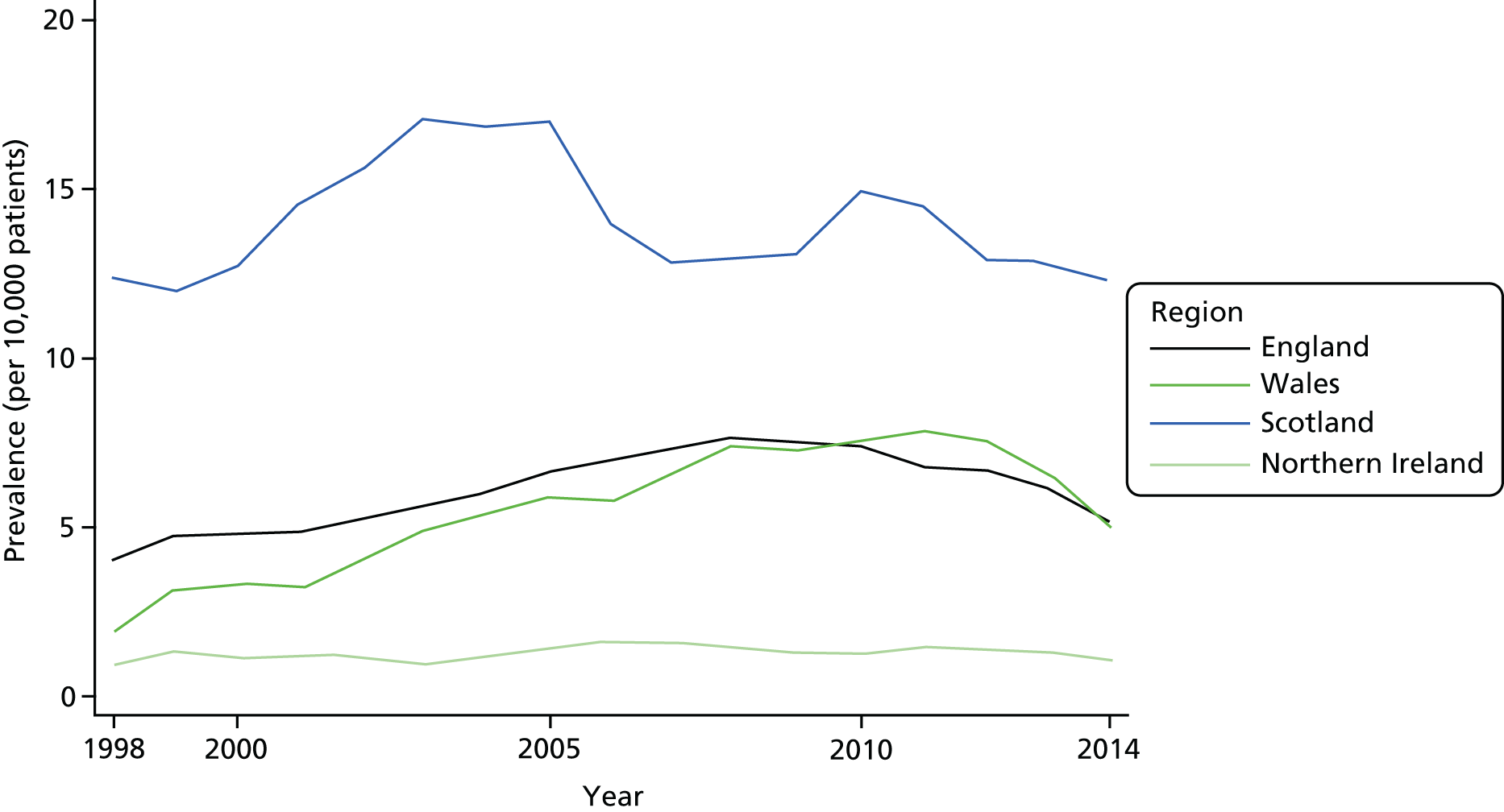
Trends in patient characteristics
The average age of OST patients increased by 10 years during the study (Table 3). About half of this increase was attributable to an ageing sample of patients receiving prolonged OST over many episodes. There was little evidence that the gender ratio varied during the study period. However, this concealed a declining trend in the ratio for the under-30-year-olds, with men representing 70% of this group in 1998 compared with 60% in 2014 (p < 0.0001).
Year | OST patients | Age (years) | Gender (% male) | Medications (% of patients) | |||||
---|---|---|---|---|---|---|---|---|---|
M | B | D | BD | ZD | PG | ||||
1998 | 1160 | 32.78 | 68.10 | 86.03 | 8.45 | 15.00 | 42.07 | 10.78 | 0.60 |
1999 | 1707 | 32.43 | 67.72 | 85.82 | 7.79 | 15.35 | 37.26 | 12.71 | 0.64 |
2000 | 1948 | 33.04 | 68.84 | 83.21 | 10.22 | 17.30 | 37.47 | 13.86 | 0.87 |
2001 | 2357 | 33.43 | 68.22 | 77.94 | 18.24 | 17.95 | 35.51 | 12.13 | 0.93 |
2002 | 2805 | 33.90 | 67.45 | 73.16 | 23.78 | 16.51 | 36.72 | 13.58 | 1.21 |
2003 | 3274 | 34.16 | 67.78 | 71.90 | 27.09 | 12.98 | 34.18 | 12.16 | 1.56 |
2004 | 3574 | 34.57 | 67.04 | 69.56 | 30.64 | 11.58 | 32.18 | 11.39 | 2.52 |
2005 | 3783 | 35.26 | 66.93 | 68.89 | 32.30 | 11.10 | 32.88 | 12.00 | 2.88 |
2006 | 3978 | 36.25 | 68.00 | 69.43 | 31.57 | 10.41 | 33.43 | 13.70 | 3.14 |
2007 | 4118 | 37.21 | 67.53 | 69.31 | 30.67 | 10.13 | 32.25 | 12.94 | 4.15 |
2008 | 4276 | 37.95 | 67.45 | 71.02 | 29.09 | 9.10 | 32.16 | 12.04 | 4.07 |
2009 | 4188 | 38.60 | 66.52 | 72.11 | 27.72 | 8.55 | 31.45 | 12.30 | 4.75 |
2010 | 4129 | 39.42 | 67.35 | 73.12 | 26.64 | 8.09 | 30.35 | 12.62 | 6.32 |
2011 | 3919 | 40.16 | 67.44 | 73.03 | 25.85 | 7.76 | 30.62 | 12.78 | 7.76 |
2012 | 3681 | 40.82 | 67.24 | 70.99 | 27.68 | 7.25 | 30.05 | 12.66 | 9.07 |
2013 | 3413 | 41.47 | 67.07 | 69.32 | 29.62 | 7.47 | 28.66 | 11.10 | 11.08 |
2014 | 2670 | 42.55 | 67.45 | 67.57 | 30.82 | 7.68 | 26.89 | 10.90 | 12.70 |
Overall | 12,780 | 36.04 | 66.63 | 78.62 | 36.03 | 15.37 | 47.44 | 25.37 | 9.20 |
Trenda | 0.681 | 0.997 | 0.971 | 1.036 | 0.930 | 0.971 | 0.995 | 1.213 | |
SE (trend) | 0.0099 | 0.0021 | 0.0023 | 0.0025 | 0.0032 | 0.0021 | 0.0030 | 0.0058 | |
p-value | < 0.0001 | 0.2119 | < 0.0001 | < 0.0001 | < 0.0001 | < 0.0001 | 0.1149 | < 0.0001 | |
Deviation | < 0.0001 | 0.9956 | < 0.0001 | < 0.0001 | < 0.0001 | 0.0790 | 0.0070 | 0.3984 |
During the study, the use of methadone, dihydrocodeine and benzodiazepines was decreasing while the use of buprenorphine and gabapentinoids was increasing (Figure 4). Deviations from linearity for methadone and buprenorphine suggested that the major changes occurred up to 2006, with less evidence of any changes after that date. Although there was evidence of a declining prevalence for the prescription of z-drugs, the effect size was small, reflecting a prevalence among OST patients of ≈12. Considering the prevalence of any of these three medications, the prevalence was declining up to 2002 but with no strong evidence of any change after that year. Overall, 78.6% [95% confidence interval (CI) 77.9% to 79.3%], 36.0% (95% CI 35.2% to 36.9%), 15.4% (95% CI 14.7% to 16.0%), 47.4% (95% CI 46.6% to 48.3%), 25.4% (95% CI 24.6% to 28.1%) and 9.2% (95% CI 6.7% to 9.7%) of patients were prescribed methadone, buprenorphine, dihydrocodeine, benzodiazepines, z-drugs or gabapentinoids, respectively.
FIGURE 4.
Opiate substitution treatment medications, benzodiazepines, z-drugs and gabapentinoids prescribed to OST patients by year. Overall, during the study, prescriptions of methadone declined, whereas those for buprenorphine increased. There may be some evidence to suggest that, after 2007, the percentage of OST patients prescribed these medications remained constant. Benzodiazepines and z-drugs were prescribed to a declining proportion of OST patients as time progressed. Gabapentinoids were increasingly prescribed, from < 1% in 1998 to > 10% in 2014.
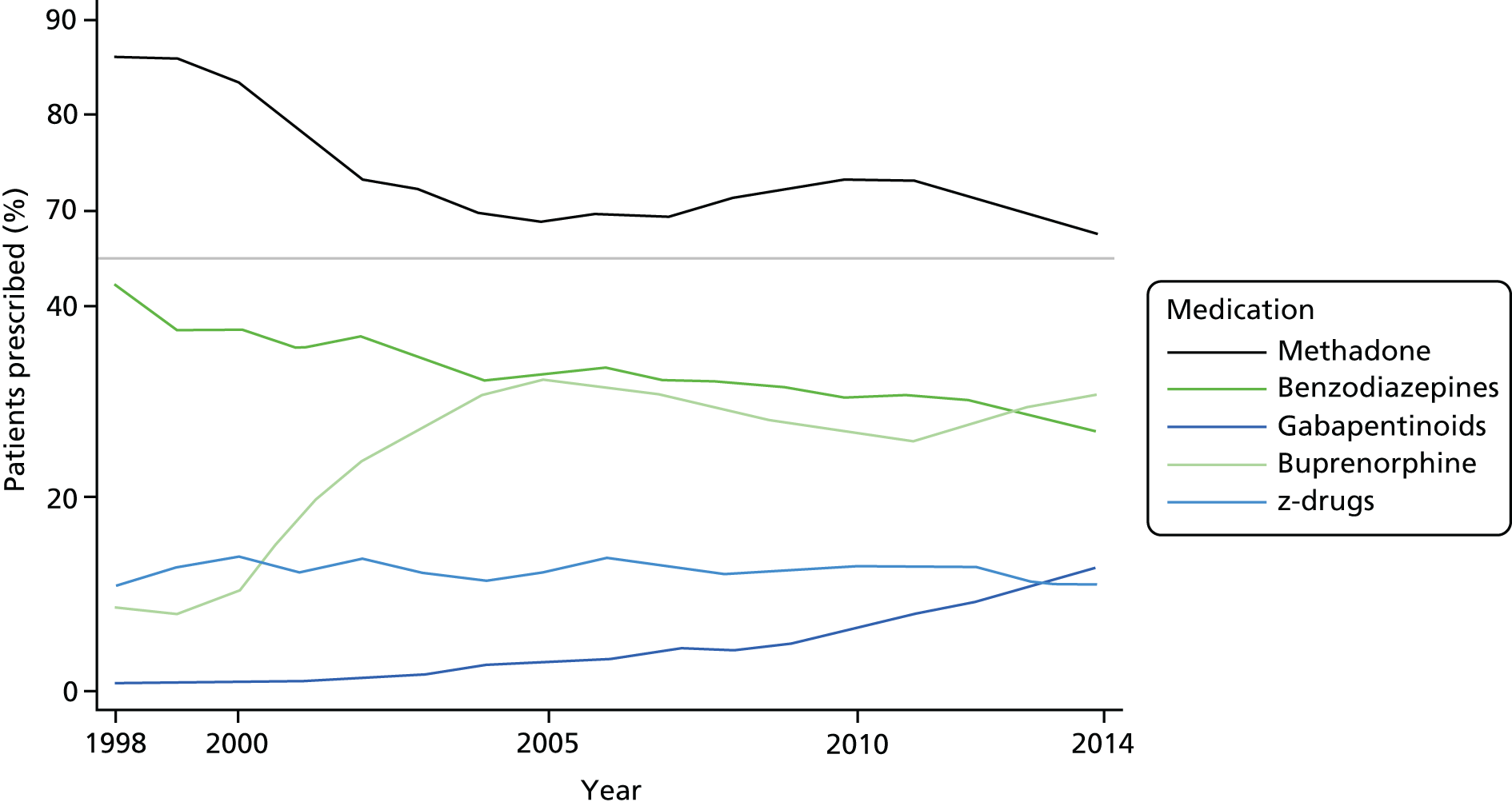
Trends in episode characteristics
Overall, average dose increased during the study, although there was evidence that this trend may have changed after 2008 (Table 4). Linear trends after this date showed a decreasing effect, with –1.057 (95% CI –1.123 to –0.966) and –0.151 (95% CI –0.177 to –0.126) for methadone and buprenorphine, respectively. Both medications showed an increasing adherence to guidelines with optimal dose across the whole study. However, the deviation statistics suggested a more complex pattern. After 2008, methadone episodes showed a declining adherence, multiplicative trend (0.96, 95% CI 0.94 to 0.99), whereas buprenorphine showed no trend (1.02, 95% CI 0.99 to 1.06).
Year | Number of episodes | Mean dose (mg) | Optimal dose (%) | On-treatment duration (days) | Off-treatment duration (days) | ||||||||
---|---|---|---|---|---|---|---|---|---|---|---|---|---|
M | B | Other | M | B | M | B | M | B | All | M | B | All | |
1998 | 1062 | 97 | 425 | 41.95 | 2.30 | 25.08 | 16.67 | 76 | 57 | 82 | 182 | 89 | 163 |
1999 | 1366 | 166 | 619 | 40.86 | 2.47 | 26.79 | 8.96 | 66 | 36 | 69 | 206 | 74 | 172 |
2000 | 1526 | 199 | 842 | 39.11 | 2.80 | 21.45 | 7.62 | 58 | 29 | 62 | 177 | 101 | 166 |
2001 | 1698 | 351 | 1146 | 40.99 | 3.04 | 27.39 | 14.80 | 77 | 41 | 75 | 179 | 125 | 173 |
2002 | 1885 | 579 | 1323 | 43.67 | 4.28 | 34.72 | 18.92 | 80 | 45 | 80 | 182 | 144 | 179 |
2003 | 2109 | 776 | 1406 | 46.21 | 5.13 | 41.59 | 23.93 | 100 | 46 | 91 | 172 | 146 | 168 |
2004 | 2180 | 885 | 1506 | 48.05 | 5.47 | 45.36 | 28.35 | 98 | 47 | 93 | 196 | 148 | 176 |
2005 | 2077 | 998 | 1609 | 51.28 | 6.21 | 51.01 | 30.15 | 122 | 48 | 103 | 204 | 142 | 175 |
2006 | 2232 | 1025 | 1682 | 53.66 | 6.46 | 56.17 | 25.80 | 134 | 48 | 108 | 195 | 142 | 175 |
2007 | 2366 | 1017 | 1686 | 55.71 | 7.30 | 60.48 | 31.40 | 141 | 42 | 102 | 199 | 165 | 181 |
2008 | 2560 | 996 | 1601 | 57.00 | 7.32 | 63.97 | 33.89 | 151 | 48 | 110 | 186 | 149 | 169 |
2009 | 2507 | 999 | 1559 | 56.30 | 7.27 | 62.69 | 27.91 | 144 | 46 | 102 | 220 | 137 | 179 |
2010 | 2497 | 931 | 1441 | 56.83 | 7.27 | 61.98 | 31.19 | 178 | 53 | 122 | 209 | 152 | 181 |
2011 | 2376 | 868 | 1288 | 54.82 | 6.70 | 63.65 | 29.57 | 140 | 52 | 107 | 259 | 150 | 197 |
2012 | 2145 | 911 | 1158 | 52.50 | 6.62 | 62.87 | 33.45 | 121 | 61 | 99 | 254 | 151 | 197 |
2013 | 1928 | 851 | 1016 | 52.20 | 6.94 | 59.38 | 32.67 | 94 | 58 | 82 | 219 | 161 | 185 |
2014 | 1405 | 626 | 716 | 52.64 | 7.17 | 55.76 | 36.36 | 68 | 62 | 64 | 293 | 198 | 248 |
Overall | 17,787 | 8497 | 8143 | 51.66 | 6.54 | 48.58 | 28.49 | 106 | 48 | 93 | 202 | 146 | 179 |
Trenda | 0.959 | 0.198 | 1.131 | 1.061 | 1.048 | 1.028 | 1.020 | 1.023 | 1.024 | 1.013 | |||
SE (trend) | 0.0030 | 0.0013 | 0.0036 | 0.0065 | 0.0033 | 0.0047 | 0.0025 | 0.0026 | 0.0039 | 0.0020 | |||
p-value | < 0.0001 | < 0.0001 | < 0.0001 | < 0.0001 | < 0.0001 | < 0.0001 | < 0.0001 | < 0.0001 | < 0.0001 | < 0.0001 | |||
Deviation | < 0.0001 | < 0.0001 | < 0.0001 | < 0.0001 | < 0.0001 | 0.0952 | < 0.0001 | < 0.0001 | < 0.0001 | 0.0002 |
The predicted median durations by year are shown in Table 4. The mean durations are not reported because of the skewed nature of the distribution. On-treatment duration reached a maximum in 2010 for methadone (Figure 5). By contrast, duration for buprenorphine was still increasing by the end of the study. Off-treatment durations were generally increasing throughout the study but there were periods when duration appeared to be constant. For methadone, there was no evidence of any increase before 2009 (HR 1.01, 95% CI 1.00 to 1.02; p = 0.180). Similarly, for buprenorphine, during the period 2002–8, off-treatment duration remained constant (HR 1.01, 95% CI 0.99 to 1.03; p = 0.306).
FIGURE 5.
Duration of OST by type and year. The median duration of buprenorphine treatment increased overall during the study, although there was some variability in the early years owing to the small number of episodes. Methadone episodes showed an increasing duration up to 2010 but then decreased such that in 2014 their durations were similar to those of buprenorphine.
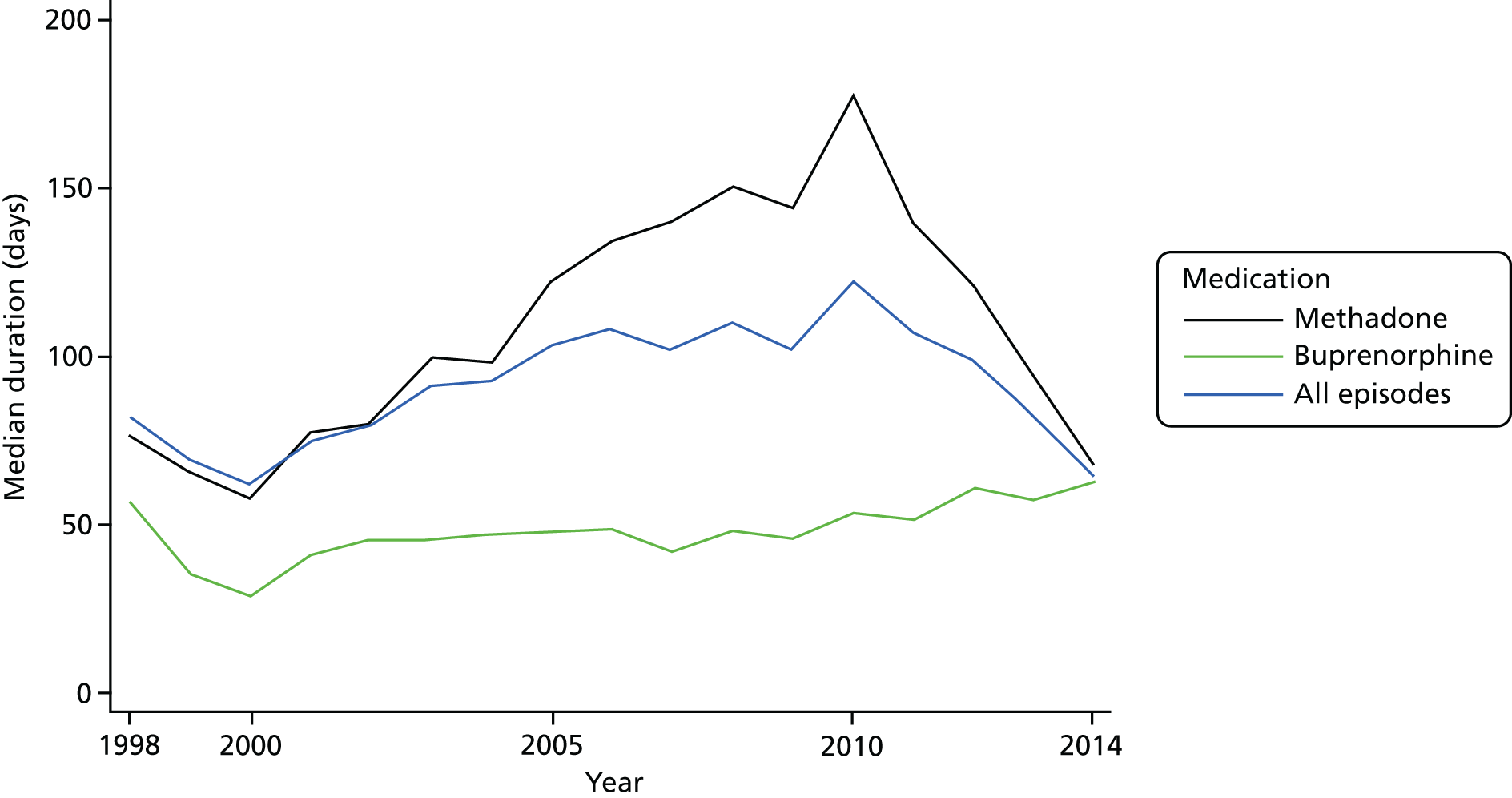
The associations of gender, age, comorbidity and region with on- and off-durations are reported in Table 5. Age and gender were associated with on-treatment duration for buprenorphine only, with older women tending to have longer durations (lower HR). Those with comorbid chronic illnesses tended to have longer durations for both medications. For off-treatment duration, gender had no association with either medication and comorbidity had no association with buprenorphine. Older patients tended to have longer intervals between treatments. Adjusting for these variables did not markedly change the duration results (Table 6).
Confounder | Category | On treatment | Off treatment | ||||||
---|---|---|---|---|---|---|---|---|---|
Methadone | Buprenorphine | Methadone | Buprenorphine | ||||||
HR (95% CI) | p-value | HR (95% CI) | p-value | HR (95% CI) | p-value | HR (95% CI) | p-value | ||
Gender | Male | 0.99 (0.94 to 1.06) | 0.8483 | 1.25 (1.16 to 1.35) | < 0.0001 | 0.97 (0.93 to 1.02) | 0.2704 | 1.01 (0.96 to 1.08) | 0.6385 |
Female | 1 (reference) | 1 (reference) | 1 (reference) | 1 (reference) | |||||
Age (years) | < 30 | 1 (reference) | 0.2908 | 1 (reference) | < 0.0001 | 1 (reference) | < 0.0001 | 1 (reference) | < 0.0001 |
30–39 | 1.06 (0.99 to 1.14) | 1.14 (1.02 to 1.27) | 0.82 (0.77 to 0.86) | 0.93 (0.85 to 1.02) | |||||
40–49 | 1.05 (0.97 to 1.15) | 0.91 (0.81 to 1.02) | 0.62 (0.57 to 0.66) | 0.61 (0.55 to 0.66) | |||||
≥ 50 | 1.10 (0.97 to 1.24) | 0.72 (0.65 to 0.81) | 0.54 (0.49 to 0.60) | 0.42 (0.38 to 0.46) | |||||
Comorbidity score | 0 | 1 (reference) | < 0.0001 | 1 (reference) | 0.0251 | 1 (reference) | < 0.0001 | 1 (reference) | 0.2837 |
1 | 1.01 (0.94 to 1.08) | 0.98 (0.90 to 1.06) | 0.91 (0.86 to 0.96) | 0.98 (0.92 to 1.05) | |||||
≥ 2 | 0.64 (0.57 to 0.73) | 0.84 (0.74 to 0.95) | 0.76 (0.69 to 0.85) | 1.07 (0.97 to 1.18) | |||||
Region | North East | 1.29 (1.00 to 1.66) | < 0.0001 | 1.38 (1.11 to 1.70) | < 0.0001 | 1.21 (0.98 to 1.48) | < 0.0001 | 0.79 (0.67 to 0.92) | < 0.0001 |
North West | 1 (reference) | 1 (reference) | 1 (reference) | 1 (reference) | |||||
Yorkshire and the Humber | 1.85 (1.61 to 2.14) | 1.89 (1.53 to 2.35) | 1.52 (1.34 to 1.71) | 1.08 (0.91 to 1.29) | |||||
East Midlands | 1.32 (1.13 to 1.53) | 1.33 (1.12 to 1.58) | 1.40 (1.24 to 1.59) | 0.95 (0.83 to 1.09) | |||||
West Midlands | 1.80 (1.62 to 2.01) | 1.12 (0.98 to 1.28) | 1.72 (1.57 to 1.88) | 1.13 (1.02 to 1.26) | |||||
East | 1.45 (1.29 to 1.62) | 1.27 (1.09 to 1.48) | 1.49 (1.36 to 1.63) | 1.17 (1.04 to 1.32) | |||||
South West | 1.88 (1.68 to 2.10) | 1.22 (1.06 to 1.41) | 1.72 (1.56 to 1.89) | 1.06 (0.95 to 1.18) | |||||
South Central | 2.31 (2.05 to 2.61) | 1.16 (1.01 to 1.32) | 1.63 (1.48 to 1.80) | 1.08 (0.97 to 1.20) | |||||
London | 2.30 (2.03 to 2.62) | 1.19 (1.03 to 1.39) | 1.46 (1.32 to 1.62) | 0.89 (0.80 to 1.00) | |||||
South East | 1.12 (0.95 to 1.33) | 1.03 (0.86 to 1.22) | 1.90 (1.66 to 2.18) | 1.11 (0.97 to 1.27) | |||||
Northern Ireland | 1.62 (0.83 to 3.16) | 1.38 (0.99 to 1.90) | 2.13 (1.20 to 3.79) | 0.61 (0.48 to 0.78) | |||||
Scotland | 3.37 (3.10 to 3.66) | 1.12 (0.92 to 1.37) | 1.45 (1.35 to 1.55) | 1.24 (1.05 to 1.46) | |||||
Wales | 1.98 (1.71 to 2.29) | 1.79 (1.52 to 2.09) | 2.27 (2.01 to 2.57) | 1.28 (1.12 to 1.45) |
Year | On-treatment duration (days) | Off-treatment duration (days) | ||||
---|---|---|---|---|---|---|
Methadone (only) | Buprenorphine (only) | Alla | Methadone (only) | Buprenorphine (only) | Alla | |
1998 | 93 | 73 | 87 | 170 | 126 | 150 |
1999 | 81 | 43 | 75 | 194 | 98 | 160 |
2000 | 71 | 33 | 68 | 175 | 126 | 158 |
2001 | 87 | 43 | 79 | 175 | 132 | 163 |
2002 | 87 | 46 | 81 | 178 | 140 | 168 |
2003 | 104 | 46 | 91 | 169 | 135 | 158 |
2004 | 106 | 48 | 95 | 193 | 139 | 167 |
2005 | 137 | 49 | 110 | 204 | 137 | 171 |
2006 | 158 | 49 | 120 | 201 | 139 | 177 |
2007 | 162 | 43 | 113 | 211 | 164 | 189 |
2008 | 171 | 51 | 122 | 197 | 155 | 180 |
2009 | 158 | 51 | 115 | 232 | 148 | 194 |
2010 | 189 | 59 | 136 | 218 | 165 | 200 |
2011 | 153 | 59 | 124 | 284 | 171 | 228 |
2012 | 137 | 67 | 114 | 279 | 164 | 224 |
2013 | 102 | 64 | 93 | 250 | 176 | 217 |
2014 | 67 | 70 | 70 | 327 | 216 | 288 |
Overall | 120 | 51 | 101 | 206 | 151 | 184 |
Trendb | 1.041 | 1.034 | 1.028 | 1.034 | 1.030 | 1.031 |
SE (trend) | 0.0034 | 0.0049 | 0.0026 | 0.0027 | 0.0038 | 0.0020 |
p-value | < 0.0001 | < 0.0001 | < 0.0001 | < 0.0001 | < 0.0001 | < 0.0001 |
Deviation | < 0.0001 | 0.0355 | < 0.0001 | < 0.0001 | 0.2703 | < 0.0001 |
In a sensitivity analysis, we also analysed duration using the start year of on or off treatment rather than each year associated with an episode. This had two consequences: (1) episodes with on/off treatment starting before the study start date were excluded and (2) an episode contributed to only 1 year however long the period on/off treatment. As a result, it was appropriate to consider each episode as only a single record. Despite these changes to the data, the median durations were very similar (Table 7).
Year | On-treatment duration (days) | Off-treatment duration (days) | ||||
---|---|---|---|---|---|---|
Methadone (only) | Buprenorphine (only) | Alla | Methadone (only) | Buprenorphine (only) | Alla | |
1998 | 72 | 65 | 78 | 208 | 81 | 180 |
1999 | 63 | 36 | 67 | 193 | 83 | 169 |
2000 | 63 | 28 | 67 | 174 | 97 | 164 |
2001 | 75 | 41 | 73 | 175 | 142 | 174 |
2002 | 85 | 47 | 85 | 186 | 152 | 182 |
2003 | 105 | 46 | 96 | 178 | 140 | 169 |
2004 | 100 | 48 | 94 | 206 | 150 | 180 |
2005 | 132 | 47 | 108 | 190 | 145 | 171 |
2006 | 143 | 50 | 110 | 192 | 138 | 169 |
2007 | 142 | 44 | 104 | 200 | 164 | 180 |
2008 | 149 | 49 | 110 | 185 | 145 | 166 |
2009 | 139 | 47 | 99 | 217 | 134 | 177 |
2010 | 168 | 51 | 115 | 221 | 159 | 187 |
2011 | 130 | 52 | 102 | 264 | 147 | 198 |
2012 | 121 | 61 | 97 | 257 | 159 | 203 |
2013 | 85 | 57 | 77 | 226 | 163 | 192 |
2014 | 73 | 58 | 69 | 362 | 199 | 279 |
Overall | 106 | 48 | 93 | 203 | 146 | 180 |
Trendb | 1.0470 | 1.0240 | 1.0186 | 1.0240 | 1.0225 | 1.0129 |
SE (trend) | 0.0033 | 0.0048 | 0.0026 | 0.0027 | 0.0039 | 0.0020 |
p-value | < 0.0001 | < 0.0001 | < 0.0001 | < 0.0001 | < 0.0001 | < 0.0001 |
Deviation | < 0.0001 | 0.0480 | < 0.0001 | < 0.0001 | < 0.0001 | < 0.0001 |
Summary
Our data suggest that the prevalence of OST has been declining since 2010. Extrapolation to the whole UK suggests that < 50,000 patients are currently being treated in primary care. This number is lower than other estimates9,11 and may reflect either other patients being treated by alternative services such as community drug agencies or that CPRD practices were not representative of all UK general practices.
Trends in medications suggest that prescribing buprenorphine has become more prevalent, with about 30% of patients in 2014 prescribed this medication. Co-prescription of gabapentinoids was rare at the start of this study but by the end it was prescribed to about 13% of patients. Benzodiazepines were more commonly co-prescribed but the prevalence of this medication among OST patients per year declined, although they were still prescribed to about 26% of patients by the end of the study. The prevalence of co-prescription of z-drugs changed very little during the study.
The mean doses and the proportion of treatment episodes reaching an optimal dose increased up to about 2008, with evidence of declining trends after this date for most outcomes. The exception was optimal dose for buprenorphine for which the evidence suggested a stable proportion post 2008. Shorter treatment duration for buprenorphine than for methadone treatment has also been reported in other studies. 16,21–23,63 Off-treatment durations increased for both methadone and buprenorphine after 2008. It is interesting to note that the shorter on-treatment duration for buprenorphine was associated with a shorter off-treatment duration.
Chapter 5 Comparison of methadone and buprenorphine use in opiate substitution treatment
As seen in the previous chapter, there has been an increase in the use of buprenorphine as part of OST. This chapter, on WP 2, presents the results comparing buprenorphine and methadone on mortality. The main report is published in Hickman et al. 61 Reproduced from Hickman et al. 61 This is an Open Access article distributed in accordance with the terms of the Creative Commons Attribution (CC BY 4.0) license, which permits others to distribute, remix, adapt and build upon this work, for commercial use, provided the original work is properly cited. See: http://creativecommons.org/licenses/by/4.0/. The text below includes minor additions and formatting changes to the original text.
Aims and objectives
Our main aim was to explore the differences in mortality risk between methadone and buprenorphine and whether or not any effects were modified by treatment period. Our secondary aim was to explore other interactions that may influence the risk of mortality.
Data set for main analyses
There were 26,546 OST episodes involving solely methadone or buprenorphine relating to 11,033 patients. For simplicity in comparing these medications, treatment episodes involving both medications or dihydrocodeine were excluded. In 30,410 person-years of follow-up there were 587 ACM deaths.
Statistical methods
Poisson regression was the main analytic method. In addition to standard adjusted analyses, three methods, more robust to residual confounding were described in the paper: IPW, IV and PSM. Further details are provided below on the IV and PSM analyses.
Sensitivity analyses were performed:
-
Restricting to patients without any evidence of comorbid illnesses. This was to explore whether or not chronic illnesses may have introduced residual confounding, perhaps via palliative care.
-
Including partial episodes involving methadone or buprenorphine. Main analyses involved only episodes involving a single medication to avoid any possible combined effect of multiple medications during an episode. Including episodes with multiple medications allowed a more continuous follow-up of patients, increasing person-years by 19% and the number of deaths by 12%.
-
Additionally adjusting for the tapering of dose during the last 28 days of treatment. Tapering may indicate a planned discharge from treatment rather than poor treatment retention.
-
Using negative binomial regression to take account of possible overdispersion in Poisson regression.
-
Using linear regression and survival analysis to compare the results for risk differences and HRs with IRRs.
-
Using multilevel models to explore whether other sources of variability, in particular between patients, modify the interpretation of results.
-
Analysing only the first or last episode for each patient to investigate whether there was a cumulative effect of multiple episodes.
-
Redefining OST episodes based on a 7-day or 56-day gap instead of the 28-day gap used in main analyses.
Items 1–4 were included in the published paper, although additional background results are included in Tables 8–11. Items 5–8 were not included in the published paper but are included now for completeness.
Propensity score matching
As well as IPW, propensity scores were also used to match buprenorphine episodes to methadone episodes using the nearest neighbour procedure. Matching was successful if the difference in logit(propensity score) was ≤ 0.25 SD. 46 For our data, the SD of the logit was 1.28, suggesting a criterion of 0.32. Owing to the limited pool of methadone episodes, it was not always possible to match every buprenorphine episode to a methadone episode using this criterion.
Matching reduced the data to 13,940 (53% of total) and 8938 (57%) episodes for ACM and DRP, respectively. The results from Poisson regressions are shown in Table 8. Adjusted results are similar to unadjusted results, suggesting that matching had reduced observed confounding below any level of practical significance. A comparison of PSM results with confounder and IPW adjusted results in the main paper61 showed similar effects in terms of the point estimates. However, the CIs for PSM results were wider.
Period | OST type | Unadjusted | Adjusteda | ||
---|---|---|---|---|---|
IRR (95% CI) | p-value | IRR (95% CI) | p-value | ||
All-cause mortality | |||||
On 1–4 weeks | 3.02 (2.06 to 4.44) | < 0.0001 | 2.77 (1.88 to 4.09) | < 0.0001 | |
On rest | 1 (reference) | 1 (reference) | |||
Off 1–4 weeks | 10.22 (7.95 to 13.13) | 10.09 (7.80 to 13.06) | |||
Off rest | 2.03 (1.59 to 2.60) | 2.60 (2.02 to 3.35) | |||
On 1–4 weeks | Methadone | 1 (reference) | 0.0003 | 1 (reference) | 0.0031 |
Buprenorphine | 0.07 (0.02 to 0.29) | 0.0003 | 0.07 (0.02 to 0.29) | 0.0002 | |
On rest (reference) | Methadone | 1 (reference) | 1 (reference) | ||
Buprenorphine | 0.36 (0.22 to 0.59) | < 0.0001 | 0.28 (0.17 to 0.46) | < 0.0001 | |
Off 1–4 weeks | Methadone | 1 (reference) | 1 (reference) | ||
Buprenorphine | 0.10 (0.06 to 0.19) | < 0.0001 | 0.09 (0.05 to 0.16) | < 0.0001 | |
Off rest | Methadone | 1 (reference) | 1 (reference) | ||
Buprenorphine | 0.39 (0.26 to 0.56) | < 0.0001 | 0.27 (0.18 to 0.39) | < 0.0001 | |
Drug-related mortality | |||||
On 1–4 weeks | 1.28 (0.30 to 5.53) | < 0.0001 | 1.30 (0.30 to 5.68) | < 0.0001 | |
On rest | 1 (reference) | 1 (reference) | |||
Off 1–4 weeks | 7.36 (3.58 to 15.16) | 7.62 (3.63 to 16.01) | |||
Off rest | 2.52 (1.35 to 4.69) | 2.61 (1.38 to 4.96) | |||
On 1–4 weeks | Methadone | 1 (reference) | 0.1391 | 1 (reference) | 0.1186 |
Buprenorphine | n/e | n/e | |||
On rest (reference) | Methadone | 1 (reference) | 1 (reference) | ||
Buprenorphine | 0.61 (0.20 to 1.87) | 0.3893 | 0.54 (0.17 to 1.65) | 0.2781 | |
Off 1–4 weeks | Methadone | 1 (reference) | 1 (reference) | ||
Buprenorphine | 1.46 (0.48 to 4.46) | 0.5078 | 1.42 (0.46 to 4.35) | 0.5382 | |
Off rest | Methadone | 1 (reference) | 1 (reference) | ||
Buprenorphine | 0.34 (0.13 to 0.85) | 0.0206 | 0.31 (0.12 to 0.78) | 0.0130 |
Owing to the reduced number of data for these analyses, for DRP, the full interaction between period and type could not be estimated because there were zero deaths observed for buprenorphine treatment in the first 4 weeks. Estimates of the partial interaction (with 2 df instead of 3 df) were obtained by excluding all data for buprenorphine in the first 4 weeks of treatment.
Instrumental variable analysis
We explored the usefulness of a GP’s previous prescription as an IV. 58,59 Because a treatment episode typically involved many prescriptions, we concentrated on the initiation of treatment as the critical time when the choice of medication was made. There were 3409 GPs associated with prescribing methadone or buprenorphine at this time. Their histories of treatment initiation covered 26,546 episodes, with an additional 728 episodes predating the study period (Figure 6). Because the time of day was unavailable for prescriptions within CPRD, 4560 episodes (13%) initiated on the same day by the same GP were assigned a random generation sequence. There were sufficient histories to provide information on the previous prescription for 2213 GPs (65%).
FIGURE 6.
Flow chart of data available for IV analyses. The previous GP prescription was used as an IV. About 35% of GPs had initiated an OST episode on only one occasion and so were excluded from the analyses. For 33–40% of GPs, data were available before the study start date. Hence, the number of available episodes did not reduce by the number of GPs. GPs who prescribed only one medication, either methadone or buprenorphine, were also excluded as the IV would be equivalent to the prescribed medication and its associated confounding.
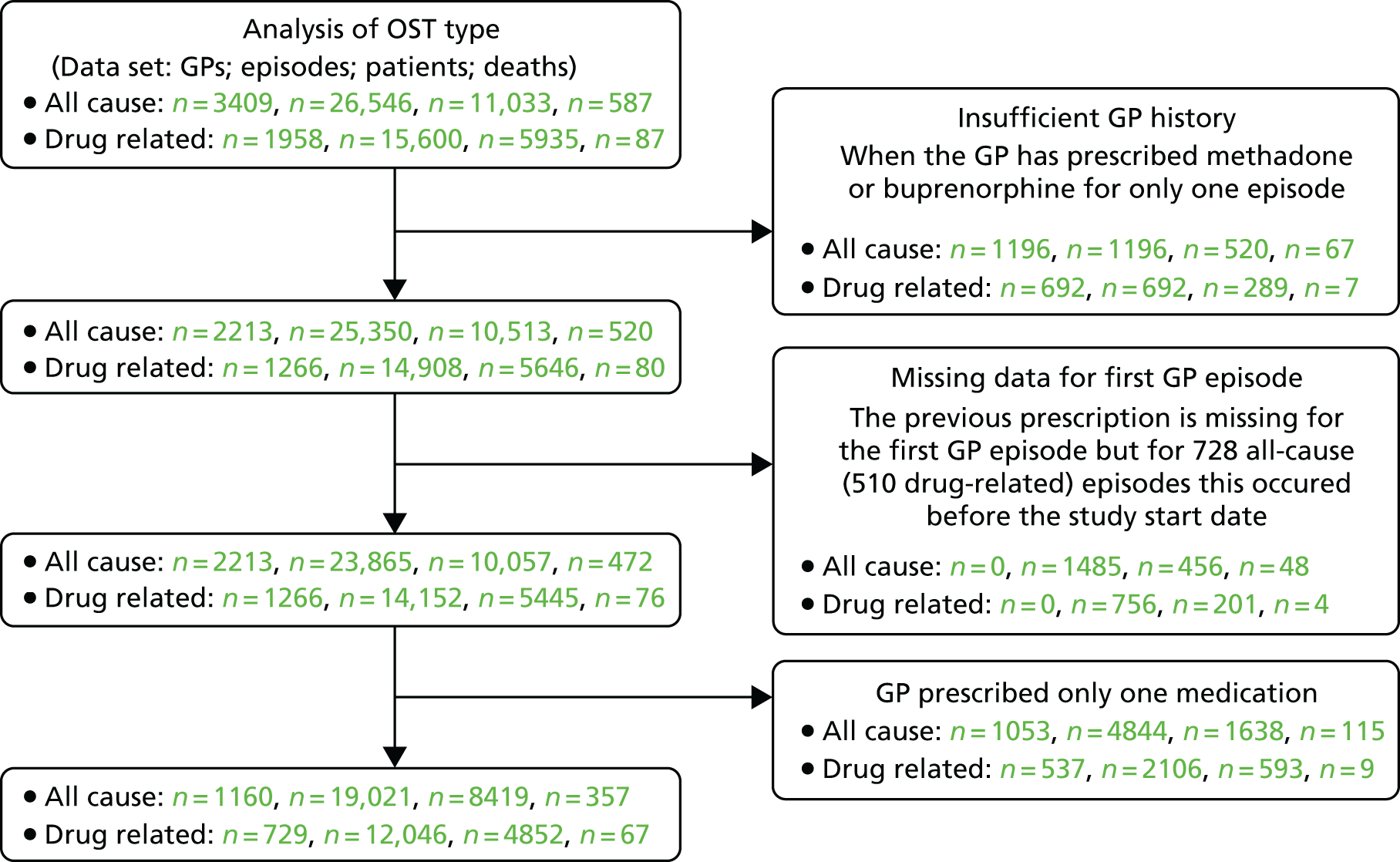
The majority of GPs tended to have a history of prescribing only one type of medication at the initiation of treatment, with 1269 (37%) prescribing methadone and 959 (28%) prescribing buprenorphine. However, these GPs were generally associated with shorter histories such that these 65% of GPs accounted for only 8606 (25%) episodes. These GPs were excluded from the IV analyses.
Using the previous prescription as an IV reduced the sample to 8419 patients, 19,021 episodes and 357 deaths in ACM analyses, and 4852 patients, 12,046 episodes and 67 deaths in DRP analyses. The proportions of buprenorphine episodes, 34%, remained similar to the 35% for current prescriptions. By contrast, the proportion of those episodes associated with deaths, 31%, doubled from the 14% with current prescriptions.
An investigation of the criteria for a valid IV showed strong associations between the choice of prescribed medication and IVs. Agreement occurred in 71% of episodes for ACM and in 70% of episodes for DRP. However, the IV remained associated with confounders with an R2 of 10.5% and 6.1% for ACM and DRP, respectively. These associations were similar to those observed between OST type and confounders (Table 9a). These results suggested that previous prescription would act as a poor IV. Similar conclusions were reached when regression coefficients were compared (Table 9b).
Confoundinga | Type of analysis | |||
---|---|---|---|---|
Adjusted | IPW | PSM | IV | |
Gender | 0.33 | 0.01 | 0.05 | 0.19 |
Age | 5.64 | 0.04 | 0.17 | 4.45 |
Year | 0.01 | 0.69 | 1.21 | 0.63 |
Comorbidity score | 2.90 | 0.52 | 0.79 | 2.27 |
Region | 5.75 | 1.72 | 1.53 | 6.47 |
All | 11.38 | 3.16 | 3.60 | 10.52 |
Matchingb | 1.27 | 0.17 | 0.01 | 1.22 |
n (episodes) | 26,546 | 26,546 | 13,940 | 19,021 |
n (patients) | 11,033 | 11,033 | 5921 | 7582 |
n (deaths) | 587 | 587 | 410 | 357 |
Variable | Category | Type of analysis | |||
---|---|---|---|---|---|
Adjusted | IPW | PSM | IV | ||
Gender | Male | –0.017 | 0.008 | 0.005 | –0.016 |
Female | 0 (reference) | ||||
Age (years) | < 30 | 0 (reference) | |||
30–39 | 0.018 | 0.023 | 0.014 | –0.010 | |
40–49 | 0.059 | 0.042 | 0.054 | 0.011 | |
≥ 50 | 0.162 | 0.001 | 0.014 | 0.145 | |
Year | 1998–9 | 0 (reference) | |||
2000–4 | 0.002 | –0.103 | –0.149 | 0.018 | |
2005–9 | –0.015 | –0.133 | –0.183 | 0.093 | |
2010–14 | –0.029 | –0.140 | –0.190 | 0.062 | |
Comorbidity score | 0 | 0 (reference) | |||
1 | –0.004 | –0.010 | 0.001 | 0.001 | |
≥ 2 | 0.057 | 0.068 | 0.080 | 0.066 | |
Region | North East | 0.362 | 0.159 | 0.160 | 0.336 |
North West | 0 (reference) | ||||
Yorkshire and the Humber | 0.110 | 0.029 | 0.035 | 0.064 | |
East Midlands | 0.146 | 0.066 | 0.086 | 0.181 | |
West Midlands | 0.089 | –0.005 | –0.006 | 0.063 | |
East | 0.052 | 0.009 | 0.017 | 0.050 | |
South West | 0.006 | –0.045 | –0.044 | 0.079 | |
South Central | 0.130 | 0.026 | 0.029 | 0.127 | |
London | –0.028 | –0.052 | –0.064 | –0.043 | |
South East | 0.096 | –0.001 | 0.074 | 0.093 | |
Northern Ireland | 0.045 | –0.140 | –0.167 | 0.097 | |
Scotland | –0.053 | –0.041 | 0.011 | –0.180 | |
Wales | 0.081 | –0.039 | –0.005 | 0.146 | |
R2 (%) | 11.38 | 3.16 | 3.60 | 10.52 |
Confounding
In the main report, confounding was assessed using unadjusted associations of predictors (in the propensity score model) with OST type. Comparing the predicted probabilities of being prescribed buprenorphine, the differences between categories for each predictor were reduced in IPW and PSM models compared with an unadjusted model (see table S2 in Hickman et al. 61). For instance, the differences between men and women in being prescribed buprenorphine reduced from 7% in an unadjusted model to 0.5% in the IPW model. In Table 9, we extend these analyses to report the multivariable associations between OST type and all confounders. These analyses were based on linear regressions between OST type (or the IV acting as a proxy for OST type) and confounders using the weights implicit in the Poisson regressions on mortality. Comparisons were made with the standard covariate adjusted model. In part (a), R2 statistics are reported for individual confounders and overall. In part (b), the regression coefficients for the multivariable models are reported. Both PSM and IPW reduced the associations with observed confounders, although the association with calendar year increased.
A comparison of confounder associations with mortality showed that all variables were associated with either ACM or DRP. Gender had a stronger association with DRP, whereas age and region had a stronger association with ACM (Table 10).
Confounder | Category | All-cause mortality | Drug-related mortality | ||
---|---|---|---|---|---|
IRR (95% CI) | p-value | IRR (95% CI) | p-value | ||
Gender | Male | 1.13 (0.95 to 1.34) | 0.1769 | 4.08 (2.04 to 8.15) | 0.0001 |
Female | 1 (reference) | 1 (reference) | |||
Age (years) | < 30 | 1 (reference) | < 0.0001 | 1 (reference) | 0.5135 |
30–39 | 1.20 (0.85 to 1.71) | 1.12 (0.62 to 2.02) | |||
40–49 | 2.06 (1.45 to 2.92) | 0.89 (0.44 to 1.79) | |||
≥ 50 | 3.27 (2.31 to 4.65) | 0.65 (0.28 to 1.54) | |||
Year | 1998–9 | 1 (reference) | 0.0028 | 1 (reference) | 0.0210 |
2000–4 | 0.72 (0.50 to 1.03) | 0.40 (0.20 to 0.82) | |||
2005–9 | 0.65 (0.46 to 0.93) | 0.33 (0.16 to 0.68) | |||
2010–14 | 0.54 (0.37 to 0.77) | 0.37 (0.18 to 0.79) | |||
Comorbidity score | 0 | 1 (reference) | < 0.0001 | 1 (reference) | 0.0007 |
1 | 1.39 (1.08 to 1.79) | 1.50 (0.92 to 2.46) | |||
≥ 2 | 11.68 (9.48 to 14.40) | 3.85 (1.90 to 7.81) | |||
Region | North East | 1.03 (0.53 to 2.01) | 0.0003 | n/e | 0.2529 |
North West | 1 (reference) | 1 (reference) | |||
Yorkshire and the Humber | 1.64 (1.07 to 2.53) | 0.25 (0.03 to 1.81) | |||
East Midlands | 1.69 (1.10 to 2.61) | 0.87 (0.26 to 2.90) | |||
West Midlands | 0.92 (0.62 to 1.35) | 0.60 (0.28 to 1.25) | |||
East | 1.88 (1.36 to 2.61) | 1.46 (0.75 to 2.84) | |||
South West | 1.08 (0.74 to 1.59) | 0.91 (0.46 to 1.77) | |||
South Central | 0.93 (0.63 to 1.38) | 0.44 (0.17 to 1.15) | |||
London | 1.31 (0.92 to 1.87) | 0.93 (0.43 to 2.00) | |||
South East | 1.50 (0.95 to 2.38) | 1.43 (0.59 to 3.49) | |||
Northern Ireland | 0.53 (0.13 to 2.17) | n/a | |||
Scotland | 1.39 (1.03 to 1.87) | n/a | |||
Wales | 0.95 (0.63 to 1.43) | n/a |
Key findings from the published paper
The overall mortality rates for ACM and DRP were 1.93 and 0.53 per 100 person-years, respectively. DRP was elevated in the first 4 weeks of treatment (IRR 1.93, 95% CI 0.97 to 3.82), the first 4 weeks after treatment ceased (IRR 8.15, 95% CI 5.45 to 12.19) and the remainder of time out of treatment (IRR 2.13, 95% CI 1.47 to 3.09), compared with mortality risk from 4 weeks to the end of treatment. Similar patterns of elevated risks by period were also observed for ACM, although the tendency was for higher IRRs than for DRP (first 4 weeks of treatment, IRR 2.98, 95% CI 2.44 to 3.64; first 4 weeks after treatment ceased, IRR 10.40, 95% CI 9.07 to 11.92; and the remainder of time out of treatment, IRR 2.77, 95% CI 2.42 to 3.17).
Patients on buprenorphine had lower ACM rates in each treatment period than those on methadone, with the strongest beneficial effect being associated with the first 4 weeks of treatment (IRR 0.04, 95% CI 0.01 to 0.15). After IPW adjustment, there was evidence of a lower DRP risk for patients on buprenorphine than for those on methadone at treatment initiation (IRR 0.08, 95% CI 0.01 to 0.48) and for the rest of time on treatment (IRR 0.37, 95% CI 0.17 to 0.79). Model estimates suggested that there was a low probability that methadone or buprenorphine reduced the number of DRPs in the population: 28% and 21%, respectively.
There was evidence that age and comorbidity interacted with OST type (p < 0.0024) such that buprenorphine may have lower ACM and DRP risks in older and more comorbid patients.
Sensitivity analyses did not change the conclusions (see table S4 in Hickman et al. 61).
Other sensitivity analyses not reported in published paper
An analysis of risk differences involved linear regression on the mortality rate associated with each record in the data file. The length of time associated with each record was used as a weight in the analysis. These analyses might provide an alternative description of risks to IRRs. Although the ordinal properties of these results matched those from Poisson regression (e.g. the treatment period with the lowest risk was on treatment after the first 4 weeks, and the highest was off treatment in the first 4 weeks), there were power issues for the treatment period effect for DRP (Table 11). This possibly reflected the low mortality rate for the reference period in DRP, producing a smaller range of differences in the linear regressions but a larger range in the ratios for the Poisson regressions.
Period | OST type | Unadjusted | Adjusteda | ||
---|---|---|---|---|---|
RD (95% CI) | p-value | RD (95% CI) | p-value | ||
All-cause mortality | |||||
On 1–4 weeks | 2.13 (–0.03 to 4.30) | < 0.0001 | 2.31 (0.13 to 4.50) | < 0.0001 | |
On rest | 0 (reference) | 0 (reference) | |||
Off 1–4 weeks | 8.56 (6.50 to 10.61) | 8.70 (6.62 to 10.78) | |||
Off rest | 1.21 (0.15 to 2.26) | 1.67 (0.58 to 2.76) | |||
On 1–4 weeks | Methadone | 0 (reference) | < 0.0001 | 0 (reference) | < 0.0001 |
Buprenorphine | –4.05 (–8.47 to 0.38) | 0.0734 | –5.95 (–10.41 to –1.50) | 0.0088 | |
On rest (reference) | Methadone | 0 (reference) | 0 (reference) | ||
Buprenorphine | –0.46 (–1.98 to 1.06) | 0.5513 | –2.11 (–3.70 to –0.52) | 0.0093 | |
Off 1–4 weeks | Methadone | 0 (reference) | 0 (reference) | ||
Buprenorphine | –11.40 (–15.47 to –7.34) | < 0.0001 | –13.63 (–17.73 to –9.53) | < 0.0001 | |
Off rest | Methadone | 0 (reference) | 0 (reference) | ||
Buprenorphine | –1.05 (–2.91 to 0.80) | 0.2667 | –2.95 (–4.86 to –1.05) | 0.0024 | |
Drug-related mortality | |||||
On 1–4 weeks | 0.60 (–0.76 to 1.95) | 0.1327 | 0.61 (–0.76 to 1.98) | 0.1387 | |
On rest | 0 (reference) | 0 (reference) | |||
Off 1–4 weeks | 1.43 (0.16 to 2.69) | 1.45 (0.17 to 2.73) | |||
Off rest | 0.35 (–0.32 to 1.02) | 0.36 (–0.34 to 1.05) | |||
On 1–4 weeks | Methadone | 0 (reference) | 0.8816 | 0 (reference) | 0.8928 |
Buprenorphine | –0.94 (–3.62 to 1.73) | 0.4891 | –0.87 (–3.56 to 1.81) | 0.5242 | |
On rest (reference) | Methadone | 0 (reference) | 0 (reference) | ||
Buprenorphine | –0.15 (–1.09 to 0.79) | 0.7487 | –0.16 (–1.12 to 0.80) | 0.7438 | |
Off 1–4 weeks | Methadone | 0 (reference) | 0 (reference) | ||
Buprenorphine | 0.27 (–2.16 to 2.71) | 0.8252 | 0.36 (–2.10 to 2.82) | 0.7742 | |
Off rest | Methadone | 0 (reference) | 0 (reference) | ||
Buprenorphine | –0.51 (–1.62 to 0.61) | 0.3704 | –0.46 (–1.60 to 0.68) | 0.4281 |
We also more formally took account of the effect of the censoring of follow-up by performing survival analysis instead of Poisson regression (Table 12). Right-censoring occurred when patients left a CPRD primary care practice, or if treatment episodes extended beyond the study end or CPRD’s last data collection dates. The results of this analysis were very similar to the adjusted Poisson results.
Period | OST type | Unadjusted | Adjusteda | ||
---|---|---|---|---|---|
HR (95% CI) | p-value | HR (95% CI) | p-value | ||
All-cause mortality | |||||
On 1–4 weeks | 3.05 (2.21 to 4.20) | < 0.0001 | 3.06 (2.21 to 4.25) | < 0.0001 | |
On rest | 1 (reference) | 1 (reference) | |||
Off 1–4 weeks | 8.62 (6.96 to 10.68) | 9.49 (7.59 to 11.86) | |||
Off rest | 2.44 (1.99 to 3.00) | 2.79 (2.26 to 3.44) | |||
On 1–4 weeks | Methadone | 1 (reference) | < 0.0001 | 1 (reference) | 0.0002 |
Buprenorphine | 0.03 (0.01 to 0.12) | < 0.0001 | 0.04 (0.01 to 0.15) | < 0.0001 | |
On rest (reference) | Methadone | 1 (reference) | 1 (reference) | ||
Buprenorphine | 0.25 (0.16 to 0.39) | < 0.0001 | 0.25 (0.15 to 0.39) | < 0.0001 | |
Off 1–4 weeks | Methadone | 1 (reference) | 1 (reference) | ||
Buprenorphine | 0.05 (0.03 to 0.09) | < 0.0001 | 0.07 (0.04 to 0.12) | < 0.0001 | |
Off rest | Methadone | 1 (reference) | 1 (reference) | ||
Buprenorphine | 0.21 (0.14 to 0.29) | < 0.0001 | 0.21 (0.15 to 0.30) | < 0.0001 | |
Drug-related mortality | |||||
On 1–4 weeks | 3.09 (1.40 to 6.83) | < 0.0001 | 2.94 (1.32 to 6.59) | < 0.0001 | |
On rest | 1 (reference) | 1 (reference) | |||
Off 1–4 weeks | 6.05 (3.32 to 11.03) | 5.98 (3.23 to 11.07) | |||
Off rest | 2.27 (1.36 to 3.78) | 2.27 (1.35 to 3.83) | |||
On 1–4 weeks | Methadone | 1 (reference) | 0.1705 | 1 (reference) | 0.1552 |
Buprenorphine | 0.27 (0.03 to 2.18) | 0.2178 | 0.27 (0.03 to 2.23) | 0.2248 | |
On rest (reference) | Methadone | 1 (reference) | 1 (reference) | ||
Buprenorphine | 0.57 (0.20 to 1.66) | 0.3064 | 0.57 (0.19 to 1.65) | 0.2973 | |
Off 1–4 weeks | Methadone | 1 (reference) | 1 (reference) | ||
Buprenorphine | 1.49 (0.58 to 3.87) | 0.4101 | 1.62 (0.63 to 4.19) | 0.3207 | |
Off rest | Methadone | 1 (reference) | 1 (reference) | ||
Buprenorphine | 0.39 (0.16 to 0.96) | 0.0403 | 0.42 (0.17 to 1.04) | 0.0613 |
We also considered whether or not clustering by patient biased the standard errors (SEs) used in standard analyses. There was some evidence of inflated SEs compared with standard Poisson analyses, but the changes were minor (Table 13). These analyses also changed IRR estimates, but, again, the changes were minor.
Period | OST type | Unadjusted | Adjusteda | ||
---|---|---|---|---|---|
IRR (95% CI) | p-value | IRR (95% CI) | p-value | ||
All-cause mortality | |||||
On 1–4 weeks | 2.60 (1.85 to 3.64) | < 0.0001 | 1.96 (1.37 to 2.80) | < 0.0001 | |
On rest | 1 (reference) | 1 (reference) | |||
Off 1–4 weeks | 10.51 (8.29 to 13.32) | 9.42 (7.42 to 11.95) | |||
Off rest | 3.03 (2.35 to 3.91) | 3.25 (2.57 to 4.12) | |||
On 1–4 weeks | Methadone | 1 (reference) | 0.0026 | 1 (reference) | 0.0022 |
Buprenorphine | 0.09 (0.02 to 0.36) | 0.0008 | 0.06 (0.01 to 0.27) | 0.0002 | |
On rest (reference) | Methadone | 1 (reference) | 1 (reference) | ||
Buprenorphine | 0.44 (0.26 to 0.75) | 0.0025 | 0.27 (0.16 to 0.47) | < 0.0001 | |
Off 1–4 weeks | Methadone | 1 (reference) | 1 (reference) | ||
Buprenorphine | 0.14 (0.08 to 0.24) | < 0.0001 | 0.07 (0.04 to 0.13) | < 0.0001 | |
Off rest | Methadone | 1 (reference) | 1 (reference) | ||
Buprenorphine | 0.36 (0.24 to 0.55) | < 0.0001 | 0.16 (0.10 to 0.25) | < 0.0001 | |
Drug-related mortality | |||||
On 1–4 weeks | 3.21 (1.44 to 7.17) | < 0.0001 | 3.04 (1.31 to 7.06) | < 0.0001 | |
On rest | 1 (reference) | 1 (reference) | |||
Off 1–4 weeks | 6.59 (3.51 to 12.36) | 7.05 (3.64 to 13.68) | |||
Off rest | 2.42 (1.40 to 4.20) | 2.87 (1.53 to 5.40) | |||
On 1–4 weeks | Methadone | 1 (reference) | 0.2612 | 1 (reference) | 0.1687 |
Buprenorphine | 0.26 (0.03 to 2.11) | 0.2058 | 0.29 (0.03 to 2.58) | 0.2681 | |
On rest (reference) | Methadone | 1 (reference) | 1 (reference) | ||
Buprenorphine | 0.58 (0.19 to 1.77) | 0.3395 | 0.69 (0.20 to 2.43) | 0.5659 | |
Off 1–4 weeks | Methadone | 1 (reference) | 1 (reference) | ||
Buprenorphine | 1.27 (0.47 to 3.38) | 0.6369 | 1.62 (0.56 to 4.72) | 0.3776 | |
Off rest | Methadone | 1 (reference) | 1 (reference) | ||
Buprenorphine | 0.38 (0.16 to 0.95) | 0.0390 | 0.40 (0.14 to 1.13) | 0.0836 |
In addition, we analysed subsets of data relating to either the first or the last episode. Any differences between these two sets of results might indicate a cumulative effect of repeated treatment on the risk of death. As the number of episodes increase, the first 4 weeks after treatment may be associated with an increased risk of ACM (Tables 14 and 15).
Period | OST type | Unadjusted | Adjusteda | ||
---|---|---|---|---|---|
IRR (95% CI) | p-value | IRR (95% CI) | p-value | ||
All-cause mortality | |||||
On 1–4 weeks | 4.87 (3.54 to 6.69) | < 0.0001 | 3.98 (2.88 to 5.51) | < 0.0001 | |
On rest | 1 (reference) | 1 (reference) | |||
Off 1–4 weeks | 19.57 (15.84 to 24.18) | 17.31 (13.90 to 21.57) | |||
Off rest | 2.82 (2.30 to 3.45) | 2.91 (2.36 to 3.59) | |||
On 1–4 weeks | Methadone | 1 (reference) | 0.0021 | 1 (reference) | 0.0040 |
Buprenorphine | 0.13 (0.03 to 0.52) | 0.0041 | 0.09 (0.02 to 0.37) | 0.0008 | |
On rest (reference) | Methadone | 1 (reference) | 1 (reference) | ||
Buprenorphine | 0.68 (0.43 to 1.06) | 0.0893 | 0.43 (0.27 to 0.67) | 0.0002 | |
Off 1–4 weeks | Methadone | 1 (reference) | 1 (reference) | ||
Buprenorphine | 0.24 (0.14 to 0.40) | < 0.0001 | 0.13 (0.08 to 0.22) | < 0.0001 | |
Off rest | Methadone | 1 (reference) | 1 (reference) | ||
Buprenorphine | 0.62 (0.44 to 0.87) | 0.0055 | 0.27 (0.19 to 0.39) | < 0.0001 | |
Drug-related mortality | |||||
On 1–4 weeks | 4.90 (2.23 to 10.79) | < 0.0001 | 4.41 (1.98 to 9.80) | < 0.0001 | |
On rest | 1 (reference) | 1 (reference) | |||
Off 1–4 weeks | 12.22 (6.73 to 22.19) | 10.58 (5.72 to 19.57) | |||
Off rest | 2.77 (1.67 to 4.59) | 2.44 (1.44 to 4.14) | |||
On 1–4 weeks | Methadone | 1 (reference) | 0.1848 | 1 (reference) | 0.1725 |
Buprenorphine | 0.32 (0.04 to 2.61) | 0.2887 | 0.35 (0.04 to 2.82) | 0.3211 | |
On rest (reference) | Methadone | 1 (reference) | 1 (reference) | ||
Buprenorphine | 0.66 (0.23 to 1.90) | 0.4407 | 0.64 (0.22 to 1.86) | 0.4089 | |
Off 1–4 weeks | Methadone | 1 (reference) | 1 (reference) | ||
Buprenorphine | 1.53 (0.60 to 3.88) | 0.3684 | 1.67 (0.65 to 4.33) | 0.2869 | |
Off rest | Methadone | 1 (reference) | 1 (reference) | ||
Buprenorphine | 0.40 (0.17 to 0.96) | 0.0411 | 0.43 (0.17 to 1.06) | 0.0667 |
Period | OST type | Unadjusted | Adjusteda | ||
---|---|---|---|---|---|
IRR (95% CI) | p-value | IRR (95% CI) | p-value | ||
All-cause mortality | |||||
On 1–4 weeks | 3.94 (2.64 to 5.87) | < 0.0001 | 3.03 (2.01 to 4.56) | < 0.0001 | |
On rest | 1 (reference) | 1 (reference) | |||
Off 1–4 weeks | 12.69 (9.77 to 16.49) | 10.66 (8.12 to 14.00) | |||
Off rest | 2.01 (1.54 to 2.60) | 2.10 (1.60 to 2.75) | |||
On 1–4 weeks | Methadone | 1 (reference) | 0.0103 | 1 (reference) | 0.0473 |
Buprenorphine | 0.10 (0.01 to 0.71) | 0.0215 | 0.07 (0.01 to 0.49) | 0.0080 | |
On rest (reference) | Methadone | 1 (reference) | 1 (reference) | ||
Buprenorphine | 0.55 (0.30 to 0.99) | 0.0477 | 0.29 (0.16 to 0.53) | 0.0001 | |
Off 1–4 weeks | Methadone | 1 (reference) | 1 (reference) | ||
Buprenorphine | 0.17 (0.08 to 0.37) | < 0.0001 | 0.09 (0.04 to 0.19) | < 0.0001 | |
Off rest | Methadone | 1 (reference) | 1 (reference) | ||
Buprenorphine | 0.66 (0.41 to 1.05) | 0.0817 | 0.26 (0.16 to 0.42) | < 0.0001 | |
Drug-related mortality | |||||
On 1–4 weeks | 4.73 (1.53 to 14.67) | < 0.0001 | 3.90 (1.24 to 12.26) | < 0.0001 | |
On rest | 1 (reference) | 1 (reference) | |||
Off 1–4 weeks | 11.36 (5.01 to 25.75) | 8.37 (3.61 to 19.42) | |||
Off rest | 2.69 (1.29 to 5.63) | 2.01 (0.94 to 4.31) | |||
On 1–4 weeks | Methadone | 1 (reference) | 0.8840 | 1 (reference) | 0.9537 |
Buprenorphine | 0.73 (0.08 to 7.02) | 0.7852 | 0.82 (0.08 to 8.06) | 0.8679 | |
On rest (reference) | Methadone | 1 (reference) | 1 (reference) | ||
Buprenorphine | 1.29 (0.35 to 4.78) | 0.6993 | 1.33 (0.35 to 5.04) | 0.6721 | |
Off 1–4 weeks | Methadone | 1 (reference) | 1 (reference) | ||
Buprenorphine | 0.73 (0.19 to 2.77) | 0.6472 | 0.90 (0.23 to 3.53) | 0.8851 | |
Off rest | Methadone | 1 (reference) | 1 (reference) | ||
Buprenorphine | 0.66 (0.21 to 2.02) | 0.4635 | 0.83 (0.26 to 2.63) | 0.7479 |
Finally, we analysed ACM using different gaps in patient prescription histories to indicate a new treatment episode. Decreasing the gap from 28 days in main analyses increased the number of episodes but also increased the number of patients (Table 16). This was a result of treatment durations being shortened, thereby increasing the chance that only one medication was prescribed. Conversely, increasing the gap decreased the number of episodes and patients. The interaction effects were relatively robust to changes in the definition of episodes with changes in effect sizes restricted to the treatment period main effect.
Period | OST type | 7 days | 56 days | ||
---|---|---|---|---|---|
IRR (95% CI) | p-value | IRR (95% CI) | p-value | ||
On 1–4 weeks | 2.05 (1.53 to 2.74) | < 0.0001 | 3.76 (2.66 to 5.32) | < 0.0001 | |
On rest | 1 (reference) | 1 (reference) | |||
Off 1–4 weeks | 5.87 (4.72 to 7.31) | 15.00 (12.06 to 18.66) | |||
Off rest | 2.82 (2.29 to 3.46) | 2.86 (2.32 to 3.52) | |||
On 1–4 weeks | Methadone | 1 (reference) | < 0.0001 | 1 (reference) | 0.0029 |
Buprenorphine | 0.06 (0.02 to 0.16) | < 0.0001 | 0.03 (0.00 to 0.21) | 0.0005 | |
On rest (reference) | Methadone | 1 (reference) | 1 (reference) | ||
Buprenorphine | 0.30 (0.19 to 0.48) | < 0.0001 | 0.24 (0.15 to 0.38) | < 0.0001 | |
Off 1–4 weeks | Methadone | 1 (reference) | 1 (reference) | ||
Buprenorphine | 0.08 (0.05 to 0.13) | < 0.0001 | 0.08 (0.05 to 0.14) | < 0.0001 | |
Off rest | Methadone | 1 (reference) | 1 (reference) | ||
Buprenorphine | 0.23 (0.17 to 0.33) | < 0.0001 | 0.21 (0.15 to 0.31) | < 0.0001 | |
Episodes; patients; deaths | 56,058; 11,590; 617 | 19,277; 10,706; 573 |
Summary
All analyses showed an increased risk of mortality in the first 4 weeks of treatment and the first 4 weeks after treatment ceased, compared with after 4 weeks on treatment. These results are consistent with other reports. 53,64 Overall, mortality rates were higher during off treatment than during on treatment. 30,64–66
All analyses for ACM and IPW analyses for DRP showed the presence of an interaction with OST type, suggesting that the association in the first 4 weeks of treatments was more pronounced with methadone than with buprenorphine. These results are consistent with a recent Australian study. 53 However, although we found a similar difference in risk for the remainder of time on treatment, that study found no differences between methadone and buprenorphine. Our IRRs comparing buprenorphine with methadone for on- and off-treatment periods are consistent with pooled estimates from a recent systematic review. 64
The presence of an OST type by period interaction only in IPW for DRP may reflect the reduced power associated with only 87 deaths. Although this may be an indication of under-reporting of DRP in causes of death, the availability of such information for only 50% of patients within CPRD at the time of this study was a major contributory factor to the reduction in power.
As previously reported,15–17,25,53 we found increased mortality risks immediately following the cessation of treatment, but, in comparing different OST types, there were some differences. Whereas our data suggested lower risks for buprenorphine with ACM but not with DRP, by contrast, the Australian study reported a lower risk for methadone with DRP during the first 4 weeks following cessation. 53
It is likely that the differences during treatment cessation after the first 4 weeks are indicative of residual confounding or confounding by indication. Owing to the half-lives of OST medications, any direct pharmacological effects of either medication are likely to be small. 7 However, there may be indirect effects, such as reduced opioid tolerance, with consequences for any relapse to drug abuse. 36 Whether methadone and buprenorphine treatment differ in these respects is unclear. In addition, one would expect any differences in medication to diminish with time. Although this was observed for ACM, with differences halving compared with the first 4 weeks, such a change was not observed for DRP.
Various sensitivity analyses involving different analysis techniques and different subsets of data did not change these conclusions. Although propensity score and IV methods are well-established techniques, we also explore modifications to the SCCS methods in Chapter 8.
It should be noted that we lacked information about patients’ illicit drug use during their treatment. It is possible that some patients resorted to this to overcome withdrawal symptoms, potentially increasing mortality. Another limitation was the use of naloxone, which may have reduced overdose deaths.
Chapter 6 Co-prescription of benzodiazepines, z-drugs and gabapentinoids with opiate substitution treatment
In Chapter 4, we reported that about 50% of patients received benzodiazepines, z-drugs or gabapentinoids in addition to OST. Given that current treatment guidelines discourage this practice,34,35 in this chapter on WP 3 we will explore the effects on mortality of co-prescription.
Aims and objectives
Our main aim was to explore the effects of co-prescription on mortality risk. For comparison with a recent study,31 we also analysed deaths from non-drug related causes in addition to ACM and DRP. Given the strong effects on OST type and treatment period noted in WP 2, we also investigated whether or not the co-prescription effects varied with these OST factors.
Data set for the main analyses
There were 29,540 OST episodes involving methadone or buprenorphine (see Figure 2). As our primary aim was not to compare OST medications, we included partial episodes of methadone and buprenorphine to increase the power of comparisons. These episodes related to 12,118 patients, of whom 42% received benzodiazepine co-prescription, 20% received z-drug co-prescription and 8% received gabapentinoid co-prescription. In 36,126 person-years of follow-up there were 657 ACM deaths.
Key findings from the submitted paper
The results for co-prescription of the three medications with OST are summarised in Table 17. All co-prescribed medications were related to ACM and DRP such that co-prescription increased the risk of mortality. There was evidence of a dose–response relationship for benzodiazepines with DRP but not with ACM. Only gabapentinoids were associated with an increase in risk of non-drug-related mortality.
Co-prescribed | Mortality | ||
---|---|---|---|
All cause | Drug related | Non-drug related | |
Benzodiazepines | 1.16 | 2.02 (D) | |
z-drugs | 1.83 | 3.31 | |
Gabapentinoids | 1.99 | 1.60 | 2.15 |
Concurrent prescription of benzodiazepine was associated with an approximate doubling of the duration of methadone treatment (adjusted mean duration of treatment episode 444 days compared with 288 days). In analyses considering this increased duration of OST, the overall adverse effect on mortality risk was still apparent (DRP with benzodiazepine concurrent prescription compared with patients with no concurrent exposure; adjusted IRR 4.04, 95% CI 2.35 to 6.95).
Sensitivity analyses were performed using survival analysis and Poisson regression excluding the first OST episode. Those results were consistent with those of the main analyses.
Interactions with opiate substitution treatment type and period
In the previous chapter, we found OST type to interact with a number of factors, including OST period, age and comorbidity. Consequently, it seemed plausible that OST type may also interact with these three co-prescribed medications. In addition, there were key questions concerning the timing of these medications relating to whether or not the exposure was concurrent with OST. A priori, one might have expected a greater effect concurrent with OST than during periods after OST has ceased. These issues were explored using an interaction with OST period.
The results from these analyses are summarised in Table 18. To take account of the possibility that OST period with four categories may be underpowered to detect concurrent specific effects, interactions were also fitted using a two-level factor reflecting on and off OST. Overall, there was no evidence of any interactions. The strongest evidence was for gabapentinoids with ACM. These data suggested that gabapentinoids had an adverse association with mortality for methadone only, with no effect for buprenorphine episodes. The interaction with period suggested an adverse effect only for the period of treatment after the first 4 weeks and the first 4 weeks after treatment cessation. However, given the number of statistical tests being employed, both of these results may be chance events.
Medication | All cause | Drug related | Non-drug related | ||||||
---|---|---|---|---|---|---|---|---|---|
Type | Period4 | Period2 | Type | Period4 | Period2 | Type | Period4 | Period2 | |
B on/off | 0.7437 | 0.4499 | 0.5014 | 0.2006 | 0.2781 | 0.5665 | 0.9805 | 0.7949 | 0.5811 |
B low/high | 0.1051 | 0.3771 | 0.2885 | 0.6201 | 0.2054 | 0.2337 | 0.5236 | 0.9148 | 0.5807 |
B linear | 0.3009 | 0.3954 | 0.2822 | 0.1505 | 0.4055 | 0.5145 | 0.7532 | 0.6707 | 0.4324 |
Z on/off | 0.4284 | 0.3633 | 0.2646 | 0.1268 | 0.4070 | 0.7308 | 0.6749 | 0.6550 | 0.3995 |
Z low/high | 0.5722 | 0.1932 | 0.0648 | 0.2565 | 0.8235 | 0.9106 | 0.8477 | 0.2834 | 0.0763 |
Z linear | 0.6374 | 0.1633 | 0.0822 | 0.1765 | 0.6945 | 0.7636 | 0.7826 | 0.3953 | 0.1031 |
G on/off | 0.0059 | 0.0022 | 0.0419 | 0.0979 | 0.0795 | 0.1412 | 0.1807 | 0.6647 |
Co-prescription and the opiate substitution treatment type × period interaction
Given that the OST type propensity score included a contribution from co-prescription, it was expected that further adjustment for co-prescription would have little effect on this interaction. In addition, given the similarity in IPW analyses with standard Poisson regression for ACM, it might also be expected that these results would not be materially affected. These assumptions were verified in Table 19.
Period | OST type | All-cause mortalitya | Drug-related mortalitya | ||
---|---|---|---|---|---|
IRR (95% CI) | p-value | IRR (95% CI) | p-value | ||
On 1–4 weeks | 3.35 (2.44 to 4.60) | < 0.0001 | 3.24 (1.54 to 6.79) | < 0.0001 | |
On rest | 1 (reference) | 1 (reference) | |||
Off 1–4 weeks | 11.82 (9.58 to 14.57) | 7.36 (4.22 to 12.83) | |||
Off rest | 3.43 (2.82 to 4.17) | 3.33 (2.12 to 5.25) | |||
On 1–4 weeks | Methadone | 1 (reference) | 0.0007 | 1 (reference) | 0.0799 |
Buprenorphine | 0.04 (0.01 to 0.18) | < 0.0001 | 0.23 (0.03 to 1.83) | 0.1643 | |
On rest (reference) | Methadone | 1 (reference) | 1 (reference) | ||
Buprenorphine | 0.25 (0.16 to 0.39) | < 0.0001 | 0.37 (0.13 to 1.07) | 0.0661 | |
Off 1–4 weeks | Methadone | 1 (reference) | 1 (reference) | ||
Buprenorphine | 0.09 (0.06 to 0.15) | < 0.0001 | 1.49 (0.62 to 3.59) | 0.3700 | |
Off rest | Methadone | 1 (reference) | 1 (reference) | ||
Buprenorphine | 0.24 (0.17 to 0.33) | < 0.0001 | 0.43 (0.21 to 0.90) | 0.0256 |
Summary
We found that co-prescription of benzodiazepines was associated with an increased risk of DRP. This was consistent with most studies,26,28–30 although one found no association. 31 Although we also found weak associations with ACM, the evidence from other studies was more equivocal. 26,31,67 We found z-drugs and gabapentinoids to be associated with DRP, as found in one other study. 31 For ACM, our positive association was inconsistent with the only other study involving OST patients,31 although there was some evidence of increased risk for these medications for opioid users. 32,33 Whether the association with gabapentinoids is a reflection of the medication itself or confounding by the illnesses it is trying to treat remains unclear.
Our results have implications for clinical practice for OST. With over one-quarter of OST patients receiving benzodiazepines, the increased mortality risk associated with their co-prescription suggests that this may include an avoidable number of risks if suitable alternative treatment can be found. For instance, if they have been prescribed for psychological stress, other means of support may be available. For z-drugs, similar drug-related mortality risks and higher ACM risks were observed in this study, suggesting that similar warnings concerning co-prescription are warranted for this class of medications. With the co-prescription of gabapentinoids being relatively rare in this study, and with few other studies examining these medications, the implications for policy are less clear for gabapentinoids.
Chapter 7 Initiation and cessation of opiate substitution treatment
Initiation and cessation of OST are key periods in the treatment of opioid abuse when risks of overdose and withdrawal need to be minimised. Current clinical guidance recommends a low initial dose with steady increases to a maintenance level and gradual reduction in dose leading to a low final dose. 7,36 As adherence to these guidelines was poor, as seen in Chapter 4, in this chapter on WP 4, we explore whether or not these deviations had any adverse impact on mortality.
Aims and objectives
In this WP, we planned to investigate whether or not the risk of mortality is reduced with:
-
supervised consumption of OST medication
-
low initial doses with increasing doses over the first 28 days of treatment
-
low final doses with decreasing doses over the last 28 days of treatment.
Our primary aims were to explore the associations of these factors with mortality during the first 28 days of treatment for initiation and the first 28 days following the end of treatment for cessation. Analyses would explore whether or not these associations differed by OST type.
In addition, we explored whether or not adherence to current guidelines for initiation and cessation varied over time.
Data set
As we were concerned with only the first or last 28 days of a treatment episode, we restricted episodes to those involving only methadone or buprenorphine during these times. The data used in these analyses related to 11,289 patients and 26,544 episodes (see Figure 2). However, owing to differing criteria for initiation or cessation (see Initiation of opiate substitution treatment and Cessation of opiate substitution treatment), and to some episodes being only partially included within the study period (and hence the initiation or cessation periods may be excluded), not all of these data were used in any one analysis.
Supervised consumption
From the supplied CPRD data, it was possible to identify supervised consumption only from the free text associated with each prescription. Searches revealed 4071 prescriptions, with 3831 using the phrase ‘supervised administration’ and 240 using ‘supervised consumption’. These related to 184 patients and were all for methadone. Searches involving likely spelling mistakes or abbreviations discovered no additional prescriptions.
We requested CPRD to search its databases, including information not normally released. It identified 16,750 prescriptions for 591 patients for the whole database (not just our data set) covering the period 1987 to March 2015.
Overall, we concluded that the prevalence of supervised consumption was seriously under-reported and that using the available data would be underpowered to address research objectives as a result of both the low observed prevalence and the dilution of effects as a result of misclassification.
Initiation of opiate substitution treatment
We characterised the initiation of treatment using two measures: the starting dose and the change in dose during the first 28 days. Episodes were included in the analyses where only one medication was prescribed in the first 28 days, there was valid dose information for at least part of this time and some part of the first 28 days of treatment occurred between the study dates. This led to 10,817 patients providing data for 25,246 episodes.
Cessation of opiate substitution treatment
Similarly, ending dose and the change in dose over the last 28 days were used to describe cessation. Treatment could end by planned cessation, dropout or death. Episodes were included in the analyses where only one medication was prescribed during the last 28 days, there was valid dose information for at least part of this time, the duration exceeded 90 days and some part of the first 28 days following cessation occurred between the study dates. It should be noted that episodes were excluded where treatment had not ceased but data were unavailable owing to loss to follow-up. This led to 6491 patients providing data for 10,811 episodes.
Analyses
We investigated the impact of initiation and cessation characteristics on ACM and DRP.
Initiation and cessation characteristics were analysed both as linear covariates and as categorical factors to allow for non-linear effects. Methadone doses for the linear covariates were divided by five to achieve a dose more equivalent to buprenorphine. For starting/ending doses, a four-level factor was derived, with the lowest dose category defined as ≤ 20 mg (≤ 4 mg) of methadone (buprenorphine) and increasing by 20 mg (4 mg) per category until the highest dose category of > 60 mg (> 12 mg). For change in dose, this was categorised as binary variables, with any increase in dose for initiation or any decrease in dose for cessation being expected to be more favourable in terms of mortality than no increase or decrease, respectively.
Although standard adjustment was made for gender, age, year, comorbidity and UK region, we also adjusted for OST type as an additional model. The inclusion of OST type allowed proportional effects (parallel effects on a log scale) to be investigated. Previous interactions with OST type have been observed in WP 2 and it seemed advisable also to consider the possibility that initiation and cessation characteristics might interact with OST type.
Adherence to the guidelines7 was based on low starting or ending doses and optimal changes in dose during the first 28 days after either the start or the end of treatment.
Results
Initiation and cessation characteristics
The results from the linear growth models are shown in Table 20. Characteristics differed between methadone and buprenorphine. Both starting and ending doses for methadone episodes were evenly distributed across the four categories, with 21% to 27% of episodes being associated with the lowest dose. By contrast, for buprenorphine, ≈50% of episodes started or ended with the lowest dose category. Most episodes showed little evidence of any change in dose, with only about 30% of episodes showing increases during initiation or decreases during cessation. The exception appeared to be the change in dose for methadone episodes during the first 28 days. Here, 68% showed some increase in dose, but, as the mean suggests, the overall increases were small.
Initiation (first 28 days) | Cessation (last 28 days) | ||||
---|---|---|---|---|---|
Daily dose | Methadone | Buprenorphine | Daily dose | Methadone | Buprenorphine |
Lowesta | 3564 (20.75) | 3879 (47.27) | Lowesta | 2258 (27.79) | 1407 (51.31) |
6028 (35.10) | 1701 (20.73) | 1969 (24.24) | 726 (26.48) | ||
4054 (23.61) | 1488 (18.13) | 1695 (20.86) | 252 (9.19) | ||
Highest | 3527 (20.54) | 1138 (13.87) | Highest | 2202 (27.10) | 357 (13.02) |
Mean (SD) | 42.56 (30.39) | 6.08 (6.51) | 43.12 (34.55) | 5.57 (6.04) | |
n (episodes) | 17,173 | 8206 | 8124 | 2742 | |
Change in dose | |||||
No increase | 5494 (31.99) | 5044 (61.47) | No decrease | 5674 (69.84) | 1934 (70.53) |
Any increase | 11,679 (68.01) | 3162 (38.53) | Any decrease | 2450 (30.16) | 808 (29.47) |
Mean (SD) | 1.20 (23.29) | –0.28 (5.25) | 2.87 (27.89) | 0.25 (4.42) | |
n (episodes) | 17,173 | 8206 | 8124 | 2742 |
Initiation and mortality
Only about 7% of the deaths available in WP 3 were valid for these analyses. This led to 48 ACM and eight DRP deaths (Table 21).
Dose | Category | Deaths | PY | Unadjusted | Adjusteda | Adjusteda + OST type | ||||
---|---|---|---|---|---|---|---|---|---|---|
MR | HR (95% CI) | p-value | HR (95% CI) | p-value | HR (95% CI) | p-value | ||||
ACM | ||||||||||
Starting | Linearb | 1.07 (1.03 to 1.10) | 0.0001 | 1.05 (1.02 to 1.09) | 0.0044 | 1.04 (1.00 to 1.09) | 0.0413 | |||
Starting | Lowestc | 13 | 434 | 3.00 | 1 (reference) | 0.0795 | 1 (reference) | 0.2168 | 1 (reference) | 0.5574 |
15 | 499 | 3.01 | 2.31 (0.99 to 5.34) | 1.76 (0.65 to 4.76) | 0.76 (0.25 to 2.28) | |||||
9 | 360 | 2.50 | 2.27 (0.87 to 5.92) | 3.03 (0.99 to 9.21) | 1.56 (0.48 to 5.08) | |||||
Highest | 11 | 297 | 3.70 | 3.03 (1.27 to 7.26) | 2.39 (0.86 to 6.69) | 1.32 (0.45 to 3.90) | ||||
Change | Linearb | 0.94 (0.89 to 0.99) | 0.0195 | 0.94 (0.89 to 0.99) | 0.0238 | 0.95 (0.90 to 1.00) | 0.0655 | |||
Change | No increase | 14 | 650 | 2.15 | 0.54 (0.28 to 1.02) | 0.0565 | 0.69 (0.33 to 1.45) | 0.3249 | 1.04 (0.46 to 2.36) | 0.9279 |
Any increase | 34 | 939 | 3.62 | 1 (reference) | 1 (reference) | 1 (reference) | ||||
Drug-related mortality | ||||||||||
Starting | Linearb | 1.02 (0.91 to 1.15) | 0.6979 | 1.04 (0.88 to 1.23) | 0.6227 | 1.03 (0.86 to 1.23) | 0.7421 | |||
Starting | Lowestc | 2 | 278 | 0.72 | 1 (reference) | 0.8202 | 1 (reference) | 0.4125 | 1 (reference) | 0.3297 |
3 | 296 | 1.01 | 1.33 (0.20 to 8.87) | 0.21 (0.01 to 6.58) | 0.02 (0.00 to 3.62) | |||||
2 | 215 | 0.93 | 2.72 (0.34 to 22.11) | 3.52 (0.21 to 58.18) | 2.16 (0.13 to 34.79) | |||||
Highest | 1 | 148 | 0.67 | 1.73 (0.13 to 23.07) | 0.97 (0.03 to 30.81) | 0.32 (0.01 to 12.88) | ||||
Change | Linearb | 1.00 (0.81 to 1.25) | 0.9902 | 0.97 (0.70 to 1.34) | 0.8436 | 0.93 (0.68 to 1.27) | 0.6436 | |||
Change | No increase | 2 | 412 | 0.49 | 0.50 (0.08 to 3.01) | 0.4479 | 0.98 (0.12 to 8.35) | 0.9873 | 1.69 (0.18 to 15.59) | 0.6447 |
Any increase | 6 | 525 | 1.14 | 1 (reference) | 1 (reference) | 1 (reference) |
Higher starting doses were associated with an increased risk of ACM. Unadjusted results suggested a 7% increase in risk for every 5-mg increase in the starting dose of methadone (1-mg increase in buprenorphine) (HR 1.07, 95% CI 1.03 to 1.10). Adjustment for confounders and then OST type attenuated the results, but the association remained (HR 1.04, 95% CI 1.00 to 1.09). Categorising the starting dose may suggest that the biggest change in risk was exceeding low doses, although the CIs were too wide to obtain robust statistical evidence. There was some evidence that increasing the dose over the first 28 days also appeared to be beneficial, reducing mortality risks by 5% (HR 0.95, 95% CI 0.90 to 1.00).
An analysis of DRP produced similar results to that of ACM in terms of point estimates, with a 3% increase in risk being associated with the linear starting dose effect and a 7% reduction in risk for the linear change in dose effect. However, with only eight deaths, the CIs were wide and the statistical evidence weak.
There was no evidence that these effects varied between patients prescribed methadone and those prescribed buprenorphine (interaction p > 0.39).
Cessation and mortality
Only about 11% of the deaths available in WP 3 were valid for these analyses. This led to 75 ACM and 12 DRP deaths (Table 22).
Dose | Category | Deaths | PY | Unadjusted | Adjusteda | Adjusteda + OST type | ||||
---|---|---|---|---|---|---|---|---|---|---|
MR | HR (95% CI) | p-value | HR (95% CI) | p-value | HR (95% CI) | p-value | ||||
ACM | ||||||||||
Ending | Linearb | 1.06 (1.03 to 1.09) | 0.0001 | 1.04 (1.01 to 1.08) | 0.0158 | 1.03 (0.99 to 1.07) | 0.0926 | |||
Ending | Lowestc | 8 | 273 | 2.93 | 1 (reference) | 0.0005 | 1 (reference) | 0.0262 | 1 (reference) | 0.1041 |
24 | 196 | 12.26 | 4.49 (1.94 to 10.42) | 3.41 (1.41 to 8.26) | 2.94 (1.21 to 7.13) | |||||
16 | 141 | 11.38 | 4.91 (1.98 to 12.21) | 3.73 (1.44 to 9.65) | 2.79 (1.06 to 7.35) | |||||
Highest | 27 | 183 | 14.77 | 5.72 (2.46 to 13.30) | 3.32 (1.35 to 8.17) | 2.43 (0.97 to 6.10) | ||||
Change | Linearb | 1.00 (0.95 to 1.05) | 0.9607 | 0.99 (0.93 to 1.05) | 0.7257 | 0.99 (0.94 to 1.05) | 0.7338 | |||
Change | No decrease | 61 | 552 | 11.06 | 1.34 (0.71 to 2.53) | 0.3674 | 1.35 (0.69 to 2.66) | 0.3824 | 1.47 (0.73 to 2.95)d | 0.2790 |
Any decrease | 14 | 240 | 5.83 | 1 (reference) | 1 (reference) | 1 (reference) | ||||
Drug-related mortality | ||||||||||
Ending | Linearb | 1.06 (0.97 to 1.16) | 0.1833 | 1.08 (0.98 to 1.19) | 0.1227 | 1.17 (1.02 to 1.33) | 0.0209 | |||
Ending | Lowestc | 3 | 166 | 1.81 | 1 (reference) | 0.8374 | 1 (reference) | 0.8032 | 1 (reference) | 0.2426 |
3 | 118 | 2.53 | 1.40 (0.27 to 7.33) | 1.57 (0.20 to 12.14) | 1.41 (0.16 to 12.28) | |||||
3 | 84 | 3.57 | 2.05 (0.38 to 11.04) | 1.80 (0.32 to 10.21) | 4.24 (0.63 to 28.66) | |||||
Highest | 3 | 87 | 3.43 | 1.86 (0.36 to 9.68) | 2.42 (0.39 to 14.94) | 11.59 (0.93 to 144) | ||||
Change | Linearb | 1.00 (0.86 to 1.16) | 0.9881 | 1.01 (0.85 to 1.21) | 0.8935 | 1.03 (0.84 to 1.25) | 0.8044 | |||
Change | No decrease | 7 | 310 | 2.26 | 0.72 (0.22 to 2.40) | 0.5932 | 0.69 (0.19 to 2.55) | 0.5802 | 0.88 (0.22 to 3.52) | 0.8555 |
Any decrease | 5 | 146 | 3.43 | 1 (reference) | 1 (reference) | 1 (reference) |
For ACM, higher ending doses were associated with increased risk. Similar to initiation, adjustment attenuated linear effect sizes by about 50%. With full adjustment, the results were perhaps underpowered, but suggested a 3% increase in mortality risk associated with every 5-mg increase in ending dose of methadone (1-mg increase for buprenorphine) (HR 1.03, 95% CI 0.99 to 1.07). As for initiation, the biggest change in risk was to exceed the low dose. For DRP, adjustment amplified effect sizes, almost trebling the linear effect size. Here, there was a 17% increase in risk associated with every 5-mg methadone (1-mg buprenorphine) increase in ending dose (HR 1.17, 95% CI 1.02 to 1.33). Unlike ACM, the biggest change in risk may be associated with exceeding medium doses.
There was no evidence that changing dose over the last 28 days had any effect on mortality risks.
There was some evidence that the change in dose effect varied with OST type for ACM (interaction p = 0.022). Whereas no decrease in dose appeared to be associated with an increase risk by 100% for methadone (p = 0.088), there was a 75% reduction in risk for buprenorphine (p = 0.091) compared with any decrease.
Trends in adherence for initiation and cessation
Prior to the guidelines in 2007,7 there was declining adherence or no change in adherence (Table 23). In particular, starting and ending doses and change in dose for methadone during cessation showed evidence of declining adherence. After the guidelines, this decline was generally halted for initiation starting doses and may be improving for most cessation characteristics.
Year | Initiation [Total number of episodes (% adherence)] | Cessation [Total number of episodes (% adherence)] | ||||||
---|---|---|---|---|---|---|---|---|
Starting dose | Increase in dose | Ending dose | Decrease in dose | |||||
Methadone | Buprenorphine | Methadone | Buprenorphine | Methadone | Buprenorphine | Methadone | Buprenorphine | |
2001 | 1235 (25.51) | 318 (60.38) | 1235 (68.02) | 318 (36.16) | 463 (32.18) | 69 (66.67) | 463 (37.80) | 69 (30.43) |
2002 | 1291 (24.09) | 497 (46.68) | 1291 (67.70) | 497 (35.01) | 500 (29.40) | 120 (56.67) | 500 (33.60) | 120 (30.00) |
2003 | 1389 (21.74) | 637 (44.90) | 1389 (69.26) | 637 (41.44) | 509 (25.93) | 180 (54.44) | 509 (28.09) | 180 (30.56) |
2004 | 1271 (21.32) | 718 (38.86) | 1271 (67.19) | 718 (37.19) | 653 (27.72) | 215 (55.35) | 653 (28.02) | 215 (29.77) |
2005 | 1152 (22.14) | 737 (41.79) | 1152 (67.97) | 737 (37.04) | 564 (25.35) | 242 (47.93) | 564 (26.06) | 242 (28.93) |
2006 | 1209 (19.35) | 718 (39.69) | 1209 (68.73) | 718 (39.00) | 588 (25.17) | 233 (43.78) | 588 (27.21) | 233 (33.91) |
2007 | 1175 (20.68) | 695 (44.03) | 1175 (68.85) | 695 (40.14) | 533 (25.52) | 243 (44.86) | 533 (25.70) | 243 (33.74) |
2008 | 1200 (20.42) | 648 (43.06) | 1200 (68.58) | 648 (36.88) | 548 (24.27) | 237 (43.88) | 548 (25.91) | 237 (29.96) |
2009 | 974 (16.94) | 625 (51.04) | 974 (68.89) | 625 (37.44) | 542 (25.46) | 202 (48.02) | 542 (29.34) | 202 (24.75) |
2010 | 1024 (17.58) | 552 (50.00) | 1024 (71.09) | 552 (41.85) | 464 (27.37) | 199 (42.21) | 464 (30.60) | 199 (26.63) |
2011 | 797 (15.68) | 496 (52.62) | 797 (64.62) | 496 (40.12) | 519 (25.43) | 182 (51.65) | 519 (31.79) | 182 (29.67) |
2012 | 717 (18.27) | 517 (48.16) | 717 (66.11) | 517 (40.81) | 491 (27.90) | 222 (54.95) | 491 (30.14) | 222 (35.59) |
2013 | 624 (17.31) | 432 (39.81) | 624 (65.87) | 432 (35.65) | 393 (24.68) | 199 (53.27) | 393 (27.23) | 199 (28.64) |
2014 | 299 (23.41) | 236 (44.49) | 299 (66.56) | 236 (37.71) | 229 (25.33) | 96 (52.08) | 229 (26.20) | 96 (30.21) |
Trends | ||||||||
2001–6 | –0.061, 0.0002 | –0.121, < 0.0001 | 0.003, 0.8416 | 0.015, 0.4874 | –0.063, 0.0068 | –0.156, 0.0002 | –0.100, < 0.0001 | 0.025, 0.5648 |
2007–14 | –0.021, 0.1576 | 0.002, 0.8808 | –0.025, 0.0423 | –0.004, 0.7914 | 0.007, 0.6688 | 0.066, 0.0041 | 0.019, 0.2671 | 0.000, 0.9946 |
Equality | 0.0693 | < 0.0001 | 0.1443 | 0.4692 | 0.0152 | < 0.0001 | < 0.0001 | 0.6185 |
Summary
Evidence that OST initiation and cessation followed current guidelines has historically been poor. Although there was evidence that adherence is improving, evidence of planned titration and discharge remains low. There was considerable variability in initiation and cessation characteristics. This may reflect errors in recorded or imputed daily doses, missing prescription data misclassifying one episode as two episodes or, for initiation, accelerated detoxification.
The trend towards lower starting and ending doses being associated with lower risk of mortality was found for ACM. For DRP, the same trends were observed but the evidence was weaker as result of the lack of power associated with few deaths. This is consistent with other studies reporting the lowest incidence of mortality among those starting on < 30mg/day methadone and those completing detoxification. 68,69
We found weak evidence for a beneficial association on mortality risk for an increasing dose during the first 28 days of treatment. Although our data did not exhibit large rapid increases in dose, one study has reported an increased risk of mortality associated with too-rapid increases in methadone dose. 68 There was no evidence that changes in dose during cessation were associated with mortality. This may reflect that a period of 28 days was too short to capture optimal changes in dose.
Although it was interesting that effect modification for change in dose with OST type for cessation appeared to be present for ACM, the interaction was difficult to interpret and was probably a chance event among the multiple comparisons being tested.
Chapter 8 Development of self-controlled case series methods for opiate substitution treatment data
In this project we have attempted to use a number of methods to obtain more robust results. In this chapter, on WP 5, we describe the modification of two SCCS methods48,50 and the results comparing these methods using simulations.
Aims and objectives
Self-controlled case series methods have been developed to study the adverse reactions to vaccinations. 48,50 In these scenarios, treatment episodes consist of a series of very short exposures (the injections) at regular intervals. This is in stark contrast to therapeutic data, where exposure times are variable and can last for months and where the sequence of episodes is not predetermined and will vary both in frequency and number.
In the context of OST data, there are two main problems. First, having a variable treatment period leads to an open-ended risk period following the first 4 weeks of treatment. This period would need to be estimated if death occurs during treatment. The equivalent open-ended period after treatment had ceased for more than 4 weeks was not originally considered to raise major issues. Second, there were two main types of treatment involving methadone or buprenorphine with their effects on mortality varying with the treatment period.
The aims of this WP were to extend existing methods to cater for these additional characteristics of OST data. We used simulations to verify that the modifications were robust and finally to apply the modified methods to the real data relating to WP 2 to investigate the OST type × period interaction.
The implications of these facets of the OST data are discussed in the next section as we describe some of features of the SCCS methods. Other characteristics of the OST data and their implications to SCCS analyses are described in the report under submission.
Implications of the existing self-controlled case series methods for opiate substitution treatment data
The two methods are referred to as the Farrington48 and Kuhnert50 methods, both of which are relevant to censored data as arise in the study of mortality. Both methods analyse only cases using fixed-effects Poisson regression clustering on patients. Using this technique has advantages in that the fixed effects can adjust for all non-time-varying factors, whether or not observed, associated with the patient. Both methods attempt to reconstruct the last episode, when death occurred, as if death had not occurred. In the context of vaccination data, this is straightforward, as typically there is a single risk period for each episode of fixed duration. Although the subsequent control period is technically open-ended, this is catered for differently by the two methods. For the Kuhnert method, there is usually a known earliest date for the next vaccination, whereas for the Farrington method there is usually a known date when follow-up was planned to cease. These dates are used to set the end of the control period.
Differences between the two methods reflect the exclusion of cases and the derivation of pseudo-individuals. For the Kuhnert method, patients who died after the earliest date for the next vaccination are excluded but all included patients appear only once. By contrast, for the Farrington method, all cases are included but copies of each patient’s data are generated to reflect unobserved scenarios in which each observed treatment episode was the only episode to occur.
For the OST data, the open-ended treatment period was imputed using treatment duration data for patients who survived. Imputations were based on matched patients receiving the same medication at a similar time and of a similar age to those patients who died. For the open-ended period after treatment ceased, a date 1 year after the last treatment ended was used for the Farrington method. For the Kuhnert method, although 28 days was the observed minimum interval between treatment episodes, this interval was too short to allow estimation of the open-ended period. Hence, a range of intervals from 35 to 56 days was tried.
The presence of two medications had consequences for estimating the type × period interaction. Because only one episode and hence one medication was used for all patients in the Kuhnert method and all pseudo-individuals in the Farrington method, 1 df was aliased between the interaction and the clustered fixed effect. To overcome this, the open-ended off-treatment period was assumed to be equal for methadone and buprenorphine.
Data set used in simulations
Data relating to 11,033 patients as used in WP 2 were also used in this WP, but instead of using the observed deaths, simulated deaths were generated using the observed risks for covariates obtained from WP 2 using Poisson regression. In all, 1000 simulated data sets were generated, producing results for both methods under different scenarios.
Key findings from the submitted paper
Simulations suggested that the Farrington method, assuming a projected end to follow-up of 150 days after the last treatment ended, produced estimated effects closest to the true values. For the Kuhnert method, the shortest interval performed best, although the differences between 35 and 56 days were small. This characteristic was important, as there may be insufficient numbers of death for shorter intervals to allow the interactions to be modelled.
Using these modified methods with the observed data allowed comparisons with an alternative robust method, IPW, as reported in WP 2. 61 For ACM, these methods also showed evidence of a OST type × period interaction, although stronger evidence came from the Farrington method, probably because of the greater number of deaths that were valid for this method. There was perhaps some deviation from results reported in WP 2 in that differences between methadone and buprenorphine were smaller and statistically equivalent for the period following the first 4 weeks of treatment for the Farrington method (interaction IRR 1.04, 95% CI 0.55 to 1.94) and for the Kuhnert method (interaction IRR 2.34, 95% CI 0.56 to 9.84). At other times, as reported in WP 2, buprenorphine was protective compared with methadone. For DRP, no evidence of an interaction was found with either method, in contrast to results from IPW analyses. However, SEs were large in these analyses, making the detection of any potential interaction effects difficult.
Summary
Simulations have suggested that modifications to the SCCS methods have produced robust results. As a consequence, these methods can be applied not only to vaccination data but also to therapeutic prescription-based data. This may be particularly helpful for databases that contain limited additional information on patients’ histories, severity of symptoms and treatment quality. However, robust results were achieved only with a relatively short intertreatment gap for the Farrington method and a very short gap for the Kuhnert method, although, for the Kuhnert method, this was to be expected as this interval should reflect the minimum gap between treatment episodes. The use of such constraints inevitably reduced the number of analysed deaths. Applying the revised methods to the observed data on mortality and episodes of methadone and buprenorphine treatment reduced the number of deaths in the constrained sample by about 10% for the Farrington method and about 30% for the Kuhnert method compared with the original Poisson regressions reported in Chapter 5. The results suggested an OST type × period interaction for ACM but were too underpowered to evaluate DRP.
Chapter 9 Conclusions
This chapter considers the implications for clinical practice arising from the results of this study, a review of the potential limitations of this study and recommendations for future work.
Patient and public involvement
Our patient and public involvement (PPI) work demonstrates why we need to be very cautious in drawing conclusions on which form of OST is safer and more likely to reduce mortality risk in the population (see Appendix 3 for more details). Drug workers and people who use opioids highlighted that there may be multiple reasons why people who choose or are prescribed buprenorphine are different from people who choose or are prescribed methadone, in terms of stability, mood and use of heroin and other drugs during treatment. For this reason, our PPI group were not surprised that fewer people died in the first 4 weeks of buprenorphine treatment than methadone treatment – even after adjustment for multiple confounders (differences in patient and practice characteristics between patients on methadone and those on buprenorphine). There was some, but not widespread, support for a trial that seeks to induct all patients onto buprenorphine where patients did not express a strong preference for methadone. However, others raised concerns over the probable need for additional psychological support and the potentially unintended consequences of such a trial (in terms of patients withdrawing from treatment or moving services). The PPI group confirmed that there is no clear and quick fix to reduce drug-related deaths in the population through changing the way in which OST is delivered. More development is needed to establish an acceptable intervention and trial of OST delivery (see Chapter 9, Future research).
Drug-related poisoning and all-cause mortality rates
The average annual ACM and DRP in our study, at 1.9% and 0.8%, respectively, were slightly higher than in some earlier studies of mortality in cohorts obtained from community drug treatment agencies (0.34 per 100 person-years based on the NDTMS30,44), although our findings were consistent with recent systematic review evidence. 64 It is likely that the NDTMS population is a mix of opioid users who may not all be receiving OST, in contrast to the CPRD population, which comprises people with opioid disorders in OST. There may also be differences in morbidity between CPRD and NDTMS populations, but we lack consistent data between the two systems to allow us to compare morbidity (see Future research).
Clinical implications and recommendations
Our data suggest that there was an increased risk of mortality during the first 4 weeks of treatment, with lower risks being associated with buprenorphine treatment. Although this may suggest advantages in prescribing buprenorphine during induction and switching to methadone later if necessary, our investigations into such strategies showed only a low probability of reducing DRP.
The increased risk of ACM mortality following cessation of treatment may indicate poor retention in treatment or the need for greater patient support when the impact of reduced opioid tolerance is most acute. The lower risk for buprenorphine during this period may suggest benefits in switching to buprenorphine during the final stages of treatment.
We identified particular groups of patients in our data, namely those who were older and had more comorbidity, who appeared to particularly benefit from buprenorphine treatment. This was a novel result and not specified a priori. Further work is needed to confirm these interactions.
As in other studies, our study also suggested shorter treatment duration for buprenorphine than methadone treatment, but the intervals between treatments also differed, with buprenorphine having a shorter off-treatment duration. Overall, current estimates indicate that buprenorphine patients have a lower percentage time on treatment. There is a clear public health need to retain people on OST longer to reduce the number of deaths in the population.
Our findings do not advocate prescribing benzodiazepines or z-drugs to OST patients. Gabapentinoids may also be detrimental, but further replication is needed. Although trends differed by medication, with benzodiazepine decreasing and gabapentinoids increasing in prevalence during the study period, overall, for each of these three medications, data since 2002 have suggested that the prevalence of affected patients remained constant. Our data would suggest that further decreases in co-prescribing would be beneficial.
Our analyses were completed before new clinical guidance on drug treatment was issued. 43 This guidance suggested caution in prescribing OST to patients if there is associated alcohol or benzodiazepine dependence, or use of other depressant drugs such as pregabalin or gabapentin or some major tranquillisers. Our findings have shown similar problems in prescribing benzodiazepines, z-drugs or gabapentinoids to patients with an ongoing opioid disorder irrespective of whether they are on or off OST.
Unfortunately, other critical aspects of OST delivery in CPRD data could not be measured, such as supervised consumption, availability and intensity of adjunct psychosocial support, and frequency of care-plan assessments during OST. Further research and alternative methodological approaches are required to measure the intensity and quality of OST on retention and mortality outcomes. It was found, however, that very few OST episodes seem to correspond to maintenance therapy, as such a large proportion of OST episodes lasted < 3 months. Furthermore, only a minority of OST episodes lasting < 3 months had any evidence of tapering of dose indicative of planned discharge and detoxification. The guidance suggests that ‘duration of maintenance should reflect the patient’s own preferences and their clinical circumstances (which may include the opportunities available to them to support their recovery and management of risk)’ (contains public sector information licensed under the Open Government Licence v3.0). 43 It has been shown that, in the majority of episodes, OST is ceased prematurely and that there is a need to provide additional interventions to retain people on OST to achieve population benefit of reducing drug-related deaths. Short OST episodes are not unique to primary care, as we show when comparing average treatment duration between CPRD and a major non-governmental organisation.
Limitations of the study design, data sources and analytic methods
The key limitation of this project was the potential for residual confounding through either imperfectly measured observed confounders or omitted/unobserved confounders. Several analytic approaches have been presented to consider the extent of confounding, to aid interpretation of the analyses and to reduce the bias from such effects. These analyses, such as IPW, tended to strengthen confidence in the results.
There were likely to have been missing prescriptions in the CPRD data. For instance, some patients may have been treated in specialist drug treatment clinics as well as in primary care. Treatment during periods in prison are also unlikely to be recorded in primary care. This would lead to not only misclassification of periods on and off treatment but also possibly misclassification between the first 4 weeks and the remainder of time on treatment if the missing data had erroneously led to one episode being considered as two. To explore this further, sensitivity analyses were performed using different criteria for the derivation of episodes, but the impact on the OST type × period interaction was small. These misclassification of treatment periods would have also biased mean on- and off-treatment durations. However, it was expected that median durations would have been less susceptible to any bias.
Periods in prison may also introduce bias and confer additional risks; for example, the period in prison will be one of lower mortality risk but the period immediately following prison release is of high risk. 70 However, prison history was incorporated into the propensity scores as a method of reducing this bias.
The small number of DRP deaths among a high-risk group such as OST patients may indicate that these deaths were under-reported. Although this allowed some conclusions to be drawn relating to the OST type × period interaction and other interactions with age and comorbidity, other analyses on initiation and cessation were severely hampered.
Critically, it was not possible to characterise the intensity and quality of OST, and clinical data on addiction severity or drug use patterns over the 16 years of this study. However, these data are also absent from all other large-scale drug treatment cohorts. For example, treatment cohorts in New South Wales have fewer confounders than CPRD and adjusted only for age, gender and treatment history; and NDTMS lacks data on morbidity and detailed information on type of OST. 30,53 There is a clear tension between well-characterised cohorts or trials of OST and power to detect changes in mortality risk, and it seems unlikely in the immediate future that refined clinical information can be obtained on large numbers of patients. An alternative approach, undertaken in the VEdeTTE study,17 is to conduct nested case–control studies within the cohort, and to collect more detailed clinical information on a smaller number of cases (DRP) and controls (three or four people with an opioid disorder who at the time of the case were known to be alive) (unpublished document: Professor Fabrizio Faggiano, University of Eastern Piedmont, and Dr Marina Davoli, Lazio Regional Health Service Rome 2018).
Since 2007 there have been changes to drug treatment and policy. 7,36,71 These include a greater emphasis on recovery and re-engagement with the community through employment, take-home naloxone to counteract overdose and a greater diversity of treatment agencies. These factors potentially may confound our results, particularly the longitudinal trends.
Future research
The results from this project suggest four main areas of future research.
First, replication and strengthening power: the analyses on DRP were limited because only just above half of the patient episodes were linked to Office for National Statistics data on cause of death. Several of the analyses involving SCCS methods and for initiation and cessation were underpowered and may provide clearer evidence if conducted on a larger data set. In addition, novel interactions between age and OST modality and between comorbidity and OST modality were identified that need to be tested in other data sets and studies.
Second, cross-cohort comparison: these results relate to UK primary care. There are benefits (in terms of both replication and identifying potential differences in OST delivery that may increase or decrease mortality risk) of comparing the CPRD findings with those for cohorts from community drug agencies in the UK and internationally. This will test whether there are differences between mortality risk in patients managed in primary care and those managed by community drug agencies, as well as raise hypotheses on how OST might be better delivered in the UK if differences are found across international cohorts.
Third, intensity of treatment: additional studies are needed both to determine the extent to which OST is delivered as recommended in current guidelines and to assess whether or not intensity of OST, including adjunct interventions, protects against DRP during and immediately after treatment cessation compared with low-intensity/low-threshold OST.
Fourth, interventions: retention in OST is critical to reducing the number of DRP in the population, and more work with patient groups and clinicians is required on how to deploy buprenorphine and methadone and other strategies both to reduce mortality risk at the beginning of OST and to retain patients on OST for prolonged periods to minimise mortality risk long enough to generate public health benefit.
Acknowledgements
We are grateful for comments and contributions from patients and drug workers at Bristol Drug Project and Horizon Drug and Alcohol Service Blackpool, and in particular Rachel Ayres and Louisa Chowen at Bristol Drug Project for organising PPI.
Contributions of authors
Colin D Steer (Senior Research Fellow, Medical Statistics) contributed to the analysis plan, the development of SCCS methods, the drafting of the report, the interpretation of the results and the critical revision of the report; he analysed the CPRD data and performed the SCCS simulations.
John Macleod (Professor of Clinical Epidemiology and Primary Care) contributed to the study design, the drafting of the report, PPI, the interpretation of the results and the critical revision of the report.
Kate Tilling (Professor of Medical Statistics) contributed to the analysis plan, the interpretation of the results and the critical revision of the report.
Aaron G Lim (Senior Research Associate, Infectious Disease Mathematical Modelling) performed the simulations of different treatment regimens on mortality rates, and contributed to the interpretation of the results and the critical revision of the report.
John Marsden (Professor of Addiction Psychology) contributed to the interpretation of the results and the critical revision of the report.
Tim Millar (Professor of Substance Use and Addictions) analysed treatment duration from a special drug agency database for comparison with CPRD, and contributed to the interpretation of the results and the critical revision of the report.
John Strang (Head of Addictions Department, Professor of the Psychiatry of Addictions) contributed to the interpretation of the results and the critical revision of the report.
Maggie Telfer (Chief Executive Officer of Bristol Drugs Project) contributed to PPI.
Heather Whitaker (Senior Lecturer, Statistics and SCCS methods) contributed to the development of SCCS methods for the CPRD data, the interpretation of the results and the critical revision of the report.
Peter Vickerman (Professor of Infectious Disease Modelling) contributed to the simulations of different treatment regimens on mortality rates, the interpretation of the results and the critical revision of the report.
Matthew Hickman (Head of Population Health Sciences, Professor in Public Health and Epidemiology) contributed to study design, the drafting of the report, the analysis plan, PPI, the interpretation of the results and the critical revision of the report.
Publication
Hickman M, Steer C, Tilling K, Lim AG, Marsden J, Millar T, et al. The impact of buprenorphine and methadone on mortality: a primary care cohort study in the United Kingdom. Addiction 2018;113:1461–76.
Data-sharing statement
This study utilised CPRD data that cannot be disseminated further as a result of conditions attached to their initial release to the authors. These data can be requested directly from CPRD (www.cprd.com). All queries should be submitted to the corresponding author.
Patient data
This work uses data provided by patients and collected by the NHS as part of their care and support. Using patient data is vital to improve health and care for everyone. There is huge potential to make better use of information from people’s patient records, to understand more about disease, develop new treatments, monitor safety, and plan NHS services. Patient data should be kept safe and secure, to protect everyone’s privacy, and it’s important that there are safeguards to make sure that it is stored and used responsibly. Everyone should be able to find out about how patient data are used. #datasaveslives You can find out more about the background to this citation here: https://understandingpatientdata.org.uk/data-citation.
Disclaimers
This report presents independent research funded by the National Institute for Health Research (NIHR). The views and opinions expressed by authors in this publication are those of the authors and do not necessarily reflect those of the NHS, the NIHR, NETSCC, the HS&DR programme or the Department of Health and Social Care. If there are verbatim quotations included in this publication the views and opinions expressed by the interviewees are those of the interviewees and do not necessarily reflect those of the authors, those of the NHS, the NIHR, NETSCC, the HS&DR programme or the Department of Health and Social Care.
References
- Ward J, Hall W, Mattick RP. Role of maintenance treatment in opioid dependence. Lancet 1999;353:221-6. https://doi.org/10.1016/S0140-6736(98)05356-2.
- Gowing L, Farrell MF, Bornemann R, Sullivan LE, Ali R. Oral substitution treatment of injecting opioid users for prevention of HIV infection. Cochrane Database Syst Rev 2011;8. https://doi.org/10.1002/14651858.CD004145.pub4.
- Mattick RP, Breen C, Kimber J, Davoli M. Methadone maintenance therapy versus no opioid replacement therapy for opioid dependence. Cochrane Database Syst Rev 2009;3. https://doi.org/10.1002/14651858.CD002209.pub2.
- Turner KM, Hutchinson S, Vickerman P, Hope V, Craine N, Palmateer N, et al. The impact of needle and syringe provision and opiate substitution therapy on the incidence of hepatitis C virus in injecting drug users: pooling of UK evidence. Addiction 2011;106:1978-88. https://doi.org/10.1111/j.1360-0443.2011.03515.x.
- Amato L, Davoli M, Perucci CA, Ferri M, Faggiano F, Mattick RP. An overview of systematic reviews of the effectiveness of opiate maintenance therapies: available evidence to inform clinical practice and research. J Subst Abuse Treat 2005;28:321-9. https://doi.org/10.1016/j.jsat.2005.02.007.
- Robertson JR, Raab GM, Bruce M, McKenzie JS, Storkey HR, Salter A. Addressing the efficacy of dihydrocodeine versus methadone as an alternative maintenance treatment for opiate dependence: a randomized controlled trial. Addiction 2006;101:1752-9. https://doi.org/10.1111/j.1360-0443.2006.01603.x.
- Drug Misuse and Dependence: UK Guidelines on Clinical Management. London: Department of Health and Social Care; 2007.
- Methadone and Buprenorphine for the Management of Opioid Dependence. London: National Institute for Health and Care Excellence; 2007.
- National Treatment Agency for Substance Misuse . Statistics from the National Drug Treatment Monitoring System 1 April 2011–31 March 2012 2012. www.nta.nhs.uk/uploads/statisticsfromndtms201112vol1thenumbersfinal.pdf (accessed July 2017).
- Hay G, Gannon M, MacDougall J, Eastwood C, Williams K, Millar T. Capture – recapture and anchored prevalence estimation of injecting drug users in England: national and regional estimates. Stat Methods Med Res 2009;18:323-39. https://doi.org/10.1177/0962280208094687.
- Hay G, Rael dos Santos A, Worsley J. Estimates of the Prevalence of Opiate Use and/or Crack Cocaine Use, 2011/12. Liverpool: John Moores University; 2014.
- De Angelis D, Hickman M, Yang S. Estimating long-term trends in the incidence and prevalence of opiate use/injecting drug use and the number of former users: back-calculation methods and opiate overdose deaths. Am J Epidemiol 2004;160:994-1004. https://doi.org/10.1093/aje/kwh306.
- Godfrey C, Stewart D, Gossop M. Economic analysis of costs and consequences of the treatment of drug misuse: 2-year outcome data from the National Treatment Outcome Research Study (NTORS). Addiction 2004;99:697-70. https://doi.org/10.1111/j.1360-0443.2004.00752.x.
- Caplehorn JR, Dalton MS, Haldar F, Petrenas AM, Nisbet JG. Methadone maintenance and addicts’ risk of fatal heroin overdose. Subst Use Misuse 1996;31:177-96. https://doi.org/10.3109/10826089609045806.
- Cornish R, Macleod J, Strang J, Vickerman P, Hickman M. Risk of death during and after opiate substitution treatment in primary care: prospective observational study in UK General Practice Research Database. BMJ 2010;341. https://doi.org/10.1136/bmj.c5475.
- Degenhardt L, Randall D, Hall W, Law M, Butler T, Burns L. Mortality among clients of a state-wide opioid pharmacotherapy program over 20 years: risk factors and lives saved. Drug Alcohol Depend 2009;105:9-15. https://doi.org/10.1016/j.drugalcdep.2009.05.021.
- Davoli M, Bargagli AM, Perucci CA, Schifano P, Belleudi V, Hickman M, et al. Risk of fatal overdose during and after specialist drug treatment: the VEdeTTE study, a national multi-site prospective cohort study. Addiction 2007;102:1954-9. https://doi.org/10.1111/j.1360-0443.2007.02025.x.
- Degenhardt L, Bucello C, Mathers B, Briegleb C, Ali H, Hickman M, et al. Mortality among regular or dependent users of heroin and other opioids: a systematic review and meta-analysis of cohort studies. Addiction 2011;106:32-51. https://doi.org/10.1111/j.1360-0443.2010.03140.x.
- White JM, Irvine RJ. Mechanisms of fatal opioid overdose. Addiction 1999;94:961-72. https://doi.org/10.1046/j.1360-0443.1999.9479612.x.
- Mattick RP, Kimber J, Breen C, Davoli M. Buprenorphine maintenance versus placebo or methadone maintenance for opioid dependence. Cochrane Database Syst Rev 2008;2. https://doi.org/10.1002/14651858.CD002207.pub3.
- Bell J, Trinh L, Butler B, Randall D, Rubin G. Comparing retention in treatment and mortality in people after initial entry to methadone and buprenorphine treatment. Addiction 2009;104:1193-200. https://doi.org/10.1111/j.1360-0443.2009.02627.x.
- Burns L, Gisev N, Larney S, Dobbins T, Gibson A, Kimber J, et al. A longitudinal comparison of retention in buprenorphine and methadone treatment for opioid dependence in New South Wales, Australia. Addiction 2015;110:646-55. https://doi.org/10.1111/add.12834.
- Hser YI, Saxon AJ, Huang D, Hasson A, Thomas C, Hillhouse M, et al. Treatment retention among patients randomized to buprenorphine/naloxone compared to methadone in a multi-site trial. Addiction 2014;109:79-87. https://doi.org/10.1111/add.12333.
- Auriacombe M, Fatséas M, Dubernet J, Daulouède JP, Tignol J. French field experience with buprenorphine. Am J Addict 2004;13:17-28. https://doi.org/10.1080/10550490490440780.
- Buster MC, van Brussel GH, van den Brink W. An increase in overdose mortality during the first 2 weeks after entering or re-entering methadone treatment in Amsterdam. Addiction 2002;97:993-1001. https://doi.org/10.1046/j.1360-0443.2002.00179.x.
- McCowan C, Kidd B, Fahey T. Factors associated with mortality in Scottish patients receiving methadone in primary care: retrospective cohort study. BMJ 2009;338. https://doi.org/10.1136/bmj.b2225.
- Strang J, McCambridge J, Best D, Beswick T, Bearn J, Rees S, et al. Loss of tolerance and overdose mortality after inpatient opiate detoxification: follow up study. BMJ 2003;326:959-60. https://doi.org/10.1136/bmj.326.7396.959.
- Jones JD, Mogali S, Comer SD. Polydrug abuse: a review of opioid and benzodiazepine combination use. Drug Alcohol Depend 2012;125:8-18. https://doi.org/10.1016/j.drugalcdep.2012.07.004.
- Leece P, Cavacuiti C, Macdonald EM, Gomes T, Kahan M, Srivastava A, et al. Predictors of opioid-related death during methadone therapy. J Subst Abuse Treat 2015;57:30-5. https://doi.org/10.1016/j.jsat.2015.04.008.
- Pierce M, Bird SM, Hickman M, Marsden J, Dunn G, Jones A, et al. Impact of treatment for opioid dependence on fatal drug-related poisoning: a national cohort study in England. Addiction 2016;111:298-30. https://doi.org/10.1111/add.13193.
- Abrahamsson T, Berge J, Öjehagen A, Håkansson A. Benzodiazepine, z-drug and pregabalin prescriptions and mortality among patients in opioid maintenance treatment – a nation-wide register-based open cohort study. Drug Alcohol Depend 2017;174:58-64. https://doi.org/10.1016/j.drugalcdep.2017.01.013.
- Darke S, Deady M, Duflou J. Toxicology and characteristics of deaths involving zolpidem in New South Wales, Australia 2001–2010. J Forensic Sci 2012;57:1259-62. https://doi.org/10.1111/j.1556-4029.2012.02117.x.
- Chiappini S, Schifano F. A decade of gabapentinoid misuse: an analysis of the European Medicines Agency’s ‘Suspected Adverse Drug Reactions’ database. CNS Drugs 2016;30:647-54. https://doi.org/10.1007/s40263-016-0359-y.
- Lingford-Hughes AR, Welch S, Peters L, Nutt DJ. Expert Reviewers Group . BAP updated guidelines: evidence-based guidelines for the pharmacological management of substance abuse, harmful use, addiction and comorbidity: recommendations from BAP. J Psychopharmacol 2012;26:899-952. https://doi.org/10.1177/0269881112444324.
- Schuckit MA. Treatment of opioid-use disorders. N Engl J Med 2016;375:357-68. https://doi.org/10.1056/NEJMra1604339.
- Guidance for the Use of Substitute Prescribing in the Treatment of Opioid Dependence in Primary Care. London: Royal College of General Practitioners; 2011.
- Morgan O, Griffiths C, Hickman M. Association between availability of heroin and methadone and fatal poisoning in England and Wales 1993–2004. Int J Epidemiol 2006;35:1579-85. https://doi.org/10.1093/ije/dyl207.
- Strang J, Hall W, Hickman M, Bird SM. The impact of supervised methadone consumption on opiate overdose deaths in England and Scotland: analysis using the OD4 Index. BMJ 2010;341. https://doi.org/10.1136/bmj.c4851.
- Morgan O, Vicente J, Griffiths P, Hickman M. Trends in overdose deaths from drug misuse in Europe: what do the data tell us?. Addiction 2008;103:699-700. https://doi.org/10.1111/j.1360-0443.2007.02102.x.
- Hickman M, Vickerman P, Robertson R, Macleod J, Strang J. Promoting recovery and preventing drug-related mortality: competing risks?. J Public Health 2011;33:332-4. https://doi.org/10.1093/pubmed/fdr055.
- National Treatment Agency for Substance Misuse . Medications in Recovery: Re-Orientating Drug Dependence Treatment 2012.
- Drug Strategy 2017. London: HMSO; 2017.
- Drug Misuse and Dependence: UK Guidelines on Clinical Management. London: Department of Health and Social Care; 2017.
- Pierce M, Bird SM, Hickman M, Millar T. National record linkage study of mortality for a large cohort of opioid users ascertained by drug treatment or criminal justice sources in England, 2005–2009. Drug Alcohol Depend 2015;146:17-23. https://doi.org/10.1016/j.drugalcdep.2014.09.782.
- Using Natural Experiments to Evaluate Population Health Interventions: Guidance for Producers and Users of Evidence. London: Medical Research Council; 2011.
- D’Agostino RB . Propensity score methods for bias reduction in the comparison of a treatment to a non-randomized control group. Stat Med 1998;17:2265-81. https://doi.org/10.1002/(SICI)1097-0258(19981015)17:19<2265::AID-SIM918>3.0.CO;2-B.
- Williamson E, Morley R, Lucas A, Carpenter J. Propensity scores: from naive enthusiasm to intuitive understanding. Stat Methods Med Res 2012;21:273-93. https://doi.org/10.1177/0962280210394483.
- Farrington CP, Whitaker HJ, Hocine MN. Case series analysis for censored, perturbed, or curtailed post-event exposures. Biostatistics 2009;10:3-16. https://doi.org/10.1093/biostatistics/kxn013.
- Whitaker HJ, Farrington CP, Spiessens B, Musonda P. Tutorial in biostatistics: the self-controlled case series method. Stat Med 2006;25:1768-97. https://doi.org/10.1002/sim.2302.
- Kuhnert R, Hecker H, Poethko-Müller C, Schlaud M, Vennemann M, Whitaker HJ, et al. A modified self-controlled case series method to examine association between multidose vaccinations and death. Stat Med 2011;30:666-77. https://doi.org/10.1002/sim.4120.
- Clinical Practice Research Datalink . Research Services 2017. www.cprd.com/researcher/ (accessed 31 July 2017).
- Herrett E, Gallagher AM, Bhaskaran K, Forbes H, Mathur R, van Staa T, et al. Data Resource Profile: Clinical Practice Research Datalink (CPRD). Int J Epidemiol 2015;44:827-36. https://doi.org/10.1093/ije/dyv098.
- Kimber J, Larney S, Hickman M, Randall D, Degenhardt L. Mortality risk of opioid substitution therapy with methadone versus buprenorphine: a retrospective cohort study. Lancet Psychiatry 2015;2:901-8. https://doi.org/10.1016/S2215-0366(15)00366-1.
- Statistical Bulletin: Deaths Related to Drug Poisoning in England and Wales, 2014 Registrations. Newport: Office for National Statistics; 2015.
- Cousins G, Teljeur C, Motterlini N, McCowan C, Dimitrov BD, Fahey T. Risk of drug-related mortality during periods of transition in methadone maintenance treatment: a cohort study. J Subst Abuse Treat 2011;41:252-60. https://doi.org/10.1016/j.jsat.2011.05.001.
- Khan NF, Perera R, Harper S, Rose PW. Adaptation and validation of the Charlson Index for Read/OXMIS coded databases. BMC Fam Pract 2010;11. https://doi.org/10.1186/1471-2296-11-1.
- Martens EP, Pestman WR, de Boer A, Belitser SV, Klungel OH. Instrumental variables: application and limitations. Epidemiology 2006;17:260-7. https://doi.org/10.1097/01.ede.0000215160.88317.cb.
- Thomas KH, Martin RM, Davies NM, Metcalfe C, Windmeijer F, Gunnell D. Smoking cessation treatment and risk of depression, suicide, and self harm in the Clinical Practice Research Datalink: prospective cohort study. BMJ 2013;347. https://doi.org/10.1136/bmj.f5704.
- Brookhart MA, Wang PS, Solomon DH, Schneeweiss S. Evaluating short-term drug effects using a physician-specific prescribing preference as an instrumental variable. Epidemiology 2006;17:268-75. https://doi.org/10.1097/01.ede.0000193606.58671.c5.
- Brown H, Prescott R. Applied Mixed Models in Medicine. Chichester: John Wiley & Sons, Ltd; 2015.
- Hickman M, Steer C, Tilling K, Lim AG, Marsden J, Millar T, et al. The impact of buprenorphine and methadone on mortality: a primary care cohort study in the United Kingdom. Addiction 2018;113:1461-76. https://doi.org/10.1111/add.14188.
- Benchimol EI, Smeeth L, Guttmann A, Harron K, Moher D, Petersen I, et al. The REporting of studies Conducted using Observational Routinely-collected health Data (RECORD) statement. PLOS Med 2015;12. https://doi.org/10.1371/journal.pmed.1001885.
- Connock M, Juarez-Garcia A, Jowett S, Frew E, Liu Z, Taylor RJ, et al. Methadone and buprenorphine for the management of opioid dependence: a systematic review and economic evaluation. Health Technol Assess 2007;11. https://doi.org/10.3310/hta11090.
- Sordo L, Barrio G, Bravo MJ, Indave BI, Degenhardt L, Wiessing L, et al. Mortality risk during and after opioid substitution treatment: systematic review and meta-analysis of cohort studies. BMJ 2017;357. https://doi.org/10.1136/bmj.j1550.
- White M, Burton R, Darke S, Eastwood B, Knight J, Millar T, et al. Fatal opioid poisoning: a counterfactual model to estimate the preventive effect of treatment for opioid use disorder in England. Addiction 2015;110:1321-9. https://doi.org/10.1111/add.12971.
- Fugelstad A, Stenbacka M, Leifman A, Nylander M, Thiblin I. Methadone maintenance treatment: the balance between life-saving treatment and fatal poisonings. Addiction 2007;102:406-12. https://doi.org/10.1111/j.1360-0443.2006.01714.x.
- Bakker A, Streel E. Benzodiazepine maintenance in opiate substitution treatment: Good or bad? A retrospective primary care case-note review. J Psychopharmacol 2017;31:62-6. https://doi.org/10.1177/0269881116675508.
- Pilgrim JL, McDonough M, Drummer OH. A review of methadone deaths between 2001 and 2005 in Victoria, Australia. Forensic Sci Int 2013;226:216-22. https://doi.org/10.1016/j.forsciint.2013.01.028.
- Stimmel B, Goldberg J, Cohen M, Rotkopf E. Detoxification from methadone maintenance: risk factors associated with relapse to narcotic use. Ann N Y Acad Sci 1978;311:173-80. https://doi.org/10.1111/j.1749-6632.1978.tb16774.x.
- Farrell M, Marsden J. Acute risk of drug-related death among newly released prisoners in England and Wales. Addiction 2008;103:251-5. https://doi.org/10.1111/j.1360-0443.2007.02081.x.
- UK Drug Policy Commission . A Fresh Approach to Drugs 2012. www.ukdpc.org.uk/wp-content/uploads/a-fresh-approach-to-drugs-the-final-report-of-the-uk-drug-policy-commission.pdf (accessed 26 April 2018).
Appendix 1 Definition of drug-related deaths
Description | ICD-9 codes | ICD-10 codes |
---|---|---|
Mental and behavioural disorders | ||
Attributable to drug use (excluding alcohol and tobacco)a | 292, 304, 305.2–305.9 | F11–F16, F18–F19 |
Unspecified cause/disorder | F99 | |
Accidental self-harm | ||
Poisoning by drugs, medicaments and biological substancesa | E850–E858 | X40–X44 |
Poisoning, other or unspecified exposure | E866.8, E866.9 | X49 |
Other or unspecified means | E928.8, E928.9 | X58, X59.9 |
Intentional self-harm | ||
Poisoning by drugs, medicaments and biological substancesa | E950.0–E950.5 | X60–X64 |
Poisoning, other or unspecified exposure | E950.9 | X69 |
Other or unspecified means | E958.8, E958.9 | X83, X84 |
Assault by | ||
Poisoning by drugs, medicaments and biological substancesa | E962.0 | X85 |
Poisoning, other or unspecified exposure | E962.9 | X90 |
Other or unspecified means | E968.8, E968.9 | Y08, Y09 |
Self-harm, undetermined intent | ||
Poisoning by drugs, medicaments and biological substancesa | E980.0–E980.5 | Y10–Y14 |
Poisoning, other or unspecified exposure | E980.9 | Y19 |
Other or unspecified means | E988.8, E988.9 | Y33, Y34 |
External cause | ||
Poisoning by drugs, medicaments and biological substances | 960–979 | T36-T50 |
Poisoning, other or unspecified exposure | 989.89, 989.9 | T65.8, T65.9 |
Other or unspecified cause | 995.89 | T78.8, T78.9 |
Ill-defined, unspecified or unknown cause | 798.1–798.9, 799.89, 799.9 | R68.8, R69, R96–R99 |
Appendix 2 Definition of psychosocial adversity using Clinical Practice Research Datalink medcodes
Self-harm
medcode | Description |
---|---|
10057 | Deliberate self-harm |
10464 | Self-harm |
17046 | [X]Intentional self-harm |
22107 | [V]Personal history of self-harm |
32267 | Self-mutilation |
35123 | Self-mutilation of hands |
45796 | [X]Sequel intentn self-harm assault+event of undeterm intent |
57479 | Self-mutilation of genitalia |
64200 | Self-mutilation of ears |
69263 | [X]Sequelae of intentional self-harm |
Overdose
medcode | Description |
---|---|
171 | Overdose of drug |
1493 | Cause of overdose – accidental |
6595 | Cause of overdose – deliberate |
11708 | Overdose of biological substance |
13568 | H/O: repeated overdose |
18379 | [X]Overdose – paracetamol |
28710 | [X]Overdose – heroin |
29861 | [X]Overdose – aspirin |
34703 | [X]Overdose – amitriptyline |
44886 | [X]Overdose – ibuprofen |
45748 | [X]Overdose – diazepam |
46280 | [X]Overdose – antidepressant |
48324 | [X]Overdose – benzodiazepine |
49552 | [X]Overdose – barbiturate |
51381 | [X]Overdose – temazepam |
52931 | [X]Overdose – nitrazepam |
55395 | [X]Overdose – sleeping tabs |
60559 | [X]Overdose – SSRI |
94725 | [X]Overdose – amobarbital |
99775 | Intentional overdose of prescription only medication |
Alcohol problems
medcode | Description |
---|---|
1399 | Alcohol problem drinking |
2081 | Alcoholism |
2082 | Alcohol withdrawal syndrome |
2083 | Alcohol detoxification |
2084 | Alcohol dependence syndrome |
2925 | Alcoholic polyneuropathy |
3216 | Acute alcoholic hepatitis |
4500 | Korsakov's alcoholic psychosis |
4506 | Alcoholic gastritis |
4743 | Alcoholic cirrhosis of liver |
4915 | Alcoholic cardiomyopathy |
5611 | [X]Mental and behavioural disorders due to use of alcohol |
5740 | Acute alcoholic intoxication in alcoholism |
5758 | [X]Chronic alcoholism |
6169 | Alcohol dependence syndrome NOS |
6467 | [X]Alcoholic hallucinosis |
7123 | [V]Personal history of alcoholism |
7602 | Chronic alcoholic hepatitis |
7885 | Alcoholic liver damage unspecified |
7943 | Alcoholic hepatitis |
8030 | [V]Alcohol abuse counselling and surveillance |
8363 | Oesophageal varices in alcoholic cirrhosis of the liver |
8388 | [V]Alcohol rehabilitation |
8430 | H/O: alcoholism |
9169 | [D]Alcohol blood level excessive |
9489 | Under care of community alcohol team |
9508 | [X]Acute alcoholic drunkenness |
9849 | Referral to community alcohol team |
10463 | [X]Intent self poison/exposure to alcohol |
10691 | Alcoholic fatty liver |
11106 | Korsakov's alcoholic psychosis with peripheral neuritis |
11670 | [X]Korsakov's psychosis, alcohol induced |
11740 | Alcohol misuse – enhanced services administration |
12353 | [X]Mental & behav dis due to use alcohol: psychotic disorder |
12554 | Referral to community drug and alcohol team |
12976 | Suspect alcohol abuse – denied |
12982 | Alcohol intake above recommended sensible limits |
16225 | Alcohol withdrawal delirium |
16237 | Alcoholic psychoses |
17259 | [X]Delirium tremens, alcohol induced |
17330 | Alcoholic hepatic failure |
17607 | [X]Alcoholic psychosis NOS |
18156 | Alcoholics anonymous |
18252 | Accidental poisoning by alcohol, NEC |
19217 | Alcohol causing toxic effect |
20514 | [X]Mental and behav dis due to use alcohol: withdrawal state |
20762 | Alcohol amnestic syndrome |
21412 | Adverse reaction to alcohol deterrents |
21624 | Episodic acute alcoholic intoxication in alcoholism |
21650 | Admitted to alcohol detoxification centre |
21713 | Alcoholic fibrosis and sclerosis of liver |
21879 | [X]Mental and behav dis due to use of alcohol: harmful use |
23978 | [X]Evid of alcohol involv determind by level of intoxication |
24064 | Continuous chronic alcoholism |
24485 | Chronic alcoholism in remission |
24984 | Alcohol-induced chronic pancreatitis |
25110 | Alcohol withdrawal hallucinosis |
26106 | Episodic chronic alcoholism |
26323 | [X]Alcoholic dementia NOS |
27342 | Alcoholic dementia NOS |
28780 | [X]Alcohol addiction |
29691 | Aversion therapy – alcoholism |
30036 | [X]Poisoning/exposure, ? intent, to alcohol |
30162 | [X]Alcoholic paranoia |
30404 | Alcoholic paranoia |
30460 | Alcoholism counselling |
30604 | Alcohol-induced epilepsy |
31443 | Chronic alcoholism |
31605 | [X]Accident poisoning/exposure to alcohol |
31742 | Alcoholic myopathy |
32927 | [X]Alcohol withdrawal-induced seizure |
33635 | Chronic alcoholism NOS |
33839 | Cerebellar ataxia due to alcoholism |
36296 | Acute alcoholic intoxication in alcoholism NOS |
36499 | Alcohol causing toxic effect NOS |
36687 | Alcohol deterrent poisoning |
36748 | Alcoholic encephalopathy |
37691 | [X]Chronic alcoholic brain syndrome |
37946 | Chronic alcoholic brain syndrome |
38061 | Alcohol induced hallucinations |
39327 | [X]Mental and behav dis due to use alcohol: dependence syndr |
39799 | [X]Mental and behav dis due to use alcohol: amnesic syndrome |
40530 | Acute alcoholic intoxication, unspecified, in alcoholism |
40541 | Accidental poisoning by alcoholic beverages |
41638 | [X]Int self poison/exposure to alcohol at home |
41920 | Alcohol amnestic syndrome NOS |
41983 | Alcohol detoxification |
43193 | Unspecified chronic alcoholism |
44299 | [X]Mental & behav dis due to use alcohol: acute intoxication |
45169 | [X]Men & behav dis due to use alcohol: oth men & behav dis |
46677 | Alcohol withdrawal regime |
47555 | Cerebral degeneration due to alcoholism |
48241 | [X] Adverse reaction to alcohol deterrents |
48514 | Denatured alcohol causing toxic effect |
55415 | Accidental poisoning by alcohol NOS |
56410 | Delivery of rehabilitation for alcohol addiction |
56947 | Continuous acute alcoholic intoxication in alcoholism |
57714 | Alcohol dependence with acute alcoholic intoxication |
57939 | Pathological alcohol intoxication |
59414 | [X]Intent self poison alcohol unspecif place |
59574 | Acute alcoholic intoxication in remission, in alcoholism |
60752 | Accidental poisoning by secondary propyl alcohol |
61187 | [X]Accid poison/expos to alcohol unspecif place |
61190 | [X]Pois/expos ?intent to alcohol unspecif place |
63306 | [X]Accident poison/exposure to alcohol at home |
63529 | Alcohol misuse – enhanced service completed |
63876 | [X]Accid poison/expos alcohol in street/highway |
64389 | [X]Ment & behav dis due use alcohol: unsp ment & behav dis |
65754 | Alcohol-induced pseudo-Cushing's syndrome |
65932 | [X]Alcoholic jealousy |
67651 | Alcoholic psychosis NOS |
68159 | [X]Poison/exposure ?intent, to alcohol at home |
69407 | [X]Pois/exp ?intent alcohol school/pub admin area |
73876 | [X]Alcohol deterrents caus adverse effects in therapeut use |
92908 | [X]Accid poison/expos alcohol trade/service area |
94553 | Referral to specialist alcohol treatment service |
94670 | Alcohol misuse |
95181 | Alcohol reduction programme |
96053 | Brief intervention for excessive alcohol consumptn completed |
96054 | Extended intervention for excessive alcohol consumptn complt |
96219 | [X]Pois/expos ?intent alcohol in street/highway |
96993 | Referral to alcohol brief intervention service |
103069 | [X]Acc poison/expos alcohol school/pub admin area |
104611 | Alcohol-induced acute pancreatitis |
104702 | [X]Accid pois/expos alcohol in sport/athletic area |
Prison
medcode | Description |
---|---|
1123 | In prison |
10269 | Released from prison |
21521 | Prison record |
26013 | Prison sentence |
28655 | [V]Imprisonment |
29760 | [V]Problems related to release from prison |
37738 | Imprisonment record |
52682 | Prison medical examination |
53439 | [V]Prison medical |
54399 | Place of occurrence of accident or poisoning, jail |
59330 | Place of occurrence of accident or poisoning, prison |
105175 | Medically fit for activity outside prison |
Homeless
medcode | Description |
---|---|
2562 | Homeless |
25452 | Homeless single person |
67112 | Homeless – enhanced services administration |
96605 | Homeless – enhanced service complete |
97757 | Sofa surfer – person of no fixed abod |
104962 | Length of time homeless |
107393 | Under care of homeless advocacy service |
Appendix 3 Report on public and patient involvement
Appendix 4 Registered patients within Clinical Practice Research Datalink and the UK by year
Year | CPRD patients by country (0000) | Registered patients by country (0000) | ||||||||
---|---|---|---|---|---|---|---|---|---|---|
England | Wales | Scotland | Northern Ireland | UK | England | Wales | Scotland | Northern Ireland | UK | |
1998 | 236 | 19 | 17 | 8 | 280 | 5112 | 300 | 536 | 176 | 6124 |
1999 | 290 | 25 | 21 | 10 | 346 | 5090 | 298 | 537 | 177 | 6102 |
2000 | 329 | 30 | 24 | 12 | 395 | 5134 | 301 | 535 | 178 | 6148 |
2001 | 359 | 34 | 31 | 14 | 439 | 5125 | 301 | 535 | 177 | 6139 |
2002 | 384 | 35 | 38 | 16 | 473 | 5152 | 304 | 535 | 178 | 6168 |
2003 | 393 | 38 | 48 | 16 | 495 | 5271 | 302 | 534 | 178 | 6286 |
2004 | 403 | 40 | 51 | 17 | 511 | 5253 | 304 | 537 | 179 | 6273 |
2005 | 409 | 41 | 51 | 17 | 518 | 5282 | 305 | 539 | 179 | 6305 |
2006 | 419 | 41 | 52 | 17 | 529 | 5324 | 309 | 541 | 181 | 6355 |
2007 | 422 | 43 | 52 | 17 | 534 | 5307 | 309 | 542 | 183 | 6342 |
2008 | 417 | 44 | 53 | 16 | 529 | 5361 | 311 | 547 | 185 | 6404 |
2009 | 414 | 44 | 53 | 16 | 527 | 5414 | 313 | 550 | 187 | 6464 |
2010 | 413 | 44 | 53 | 16 | 526 | 5502 | 315 | 552 | 188 | 6557 |
2011 | 399 | 44 | 54 | 16 | 513 | 5531 | 316 | 553 | 190 | 6589 |
2012 | 386 | 45 | 54 | 16 | 500 | 5574 | 317 | 555 | 191 | 6637 |
2013 | 379 | 47 | 54 | 16 | 495 | 5601 | 318 | 557 | 192 | 6667 |
2014 | 335 | 46 | 55 | 16 | 451 | 5647 | 317 | 560 | 194 | 6718 |
List of abbreviations
- ACM
- all-cause mortality
- CI
- confidence interval
- CPRD
- Clinical Practice Research Datalink
- df
- degrees of freedom
- DRP
- drug-related poisoning
- GP
- general practitioner
- HR
- hazard ratio
- ICD-9
- International Classification of Diseases, Ninth Edition
- ICD-10
- International Classification of Diseases, Tenth Edition
- IPW
- inverse probability weighted
- IRR
- incidence rate ratio
- IV
- instrumental variable
- NDTMS
- National Drug Treatment Monitoring System
- OST
- opiate substitution treatment
- PPI
- patient and public involvement
- PSM
- propensity score matching
- SCCS
- self-controlled case series
- SD
- standard deviation
- SE
- standard error
- WP
- work package