Notes
Article history
The research reported in this issue of the journal was funded by the HS&DR programme or one of its preceding programmes as project number 14/70/96. The contractual start date was in January 2016. The final report began editorial review in May 2018 and was accepted for publication in March 2019. The authors have been wholly responsible for all data collection, analysis and interpretation, and for writing up their work. The HS&DR editors and production house have tried to ensure the accuracy of the authors’ report and would like to thank the reviewers for their constructive comments on the final report document. However, they do not accept liability for damages or losses arising from material published in this report.
Declared competing interests of authors
none
Permissions
Copyright statement
© Queen’s Printer and Controller of HMSO 2020. This work was produced by Aldus et al. under the terms of a commissioning contract issued by the Secretary of State for Health and Social Care. This issue may be freely reproduced for the purposes of private research and study and extracts (or indeed, the full report) may be included in professional journals provided that suitable acknowledgement is made and the reproduction is not associated with any form of advertising. Applications for commercial reproduction should be addressed to: NIHR Journals Library, National Institute for Health Research, Evaluation, Trials and Studies Coordinating Centre, Alpha House, University of Southampton Science Park, Southampton SO16 7NS, UK.
2020 Queen’s Printer and Controller of HMSO
Chapter 1 Background and introduction
Dementia
Dementia is a syndrome of progressive loss of both cognitive and everyday functional ability. 1 It is associated with a number of underlying brain pathologies, including Alzheimer’s disease-type pathologies, cerebrovascular disease and Lewy body disease among many others. 2,3 Memory impairment is a characteristic of Alzheimer’s disease, but other dementias, particularly those presenting at younger ages, can have different profiles of cognitive impairment and non-cognitive symptoms, including psychosis, personality changes and behaviour changes.
Dementia has an enormous social and economic impact;4,5 as it is a major cause of disability, there is a need for long-term residential care and it is increasingly recognised as a cause of death. Dementia is more common after the age of 65 years, after which its incidence roughly doubles every 5 years. 6
It is estimated that there are 50 million people living with dementia globally,1 with an economic cost of US$1T. 7 The prevalence of dementia is difficult to estimate, but as of 2018, in England, there are around 650,000 people living with dementia and around 540,000 carers of people with dementia. 8
Diagnosis of dementia in the UK and internationally
Dementia can be formally diagnosed when a patient meets certain clinical criteria. These criteria vary but typically require (1) impairment in at least two aspects of cognitive function and (2) an associated impact on daily life, such as disability or loss of occupation caused by cognitive impairment. A diagnosis of dementia is valid only where symptoms of the impairment are not reversible (e.g. symptoms arising as a result of depression or from the side effects of prescribed medication) or transient (e.g. delirium), and cognitive impairment is likely to worsen as the underlying pathology advances. There is no single accepted operational definition of dementia, with different interpretations and thresholds used to make a diagnosis in different research and clinical contexts.
The benefits and harms of receiving a clinical diagnosis for a person with dementia are not well understood and are likely to vary substantially between individuals. 9 There is usually no medical treatment that will alter the course of the neurodegeneration, but some symptomatic treatment to improve cognitive function is available. It is also suggested that a clinical diagnosis of dementia may improve quality of life by enabling people with dementia and their families and carers to receive appropriate support and information that may help with daily living, delay institutionalisation or avert health crises. 10
Diagnosing more people with dementia has been a priority in dementia care policy both in the UK and internationally. Across the developing world, the vast majority of people with dementia do not have access to services providing dementia diagnosis. 11 The World Health Organization Global Action Plan 2017–2512 set a global target (global target 4): in at least 50% of countries, 50% of the estimated number of people with dementia will be diagnosed by 2025 (as a minimum). In the UK, the 2009 National Dementia Strategy set a target that 67% of people living with dementia should have a formal diagnosis recorded in their primary care record.
Estimating dementia diagnosis rates
Current estimates of the proportion of people with a diagnosis (often known as the ‘diagnosis rate’) are made by dividing the number of reported diagnosed cases by the number of cases that there are expected to be in a population, with this expected number calculated using prevalence estimates from epidemiological research. 13 Clearly this diagnosis rate estimate relies on having precise, unbiased estimates for prevalence that are up to date and are directly applicable in the areas for which the diagnosis rate is being calculated.
However, estimating the prevalence of dementia, and hence the true number of people living with dementia, in any population is extremely difficult. National population-representative epidemiological studies of dementia require huge resources and have been commissioned only in the UK through the Cognitive Function and Ageing Studies. 14 These studies, and similar studies in other countries, proceed by selecting large representative samples of target populations (say, all adults over the age of 65 years). Then, by applying standardised diagnostic criteria to each member of the sample, in theory, the prevalence of dementia in that population can be estimated.
This approach also has several technical limitations. Even with standardised assessments being conducted among many thousands of participants, the number of people identified with dementia, and hence the precision of prevalence estimates, will be relatively small. This is particularly true when studies produce prevalence rates using smaller subgroups of their samples, such as within age groups or specific residential settings.
Medical settings cannot be used to recruit samples for prevalence studies owing to biases inherent in help-seeking and diagnosis, and so prevalence estimates must be made using samples drawn from community settings. However, people with dementia are difficult to access for research in a community setting, with many barriers to participation. Therefore, if those with dementia are less likely to participate than those without, then assumptions regarding this process must be made to attempt to produce unbiased estimates of prevalence.
Dementia is difficult to diagnose, particularly in its early stages. As a result, different operational definitions of dementia can lead to vastly different numbers of cases being identified and therefore large variation in estimates of dementia prevalence. 15 If different definitions of dementia are used in establishing the numerator and denominator of a diagnosis rate calculation, then the result will be questionable. Because there is no standardised operational definition of dementia in use across England, and prevalence estimates each use different epidemiological definitions, this is a clear limitation of current diagnosis rate estimates.
Finally, recent evidence suggests a fall in dementia prevalence across all age groups since 1990. 16 This means that older pan-European estimates of prevalence, on which UK and regional prevalence had previously been based, may overestimate the true number of current cases (the denominator for a diagnosis rate calculation) and, hence, underestimate the proportion of people living with dementia who have a diagnosis. This has now been corrected by incorporating the more recent and England-specific Cognitive Function and Ageing Study II (CFAS II) prevalence estimates in the diagnosis rate calculation, but if dementia prevalence has continued to fall since 2008 then these estimates will now also be unreliable.
Limitations of current approaches for estimating diagnosis rate
Estimates of dementia diagnosis rates based on comparing the numbers of cases with estimates of dementia prevalence are limited in a number of important ways.
First, it is not possible to disaggregate rates by socioeconomic or clinical characteristics. Therefore, it is not possible to currently say what the care needs of the undiagnosed population are likely to be compared with the diagnosed population, and it is not possible to describe inequalities in access to diagnosis. It is well known that those with very mild dementia are far less likely to have received a diagnosis than those with severe dementia. Reporting a single diagnosis rate of, say, 60%, implies that the characteristics and needs of the 60% diagnosed and 40% undiagnosed populations are more or less the same. It ignores the fact that the majority of those who are undiagnosed are likely to have very mild dementia, whereas the overwhelming majority of those with severe dementia and at greatest need are likely to have a diagnosis, or at least clinical support that recognises their cognitive impairment without a formal primary care diagnosis being necessary. Similarly, those who are undiagnosed may be so because they are managing well with fewer psychological symptoms or difficulties with activities of daily living. Directly assessing the population with undiagnosed population is essential for this to be meaningful.
As described above, dementia prevalence estimates are unreliable when applied to smaller areas and so diagnosis rates at the regional and especially the practice level will have large ranges of uncertainty, particularly if estimates from other regions with different demographic characteristics are applied. If a particular region has lower rates of factors linked to dementia incidence, then this is likely to depress the number of observed cases, but the expected number based on national prevalence figures will remain unchanged. Hence, the estimate of diagnosis rate might be adversely affected.
Third, if any primary care cases are ‘false positives’, that is people with a record of dementia but who do not in fact have dementia, this will bias estimates of diagnosis rate upwards. Conversely, using ‘diagnosis rate’ as a measure of quality of care or a metric on which to base incentives at practice or regional level might incentivise diagnoses to be made for reasons other than direct patient benefit.
Finally, in using routine records of recorded cases, there is an implicit dichotomisation of individuals into those who are ‘diagnosed’ and receiving support and those who are ‘undiagnosed’ and so are assumed to be unknown to health services (with respect to their cognitive impairment). This may not reflect the reality that many individuals are likely to be in a process of help-seeking and diagnosis, or have a cognitive impairment that is known and managed but is not formally diagnosed and recorded as dementia. It also does not reflect the substantial number of patients with a dementia diagnosis in a hospital record but not their primary care record. Epidemiological studies have found that large proportions of the undiagnosed population were known to general practitioner (GPs) as having a cognitive impairment or ‘suspected dementia’,17,18 or were receiving dementia treatment,19 and that this group could be as large the group with a formal diagnosis.
Current trends in reported diagnosis rate in England
In January 2018, NHS Digital20 estimated the number of people aged > 65 years with dementia in England to be 645,101. However there were only 438,142 coded dementia diagnoses among those aged > 65 years at that time. This corresponds to a ‘diagnosis rate’ of 67.9%. 13 This represents a considerable change from 33% reported in 2007,21 but masks a wide variation across the UK, with some regions recording up to 90% of their estimated number of dementia cases and others around 50%. The reasons for this variation are not clear. Even though many Clinical Commissioning Groups (CCGs) have devoted substantial resource to raising awareness and encouraging dementia diagnosis in general practice, it is likely that at least some of the variation is linked to one or more of the difficulties in estimating and comparing diagnosis rates described above.
Why do people with dementia remain undiagnosed?
Despite the difficulties in estimating diagnosis rates outlined above, it is certain that there are a substantial number of people living with dementia who are not diagnosed. The reasons for this among individual patients, their families and their doctors have been extensively explored in the qualitative literature. There are many complex factors influencing presentation and diagnosis. 22,23 For example, some individuals will be fearful or antagonistic towards seeking medical advice. They may deny that there is a problem and reject suggestions from family members that they should see a doctor or get help. Social and cultural factors are also important. For example, some ethnic groups may regard dementia as something to be concealed within the family network. Some of the stated ‘barriers’ to presentation are the belief that dementia symptoms are a normal aspect of ageing, the ability to manage without help, stigma, lack of knowledge around dementia, lack of treatment, as well as the possibility that a diagnosis of dementia would do harm and be a negative development for the individual and their context (possibly from observation of support to others in similar situations). GPs consistently attribute missed diagnosis to a lack of education and support for themselves and carers, their own concern about the availability of secondary or support services, financial and time constraints, and the difficulty in making the diagnosis or breaking bad news. 24
In contrast, acute events such as hospitalisation or interaction with health-care services, change in circumstances, behavioural disturbance or concerned family can act as triggers to assessment and ultimately diagnosis. 22,25,26
Our conversations with public and patient involvement (PPI) representatives have reinforced the possibility that help-seeking is inhibited by a fear of the consequences of diagnosis and a determination among some family units to cope without outside interference until help-seeking becomes unavoidable (see Chapter 12). Management of other health conditions was also suggested by our PPI representatives as a potential distraction that meant cognitive impairments are not fully explored by GPs (so-called diagnostic overshadowing) and the possibility that formal diagnoses are not made if they are seen to be likely to impact on care arrangements in which an individual and those around them are coping well.
The benefits and harms of diagnosis for people with dementia
Central to the debate around the need for timely diagnosis (hence the political imperative for increased diagnosis rate) is whether a diagnosis is ultimately helpful or harmful to a person with dementia and those around them. At present, there is no treatment that can delay the progress of any dementia. Symptomatic treatments exist for various stages of Alzheimer’s disease that have been shown to improve cognitive function and ability in some cases. Although effects are typically small and side effects can be significant, such treatments are generally regarded as offering a net benefit for a significant number of people with Alzheimer’s disease. Non-pharmacological interventions to support people with dementia and their families and carers have stronger support.
However, the fact of the existence of programmes to support people with dementia who seek diagnosis does not automatically translate into a net benefit of diagnosis.
Psychosocial and occupational benefits and harms of dementia diagnosis are also reported.
Although many people with dementia report feeling relief at understanding their symptoms and being able to plan for the future, they may also experience increased tension while negotiating their new identity, feel the need for increased social and psychological support (which may or may not be available to them) or have a heightened fear of what the future holds for them. 22
Hence, to understand the impact of diagnosis it is essential to compare people living with dementia who are diagnosed with those who are not diagnosed. At the time of writing, two epidemiological studies of the benefits or harms of dementia showed no improvement with respect to mortality or functional decline, but did suggest that those seeking referral or diagnosed earlier were more likely to transfer to institutional care. 27,28
Why is further research needed?
Globally and nationally, substantial resources are being directed towards diagnosing more people with dementia. Nationally, this has led to a significantly higher number of people with dementia being diagnosed, although there remains a substantial gap between the expected number of cases and the number of cases reported in some areas.
The James Lind Alliance priorities for dementia research include the consequences of the emphasis on early diagnosis, with questions about the impact of early diagnosis and what the appropriate role of primary care in this would be (this was rated in the top three priorities). 29
Local authorities and CCGs are tasked with examining areas where the number of patients diagnosed is significantly lower than the target based on the number expected from prevalence data. Yet local commissioning groups, GPs and the Alzheimer’s Society have expressed concern over the validity of comparing local dementia registers with national or international estimated prevalence data. Additional concerns are the possibility of overdiagnosis and the large number of people who are known to services but who are not diagnosed. 30 Without understanding any inequalities in access to dementia diagnosis, it will be difficult to design strategies to make this more accessible to those who would benefit. Lessons may be learnt from the management of other chronic long-term illnesses.
Reviews of whether or not dementia meets the internationally accepted criteria for the introduction of a screening programme also highlight the lack of evidence for the benefit of diagnosis in the context of currently available treatment, and the lack of evidence for the acceptability or effectiveness of any potential screening or case-finding programme. 31,32 Despite this, the case is often forcefully made for earlier diagnosis of dementia based on supposed social and economic benefits without any direct evidence for these,10 and targets for the numbers of people who health services are expected to diagnose remain in place.
Hence, a considerable number of questions remain unanswered. Central to these is the characterisation of the population with undiagnosed dementia and estimates of the possible social and economic benefits of diagnosis, which we begin to address in the current work.
Chapter 2 Aims and objectives
Broad aims
Our main aim was to estimate the prevalence and distribution of dementia not recorded in primary care among the UK population aged ≥ 65 years. To achieve this, we linked data from an epidemiological study to primary care records of the study participants. The study used was CFAS II,16 which is the most recently recruited cohort study representative of the primary care population of England to study dementia and which underpins current UK dementia prevalence estimates. CFAS II is fully described at www.cfas.ac.uk/ (accessed 11 February 2019).
In particular, we linked and compared objectively and independently conducted CFAS II dementia assessments with records of cognitive complaints and dementia diagnoses recorded in primary care.
Crucially, this means that we were able to establish the primary care dementia diagnosis status of a population-representative cohort of people who independently met an objective, standardised and validated assessment of dementia (henceforth ‘people with dementia’). This enables us to estimate the prevalence, correlates and consequences of undiagnosed dementia in the older population of England.
This study is reported in accordance with Strengthening the Reporting of Observational Studies in Epidemiology (STROBE) guidelines.
Specific research questions
This linkage was used to address the following specific questions.
Research question 1a (including subquestions)
What was the prevalence of undiagnosed dementia between 2008 and 2013? How many undiagnosed people with dementia had a GP record of cognitive complaint/impairment? How many had been referred to specialist services? How many had no record of any cognitive impairment?
Research question 1b
What are the social and clinical characteristics of the population without dementia but with a dementia diagnosis recorded in primary care?
Research question 2
What are the independent predictors of help-seeking and diagnosis among people with dementia?
Research question 3 (including subquestions)
What is the incidence of new diagnosis among the population with undiagnosed dementia? What are the predictors of getting a diagnosis among people with undiagnosed dementia?
Research question 4
How are clinical, psychosocial and health-care utilisation trajectories associated with diagnosis status among people with dementia?
Study design and objectives
This study, henceforth the Cognitive Function and Ageing Study II Dementia Diagnosis Study (CADDY), is a record linkage study of a population-representative cohort of older people in England. In short, we linked data from GP records with epidemiologically determined dementia diagnoses from a population-representative cohort of older people to estimate the prevalence of undiagnosed dementia and the causes and consequences of diagnosis among people living with dementia.
To answer our research questions our study required a sample of people with dementia who:
-
are representative of the population with dementia
-
are known to have dementia but with their dementia status determined independently of health-care services
-
had primary care records that we could access and extract data from.
Recruiting this sample specifically for this research was prohibitively expensive, and CFAS II is the only study in England that meets these criteria. Although other English studies of older people, such as the English Longitudinal Study on Ageing, do include people with dementia, dementia is typically not systematically assessed using validated algorithms (www.elsa-project.ac.uk/; accessed 14 June 2019). Epidemiological studies do not often include the population in residential care settings and often it is not possible to access health records.
CFAS II consented participants for medical record linkage but did not attempt to collect any data from primary care beyond that needed for recruitment into the study and ongoing contact with participants. Hence, the main objectives of CADDY were to:
-
identify a subsample of the CFAS II participants for inclusion in the current study, the ‘CADDY’ sample
-
contact the GPs of these participants (their GP at the time of study recruitment) and extract data on recorded cognitive concerns, referral and dementia diagnoses
-
encode and link data extracted from primary care medical records to Cognitive Function and Ageing Study (CFAS) assessment and interview data, and additional data supplied by CFAS on mortality and area-level deprivation from the Office for National Statistics (ONS)
-
conduct an analysis of the linked data set to estimate each of the research questions above.
Figure 1 illustrates the relationship between the pre-existing CFAS II (shaded boxes) and CADDY (white boxes).
FIGURE 1.
The design of CADDY with respect to the CFAS II.
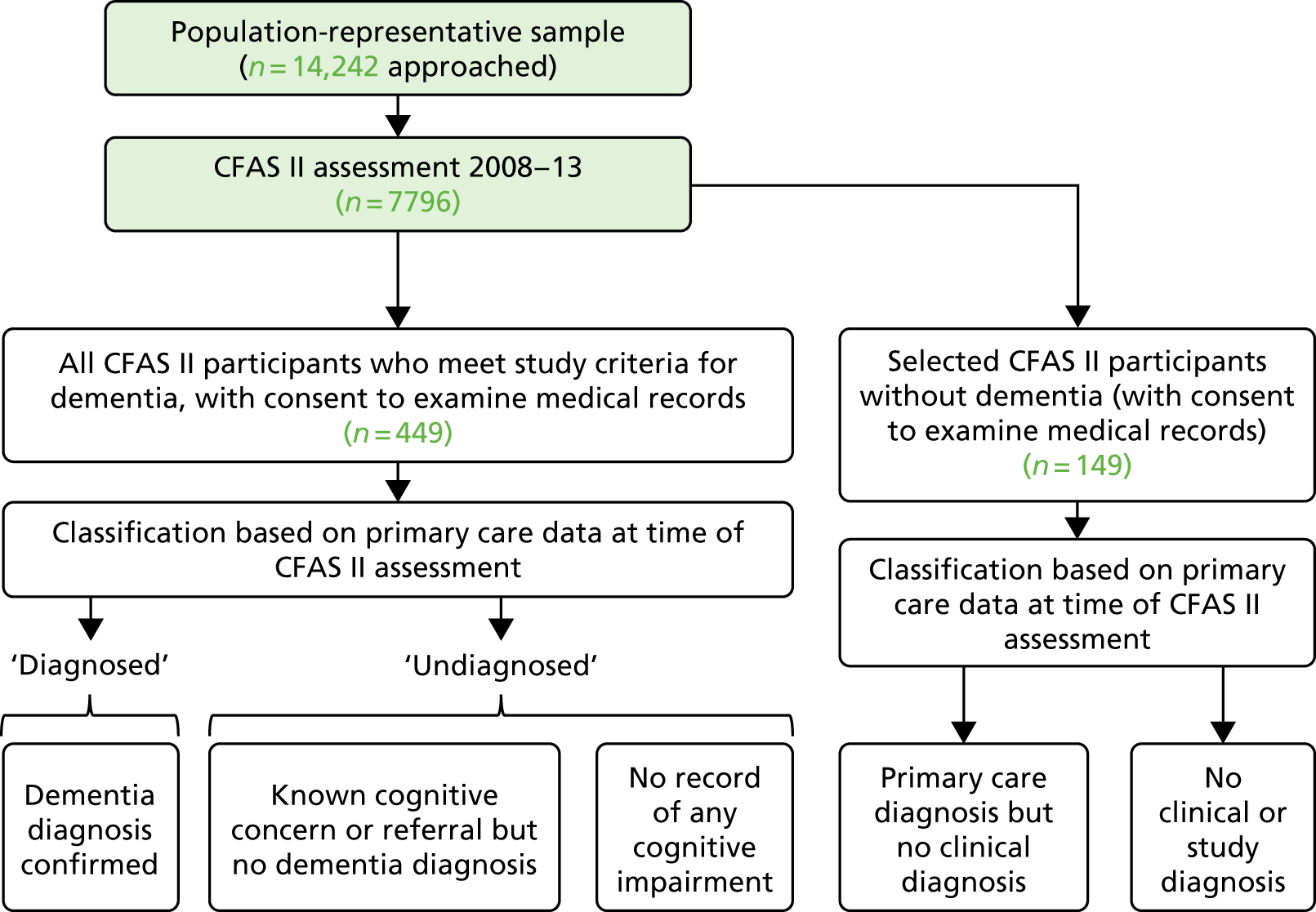
Chapter 3 Setting, population and sample: the Cognitive Function and Ageing Study II
This record linkage study was carried out at the University of East Anglia (UEA) in the period January 2016 to April 2018. Between July 2016 and September 2017 the CADDY team sought data from the primary care records of a subset of CFAS II participants.
CFAS II design
CFAS II is an epidemiological study of ageing and dementia conducted in three geographic regions of England. The study has been described in detail previously,16 but, in short, the study aimed to recruit 7500 individuals aged ≥ 65 years, selected at random from primary care registers in three geographic areas, with samples of around 2500 individuals aged ≥ 65 years in each area (Nottingham, Newcastle and rural Cambridgeshire). The sample was stratified to recruit equal numbers of those aged 65–74 years and those aged ≥ 75 years.
Those with severe cognitive impairment or living in care homes were included in the study. The only exclusion criterion was presence of a terminal illness resulting in life expectancy of < 6 months at time of sampling, as assessed by the participant’s GP.
Each participant received a letter informing them about the study and was then visited up to three times by a CFAS interviewer. Once consent had been ascertained, the participant and, where appropriate, an informant, or, in the case of those who could not give consent, a proxy, were interviewed using standardised assessments as described in Chapter 5 (with full details available at www.cfas.ac.uk; accessed 26 June 2019).
The W1 interview data were available for 7762 participants of the 14,242 participants who were approached. The W1 assessments were conducted between 2008 and 2011. Before carrying out a 2-year follow-up assessment, each participant’s primary care practice was contacted to determine their vital status. Each surviving participant was then invited to undertake a similar assessment approximately 2 years after W1 [wave 2 (W2), conducted between 2011 and 2013]. A total of 5156 participants took part at W2.
At the W1 assessment, consent for the research team to access medical records and to link this to study data was sought. Although consent to the CFAS II could be made by proxy, medical record consent was sought only if the participant had sufficient mental capacity to consent personally at W1, meaning that those with moderate or severe dementia are under-represented among those with record linkage at W1.
CFAS II ascertainment of dementia
CFAS II assessments were designed to include the ascertainment of dementia based on the Geriatric Mental State-Automated Geriatric Examination for Computer Assisted Taxonomy (GMS-AGECAT) algorithm, which has been shown to be valid with respect to the Diagnostic and Statistical Manual of Mental Disorders, Third Edition (DSM-IIIR), and was directly validated by full clinical work-up in a subsample during the earlier Medical Research Council (MRC) CFAS (Lu Gao, MRC Biostatistics Unit, 2016, personal communication).
Each CFAS II assessment included a face-to-face interview with the participant and administration of a series of tests of cognitive function, including the Geriatric Mental State Examination. A second interview with a knowledgeable informant was requested in the case of 20% of participants, stratified such that those with more cognitive impairment were over-represented and including informants for all participants with significant cognitive impairment. Informants were predominantly the participant’s spouse (35%), son or daughter (39%), friend or other family member (17%), or caretaker, warden or paid carer (9%). At least 90% of informants saw the participant several times per week or more. A proxy respondent could attend the face-to-face interview and answer some sections where appropriate. Interviewers also provided judgement on some aspects (e.g. their perception of demeanour or the ability of a participant to respond). The classification of dementia status was in most cases based on the algorithmic approach, but, if data were missing from the algorithm, the entire interview and vignettes from the interviewers were reviewed by the same diagnostician as in the original CFAS to maintain consistency using DSM-IIIR criteria. Most such individuals were seriously impaired and had moderate to severe dementia. Crucially, the algorithmic study diagnosis is made without information gleaned from health-care services. In the case of a small number of participants with missing algorithmic classification, the nature of the missingness, such as care settings, combined with vignettes, made this blinding impossible. This applies to 16 W1 participants of CADDY and seven W2 participants. Full details of all assessments are available on the CFAS website (URL: www.cfas.ac.uk). In accordance with CFAS II protocol and ethics approvals, no individual considered to be cognitively impaired or who received a study diagnosis of dementia under CFAS II was referred to health-care professionals.
Selection of CADDY participants
The main aim of CADDY is to link the knowledge an individual participant’s GP has about their cognitive or dementia status with the dementia status objectively ascertained under the CFAS study for the same individual.
Sample with dementia
All CFAS II participants (1) with dementia diagnosed at W1 or W2 and (2) who gave consent to link interview data to medical records were selected to be included in CADDY. Thus, CADDY includes a cohort of those with dementia sampled to be representative of the population with dementia in England.
Selection of sample without dementia
A group without dementia were also included in CADDY. This group was included to (1) blind participating practices to the study dementia status of individual patients (i.e. to ensure that practices could not identify which patients had received a study diagnosis of dementia) and (2) allow us to estimate the number of ‘false-positive’ dementia cases. Data for these participants were used primarily to address research question 1b. CADDY participants who had dementia at W2 but not at W1 were included as ‘no dementia’ cases at W1, along with an additional group of 149 participants without dementia at either W1 or W2. This group was weighted towards those who were cognitively impaired and selected such that at least one participant with no dementia at either W1 or W2 was selected from each practice and all participants who reported a dementia-specific medication were also included. For full details of this selection see Appendix 1.
Hence, CADDY eligibility criteria were as follows.
Inclusion criteria
-
Participation in CFAS II.
-
Consent provided by the participant to use medical records for research.
-
Consent provided for data to be held long term and used for research purposes, including in the event of the participant’s incapacity or death.
-
Study diagnosis of dementia (made using GMS-AGECAT algorithm from CFAS II assessment) or selected participant without dementia.
Exclusion criteria
-
Withdrawal of consent at any point.
Chapter 4 CADDY data collection and management
Primary care data collection
For all participants we requested, from their general practice, information from their primary care records about different aspects of dementia and cognitive impairment. The case report form (CRF) is appended (see Appendix 2). This was to be completed by practice staff either electronically via a web-form or by hand and posted back to the study team.
Data collection
Principles for maintaining confidentiality
In collaboration with the Norwich Clinical Trials Unit (CTU), we developed a system to ensure that confidentiality would be maintained and to reduce the risk of any confidentiality breach. In particular:
-
Clinical data were not linked to NHS number on any database held outside the GP practice.
-
NHS number or patient and practice contact information were not linked to a CFAS identifier outside the existing administrative database held by the University of Cambridge.
-
No NHS number was stored unencrypted at the Norwich CTU or the UEA.
-
NHS secure e-mail was used in all correspondence carrying personal identifiers.
Generation of CADDY identifiers
A new identifier was created for each CADDY participant. This was particularly important to ensure confidentiality when patient information was transmitted electronically from general practices to the CADDY team. This identifier was generated by the CFAS II team.
Data management and storage
CFAS II participant-identifying information is held in an administrative database at the University of Cambridge Department of Public Health and Primary Care. It is held separately to participants’ interview and assessment data, which are held by the University of Cambridge Biostatistics Unit. These databases are indexed by a CFAS identification (ID) number. Three CADDY databases and an encrypted spreadsheet linking CFAS II participant ID with CADDY ID were created:
-
CADDY Administrative Database for participant NHS numbers, gender, month and year of birth and general practice identifiers. This database was extracted from the CFAS II administrative database by CFAS II staff and provided to the CADDY team in person encrypted on an NHS-recommended flash drive. Data were used by the study manager to identify patients to their general practices by NHS number. At study completion, the CADDY Administrative Database was destroyed.
-
CADDY Clinical Management Database for patient primary care data hosted at the Norwich CTU, using a Research Data Capture system (REDCap version 6.10.17; Vanderbilt University, Nashville, TN, USA) and populated by practice staff from participant records using a web-based electronic CRF. This database enabled population of an electronic CRF that was accessible by participating GPs and the CADDY study manager, to ensure that data for the correct participant are entered into the database, to generate queries when inconsistent or incomplete data are detected and finally as a secure repository of primary care data. No personal identifiers were held in this database.
-
CADDY Analysis Database held primary care data extracted from the Clinical Management Database and CFAS II demographic, assessment and interview data extracted from the CFAS II data set. The primary function of this database was to act as a repository of linked data to be analysed. No personal identifiers were held in this database. This database was indexed by CFAS II ID. A copy of the CADDY Analysis Database indexed only by CFAS ID was returned to the University of Cambridge and is available to researchers on request and by approval of the CFAS II oversight and management committee, following the process for data sharing current at the time of application.
Electronic case report form
The CADDY data were collected and managed using a research data capture tool, REDCap, hosted at the Norwich CTU. REDCap is a secure, web-based application designed to support data capture for research studies. It provides (1) an intuitive interface for validated data entry, (2) audit trails and (3) automated export to common statistical packages. 33 REDCap enabled practice staff to enter the requested information directly into the clinical management database. The NHS number was not shown or transmitted with any returned data.
Pilot data collection phase
A 2-month pilot phase was undertaken with four general practices to ensure that the primary care data collection process was robust and to revise CRFs, CRF guidance and data management procedures. No major revisions to the data collection process were made as a result of this.
Primary care data collection
A standard operating procedure was developed to standardise contact with practices.
Contact details for the practices from which participants were originally recruited were verified. Each practice was initially contacted up to three times by telephone by the study manager or study administrator. During this initial stage, we established the most appropriate contact person and discussed practice willingness and ability to participate, addressed any initial questions about the study and established the practice preference for either paper or electronic communication of study information and data collection.
Practices received study information packs comprising a letter of introduction to the study, a participant information sheet, an example CRF and guidance notes for CRF completion, the NHS Health Research Authority (HRA) letter of approval, and the HRA statement of activities.
Once practices agreed to take part in CADDY, each was sent a key linking CADDY ID to NHS numbers for participants registered with their practice. Practices preferring electronic communication also received a unique link to an electronic CRF hosted through REDCap. E-mail communication was through secure NHS.net accounts.
When the practice requested paper communication, a hard-copy CRF was generated for each participant, using information held on the administrative database. The practice completed the paper CRF and returned this to the research team at the UEA, who subsequently entered data from the paper CRF into REDCap.
It is usual practice for the primary care records of patients who are deceased to be returned by their general practice to a primary care data repository. The data repository for Cambridgeshire, Nottinghamshire and Newcastle was Primary Care Support England (PCSE). 34 When patient records were unavailable at the patient’s primary care practice, regional Clinical Research Network (CRN) staff applied to PCSE for access to the study records for patients within their region. PCSE returned patient records in hard copy. CRN staff members extracted and returned data to the study team via paper-based forms or REDCap. When practices had merged, details for their participants were sought from the new partnership. When practices were closed, patient records were sought from PCSE.
Support for practices
We made every effort to support practice staff to make the data collection process as easy and efficient as possible.
Practices were reimbursed for the time spent on extracting data for the study. Each practice was paid £75 for participating in CADDY, and an additional £25 for each CRF completed.
When practices wanted to take part but did not have the capacity to do so, staff from their regional CRN visited the practice to extract the required data. Although usually keen to participate, most practices had limited capacity owing to workload and so by prior arrangement many were provided with weekly or fortnightly telephone reminders to complete data collection. At each reminder contact, the research team tried to determine barriers to data return and acted to resolve any issues. Some practices requested copies of original consent forms, which were provided directly to practices by the CFAS administration team. CADDY researchers were available to address queries encountered by practice staff while completing the forms.
Validation of records
Two distinct processes helped us to ensure that only the correct patient record was returned and to help us to understand the accuracy of data received.
First, three validation questions were included in each CRF, relating to gender and month and year of birth of each participant. This step confirmed that data for the correct patient were returned. Responses were automatically checked against the details held in the CADDY administrative database. If validation details did not match, the electronic CRF prompted the data entry person to check they were using the correct patient record. For paper CRFs, any inconsistency prompted a telephone call to the practice to resolve the discrepancy. If inconsistencies could not be resolved through discussion, data were accepted as correct if two of the three validation details were correct.
Official date of death statistics were used as an indicator of the accuracy of data entry. When practices provided a date of death which was within 2 months of the official date, the record was considered accurate. Two months was chosen as the threshold as it is recognised that dates of death recorded in GP notes are often approximate.
Chapter 5 Definition of variables
CADDY outcome measures
Ascertainment and coding of dementia diagnosis
For each CADDY participant, we received primary care data on the presence or absence of (1) dementia diagnoses, (2) any concerns about cognitive function and (3) referrals to specialist services following cognitive concerns (see Appendix 2 for detail of the CRF). The earliest date and specific clinical code used for each clinical diagnosis, concern or referral were also recorded.
By comparing dates recorded in primary care with the dates of each participant’s CFAS assessments, we encoded the status of each participant at the date of their W1 and W2 assessment into one of four mutually exclusive categories as described as follows:
-
diagnosed (clinical diagnosis) on the interview date if the GP had recorded a clinical diagnosis of dementia before that date
-
referred if no clinical diagnosis had been made but a referral to a specialist service following a cognitive concern had been noted before the interview date, irrespective of whether the patient had attended or the referral result had been noted
-
concern if there was any record of a cognitive concern before the date of interview, but without a clinical diagnosis or referral having been made
-
unknown if there was no report of any cognitive concern, referral or clinical diagnosis up to the interview date (note that unknown is not a missing category but means that the practice reported no cognitive concerns up to the corresponding CFAS assessment date).
Dementia status
Each participant is defined as living with dementia or not having dementia at each wave, as defined by the CFAS II classification. So, based on this and the definition of diagnosis status above, we classify people at each wave as having:
-
diagnosed dementia if they meet the CFAS II criteria for dementia and have a record of a dementia diagnosis in their GP notes on or before the interview date, or
-
undiagnosed dementia if they meet the CFAS II criteria for dementia but have no record of a dementia diagnosis on or before the interview date.
Note that this definition treats the CFAS II diagnosis as a gold standard, against which we are comparing the accuracy of GP diagnoses. Although the CFAS II study diagnosis has been validated against the DSM-IIIR diagnosis of dementia, we acknowledge that it may not be sensitive, that is there are likely to be people with dementia who are not classified as such. However, the CFAS II diagnosis is thought to be specific and to have a high positive predictive value, in that those reported as having dementia using this algorithm are very likely to have dementia. Hence, it is well suited to the purpose of identifying a cohort of people living with dementia. The CFAS study assessment is also administered in a standardised way across all participants and so will not introduce a bias when estimating the effects of factors potentially associated with diagnosis.
Selection and coding of covariates
CFAS II assessed many social, demographic, clinical and health service use factors for each participant. Many were included as potential predictors or consequences of dementia diagnosis. These were selected based on a review of previous literature, theoretical considerations and discussions with PPI advisory groups. Data collection methods are fully described in Chapter 3, CFAS II design.
Sociodemographic factors
Date of birth, sex and years of completed education were ascertained or confirmed at the baseline interview. Education was coded into three groups: (1) < 10 years of formal education, (2) 10 years of formal education and (3) > 10 years of formal education.
Cambridge Cognitive Examination
The Cambridge Cognitive Examination (CAMCOG)35 is a more comprehensive assessment than the short cognitive assessment that has been in widespread use throughout the NHS until relatively recently, the Mini Mental State Examination (MMSE). 36
The CAMCOG has a range of total scores on a 0–103 scale and has subscores in 10 cognitive subdomains. These are:
-
orientation
-
language (comprehension)
-
language (expression)
-
memory (remote)
-
memory (recent)
-
memory (learning)
-
attention and calculation
-
praxis
-
abstract thinking
-
perception.
The CAMCOG was administered as part of the total interview to as many participants as possible, but if the interviewer considered that the participant might refuse to continue the interview, or the participant failed early items in the questionnaire on orientation, a shorter ‘emergency’ assessment was conducted, in which the MMSE but not the CAMCOG was administered.
Any missed questions were coded as failed for the purpose of this study, if the participant attempted any of the CAMCOG questions. Twenty-five CADDY sample participants did not attempt any of the CAMCOG assessments, in which case all subscales and the total score are coded as missing.
A CAMCOG score of 80 has been reported as the optimal threshold for identifying people with dementia,37 whereas CAMCOG scores of 60, 40 and 30 are typical of minimal, mild and moderate/severe dementia, respectively. 38
Memory impairment (informant report only)
The informant is asked whether or not the participant has any difficulty with memory (in general) and then specifically with (1) remembering short lists of items when shopping, (2) remembering what happened yesterday, (3) remembering where they put things or (4) remembering the names of people. All positive responses to any of these questions are summed, leading to a score of 1–5.
Changes in personality (informant report only)
This is determined from responses to the binary question ‘Have you noticed any changes in his/her personality, such as the way she/he behaves socially?’.
Changes in behaviour (informant report only)
This is determined from the answers to two questions on whether or not behaviour has changed and participants’ awareness of their behaviour. Responses to each question about change in a specific behaviour were scored as none (0), mild (1) or severe (2). Values were based on the maximum reported score for either question.
Problem with eating or drinking
This is defined as any report of eating unusual things, overeating or any problem that the participant experiences with physically feeding themselves.
Incontinence
This is a binary variable corresponding to any report from the participant or informant of difficulty with getting to the toilet or with bladder control.
Sleep disturbance
This is recorded if either the participant or the informant reports any sleep disturbance, which could include difficulty sleeping/getting to sleep or waking early.
Communication difficulty (carer’s report only)
This is defined as any mention of a problem with communicating. We used five questions with binary responses on word-finding and talkativeness, and three questions on repeating words, which were added to produce a single score (0–5) of communication difficulties.
Blessed Dementia Scale
The Blessed Dementia Scale (BDS)39 was developed as a screening instrument for the detection of mild cognitive impairment and dementia, but is also used as a measure of severity of functional impairments associated with dementia. CFAS uses a modified version of the BDS, including 11 questions on the performance of household tasks, managing money, navigating indoors and outdoors, remembering lists, interpreting surroundings, recall of recent events, dwelling in the past, difficultly eating, dressing and continence. The BDS is administered at the informant interview, and so reflects both the severity of dementia and the informant’s knowledge of these symptoms of dementia in the respondent. It is scored from 0 (not impaired) to 17 (extremely impaired). The BDS cannot be administered in the case of participants with no informant interview. In CADDY, the BDS was used for generating weights, as elements of the scale are included in other predictor variables.
Inpatient hospital stays
The CFAS II does not specifically ask about unplanned hospital admissions, but does ascertain at each wave the number of separate inpatient stays in the year before the assessment, and the number of nights for up to seven visits. These are reported either by the participants themselves or by a proxy.
Anxiety and depression
Anxiety and depression were both coded based on interview data according to the Automated Geriatric Examination for Computer Assisted Taxonomy (AGECAT) algorithm. 40 Both were coded at each assessment as being absent ‘none’, ‘subthreshold’ or ‘case level’, similar to previous analyses using the MRC CFAS. 41
Sensory impairment
Visual impairment was dichotomised from participant report and interviewer observations as the presence or absence of any degree of visual impairment that interferes with reading, writing or drawing after correction with glasses.
Hearing impairment was dichotomised from the interviewer’s subjective assessment as presence or absence of a hearing impairment that interferes with the questioning or from the informant report of whether or not the respondent wears a hearing aid. A positive response to either question was considered to indicate a hearing impairment.
Social participation
Participant’s or proxy’s report of the number of activities they participate in, from the following list:
-
political parties
-
trade unions
-
environmental groups
-
tenants’ or residents’ groups
-
Neighbourhood Watch
-
evening classes
-
University of the Third Age
-
other adult learning
-
arts, music or singing groups
-
charity, voluntary or community groups
-
groups for elderly (e.g. lunch club)
-
youth groups (e.g. Scouts/Guides/youth club)
-
Women’s Institute, Townswomen’s Guild
-
social clubs (Rotary, working men’s club)
-
sports clubs, gym, exercise/dance groups
-
other groups or organisations.
Social contact
This was determined from the participant’s or proxy’s report of the frequency of contact between the participant and friends or family and was coded as ‘daily’, ‘two or three times per week’, ‘weekly’, ‘monthly’, ‘less often’ or ‘never’. This variable is coded only for participants not in a residential care setting.
Comorbidity
Participants and informants were asked whether or not participants had even been diagnosed with any of the following comorbid health conditions:
-
heart attack
-
stroke
-
angina
-
high blood pressure
-
intermittent claudication
-
bronchitis
-
other respiratory disease
-
asthma
-
arthritis
-
pernicious anaemia
-
diabetes
-
thyroid problem.
Each comorbidity was coded as a binary variable, reflecting any prior diagnosis. The comorbidities were then summed into two variables: cardiovascular comorbidity (sum of conditions 1–5) and non-cardiovascular comorbidity (sum of conditions 6–12).
Chapter 6 Statistical analysis
Research question 1a: distribution of diagnosis among people with dementia
The distribution of the four levels of primary care diagnosis status among participants with dementia and the characteristics of the undiagnosed population were estimated separately for each wave, using inverse probability weights to correct for missing diagnosis status.
Each potential covariate was tabulated across each outcome group for all people with dementia, combining records from W1 and W2. Inverse probability weights were applied to estimate proportions (see Missing data).
Research question 1b: diagnosis among people without dementia
The number of people at W1 and W2 who did not have dementia (by the CFAS II criteria) but did have a diagnosis in their primary record was reported. The sample used for this research question included participants selected as control and participants selected because they had dementia at W2 but did not have dementia at W1. For people without dementia but with a diagnosis at W1, their W2 dementia status was also described. Numbers were very small and no further analysis was conducted with this group.
Research question 2: correlates of diagnosis
Estimating predictors of diagnosis
The distribution of diagnosis status was described, stratified by levels of each covariate independently. We describe how the proportion of people with dementia who are diagnosed varies with levels of each potential predictor. Logistic regression was then used to create a multivariable model, estimating the independent contribution of each predictor when controlling for each of the others. Here, multiple imputation was used to account for missing data in both the covariates and the outcomes (see Missing data).
Predictors of individual elements of the diagnostic pathway
The final model for diagnosis was then broken down into two components: the probability of help-seeking and the probability of clinical diagnosis given help-seeking. That is, because we make the assumption that the probability of clinical diagnosis without help-seeking is zero:
where D represents diagnosis for an individual, H is help-seeking and X are the variables representing potential predictors of either help-seeking or diagnosis. The first term on the right-hand side is the probability of clinical diagnosis given help-seeking and predictors, whereas the second term refers to the probability of help-seeking. A logistic regression model for each term was estimated separately using the same set of potential predictors as for the full model of overall probability of diagnosis.
Research question 3: the time to diagnosis
To estimate the average time between dementia incidence and clinical diagnosis, a time-to-event analysis was performed. The analysis was restricted to patients who had developed dementia between W1 and W2 and therefore for whom an approximate date of onset was known. Patients were entered into the analysis 4 years prior to the date of their W2 interview, and were included until the earliest of death, new diagnosis, or being censored on the date that their practice submitted data to CADDY. The timescale used was years since entry into the analysis. The cumulative incidence of new diagnosis, and hence the median time to diagnosis (time at which 50% of cases had been diagnosed) was then estimated, with death prior to diagnosis considered as a competing risk.
To estimate predictors of new clinical diagnosis among those undiagnosed, we defined an ‘index assessment’ as the first CFAS II assessment at which a participant met the study criteria for dementia. In the case of patients who were undiagnosed at their index assessment, Cox proportional hazards regression was used to estimate predictors of new diagnosis over the subsequent 2 years, using the same set of covariates as for research question 2 (see Research question 2: correlates of diagnosis).
Research question 4: the consequences of diagnosis
To estimate the effect of diagnosis on people with dementia (compared with remaining undiagnosed), we selected outcomes that reflected hypothesised benefits or harms of clinical diagnosis based on previous literature and discussions with carers of people with dementia. These were mortality, anxiety, health-related worry, social contact, new institutional placement and stays in hospital.
Effect of diagnosis on mortality
Mortality was estimated using a time-to-event analysis, with participants deemed ‘at risk’ from the date of the first assessment at which they received a study diagnosis of dementia, and being followed up until death or until a censoring date of 6 years following the index assessment. In this analysis, a participant’s diagnosis status was fixed as their clinical diagnosis status on their index assessment date. We did not consider diagnosis status as a time-varying exposure, as we could not measure confounding factors accompanying any change in diagnosis status, and it is possible that diagnosis after the interview date is associated with unmeasurable changes in health, which would also be linked to mortality.
Effect of diagnosis on mood and social contact
Three potential psychosocial impacts of dementia diagnosis were measurable using the CFAS II assessments: generalised anxiety, health-related worry and the number of social activities undertaken by the participant. To estimate the effect of diagnosis on each of these three measures, we used data from any CADDY participant who had taken part in both a W1 and a W2 CFAS interview, and we measured the changes in each of the three aspects between W1 and W2, stratified by diagnosis status. Participants were divided into three groups: (1) those who were not diagnosed and remained undiagnosed, (2) those who were undiagnosed at W1 but were diagnosed by W2 and (3) those who were diagnosed at W1.
For each outcome, patients were further classified into three groups corresponding to no change, improvement or worsening. The effect of clinical diagnosis status on outcome status was measured by a descriptive analysis and by multinomial logistic regression comparing each outcome across groups, adjusted for cognitive function, age, sex and living arrangement. We also described the distribution of each outcome at W1 and W2 for all participants who completed both W1 and W2 assessments, stratified by clinical diagnosis status.
Effect of diagnosis on hospital admission and move to residential care setting
To test whether or not diagnosis delayed transfer to a residential care setting from a participant’s own home, we used multivariable logistic regression. We estimated the relationship between diagnosis status at W1 and new institutional placement between W1 and W2 among those living at home at W1, adjusting for potential covariates. To estimate how diagnosis affects the rate of hospital admissions, we used Poisson regression to model the number of admissions and the total nights in hospital in the year before W2 regressed on W1 primary care diagnosis status and potential covariates.
To avoid reverse causation, we did not consider new diagnoses between W1 and W2 in this analysis. The inclusion of new diagnoses between W1 and W2 would probably have resulted in the inclusion of diagnoses that were made after or as a result of the hospitalisation or institutional placement. Hence, the comparison made is between diagnosis status at W1 and the change in residential status or hospital admissions between W1 and W2.
Common issues for all analyses
Representativeness of the sample
The CFAS II participants were stratified by age at recruitment. Within those strata, a random sample from primary care lists was drawn, and so the age, sex and postcode of those who did and did not participate is known. Based on this, an inverse probability weight was calculated such that when applied the CFAS II sample is representative of its target population.
People with more severe cognitive impairment were less likely to have been consented to link their medical records, and hence were less likely to appear in the CADDY. A CADDY-specific weight at W1 and W2 was generated using a logistic regression model estimated in all CFAS participants with dementia, with participation in CADDY as the outcome and with age, sex, dementia severity, living arrangement and geographic centre of each participant as predictors. For weighting, dementia severity was measured by the BDS, as this is assessed in all cases irrespective of patient capacity. The BDS is not measured for those without an informant interview and, so, for weighting purposes, these participants are assigned to a ‘missing severity’ group.
Dimension reduction for covariates
The potential list of covariates is too long for each to be included in analyses independently. Following theoretical and empirical correlations, groups of covariates were combined into composites as described below.
Objective measures of cognitive function
Cognitive function was measured using the CAMCOG scale. This includes subscores on 10 different domains of cognitive function. Missing items are coded as 0 if the participant completed at least some of the assessment. When none of the CAMCOG is completed, all subscales are recorded as missing.
An exploratory factor analysis was performed on untransformed scores to test whether or not these scores could reflect fewer subdomains of cognitive function. This analysis revealed a single dominant factor reflecting overall dementia severity. As our aim was to understand the impact of different domains of cognitive function on clinical diagnosis, a one-factor solution would not be helpful and so we estimated a two-factor solution. The second factor reflected the difference between impairments predominantly in memory and orientation compared with impairments in other domains of cognitive function. Several different algorithms and rotations were applied, but this factor structure was consistent, suggesting that it is not an artefact of any particular analysis. When a varimax rotation was applied, this factor structure comprised one factor reflecting impairments in memory and orientation and another reflecting impairments in other CAMCOG domains.
For ease of interpretation, instead of using factors scores in analysis, we created two variables: one the sum of the ‘orientation’, ‘memory: learning’, ‘memory: recent’ and ‘memory: remote’ subscores, and the second including the sum of the remaining subscores. For regression analysis, each subscore was standardised to mean 0 and standard deviation (SD) 1 before summing, and then the composite ‘memory’ and ‘non-memory’ scores were themselves standardised to mean 0 and SD 1. The distribution of participants with respect to these two factors is shown in Figure 8.
Informant reports of cognitive and non-cognitive symptoms of dementia
Following the classification of objective memory impairment, we classified each of the informant-reported symptoms of dementia as being primarily memory or non-memory related, and created two variables corresponding to the number of memory or non-memory symptoms endorsed (Table 1).
Memory symptom | Non-memory symptom |
---|---|
Trouble remembering short shopping list | Changes in personality |
Trouble remembering what happened yesterday | Changes in behaviour |
Trouble remembering people’s names | Incontinence |
Trouble remembering where they (participant) put things | Sleep disturbance |
Any memory difficulty | Loss of interest |
Loss of or excess appetite |
Missing data
Missing outcome variable
At W1, participants were asked to consent to medical record linkage only if they had capacity to do so personally. Figure 2 shows the link between measures of dementia severity and diagnosis data availability at each wave. Participants without consent for linkage had more severe dementia at W1 and were also the least likely to have been able to complete cognitive tests or directly answer other interview questions. However, those with severe dementia at W2 were often consented at W1, at which time they did have capacity and so are included in the study. We correct for cases without consent, either through the use of multiple imputation or by the use of inverse probability weights, depending on the analysis (the generation of weights is discussed in Chapters 7–11 and multiple imputation is discussed in Multiple imputation by chained equations).
FIGURE 2.
The proportion of people with dementia at CFAS II W1 and W2 for whom diagnosis data were available in CADDY, stratified by BDS quintile (higher value = more severe dementia). (a) W1 and (b) W2.
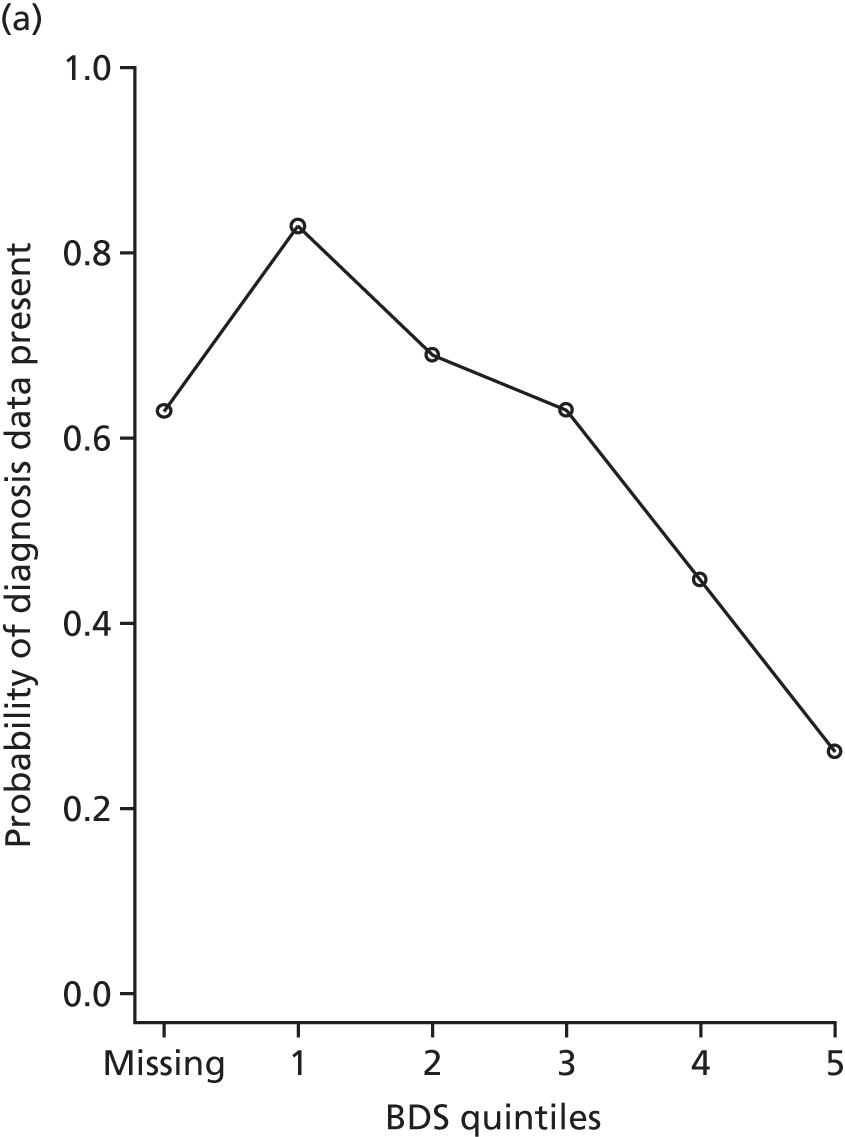
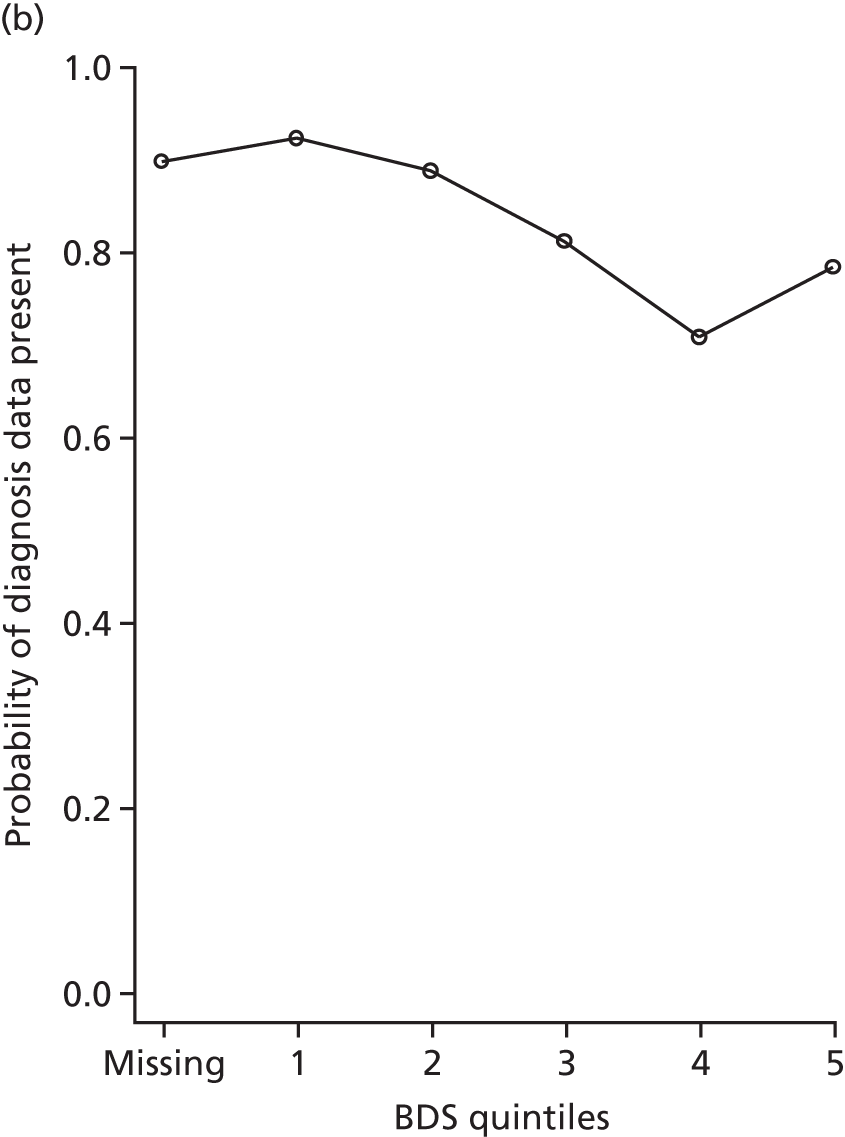
Missing predictor variables
Proxies were available to answer some but not all questions. Variables are typically missing when either (1) the respondent is not able to answer and there is no proxy or (2) the questions rely on an informant assessment and no informant exists or is available.
Multiple imputation by chained equations
Multiple imputation by chained equations (MICE) was used to infer the study diagnosis status and other covariate data when they were missing. 42 For continuous, binary and ordinal variables, predictive mean matching was used to impute new values; for nominal values with more than two levels, multiple logistic regression models were used. In short, MICE works by imputing missing cases of each variable in turn using a regression model based on all other variables in the planned analysis, including the outcome. When predictive mean matching is used, the regression model is applied to all individuals and for each missing value an imputed value is selected from the complete cases with similar fitted values. When multinomial regression is used, imputed values are sampled directly using the predicted probabilities from the model. MICE allows imputed values for predictors to be used and iterates imputations on each variable a fixed number of times before imputed values are recorded for analysis. In this way, any pattern of missing data, including the primary outcome of interest, can be imputed.
For imputation models, data from W1 and W2 are combined. In practice, this means that the study diagnosis status among those not able to consent at W1 is imputed based on the relationship between diagnosis status and other predictor variables at W2 and among W1 cases when consent was taken. As patients with severe dementia at W2 were able to give consent to medical record linkage on recruitment to the study, this means that the imputation model for diagnosis study in severe dementia can be estimated and applied to W1 participants. The number of imputed cases is specified alongside each analysis. Only data for those who are alive and participated in the relevant CFAS II wave are imputed.
Clustering
Eighty-five participants received study diagnoses of dementia and were included in both W1 and W2. In all inferential analyses (regression models) in which waves are combined, we corrected for this using robust standard errors to account for within-participant correlation, by specifying the ‘cluster’ option in Stata® 14.1 (StataCorp LP, College Station, TX, USA). 43
Software
All analysis was conducted using Stata 14.1.
Chapter 7 Results: data collection
Primary care data collection
Our research relied heavily on persuading GP practices to extract and supply data from patient records. This was successful, but at considerable cost in terms of researcher time, and was dependent on building and maintaining good relationships with our contributing practices. The remainder of this chapter is dedicated to a quantitative and qualitative description of the reality of the process of collecting data from primary care, along with insights and suggestions arising from our experience that should be of help to future researchers undertaking similar tasks.
Engagement with primary care practice staff members
The primary contact person at practices was most often the practice manager. Direct contact with GPs was rare. The majority of practices expressed a desire for electronic communication, but several appeared unfamiliar with this, and some had reservations about using the weblink for data upload. Practices were keenly aware of their responsibilities surrounding data security.
Most practices were willing to participate, but some had reservations about their capacity to undertake any extra work. Of the 88 practices contacted, five initially declined to take part, but four of those five subsequently agreed to take part with CRN support for data extraction. The remaining practice was a single-handed GP who declined both CRN support and the invitation to take part because of workload. He was not recontacted.
Data were returned from practices by many different types of staff, but most commonly by practice managers and GPs. CRN facilitators reported difficulty in extracting the required data from large and complex paper records provided by PCSE.
Most practices returned data via the electronic form, with only five practices returning forms by post.
Clinical Research Network facilitators collected data at 12 practices, as well as extracting any records provided by PCSE.
Frequency and timing of contacts needed to obtain data
It was difficult to maintain regular contact with practices, as telephone calls were frequently queued for prolonged periods. We made 1079 reminder telephone calls and sent 69 reminder e-mails to practices; 597 of the telephone calls reached a contact at the practice and 214 telephone calls reached the primary contact. Primary contacts received, on average, 2.5 reminders (range 0–8 reminders).
Potential barriers to data collection and their resolution
Good communication with practices allowed us to identify a number of barriers to return of data and implement ways to overcome these:
-
The main barrier was lack of capacity for anticipated additional work. For example, during preparations for Care Quality Commission inspection, there was no capacity for any member of the practice staff to carry out any work for CADDY. In these cases, we negotiated discussions after the Care Quality Commission inspection at a date and time selected by the contact.
-
At another practice, on request for some missing CRFs, we identified a misunderstanding: the GP had mistakenly understood that we were looking for records only of people with dementia and so the records for patients we had identified but who had no recorded clinical diagnosis of dementia were not returned. Further explanation of the aims of the study was sufficient to resolve this issue.
-
In other cases, contacts were not confident about using the web-based form. Researchers booked time with practices to walk them through the process step by step. Once the first form had been completed, practices were usually quick to complete the remaining CRFs.
-
In most cases, the primary contact person had not been involved in CFAS II in the period 2008–11, and many requested copies of the signed consent forms for the original study.
Validation of records
Automatic validation identified 12 instances of non-matching validation data. Most were inadvertent entry of incorrect data and all were successfully resolved. In only one case (< 0.2%) was a true discrepancy between the administrative database and the primary care record identified: a GP was unable to enter the records for one patient whose year of birth in the administrative database did not match that in the patient record. The discrepancy was 1 year and other details matched. All validation details for 28 other records for this practice matched. We accepted that there was true discrepancy between the records. The form was re-sent and the data entered by the GP.
Further validation was carried out comparing date of death data from the ONS (supplied to CADDY investigators as part of the CFAS data set) with dates of death supplied by GPs. ONS data on date of death were available for 356 out of 598 patients (60%), of whom 277 (78%) were reported by practices as deceased, with date of death provided for 272 of these patients (76%). In 211 cases, there was no discrepancy between the GP-recorded and ONS-reported dates of death. For a further 50 patients, there was a discrepancy of between 1 and 60 days (median 3 days, mean 7.4 days); dates of death reported by practices for the remaining 11 patients did not closely match ONS data. Of these, the date of death for five (1.8%) patients matched those provided by the ONS in all but one digit (possibly reflecting typographical error), whereas dates of death for six (2.2%) patients did not closely reflect ONS data. However, owing to the high concordance in date of birth, gender and NHS number data for these patients, no participants were excluded based on any validation results.
Data flow
The CFAS II participants were initially recruited at 105 general practices. However, 14 of the original practices had merged into other participating practices or health centres, one practice was in the process of merging and two practices had since been closed. Patient lists for closed practices had been distributed among other local practices, some of which were CFAS II practices. We therefore worked with 88 practices. Details of practice participation by region are tabulated in Table 2.
CFAS centre | Participants for whom data sought, n | Participants per practice, mean (range) | Returned via practice, n | Returned via CRN, n | Returned via PCSE, n | Not returned, n |
---|---|---|---|---|---|---|
Cambridgeshire | 170 | 14.2 (1–40) | 116 | 37 | 17 | 0 |
Nottingham | 199 | 3.8 (1–16) | 159 | 9 | 8 | 23 |
Newcastle | 229 | 9.2 (1–21) | 136 | 80 | 5 | 8 |
All regions | 598 | 5.7 (0–40) | 411 | 126 | 30 | 31 |
Participant data collection statistics
Participant data were returned by general practice staff (n = 411), CRN representatives at general practices that did not have capacity to participate (n = 126) or in the form of PCSE-returned hard-copy records (n = 30). Records for 31 patients were not returned.
Consent to link medical records and primary care response rate among people with dementia
Of 7762 participants in CFAS II, 461 met the study criteria for dementia at the W1 assessment. Of those, 285 (62%) gave consent to have their medical records accessed by the study team and linked to study data. At W2, 5265 participants remained in the study, of whom 274 were assessed to have dementia at the W2 assessment, and of this number 249 (91%) had given consent to link their medical records.
Table 3 shows the breakdown of diagnosis status at the time of the W1 and W2 interviews for each study participant who took part in assessment at that wave, as well as the number with dementia in each wave who could not be consented for medical record linkage or for whom primary care data were not available.
Primary care diagnosis status at time of respective CFAS interview | W1 CADDY sample | W2 CADDY sample | ||
---|---|---|---|---|
NDa | D | NDa | D | |
Status | ||||
Unknown (no record of cognitive concern in primary care record) | 240 | 130 | 69 | 90 |
Concern | 24 | 31 | – | 26 |
Referral in progress | 10 | 22 | – | 23 |
Diagnosed | 22 | 86 | – | 93 |
Number with diagnosis data | 296 | 269 | 78 | 232 |
Missing data | ||||
Not consented | 9 | 176 | – | 25 |
Data not returned | 15 | 16 | – | 10 |
Censoredb | 2 | 0 | – | 7 |
Total including missing cases | 322 | 461 | 84 | 274 |
Primary care diagnosis status was received for 269 (94%) CFAS II participants with dementia who consented to linkage at W1, representing 58% of all those with dementia at W1. Data were received for 96% of those with dementia at W2 and consent to linkage, representing 87% of those with dementia at W2 (Table 3). Table 3 shows the breakdown of diagnosis status at the time of the W1 and W2 interviews for each study participant who took part in assessment at that wave, as well as the number with dementia in each wave who could not be consented for medical record linkage or for whom primary care data were not available.
At W1, those who gave consent were less severely functionally impaired. At W2, the link between dementia severity and consent to linkage was greatly reduced, as many participants with severe dementia at W2 had been able to consent at W1. There is an expected strong relationship between dementia severity and primary care diagnosis status at both W1 and W2. Previous studies have also shown that people with more severe dementia are more likely to be diagnosed, so missing more severe cases might cause the proportion with a diagnosis to be underestimated.
An inverse probability weight was generated at each wave using a logistic regression model to estimate the probability of inclusion in the data set, given the BDS score, age, sex, living in institutional care and the centre that each patient was recruited from. Modelled probability of participation matched the observed probability of participation well (Hosmer–Lemeshow goodness-of-fit tests: p = 0.96 for W1 and p = 0.52 for W2). At each wave, the weight was multiplied by the supplied CFAS II sampling weight and then each weight was bounded above at 20 to prevent any particular individuals from having an extreme influence on the study findings. The distribution of the W1 and W2 weights are shown in Figure 3.
FIGURE 3.
The distribution of inverse probability weights of participants with dementia at (a) W1 and (b) W2.
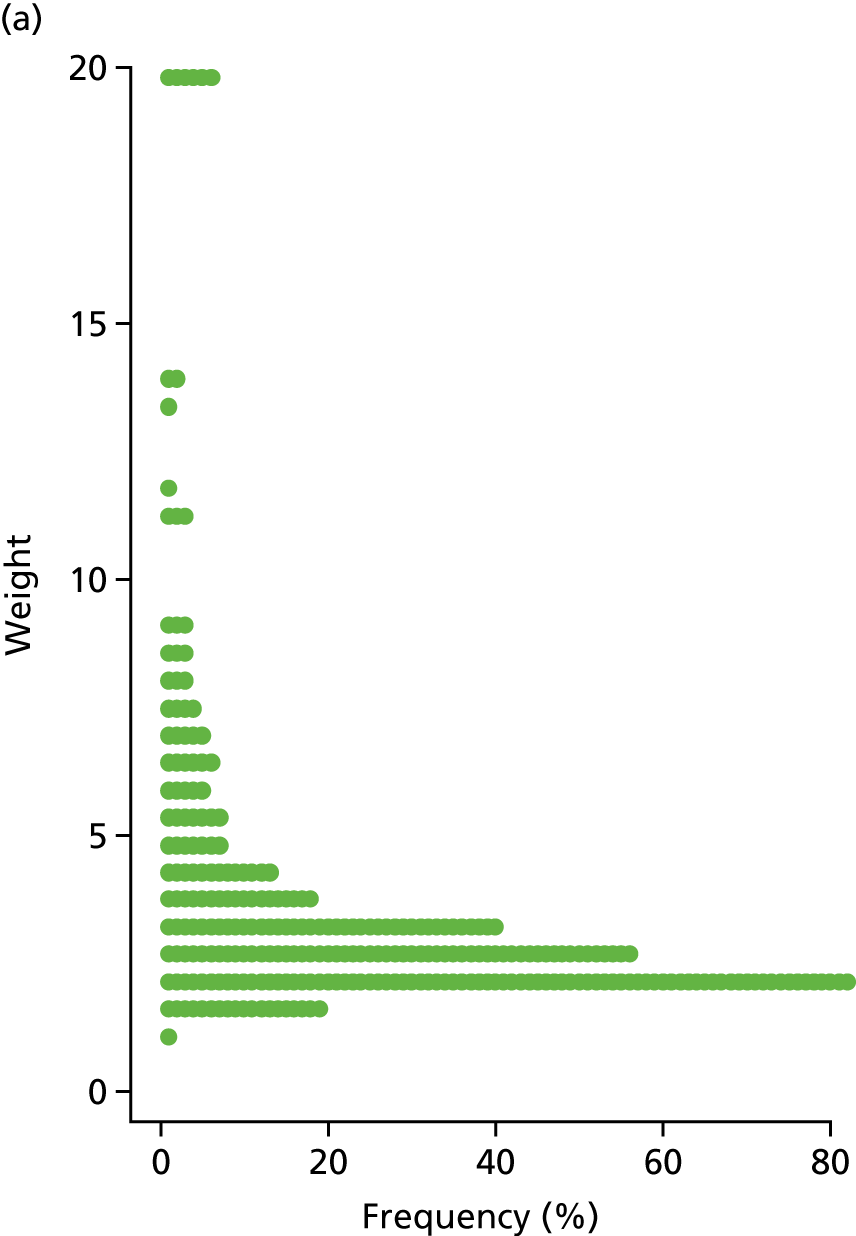
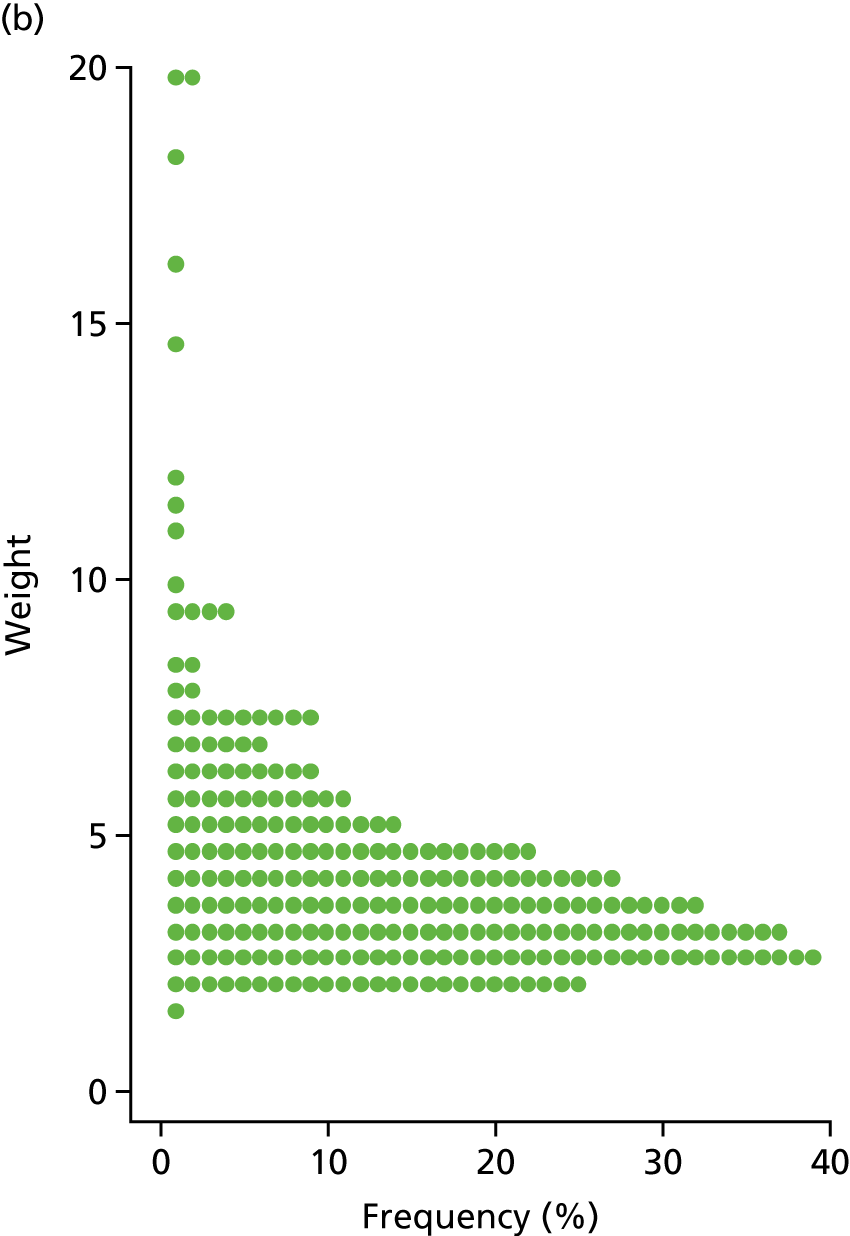
Figure 4 shows the flow of CADDY participants through the CFAS II, showing the numbers of dementia cases and those who consented to medical record linkage.
FIGURE 4.
Flow of CADDY participants through the CFAS II, showing the numbers of dementia cases and those who consented to medical record linkage. Not shown: three patients assessed to have dementia at W1 but not at W2.
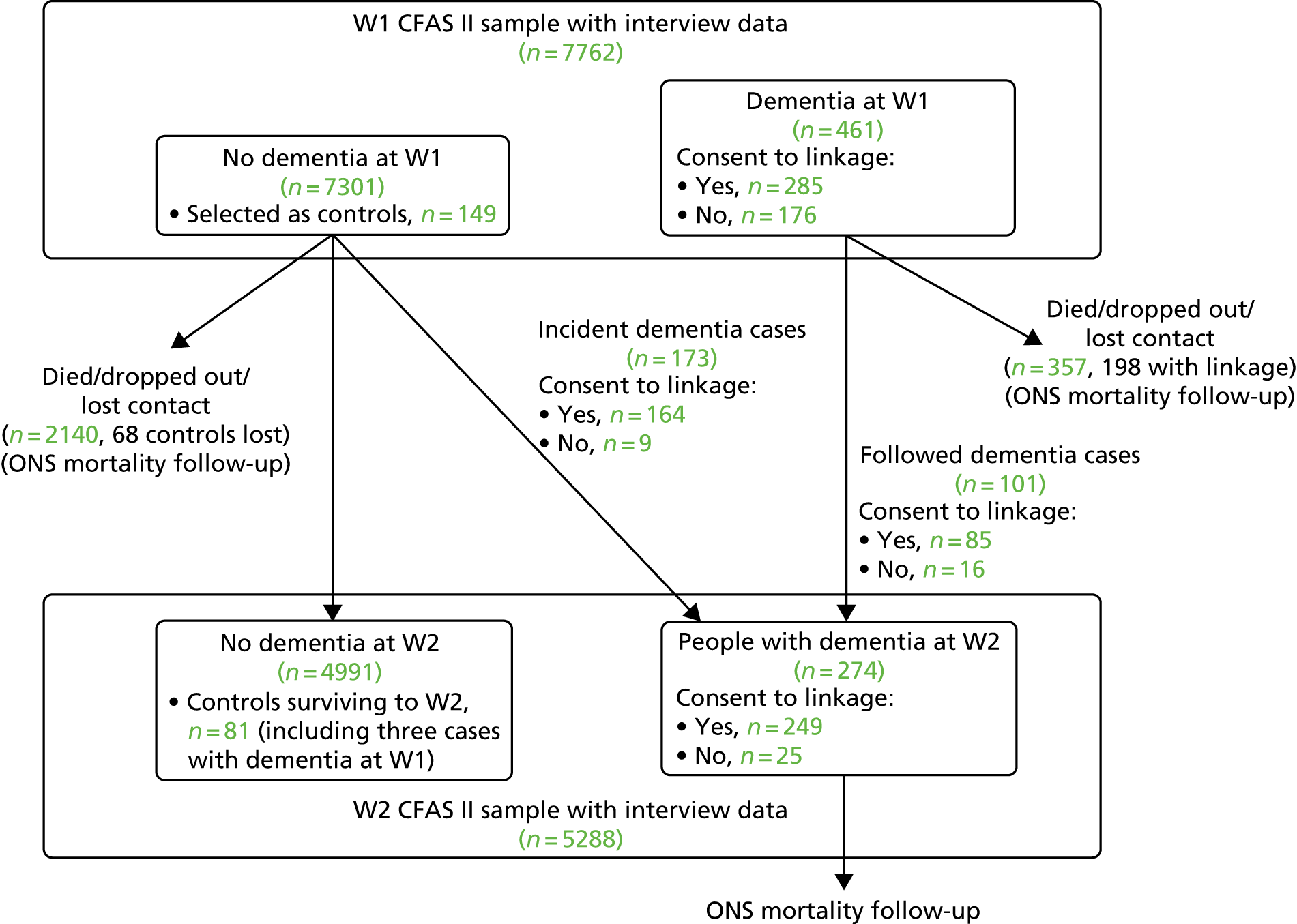
Chapter 8 Results (research question 1): prevalence of cognitive concerns, ongoing referral and diagnosis among people with dementia
Prevalence of diagnosis, concern or referral among people with dementia
Weighted estimates of the proportion of participants diagnosed, referred or with cognitive concern noted are shown in Table 4. The proportion diagnosed increased from 35% [95% confidence interval (CI) 28% to 43%] at W1 to 43% (95% CI 36% to 51%) at W2. At both waves, around 10% of the sample had been referred to specialist services but had not yet received a diagnosis of dementia, and 11% had a record of a cognitive concern being raised but had no subsequent referral or diagnosis. Hence, the proportion of the population with dementia whose GP had no record of any cognitive concern was 46% (95% CI 38% to 53%) at W1 and 35% (95% CI 29% to 42%) at W2.
Primary care-recorded diagnosis status | W1 | W2 | ||||
---|---|---|---|---|---|---|
n | Weighted proportion (%) | 95% CI | n | Weighted proportion (%) | 95% CI | |
Unknown | 130 | 45.6 | 38.1 to 53.3 | 90 | 35.2 | 28.6 to 42.3 |
Concern | 31 | 11.6 | 7.7 to 17.2 | 26 | 11.6 | 7.3 to 17.8 |
Referral | 22 | 8.0 | 4.5 to 13.9 | 23 | 10.1 | 6.4 to 15.6 |
Diagnosed | 86 | 34.8 | 27.6 to 42.6 | 93 | 43.2 | 35.9 to 50.8 |
Characteristics of the population with diagnosed versus undiagnosed dementia
The weighted distribution of demographic, social and clinical characteristics of participants with diagnosed compared with undiagnosed dementia for both waves combined is shown in Appendix 3, Table 11. Here, we discuss these numbers from the point of view of comparing the population that had a GP diagnosis with the population that did not. For a multivariable analysis of these data, we aimed to identify statistically significant independent effects of predictors of help-seeking and diagnosis (see Chapter 9). The following relates only to the group with a CFAS II diagnosis of dementia.
Demographic characteristics
Although the median age of the diagnosed group [median = 83 years, interquartile range (IQR) = 78–87 years] does not differ substantially from that of the ‘unknown’ group (median = 85 years, IQR = 79–89 years), it appears that the oldest age group (those aged ≥ 90 years) is proportionally under-represented among the ‘diagnosed’ and ‘referred’ groups compared with the ‘unknown’ and ‘concern’ groups. Likewise, it appears that there is a higher proportion of men in the ‘unknown’ group (40.2%) than in the ‘diagnosed’ (32.3%), ‘concern’ (20.3%) or ‘referred’ (36.7%) groups.
Although those in the unknown group are more likely than people with diagnosed dementia to be living alone (42.5% vs. 29.4%) and are less likely to be living with a long-term partner, a substantial proportion of each group, including the ‘unknown’ group (28.7%), are living in a care setting.
Clinical characteristics
The median cognitive function was considerably lower among those with diagnosed dementia (CAMCOG score: median 28, IQR = 1–54) than among the other three dementia groups (CAMCOG score for the ‘unknown’ group: median 55, IQR = 38–67). Nevertheless, more than 25% of those without a recorded cognitive concern have CAMCOG scores < 40 (including those with missing scores); this suggests a significant degree of impairment within the undiagnosed dementia groups (Figure 5).
FIGURE 5.
Cambridge Cognitive Examination (CAMCOG) scores among people with dementia stratified by GP diagnosis status, combining W1 and W2.
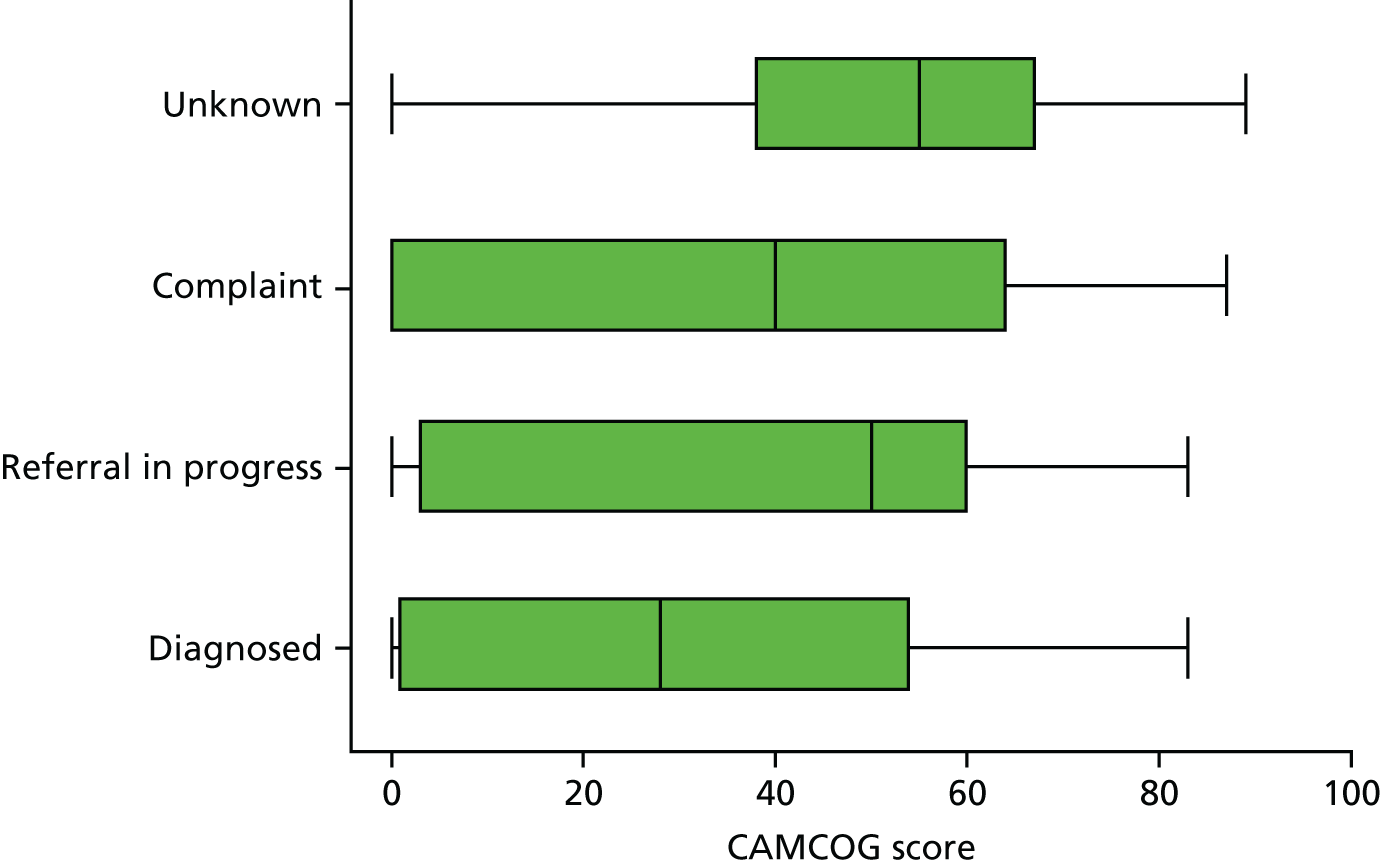
Similarly, informants for those with a diagnosis reported considerably more memory and non-memory symptoms than informants of the ‘unknown’ group, although, as with objectively measured cognitive impairment, a substantial proportion of informants (often carers) across all diagnosis groups endorsed most or all of the symptoms that were assessed. This indicates that symptoms are recognised by carers and are potentially problematic in many cases, even where no concern has been raised with a GP.
There was little difference in the levels of depression and anxiety across groups, but those with diagnosed dementia had substantially fewer diagnosed cardiovascular comorbidities than those in other groups.
Social factors
There was no substantial association between level of diagnosis status and frequency of social contact or number of social activities. Around 30% of each group were in institutional care, a further 30% were seeing friends or a member of their family at least daily and a further 20% at least two or three times per week, although around 10% of each group saw friends or family monthly or less often. Most people with dementia (60–70% across groups) reported no participation in structured social activity using our assessment, but this did not vary with diagnosis status.
Research question 1b: prevalence and characteristics of the cohort without dementia but with a dementia diagnosis
Of the 296 participants without dementia at the W1 interview, 22 had a GP-recorded diagnosis of dementia on or before that date. Of the 16 participants from this group who survived to W2, 14 met the CFAS criteria for dementia at W2, suggesting that in most such patients the GP had diagnosed dementia before the CFAS assessment and not that these patients constituted a substantial population with a misdiagnosis of dementia. Of the 78 participants without dementia at W2, three had a GP diagnosis of dementia recorded. There were no assessments beyond W2, so we do not know whether or not these participants would have met the study criteria for dementia at a later date, but two of the three had MMSE scores of ≤ 21 at W2, suggesting a substantial cognitive impairment, whereas one had MMSE score of 28. Hence, our study did not reveal a substantial number of GP diagnoses of dementia that might be considered false positive; we did not investigate this group further.
Chapter 9 Results (research question 2): independent predictors of diagnosis, concern and referral among people with dementia
Predictors of diagnosis of dementia in general practitioner records
Having demonstrated that the population with diagnosed dementia differs in several characteristics from the population with undiagnosed dementia, here we estimate the probability of diagnosis conditional on patient characteristics. We then develop multivariable models to establish which factors independently predict if a patient seeks help and the factors that predict diagnosis conditional on help-seeking.
Sample
The sample used is all participants with dementia at either W1 or W2. Each patient at each wave is considered as a separate observation, as outcome and covariate data can change between assessments. Participants who are censored because they left their practice before interview date are excluded, as are those who could not complete any element of the cognitive assessments; however, those without consent to link records or no data from GPs are included when missing data are imputed. The total number of potential observations is therefore 703 [461 people with dementia at W1, 274 people with dementia at W2 (with 173 participants contributing twice), excluding 25 observations with no cognitive data and seven censored observations] (see Figure 4 and Table 3).
Age, sex, educational attainment, area-level deprivation and living arrangement
Appendix 3, Table 10, shows primary care diagnosis status by levels of each potential predictor.
The description of the diagnosed versus undiagnosed population provided in Chapter 8 suggests that demographic factors, such as age, sex, socioeconomic status (as measured by educational attainment) and area-level deprivation and current living arrangement, might independently predict whether or not a person with dementia seeks and receives a diagnosis.
Figure 6 shows that people with dementia are most likely to have a primary care diagnosis if they live with a long-term partner or live in residential care, compared with those who live alone or with others (not spouse). Women are more likely to be diagnosed than men, and there is a relationship with age such that the youngest (aged 65–74 years) and oldest (aged ≥ 90 years) groups are less likely to be diagnosed than other age groups (aged 75–89 years).
FIGURE 6.
The proportion of people with dementia who are diagnosed, stratified by (a) sex; (b) age; (c) living arrangement; (d) area-level deprivation; (e) years in education; and (f) cognitive function measured by CAMCOG score. Error bars correspond to 95% CIs.
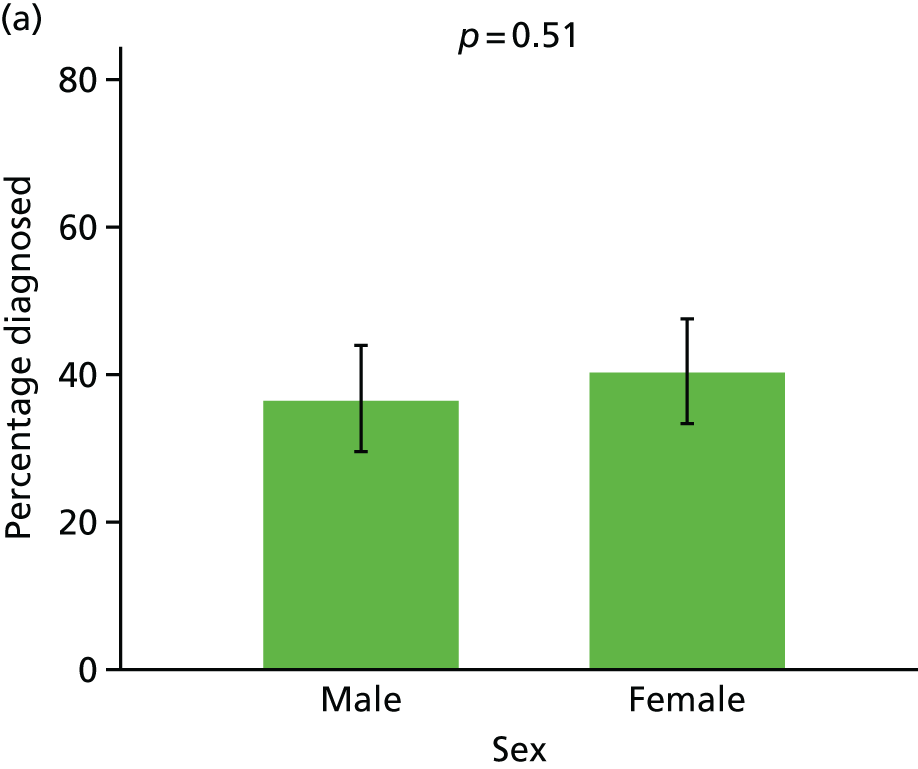
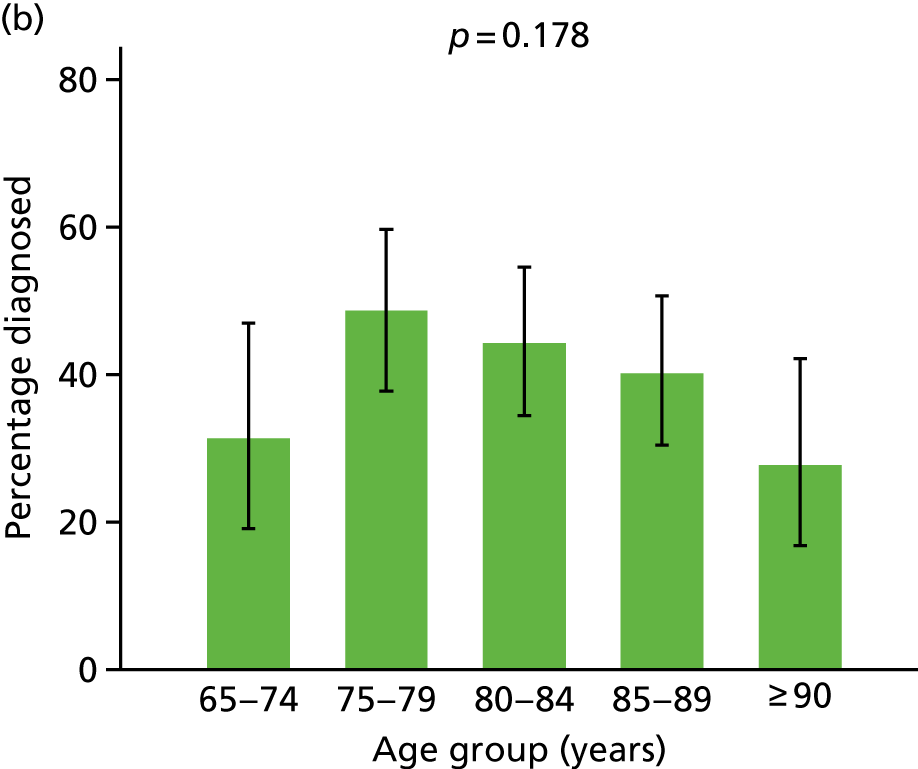
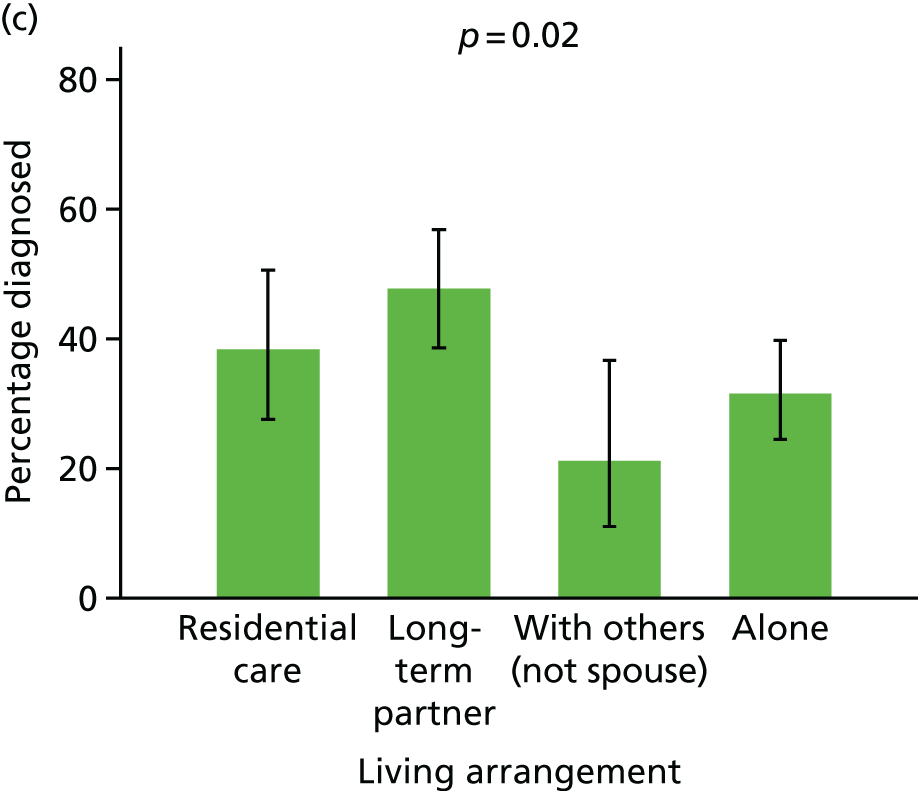
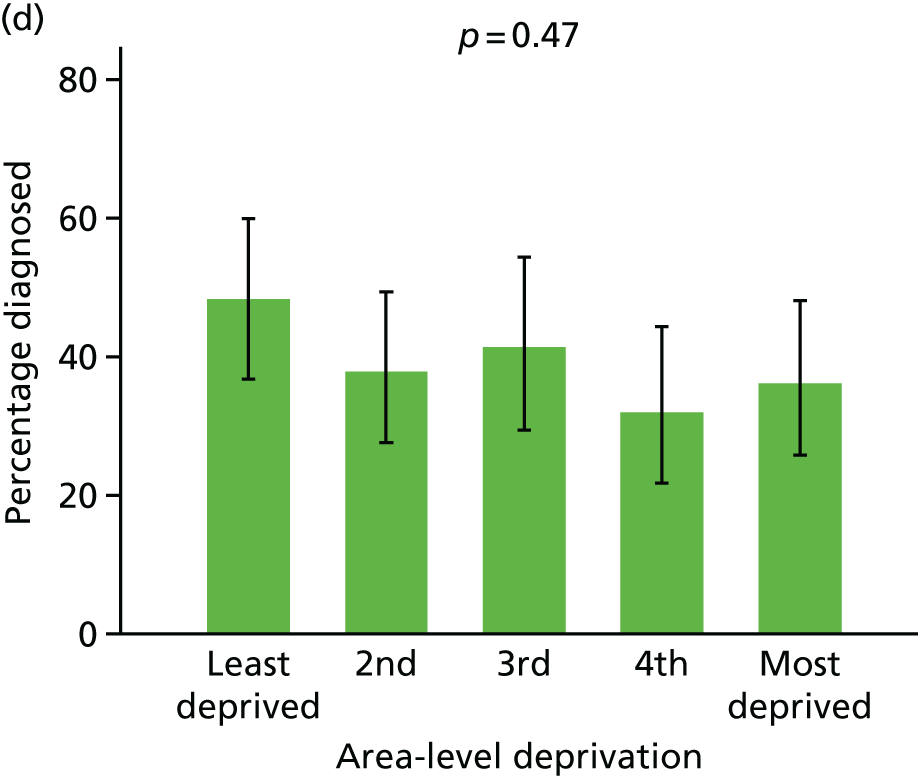
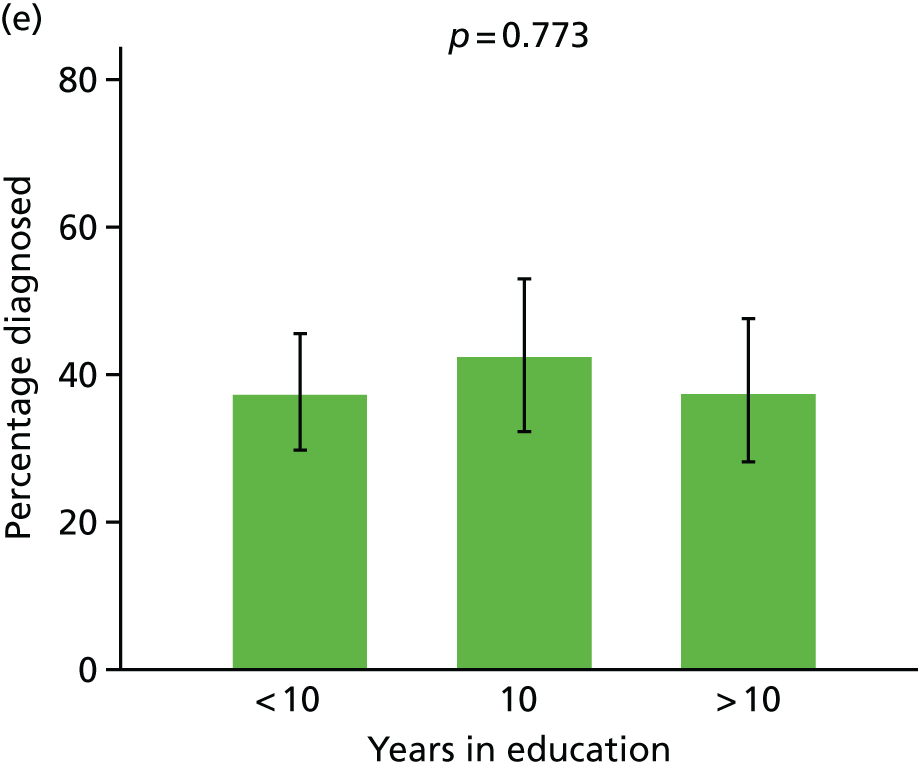
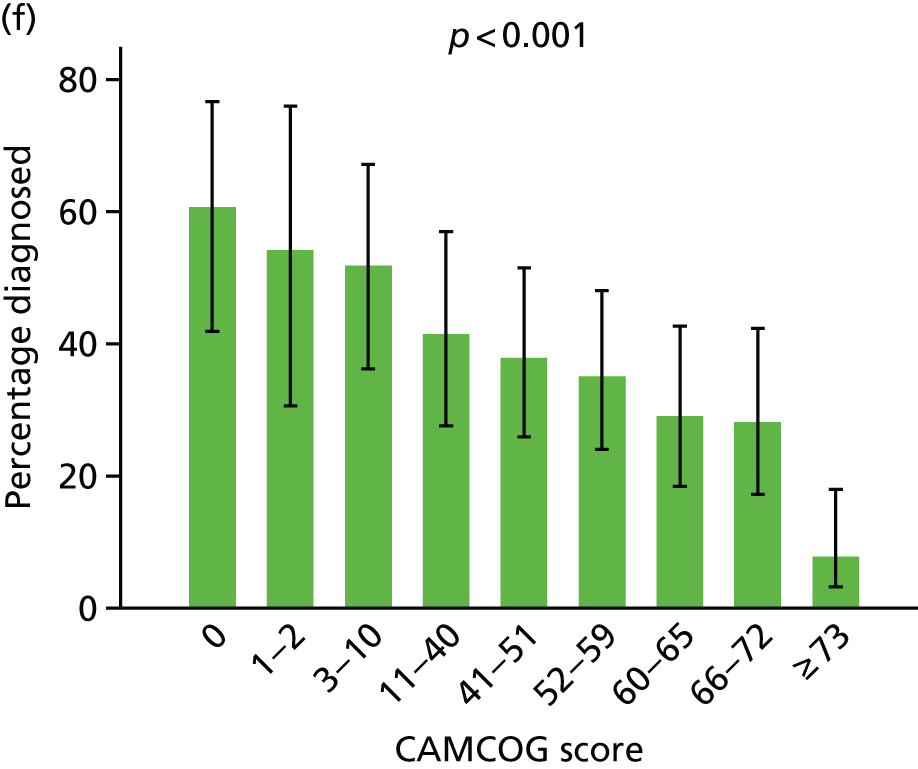
Cognitive function
The proportion of people with dementia who have a primary care diagnosis increases with poorer cognition as measured by CAMCOG score. Figure 7 clearly shows that people with more severe cognitive impairment are more likely to be diagnosed, with only 4 of 63 (6%) people with a CAMCOG score > 73 and 17 of 66 (25%) of those with a CAMCOG score between 66 and 72 being diagnosed. However, > 40% of people with the most severe cognitive impairment did not have a GP record of dementia.
FIGURE 7.
The proportion of people with dementia who are diagnosed, stratified by number of reported signs and symptoms. (a) Memory symptoms; (b) non-memory symptoms; (c) depression; (d) anxiety; (e) cardiovascular comorbidity; (f) other comorbidity; (g) frequency of social contact; and (h) number of social activities. Error bars correspond to 95% CIs.
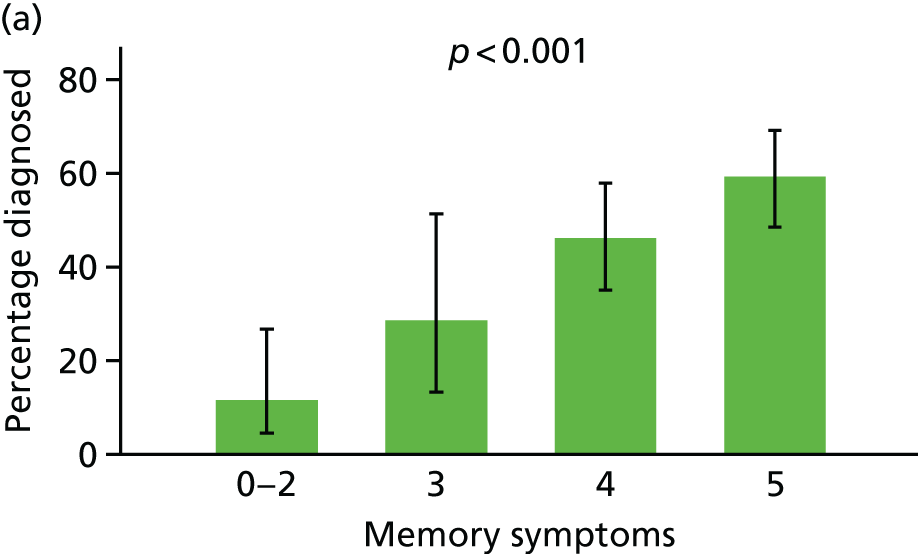
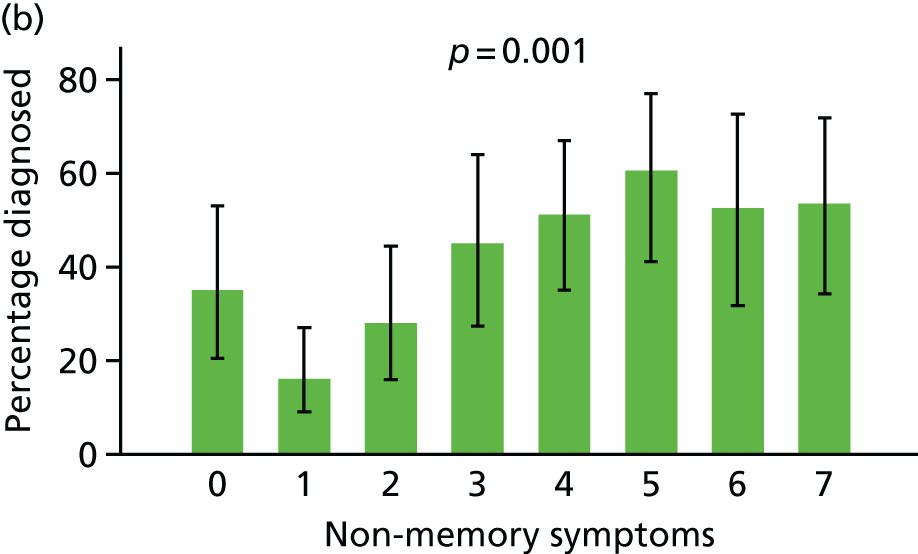
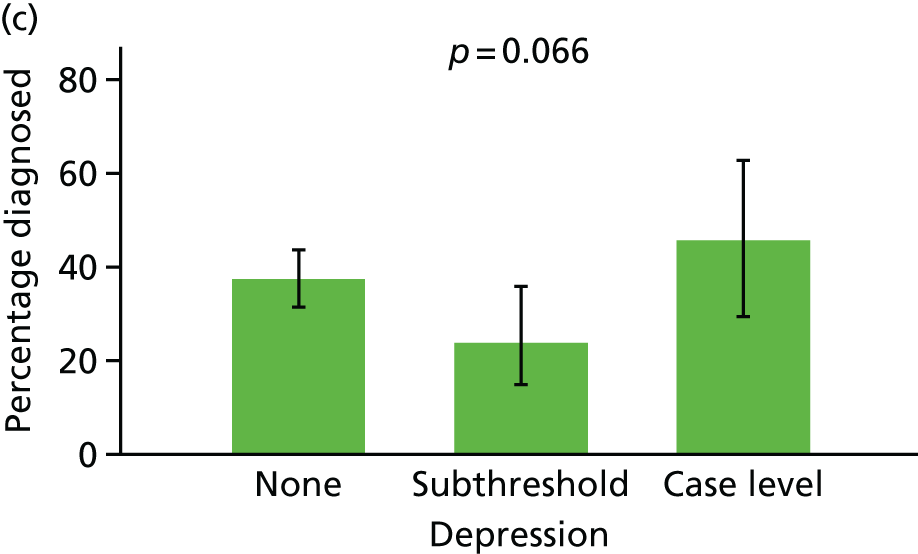
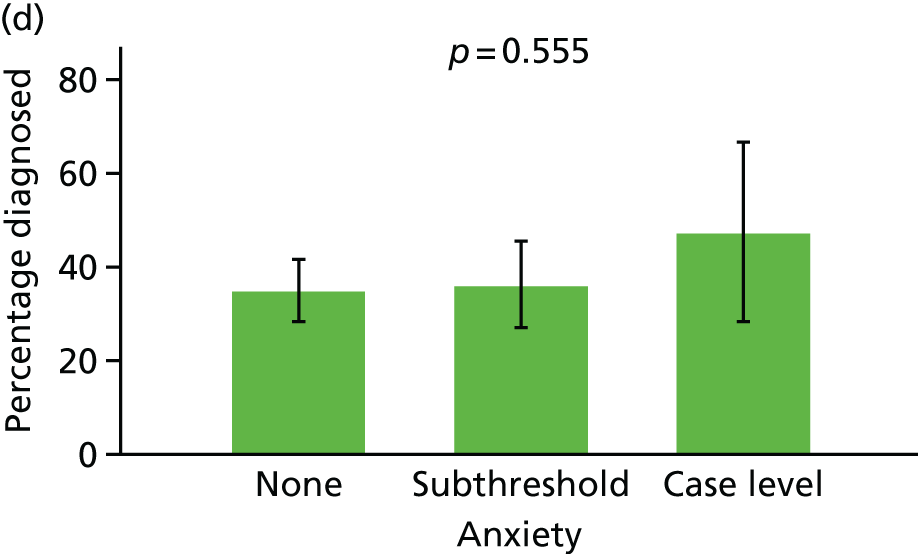
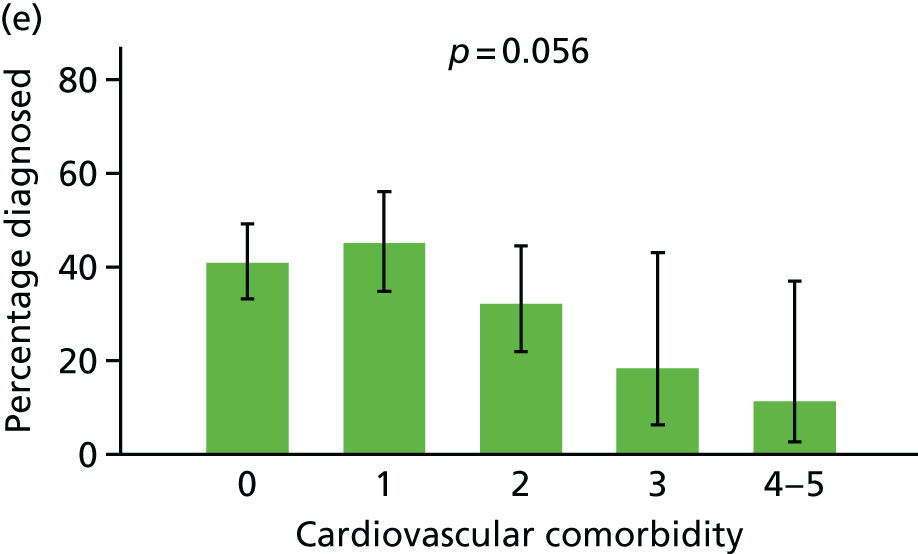
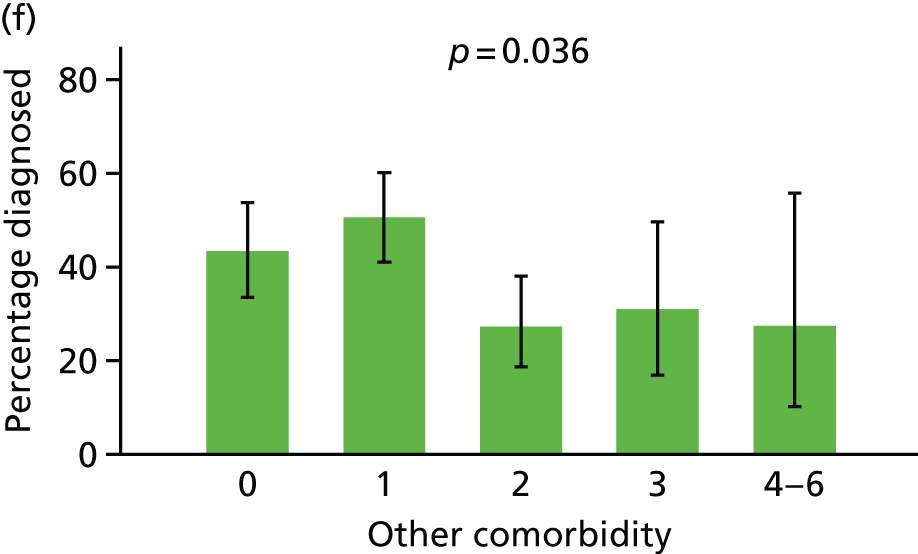
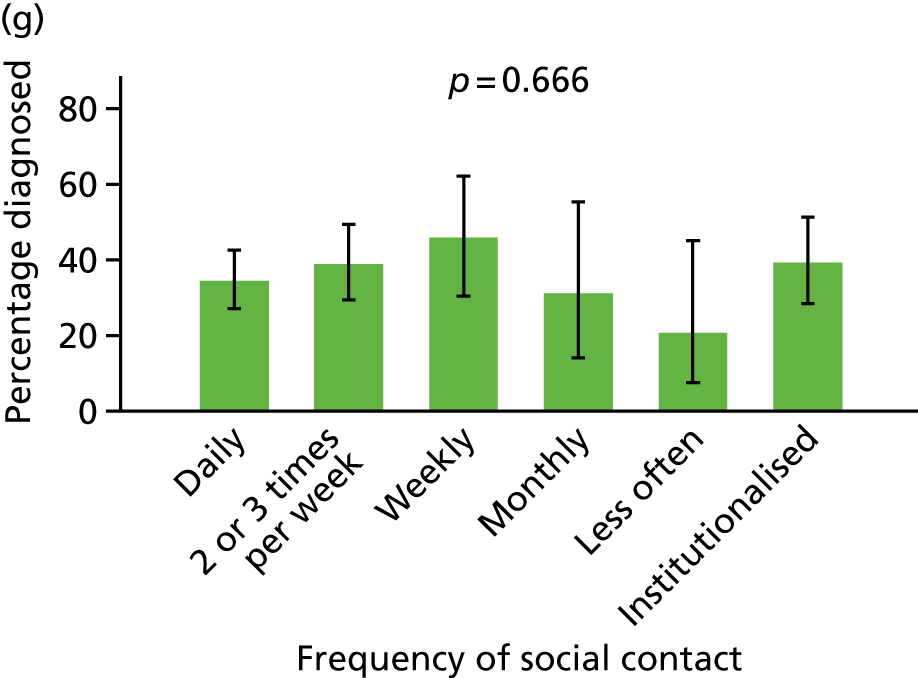
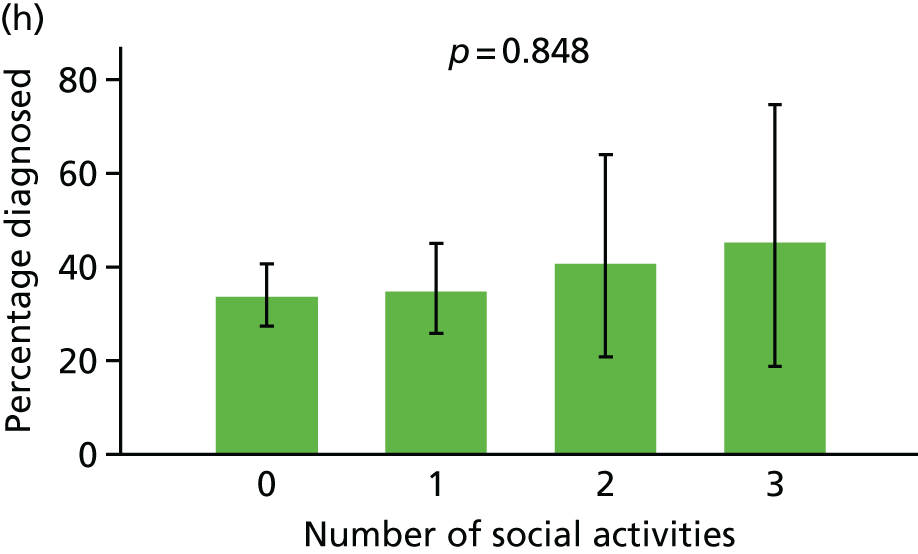
The relationship between subdomains of cognitive function, split into memory/orientation and other subdomains, and stratified by diagnosis status, is shown in Figure 8. Memory and non-memory scores are strongly associated, as would be expected. A logistic regression model was estimated to predict diagnosis based on the memory/orientation and non-memory domains, as well as the interaction effect between the two. This model shows that, once memory/orientation is taken into account, there is no further effect of cognitive function on the likelihood of having a primary care diagnosis and no significant interaction. This is illustrated by the contour lines overlying Figure 8, which indicate how the predicted probability of diagnosis under this model varies with both domains. The fact that the lines are broadly horizontal indicates that the probability of diagnosis is determined strongly by the level of impairment in memory and orientation, and not at all by other domains.
FIGURE 8.
Diagnosed and undiagnosed people with dementia in CFAS II, plotted with respect to their CAMCOG score in memory/orientation and non-memory/orientation domains. Contour lines indicate equal probability of diagnosis given the level of memory and non-memory function.
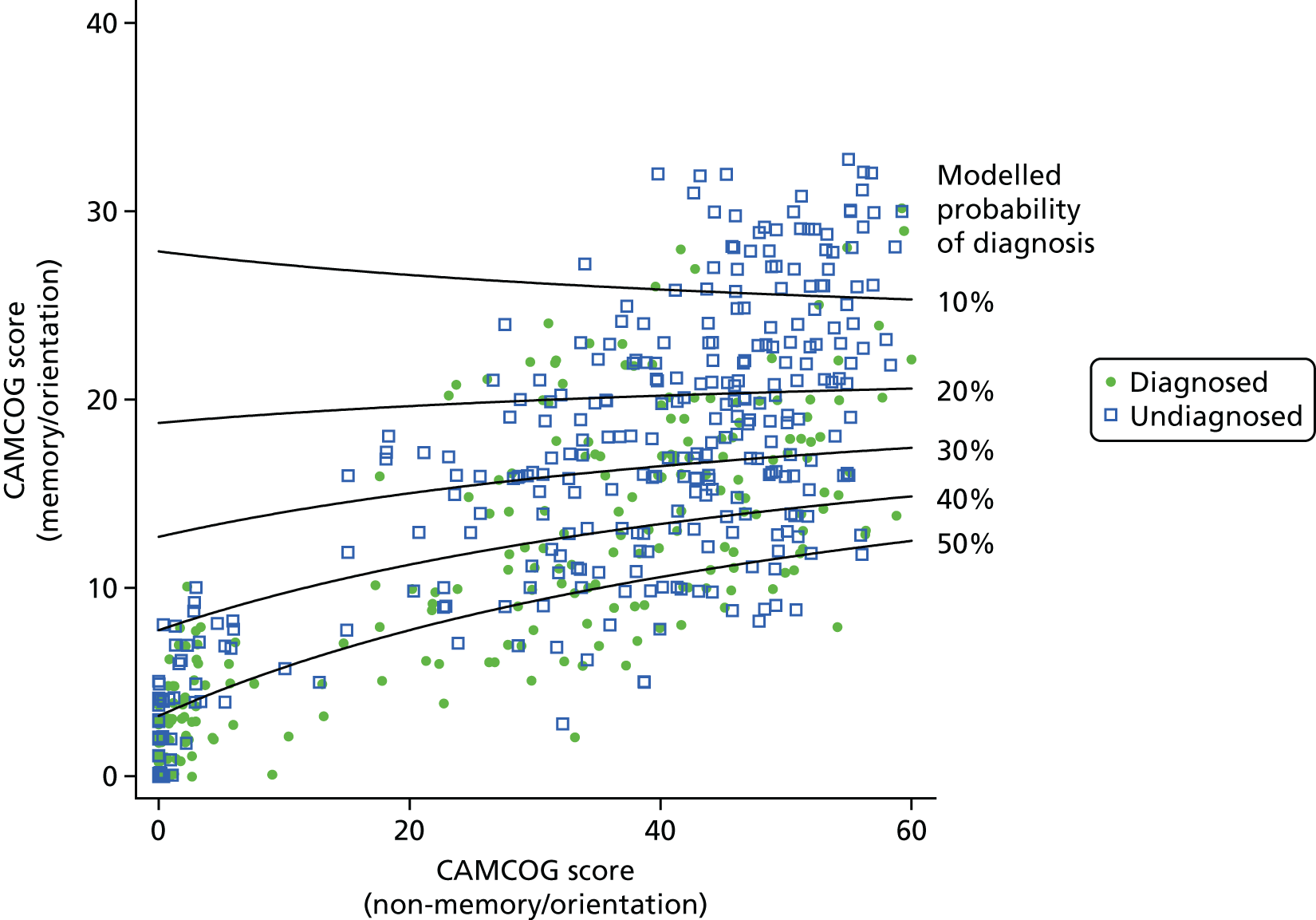
To explore this further and to identify specifically which domains of impairment were linked to diagnosis, a regression analysis was conducted, regressing diagnosis on all 10 CAMCOG subdomains simultaneously. The absolute difference in probability of diagnosis independently associated with a 1-SD change in each domain is shown in Figure 9. Only impairments in memory and orientation are positively linked to diagnosis.
FIGURE 9.
Association between CAMCOG domain scores (standardised) and the absolute probability of diagnosis. Positive scores indicate more impairment.
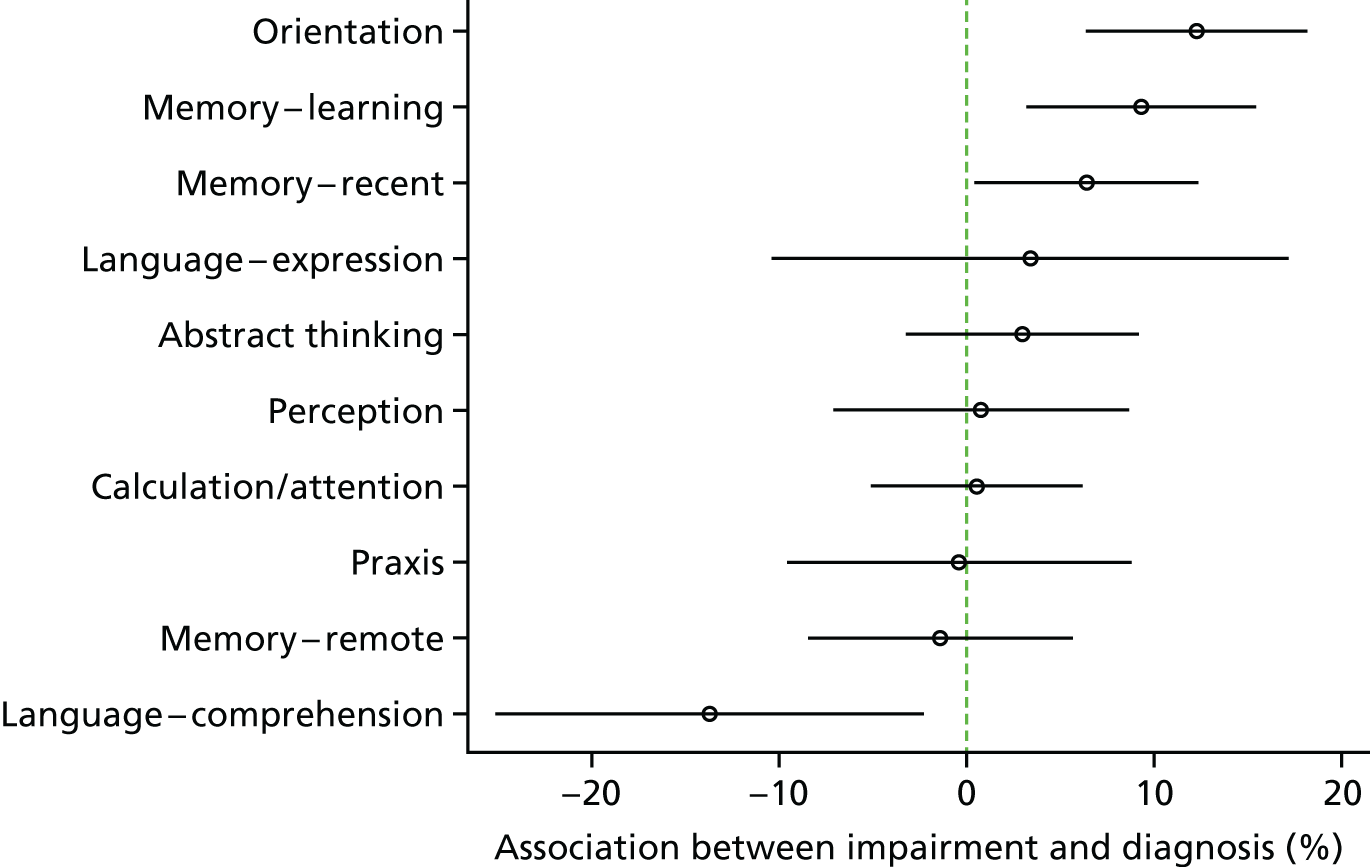
This analysis was repeated removing the cluster of cases with very low CAMCOG scores (CAMCOG < 10 or missing). This led to no appreciable difference in results (not shown).
Informant report of symptoms of dementia
As with objectively measured cognitive function, there is a strong association between symptoms of dementia reported by an informant and the chance of being diagnosed, seen for both memory and non-memory changes associated with dementia. Only 7 of the 61 participants whose informant endorsed zero to two of the memory symptoms had been diagnosed, compared with 77 of 136 (57%) participants endorsing all five memory questions. For non-memory changes, this association remains, from 31% among those endorsing no symptoms to 55–60% for those endorsing between five and seven symptoms.
However, as with objectively measured impairment, there is no independent effect of the number of non-memory symptoms reported when controlling for the severity of non-memory impairment (not shown).
Comorbid health conditions
Figure 7 shows the association between diagnosis and the number of cardiovascular comorbidities compared with non-cardiovascular comorbidities reported. There is some evidence that the number of diagnosed comorbidities is negatively correlated with the probability of being diagnosed, with borderline statistical significance for both cardiovascular (p = 0.045) and non-cardiovascular (p = 0.068) comorbidity. However, the numbers with four or five cardiovascular (n = 14) or four to six non-cardiovascular comorbidities (n = 17) are small, so this may be of limited practical significance.
There was no evidence that the likelihood of being diagnosed with dementia differed significantly between those with comorbid depression or anxiety and those without.
Social contact and activity
There is no evidence that that the frequency of contact with friends or family was linked to diagnosis. The chance of being diagnosed appears to rise slightly with the number of social activities, but this finding is not statistically significant.
Multivariable model
Finally, we estimated a model including each of the predictors discussed above simultaneously. All potential predictors are included, rather than those that are statistically significant in univariable analysis, because of a strong possibility of the suppression of effects meaning that potentially important factors might not appear important in univariate analysis.
Effects are shown in Appendix 3, Table 12, both for complete cases only (n = 270) and for all cases (n = 703) when imputing missing predictors and outcomes. There was no substantial difference in the pattern of point estimates of effects between complete case and multiply imputed models, although the precision of estimate under the imputed model was higher, as much more outcome and covariate information is available for analysis. Hence, the multiple imputation model is the primary result.
From this model, the strong and clearly significant independent predictors of diagnosis are the objectively measured level of memory impairment of the person [odds ratio (OR) 0.36, 95% CI 0.21 to 0.61 per SD]; the degree of memory impairment reported by the informant; the level of cardiovascular comorbidity (for three or more comorbidities); and W2 compared with W1 (OR 1.68, 95% CI 1.07 to 2.64). The effect of care setting is not statistically significant (p = 0.130), although it appears that people living with a long-term partner are twice as likely to be diagnosed with dementia than people living in other settings. When considered individually, the difference in likelihood of diagnosis between those living with a spouse and those living in long-term care is statistically significant (OR 2.2, 95% CI 1.2 to 4.0).
Although the effect of the number of non-memory symptoms reported by informants was not statistically significant when each factor is compared with a baseline of 0, or when the factor is tested globally, there is evidence of an increasing chance of diagnosis with an increasing number of non-memory symptoms. As a post hoc analysis, we tested this as a single continuous linear variable and observed a statistically significant effect (OR 1.20 per symptom, 95% CI 1.05 to 1.37).
A similar finding was observed for age group; although there appears to be a non-linear relationship between age and diagnosis, in that those in the 65–74 years and ≥ 90 years age groups are less likely to be diagnosed with dementia than those aged 75–89 years. When we estimate the effect of age as a continuous variable with a linear and quadratic term in the model, the joint effect of these terms is statistically significant (p = 0.030).
The apparent increase in the proportion diagnosed with the number of social activities is also seen in a multivariable analysis, but is based on very few participants undertaking two or more activities and is not statistically significant. Likewise, those with case-level anxiety had twice the chance of being diagnosed in this data set but, again, numbers with dementia and case-level anxiety are small and this finding is not statistically significant so may not reflect a difference in the wider population.
There is no effect of non-memory cognitive function on diagnosis rate, no effect of sex and no effect of socioeconomic status as measured either by educational attainment or by area-level deprivation. There also does not appear to be an effect of the number of non-cardiovascular comorbidities reported.
Predictors of raising cognitive concern and of diagnosis once a concern is raised
This multivariable model was then disaggregated into two: (1) a model for the probability of help-seeking or referral among the whole population with dementia; and (2) a model for the probability of diagnosis among the subset with at least help-seeking or referral.
Appendix 3, Table 13 shows the association between each factor and (1) help-seeking and (2) diagnosis conditional on help-seeking. There is no clear distinction between the factors that affect either aspect of the diagnostic process. Subjective and objective memory impairment, comorbidity and living arrangement each appear to significantly affect both the chance of having a cognitive concern recorded and the probability of having a diagnosis once the concern is raised.
Chapter 10 Results (research question 3): the rate of new diagnosis
The time to diagnosis for people with incident dementia
This chapter describes the time to primary care diagnosis for participants with incident dementia between waves (i.e. those who did not meet the criteria for dementia at the W1 assessment but did at the W2 assessment).
There were 152 participants in CADDY with incident dementia in whom primary care diagnosis status at the time of their W2 interview was known (164 consented, of whom eight did not have data returned and four were censored). Of these participants, 14 (9%) had a diagnosis of dementia recorded in primary care before the W1 interview (see Chapter 8, Research question 1b: prevalence and characteristics of the cohort without dementia but with a dementia diagnosis) and seven (5%) had a diagnosis recorded more than 4 years before the W2 interview. A further 36 participants with incident dementia at W2 (24%) had a first primary care diagnosis between W1 and W2, leaving 102 of the 152 participants with incident dementia alive and without clinical diagnosis at the time of the W2 interview. Between the W2 interview date and the date at which primary care records were ascertained (median 3.9 years, IQR 3.5–4.6 years), 38 of these participants received a diagnosis, 26 died without ever having a dementia diagnosis and 38 remained alive and undiagnosed.
Figure 10 shows the cumulative incidence of dementia diagnosis up to 4.5 years after the W2 assessment for participants whose index assessment with dementia was at W2. The cumulative incidence of death without diagnosis is also shown. Approximately 4.5 years after the index assessment (hence, approximately 5.5 years from the point when the participant would meet CFAS II criteria for a dementia diagnosis), around 60% of participants had a diagnosis in their GP record. A further 20% had died with dementia but without a GP-recorded diagnosis, leaving a further 20% of participants with dementia alive but undiagnosed at that time.
FIGURE 10.
The cumulative incidence of diagnosis (black dashed line) and death without diagnosis (green dotted line) for participants who developed dementia between W1 and W2.
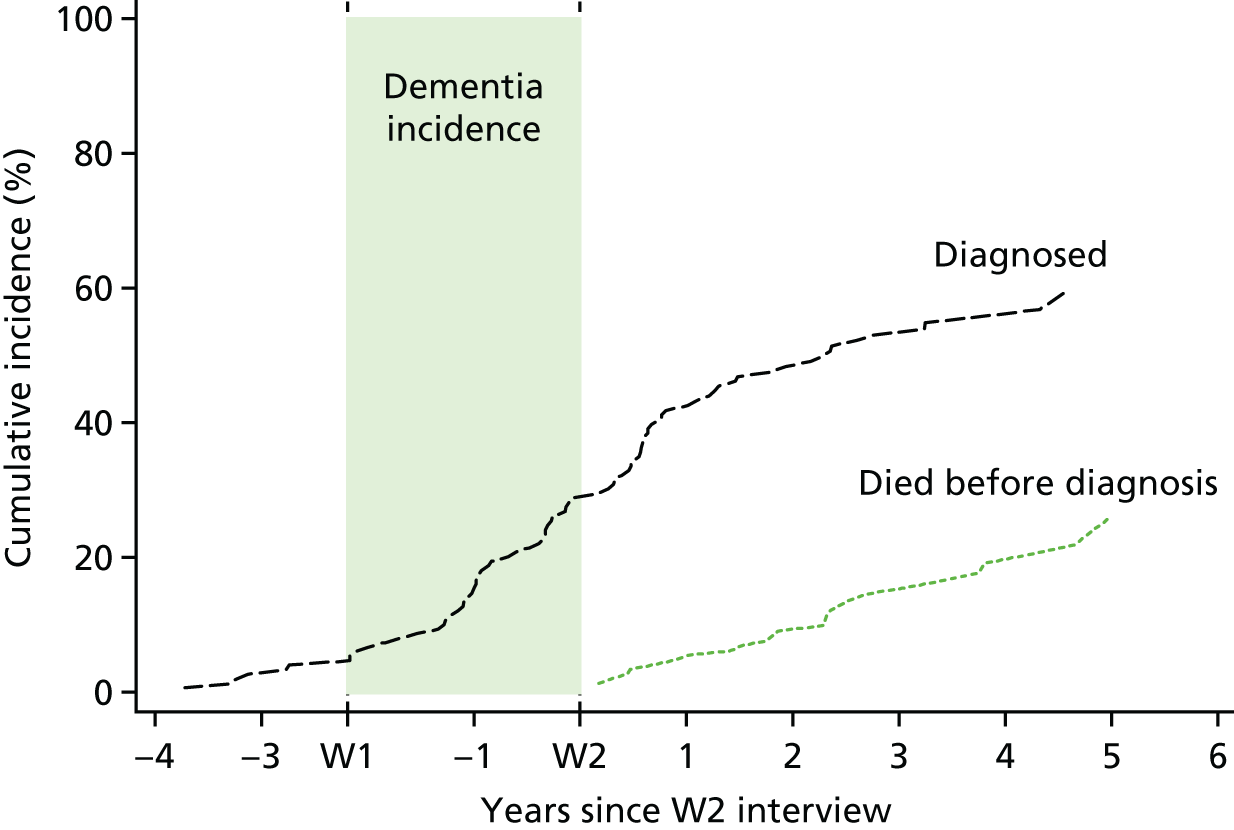
The median time to GP record of a diagnosis (time by which 50% of participants have a diagnosis) is 2.35 years from the W2 interview. The exact date of dementia onset for these participants (the date at which a participant would consistently meet the clinical criteria for dementia) is unknown, but if we assume it is at the mid-point between the W1 and W2 interviews (around 1.1 years before W2 interview), then this suggests that the median time to a diagnosis being recorded in primary care from dementia onset is approximately 3.5 years.
A further implication is that the rate of new GP diagnoses among those undiagnosed slows considerably after the period around dementia onset. Figure 11 shows the hazard function for diagnosis, that is the rate of new diagnoses among those undiagnosed as a function of time since the W2 interview. The rate of diagnosis increases sharply after a low start during the period around CFAS dementia onset, until it peaks at around 0.2 diagnoses per year (that is, the chance of getting a GP diagnosis over the course of a year for somebody without a GP diagnosis peaks at about 20%). Following this period, the rate of new GP diagnoses among the undiagnosed falls rapidly, to around half the maximum rate of 3–4 years after the W2 interview. This suggests that, if a person with dementia does not receive a diagnosis around the time of dementia onset (when they would meet the criteria for dementia), then they are increasingly unlikely ever to do so.
FIGURE 11.
The smoothed hazard (probability of being diagnosed over the next year) as a function of the time since the W2 assessment for people with incident dementia between W1 and W2.
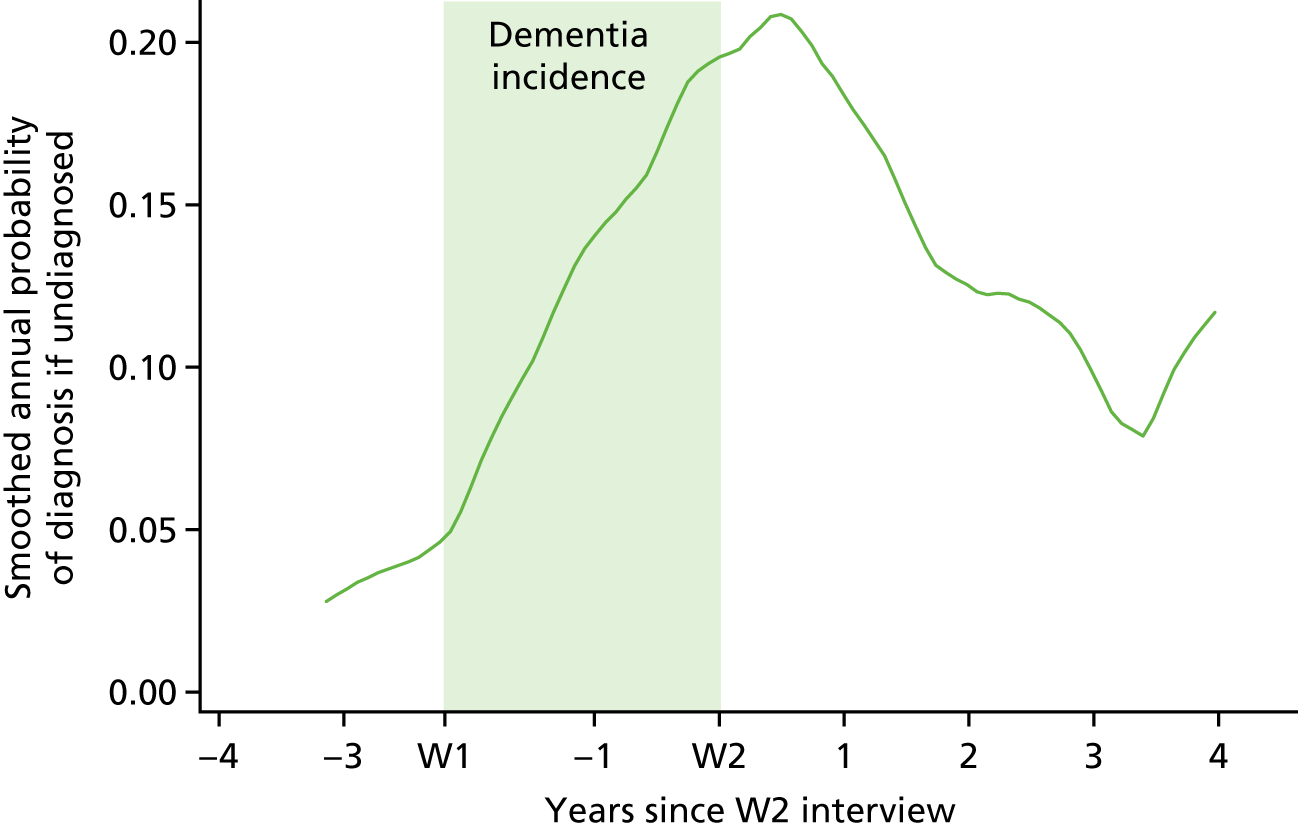
Factors affecting the rate of new diagnosis
To understand which patient characteristics affected the probability of a new diagnosis among those who were undiagnosed, we conducted an analysis among the CADDY sample with dementia but no GP diagnosis. We conducted a time-to-event analysis, including people from the point of their index assessment, that is the first date on which they were assessed to have dementia in CFAS II, and included only people without a GP diagnosis on that date.
There were 285 participants with dementia first seen at either W1 or W2 who did not have a primary care diagnosis recorded at the time of their CFAS assessment. In this analysis, we compare the characteristics of those who were diagnosed with the characteristics of those who were not diagnosed over the subsequent 2 years.
This is in contrast with the analysis in Chapter 9, in which we considered which factors independently predicted which participants had or had not received a diagnosis up to the point of their CFAS assessment (could be considered prevalent diagnosis). Here we consider which factors can predict which of the participants who are undiagnosed at the time of their CFAS assessment go on to receive a diagnosis in the subsequent 2 years (incident diagnosis). Two years is chosen to allow a sufficient number of participants to receive a diagnosis for this to be a meaningful analysis, but also to ensure that patient characteristics are unlikely to change substantially.
Patient characteristics
Of the 285 participants included in the current analysis, 66 (23%) received a GP-recorded diagnosis over the next 2 years, whereas 56 (20%) died without a GP-recorded diagnosis in that time. The characteristics of participants stratified into those who received diagnosis, those who died before diagnosis and those who remained alive but undiagnosed after 2 years are shown in Appendix 3, Table 14. Outcome after 2 years is linked to cognitive function, subjective reports of memory and non-memory complaints, years of education, age and living arrangement.
Multivariable regression
The results of the multivariable competing risks regression analysis are shown in Appendix 3, Table 15. As with prevalent diagnosis, new diagnosis is strongly linked to memory function and non-memory function; however, in contrast to the effect on prevalent GP diagnosis, conditional on memory impairment, the effect of non-memory impairment on incident diagnosis is significant and in the opposite direction, that is a higher non-memory function score predisposes to diagnosis. However, this result is of borderline statistical significance (OR = 2.2 per SD; p = 0.036). The pattern of subjective impairments being linked to diagnosis is also present but not as strong, with none of the individual effects being statistically significant although the pattern is consistent with the effect of prevalent diagnosis. Women are significantly more likely to have a new diagnosis than men, but there is no statistically significant association between educational attainment, area-level deprivation and new diagnosis. The pattern with respect to age group mirrors that seen for prevalent diagnosis, with the youngest (65–74 years) and oldest (85–89 years and ≥ 90 years) groups being less likely to receive a new diagnosis than those aged 75–85 years. As with prevalent diagnosis, there is a suggestion that case-level anxiety is linked to a higher rate of diagnosis, whereas non-cardiovascular comorbidity appears to make a new GP-recorded diagnosis less likely, but these effects are not statistically significant.
In contrast to the findings for prevalent analysis, there is no effect of cardiovascular comorbidity on new diagnosis. For those whose index interview was W2, GP diagnosis in the next 2 years was around three times more likely than for those included at W1 (OR 3.03, 95% CI 1.44 to 6.63; p = 0.003). Most strikingly, among those living in residential care there were almost no new GP-recorded diagnoses among people with dementia not already diagnosed (OR 0.07, 95% CI 0.01 to 0.45; p = 0.005) compared with those living alone.
Chapter 11 Results (research question 4): the consequences of dementia diagnosis
Does a general practitioner-recorded diagnosis affect subsequent mortality?
As in the time-to-event analysis reported in Chapter 10, Factors affecting the rate of new diagnosis, survival was estimated from the first CFAS interview at which a participant met the study criteria for dementia (the index assessment, reflecting prevalent dementia cases at W1 and incident dementia cases at W2). There were 445 such CADDY participants in total, with GP diagnosis status known for 421 participants (136 with a GP-recorded diagnosis at the time of the index CFAS assessment, 285 without a GP-recorded diagnosis at that time). In the current analysis, survival was estimated up to 6 years post index assessment. Those who do not meet the CFAS II criteria for dementia are not included in this analysis.
A total of 278 participants died within 6 years of their index assessment, of whom 267 had known diagnosis status at their index assessment. The characteristics of those who died and those who did not are shown in Appendix 3, Table 16.
Figure 12 shows Kaplan–Meier plots comparing the survival from index assessment between those who did and those who did not have a diagnosis recorded in primary care at that time. There is little difference in the survival curves (Kaplan–Meier test, p = 0.083). The hazard ratio (HR) associated with diagnosis is 1.25 (95% CI 0.97 to 1.61; p = 0.083), suggesting a slightly increased mortality rate among the diagnosed that is not statistically significant.
FIGURE 12.
The proportion of diagnosed (green dotted line) and undiagnosed (black line) participants surviving up to 6 years following their index interview.
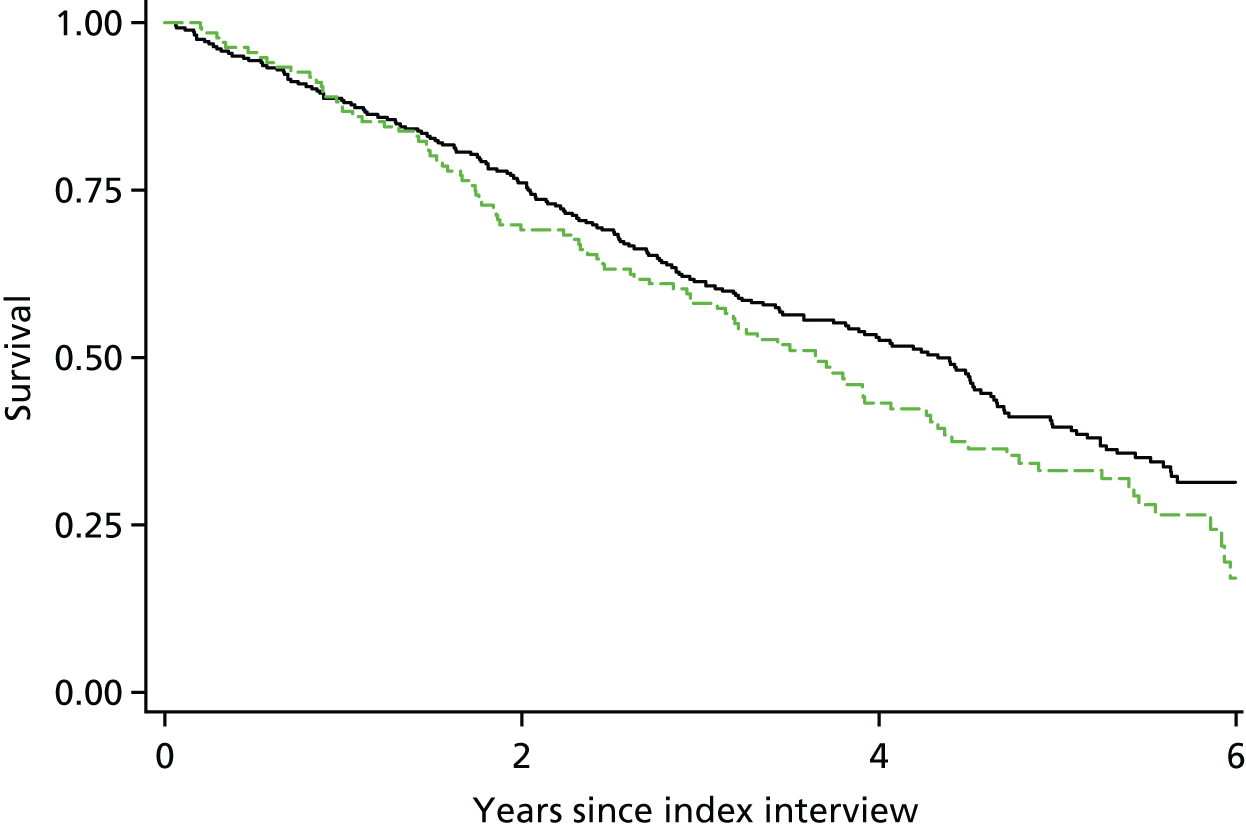
However, we know from previous chapters that those who are diagnosed tend to have more severe dementia, different living circumstances and other factors that could predispose to mortality, and these factors might suppress any benefit of diagnosis on survival.
The estimates of the effect of diagnosis and covariates on subsequent mortality, estimated using a multivariable Cox proportional hazard regression with multiple imputation used to recover missing covariate data are shown in Appendix 3, Table 17. After adjusting for all potential prognostic indicators that could also be linked to diagnosis status in a multiple imputation model, the HR associated with diagnosis is unchanged from the univariable analysis (HR 1.25, 95% CI 0.91 to 1.71; p = 0.169). Other factors significantly associated with increased mortality were increased age; living in residential care or with other family members compared with living alone or with a partner; increasing number of subjectively reported non-memory impairments; and lower educational attainment.
When a complete-case-only analysis with 236 participants was conducted, the results did not qualitatively change (HR for diagnosis 1.07, 95% CI 0.69 to 1.66). The proportional hazard model fitted well. The global test for proportional hazards assumption was χ432=41.0; p=0.557.
Social contact and mood
Changes in frequency of social contact and mood (generalised anxiety and health-related worry) following diagnosis were measured using data from participants who had both W1 and W2 assessments, and who had dementia at W2 (n = 232). Patients were divided into three groups depending on their diagnosis status at W1 and W2: (1) diagnosed at baseline (n = 42); (2) newly diagnosed between waves (n = 51); and (3) remained undiagnosed (n = 139). Mean average levels of social activity, health-related worry and anxiety among these groups at both waves are shown in Table 5. Only participants for whom data are available at both assessments are included. The proportions improving or worsening with respect to each outcome are plotted in Figure 13.
Wave | No diagnosis at W1 or W2 (n = 139) | Newly diagnosed (n = 51) | Diagnosed at W1 (n = 42) |
---|---|---|---|
Social activity (number of activities), mean (95% CI) | |||
W1 | 0.51 (0.36 to 0.64) | 0.74 (0.51 to 0.96) | 0.68 (0.41 to 0.95) |
W2 | 0.47 (0.34 to 0.59) | 0.59 (0.32 to 0.85) | 0.57 (0.23 to 0.91) |
Health-related worry, % (95% CI) | |||
W1 | 28 (19 to 36) | 35 (19 to 52) | 30 (11 to 50) |
W2 | 28 (19 to 36) | 32 (16 to 48) | 22 (4 to 39) |
Anxiety (subthreshold or clinical), % (95% CI) | |||
W1 | 38 (30 to 46) | 39 (24 to 54) | 40 (23 to 57) |
W2 | 33 (25 to 42) | 37 (22 to 52) | 34 (18 to 50) |
FIGURE 13.
The proportions of participants improving or worsening between waves with respect to (a) anxiety; (b) health-related worry; and (c) social activity; stratified by diagnosis status at W1 and W2.
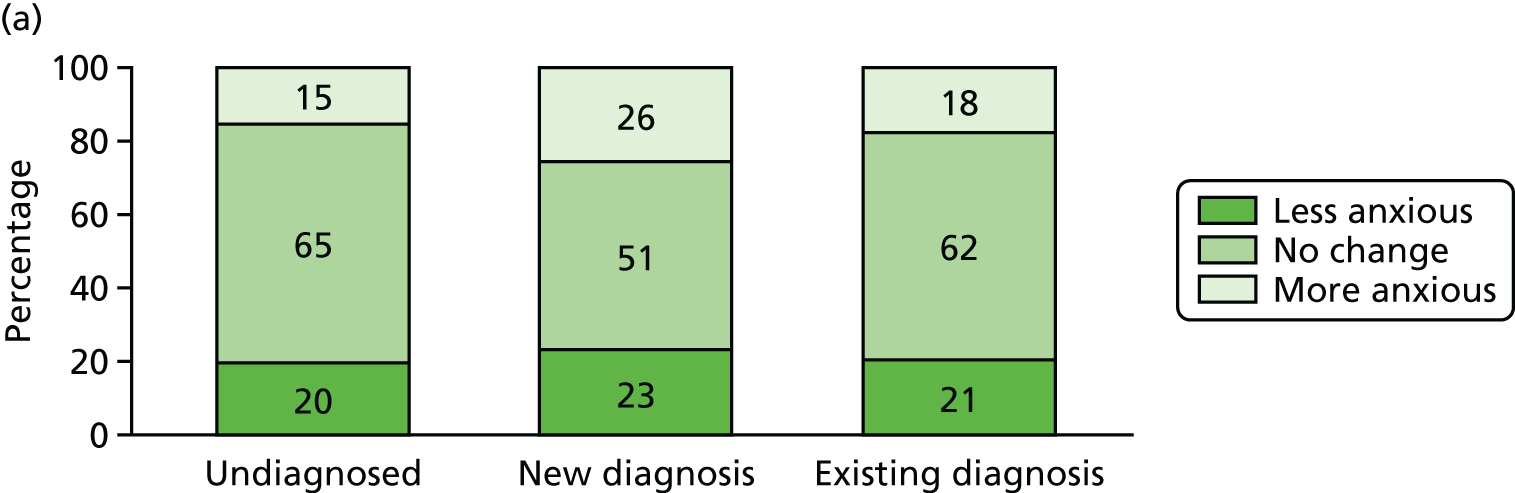
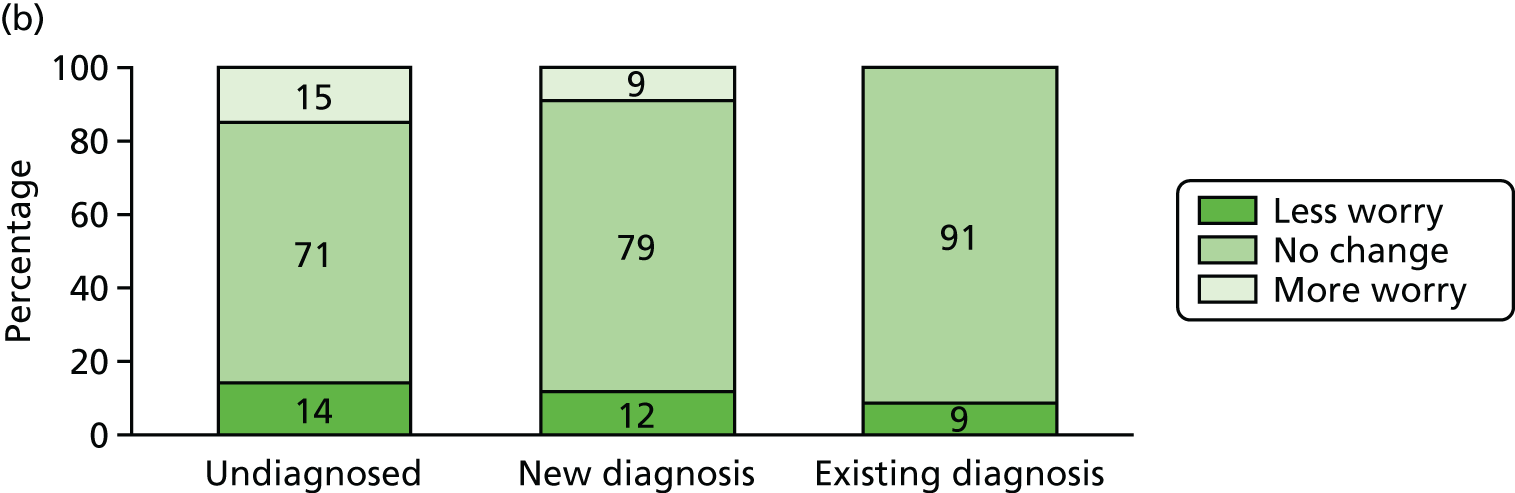
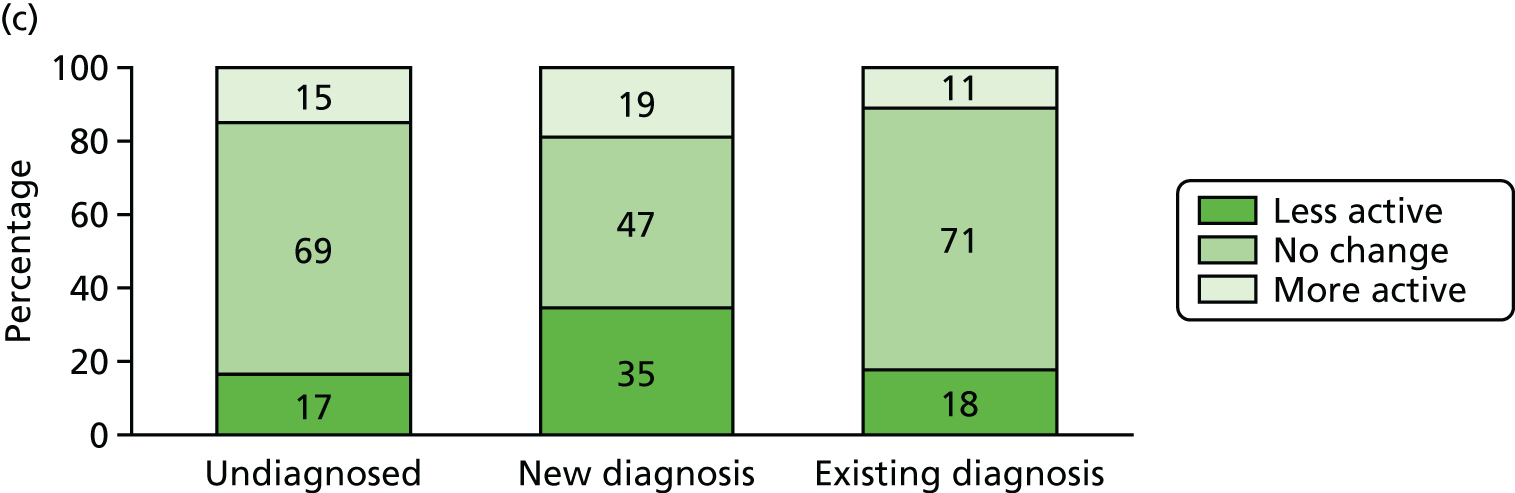
Table 6 shows the numbers of participants whose data suggested improvement or worsening with respect to each outcome and ORs from multinomial regression estimating the effects of new diagnosis or existing diagnosis on the chance of improving or worsening each outcome, adjusted for age, sex, living arrangement and cognitive function.
Change in social contact and mood | Undiagnosed (N = 139) | New diagnosis (N = 51) | Previous diagnosis (N = 42) | |||
---|---|---|---|---|---|---|
n (%) | OR (reference value) | n (%) | OR (95% CI) | n (%) | OR (95% CI) | |
Social activity | ||||||
Fewer social activities | 20 (16.1) | 1 | 16 (34.8) | 3.09a (1.26 to 7.59) | – | 1.15 (0.34 to 3.85) |
No change | 85 (68.6) | 1 | 22 (47.8) | 1 | 20 (71.4) | 1 |
More social activities | 19 (15.3) | 1 | 8 (17.4) | 1.88 (0.66 to 5.41) | – | 1.24 (0.29 to 5.26) |
Total | 124 (100) | 46 (100) | 28 (100) | |||
Health-related worry | ||||||
Less worry | 17 (14.7) | 1 | – | 0.63 (0.18 to 2.17) | – | 0.35 (0.07 to 1.78) |
No change | 82 (70.7) | 1 | 27 (79.4) | 1 | 21 (91.3) | 1 |
More worry | 17 (14.7) | 1 | – | 0.44 (0.11 to 1.78) | – | 0 |
Total | 116 (100) | 34 (100) | 23 (100) | |||
Anxiety | ||||||
Less anxiety | 25 (19.4) | 1 | 9 (22) | 1.33 (0.52 to 3.42) | 7 (20) | 0.82 (0.29 to 2.36) |
No change | 85 (65.9) | 1 | 22 (53.7) | 1 | 22 (62.9) | 1 |
More anxiety | 19 (14.7) | 1 | 10 (24.4) | 1.88 (0.70 to 5.05) | 6 (17.1) | 1.29 (0.40 to 4.23) |
Total | 129 (100) | 41 (100) | 35 (100) |
In general, there are no clear patterns of benefit or harms associated with diagnosis in this data set. Although those with a new diagnosis are more likely than those in the other two groups to be reported to have fewer social activities after their diagnosis (OR for reducing social activity associated with new diagnosis = 3.09, 95% CI 1.26 to 7.59), this is an analysis which emerges from a large number of independent hypothesis being tested. Similarly, although more newly diagnosed participants had an increase in anxiety between waves compared with the other two groups, numbers were small and the difference was not statistically significant. Both of these possible effects should be tested in larger dedicated studies.
Note these data are limited owing to small numbers of participants, but are included for completeness and transparency as this analysis was specified in the original study plan. There is a large amount of missing data particularly for the items that rely on self-report; we did not feel that information on worry or anxiety would be meaningful among those who were not able to provide their own responses.
Transfer to residential care setting
There were 218 participants living in their own home at W1 for whom we have primary care diagnosis data and who had dementia at W2. Between W1 and W2, 36 participants were moved to a care setting. Of the 181 participants who were undiagnosed at W1, 15% (n = 27) were institutionalised between waves [compared with 24% (n = 9) of those with a diagnosis]. However, we know from previous chapters that those with a diagnosis are more likely to have more advanced dementia, which would also predispose to a move to a care setting, and so we conducted a multivariable regression analysis to control for this difference. Without adjustment, the OR for new institutional placement associated with being diagnosed with dementia is 1.83 (95% CI 0.77 to 4.31). After adjustment for baseline cognitive function, age and sex, this OR becomes stronger at 2.15 (95% CI 0.82 to 5.59).
If we restrict this analysis to participants with dementia at W1 and W2 (n = 72), then the pattern of results is similar. Seven of 26 (27%) participants with a diagnosis moved to a care setting during follow-up [compared with 10 of 46 (22%) who did not have a diagnosis]. The OR for the association between diagnosis and move to a care setting is 1.32 (95% CI 0.44 to 4.04), which, after adjusting for cognitive function, age and sex, becomes 1.95 (95% CI 0.52 to 7.29).
In summary, although numbers of participants available for this analysis are low, there is no evidence that being diagnosed prevents or delays a move to a care home among people with dementia not already in a care setting.
Inpatient hospital visits
There is also no clear evidence that being diagnosed affects the chance of a subsequent inpatient hospital stay for a person with dementia. Table 7 shows the number of patients with dementia at W2 who had hospital stays in the year prior to W2, stratified by the diagnosis status at W1. Of 193 patients with no diagnosis, 12% (n = 23) had one hospital admission and 8% (n = 15) more than one [compared with 7% (n = 3) and 19% (n = 8) of the 43 patients who were already diagnosed at W1].
No dementia diagnosis at W1 (N = 193) | Diagnosed at W1 (N = 43) | Rate ratio | |||
---|---|---|---|---|---|
Unadjusted (95% CI) | Adjusteda (95% CI) | ||||
Inpatient visits to hospital, n (%) | 0 | 155 (80) | 32 (74) | ||
1+ | 38 (20) | 11 (26) | 1.19 (0.63 to 2.23) | 0.72 (0.40 to 1.31) | |
Total nights, mean (SD) | 5.7 (23.1) | 4.7 (11.7) | 0.83 (0.33 to 2.1) | 0.58 (0.24 to 1.43) | |
Total nights if any stay, mean (SD) | 29.7 (45.8) | 18.5 (17.2) | 0.62 (18.1 to 48.7) | 0.37 (0.16 to 0.84) |
The corresponding rate ratio for hospital stays associated with diagnosis was 1.19 (95% CI 0.63 to 2.23); when adjusted for age, sex, overall cognitive function and living arrangement at W1, this reduced to 0.72 (95% CI 0.40 to 1.13). When the analysis is restricted to those with a study diagnosis of dementia at W1, the pattern is similar (Table 8), with a rate ratio of 1.37 (95% CI 0.53 to 3.54) attenuated to 0.63 (95% CI 0.19 to 2.07) in multivariable analysis.
No dementia diagnosis at W1 (N = 53) | Diagnosed at W1 (N = 29) | Rate ratio | |||
---|---|---|---|---|---|
Unadjusted (95% CI) | Adjusteda (95% CI) | ||||
Inpatient visits to hospital, n (%) | 0 | 44 (83) | 22 (76) | ||
1+ | 9 (17) | 7 (24) | 1.37 (0.53 to 3.54) | 0.63 (0.19 to 2.07) | |
Total nights, mean (SD) | 0.8 (2.8) | 6.7 (13.9) | 8.28 (2.45 to 28.0) | 7.01 (1.46 to 33.7) | |
Total nights if any stay, mean (SD) | 5.3 (5.8) | 27.7 (14.8) | 5.28 (2.31 to 12.1) | 24.3 (4.4 to 133.7) |
The distribution of length of stay across groups is also shown in Tables 7 and 8. Among all participants with dementia at W2, being diagnosed at W1 appears to be associated with a shorter length of stay among those with at least one admission [mean 29.7 days for undiagnosed compared with mean 18.5 days for diagnosed participants; adjusted rate ratio 0.37 (95% CI 0.16 to 0.84) days]. However, this pattern is reversed if the analysis is restricted to those with dementia at W1, with a much higher average length of stay among participants with a diagnosis than among participants who are undiagnosed. Figure 14 shows how individual data points may account for this discrepancy. When all participants are included (see Figure 14a), then the mean length of stay is affected by several large values (seven participants each with > 75 nights in hospital in the year before W2). If medians rather than means are compared, then it appears those who are diagnosed have longer stays than those who are undiagnosed (median of nine nights in hospital for the undiagnosed, compared with 17 for those diagnosed). This is consistent with the pattern seen when restricting to those with dementia at W1 (see Figure 14b), although that group includes only 17 participants.
FIGURE 14.
The total number of nights in hospital in the year before W2 among participants with at least one overnight stay, stratified by W1 diagnosis status. (a) All participants with dementia at W2; and (b) participants with dementia at W1 and W2.
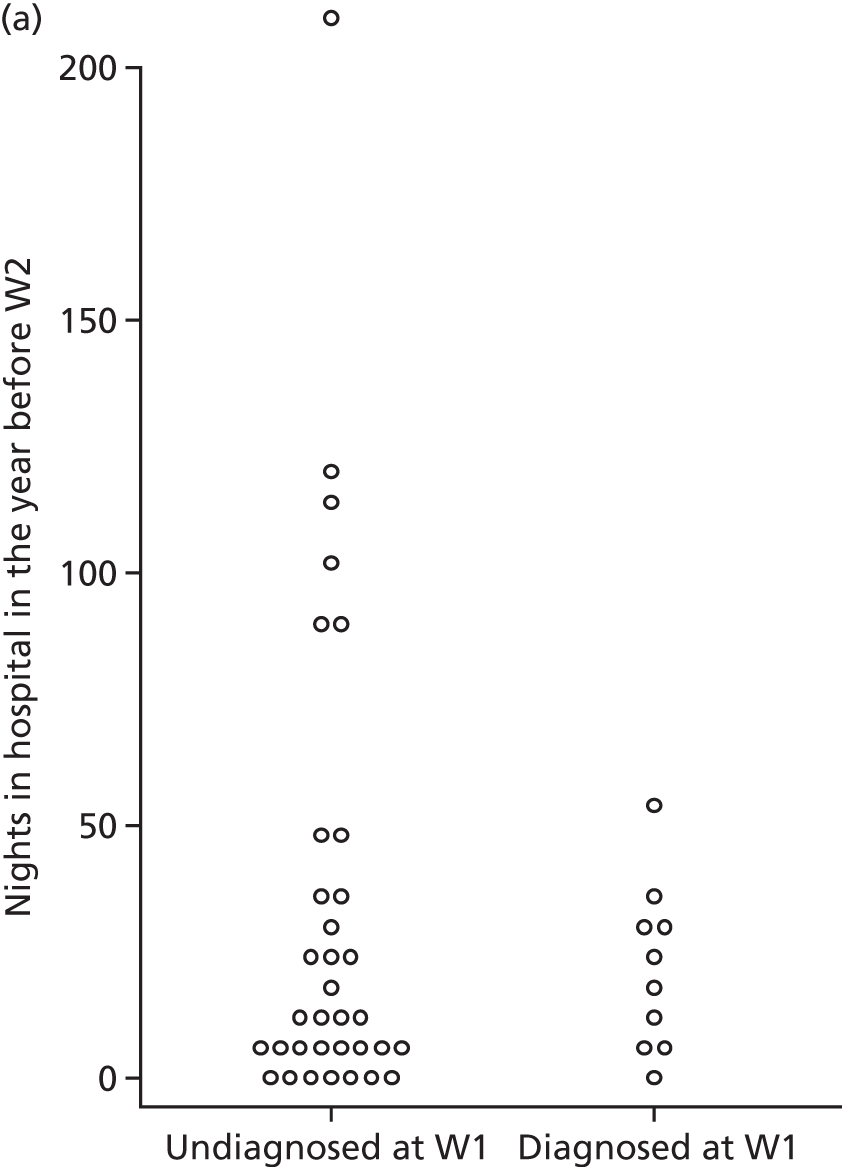
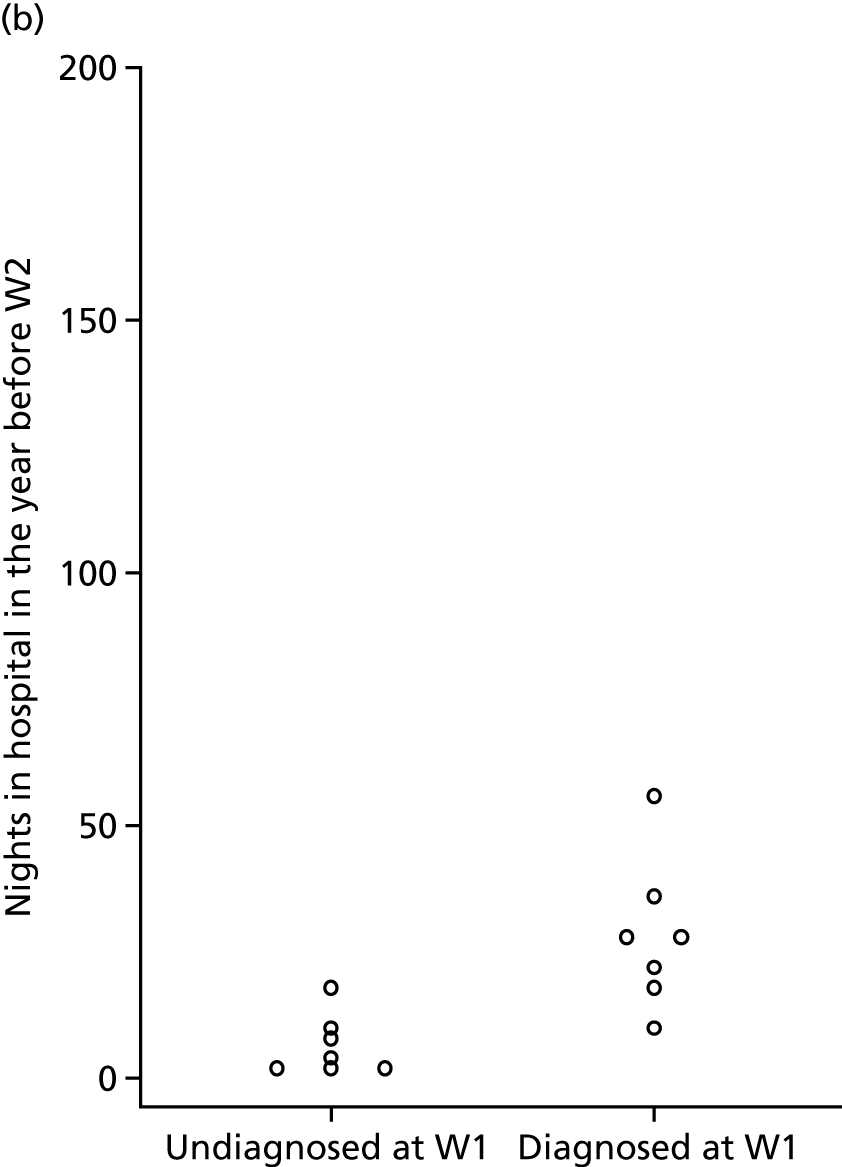
Few strong conclusions can be drawn from these data regarding the effect of diagnosis on the rate of subsequent hospital visits or length of stay because of the small numbers; however, no evidence emerges from this analysis to suggest that receiving a diagnosis prevents hospitalisation or costs associated with overnight stays among people with dementia.
Chapter 12 Public and patient involvement in CADDY
Introduction
Public and patient involvement in research is helpful to ensure that it is well conducted and to realise its potential society benefit.
Public and patient involvement in CADDY conceptualisation and development
Patient and public views shaped CADDY from the outset. Improving dementia diagnosis and understanding its impact has been consistently raised as a priority by patient and public groups. The National Institute for Health Research (NIHR) Dementia and Neurodegeneration Research Network Primary Care Clinical Studies Group identified diagnosis of dementia as a top five research priority in 2012. Alzheimer’s Society lay members contributed to the 2013 James Lind Alliance priority-setting exercise for dementia and identified ‘What is the impact of an early diagnosis of dementia and how can primary care support a more effective route to diagnosis?’ as a top 10 priority for dementia research. Delayed dementia diagnosis was consistently raised by members of the Norfolk Older Peoples Strategic Partnership Forum in 2013. The concept for CADDY was developed with the support of the Public and Patient Involvement in Research (PPIRes) group; this chapter describes the PPI throughout the course of the study.
In the development of the CADDY funding bid, we consulted lay members of the Alzheimer’s Society and met with and consulted with two local PPI groups (inspire and PPIRes), who all echoed the need for this study. PPI group members identified the role of comorbid mental and physical health in their own experience as predictors of dementia diagnosis; the huge fear of diagnosis for many people; the individuality of the decision to seek help; and the importance of considering those less likely to participate in a survey. We refined the research questions and methods accordingly and when the study was running, developed an advisory group of 11 PPI members and invited three members to join the Study Steering Committee (SSC) to ensure that the views of our PPI partners were included at all stages.
Public and patient involvement during the study
Public and patient involvement members joined the CADDY advisory group and SSC from three PPI associations:
-
PPIRes (this group, co-ordinated by NHS South Norfolk CCG, includes a panel of volunteers from Norfolk and Suffolk with a range of experiences as patients and carers of people with dementia)
-
Inspire (this group, co-ordinated by NHS Norfolk and Suffolk Foundation Trust, is an initiative to involve service users and carers in mental health research)
-
the Alzheimer’s Society Research Network (this group includes a large team of carers, former carers and people living with dementia).
Members were invited to join the CADDY Public and Patient Involvement Advisory Group (PPIAG) via organisers of their respective PPI associations. Five members joined from PPIRes, five from Inspire and one from the Alzheimer’s Society Research Network. One representative from each PPI association also joined the SSC to represent the views of the PPIAG and to provide PPI oversight to the study.
Ten of the 11 PPIAG members had direct experience of dementia care and one member had indirect experience through supporting friends who have parents with dementia. Nine members had been directly involved in the dementia diagnosis process and six had previously been participants of a research study. As well as experience of caring for people with dementia, PPI SSC members brought a range of professional experience from public, private, health and social care organisations, which was also helpful in terms of providing a managerial oversight.
Public and patient involvement activities
The PPIAG met twice, in February 2016 and in July 2017. George Savva chaired and Clare Aldus facilitated the meetings. Members received reimbursement for their time and travel costs in accordance with INVOLVE guidelines. The PPIAG was updated through newsletters.
The CFAS II national co-ordinator (Linda Barnes) provided training in the CFAS II data set for PPI SSC members. PPI members of the SSC met George Savva and Clare Aldus ahead of each SSC meeting to ensure that PPI and research team members worked together effectively and had the opportunity ask questions in an informal setting ahead of each meeting.
The first PPI advisory group (PPIAG 1) meeting was held while the study protocol was being developed, before our application for ethics approval was made. We consulted PPIAG 1 with respect to ethical issues and their experiences of dementia diagnosis.
The PPIAG met again in July 2017 (PPIAG 2), following the completion of data collection, preliminary analysis and early presentation of the results at a public seminar. PPIAG 2 reflected on the early results of the study and the presentation style. Key themes were identified, summarised and shared with our SSC and research team to develop a final analysis plan and to refine presentation.
Eleven members of the advisory group attended the first meeting (February 2016) and five attended the second meeting (July 2017). Each meeting ran for approximately 2 hours. Each PPIAG meeting was audio-recorded and transcribed. Transcripts were anonymised, key themes were identified and these were returned to each PPIAG for comment.
Influence of PPIAG on CADDY
The validity of continuing consent to inspect medical records
Although CFAS II had consented participants for medical record linkage at baseline, and for research data to be held indefinitely after their death, there was a question as to whether or not CFAS II participants would have understood this to mean that their records might be accessed several years later or after their death or incapacity. This question was put to the PPIAG, along with the relevant consent forms and a description of the basic design of the study, to explore any unforeseen concerns that might emerge.
PPIAG 1 participants unanimously supported the idea that the CFAS II consent remained valid and should apply to CADDY. Participants understood the nature of longitudinal research and their view was that CFAS II participants would expect their data to be used in this way. One PPIAG 1 member raised the issue that the wording on the original consent for access to medical records form gave consent to members of the CFAS II team and asked if this should also apply to the CADDY research team.
As a consequence of this, we developed our study documents to clarify the relationship between CFAS II and CADDY in terms of overall objectives (the aims of CADDY fall into the original broad remit of CFAS) and overlap in CFAS II and CADDY research team membership. Comments from PPIAG 1 were used in direct support for our application for ethics approval.
Help-seeking and diagnosis of dementia
Factors identified by PPIAG that might encourage seeking a diagnosis included the access to health and social services that a diagnosis can provide; encouragement of friends and relatives; positive attitudes to addressing or fighting dementia; crisis or social problems (e.g. aggression); more reliable, available and standardised diagnostic tests; and information, public awareness and education.
Factors the PPIAG considered might delay seeking a diagnosis were more complex and interlinked. Many were linked to the perceived or experienced potential harms of diagnosis. For example, although it was acknowledged that diagnosis could provide access to services, it was strongly believed to be stigmatising. Fear of diagnosis and denial of symptoms were recurring themes. These were raised indirectly (e.g. fear of being unable to do usual activities or losing independence through loss of employment, mobility, driving licence and travel insurance). Because of the lack of effective treatments, many of the group perceived diagnosis to have little benefit. Several expressed concern about GP incentivisation and consequent loss of trust in the diagnostic process. Some recognised that many would not wish to know if they had dementia and that that wish should be respected.
Interpersonal relationships and living situation were thought to play a complex role in the decision to seek help. It was thought that diagnosis may be delayed by living alone or, conversely, by living with people who might enable an individual to live well with significant cognitive impairment. The dynamics of specific relationships were felt likely to be important.
Perceived benefits and harms of diagnosis
The PPIAG 1 members identified several benefits and harms of diagnosis, to both people with dementia and their families, from their experience or personal expectations. A recurring theme was that these benefits or harms were likely to vary with individuals.
A diagnosis was thought to provide benefit through access to formal health and social care services. For some, having a diagnosis or ‘label’ was also thought to be useful in maintaining good social interaction, support from the wider community and the ability to proactively improve safety for a person living with dementia and their carers. However others referred to this negatively as the ‘label syndrome’. Being labelled was considered potentially stigmatising and a possible cause of social withdrawal.
Perceived harms of diagnosis were related to very practical matters or to mental state and changes in behaviour, and were strongly linked to the reasons for reluctance to seek diagnosis discussed above. Participants suggested that, from experience, diagnosis can harm well-being and that as a result of diagnosis patients may become fearful about the future. Themes emerging from this discussion were used to identify potentially important hypotheses to test and, thus, the design of the data collection process and analysis to ensure that these hypotheses could be tested wherever possible.
Data collection and management
Public and patient involvement members of the SSC reviewed all study documentation, in particular the CRF and documents for practice staff, and contributed to the evaluation of the pilot data collection and the development of the data collection standard operating procedures. As members of the SSC, PPI members were able to fully participate in decision-making regarding all aspects of the data collection process.
Interpretation and presentation of preliminary data
The PPIAG 2 members were invited to reflect on the preliminary results of the study following a short presentation by George Savva. This discussion helped to identify aspects of the study design that were not well understood, further hypotheses to test, potential interpretations of results and how to present results most effectively.
Outcomes from this discussion were a need to relabel the ‘cognitive complaint’ group as ‘cognitive concern’ and also the insight that the differential effect of different cognitive function domains on diagnosis may reflect a public awareness gap. The group also expressed a clear preference for bar charts rather than dot plots or line graphs to compare the proportions diagnosed across groups; therefore, we have used these graphs throughout subsequent presentations and reports.
Dissemination
Dissemination activity is described in detail in Chapter 13. PPI SSC members have reviewed all study outputs so far prior to their publication. As members of the SSC, they contributed to the planning of our dissemination event ‘Dementia Diagnosis in Primary and Secondary Care: Policy and Practice’. PPI SSC members will be invited to review or co-produce all further outputs as appropriate.
Facilitating meaningful participation
At the outset, we had proposed to train PPI SSC members in the necessary epidemiological methods required to understand the study. However, on discussion with PPIAG members, it became apparent that this was not necessary as the important aspects of study design were easily understood and the range of professional backgrounds meant that any generic training would not have been useful. Instead, pre-SSC meetings (held on the same day as SSC meetings) were used to address any questions or concerns that PPI SSC members wanted to clarify ahead of the meeting. Following each meeting, George Savva and Clare Aldus checked that PPI SSC members were satisfied that they had been able to participate. The PPI SSC members have been proactive in raising questions regarding the scientific and procedural aspects of the study, which have led to substantial changes in the study. We are therefore confident that their contributions have been both genuine and meaningful.
Public and patient involvement in epidemiology
The CADDY PPI contributions have been valuable in many respects, specifically:
-
in support of our ethics application
-
insight with respect to the variety of patient experiences in health services, and how varied and complex decisions surrounding help-seeking and diagnosis are, and the effects of diagnosis for individuals
-
revision of the study materials from the point of view of non-researchers
-
support with interpretation of data in light of their experience
-
guidance on presentation of data
-
support for the design and implementation of dissemination activity
-
identification of key messages arising from findings
-
oversight and suggestions as to the management of the study
-
assurance from those directly affected by the issues being studied that the work is valuable beyond academic boundaries.
Public and patient involvement is underdeveloped in epidemiological research, and many volunteers and researchers are unsure of the value of their participation because epidemiological research is most often focused on data analyses and does not usually include recruitment of ‘real’ participants. Nevertheless, CADDY has benefited enormously from this interaction, often in unexpected ways, owing to the diversity of the personal and professional expertise that was brought into the study through the PPI group. As PPI members are independent of the research team, they provide a level of oversight to an ongoing research study that may not otherwise be present depending on the specific requirements for composition of the SSC.
There have been some challenges, particularly around PPI members feeling initially nervous about contributing to discussions regarding work that is mainly statistical in nature, and, at the outset, some PPIAG members not understanding that we are largely unable to alter the nature of the data available to us or the experience of the participants of the wider CFAS II. However, these challenges were overcome and as the public become more aware of ‘big data’ research and PPI groups participate more often in epidemiological studies, we believe that the value of PPI in epidemiology will be better understood.
Reflection from a public and patient involvement Study Steering Committee member
The following is reflection on the PPI elements of CADDY from one of the study’s PPI SSC members:
It is impossible to summarise just how good the PPI aspects of the CADDY project have been. Right from the start, George and Clare recognised the importance of involving ‘real’ people, with direct experience of dementia, at every stage of the project. CADDY was refined to reflect the comments and concerns expressed by us all (not just those who spoke the loudest!), and, more importantly, we could actually see how our suggestions and comments were being incorporated/used to shape the project as it moved forward – particularly when it came to investigating and reporting back on the ‘why’ aspects of the research data and findings. Statisticians may love their numbers, and of course they are an important part of the picture (it is often useful to know how often something happens), but ordinary, ‘real’ people usually want to know why something happens: what can I/we learn from that? How is that relevant to me/my loved ones/our everyday lives? The CADDY findings and final report make it ‘real’. As one of the PPI representatives on the project’s Steering Committee, I was also able to contribute to the project on a more strategic level (in terms of governance, ethics and transparency, etc.) and once again my comments were well received and acted upon, and so that also demonstrates true partnership working. Whilst it is clear that the CADDY project has undoubtedly benefited from its PPI involvement, I think it is also fair to say that being able to contribute so meaningfully to a project has also benefited the PPI people involved: being able to share our insights, experiences and concern(s), and then actually be able to see that they have been taken on board/incorporated into such an important piece of research work, is very empowering and uplifting. I have been involved in PPI for almost 15 years now, and without doubt the CADDY project, and working with the CADDY team, is the best PPI experience I have ever had: if ever there was a ‘model of best PPI practice’ the CADDY project is it.
Chapter 13 Dissemination and impact
Introduction
The CADDY dissemination plan was designed to lead to both academic and societal impact from research. Specific activities planned or completed are academic publications, participation in academic conferences and an event to encourage broad discussion on dementia diagnosis across stakeholder groups. We are also working closely with the public policy section of the Alzheimer’s Society.
Conferences were selected to enable discussion of preliminary results with the international dementia and UK primary care research communities. Hence, in 2017, early findings were presented at the Alzheimer’s Association International Conference, the Alzheimer’s Society Research and Practice Conference, the Society of Academic Primary Care and the Dementias 2018 conference in London. Early findings were also shared locally through an Alzheimer’s Research UK public event held at UEA and with PPI groups. CADDY has also been presented at conferences: AgeNet, University of Hertfordshire, UK, 2018; Alzheimers Europe, Barcelona, Spain, 2018; Dementias 2019, London, UK, 2019; Contemporary Populations and Dementia, What Have we Learnt and Where are we Going? Alzheimer’s Disease Forum, Tasmania, Australia, 2019; Dementia Care Research Centres & Dementia Training Australia (part of the Government’s Australia Dementia Research Institute), Webinar, Australia, 2019.
Impact objectives
Broad impact objectives are summarised in Table 9.
Impact objective | Relevant CADDY finding/dissemination activity |
---|---|
Evidence the national conversation and policy around timely diagnosis of dementia | Highlighting the lack of quantitative evidence for the supposed benefits of diagnosis |
Inform the design and delivery of local action plans aimed to improve the process of diagnosis | Describing the population with dementia that is not recorded in primary care |
Raise public awareness of dementia | Dissemination events, social media, news media, work with the Alzheimer’s Society |
For researchers studying dementia in populations |
Description of the population with undiagnosed dementia and their clinical and social characteristics to support those designing and assessing the feasibility of future studies of dementia diagnosis services Evaluation of dementia diagnoses in primary care records as a proxy for dementia in the population, hence enabling researchers to develop better models to correct for misclassification of dementia |
Dissemination event
An event was held in London in December 2017 with the main aim of promoting the study findings among relevant stakeholder groups, including patients and the public, health-care professionals, local and national policy-makers and managers, and academic researchers in related areas. The event was participatory to ensure that the views of each stakeholder group and not just researchers were aired, and so that research teams could gauge reaction to their early findings from a diverse audience to help dissemination of final results. This event was called ‘Dementia Diagnosis in Primary and Secondary Care: Policy and Practice’ and was held at the Royal College of General Practitioners, London.
To maximise engagement with key stakeholders, the event was widened to other research studies beyond the CADDY. Speakers were invited to represent three other active studies on dementia diagnosis, in order to build an attractive and engaging forum. The findings of each study were complementary and this promoted (1) good exposure for each study to a wider audience; (2) more incentive and better value for delegates attending; (3) a research community networking opportunity; and (4) opportunity for each study to inform the others.
Practical considerations to encourage attendance were (1) using a central London venue; (2) securing influential speakers; (3) making attendance free of charge, with some support available for travel for specific delegates; (4) providing a coherent dementia diagnosis focus; (5) securing continuing professional development accreditation; and (6) employing social media, personal and professional contacts to promote the event.
Programme
Professor Alistair Burns, NHS England’s National Clinical Director for Dementia and Older People’s Mental Health, set out the national context for dementia diagnosis. This was followed by research presentations. Two hours was set aside for a workshop and expert panel. The workshop was followed by a talk from a well-known expert, by experience, who is living with a diagnosis of dementia, a presentation from Dr Jill Rasmussen, the Royal College of General Practitioners clinical dementia champion, and a short closing talk.
Delegates and presentations
Seventy-six people attended the event: eight health-care commissioners, six PPI members, 23 health-care professionals from primary, secondary and domiciliary care, 11 policy-makers, 22 academics, one journalist and three people supporting event management, and two representatives from the NIHR.
The other research studies, all of which had connections to UEA, contributing presentations were CASCADE (Dementia Case Finding), PERFECTED (Peri-operative Enhanced Recovery hip fracture Care of patients with dementia) and PRIDE (Promoting Independence in Dementia).
Workshop and expert panel
We made the event participatory by building both a workshop and generous discussion opportunities into the programme. An expert panel included researchers, people with dementia and their carers, and health-care professionals.
Leading up to the event, topics were proposed for the workshop and delegates were asked to select those they were most interested in discussing or suggest alternatives. Three topic areas were identified for discussion. These were (1) identifying and overcoming barriers to diagnosis, (2) what makes a good diagnosis of dementia and (3) how can we improve post-diagnostic support.
Each topic area was discussed for 20 minutes by delegates in discussion groups of 8–10. For each topic area, groups fed back key discussion points, the panel of experts were invited to respond and the discussion was returned to the floor. Rolling summarised feedback was typed directly onto the screens by a facilitating researcher. After the event, discussion points were collated into one document and themes were identified and summarised for each topic area.
Cost of the event
The total event cost £15,800, including venue hire; travel, subsistence and some accommodation; catering and information technology support (including filming of the event); and advertising and delegate packs. Costs were supported by project grants from the consortium and leveraged additional impact funding from the UEA.
Evaluation of the event
Evaluation by delegates
The event was evaluated via feedback from participants. Twenty-six participants completed event evaluation forms, with mean scores of 8.7 out of 10 for both ‘Did you find the event valuable?’ and ‘Did the event meet your expectations?’. Participants acknowledged the expertise, research quality and new knowledge. They also appreciated being able to network with other professionals and people with dementia knowledge and our consortium approach.
Participants appreciated the range and diversity of speakers, the range and accessibility of presentation topics, being able to participate in each presentation and the opportunity to discuss complex areas of dementia with generous time for questions.
Suggestions for improvement included access to presentations prior to the session, handouts supplied to aid sharing, and information on delegate roles and contact details. Some thought it may have been better to have given group discussions more time and to give more time to discussing solutions rather than issues.
Summary and future impact activities
We have used conference presentation and our own dissemination event to begin to generate interest in the study and refine our final analyses and outputs. Dissemination of CADDY findings and resulting translation into public awareness, policy and practice will take place following the conclusion of the study. Future research will be generated by the CADDY data set that will be made available to researchers.
One of our impact objectives is to evidence the national conversation and policy around timely diagnosis of dementia. The Dementia Diagnosis in Primary and Secondary Care: Policy and Practice meeting highlighted the lack of quantitative evidence for the supposed benefits of diagnosis. Since then we have had an ongoing conversation with NHSE regarding dementia screening in hospitals via the Dementia Assessment and Referral data collection, a national statistic (formerly a CQUIN), under which three measures are reported: the number and proportion of patients aged ≥ 75 years admitted as an emergency for more than 72 hours who (1) have a diagnosis of dementia or delirium or to whom case finding is applied, (2) if identified as potentially having dementia or delirium, are appropriately assessed, and (3) where the outcome was positive or inconclusive, are referred on to specialist services. NHSE have since received all the necessary internal approvals to retire the Dementia Assessment and Referral subject to public consultation (summer 2019).
We continue to liaise with dementia advocacy groups including Alzheimer’s Research UK and the Alzheimer’s Society with a view to raising public, clinical and policy-maker awareness of non-memory symptoms of dementia through public awareness campaigns.
Our next steps are to translate the study findings into academic papers, liaise with charities to translate the findings into local and national policy, and prepare secondary outputs that are accessible to all stakeholders.
Chapter 14 Discussion
To the best of our knowledge, CADDY is the largest study of undiagnosed dementia in the Western world conducted to date. Linking epidemiological data to medical records has enabled a rich set of comparisons to advance knowledge of the population with undiagnosed dementia and the impact of dementia diagnosis, including understanding of the dementia diagnosis process for people who have dementia but have not yet received a formal diagnosis. This will inform future policy and practice around dementia diagnosis.
Summary of findings
We successfully ascertained the details of dementia diagnoses, cognitive concerns and referrals to memory services recorded in primary care of a cohort of 449 people independently assessed to have dementia between 2008 and 2011 (W1) and 2011 and 2013 (W2).
Distribution of primary care diagnosis status among people with and without dementia
The proportion of people with dementia who had a diagnosis recorded in primary care rose from 34.8% to 43.1% between CFAS II W1 (2008–11) and W2 (2011–13). This difference was statistically significant in a multivariable analysis (OR 1.68, 95% CI 1.07 to 2.64). At both time points, around 21% of people with dementia had a cognitive concern or referral for cognitive concern noted, but had not received a diagnosis. Hence, a substantial but falling proportion of people with dementia had no record of any concern regarding cognitive function, referral or diagnosis in their medical record. There were very few people with a diagnosis of dementia in the primary care record who did not meet the CFAS II criteria for dementia.
Among people with dementia, having a recorded diagnosis was very strongly associated with increased severity of impairment in memory and orientation, but not with severity of impairment in other cognitive domains. Likewise, an informant’s perception of a participant’s memory impairment is a substantial independent predictor of diagnosis (independent of objectively measured cognitive impairment), as is the number of non-memory-related symptoms of dementia.
People with dementia living with a long-term partner were twice as likely to be diagnosed than those in other living arrangements (OR 2.20, 95% CI 1.20 to 4.01 compared with those in a residential care setting) and to have a higher probability of help-seeking (OR 1.97, 95% CI 1.09 to 3.55). People with dementia aged ≥ 90 years were less likely to be diagnosed than those in younger age groups. There was no evidence that the likelihood of diagnosis was independently linked to area-level deprivation or maximum educational attainment of the participant. With respect to comorbidity, there is some evidence that comorbid anxiety is associated with twice the odds of being diagnosed, but there was no effect of comorbid depression, and there is some evidence that cardiovascular comorbidity is inversely associated with diagnosis.
Although men with dementia were less likely to be diagnosed than women, this difference is not statistically significant after controlling for other demographic and clinical factors. The effect of age (as a continuous variable) was seen in multivariable models, suggesting that severity of dementia, living arrangement and comorbidity do not account for this effect of age on diagnosis and that there is another unmeasured factor underlying the effect of age on the probability of diagnosis.
The median time to clinical diagnosis from the time at which a participant meets clinical criteria for dementia is around 3.5 years. However, this time varies greatly; many were diagnosed close to when they first met the criteria for dementia, but around 20% were alive and undiagnosed more than 6 years after this time. Analysis of data on the time to new diagnosis among those who were undiagnosed at their CFAS II assessment suggests that men, those in the youngest and oldest age groups and those in residential care were least likely to become diagnosed.
There was no evidence that being diagnosed affected subsequent mortality or delayed the move to a residential care setting. Data on changes in social contact, mood and inpatient hospital visits were limited; there were not enough data to reliably estimate the effect of diagnosis on social participation, generalised anxiety, health-related worry, or the number and length of inpatient hospital visits.
Strengths and limitations
This study was based on a large population-representative cohort with an objective and independent dementia status determined using a validated algorithm, and with data on a wide range of other social- and health-related characteristics. It is the largest study to examine prevalence, causes and consequences of diagnosis among people with dementia. However, the study does have several important limitations.
Although the response rate from general practices was very high, a substantial proportion of participants with moderate to severe dementia at W1 could not consent to the use of their medical records and CFAS II itself has a response rate of 56%. Weights and multiple imputation were used, as appropriate, to reduce the impact of these missing data on estimates.
Diagnosis status was extracted from primary care records by practice staff or CRN nurses. Although the data were validated with respect to the correct individual being identified, data entry was not independently verified.
Few participants fell into the ‘referred’ and ‘cognitive concern’ groups that were not diagnosed but had some record of cognitive concern in their primary care record, and so it was difficult to draw strong conclusions about these groups, or to identify separate predictors of help-seeking and diagnosis conditional on help-seeking. Likewise, the sample size for estimation of predictors for those with undiagnosed dementia but diagnosis in the subsequent 2 years is also small (based on only 66 new diagnoses).
For some analyses, the numbers of people with dementia falling into many of the categories were small; hence, the power to detect subtle associations is limited, particularly for risk factors that were rare in the data (e.g. very high levels of comorbidity or social participation).
The CFAS assessments covered a wide range of personal circumstances. However, with the exception of cognitive testing, each of the risk factors we tested relied on the report of the participant themselves, a proxy or an informant for their ascertainment. People with dementia may not have answered all questions correctly or have been able to provide any response at all. In some cases, only limited cognitive testing is available, with informant questionnaire providing much of the missing data where possible. Some of the variables of interest were taken from the informant questionnaire and were missing for many people. The CFAS assessment covered many aspects of health and well-being, but many areas were not covered in depth, including, for example, the quality and nature of social interaction.
A strength of CFAS II is its inclusivity, meaning that those with very severe cognitive impairments and people living in care settings are included. However, people with severe dementia are likely to be unable to complete cognitive assessments or interviews and so covariate data are often missing for this group.
We assumed throughout that the CFAS II classification of dementia status truly represents whether or not a person had dementia on the date of their interview. Although the CFAS II algorithm is validated against DSM-IIIR diagnostic criteria, it does not include a complete clinical workup and it is possible that some individuals are misclassified. A substudy of the first MRC CFAS study was conducted to validate this algorithm (Lu Gao, personal communication) and found that CFAS diagnosis of dementia is specific, in that those classified as having dementia are very likely to have dementia, but may not be sensitive, in that some cases of dementia might not be detected. For our purpose, this specificity is important, in that we are confident that the vast majority those identified in CADDY as having dementia do so. However, our ‘control’ sample of those without a CFAS diagnosis may have included some people with dementia and our control sample was not large enough to detect a small prevalence of false-positive dementia diagnoses. In any case, the threshold for dementia varies considerably with definition and the severity of symptoms fluctuates within individuals, so to attempt to be definitive about whether or not a person has dementia at any particular time is not meaningful, particularly in the milder stages.
Similarly, we have assumed that the primary care record represents what is known in health-care services with respect to a person’s cognitive status. There is evidence that some dementia is diagnosed and recorded in hospital records that does not appear in primary care record. It is also possible that cognitive impairment is recognised and managed without being coded in a patient’s notes. However, maintaining a dementia register has been an aspect of the GP contract monitored through the Quality and Outcomes Framework and there is a financial incentive for its maintenance, so it is likely to be a relatively good reflection of a GP’s knowledge of a patient.
Comparison with previous literature
Prevalence of diagnosis
A systematic review of the global prevalence and determinants of undetected dementia from worldwide studies discovered 23 studies, published between 1988 and 2015, linking standardised assessments of dementia to diagnoses recorded in primary care, 21 of which were conducted in Europe or North America. 11 The average proportion of people with dementia who were undiagnosed across studies was 61.7% (95% CI 55% to 68%), although heterogeneity across studies was high (I2 = 96.4%) (Figure 15). Only two single-site studies had been conducted in England: one in Cambridge in 1998, among 208 people with dementia recruited as part of the Cambridge City over 75 Study;17 and the other in Newcastle in 2009, among 105 people from six general practices determined to have dementia on the basis of MMSE score alone (that is without using a validated dementia assessment), recruited as part of the Newcastle 85+ study. 44 Both studies are consistent with our results. Most recently, the National Health and Aging Trends Study45 in the USA found that, in 2011, in a study of 585 people with dementia, 39.5% were undiagnosed (based on diagnoses in their Medicare records) and 19.2% were diagnosed but were unaware of their diagnosis.
FIGURE 15.
Meta-analysis of estimates of the proportion of people with dementia who do not have a recorded diagnosis in primary care. See Lang et al. 11 for full reference details of these studies. Reproduced with permission from Lang et al. 11 This is an Open Access article distributed in accordance with the terms of the Creative Commons Attribution (CC BY 4.0) license, which permits others to distribute, remix, adapt and build upon this work, for commercial use, provided the original work is properly cited. See: http://creativecommons.org/licenses/by/4.0/.
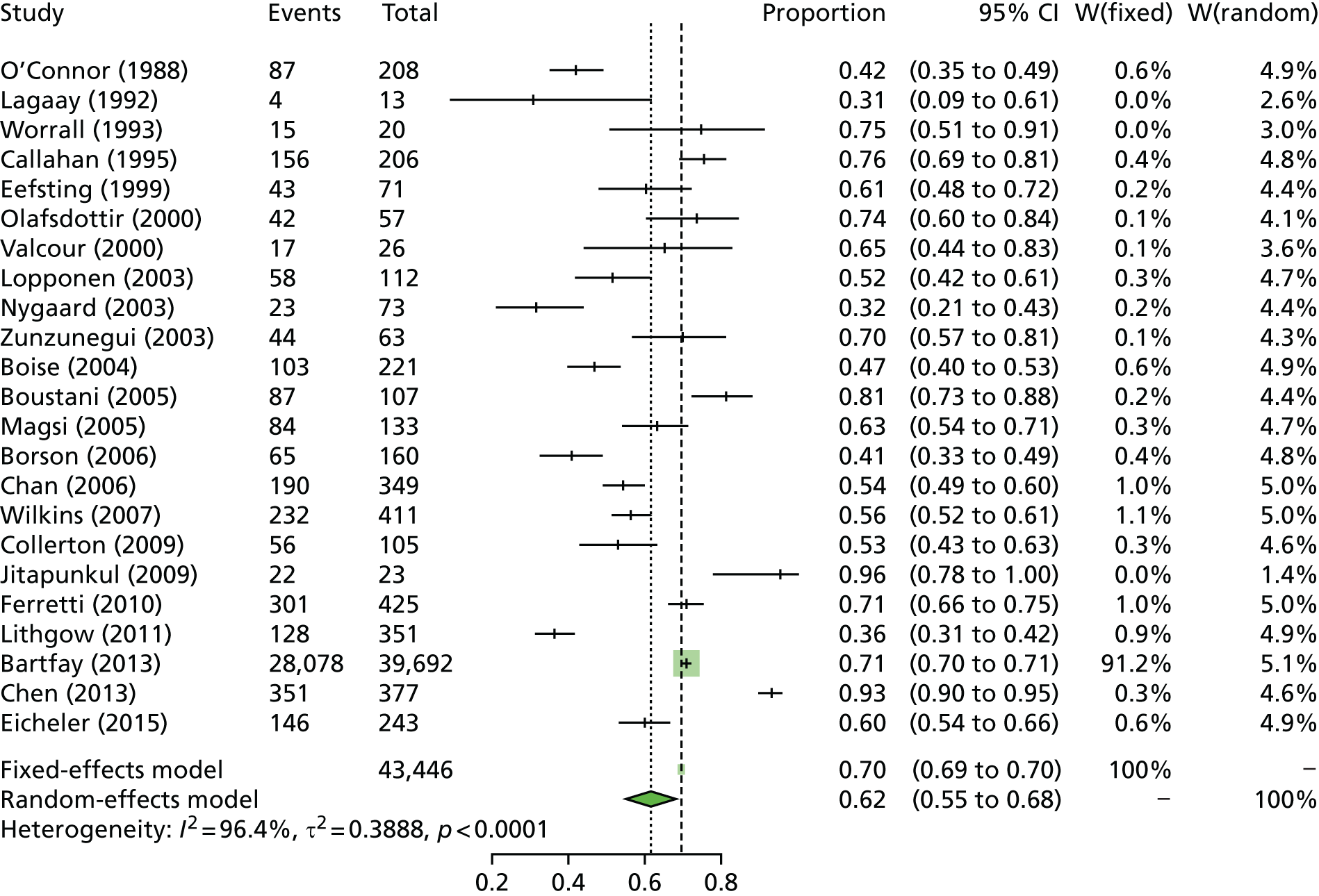
Official estimates of the proportion of people with dementia who are diagnosed are usually calculated by dividing the number of recorded cases by the expected number of dementia cases in the population, which is in turn derived using CFAS II estimates for age- and sex-specific prevalence. A comparison of the proportion calculated in this way across the years from 2006 to 2012 in England and Wales shows a stable proportion of around 36% between 2006 and 2009, then a steady increase of 2% per year between 2009 and 2012. 46 This is consistent with our estimate of 35% in 2008–11 and 43% in 2011–13.
Prevalence of concern and referral
Our results for the proportion with undiagnosed dementia are consistent with official statistics for the same periods, but official statistics do not include data on the group of people with no diagnosis but with some note of a cognitive concern or a referral in their record. This was partly addressed by O’Connor et al.,17 who reported the proportion of cases with possible as well as definite dementia, and found that, although only 22% of people with dementia were reported as having ‘definite dementia’ by their GP, this number rose to 58% of people when ‘possible dementia’ records are also included. It is possible that recent apparent increases in the proportion of people diagnosed with dementia are at least in part attributable to a reclassification, to ‘definite dementia’, of people who would otherwise be recorded as possibly or probably living with dementia.
Findings from the Three-City Study47 of 9294 participants from Bordeaux, Dijon and Montpellier showed that, in 2005, 34.4% of 253 people with incident dementia had not consulted any physician about their condition, 34% had consulted only their GP and 31.6% had consulted a specialist (in France referral to a consultant is usually via a GP), indicating that 65.6% of people were in the diagnostic process (including those diagnosed). Our results indicated that 65% of people with dementia were in the diagnostic process (including diagnosed). Around 21% of people with dementia were in the diagnostic process but had not received a diagnosis. Of these, half had been referred to specialist services. These findings are also broadly consistent with our findings that, in 2011–13, approximately one-third (37%) of people with dementia in our study were unknown to their GP, with respect to dementia or cognitive impairment.
Severity and nature of cognitive impairment
Dementia severity is consistently positively associated with the chance of being diagnosed. 11,23 To our knowledge, only one previous study45 has disaggregated domains of cognitive impairment to explore which specific impairments are most likely to lead to diagnosis, or which dementia subtypes are least likely to be diagnosed. Diagnostic accuracy and awareness of different symptoms may play a role in the association between the severity and nature of cognitive function and diagnosis; a meta-analysis of physician accuracy identified severity of dementia as the main predictor for accurate diagnosis. 48
Age
We found a consistent gradient such that older people with dementia were less likely to be diagnosed than younger people with dementia, with the exception of the youngest group studied (age 65–70 years), who were also relatively unlikely to have a diagnosis. Many previous studies also suggest that older people with dementia are less likely to be diagnosed than younger people. 11
Socioeconomic status
Low socioeconomic status is a known risk factor for dementia, as well as for other chronic diseases and mortality. We found no evidence that the chance of diagnosis among people with dementia differed across groups classified by educational attainment or by area-level deprivation (as measured by Townsend index). NHS Digital reports a higher ‘diagnosis rate’ among those in the lowest decile of deprivation (74.4%, 95% CI 66.9% to 80.6%) than those in the highest decile of deprivation (64.4%, 95% CI 58% to 69.8%),13,49 but this could reflect a failure to correctly adjust the expected prevalence across areas to take into account socioeconomic status. Direct estimates, such as those reported in the current study and based on cohorts known to have dementia, would not suffer the same bias, as such studies consistently show no effect of educational attainment (a useful proxy for socioeconomic status) on dementia diagnosis. 11 There is no difference in the level of health-care services received by people with diagnosed dementia across regions of the UK stratified by Townsend deprivation level. 50 Together, this suggests that there is no substantial social inequality in access to dementia diagnoses or dementia services in England.
Living arrangement
Those living with a long-term partner are more likely to be diagnosed than those living alone or with others; this finding is repeated in other studies of dementia and other chronic diseases. 11,51 Within residential care settings in England, dementia prevalence is around 65%. 16 Our results have shown that many such cases did not have a formal record of dementia in their primary care notes, and, once in a residential care setting, having a new diagnosis recorded is unlikely. Although it is likely that dementia is recognised and managed in many of these cases, a study in south-east England found that nursing home staff did not recognise dementia among their residents in more than half of cases,52 suggesting that much of the undiagnosed dementia in residential care settings will be unrecognised by staff. The recent review by Lang et al. 11 stratified studies into those conducted in the community and those conducted in care settings. Although none of the included studies of the prevalence of undetected dementia in care setting was conducted in the UK, globally those studies conducted in care homes report an average proportion diagnosed of 50% compared with 36% in community settings.
Comorbidity
Dementia is often complicated by frailty and multiple comorbidities, particularly among the oldest. Our findings suggest that cardiovascular comorbidity was inversely related to diagnosis, with those reporting three or more cardiovascular conditions much more unlikely to have received a diagnosis than others. However, no such association was seen for other comorbidities, and very few people with dementia reported three or more cardiovascular conditions. The association between dementia diagnosis and comorbidity has not been extensively studied elsewhere;11 a study of a cohort with cognitive impairment referred to a functional impairment support programme in the USA found a higher rate of hypertension among the non-diagnosed, but no other difference in comorbidity profile. 53
Carers
The presence of neuropsychiatric symptoms in older people and people with dementia is related to increased stress54 and distress in caregivers. 55 We found a positive association between informant reports of both cognitive and non-cognitive symptoms of dementia with the chance of being diagnosed.
Benefits or harms of diagnosis
There is very little quantitative evidence to support claims of benefits or harms of diagnosis, but in recent years some studies have emerged from epidemiological studies or routine hospital data comparing outcomes between those with known and recorded dementia and others whose cognitive impairment is first detected though screening on hospital admission or in a research setting.
Mortality
We found no evidence that people with diagnosed dementia live longer than those with undiagnosed dementia. Consistent with this, an analysis of 253 cases of incident dementia in France found that, compared with those who did not consult a medical practitioner, survival among those who consulted their GP was lower (HR 1.51, 95% CI 0.99 to 2.30) and survival among those who consulted a specialist was significantly lower (HR 1.85, 95% CI 1.21 to 2.82). 56
Move to residential care setting
Recent findings from the Three-City Study27 and the Swedish National study on Aging and Care in Kungsholmen (SNAC-K)28 suggest that early help-seeking is associated with a higher rate of subsequent move to a residential care setting. Our study is consistent with this higher rate of transfer to institutional care among those with a diagnosis than among those without. Although adjusted for cognitive function and demographic factors at baseline, residual confounding cannot be ruled out. Nevertheless, no study has found any evidence of diagnosis leading to a delay in moving to residential care among people with dementia.
Inpatient hospital visits
We found no clear evidence to suggest that receiving a diagnosis of dementia prevents hospital admission or reduces overnight stays. A study based on the Adult Changes in Thought Study57 showed that people with diagnosed dementia used more health care over the previous 2 years than those with undiagnosed dementia, but the study did not adjust for any other patient characteristics, such as severity of dementia or comorbidity. The same study57 found that those with undiagnosed dementia use more health care than healthy control subjects and suggested that ‘identifying’ people with undiagnosed dementia might prevent some of this excess; however, no evidence was provided to support this.
With respect to outcomes following hospital admission, a study of routine data from a single hospital in England between 2014 and 2015 showed that patients with cognitive impairment and dementia have worse outcomes following hospital admission than people without any cognitive impairment, and that inpatient mortality and length of stay are moderately higher for people with ‘cognitive impairment’ detected by screening on admission than for those with previously diagnosed dementia. 58 Although this may imply that hospital interventions put in place for those with diagnosed dementia could lower mortality and length of stay, there was no adjustment for other patient characteristics, and it is possible that undiagnosed cognitive impairment represented a more acute problem, such as delirium. Other hospital-based studies in Scotland and Ireland have confirmed that those with cognitive impairment on hospital admission have worse outcomes than those without, and that a substantial proportion of those with cognitive impairment have no prior diagnosis, but find no difference in mortality or length of stay between those with or without a diagnosis. 59,60
Meaning and implications
Our estimate for the proportion of people with dementia who have a GP-recorded diagnosis align closely with those reported by NHS Digital in the same time periods. This suggests that the indirect approach taken by the NHS to calculating the dementia ‘diagnosis rate’ was broadly accurate at the national level. However, the indirect approach does appear to suggest a socioeconomic gradient in diagnosis that was not seen in epidemiological studies (including CADDY), suggesting that, at the regional level, the estimate of the numbers of people with dementia might not be accurate with respect to comparison between regions.
An important implication of this study is that people with dementia but without the memory impairments characteristic of Alzheimer’s disease are less likely to have a primary care-recorded diagnosis than those whose dementia primarily affects their memory. Our analyses also highlight some particular demographic circumstances that may delay dementia diagnosis. The youngest and oldest age groups appear less likely to be diagnosed, as are those not living with a spouse or other long-term partner. Our findings also suggest that dementia may go undiagnosed among those with many other comorbid conditions. There was no evidence for a difference in the factors that determine the presence of a cognitive concern in a patient’s record or a diagnosis given that cognitive concern.
There are many possible reasons for GPs being unaware of cognitive impairment among their patients. Many psychosocial factors among patient, carer and health-care professionals contribute to a diagnosis of dementia. 22 Even when patients do present to their GPs, GPs’ decisions to diagnose and to communicate a diagnosis are influenced by their own beliefs, patient circumstances, health system access and cultural norms. 61 For example, a multicentre randomised controlled trial of an intervention to improve GP diagnosis rates by empowering people from the general population in the south-east of England aged ≥ 70 years to seek help from their GP if they were concerned found that the intervention did not increase diagnosis rate. 62
Evidence suggests that some GPs recognise and manage patients as having dementia without recording them in primary care record systems as having dementia. 19,48 It is possible that recent increases in the number of people diagnosed with dementia simply reflect an improvement in the coding of existing knowledge; further ongoing epidemiological studies such as CFAS and CADDY are needed to monitor this.
This work is also closely aligned with recommendations of the roadmap to advance dementia research in prevention, diagnosis, intervention and care by 2025,63 most specifically those of goal 2, which aims to maximise the benefits to people living with dementia and their families of seeking and receiving a diagnosis of dementia by helping to define what a ‘timely’ and ‘quality’ diagnosis of dementia means; to identify unmet needs for support and help; and to clarify differences in diagnosis rates and service usage.
In recent years there have been a number of initiatives to promote dementia recognition and management in primary care. 64 However, initiatives and financial incentivisation to increase diagnosis rates can lead to concerns about overdiagnosis or misdiagnosis. 30,65,66 Our study data collection took place before the introduction of incentives for dementia diagnosis, and we found no substantial evidence of people without dementia receiving dementia diagnosis. However it is important, with continuing pressure on local health authorities to diagnose more cases, that the risks and potential harm associated with overdiagnosis are monitored.
It has been suggested that missed diagnosis of dementia might contribute to an earlier move to residential care, or, conversely, that diagnosing more people with dementia could delay admission to residential care services. 10 Yet the only evidence in support of this claim is the fact that some psychosocial interventions, if optimally applied, have been found to delay a move to care settings, followed by the supposition that increased diagnosis would automatically increase provision and optimal uptake of these interventions by the people who might benefit from them. It would also be necessary to assume that the benefits of diagnosis would apply equally among those who had sought help and those who had not. On the other hand, claims about the possible psychosocial or practical harms of dementia diagnosis are also often made without a direct quantitative evidence base to support them. This report highlights the lack of such evidence and the difficulty in obtaining it.
We found that people with dementia living in care homes were also likely to not have a formal diagnosis in their GP record. It is acknowledged that the usual routes to formal dementia diagnosis in England, that is memory clinics or specialist mental health services, may not be accessible or appropriate for people with more severe impairments living in residential care, which may have contributed to lack of formal diagnosis among this group. More emphasis has been placed on diagnosis in care homes in recent years and so it is likely that diagnosis rates have increased in this setting alongside those in the community. However, in common with the wider community of people living with dementia, the benefits and harms of diagnosis in long-term care settings remain poorly understood.
Although calls for dementia screening intensified around a decade ago, lately there has been an acknowledgement that early diagnosis of dementia might not be optimal for everybody and that more research is needed into when a diagnosis is timely (i.e. made at the right time for an individual). Recently, the World Alzheimer Report 2016 has acknowledged that screening for dementia cannot be recommended at present, owing to a lack of evidence of benefit and low uptake of screening programmes where they have been implemented at regional level. 67 To our knowledge, the only screening trial for dementia is yet to report; 4000 older people have been recruited to the Indiana University Cognitive Health Outcomes Investigation of the Comparative Effectiveness of dementia screening (IU-CHOICE) trial (ClinicalTrials.gov identifier: NCT01699503)68 and have undertaken a short cognitive function test, with onward referral where indicated and diverse outcomes, including quality of life, to be measured. In any case, it is likely that whether there is a net benefit or harm of diagnosis will depend enormously on the individuals being screened, how the screening and diagnosis process works and the quality of post-diagnostic support available. Rightly, it is now acknowledged that establishing good care and support for those already known to have dementia, and establishing the benefits of diagnosis, should be prerequisite ahead of expensive programmes aimed at detecting more cases. 31,69
Generalisability of the findings
This work is directly applicable to England. Although CFAS II was conducted in three diverse centres across England, the dementia diagnosis rate is likely to vary substantially across the country and so our estimates of the proportion without a diagnosis are unlikely to be directly applicable within regions. Although health services vary in terms of diagnostic pathways, incentives for diagnosis and awareness of dementia among the public and clinicians, many of the factors that lead people to seek help for symptoms of dementia, and the effects on people of receiving a diagnosis, are likely to be similar across the Western world. Policy changes, GP and public attitudes and awareness of dementia have changed in recent years and, as such, the overall prevalence of undiagnosed dementia and numbers of people with a false diagnosis are very likely to have changed in the years since the CFAS II interview data were collected. However, our findings on the benefits and harms of diagnosis and of the predictors of diagnosis are likely to be applicable today.
Conclusion
Dementia diagnosis rates identified under CADDY for the period 2008–13 are similar to those reported at the time nationally through NHS Digital. This provides confidence in the data provided to policy-makers and commissioners through NHS Digital, at least at the national level. We identified no evidence of overdiagnosis of patients with dementia and that around 20% of patients with dementia were not diagnosed but were in the diagnostic pathway at any one time.
Despite the limitations of this analysis, the associations between diagnosis and cognitive function appear robust. The major implication is that people with dementia but without the memory impairments characteristic of Alzheimer’s disease are much more likely to be undiagnosed than those whose dementia primarily affects their memory.
Our analysis also highlights some particular demographic circumstances that may delay dementia diagnosis, and so suggests areas in which health education and awareness campaigns could be directed if benefits of diagnosis were identified. The youngest and oldest age groups appear less likely to be diagnosed, as are those who have completed fewer years of education and those not living with a spouse or other long-term partner. Our findings also suggest that dementia may go undiagnosed in those with many other comorbid conditions.
Implications for practice
-
There is a gap for many people between having manifest signs of dementia and having a diagnosis of dementia recorded in primary care.
-
Non-memory presentations of dementia appear to be more readily overlooked. This could be evidence of a gap in public and clinical awareness of other symptoms of dementia. Campaigns to raise awareness of non-memory symptoms of dementia could be considered.
-
People with dementia aged ≥ 90, those living alone and those with comorbidity may also be underdiagnosed, and practitioners should be aware of the possibility of undiagnosed dementia among these groups.
Recommendations for research
-
At present, there is little objective quantitative evidence that obtaining a diagnosis confers any benefit on the individual. This may reflect the shortcomings of current post-diagnostic support, but also the lack of studies specifically designed to quantitatively explore the diagnostic pathway and estimate the effect of dementia diagnosis on patient outcomes. Further research with longer and more systematically measured follow-up post-diagnostic support than the current study is required to bridge this evidence gap.
-
Researchers should be keenly aware of the large average lag and large variation in the distribution of the time between dementia incidence and the time to diagnosis of dementia in primary care, particularly when first record of a dementia diagnosis is used as the basis for recruitment or as a proxy for dementia incidence in epidemiological studies.
Chapter 15 End notes
Study governance
The study was carried out at the UEA and the sponsor for this study was South Norfolk CCG.
The SSC met on four occasions. It was not independent of the research team. It was chaired by Professor Frances Bunn (University of Hertfordshire; independent member). Ordinary members were study co-applicants: George Savva, Antony Arthur, Carol Brayne, Tom Dening, Chris Fox, Fiona Matthews, Louise Robinson and Blossom Stephan. Other members were Judy Henwood (sponsor representative), Katy Blakely (independent local commissioner), Gavin Terry (Alzheimer’s Society Policy Division), Paul Millac, Abi Dennington-Price and Peter Richmond (PPI representatives) and Clare Aldus (researcher).
The Management Committee met on six occasions. Membership comprised study co-applicants (George Savva, Antony Arthur, Carol Brayne, Tom Dening, Chris Fox, Fiona Matthews, Louise Robinson and Blossom Stephan), representatives from the Norwich CTU (Martin Pond and Antony Colles), a sponsor representative (Clare Symms) and a researcher (Clare Aldus).
Ethics considerations and approvals
We sought data for patients who had dementia or cognitive impairment. We were mindful of the extremely sensitive nature of the data we sought and of the fact that many of the patients who had given permission for the use of their data had since died. To mitigate risk to patient data we discussed ethics issues with the SSC, our PPI advisory group and the sponsor representative, and we designed a system of data management to ensure confidentiality and accuracy of data.
The study received favourable opinion at first review on 29 April 2016 from the London-Bromley Research Ethics Committee, with HRA approval on 6 May 2016 (National Research Ethics Service 16/LO/0667; Integrated Research Application System Project ID 186510; protocol v1.0 08032016).
We gained HRA approval for a minor amendment (CADDY minor amendment 1; protocol v1.1 5 January 2017), to enable members of the CRN teams to retrieve records that had been returned to the patient record repository at PCSE (from 8 February 2017).
Study registration
This study is registered with the NIHR CRN Central Portfolio Management System (CPMS 30655).
Funding
This study was funded by the NIHR under their Health Services and Delivery Research programme. This study constitutes independent research and the views expressed in this report are those of the authors and not necessarily those of the NHS, NIHR or the Department of Health and Social Care.
Acknowledgements
Many people have contributed to this research and we are very grateful to them all. We would particularly like to thank the following for their participation and support.
We are most grateful to the people who took part in the CFAS II and who agreed that their medical records could be used as part of CFAS II research programme. Without them this study could not have taken place. We are also grateful to GPs and a range of other staff of general practices who provided us with data from medical records.
We are also very grateful for the generous contributions our PPI advisory group members have made to CADDY, including Peter Richmond, Lesley Evans, Amanda Wellings, Heather Edwards, Julian Ashton, Abi Dennington-Price, June Blythe, Jennifer Griffiths, Helen Jackson, Penny Vicary and Paul Millac. Their real-life experience and knowledge has provided an invaluable contribution to the success of the study. We would also like to thank PPI group co-ordinators Jacqueline Romero (PPIRes), Rhianna Broadway (inspire) and Matt Murray (Alzheimer’s Society), who have supported us and our members in this work.
We would like to thank the many stakeholder representatives who contributed to the December 2017 Dementia Diagnosis dissemination and impact event, and our speakers and guest experts Professor Alistair Burns, Dr Jane Fleming, Professor Fiona Poland, Mr Chris Roberts, Dr Jill Rasmussen, Mrs Jane Goodrick, Professor Steve Iliffe and Ms Xanthe Townend, who gave up their time to support this activity so generously.
We would like to thank members of our steering and management groups for their contribution to CADDY.
We are also grateful for the additional financial support we received from UEA and the University of Cambridge in support of the impact event, Dementia Diagnosis in Primary and Secondary care: Policy and Practice, and for the practical support from the UEA impact team.
We would particularly like to thank Patrick Daniel (Researcher Administrator) who so ably encouraged general practice staff to take part in the study and Suzanna Dell (Administrator) for their contribution to the success of this study.
We are also grateful for the support provided by members of the CFAS secretariat, particularly Linda Barnes (CFAS National Co-ordinator), Rachael Brooks (CFAS Project Manager) and Emma Green (CFAS Database Manager).
The sponsor for this study was NHS South Norfolk Clinical Commissioning Group. CFAS II has received funding from the UK Medical Research Council (G0601022; April 2008–November 2016) and Alzheimer’s Society UK (project grant number 294; November 2016–November 2018).
Contributions of authors
Clare F Aldus (Research Fellow, Health Sciences, UEA) was study manager (January 2016–September 2017) and chief investigator (October 2017–October 2018). She contributed to research design, managed the research and administrative team, led data collection processes and liaised with general practices. She also managed governance, including ethics approval, dissemination and impact creation, and PPI activity. She contributed to statistical design and analyses.
Antony Arthur (Professor of Nursing Science, UEA) developed the study concept with George Savva. He was co-applicant, contributed to study design and oversaw leadership of the study in the period October 2017 to March 2018. He was a member of study management and steering groups, contributing to all aspects of governance, research, dissemination and reporting. He contributed to the research design, statistical design and reporting.
Abi Dennington-Price (patient and service user representative) was a member of the SSC in a PPI advisory role and a member of the CADDY PPIAG. She contributed to governance, research design, dissemination and reporting.
Paul Millac (patient and service user representative) was a member of the SSC in a PPI advisory role and a member of the CADDY PPIAG. He contributed to governance, research design, dissemination and reporting.
Peter Richmond (patient and service user representative) was a member of the SSC in a PPI advisory role and a member of the CADDY PPIAG. He contributed to governance, research design, dissemination and reporting.
Tom Dening (Professor of Dementia Research, University of Nottingham) was a co-applicant and contributed to study design. He was a member of study management and steering groups, contributing to all aspects of governance, research and reporting. He contributed to research design, dissemination, statistical design and reporting.
Chris Fox (Professor in Psychiatry, UEA) was a co-applicant and contributed to study design and the funding application. He was a member of study management and steering groups, contributing to all aspects of governance, research and reporting. He contributed to research design, dissemination, statistical design and reporting.
Fiona E Matthews (Professor of Epidemiology, University of Newcastle) was a co-applicant and contributed to study design and the funding application. She was a member of study management and steering groups, contributing to all aspects of governance, research and reporting. She contributed to research design, dissemination and reporting, with particular focus on statistical design.
Louise Robinson (Professor of Primary Care and Ageing, Regius Professor of Ageing, University of Newcastle) was a co-applicant and contributed to study design and the funding application. She was a member of study management and steering groups, overseeing all aspects of governance, research and reporting. She contributed to research design, dissemination, statistical design and reporting, with particular focus on dementia in primary care.
Blossom CM Stephan (Senior Lecturer, University of Newcastle) was a co-applicant and contributed to study design. She was a member of study management and steering groups, overseeing all aspects of governance, research and reporting. She contributed to research design, dissemination, statistical design and reporting.
Carol Brayne (Professor of Public Health Medicine, University of Cambridge, and Principal Investigator for CFAS) was responsible for developing the concept of CFAS II, which was pivotal to this work. She was a co-applicant and contributed to study design and the funding application. She was a member of study management and steering groups, overseeing all aspects of governance, research and reporting. She contributed to research design, dissemination, statistical design and reporting.
George M Savva (Institute Statistician; Quadram Institute Bioscience, formerly Senior Lecturer in Applied Statistics, UEA) was chief investigator for the study in the period January 2016 to September 2017. He developed the study concept, and led study design and the funding application. He built and led the study team, overseeing all aspects of governance, research and reporting. He led the statistical design and analyses for this study.
Data-sharing statement
We aim to share data with the scientific community with as few restrictions as possible. CADDY data are held by the CFAS secretariat and will be made available to researchers on request, subject to the approval of the CFAS II Oversight and Management Committee, in accordance with the process for data sharing current at the time of application. In the first instance, data requests should be submitted to the corresponding author. Access to anonymised data may be granted following review.
Patient data
This work uses data provided by patients and collected by the NHS as part of their care and support. Using patient data is vital to improve health and care for everyone. There is huge potential to make better use of information from people’s patient records, to understand more about disease, develop new treatments, monitor safety, and plan NHS services. Patient data should be kept safe and secure, to protect everyone’s privacy, and it’s important that there are safeguards to make sure that it is stored and used responsibly. Everyone should be able to find out about how patient data are used. #datasaveslives You can find out more about the background to this citation here: https://understandingpatientdata.org.uk/data-citation.
Disclaimers
This report presents independent research funded by the National Institute for Health Research (NIHR). The views and opinions expressed by authors in this publication are those of the authors and do not necessarily reflect those of the NHS, the NIHR, NETSCC, the HS&DR programme or the Department of Health and Social Care. If there are verbatim quotations included in this publication the views and opinions expressed by the interviewees are those of the interviewees and do not necessarily reflect those of the authors, those of the NHS, the NIHR, NETSCC, the HS&DR programme or the Department of Health and Social Care.
References
- World Health Organization (WHO) . Dementia: A Public Health Priority 2012.
- White LR, Edland SD, Hemmy LS, Montine KS, Zarow C, Sonnen JA, et al. Neuropathologic comorbidity and cognitive impairment in the Nun and Honolulu-Asia Aging Studies. Neurology 2016;86:1000-8. https://doi.org/10.1212/WNL.0000000000002480.
- Savva GM, Wharton SB, Ince PG, Forster G, Matthews FE, Brayne C. Medical Research Council Cognitive Function and Ageing Study . Age, neuropathology, and dementia. N Engl J Med 2009;360:2302-9. https://doi.org/10.1056/NEJMoa0806142.
- Alzheimer’s Society . Dementia UK: Update 2014.
- Savva G, Brayne C, Weiner MF, Lipton AM. Textbook of Alzheimer’s Disease and Other Dementias. Washington, DC: American Psychiatric Association Publishing; 2009.
- Matthews F, Brayne C. Medical Research Council Cognitive Function and Ageing Study Investigators . The incidence of dementia in England and Wales: findings from the five identical sites of the MRC CFA Study. PLOS Med 2005;2. https://doi.org/10.1371/journal.pmed.0020193.
- Alzheimer’s Disease International . World Alzheimer Report 2009: The Global Prevalence of Dementia 2009. www.alz.co.uk/research/files/WorldAlzheimerReport.pdf (accessed 26 June 2019).
- NHS England . Dementia n.d. www.england.nhs.uk/mental-health/dementia/ (accessed 3 May 2018).
- Fox C, Lafortune L, Boustani M, Brayne C. The pros and cons of early diagnosis in dementia. Br J Gen Pract 2013;63:e510-2. https://doi.org/10.3399/bjgp13X669374.
- Prince M, Bryce R, Ferri C. World Alzheimer Report 2011: The Benefits of Early Diagnosis and Intervention 2011. www.alz.co.uk/research/WorldAlzheimerReport2011.pdf (accessed 26 June 2019).
- Lang L, Clifford A, Wei L, Zhang D, Leung D, Augustine G, et al. Prevalence and determinants of undetected dementia in the community: a systematic literature review and a meta-analysis. BMJ Open 2017;7. https://doi.org/10.1136/bmjopen-2016-011146.
- World Health Organization (WHO) . Global Action Plan on the Public Health Response to Dementia 2017–2025 n.d. www.who.int/mental_health/neurology/dementia/action_plan_2017_2025/en/ (accessed 4 May 2018).
- NHS Digital . Dementia: 65+ Estimated Diagnosis Rate n.d. https://digital.nhs.uk/data-and-information/national-indicator-library/dementia-65-estimated-diagnosis-rate (accessed 26 June 2019).
- Brayne C, McCracken C, Matthews FE. Medical Research Council Cognitive Function and Ageing Study (CFAS) . Cohort profile: the Medical Research Council Cognitive Function and Ageing Study (CFAS). Int J Epidemiol 2006;35:1140-5. https://doi.org/10.1093/ije/dyl199.
- Erkinjuntti T, Ostbye T, Steenhuis R, Hachinski V. The effect of different diagnostic criteria on the prevalence of dementia. N Engl J Med 1997;337:1667-74. https://doi.org/10.1056/NEJM199712043372306.
- Matthews FE, Arthur A, Barnes LE, Bond J, Jagger C, Robinson L, et al. Medical Research Council Cognitive Function and Ageing Collaboration . A two-decade comparison of prevalence of dementia in individuals aged 65 years and older from three geographical areas of England: results of the Cognitive Function and Ageing Study I and II. Lancet 2013;382:1405-12. https://doi.org/10.1016/S0140-6736(13)61570-6.
- O’Connor DW, Pollitt PA, Hyde JB, Brook CP, Reiss BB, Roth M. Do general practitioners miss dementia in elderly patients?. BMJ 1988;297:1107-10. https://doi.org/10.1136/bmj.297.6656.1107.
- Eefsting JA, Boersma F, Van den Brink W, Van Tilburg W. Differences in prevalence of dementia based on community survey and general practitioner recognition. Psychol Med 1996;26:1223-30. https://doi.org/10.1017/S0033291700035947.
- Donegan K, Fox N, Black N, Livingston G, Banerjee S, Burns A. Trends in diagnosis and treatment for people with dementia in the UK from 2005 to 2015: a longitudinal retrospective cohort study. Lancet Public Health 2017;2:e149-e156. https://doi.org/10.1016/S2468-2667(17)30031-2.
- NHS Digital . Recorded Dementia Diagnoses, January 2018 2018. https://digital.nhs.uk/data-and-information/publications/statistical/recorded-dementia-diagnoses/january-2018 (accessed 12 July 2017).
- National Audit Office . Improving Service and Support for People With Dementia 2007. www.nao.org.uk/wp-content/uploads/2007/07/0607604.pdf (accessed 26 June 2019).
- Bunn F, Goodman C, Sworn K, Rait G, Brayne C, Robinson L, et al. Psychosocial factors that shape patient and carer experiences of dementia diagnosis and treatment: a systematic review of qualitative studies. PLOS Med 2012;9. https://doi.org/10.1371/journal.pmed.1001331.
- Bradford A, Kunik ME, Schulz P, Williams SP, Singh H. Missed and delayed diagnosis of dementia in primary care: prevalence and contributing factors. Alzheimer Dis Assoc Disord 2009;23:306-14. https://doi.org/10.1097/WAD.0b013e3181a6bebc.
- Koch T, Iliffe S. EVIDEM-ED project . Rapid appraisal of barriers to the diagnosis and management of patients with dementia in primary care: a systematic review. BMC Fam Pract 2010;11. https://doi.org/10.1186/1471-2296-11-52.
- Eustace A, Bruce I, Coen R, Cunningham C, Walsh C, Walsh JB, et al. Behavioural disturbance triggers recognition of dementia by family informants. Int J Geriatr Psychiatry 2007;22:574-9. https://doi.org/10.1002/gps.1718.
- Rapp T. Patients’ diagnosis decisions in Alzheimer’s disease: the influence of family factors. Soc Sci Med 2014;118:9-16. https://doi.org/10.1016/j.socscimed.2014.07.052.
- Pimouguet C, Le-Goff M, Rizzuto D, Berr C, Leffondré K, Pérès K, et al. Effect of early referral to specialist in dementia on institutionalization and functional decline: findings from a population-based study. J Alzheimers Dis 2016;49:819-28. https://doi.org/10.3233/JAD-150574.
- Pimouguet C, Rizzuto D, Fratiglioni L, Xu W. Does timely diagnosis influence dementia prognosis? A population-based study from Snac in Kungsholmen. Alzheimers Dement J Alzheimers Assoc 2016;12. https://doi.org/10.1016/j.jalz.2016.06.543.
- James Lind Alliance . Dementia Top 10 n.d. www.jla.nihr.ac.uk/priority-setting-partnerships/dementia/top-10-priorities/ (accessed 10 May 2018).
- Brunet M. Targets for dementia diagnoses will lead to overdiagnosis. BMJ 2014;348. https://doi.org/10.1136/bmj.g2224.
- GOV.UK . Recommendation Against National Dementia Screening n.d. www.gov.uk/government/news/recommendation-against-national-dementia-screening (accessed 2 May 2018).
- Martin S, Kelly S, Khan A, Cullum S, Dening T, Rait G, et al. Attitudes and preferences towards screening for dementia: a systematic review of the literature. BMC Geriatr 2015;15. https://doi.org/10.1186/s12877-015-0064-6.
- Harris PA, Taylor R, Thielke R, Payne J, Gonzalez N, Conde JG. Research electronic data capture (REDCap) – a metadata-driven methodology and workflow process for providing translational research informatics support. J Biomed Inform 2009;42:377-81. https://doi.org/10.1016/j.jbi.2008.08.010.
- Primary Care Support England n.d. https://pcse.england.nhs.uk/ (accessed 11 February 2019).
- Huppert FA, Brayne C, Gill C, Paykel ES, Beardsall L. CAMCOG – a concise neuropsychological test to assist dementia diagnosis: socio-demographic determinants in an elderly population sample. Br J Clin Psychol 1995;34:529-41. https://doi.org/10.1111/j.2044-8260.1995.tb01487.x.
- Folstein MF, Folstein SE, McHugh PR. ‘Mini-mental state’. A practical method for grading the cognitive state of patients for the clinician. J Psychiatr Res 1975;12:189-98. https://doi.org/10.1016/0022-3956(75)90026-6.
- Huppert A, Jorm AF, Brayne C, Girling DM, Barkley C, Beardsall L, et al. Psychometric properties of the CAMCOG and its efficacy in the diagnosis of dementia. Aging Neuropsychol Cogn 1196;3:201-14. https://doi.org/10.1080/13825589608256624.
- Lindeboom J, Ter Horst R, Hooyer C, Dinkgreve M, Jonker C. Some psychometric properties of the CAMCOG. Psychol Med 1993;23:213-19. https://doi.org/10.1017/S0033291700039003.
- Blessed G, Tomlinson BE, Roth M. The association between quantitative measures of dementia and of senile change in the cerebral grey matter of elderly subjects. Br J Psychiatry 1968;114:797-811. https://doi.org/10.1192/bjp.114.512.797.
- Copeland JR, Dewey ME, Griffiths-Jones HM. A computerized psychiatric diagnostic system and case nomenclature for elderly subjects: GMS and AGECAT. Psychol Med 1986;16:89-9. https://doi.org/10.1017/S0033291700057779.
- Kvaal K, McDougall FA, Brayne C, Matthews FE, Dewey ME, MRC CFAS. Co-occurrence of anxiety and depressive disorders in a community sample of older people: results from the MRC CFAS (Medical Research Council Cognitive Function and Ageing Study). Int J Geriatr Psychiatry 2008;23:229-37. https://doi.org/10.1002/gps.1867.
- White IR, Royston P, Wood AM. Multiple imputation using chained equations: issues and guidance for practice. Stat Med 2011;30:377-99. https://doi.org/10.1002/sim.4067.
- Williams RL. A note on robust variance estimation for cluster-correlated data. Biometrics 2000;56:645-6. https://doi.org/10.1111/j.0006-341X.2000.00645.x.
- Collerton J, Davies K, Jagger C, Kingston A, Bond J, Eccles MP, et al. Health and disease in 85 year olds: baseline findings from the Newcastle 85+ cohort study. BMJ 2009;339. https://doi.org/10.1136/bmj.b4904.
- Amjad H, Roth DL, Sheehan OC, Lyketsos CG, Wolff JL, Samus QM. Underdiagnosis of dementia: an observational study of patterns in diagnosis and awareness in US older adults. J Gen Intern Med 2018;33:1131-8. https://doi.org/10.1007/s11606-018-4377-y.
- Mukadam N, Livingston G, Rantell K, Rickman S. Diagnostic rates and treatment of dementia before and after launch of a national dementia policy: an observational study using English national databases. BMJ Open 2014;4. https://doi.org/10.1136/bmjopen-2013-004119.
- Helmer C, Pérès K, Pariente A, Pasquier F, Auriacombe S, Poncet M, et al. Primary and secondary care consultations in elderly demented individuals in France. Results from the Three-City Study. Dement Geriatr Cogn Disord 2008;26:407-15. https://doi.org/10.1159/000164692.
- Mitchell AJ, Meader N, Pentzek M. Clinical recognition of dementia and cognitive impairment in primary care: a meta-analysis of physician accuracy. Acta Psychiatr Scand 2011;124:165-83. https://doi.org/10.1111/j.1600-0447.2011.01730.x.
- Walker IF, Lord PA, Farragher TM. Variations in dementia diagnosis in England and association with general practice characteristics. Prim Health Care Res Dev 2017;18:235-41. https://doi.org/10.1017/S146342361700007X.
- Cooper C, Lodwick R, Walters K, Raine R, Manthorpe J, Iliffe S, et al. Inequalities in receipt of mental and physical healthcare in people with dementia in the UK. Age Ageing 2017;46:393-400. https://doi.org/10.1093/ageing/afw208.
- Savva GM, Arthur A. Who has undiagnosed dementia? A cross-sectional analysis of participants of the Aging, Demographics and Memory Study. Age Ageing 2015;44:642-7. https://doi.org/10.1093/ageing/afv020.
- Macdonald AJ, Carpenter GI. The recognition of dementia in ‘non-EMI’ nursing home residents in South East England. Int J Geriatr Psychiatry 2003;18:105-8. https://doi.org/10.1002/gps.779.
- Wilkins CH, Wilkins KL, Meisel M, Depke M, Williams J, Edwards DF. Dementia undiagnosed in poor older adults with functional impairment. J Am Geriatr Soc 2007;55:1771-6. https://doi.org/10.1111/j.1532-5415.2007.01417.x.
- Feast A, Orrell M, Charlesworth G, Melunsky N, Poland F, Moniz-Cook E. Behavioural and psychological symptoms in dementia and the challenges for family carers: systematic review. Br J Psychiatry 2016;208:429-34. https://doi.org/10.1192/bjp.bp.114.153684.
- Storti LB, Quintino DT, Silva NM, Kusumota L, Marques S. Neuropsychiatric symptoms of the elderly with Alzheimer’s disease and the family caregivers’ distress. Rev Lat Am Enfermagem 2016;24. https://doi.org/10.1590/1518-8345.0580.2751.
- Pimouguet C, Delva F, Le Goff M, Stern Y, Pasquier F, Berr C, et al. Survival and early recourse to care for dementia: a population based study. Alzheimers Dement 2015;11:385-93. https://doi.org/10.1016/j.jalz.2014.04.512.
- Lee SJ, Larson EB, Dublin S, Walker R, Marcum Z, Barnes D. A cohort study of healthcare utilization in older adults with undiagnosed dementia. J Gen Intern Med 2018;33:13-5. https://doi.org/10.1007/s11606-017-4162-3.
- Fogg C, Meredith P, Bridges J, Gould GP, Griffiths P. The relationship between cognitive impairment, mortality and discharge characteristics in a large cohort of older adults with unscheduled admissions to an acute hospital: a retrospective observational study. Age Ageing 2017;46:794-801. https://doi.org/10.1093/ageing/afx022.
- Power C, Duffy R, Bates H, Healy M, Gleeson P, Lawlor BA, et al. The detection, diagnosis, and impact of cognitive impairment among inpatients aged 65 years and over in an Irish general hospital - a prospective observational study. Int Psychogeriatr 2017;29:1879-88. https://doi.org/10.1017/S1041610217001326.
- Reynish EL, Hapca SM, De Souza N, Cvoro V, Donnan PT, Guthrie B. Epidemiology and outcomes of people with dementia, delirium, and unspecified cognitive impairment in the general hospital: prospective cohort study of 10,014 admissions. BMC Med 2017;15. https://doi.org/10.1186/s12916-017-0899-0.
- Low LF, McGrath M, Swaffer K, Brodaty H. Communicating a diagnosis of dementia: a systematic mixed studies review of attitudes and practices of health practitioners. Dementia 2018. https://doi.org/10.1177/1471301218761911.
- Livingston G, Baio G, Sommerlad A, de Lusignan S, Poulimenos S, Morris S, et al. Effectiveness of an intervention to facilitate prompt referral to memory clinics in the United Kingdom: cluster randomised controlled trial. PLOS Med 2017;14. https://doi.org/10.1371/journal.pmed.1002252.
- Pickett J, Bird C, Ballard C, Banerjee S, Brayne C, Cowan K, et al. A roadmap to advance dementia research in prevention, diagnosis, intervention, and care by 2025. Int J Geriatr Psychiatry 2018;33:900-6. https://doi.org/10.1002/gps.4868.
- Iliffe S, Wilcock J. The UK experience of promoting dementia recognition and management in primary care. Z. Für Gerontol Geriatr 2017;50:63-7. https://doi.org/10.1007/s00391-016-1175-1.
- Moynihan R, Doust J, Henry D. Preventing overdiagnosis: how to stop harming the healthy. BMJ 2012;344. https://doi.org/10.1136/bmj.e3502.
- Brunet MD, McCartney M, Heath I, Tomlinson J, Gordon P, Cosgrove J, et al. There is no evidence base for proposed dementia screening. BMJ 2012;345. https://doi.org/10.1136/bmj.e8588.
- Prince M, Comas-Herrera A, Knapp M, Guerchet M, Karagiannidou M. World Alzheimer Report 2016 2016. www.alz.co.uk/research/WorldAlzheimerReport2016.pdf (accessed 26 June 2019).
- Fowler NR, Harrawood A, Frame A, Perkins AJ, Gao S, Callahan CM, et al. The Indiana University Cognitive Health Outcomes Investigation of the Comparative Effectiveness of dementia screening (CHOICE) study: study protocol for a randomized controlled trial. Trials 2014;15. https://doi.org/10.1186/1745-6215-15-209.
- Lin JS, O’Connor E, Rossom RC, Perdue LA, Burda BU, Thompson M, et al. Screening for Cognitive Impairment in Older Adults: An Evidence Update for the U.S. Preventive Services Task Force. Agency for Healthcare Research and Quality (US); 2013.
Appendix 1 Selection of the control sample
Criteria for selection of the CADDY control sample
Select a sample of around 150 people without dementia, but weighted towards those with cognitive impairment. Include all people with prescription of an anti-dementia drug at W1 or W2, if they did not meet the criteria for dementia at that time.
For each practice:
-
sample at least one W1 control (person without dementia at W1) when there is a W1 case
-
sample at least one W2 control (person without dementia at W2) if there is a W2 incident case (person who first meets the criteria for dementia at W2)
-
allow up to two controls for each practice when the number of cases is ≥ 3
-
allow up to three controls for each practice when the number of cases ≥ 4.
Algorithm for selection of the control sample
Begin with the list of practices from which there is a CADDY participant and at least one other participant, merged with the list of patients without dementia who have consented to use of their medical records.
Stages:
-
exclude participants who could not give any interview information (as likely dementia)
-
exclude participants for whom dementia status at W1 is uncertain
-
for 40 iterations, select one participant at random from the group with the following criteria:
-
their MMSE group at W1 = 1
-
they are not currently selected as a control
-
the practice they come from does not have the maximum number of controls selected
-
-
for 40 further iterations, select one participant at random from the group with the following criteria:
-
their MMSE group at W1 = 2
-
they are not currently selected as a control
-
the practice they come from does not have the maximum number of controls selected
-
-
for 30 further iterations, select one participant at random from the group with the following criteria:
-
their MMSE group at W1 = 3
-
they are not currently selected as a control
-
the practice they come from does not have the maximum number of controls selected
-
-
select any participant not already selected who reports use of a dementia-specific medication at W1
-
for 30 further iterations, select one participant at random from the group with the following criteria:
-
their MMSE group at W1 = 4
-
they are not currently selected as a control
-
the practice they come from does not have the maximum number of controls selected
-
-
finally, select all participants remaining from any practice with a case (person with dementia) but not a control.
The total number of selected cases using this algorithm was 153. When the CFAS core team reviewed the paper records of consent for medical records linkage for these selected participants, four were found to be incomplete and so the number of people without dementia included in the study was reduced to 149.
Appendix 2 Case report form
Appendix 3 Supplementary tables
Factor | Level | Total, n (%) | Diagnosis status, n (%) | |||
---|---|---|---|---|---|---|
Unknown | Concern | Referred | Diagnosed | |||
Age group (years) | 65–74 | 60 (100.0) | 27 (45.0) | 7 (11.7) | 7 (11.7) | 19 (31.7) |
75–79 | 105 (100.0) | 43 (41.0) | 8 (7.6) | 9 (8.6) | 45 (42.9) | |
80–84 | 141 (100.0) | 58 (41.1) | 16 (11.3) | 15 (10.6) | 52 (36.9) | |
85–89 | 120 (100.0) | 56 (46.7) | 13 (10.8) | 7 (5.8) | 44 (36.7) | |
≥ 90 | 75 (100.0) | 36 (48.0) | 13 (17.3) | 7 (9.3) | 19 (25.3) | |
Sex | Male | 219 (100.0) | 108 (49.3) | 17 (7.8) | 22 (10.0) | 72 (32.9) |
Female | 282 (100.0) | 112 (39.7) | 40 (14.2) | 23 (8.2) | 107 (37.9) | |
Years of education | < 10 | 230 (100.0) | 109 (47.4) | 25 (10.9) | 20 (8.7) | 76 (33.0) |
10 | 132 (100.0) | 49 (37.1) | 15 (11.4) | 15 (11.4) | 53 (40.2) | |
> 10 | 121 (100.0) | 55 (45.5) | 14 (11.6) | 8 (6.6) | 44 (36.4) | |
Living arrangement | Institutionalised | 91 (100.0) | 37 (40.7) | 13 (14.3) | 5 (5.5) | 36 (39.6) |
Lives with long-term partner | 175 (100.0) | 67 (38.3) | 18 (10.3) | 17 (9.7) | 73 (41.7) | |
Lives with others/alone | 222 (100.0) | 110 (49.5) | 26 (11.7) | 23 (10.4) | 63 (28.4) | |
CAMCOG score | 0–2 | 80 (100.0) | 17 (21.3) | 12 (15.0) | 7 (8.8) | 44 (55.0) |
3–40 | 102 (100.0) | 36 (35.3) | 12 (11.8) | 6 (5.9) | 48 (47.1) | |
41–51 | 71 (100.0) | 30 (42.3) | 7 (9.9) | 8 (11.3) | 26 (36.6) | |
52–65 | 119 (100.0) | 55 (46.2) | 9 (7.6) | 15 (12.6) | 40 (33.6) | |
≥ 66 | 129 (100.0) | 82 (63.6) | 17 (13.2) | 9 (7.0) | 21 (16.3) | |
Memory symptoms | 0–3 | 103 (100.0) | 66 (64.1) | 11 (10.7) | 7 (6.8) | 19 (18.4) |
4 | 112 (100.0) | 33 (29.5) | 15 (13.4) | 15 (13.4) | 49 (43.8) | |
5 | 136 (100.0) | 34 (25.0) | 13 (9.6) | 12 (8.8) | 77 (56.6) | |
Non-memory symptoms | 0/1 | 96 (100.0) | 52 (54.2) | 11 (11.5) | 7 (7.3) | 26 (27.1) |
2 | 43 (100.0) | 19 (44.2) | 7 (16.3) | 5 (11.6) | 12 (27.9) | |
3 | 50 (100.0) | 19 (38.0) | 6 (12.0) | 7 (14.0) | 18 (36.0) | |
4/5 | 94 (100.0) | 27 (28.7) | 8 (8.5) | 8 (8.5) | 51 (54.3) | |
6/7 | 69 (100.0) | 17 (24.6) | 7 (10.1) | 7 (10.1) | 38 (55.1) | |
Depression | None | 337 (100.0) | 151 (44.8) | 38 (11.3) | 29 (8.6) | 119 (35.3) |
Subthreshold/case level | 125 (100.0) | 61 (48.8) | 11 (8.8) | 15 (12.0) | 38 (30.4) | |
Anxiety | None | 289 (100.0) | 134 (46.4) | 32 (11.1) | 28 (9.7) | 95 (32.9) |
Subthreshold/case level | 173 (100.0) | 78 (45.1) | 17 (9.8) | 16 (9.2) | 62 (35.8) | |
Cardiovascular | 0 | 201 (100.0) | 81 (40.3) | 29 (14.4) | 19 (9.5) | 72 (35.8) |
1 | 142 (100.0) | 60 (42.3) | 12 (8.5) | 11 (7.7) | 59 (41.5) | |
2–5 | 123 (100.0) | 65 (52.8) | 10 (8.1) | 13 (10.6) | 35 (28.5) | |
Other comorbidity | 0 | 142 (100.0) | 60 (42.3) | 18 (12.7) | 14 (9.9) | 50 (35.2) |
1 | 143 (100.0) | 52 (36.4) | 16 (11.2) | 11 (7.7) | 64 (44.8) | |
2 | 122 (100.0) | 65 (53.3) | 10 (8.2) | 13 (10.7) | 34 (27.9) | |
3–5 | 59 (100.0) | 29 (49.2) | 7 (11.9) | 5 (8.5) | 18 (30.5) | |
Social contact | Daily | 181 (100.0) | 89 (49.2) | 15 (8.3) | 18 (9.9) | 59 (32.6) |
Two or three times per week | 112 (100.0) | 49 (43.8) | 12 (10.7) | 13 (11.6) | 38 (33.9) | |
Less often | 101 (100.0) | 42 (41.6) | 16 (15.8) | 8 (7.9) | 35 (34.7) | |
Institutionalised | 93 (100.0) | 38 (40.9) | 13 (14.0) | 5 (5.4) | 37 (39.8) | |
Social activities | None | 285 (100.0) | 132 (46.3) | 37 (13.0) | 25 (8.8) | 91 (31.9) |
Any | 157 (100.0) | 74 (47.1) | 14 (8.9) | 15 (9.6) | 54 (34.4) |
Factor | Level | Total, n (%a) | Diagnosis status, n (%a) | |||
---|---|---|---|---|---|---|
Unknown | Concern | Referred | Diagnosed | |||
Age group (years) | 65–74 | 60 (10.6) | 27 (10.8) | 7 (9.0) | 7 (20.5) | 19 (8.5) |
75–79 | 105 (17.7) | 43 (15.5) | 8 (12.5) | 9 (15.3) | 45 (22.1) | |
80–84 | 141 (26.0) | 58 (23.7) | 16 (20.1) | 15 (28.5) | 52 (29.5) | |
85–89 | 120 (23.0) | 56 (25.1) | 13 (18.0) | 7 (17.0) | 44 (23.7) | |
≥ 90 | 75 (22.8) | 36 (24.9) | 13 (40.4) | 7 (18.8) | 19 (16.2) | |
Age | Median (IQR) | 84 (79–89) | 85 (79–89) | 86 (80–93) | 83 (76–89) | 83 (78–87) |
Sex | Male | 219 (34.5) | 108 (40.2) | 17 (20.3) | 22 (36.7) | 72 (32.3) |
Female | 282 (65.5) | 112 (59.8) | 40 (79.7) | 23 (63.3) | 107 (67.7) | |
Years of education | < 10 | 230 (51.8) | 109 (55.4) | 25 (46.0) | 20 (50.2) | 76 (50.0) |
10 | 132 (26.0) | 49 (21.2) | 15 (27.2) | 15 (35.4) | 53 (28.5) | |
> 10 | 121 (22.2) | 55 (23.4) | 14 (26.8) | 8 (14.4) | 44 (21.5) | |
Living arrangement | Institutionalised | 91 (29.3) | 37 (28.7) | 13 (36.8) | 5 (19.3) | 36 (30.2) |
Lives with long-term partner | 175 (28.3) | 67 (21.7) | 18 (20.1) | 17 (36.2) | 73 (36.1) | |
Lives with others/alone | 222 (42.4) | 110 (49.6) | 26 (43.1) | 23 (44.5) | 63 (33.7) | |
CAMCOG score | Median (IQR) | 45 (5–62) | 55 (38–67) | 40 (0–64) | 50 (3–60) | 28 (1–54) |
Memory symptoms | 0–3 | 103 (28.6) | 66 (46.7) | 11 (39.0) | 7 (25.5) | 19 (12.3) |
4 | 112 (30.0) | 33 (27.7) | 15 (30.9) | 15 (29.5) | 49 (31.7) | |
5 | 136 (41.4) | 34 (25.6) | 13 (30.1) | 12 (45.0) | 77 (56.1) | |
Non-memory symptoms | 0/1 | 96 (22.9) | 52 (34.0) | 11 (32.9) | 7 (20.2) | 26 (12.2) |
2 | 43 (9.5) | 19 (11.8) | 7 (14.4) | 5 (10.8) | 12 (6.1) | |
3 | 50 (11.9) | 19 (12.5) | 6 (9.0) | 7 (11.6) | 18 (12.3) | |
4/5 | 94 (26.8) | 27 (22.2) | 8 (16.4) | 8 (21.7) | 51 (34.3) | |
6/7 | 69 (28.9) | 17 (19.5) | 7 (27.3) | 7 (35.8) | 38 (35.1) | |
Depression | None | 337 (70.3) | 151 (67.0) | 38 (77.4) | 29 (66.0) | 119 (73.5) |
Subthreshold/case level | 125 (29.7) | 61 (33.0) | 11 (22.5) | 15 (33.9) | 38 (26.5) | |
Anxiety | None | 289 (61.3) | 134 (60.8) | 32 (63.1) | 28 (68.7) | 95 (59.7) |
Subthreshold/case level | 173 (38.7) | 78 (39.3) | 17 (36.9) | 16 (31.3) | 62 (40.4) | |
Cardiovascular | 0 | 201 (40.6) | 81 (36.5) | 29 (49.1) | 19 (39.4) | 72 (42.7) |
1 | 142 (35.0) | 60 (31.9) | 12 (28.7) | 11 (32.9) | 59 (40.6) | |
2–5 | 123 (24.3) | 65 (31.6) | 10 (22.2) | 13 (27.6) | 35 (16.7) | |
Other comorbidity | 0 | 142 (29.8) | 60 (26.1) | 18 (31.6) | 14 (29.7) | 50 (33.1) |
1 | 143 (28.0) | 52 (22.8) | 16 (25.4) | 11 (18.6) | 64 (36.4) | |
2 | 122 (29.1) | 65 (35.8) | 10 (26.0) | 13 (40.5) | 34 (20.4) | |
3–5 | 59 (13.1) | 29 (15.3) | 7 (17.0) | 5 (11.2) | 18 (10.1) | |
Social contact | Daily | 181 (30.9) | 89 (35.2) | 15 (18.9) | 18 (36.0) | 59 (28.7) |
Two or three times per week | 112 (18.4) | 49 (18.2) | 12 (14.5) | 13 (20.6) | 38 (19.3) | |
Less often | 101 (20.7) | 42 (18.1) | 16 (29.2) | 8 (23.3) | 35 (20.4) | |
Institutionalised | 93 (30.0) | 38 (28.5) | 13 (37.4) | 5 (20.1) | 37 (31.8) | |
Social activities | None | 285 (68.4) | 132 (66.5) | 37 (76.3) | 25 (73.1) | 91 (66.9) |
Any | 157 (31.5) | 74 (33.4) | 14 (23.7) | 15 (26.9) | 54 (33.0) |
Factor | Level | Complete case (N = 270) | Multiple imputation (N = 703) | ||||
---|---|---|---|---|---|---|---|
n | OR | 95% CI | OR | 95% CI | p-valuea | ||
Memory impairment | Per SD | – | 0.37 | 0.19 to 0.74 | 0.36 | 0.21 to 0.61 | 0.0002 |
Non-memory impairment | Per SD | – | 1.27 | 0.63 to 2.55 | 1.46 | 0.91 to 2.33 | 0.1124 |
Memory symptoms | 0–2 | 54 | 1 | Reference | 1 | Reference | 0.0048 |
3 | 36 | 0.99 | 0.22 to 4.42 | 2.06 | 0.68 to 6.23 | ||
4 | 92 | 3.13 | 1.05 to 9.32 | 3.21 | 1.20 to 8.55 | ||
5 | 88 | 5.17 | 1.81 to 14.76 | 4.87 | 1.77 to 13.40 | ||
Non-memory symptoms | 0 | 38 | 1 | Reference | 1 | Reference | 0.1760 |
1 | 50 | 0.73 | 0.19 to 2.82 | 0.66 | 0.23 to 1.88 | ||
2 | 35 | 0.84 | 0.22 to 3.14 | 0.81 | 0.28 to 2.33 | ||
3 | 42 | 1.08 | 0.31 to 3.75 | 1.14 | 0.40 to 3.25 | ||
4 | 32 | 1.43 | 0.39 to 5.28 | 1.45 | 0.50 to 4.17 | ||
5 | 37 | 2.9 | 0.77 to 10.86 | 2.17 | 0.75 to 6.29 | ||
6 | 23 | 3.79 | 0.91 to 15.76 | 2.29 | 0.76 to 6.89 | ||
7/8 | 13 | 0.84 | 0.13 to 5.36 | 2.3 | 0.69 to 7.67 | ||
Sex | Male | 138 | 1 | Reference | 1 | Reference | 0.4802 |
Female | 132 | 0.95 | 0.44 to 2.05 | 1.23 | 0.70 to 2.16 | ||
Age group (years) | 65–74 | 36 | 1 | Reference | 1 | Reference | 0.1640 |
75–79 | 62 | 1.07 | 0.34 to 3.31 | 1.6 | 0.64 to 4.02 | ||
80–84 | 76 | 0.98 | 0.30 to 3.24 | 1.33 | 0.54 to 3.27 | ||
85–90 | 62 | 0.75 | 0.21 to 2.66 | 1.04 | 0.38 to 2.83 | ||
≥ 90 | 34 | 0.46 | 0.09 to 2.39 | 0.54 | 0.18 to 1.62 | ||
Years of education | < 10 | 127 | 1 | Reference | 1 | Reference | 0.999 |
10 | 78 | 0.86 | 0.34 to 2.18 | 1.01 | 0.55 to 1.87 | ||
> 10 | 65 | 1.09 | 0.40 to 2.98 | 1 | 0.53 to 1.90 | ||
Townsend deprivation index quintile | Least deprived | 67 | 1 | Reference | 1 | Reference | 0.8305 |
Second | 60 | 0.38 | 0.13 to 1.13 | 0.65 | 0.31 to 1.36 | ||
Third | 60 | 0.58 | 0.21 to 1.55 | 0.71 | 0.35 to 1.44 | ||
Fourth | 39 | 0.64 | 0.22 to 1.87 | 0.86 | 0.41 to 1.82 | ||
Most deprived | 44 | 0.42 | 0.13 to 1.34 | 0.85 | 0.39 to 1.85 | ||
Anxiety | None | 166 | 1 | Reference | 1 | Reference | 0.3137 |
Mild | 87 | 0.96 | 0.44 to 2.09 | 1.11 | 0.64 to 1.93 | ||
Moderate or severe | 17 | 2.14 | 0.37 to 12.56 | 2.25 | 0.59 to 8.62 | ||
Depression | None | 192 | 1 | Reference | 1 | Reference | 0.5049 |
Mild | 48 | 0.52 | 0.22 to 1.25 | 0.61 | 0.33 to 1.15 | ||
Moderate/severe | 30 | 0.85 | 0.24 to 3.05 | 0.69 | 0.26 to 1.86 | ||
Cardiovascular disease | 0 | 94 | 1 | Reference | 1 | Reference | 0.0226 |
1 | 96 | 0.9 | 0.41 to 1.95 | 1.08 | 0.64 to 1.83 | ||
2 | 50 | 1.01 | 0.39 to 2.61 | 0.77 | 0.39 to 1.50 | ||
3 | 21 | 0.10 | 0.02 to 0.53 | 0.25 | 0.08 to 0.82 | ||
4/5 | 9 | 0.13 | 0.02 to 0.76 | 0.15 | 0.03 to 0.78 | ||
Non-cardiovascular comorbidity | 0 | 67 | 1 | Reference | 1 | Reference | 0.3346 |
1 | 87 | 2.12 | 0.92 to 4.91 | 1.21 | 0.68 to 2.16 | ||
2 | 78 | 0.86 | 0.33 to 2.25 | 0.69 | 0.36 to 1.32 | ||
3 | 28 | 0.91 | 0.25 to 3.31 | 1.11 | 0.43 to 2.87 | ||
4–6 | 10 | 0.83 | 0.20 to 3.42 | 0.45 | 0.12 to 1.75 | ||
Social activities | 0 | 171 | 1 | Reference | 1 | Reference | 0.2298 |
1 | 80 | 0.71 | 0.30 to 1.68 | 1.18 | 0.67 to 2.07 | ||
2 | 12 | 1.79 | 0.45 to 7.08 | 2.38 | 0.80 to 7.08 | ||
≥ 3 | 7 | 2.17 | 0.38 to 12.50 | 2.87 | 0.75 to 11.03 | ||
Living arrangement | Lives alone | 85 | 1.04 | 0.36 to 3.02 | 1.18 | 0.61 to 2.29 | 0.1298 |
Lives with spouse | 123 | 2.09 | 0.92 to 4.75 | 2.20 | 1.20 to 4.01 | ||
Lives with others | 23 | 0.32 | 0.06 to 1.67 | 0.97 | 0.37 to 2.54 | ||
Residential care | 39 | 1 | Reference | 1.00 | Reference | ||
Wave | W2 vs. W1 | – | 1.95 | 1.04 to 3.66 | 1.68 | 1.07 to 2.64 | 0.0241 |
Factor | Level | Predictors of help-seeking | Predictors of diagnosis conditional on help-seeking | ||
---|---|---|---|---|---|
OR | 95% CI | OR | 95% CI | ||
Memory impairment | Per SD | 0.30*** | 0.19 to 0.48 | 0.68 | 0.36 to 1.29 |
Non-memory impairment | Per SD | 1.90** | 1.22 to 2.98 | 0.96 | 0.54 to 1.70 |
Memory symptoms | 0–2 | 1 | Reference | 1 | Reference |
3 | 1.51 | 0.61 to 3.75 | 2.25 | 0.52 to 9.67 | |
4 | 2.85** | 1.30 to 6.26 | 2.53 | 0.78 to 8.23 | |
5 | 3.58** | 1.57 to 8.15 | 4.09* | 1.19 to 14.06 | |
Non-memory symptoms | 0 | 1 | Reference | 1 | Reference |
1 | 0.78 | 0.30 to 2.00 | 0.62 | 0.17 to 2.31 | |
2 | 1.13 | 0.44 to 2.92 | 0.64 | 0.17 to 2.45 | |
3 | 1.33 | 0.53 to 3.35 | 0.8 | 0.22 to 2.94 | |
4 | 1.45 | 0.54 to 3.93 | 0.97 | 0.26 to 3.62 | |
5 | 1.98 | 0.70 to 5.59 | 1.79 | 0.50 to 6.33 | |
6 | 1.81 | 0.63 to 5.24 | 1.7 | 0.40 to 7.29 | |
7/8 | 3.14 | 0.89 to 11.06 | 1.19 | 0.30 to 4.79 | |
Sex | Male | 1 | Reference | 1 | Reference |
Female | 1.46 | 0.88 to 2.44 | 1.01 | 0.50 to 2.04 | |
Years of education | < 10 | 1 | Reference | 1 | Reference |
10 | 1.16 | 0.66 to 2.05 | 0.86 | 0.41 to 1.80 | |
> 10 | 0.88 | 0.48 to 1.62 | 1.2 | 0.54 to 2.69 | |
Age group (years) | 65–74 | 1 | Reference | 1 | Reference |
75–79 | 0.97 | 0.41 to 2.30 | 1.69 | 0.59 to 4.89 | |
80–84 | 1.04 | 0.45 to 2.43 | 1.2 | 0.43 to 3.36 | |
85–90 | 0.61 | 0.26 to 1.44 | 1.29 | 0.43 to 3.89 | |
≥ 90 | 0.5 | 0.19 to 1.31 | 0.59 | 0.18 to 1.99 | |
Depression | None | 1 | Reference | 1 | Reference |
Mild | 0.79 | 0.43 to 1.45 | 0.59 | 0.27 to 1.28 | |
Moderate | 0.75 | 0.31 to 1.82 | 0.77 | 0.26 to 2.27 | |
Anxiety | None | 1 | Reference | 1 | Reference |
Mild | 0.97 | 0.58 to 1.63 | 1.14 | 0.56 to 2.32 | |
Moderate/severe | 1.41 | 0.41 to 4.89 | 2.46 | 0.49 to 12.41 | |
Cardiovascular disease | 0 | 1 | Reference | 1 | Reference |
1 | 0.81 | 0.47 to 1.39 | 1.43 | 0.72 to 2.81 | |
2 | 0.54 | 0.28 to 1.05 | 1.57 | 0.63 to 3.92 | |
3 | 0.43 | 0.17 to 1.11 | 0.29 | 0.08 to 1.08 | |
4/5 | 0.29* | 0.09 to 0.97 | 0.19 | 0.03 to 1.24 | |
Non-cardiovascular comorbidity | 0 | 1 | Reference | 1 | Reference |
1 | 1.06 | 0.59 to 1.91 | 1.31 | 0.65 to 2.67 | |
2 | 0.64 | 0.36 to 1.16 | 0.79 | 0.32 to 1.98 | |
3 | 1.01 | 0.43 to 2.41 | 1.07 | 0.32 to 3.58 | |
4–6 | 0.51 | 0.16 to 1.59 | 0.48 | 0.09 to 2.72 | |
Social activity | 0 | 1 | Reference | 1 | Reference |
1 | 0.99 | 0.59 to 1.66 | 1.21 | 0.60 to 2.47 | |
2 | 1.43 | 0.49 to 4.14 | 2.11 | 0.40 to 11.26 | |
≥ 3 | 1.37 | 0.34 to 5.54 | 3.15 | 0.39 to 25.46 | |
Living arrangement | Residential care | 1.02 | 0.54 to 1.93 | 1.3 | 0.58 to 2.90 |
Lives with spouse | 1.97* | 1.09 to 3.55 | 1.78 | 0.82 to 3.87 | |
Lives with others | 1.49 | 0.66 to 3.34 | 0.8 | 0.27 to 2.35 | |
Lives alone | 1 | Reference | 1 | Reference | |
Wave | W1 vs. W2 | 1.85** | 1.18 to 2.89 | 1.35 | 0.78 to 2.32 |
Total cases | 703 | Varies |
Factor | Level | Remained undiagnosed (N = 163), n (%) | New diagnosis (N = 66), n (%) | Died before diagnosis (N = 56), n (%) | Total (N = 285), n (%) | p-value for association |
---|---|---|---|---|---|---|
Subjective memory impairment | 0–3 | 51 (52.0) | 10 (21.7) | 16 (44.5) | 77 (42.8) | 0.016 |
4 | 23 (23.5) | 20 (43.5) | 11 (30.6) | 54 (30.0) | ||
5 | 24 (24.5) | 16 (34.8) | 9 (25.0) | 49 (27.2) | ||
Total | 98 (100) | 46 (100) | 36 (100) | 180 (100) | ||
Subjective non-memory impairment | 0–1 | 41 (41.4) | 9 (19.5) | 8 (22.3) | 58 (32.0) | 0.027 |
2–3 | 32 (32.3) | 16 (34.7) | 11 (30.5) | 59 (32.6) | ||
4 | 9 (9.1) | 8 (17.4) | 7 (19.4) | 24 (13.3) | ||
5–7 | 17 (17.2) | 13 (28.2) | 10 (27.8) | 40 (22.1) | ||
Total | 99 (100) | 46 (100) | 36 (100) | 181 (100) | ||
Sex | Male | 80 (49.1) | 24 (36.4) | 25 (44.6) | 129 (45.3) | 0.215 |
Female | 83 (50.9) | 42 (63.6) | 31 (55.4) | 156 (54.7) | ||
Total | 163 (100) | 66 (100) | 56 (100) | 285 (100) | ||
Years of education | < 10 | 73 (46.5) | 27 (42.9) | 37 (68.5) | 137 (50.0) | 0.023 |
10 | 41 (26.1) | 22 (34.9) | 8 (14.8) | 71 (25.9) | ||
> 10 | 43 (27.4) | 14 (22.2) | 9 (16.7) | 66 (24.1) | ||
Total | 157 (100) | 63 (100) | 54 (100) | 274 (100) | ||
Townsend index quintile | Lowest | 32 (19.6) | 13 (19.7) | 8 (14.3) | 53 (18.6) | 0.549 |
Second | 43 (26.4) | 14 (21.2) | 9 (16.1) | 66 (23.2) | ||
Third | 32 (19.6) | 18 (27.3) | 14 (25) | 64 (22.5) | ||
Fourth | 31 (19.0) | 10 (15.2) | 11 (19.6) | 52 (18.2) | ||
Highest | 25 (15.3) | 11 (16.7) | 14 (25) | 50 (17.5) | ||
Total | 163 (100) | 66 (100) | 56 (100) | 285 (100) | ||
Age group (years) | 65–79 | 62 (38.1) | 22 (33.3) | 8 (14.3) | 92 (32.3) | 0.034 |
80–84 | 41 (25.2) | 24 (36.4) | 16 (28.6) | 81 (28.4) | ||
85–89 | 33 (20.2) | 13 (19.7) | 18 (32.1) | 64 (22.5) | ||
≥ 90 | 27 (16.6) | 7 (10.6) | 14 (25.0) | 48 (16.8) | ||
Total | 163 (100) | 66 (100) | 56 (100) | 285 (100) | ||
Depression | None | 104 (68.4) | 46 (70.8) | 44 (80.0) | 194 (71.3) | 0.432 |
Subthreshold or case level | 48 (31.5) | 19 (29.3) | 11 (20.0) | 78 (28.7) | ||
Total | 152 (100) | 65 (100) | 55 (100) | 272 (100) | ||
Anxiety | None | 96 (63.2) | 36 (55.4) | 37 (67.3) | 169 (62.1) | 0.709 |
Subthreshold or case level | 56 (36.8) | 29 (44.7) | 18 (32.7) | 103 (37.9) | ||
Total | 152 (100) | 65 (100) | 55 (100) | 272 (100) | ||
Cardiovascular comorbidity | 0 | 66 (43.1) | 30 (48.4) | 18 (36.0) | 114 (43.0) | 0.622 |
1 | 41 (26.8) | 17 (27.4) | 15 (30.0) | 73 (27.5) | ||
2 | 29 (19.0) | 8 (12.9) | 9 (18.0) | 46 (17.4) | ||
≥ 3 | 17 (11.2) | 7 (11.3) | 8 (16.0) | 32 (12.1) | ||
Total | 153 (100) | 62 (100) | 50 (100) | 265 (100) | ||
Non-cardiovascular comorbidity | 0 | 48 (31.4) | 20 (32.3) | 14 (28.0) | 82 (30.9) | 0.716 |
1 | 43 (28.1) | 20 (32.3) | 10 (20.0) | 73 (27.5) | ||
2 | 39 (25.5) | 15 (24.2) | 19 (38.0) | 73 (27.5) | ||
≥ 3 | 23 (15.1) | 7 (11.3) | 7 (14.0) | 37 (14.0) | ||
Total | 153 (100) | 62 (100) | 50 (100) | 265 (100) | ||
Social activities | 0 | 98 (64.5) | 41 (63.1) | 36 (69.2) | 175 (65.1) | 0.891 |
≥ 1 | 54 (35.5) | 24 (36.9) | 16 (30.7) | 94 (34.9) | ||
Total | 152 (100) | 65 (100) | 52 (100) | 269 (100) | ||
Living arrangement | Residential care | 27 (17.0) | 2 (3.0) | 12 (22.2) | 41 (14.7) | 0.006 |
Long-term partner | 54 (34.0) | 27 (40.9) | 13 (24.1) | 94 (33.7) | ||
With others (not spouse) | 12 (7.5) | 5 (7.6) | 10 (18.5) | 27 (9.7) | ||
Alone | 66 (41.5) | 32 (48.5) | 19 (35.2) | 117 (41.9) | ||
Total | 159 (100) | 66 (100) | 54 (100) | 279 (100) |
Factor | Level | Sub-hazard ratio | 95% CI | p-value |
---|---|---|---|---|
Memory impairment | Per SD | 0.34** | 0.16 to 0.72 | 0.005 |
Non-memory impairment | Per SD | 2.22* | 1.05 to 4.70 | 0.036 |
Subjective non-memory impairment | 0–2 | 1 | Reference | Reference |
3 | 2.47 | 0.44 to 14.02 | 0.307 | |
4 | 3.58 | 0.75 to 17.03 | 0.109 | |
5 | 1.82 | 0.30 to 11.01 | 0.513 | |
Subjective non-memory impairment | 0 | 1 | Reference | Reference |
1 | 0.54 | 0.09 to 3.29 | 0.502 | |
2 | 0.78 | 0.16 to 3.83 | 0.76 | |
3 | 1.21 | 0.21 to 6.83 | 0.828 | |
4 | 2.62 | 0.63 to 10.97 | 0.188 | |
5 | 4.16 | 0.79 to 21.80 | 0.091 | |
6 | 3.37 | 0.54 to 20.91 | 0.192 | |
7 | 11.91* | 1.06 to 133.46 | 0.045 | |
Missing | 1.47 | 0.33 to 6.67 | 0.614 | |
Sex | Male | 1 | Reference | Reference |
Female | 1.88* | 1.02 to 3.46 | 0.043 | |
Years in education | < 10 | 1 | Reference | Reference |
10 | 1.98 | 0.90 to 4.35 | 0.089 | |
> 10 | 1.10 | 0.46 to 2.63 | 0.825 | |
Townsend deprivation index quintile | Least deprived | 1 | Reference | Reference |
Second | 0.86 | 0.28 to 2.66 | 0.791 | |
Third | 1.71 | 0.54 to 5.45 | 0.362 | |
Fourth | 2.04 | 0.55 to 7.60 | 0.285 | |
Most deprived | 2.02 | 0.63 to 6.44 | 0.235 | |
Age group (years) | 65–74 | 1 | Reference | Reference |
75–79 | 3.34* | 1.05 to 10.61 | 0.041 | |
80–84 | 2.83* | 1.09 to 7.30 | 0.032 | |
85–89 | 1.46 | 0.41 to 5.27 | 0.559 | |
≥ 90 | 1.84 | 0.33 to 10.29 | 0.488 | |
Depression | None | 1 | Reference | Reference |
Subthreshold | 1.38 | 0.54 to 3.56 | 0.499 | |
Case level | 0.71 | 0.17 to 2.89 | 0.633 | |
Anxiety | None | 1 | Reference | Reference |
Subthreshold | 0.92 | 0.46 to 1.85 | 0.819 | |
Case level | 2.45 | 0.66 to 9.08 | 0.181 | |
Cardiovascular comorbidity | 0 | 1 | Reference | Reference |
1 | 1.01 | 0.43 to 2.40 | 0.98 | |
2 | 1.02 | 0.41 to 2.50 | 0.972 | |
3 | 1.13 | 0.30 to 4.26 | 0.855 | |
4/5 | 1.05 | 0.07 to 14.82 | 0.974 | |
Non-cardiovascular comorbidity | 0 | 1 | Reference | Reference |
1 | 0.64 | 0.25 to 1.63 | 0.352 | |
2 | 0.54 | 0.20 to 1.42 | 0.209 | |
3 | 0.48 | 0.11 to 2.10 | 0.327 | |
4–6 | 0.28 | 0.04 to 2.22 | 0.228 | |
Social activity | 0 | 1 | Reference | Reference |
1 | 1.08 | 0.51 to 2.30 | 0.845 | |
2 | 2.17 | 0.63 to 7.51 | 0.223 | |
≥ 3 | Reference | Reference | Reference | |
Living arrangement | Residential care | 0.07** | 0.01 to 0.45 | 0.005 |
Long-term partner | 0.80 | 0.35 to 1.78 | 0.577 | |
With others (not spouse) | 0.32 | 0.08 to 1.22 | 0.095 | |
Lives alone | 1 | Reference | Reference | |
Wave | W2 vs. W1 | 3.03** | 1.44 to 6.36 | 0.003 |
Patient characteristic | Level | Did not die, n (%) | Died, n (%) | Total, n (%) | p-value for association |
---|---|---|---|---|---|
Diagnosed | No | 112 (39.3) | 173 (60.7) | 285 (100) | |
Yes | 42 (30.9) | 94 (69.1) | 136 (100) | ||
Total | 154 (36.6) | 267 (63.4) | 421 (100) | ||
Subjective memory impairments | 0–2 | 30 (50.8) | 29 (49.2) | 59 (100) | 0.015 |
3 | 15 (36.6) | 26 (63.4) | 41 (100) | ||
4 | 28 (30.8) | 63 (69.2) | 91 (100) | ||
5 | 30 (27.0) | 81 (73.0) | 111 (100) | ||
Total | 103 (34.1) | 199 (65.9) | 302 (100) | ||
Subjective non-memory impairments | 0 | 24 (68.6) | 11 (31.4) | 35 (100) | < 0.001 |
1 | 20 (43.5) | 26 (56.5) | 46 (100) | ||
2 | 14 (37.8) | 23 (62.2) | 37 (100) | ||
3 | 15 (31.3) | 33 (68.8) | 48 (100) | ||
4 | 10 (22.7) | 34 (77.3) | 44 (100) | ||
5 | 9 (22.5) | 31 (77.5) | 40 (100) | ||
6/7 | 12 (22.6) | 41 (77.4) | 53 (100) | ||
Total | 104 (34.3) | 199 (65.7) | 303 (100) | ||
Sex | Male | 77 (40.7) | 112 (59.3) | 189 (100) | 0.229 |
Female | 90 (35.2) | 166 (64.8) | 256 (100) | ||
Total | 167 (37.5) | 278 (62.5) | 445 (100) | ||
Years in education | < 10 | 63 (29.6) | 150 (70.4) | 213 (100) | 0.002 |
10 | 52 (46.0) | 61 (54.0) | 113 (100) | ||
> 10 | 47 (46.5) | 54 (53.5) | 101 (100) | ||
Total | 162 (37.9) | 265 (62.1) | 427 (100) | ||
Townsend deprivation index quintile | Least deprived | 31 (34.1) | 60 (65.9) | 91 (100) | 0.292 |
Second | 30 (32.3) | 63 (67.7) | 93 (100) | ||
Third | 34 (35.8) | 61 (64.2) | 95 (100) | ||
Fourth | 34 (40.0) | 51 (60.0) | 85 (100) | ||
Most deprived | 38 (46.9) | 43 (53.1) | 81 (100) | ||
Total | 167 (37.5) | 278 (62.5) | 445 (100) | ||
Age group (years) | 65–74 | 36 (64.3) | 20 (35.7) | 56 (100) | < 0.001 |
75–79 | 49 (52.1) | 45 (47.9) | 94 (100) | ||
80–84 | 48 (38.1) | 78 (61.9) | 126 (100) | ||
85–89 | 25 (23.4) | 82 (76.6) | 107 (100) | ||
≥ 90 | 9 (14.5) | 53 (85.5) | 62 (100) | ||
Total | 167 (37.5) | 278 (62.5) | 445 (100) | ||
Depression | None | 120 (39.2) | 186 (60.8) | 306 (100) | 0.51 |
Subthreshold | 24 (33.8) | 47 (66.2) | 71 (100) | ||
Case level | 13 (31.7) | 28 (68.3) | 41 (100) | ||
Total | 157 (37.6) | 261 (62.4) | 418 (100) | ||
Anxiety | None | 100 (39.4) | 154 (60.6) | 254 (100) | 0.608 |
Subthreshold | 48 (34.3) | 92 (65.7) | 140 (100) | ||
Case level | 9 (37.5) | 15 (62.5) | 24 (100) | ||
Total | 157 (37.6) | 261 (62.4) | 418 (100) | ||
Cardiovascular comorbidity | 0 | 71 (42.0) | 98 (58.0) | 169 (100) | 0.261 |
1 | 43 (34.1) | 83 (65.9) | 126 (100) | ||
2 | 32 (41.6) | 45 (58.4) | 77 (100) | ||
≥ 3 | 10 (25.6) | 29 (74.4) | 39 (100) | ||
Total | 156 (38.0) | 255 (62.0) | 411 (100) | ||
Non-cardiovascular comorbidity | 0 | 58 (47.2) | 65 (52.8) | 123 (100) | 0.099 |
1 | 45 (35.4) | 82 (64.6) | 127 (100) | ||
2 | 35 (33.0) | 71 (67.0) | 106 (100) | ||
3 | 12 (28.6) | 30 (71.4) | 42 (100) | ||
≥ 4 | 6 (46.2) | 7 (53.8) | 13 (100) | ||
Total | 156 (38.0) | 255 (62.0) | 411 (100) | ||
Social activity | 0 | 95 (36.7) | 164 (63.3) | 259 (100) | 0.151 |
1 | 45 (37.8) | 74 (62.2) | 119 (100) | ||
≥ 2 | 15 (60.0) | 10 (40.0) | 25 (100) | ||
Total | 155 (38.5) | 248 (61.5) | 403 (100) | ||
Living arrangement | Residential care | 15 (20.8) | 57 (79.2) | 72 (100) | 0.001 |
Long-term partner | 67 (43.5) | 87 (56.5) | 154 (100) | ||
With others (not spouse) | 8 (20.5) | 31 (79.5) | 39 (100) | ||
Alone | 72 (42.6) | 97 (57.4) | 169 (100) | ||
Total | 162 (37.3) | 272 (62.7) | 434 (100) |
Factor | Level | HR | 95% CI |
---|---|---|---|
Diagnosed | Yes vs. no | 1.247 | 0.911 to 1.707 |
Memory score | Per SD | 0.939 | 0.697 to 1.264 |
Non-memory score | Per SD | 0.931 | 0.715 to 1.212 |
Subjective memory complaints | 0 | 1 | Reference |
1 | 1.055 | 0.601 to 1.851 | |
2 | 1.233 | 0.736 to 2.064 | |
3 | 1.146 | 0.662 to 1.984 | |
Subjective non-memory complaints | 0 | 1 | Reference |
1 | 1.815 | 0.884 to 3.728 | |
2 | 1.747 | 0.843 to 3.622 | |
3 | 1.899 | 0.883 to 4.083 | |
4 | 2.658* | 1.245 to 5.675 | |
5 | 2.662* | 1.253 to 5.656 | |
6 | 2.242 | 0.969 to 5.190 | |
7 | 3.187** | 1.373 to 7.394 | |
Sex | Male | 1 | Reference |
Female | 0.84 | 0.616 to 1.145 | |
Years in education | < 10 | 1 | Reference |
10 | 0.746 | 0.529 to 1.053 | |
> 10 | 0.645* | 0.451 to 0.920 | |
Age group (years) | 65–74 | 1 | Reference |
75–79 | 1.192 | 0.668 to 2.125 | |
80–84 | 1.932* | 1.102 to 3.385 | |
85–89 | 2.365** | 1.347 to 4.153 | |
≥ 90 | 3.785*** | 2.040 to 7.024 | |
Depression | None | 1 | Reference |
Subthreshold | 0.876 | 0.596 to 1.287 | |
Clinical depression | 0.878 | 0.475 to 1.622 | |
Anxiety | None | 1 | Reference |
Subthreshold | 0.99 | 0.715 to 1.371 | |
Clinical anxiety | 0.798 | 0.353 to 1.803 | |
Cardiovascular comorbidity | 0 | 1 | Reference |
1 | 0.973 | 0.695 to 1.362 | |
2 | 0.925 | 0.633 to 1.351 | |
3 | 1.129 | 0.652 to 1.955 | |
4/5 | 2.043 | 0.858 to 4.868 | |
Townsend deprivation index quintile | Least deprived | 1 | Reference |
Second | 1.067 | 0.716 to 1.590 | |
Third | 1.268 | 0.843 to 1.906 | |
Fourth | 0.948 | 0.618 to 1.453 | |
Most deprived | 0.906 | 0.575 to 1.429 | |
Non-cardiovascular comorbidity | 0 | 1 | Reference |
1 | 1.072 | 0.746 to 1.541 | |
2 | 1.386 | 0.955 to 2.012 | |
3 | 1.456 | 0.858 to 2.471 | |
4–6 | 1.178 | 0.486 to 2.856 | |
Social activities | 0 | 1 | Reference |
1 | 0.913 | 0.668 to 1.249 | |
2 | 0.508 | 0.232 to 1.111 | |
≥ 3 | 0.739 | 0.209 to 2.609 | |
Living arrangement | Residential care | 1.672** | 1.148 to 2.437 |
Lives with spouse | 1.192 | 0.826 to 1.721 | |
Lives with others | 2.077** | 1.301 to 3.315 | |
Lives alone | 1 | Reference | |
Wave | W2 vs. W1 | 0.838 | 0.620 to 1.133 |
List of abbreviations
- BDS
- Blessed Dementia Scale
- CADDY
- Cognitive Function and Ageing Study II Dementia Diagnosis Study
- CAMCOG
- Cambridge Cognitive Examination
- CCG
- Clinical Commissioning Group
- CFAS
- Cognitive Function and Ageing Study
- CFAS II
- Cognitive Function and Ageing Study II
- CI
- confidence interval
- CRF
- case report form
- CRN
- Clinical Research Network
- CTU
- Clinical Trials Unit
- DSM-IIIR
- Diagnostic and Statistical Manual of Mental Disorders, Third Edition Revised
- GMS-AGECAT
- Geriatric Mental State-Automated Geriatric Examination for Computer Assisted Taxonomy
- GP
- general practitioner
- HR
- hazard ratio
- HRA
- Health Research Authority
- ID
- identification
- IQR
- interquartile range
- MICE
- multiple imputation by chained equations
- MMSE
- Mini Mental State Examination
- MRC
- Medical Research Council
- NIHR
- National Institute for Health Research
- ONS
- Office for National Statistics
- OR
- odds ratio
- PCSE
- Primary Care Support England
- PPI
- public and patient involvement
- PPIAG
- Public and Patient Involvement Advisory Group
- PPIRes
- Public and Patient Involvement in Research
- REDCap
- Research Data Capture system
- SD
- standard deviation
- SSC
- Study Steering Committee
- UEA
- University of East Anglia
- W1
- wave 1
- W2
- wave 2