Notes
Article history
The research reported in this issue of the journal was funded by the HS&DR programme or one of its preceding programmes as project number 13/54/40. The contractual start date was in January 2015. The final report began editorial review in July 2019 and was accepted for publication in January 2020. The authors have been wholly responsible for all data collection, analysis and interpretation, and for writing up their work. The HS&DR editors and production house have tried to ensure the accuracy of the authors’ report and would like to thank the reviewers for their constructive comments on the final report document. However, they do not accept liability for damages or losses arising from material published in this report.
Declared competing interests of authors
Simon Gilbody is Deputy Chairperson of the National Institute for Health Research Health Technology Assessment Commissioning Board (2017 to present) and sits on the Health Technology Assessment Post-Funding Committee teleconference (Programme Oversight Committee members to attend) and Health Technology Assessment Funding Committee Policy Group (formerly the Clinical Studies Group). Rachael Williams is employed by Clinical Practice Research Datalink, which received funding from the University of York for access to research data and services used in this study. Clinical Practice Research Datalink additionally provided funding for access to research data and services used in this study.
Permissions
Copyright statement
© Queen’s Printer and Controller of HMSO 2020. This work was produced by Jacobs et al. under the terms of a commissioning contract issued by the Secretary of State for Health and Social Care. This issue may be freely reproduced for the purposes of private research and study and extracts (or indeed, the full report) may be included in professional journals provided that suitable acknowledgement is made and the reproduction is not associated with any form of advertising. Applications for commercial reproduction should be addressed to: NIHR Journals Library, National Institute for Health Research, Evaluation, Trials and Studies Coordinating Centre, Alpha House, University of Southampton Science Park, Southampton SO16 7NS, UK.
2020 Queen’s Printer and Controller of HMSO
Chapter 1 Background
Some of the material in this chapter is reproduced from Jacobs et al. 1 Contains information licensed under the Non-Commercial Government Licence v2.0.
Serious mental illness
Serious mental illness (SMI) encompasses a set of chronic enduring conditions, such as schizophrenia, bipolar disorder and other psychoses. Although some people make a full recovery, most will develop a lifelong illness. 2 Psychosis is a key symptom or feature of SMI, typically characterised by radical changes in personality, impaired functioning and a distorted sense of objective reality, exhibited by delusions and hallucinations. Schizophrenia is a SMI marked by severely impaired thinking, emotions and behaviours. People with schizophrenia are typically unable to filter sensory stimuli and may have altered perceptions of their environment, including delusions and hallucinations. If untreated, people with schizophrenia may gradually withdraw from interactions with other people and lose their ability to take care of their personal needs. 1 Schizophrenia is a disease that usually begins in early adulthood, and the average age at onset is 18–25 years in men and 25–35 years in women. 3 Bipolar disorder is a mood disorder that causes marked emotional changes and mood swings, whereby individuals experience alternating episodes of mania, or hypomania, and depression. The lifetime prevalence of schizophrenia is around 1%,4 whereas the prevalence of bipolar disorder is about 1–2% in the UK. 5
The total monetary costs in England have been estimated at £11.8B for schizophrenia and psychoses in 2012,6 and £5.2B for bipolar disorder in 20077 (equivalent to £6.0B in 2012), with a large proportion of the economic burden attributed to service costs, informal care and lost labour market output. Therefore, SMI creates a high cost to society, as well as to the NHS.
Life expectancy for people with schizophrenia and bipolar disorder is usually around 20 years lower than for the general population;8–12 people with SMI die prematurely, the majority from preventable physical illnesses. 13 People with SMI are at higher risk of physical ill health and, thus, hospitalisation. 14 Compared with the general population, people with SMI have double the risk of diabetes, two to three times the risk of hypertension and three times the risk of dying from coronary heart disease,15 and experience a twofold to sixfold increase in deaths from respiratory disease. 16 Owing to much higher smoking rates than the general population, smoking-related diseases, heart disease and premature death are more common in people with SMI. 17 People with SMI are at a much higher risk of obesity, as the atypical antipsychotic medications they take are associated with weight gain and their illness reduces their activities and impairs their ability to exercise. 18 Alcohol use disorders are common among people with SMI and contribute to excess morbidity and mortality in this population. 19
Poor compliance with medication is well recognised among people with these diagnoses and this may lead to relapse, poorer outcomes and hospital admissions. Schizophrenia and bipolar disorder rank among the top 10 causes of disability in developed countries worldwide. 20 Despite its prevalence, considerable disease burden, poor outcomes and costs, there has been little empirical research on the processes of care for people with SMI. They are often disenfranchised, marginalised and experience stigma, and thus do not receive the same priority as people with other chronic disease conditions.
In the English NHS, a number of different services provide care for people with SMI. There has been a general trend away from long hospital stays in favour of shorter-term pharmacological stabilisation in hospital, followed by longer multidisciplinary follow-up in the community or primary care setting. Most people with SMI are treated in primary care by their general practitioner (GP). People with SMI consult their GPs more frequently21 and are in contact with primary care services for a longer cumulative time than people without mental health problems. 22,23 Evidence suggests that around one-third of patients with SMI in the UK are treated solely by their GP or other primary care clinician. 24 Primary care is therefore central to the care of people with SMI. The GP oversees care, prescribes medication and provides both mental and physical health services.
Primary care quality
Measuring quality
There are many different conceptions and definitions of quality. One commonly used definition in health care is the degree to which services increase the likelihood of desired outcomes, consistent with current professional knowledge. 25 More specifically, health care can be considered to have six key aims: (1) safety, (2) effectiveness, (3) patient-centredness, (4) timeliness, (5) efficiency and (6) equity. 26 For practical purposes of measurement, health-care quality can be further divided into three components: (1) structures (e.g. levels of staffing), (2) processes (e.g. monitoring of cholesterol levels) and (3) outcomes (e.g. mortality rates). 27 There are multiple measures of quality, some of which are routinely recorded by health services and government agencies, and others that are measured for specific purposes by researchers or interest groups. These measures are often disease specific, reflecting the division of medicine into discrete specialties.
For primary care, a disease-specific focus is problematic because most patients have a combination of physical, psychological and social problems, and one of the core requirements of primary care providers is to provide integrated care that accounts for this complexity. 28 This approach often requires co-ordination across different disciplines and agencies, some of which may lie outside the health-care community. Measuring these aspects of care is challenging and they are therefore often neglected in favour of more mechanistic measures of quality.
Primary care quality in England
The first systematic attempt to measure quality of primary care in the UK was the Collings29 study in 1950. This was damning about the quality of care provided by many general practices, describing some as ‘bad enough to require condemnation in the public interest’. 29 The outcry raised by the report stimulated reform within the profession, leading to the establishment of the Royal College of General Practitioners in 1952. This was followed in subsequent years by mandatory vocational training in general practice as a specialty; incentives for physicians to work in groups; government financial support for practice facilities, staff costs and information systems; and limited pay for performance (P4P) (e.g. for conducting cervical screening). However, by the 1990s, quality of care was still largely unmeasured, despite accumulating evidence of wide variations in care, and the main formal check on substandard care was provided by the profession itself through the General Medical Council, which dealt with reports of negligent care. A series of high-profile scandals and subsequent public inquiries in the 1990s, including the Bristol Royal Infirmary Inquiry30 and the Shipman Inquiry,31 damaged trust in the medical profession, which finally accepted the need for reform and for more robust methods to monitor and improve quality of care.
From 1997 the government implemented a series of reforms aimed at improving quality of care, supported by additional funding for the NHS. These included the creation of the:
-
National Institute for Clinical Excellence [later the National Institute for Health and Care Excellence (NICE)] to provide clinical guidelines and to conduct cost-effectiveness analysis of medical interventions
-
Commission for Health Improvement (replaced by the Healthcare Commission and then the Care Quality Commission) to regulate and inspect health and social care services
-
National Service Frameworks to provide guidelines and standards of care for some common chronic conditions.
Against this background of quality improvement efforts, in 2003 the national contract between the NHS and GPs was renegotiated in an effort to address an emerging recruitment and retention crisis. 32 It was recognised that existing methods of remuneration (including capitation payments, which constituted most of practices’ income) were at best neutral towards the quality of care provided and, in some cases, provided perverse incentives for under- or overtreatment. At that time, P4P schemes linking remuneration directly to performance on quality indicators were emerging in the USA as a potential method for improving quality of care and incentivising efficiency. It was therefore agreed that funding for practices in the UK would be increased, but that this would be achieved through a national P4P programme aimed at rewarding high-quality care.
The Quality and Outcomes Framework
In April 2004, the Quality and Outcomes Framework (QOF) was introduced as part of a new national General Medical Services contract for primary care practices in the UK. This major P4P scheme aims to reward higher-quality primary care by offering financial incentives to general practices. Participation is voluntary, but due to the size of the incentives, the vast majority of practices participate. 33 The QOF includes > 100 quality targets for clinical, organisational and patient experience indicators, based on clinical evidence and expert opinion. Under the scheme, practices earn points according to performance between a lower target threshold (which sets the minimum level of achievement required) and an upper threshold (which represents the maximal level of achievement expected for the average practice). The maximum number of points and the threshold levels vary by indicator. For example, controlling blood pressure for patients with coronary heart disease was originally worth a maximum of 19 points, with a lower threshold of 25% (i.e. blood pressure had to be controlled for at least 25% of patients to earn any points) and an upper threshold of 70% (i.e. any achievement > 70% earned the maximum 19 points). Points are converted into annual payments, adjusted for relative practice size and disease prevalence. When the QOF was introduced in 2004/5, the price per point was £75, which increased to £157 by 2013/14. By the third year of the scheme, most practices were performing above the upper thresholds for most indicators and so attained close to the maximum 1000 points available. This translated into payments of around £125,000 for an average-sized practice.
The mental health domain of the Quality and Outcomes Framework
One of the clinical domains of the QOF is mental health, specifically SMI, which is the focus of our study. Indicators have been revised over time, but since 2006/7 practices have been required to record the number of patients with SMI on their practice list, document a care plan (CP), conduct an annual review of the patient’s physical health (AR), and monitor therapeutic levels and renal and thyroid health for patients treated with lithium. We excluded the lithium-related indicators from our analysis because they typically relate to patients with bipolar and mood affective disorder who are using this mood stabiliser, a subset of all people with SMI. Our study therefore focuses on the CP and AR indicators that are applicable to all patients on the practice SMI register. We describe these indicators in more detail in Chapter 3, Care quality indicators.
Other measures of quality
The QOF, however, covers only some aspects of quality and may neglect other important unmeasured dimensions. 34 We therefore measured the quality of care using indicators from the QOF (the CP and AR indicators) and non-QOF indicators (also derived from primary care patient records). These were selected on the basis of a systematic literature review (see Chapter 2) and informed by the views and experience of our service users on the research team and our Scientific Steering Committee (SSC). Non-QOF indicators included antipsychotic polypharmacy and the continuity of GP consultations, and are described in Chapter 4, Non-Quality and Outcomes Framework measures of quality: practitioner continuity, and Chapter 5, Definition of antipsychotic polypharmacy.
Aims and objectives
All health-care systems seek ways to improve the quality of care for their patients within the constraints of the existing health-care budget. If successful high-quality care activities can be identified and adopted more widely, they may generate patient benefit in the form of improved health-related quality of life and life expectancy and wider non-health benefits (e.g. higher employment rates). Furthermore, by improving the health of the target population, high-quality care may also prevent the need for additional health care and lead to lower resource consumption. Such advances in care quality would therefore be of great interest both to patients and to policy-makers looking to improve population well-being and contain health expenditure. 35
Successful quality improvement efforts targeted at primary care providers offers a critical opportunity to reduce avoidable morbidity and upstream resource consumption. Yet, the existing evidence about the effectiveness and cost-effectiveness of primary care activities that seek to improve quality is relatively weak and often based on either small-scale studies with unclear generalisability, or expert consensus in the absence of robust evidence (see Chapter 2). There is a lack of large-scale, nationally representative studies that provide robust evidence of the association between high-quality care activities and outcomes in a real-world setting. 36,37
Our study objectives are to examine whether or not better-quality primary care for patients with SMI can improve a broad range of outcomes [accident and emergency (A&E) attendances, emergency admissions, mortality and costs, see Chapter 3, Outcomes for details]. The quality of care is assessed with two sets of quality indicators derived from primary care patient records: (1) those used in the QOF, which cover SMI-specific indicators (the CP and AR indicators) and (2) other non-QOF measures (antipsychotic polypharmacy and the continuity of GP consultations).
Our research questions (as set out in our original research protocol) were, is better quality primary care for people with SMI associated with:
-
lower rates of SMI hospital admissions
-
lower rates of ambulatory care sensitive condition (ACSC) emergency admissions
-
lower A&E attendances
-
reduced mortality
-
improvements in health outcomes [Health of the Nation Outcome Scales (HoNOS)]
-
reduced SMI costs in primary and secondary care?
Deviations from study protocol
When considering the potential mechanisms by which each of the four quality indicators we examine (CP, AR, polypharmacy and continuity of care) might impact on outcomes, we recognised that some combinations of quality indicators and outcomes are highly unlikely to arise in practice, and so decided not to test them all explicitly. We outline our approach for which indicators are analysed against which outcomes in Chapter 3 and the rationale for the outcomes used for the respective indicators is provided in the empirical Chapters 4–7.
We deviated from the protocol in our analysis of the HoNOS, an outcome measure used by clinicians in secondary mental health services (see Chapter 6). On closer examination of the potential mechanisms through which primary care quality might impact on HoNOS outcomes within a secondary care setting, it became apparent that the causal pathway was unclear: any effect would need to be mediated by secondary care. We therefore changed this outcome to re-entry to mental health specialist community care (from primary care). Our original intention was to examine the level of severity of patients re-entering specialist mental health care using a clinician-rated outcome measure, the HoNOS. However, we faced practical challenges in implementing this analysis. HoNOS is recorded only for individuals treated in secondary care. Therefore, if primary care prevents relapse in people with SMI, the impact on HoNOS for these individuals is unknown. In addition, HoNOS is not recorded at regular intervals for people who access secondary care services and many individuals in our data set had only one HoNOS score. In theory, all patients have a HoNOS score completed on entry to mental health care, or soon after, but we found this not to be the case in the data. We looked at episodes when a patient re-entered specialist secondary mental health care after at least 12 months without secondary care activity. A HoNOS score was documented within a month either side of that date in only 23% of such episodes, and in only 36% of such episodes was a HoNOS score documented within 6 months either side. Such a sample of patients would not have been selected at random, as we could not assume that HoNOS completion was random. Rather, we suspected that those who had a HoNOS completed were different in some unobservable but systematic way from those who did not, and that any results we generated would not be generalisable to the wider SMI population.
We also deviated from the protocol in our analysis of costs (see Chapter 7). Initially, it was unclear that we would be able to obtain linked data to analyse mental health community care, but this became available during the project and we were therefore able to examine costs in primary, secondary and community mental health care.
We also added an additional outcome to the set of health-care utilisation measures, by including all unplanned admissions.
Patient and public involvement
Aim
It was determined from the outset that patient and public involvement (PPI) would be a key aspect of this project. The benefits of involving experts by experience, particularly people with SMI, were threefold: (1) it allowed us to address questions that matter to service users; (2) it enhanced the interpretation of results, ensuring that the findings are plausible; and (3) it allowed continuing support for the dissemination of findings of this research in a manner suitable for lay people (e.g. production of a video showcasing the results of the study).
Methods
Two of our team members, LA and CD, have lived experience of SMI. These experts, by experience, have actively engaged throughout the project, with LA participating in early discussions with the team to help shape and clarify the research questions, including during the grant-writing phase. This helped focus on quality indicators that matter to service users. LA and CD were also members of the SSC, which provided them a further opportunity to steer the research programme.
Both experts by experience have played a pivotal role in the research team. They attended regular research team meetings, in which research findings were discussed, allowing them the opportunity to relay their interpretation of the results. This in turn influenced further analyses. Meeting minutes were distributed to these individuals, alongside the rest of the team, so that they could keep up to date with the progress of the project.
The experts, by experience, were able to liaise with and seek the expertise of various team members, as and when needed. This enabled them to feel supported and have adequate training for the role. The principal investigator was also available to help clarify things, when desired. Both individuals were reimbursed, based on guidance provided by INVOLVE. 38
Study results
As part of the team, LA and CD actively engaged with the literature review and contributed to the identification of quality indicators in primary care. The involvement of these individuals, and their unique perspective, allowed the team to keep sight of what is most important to service users. Both experts, by experience, have felt part of the team and have co-authored four articles during the project, with other research papers and dissemination activities to follow. 39–42
Discussion and conclusions
The insight provided by LA and CD has enhanced other team members’ understanding of SMI and the nature of the illness, and has had a positive impact on the project. They have participated in the project from its inception to conclusion and have felt genuinely engaged throughout.
Reflections and critical perspective
It was beneficial to have two experts by experience working on the project, as this facilitated the engagement of at least one individual at all times, particularly during periods in which one or the other may have been unable to attend because of illness.
Chapter 2 Systematic literature review of primary care quality
This chapter is based on Kronenberg et al. 39 This is an Open Access article distributed in accordance with the terms of the Creative Commons Attribution (CC BY 4.0) license, which permits others to distribute, remix, adapt and build upon this work, for commercial use, provided the original work is properly cited. See: http://creativecommons.org/licenses/by/4.0/. This includes minor additions and formatting changes to the original text.
Introduction
Quality and Outcomes Framework indicators are targeted at high-priority disease areas for which primary care has principal responsibility for ongoing care and when there is good evidence that improved primary care will have health benefits. However, as noted in Chapter 1, Other measures of quality, the QOF may neglect important unmeasured aspects of quality of care43 and the incentives may result in tunnel vision44 or a focus on activities that are incentivised at the expense of other non-incentivised activities. 45,46
We therefore performed a systematic review of the literature and interrogated international databases to identify potential quality indicators that we could include in our study. We sought to include indicators identified in the literature into our analysis as additional measures of quality of care, rather than focusing only on QOF indicators. These additional measures could potentially supplement or even replace indicators included in the QOF for people with SMI and could potentially be incentivised in primary care. Identifying indicators of primary care quality for people with SMI could help shed light on neglected areas of care, as well as providing the basis for incentive schemes aimed at improving quality.
A major focus of our analysis was the source of the data on which the indicators were based. Those requiring primary data collection, for example via surveys of patients or health professionals, or retrospective auditing of patient records, would be very challenging to examine in routine data. This was an important consideration for inclusion in our analysis. Previous literature reviews on quality indicators have focused on SMI in secondary care,47,48 whereas our review was the first to focus specifically on people with SMI treated in primary care.
Methods
A systematic review of primary care quality indicators for people with SMI was conducted39 in February 2015, with the aim of identifying quality indicators in addition to those already included in the QOF.
The base search was constructed using MEDLINE and adapted to other resources. The following databases were searched: Applied Social Sciences Index and Abstracts (ASSIA); Cochrane Central Register of Controlled Trials (CENTRAL); Cochrane Database of Systematic Reviews; Conference Proceedings Citation Index – Science (CPCI-S); Database of Abstracts of Reviews of Effects (DARE); EMBASE; Ovid MEDLINE® In-Process & Other Non-Indexed Citations and Ovid MEDLINE®; PsycINFO and MEDLINE. The full strategy for MEDLINE as a template is available in Appendix 1.
Additionally, previous reviews with overlapping aims were searched and we contacted authors to ask for their indicators (most notably Stegbauer et al. 48 and Großimlinghaus et al. 49). The quality indicator database of the Agency for Healthcare Research and Quality50 was also searched for indicators relevant to primary care. The final selection of indicators was informed by the views of our SSC.
Inclusion and exclusion criteria
We sought published examples of potential quality indicators that could readily be collected in primary care with reference to routine data. Search terms were identified by an information specialist, in conjunction with the research team. Included papers had the terms serious mental illness AND primary care AND quality indicator, including alternative spellings and synonyms. Studies on children, and on mental illnesses other than SMI, were excluded. All studies from January 1990 to February 2015 were considered for inclusion. No language restrictions were applied, although all search terms were in English, and all studies in English, German, Dutch and Afrikaans were considered due to the authors’ combined language knowledge.
Study selection
All titles were first reviewed by MG, TK, TD, RJ and Christoph Kronenberg (CK), a former member of the research team. All studies that two members of the research team indicated as potentially relevant were included in the abstract screening process. All abstracts were screened by LA, MG, TK, TD, RJ and CK, and full papers were obtained if two members of the team judged the abstract potentially relevant or in scope (i.e. covering SMI, primary care and quality indicators). Full papers were divided into four groups and independently reviewed by MG and CD, TD and LA, TK and SG, and RJ and CK. The focus of the selection was to identify papers that included relevant quality indicators that could be applied in primary care. It was evident that the definition of primary care varies between different countries, so we included indicators with elements of shared care between primary and specialist settings (e.g. prescribing and monitoring of antipsychotic medication), while acknowledging that, in some countries, those indicators may be more applicable to secondary care. Our search strategy complied with the Preferred Reporting Items for Systematic Reviews and Meta-Analyses (PRISMA) checklist.
Data extraction and analysis
A short description of each indicator from each paper was extracted and the descriptions for similar indicators were merged. The indicators were grouped into six relevant domains [(1) continuity of care, (2) substance abuse, (3) access to care, (4) medication management, (5) mental health assessment and care and (6) physical health]. The domains were selected by the research team as representing broad areas that would encompass all the chosen indicators. Given the main focus of our study, we decided if each indicator could, in principle, be measured from routine data or if primary data collection would be necessary. Furthermore, we checked if the identified indicators had ever been included in the QOF. We also assessed the quality of the evidence of the included studies using an adaptation of the Grading of Recommendations Assessment, Development and Evaluation (GRADE) guideline,51 and rated the quality of the evidence as high [systematic reviews or randomised controlled trials (RCTs)], moderate (non-randomised control studies or unsystematic reviews), low (expert opinion or uncontrolled studies) or not applicable (measure was extracted from the grey literature).
Indicator selection
We presented the final list of indicators to our SSC and discussed the list within the research team, as well as with our PPI team members. Based on these discussions, the evidence from the literature review and how readily the indicators could be translated into appropriate quality measures from routine data, we decided to choose two quality indicators that were not already included in the QOF for analysis in this study. Although some of the remaining potential indicators were, in theory, measurable from routine data, they would be unable to be measured in the primary care data used for this study owing to limitations of the clinical coding (e.g. quality of the data, missingness).
Results
In total, 1847 studies and further database sources were identified using the search. The split was ASSIA (n = 34), CENTRAL (n = 96), Cochrane (n = 12), CPCI-S (n = 125), DARE (n = 28), EMBASE (n = 738), Ovid MEDLINE (n = 537), PsycINFO (n = 271) and six further database sources (Agency for Healthcare Research and Quality50, Stegbauer et al. ,48 Großimlinghaus et al. ,49 Parameswaran et al. 52 and NICE36). After removing duplicates using bibliographic software [EndNote X7.3 (Clarivate Analytics, Philadelphia, PA, USA) and Zotero 4.0 (Center for History and New Media, George Mason University, Fairfax, VA, USA)], 1303 records remained. Title screening reduced this to 356, excluding those that were not about quality indicators, primary care, mental illness or were not included in our definition of SMI (e.g. depression or substance abuse disorders). Abstract screening reduced the records to 113, with similar reasons for exclusion. Finally, from those 113 records, 86 were excluded and 27 records were included in the review (Figure 1).
FIGURE 1.
The PRISMA flow diagram for systematic review of quality-of-care indicators for patients with SMI. Reproduced from Kronenberg et al. 39 This is an Open Access article distributed in accordance with the terms of the Creative Commons Attribution (CC BY 4.0) license, which permits others to distribute, remix, adapt and build upon this work, for commercial use, provided the original work is properly cited. See: http://creativecommons.org/licenses/by/4.0/. The figure includes minor additions and formatting changes to the original text.
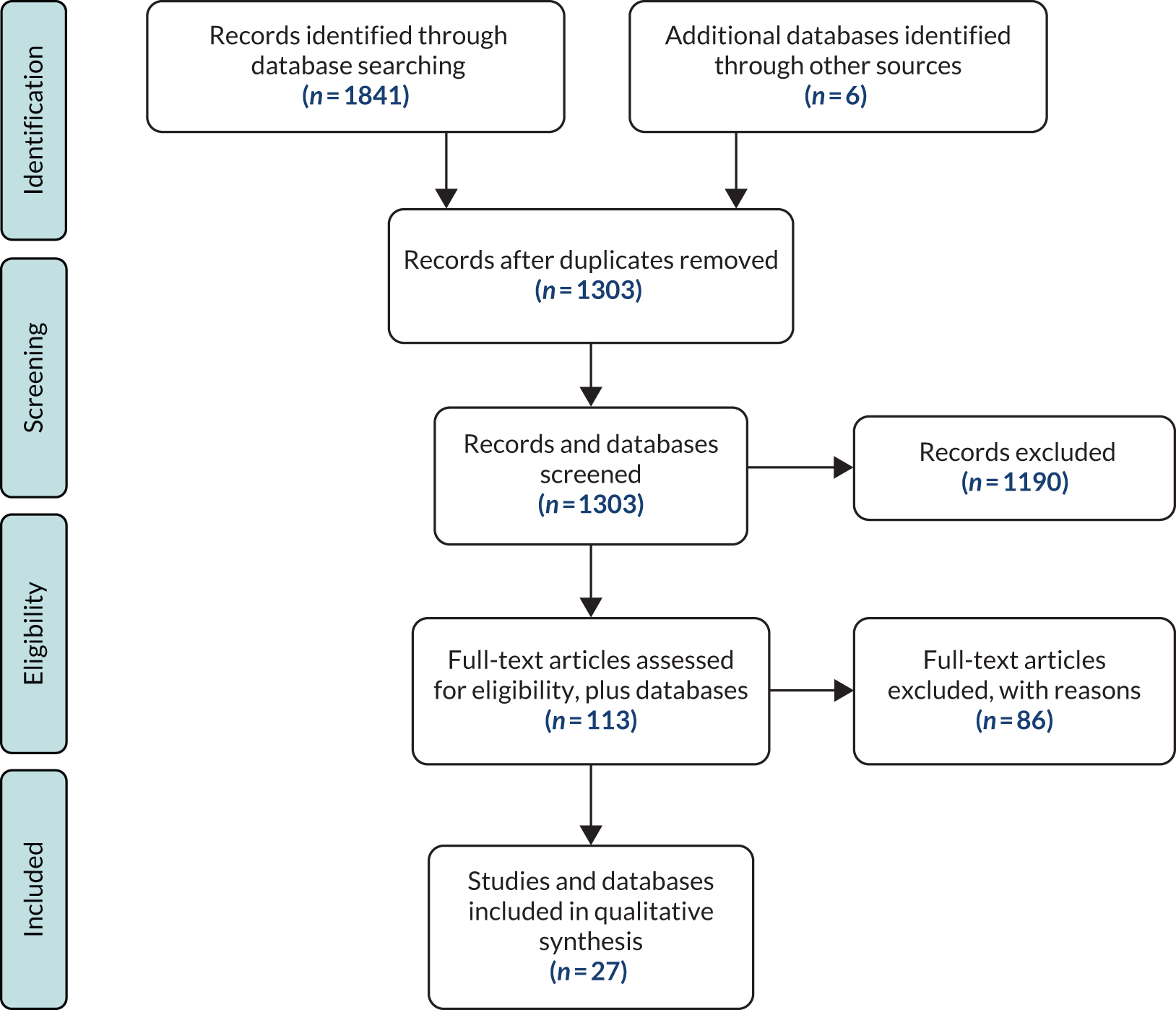
Out of these 27 records, a final set of 59 different indicators was extracted. The final list of indicators are shown in Table 1.
Number | Description | Data source | QOF | Reference(s) |
---|---|---|---|---|
Co-ordination of care | ||||
1 | Co-ordinated care: identify key worker (social worker or CPN) | Routine data | 53 | |
2 | Staff continuity: good communication between staff and infrequent staff changes | Routine data | 54 | |
3 |
Continuity and co-ordination: CONNECT is a patient questionnaire with 72 items, each rated on a 5-point scale, with 13 scales and one single-item indicator (general co-ordination – ‘overall is your mental health treatment well co-ordinated?’) Primary care scales: ‘how often is psychiatrist in contact with your primary care doctor?’ (never, rarely, sometimes, often, always) |
Primary data | 55 | |
4 | Total number of follow-up contacts during treatment episode after initial evaluation | Routine data | 56 | |
Substance misuse | ||||
5 | Patients with SMI who smoke who are offered tobacco counselling/help to stop smoking | Routine data | 36 | |
6 | Alcohol misuse screening | Routine data | ✓ | 50 |
7 | Screening for illicit drug use, type, quantity and frequency | Routine data | 50 | |
8 | Referral to substance misuse disorder specialty care, if appropriate | Routine data | 52 | |
9 | HIV screening with co-occurring substance misuse for SMI service users | Routine data | 57 | |
Service provision and access to care | ||||
10 | Practice can produce register of all patients with SMI | Routine data | ✓ | 58 |
11 | Service user registration with a primary health organisation | Routine data | 24 | |
12 | Markers of care recorded: contact with secondary health services, written CPs, 6-month mental health review, identified care co-ordinator, evidence of physical examination | Routine data | ✓ | 59 |
13 | Patients who do not attend the practice for their annual review who are identified and followed up by the practice team | Routine data | ✓ | 50 |
14 | System contact: number of patients in contact with the treatment system | Routine data | 52 | |
15 | Surveillance to prevent relapse | Routine data | 54 | |
16 | Crisis management and out of hours services | Routine data | 55 | |
17 | Access to services and range of services | Routine data | 54 | |
18 | Family care: record of families living with person with schizophrenia | Routine data | 53 | |
19 | Duration of untreated psychosis: number of recently diagnosed patients | Routine data | 52,60 | |
20 | Waiting time between registration and start of treatment | Routine data | 52 | |
Medicines management | ||||
21 | All current medication clearly available at all consultations: known drug dosages, frequencies, history of side effects, review date | Routine data | 53 | |
22 | Monitor patients suffering extra pyramidal effects, check compliance | Routine data | 61 | |
23 | Assess weight gain, use of concomitant medication | Routine data | ✓ | 62 |
24 | Use of lithium: plasma lithium levels monitored regularly | Routine data | ✓ | 63,64 |
25 | Percentages of bipolar service users prescribed antidepressants and anxiolytics | Routine data | 63,64 | |
26 | Proportion of patients who are receiving depot antipsychotics who have appropriate laboratory screening tests | Routine data | 65 | |
27 | Patients have their antipsychotic medication reviewed regularly, considering symptoms and side effects: appropriate referral to specialist | Routine data | 66 | |
28 | Polypharmacy: reduce number of patients using more than four psychotropic drugs at the same time | Routine data | 67 | |
29 | Monitoring patients with neurological, sexual, sleeping and sedation side effects | Routine data | 68 | |
Mental health assessment and care | ||||
30 | Percentage of patients given annual mental health review by a GP | Routine data | ✓ | 69 |
31 | Comprehensive mental status examination and history conducted in patients with a new treatment episode | Routine data | 50,53 | |
32 | Referral for specialist mental health assessment | Routine data | 63 | |
33 | Comprehensive assessment of comorbid psychiatric conditions and response to treatment | Routine data | 50 | |
34 | Reassess severity of symptoms | Routine data | ✓ | 70 |
35 | Examined for duration of untreated psychosis | Routine data | 60 | |
36 | Delayed diagnosis | Routine data | 71 | |
37 | Informal carer contacts | Routine data | 54 | |
38 | Information on employment status | Routine data | 53 | |
Physical health assessment and care | ||||
39 | Diabetes monitoring for people with diabetes and schizophrenia | Routine data | 50 | |
40 | Diabetes and cholesterol monitoring for people with schizophrenia and diabetes | Routine data | 50 | |
41 | Diabetes screening for people who are using antipsychotic medications | Routine data | 50 | |
42 | Blood pressure screening for patients with diabetes | Routine data | ✓ | 50,72–75 |
43 | Weight management/BMI monitoring | Routine data | ✓ | 50,72–75 |
44 | Proportion with increased BMI/abdominal waistline | Routine data | ✓ | 50,72–75 |
45 | Patients with diabetes who received education about diabetes, nutrition, cooking, physical activity or exercise | Routine data | 50 | |
46 | Counselling on physical activity and/or nutrition for those with documented elevated BMI | Routine data | ✓ | 50 |
47 | Retinal exam for patients with SMI who have diabetes | Routine data | 50 | |
48 | Foot exam for patients with SMI who have diabetes | Routine data | 50 | |
49 | Hypertension counselling: patients with hypertension who received education services related to hypertension, nutrition, cooking, physical activity or exercise | Routine data | 50 | |
50 | Hypertension: recording and monitoring patients with hypertension and high blood cholesterol (LDL) | Routine data | ✓ | 50,72–75 |
51 | Breast cancer screening for women | Routine data | 50 | |
52 | Colorectal cancer screening | Routine data | 50 | |
53 | Proportion of patients who have an increased blood pressure | Routine data | ✓ | 50,72–75 |
54 | Proportion of patients who have an increased blood glucose level | Routine data | ✓ | 50 |
55 | Proportion of patients who have low levels of glycosylated haemoglobin | Routine data | ✓ | 50 |
56 | Proportion of patients who have increased level of blood lipids | Routine data | 48 | |
57 | Comprehensive physical health assessment with appropriate advice | Routine data | ✓ | 70 |
58 | Patients with diabetes who received psychoeducation related to weight (BMI), diabetes (blood glucose levels) | Routine data | 76 | |
59 | Medical attention for nephropathy | Routine data | 77 |
Table 1 shows that of the 59 indicators, 52 could potentially be assessed using routine data and seven would require primary data collection from patients or professionals. Of these 59 indicators, 17 are, or have previously been, included in the QOF. A large proportion of the indicators relevant to primary care are in the physical health domain. Another large subset of indicators relate to the process of receiving care (e.g. continuity of care, access to services and frequency of contacts).
Table 2 shows the quality of evidence of the included studies from which the indicators were drawn. Two studies were rated as high quality (Cochrane or systematic review, RCT), three studies as moderate quality (non-randomised study or unsystematic review), 19 studies as low quality (expert opinion, uncontrolled studies) and three studies were of uncertain quality, having been identified from the ‘grey’ literature (e.g. non-government organisation documents or databases).
Study | Description of study | Strength of evidencea |
---|---|---|
Parameswaran et al.52 | A total of 656 measures of quality of mental health care identified in earlier work are rated in importance, validity and feasibility using a modified Delphi process | 3 |
NICE63 | NICE treatment guidelines for bipolar disorder | 4 |
NICE36 | NICE treatment guidelines for schizophrenia | 4 |
Agency for Healthcare Research and Quality50 | The Agency for Healthcare Research and Quality provides a database of quality indicators that was used during the grey literature search | 4 |
Lester et al.58 | Focus groups with patients, GPs and nurses were conducted to explore how to improve care in cases of acute mental health crises | 3 |
Sweeney et al.54 | Structured interviews were conducted with 167 individuals suffering from psychoses to establish a concept of service user-defined continuity of care | 3 |
Ware et al.55 | This study reports on the field testing of an interview-based measure of continuity of care | 3 |
Cerimele et al.56 | Narrative description of 740 bipolar primary care patients who participated in a mental health integration programme. Quality-of-care outcomes were derived from patient disease registry | 3 |
Pincus et al.70 | Discussion on the barriers to measuring quality of care in the mental health arena combined with a short list of potential quality measures | 3 |
Holden53 | This study audited 16 GPs on their care for 266 schizophrenia patients and observed that the audit led to improved recording of a range of quality indicators | 3 |
Swartz and MacGregor57 | The authors of this paper argue that in South Africa the role of mental health nurses has been altered to focus on violence, substance abuse and HIV/AIDS and should be refocused on psychiatry care in the primary care setting | 3 |
Ruud60 | The author summarises the literature on quality of care in mental health services in Norway for the years 2008–9 | 3 |
Highet et al.71 | Interviews with 49 bipolar patients to describe experience in primary care in Australia. Eight themes for improvement of the primary care experience are outlined | 3 |
Lader61 | Expert review of the standards of care in schizophrenia to reduce side effects, while achieving best treatment outcomes | 3 |
Haro and Salvador-Carulla62 | Observational study following 11,000 patients who were on or changing antipsychotic medication, to determine the best course of treatment with respect to symptoms, quality of life, social functioning and other outcomes | 2 |
Caughey et al.64 | Development, expert review and assessment of the evidence base for, and validity of, medication-related indicators of potentially preventable hospitalisations | 3 |
Busch et al.65 | Observational study examining trends in four measures of quality over time in the USA | 2 |
Young et al.66 | Uncontrolled study looking at differences in quality of care as variations from national guidelines | 3 |
Nayrouz et al.67 | Evaluation of an integrated care approach between primary care and community care focused on people with SMI | 3 |
McCullagh et al.59 | This observational study looks at urban vs. rural differences in quality of care for psychoses, as well as the difference in quality of care conditional on contacts with secondary care | 3 |
Rodgers et al.69 | Audit of quality of care in 822 Scottish patients with schizophrenia | 3 |
Osborn et al.72 | Randomised trial to evaluate the impact of a nurse-led treatment to improve screening for CVD in the SMI population | 1 |
Yeomans et al.73 | Evaluation of a computer-based physical health screening template vs. NICE guidelines for the SMI population | 3 |
Mitchell et al.74 | A systematic review and meta-analysis of screening practices with respect to metabolic risks for psychosis patients | 1 |
Roberts et al.75 | A retrospective view of case notes in 22 general practices to determine whether or not patients with schizophrenia receive equitable physical health care | 3 |
Mainz et al.68 | Description of the Danish National Indicator Project, which intends to document and advance quality of care | 3 |
Druss et al.77 | The study compared diabetes performance measures in US Medicaid enrolees with and without mental comorbidity | 2 |
Only a very few RCTs have evaluated quality indicators. Two RCTs were reviewed in Cimo et al. ,76 which produced evidence on the effectiveness of lifestyle interventions for people with type 2 diabetes and schizophrenia or schizoaffective disorder. However, more often, indicators were based on expert consensus or small cross-sectional studies.
Many of the indicators identified were derived from a database of indicators produced by the Agency for Healthcare Research and Quality,50 and the strength of evidence underpinning the individual indicators is variable.
From the large set of quality indicators reviewed, we identified two indicators that could potentially be created and adapted from the routine data to which we had access: continuity of care (indicator 2) and polypharmacy (indicator 28). Our SSC, our PPI team members and the literature all suggested that these two areas were important aspects of quality of primary care for people with SMI. Although the quality of the evidence for these indicators was rated as weak in this review, we felt that there was sufficient interest to suggest that they would be worth exploring as important quality measures. The two indicators we constructed are described in Chapter 3, Non-Quality and Outcomes Framework indicators.
Discussion
Summary of findings
To our knowledge, this was the first attempt to identify potential indicators of quality of primary care for people with SMI in a systematic way. Although we identified > 50 indicators that could potentially be captured and monitored using routine data, crucially, we note that the quality of the available evidence underpinning many of the indicators is relatively weak. We identified two indicators for further analysis on their association with outcomes using routine data: (1) continuity of GP care and (2) antipsychotic polypharmacy.
Strengths and limitations
The feasibility of collecting data for any set of quality indicators will vary across different health-care systems. Many countries have insurance or other systems, which routinely collect activity data in primary care. Some indicators are likely to require more effort to collect (e.g. patient questionnaires for perceived continuity of care). Our study focused specifically on finding indicators that could be monitored at relatively low cost to the UK health-care system.
The list of quality indicators identified here is much broader and more encompassing than the current list of indicators contained in the QOF SMI domain. However, even if good-quality metrics were identified for use, criticisms would remain around their adoption. These include measuring only what can be measured (in routine data), instead of focusing on measuring what matters, and the risk of unintended consequences arising from prioritising some activities at the expense of other non-incentivised activities. Moreover, there are gaps in the literature and in the indicators identified, as the service user perspective is not well represented in the literature. There is also an absence of quality indicators around aspects of the social environment, such as the stability of housing for people with SMI. Although such factors are important and may well influence health outcomes, the extent to which primary care could influence these factors may be very limited and hence it may not be appropriate to hold primary care practitioners responsible for improving quality in these domains. Finally, our search excluded non-published indicators and those written in languages other than those listed earlier (see Inclusion and exclusion criteria).
Implications for research and practice
Donabedian’s27 conceptual framework of quality of care suggests that indicators can usually be divided into three subcategories: (1) structure, (2) process and (3) outcome measures. As noted above, the vast majority of indicators included in this review relate to processes of care and although aspects of process are highly relevant, especially to patients, it is important to establish whether or not quality indicators also promote improved health outcomes. If so, there is a case for their inclusion in the QOF and other initiatives aiming to improve the care of people with SMI. For physical conditions, improvements in processes of care in primary care settings have been found to be associated with modest improvements in intermediate outcomes (e.g. cholesterol levels78) and quality of life,79 but associations with patient outcomes, such as emergency hospital admission, are weaker. 80 For SMI, the evidence is much more limited and suggests that higher provider performance on processes may not be associated with better patient outcomes. 43
This evidence gap is something that this research report explicitly addresses. We chose two areas in which we could construct a range of indicators from routine data (continuity of care and polypharmacy) and examine their respective associations with outcomes in Chapters 4–6.
Chapter 3 Study framework
Introduction
As noted in Chapter 1, Aims and objectives, the overarching aim of this research was to examine the relationship between four indicators of primary care quality (two indicators from the QOF and two non-QOF indicators) and various measures of health-care utilisation, costs and outcomes.
A key contribution of this study was the use of linked routine data, which, for the first time in England, provided linkage and coverage of the full care pathway of people with SMI. This enabled the research team to track activity and outcomes through primary, community, hospital and A&E care settings.
This chapter describes the study design, the quality-of-care indicators, the outcomes, the data sources, the covariates used as controls and the study samples used.
Study design
This retrospective observational study followed a cohort design, in which a group of people with a confirmed diagnosis of SMI are followed until they experience an outcome or an event of interest (e.g. a hospitalisation), or until the end of follow-up (end of study period or the patient moved practice). The relationship of interest was that between the frequency or timing of these outcomes or events, and the existing exposure to the indicators of quality of primary care. Information on patients’ socioeconomic characteristics, medical history, the care they received, as well as their eligibility for inclusion in this study, were taken from linked primary and secondary care administrative records (see Data sources). The Strengthening the Reporting of Observational Studies in Epidemiology (STROBE) checklist for observational studies using a cohort design is provided in Appendix 2.
Retrospective studies based on administrative data have a number of advantages over prospective studies that require primary data collection. These include the low cost and timeliness of data acquisition, long follow-up over several years, wide coverage of the patient population without the step of selection into a trial and representativeness of current clinical practice (high external validity). However, administrative data are typically collected for other purposes than health services research (e.g. reimbursement, documentation of patient care) and so may not contain all the information that ideally is required to fully investigate the research question. If, for example, important confounding factors are not measured and, therefore, cannot be controlled for in statistical analysis (i.e. omitted variables), any inference drawn from routine administrative data may contain bias (low internal validity). Other potential biases include reverse causation and (self-)selection into treatment.
We employed two approaches to reduce the potential for bias in our estimates. First, we used detailed information on patients’ characteristics and health condition, as recorded by primary care professionals (i.e. GPs, nurses, health-care assistants) to control for observed differences in health-care needs across patients. All control variables were measured at baseline, thereby eliminating the risk that they were contaminated by receipt of the treatment of interest. The construction of the control variables is described in detail in Covariates. Second, when possible, we employed statistical methods that focused on only within-subject variation in treatment exposure over time, which allowed us to control for the influence of any time-invariant unobserved patient heterogeneity (described further in Chapter 4, Statistical analysis).
Statistical methodology
The longitudinal nature of the linked data used in these analyses meant that we were able to track over time the events occurring in each patient’s health-care records. To make best use of this rich data set, we applied survival analysis (also termed ‘time-to-event’ or ‘duration’ analysis) methodology, as this approach captures not only the sequence of those events at the patient level, but also their timing. This means that the analysis took into account, for example, if a quality indicator occurred before a hospitalisation, and also how much time elapsed between the quality indicator being recorded and the start of the hospitalisation. We hypothesised that if better-quality care improved patient health, even when this did not result in complete prevention of outcomes like hospitalisation, it would take longer for patients’ health to deteriorate to the point of hospitalisation. Survival analysis is able to capture such impacts.
For some patients, we observed multiple occurrences of a particular type of event (such as A&E department attendance). It is possible to use survival analysis methods that allow for such multiple outcome events per person; however, for our main analyses we studied only the duration to the first event for each person. The reason for this was that, based on clinical advice, we hypothesised that events such as A&E department attendance, hospitalisation or re-entry to specialist care would result in changes to the patient’s care in each of those clinical settings, which would not be observed in our data set. By focusing on the occurrence to the first event, we avoid this source of potential omitted variable bias.
Two different types of survival analysis are employed in this report: continuous- and discrete-time survival analysis. The former is used in Chapter 5, when duration to event is measured in continuous time, with day-level information on events in the analysis. The latter, used in Chapters 4 and 6, allocates time into ‘discrete’ periods (such as quarters or years), with events known to occur between the start and end of a given discrete time period. This approach was taken to allow us to examine a quality-of-care indicator that is measured over discrete periods rather than on a continuous basis (as detailed in Chapter 4, Statistical analysis). The focus in survival analysis is on the instantaneous risk, or hazard of an event taking place (e.g. a hospitalisation). The results are interpreted in terms of the hazard ratio (HR). A HR > 1 (< 1) indicates an increase (decrease) in the hazard of the outcome occurring at a given point in time associated with a unit change in the explanatory variable. Alternatively, a HR > 1 (< 1) indicates a shorter (longer) time until the event occurs. The discrete-time model in Chapter 4 can be obtained by grouping time in the continuous-time Cox model and the results (exponentiated coefficients) can also be interpreted in terms of HRs. 81 The models we used are outlined in each chapter.
One complexity of these analyses is that the primary care quality indicators we study vary over time for an individual patient. This necessitated the use of survival methods, which allowed for time-varying covariates in the model. Often in survival analysis, results would be presented using graphical representation of the survival distributions of two groups of patients (such as treatment and control). Known as the Kaplan–Meier curves, these graphs compare groups of patients based on the level of a covariate. However, in our studies, the groups are based on primary care quality (e.g. receipt or not of a CP). This means that patients could switch between groups over time as their care changed. We therefore do not present the results graphically, as such depictions would be unhelpfully complex.
For the analysis of the relationship between primary care quality and NHS costs in Chapter 7, we did not use survival analysis. Instead, we constructed a panel data set of repeated observations on each patient and estimated two-part models (as detailed in Chapter 7, Statistical analysis).
All analyses were performed using the suite of survival models provided in Stata® 14 (StataCorp LP, College Station, TX, US).
Care quality indicators
The quality of primary care was assessed using four measures derived from primary care patient records: (1) CP, (2), AR, (3) GP continuity and (4) antipsychotic polypharmacy. The first two measures were QOF indicators and the last two non-QOF indicators (which we constructed based on the results of the literature review reported in Chapter 2). All indicators are described below and in subsequent chapters.
Quality and Outcomes Framework indicators
The care plan indicator
The CP indicator requires a comprehensive CP to be agreed with individuals and their families or carers, and documented in primary care records. It is designed to reflect good professional practice, and should cover the patient’s current health and social care needs and how these are met. Co-ordination arrangements with secondary care and patient preferences for contacts and treatment in the event of a clinical relapse must also be documented. If the patient is treated under the care programme approach (CPA), that CP, although arising from secondary care, can also be used to meet the requirements of the QOF in primary care. 82 The rationale for including this indicator in the study was that it is intended to reflect good-quality care for people with SMI.
The annual review indicator
The AR indicator should cover use of alcohol, drugs and smoking behaviour, and offer appropriate checks for blood pressure, cholesterol, body mass index (BMI) and diabetes. The review may also include cervical screening attendance and medication review. The rationale for including this indicator in the study is that patients with serious mental health problems are at increased risk of physical ill health,83 are less likely to be offered health promotion advice,84 and are far more likely to smoke85 and to have smoking-related diseases,86 than the general population. People with schizophrenia are also at increased risk of impaired glucose tolerance and diabetes. 87,88
Table 3 shows the development of the CP and AR indicators over time within the QOF. The CP indicator definition remained unchanged over financial years 2006/7–2010/11, after which payment thresholds were increased annually. The AR indicator definition also remained unchanged during 2006/7–2010/11. From financial year 2011/12, the indicator was split into its physical care review constituent parts: alcohol consumption, BMI, blood pressure, total cholesterol, blood glucose and cervical screening test. The construction of these indicators is described in more detail in Chapter 4, QOF indicators of quality.
Indicator | Financial yeara | |||||
---|---|---|---|---|---|---|
2006/7–2010/11 | 2011/12 | 2012/13 | 2013/14 | |||
CP | The percentage of patients on the register who have a comprehensive CP documented in the records agreed between individuals, their family and/or carers as appropriate | MH6 (25–50%) | MH10 (25–50%) | MH10 (30–55%) | MH002 (40–90%) | |
AR | Review | The percentage of patients with schizophrenia, bipolar affective disorder and other psychoses with a review recorded in the preceding 15 months. In the review there should be evidence that the patient has been offered routine health promotion and prevention advice | MH9 (40–90%) | |||
Review constituents | Alcohol consumption | MH11 (40–90%) | MH11 (50–90%) | MH007 (50–90%) | ||
BMI | MH12 (40–90%) | MH12 (50–90%) | MH006 (50–90%) | |||
Blood pressure | MH13 (40–90%) | MH13 (50–90%) | MH003 (50–90%) | |||
Total cholesterol : HDL ratio | MH14 (40–80%) | MH19 (45–80%) | MH004 (45–80%) | |||
Blood glucose | MH15 (40–80%) | MH20 (45–80%) | MH005 (45–80%) | |||
Cervical screening | MH16 (40–80%) | MH16 (45–80%) | MH008 (45–80%) |
Non-Quality and Outcomes Framework indicators
Continuity of general practitioner consultations
Continuity of practitioner care is a widely accepted core principle of primary care, and denotes the connected and coherent care that is consistent with the health needs and personal circumstances of a patient. 89 It is considered important to ensure effective and efficient health care, and is believed to be essential for high-quality patient care. Three major types of continuity of care are commonly distinguished, namely (1) management, (2) informational and (3) relational continuity.
The QOF indicator ‘presence of current comprehensive CP’ can be considered an informational measure of continuity, as it captures co-ordination arrangements and information sharing with secondary care.
We used three well-recognised and widely applied indices measuring different dimensions of GP continuity, which reflect management and relational continuity, but are not QOF indicators. Dispersion across different GPs within a practice involved in the patient’s care is measured using the Continuity of Care Index (COC), density of visits to a single GP is measured with the Usual Provider of Care Index (UPC) and the pattern of visits across a practice is measured with the Sequential Continuity Index (SECON). These are described in more detail in Chapter 4, Non-Quality and Outcomes Framework measures of quality: practitioner continuity.
Inappropriate polypharmacy
Antipsychotic polypharmacy is the co-prescription of two or more different antipsychotic drugs for an individual patient. 90 It is sometimes used in clinical practice, usually for people with established schizophrenia, when monotherapy is considered ineffectual, to improve therapeutic response. However, there is little empirical evidence that polypharmacy is more effective than monotherapy,91 and the equivocal evidence on the risks and benefits mean that it is not generally recommended for use in routine clinical practice.
Outcomes
We investigated the impact of primary care quality on four types of outcomes. The first type, measures of health-care utilisation, included unplanned hospital admissions and presentations to an A&E department. Subtypes of unplanned hospital admissions are of particular interest, including admissions for SMI, admissions for conditions thought to be particularly amenable to primary care and ACSCs, such as diabetes, angina, cellulitis and vaccine-preventable diseases. (The codes used to define SMI and ACSC admissions are listed in Appendix 2.) A&E department presentations of all causes, both physical and mental health problems, are also examined, as they are generally much higher for mental health service users than for the general population92 and are a precursor to unplanned hospital admissions. Attendances at emergency units have been increasing93 and evidence-based approaches to reduce emergency attendances are urgently needed. These outcomes are analysed in Chapters 4 and 5. We acknowledge that the context is important, and for some individual patients in specific circumstances, hospital admissions may not necessarily be inappropriate or a signal of poor outcomes. However, in general, there is widespread agreement that reducing this type of utilisation is beneficial to most patients.
The second outcome was mortality, which is a key outcome indicator for people with SMI and is analysed in Chapter 5. As described above, people with SMI have 15–20 years lower life expectancy than the general population, driven largely by premature mortality from preventable or modifiable physical illnesses.
The third outcome was (re-)entry to specialist mental health care and is analysed in Chapter 6. This outcome captures the situation in which patients who were not seen within specialist mental health services for at least 1 year – suggesting that they were stable enough to be managed fully in primary care – then re-engaged with specialist mental health services, indicating a deterioration in their mental health, requiring specialist expertise. Again, we acknowledge that this may not in all contexts be a negative outcome, but in general it is reasonable to assume that re-entry should be avoided, when possible.
The fourth type of outcome measure examined in Chapter 7 was cost. Costs of health-care utilisation were captured across the full health-care pathway for people with SMI and include costs of primary care, drug prescriptions, A&E department presentations, inpatient admissions for both physical and mental health problems, and specialist community mental health care.
Figure 2 summarises which measures of primary care quality and outcomes were analysed in each chapter. The measures of care quality included were dictated by the research question and the outcomes analysed in each specific chapter. A rationale for choice of outcomes is provided in each chapter.
FIGURE 2.
Overview of outcomes and quality indicators examined, and methods used in the report. Methods used a, discrete-time survival analysis (see Chapters 4 and 6); b, continuous-time survival analysis (see Chapter 5); and c, a two-part model (see Chapter 7).
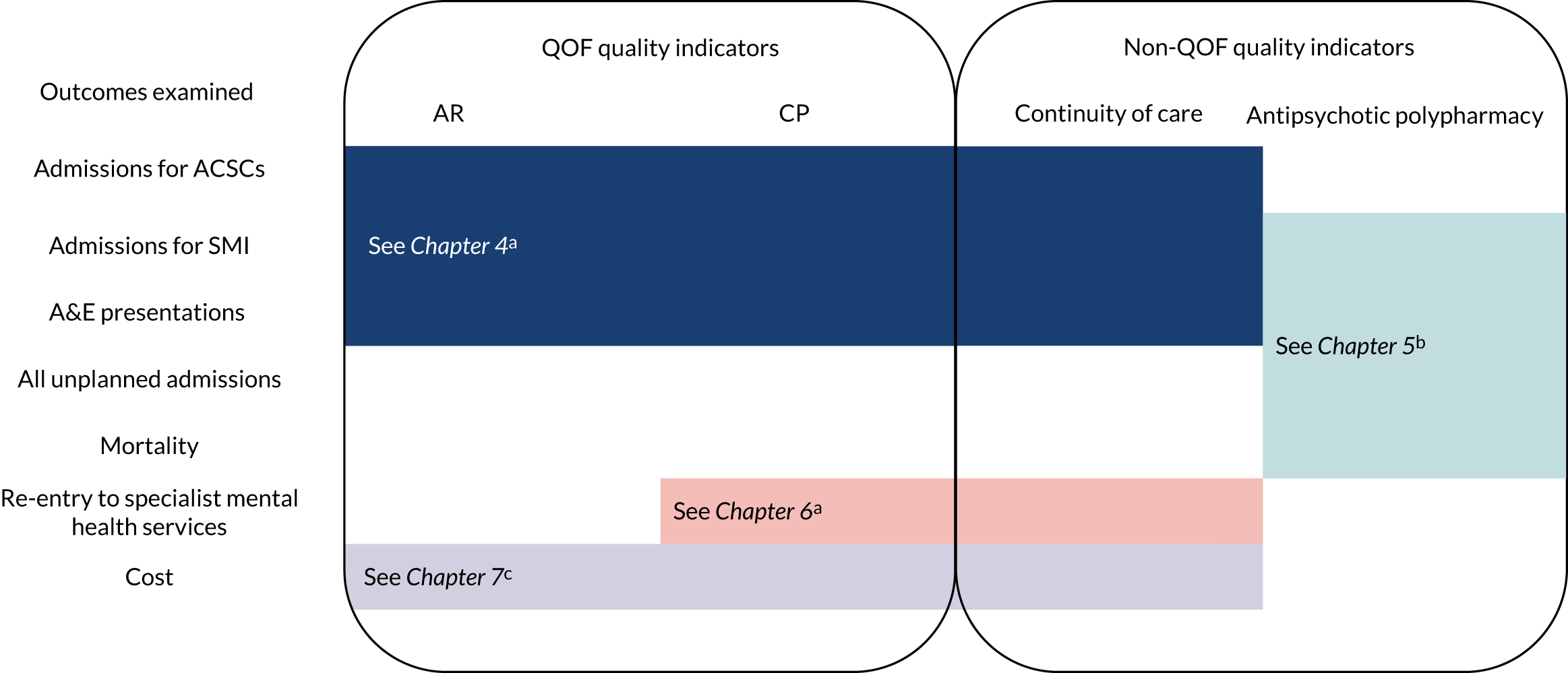
Data sources
This section describes the data sources used to compile the samples analysed in Chapters 4–7.
Clinical Practice Research Datalink
The Clinical Practice Research Datalink (CPRD) is a UK-based research service, jointly sponsored by the Medicines and Healthcare products Regulatory Agency and the National Institute for Health Research, as part of the Department of Health and Social Care. The CPRD collects fully coded and deidentified patient electronic health records from a network of general practices using the Vision® (In Practice Systems Ltd, London, UK) software system. Data for this study were extracted from the CPRD GOLD database, which is drawn from practices using the Vision software system. We used the following CPRD GOLD data files:
-
patient file – basic demographics and registration details
-
practice file – collection and region information
-
staff file – staff role and sex
-
consultation – type of consultation
-
clinical – medical history events, including symptoms, signs and diagnoses
-
additional clinical details – information entered in the structured data areas in the Vision software
-
referral – referrals to external care centres (e.g. hospitals), including specialty and referral type
-
test – qualitative and quantitative test results
-
therapy – prescriptions for drugs and devices issued by the GP.
Clinical information is captured as Read codes, which are recorded by practice staff (doctors, nurses, administrative staff, etc.) as part of routine data entry. Read codes94 are a hierarchical clinical data coding system used in primary care in the UK that classifies diseases, patient characteristics, procedures and tests. 95
A study conducted in collaboration between the CPRD and the London School of Hygiene & Tropical Medicine96 concluded that patients in the CPRD GOLD database are broadly representative of the English general population, in terms of age, sex, ethnicity and BMI. This was in line with previous research by the CPRD,98 which also demonstrated slightly lower mortality rates in younger age groups than in national rates, and a larger median practice size than the national average. 96 Owing to the geographical distribution of Vision software use, areas in the East Midlands, Yorkshire and the Humber and the north-east of England are under-represented relative to areas in Wales, Scotland and Northern Ireland.
We had access to CPRD data for the period of 1 April 2000 to 31 March 2014.
Hospital Episodes Statistics
Hospital Episodes Statistics (HES) comprises detailed records for all patients who received publicly funded care in public and private acute hospitals in England. The study data set includes HES data for those patients who had admissions and/or A&E department presentations during the study period. The HES data used in this study do not include hospital outpatient attendances. Admissions recorded in HES include those for physical health problems and for mental health problems, when the patient was admitted to an acute hospital rather than a specialist mental health inpatient facility.
Hospital admissions were classified using International Statistical Classification of Diseases and Related Health Problems, Tenth Revision (ICD-10), codes to identify SMI and ACSC admissions. (The codes used to classify admissions are listed in Appendix 2.)
Each linked HES record provides detailed information about the patient’s demographic characteristics, medical condition and care pathway. Inpatient data are reported at the level of finished consultant episodes and a new finished consultant episode is created every time a patient is discharged from the care of one consultant to another consultant. To capture the entire care pathway and derive correct admission numbers, we converted finished consultant episodes to continuous inpatient spells, which cover the entire period from admission to final discharge. Continuous inpatient spells also allow for transfers between providers of inpatient care.
We had access to HES inpatient data for the period of 1 April 2000 to 31 March 2014 and to A&E department data for the period of 1 April 2007 to 31 March 2014.
Mental Health Minimum Data Set
The Mental Health Minimum Data Set (MHMDS) (now called the Mental Health Services Data Set) is a nationally mandated routine data collection covering adults who receive care in specialist NHS mental health services, both inpatient- and community-based (outpatient) services. It comprises individual-level, administrative patient data, including data on visits, hospital admissions and other measures of service utilisation, such as contacts with health-care professionals. The study data set includes MHMDS data for all patients who received care in specialist NHS mental health services during the study period.
Mental Health Minimum Data Set data were used for the period of 1 April 2011 to 31 March 2014. MHMDS data prior to this date did not include all specific dates of activity (such as outpatient visits) required for the study.
Linked data sets
The anonymised CPRD GOLD primary care patient data can be individually linked to secondary care and other health and area-based data sets to provide a fuller picture of the patient care record. Linkages are available for English practices that have consented to participate in the linkage scheme, covering patients with a valid NHS number recorded in their GP record, who have not opted out. The CPRD is expanding its health-care data and research services to increase the number of data sets that are linked and made available on a routine basis to the research community. This study is one of the first to use the linked HES A&E data and the MHMDS. Approximately 75% of English practices participating in the CPRD permit linkage of their patients’ data in this way. 96 We test below whether there were systematic differences between practices with and without linkage (see Testing for linkage bias).
In addition to HES and MHMDS linkages, for this study, the CPRD data were also linked to the Index of Multiple Deprivation (IMD) data from 2010, for patients’ area of residence at the lower-layer super output area (LSOA) level. This provided the ranking of the patients’ area of residence according to twentiles of IMD data at the national level. Information was also linked at the practice level. Based on 2011 Census data,97 the practice postcodes held by the CPRD allowed practices to be classified in rurality. They were also classified based on postcodes in terms of estimated distances to the nearest hospital and nearest mental health inpatient facility. Exact IMD scores or distance information were not provided to the study team to avoid disclosing location information. Finally, mortality data from the Office for National Statistics (ONS) were also linked to the CPRD GOLD database.
To preserve anonymity, the data linkages were carried out by NHS Digital as the trusted third party of the CPRD. To avoid the risk of reidentification, the CPRD permitted only four data sets to be linked simultaneously. We therefore obtained the following two sets of linkages: CPRD–A&E–MHMDS–HES and CPRD–HES–ONS–MHMDS.
Information was provided by CPRD for all patients who were eligible for linkage and had an incident or prevalence diagnosis of SMI in their GP record on or before 31 March 2014.
We had two data use agreements with the CPRD approved by the International Scientific Advisory Committee, one for the CPRD GOLD database linked with HES A&E data, the MHMDS, patient IMD, distance to provider and rurality indicators (protocol number 15_213), and one for the CPRD GOLD database linked with HES inpatient data, ONS data, distance to provider and rurality indicators (protocol number 14_168).
Study samples
There are effectively five different samples used in the studies presented in this report, each derived from the same set of patients present in the CPRD data. Differences arise because studies differ in the duration over which patients were followed and due to different inclusion and exclusion criteria applied.
The full set of patients from which the samples are derived was formed of all patients with a diagnosis of SMI documented in primary care clinical notes or referral records on or before 31 March 2014, who attended participating CPRD practices and whose records were eligible for data linkages (n = 34,812). The codes used by CPRD to identify diagnoses of SMI were provided by the study team and are listed in Appendix 2. The data set was limited to patients whose records met an acceptable standard based on recording of registration, clinical events and demographic details, at practices deemed up to standard according to a CPRD algorithm. 96 There were 600 patients for whom IMD data could not be linked, most likely due to their postcode being relatively new and not appearing in the LSOA lookup, so the full combined data set comprised 34,212 patients.
The start date of observation was defined as the earliest date (after 1 April 2000) on which all of the following conditions were met:
-
Patient has a diagnosis of SMI already documented in primary care records (using Read codes).
-
Patient was aged ≥ 18 years.
-
Patient had been registered with a participating practice for at least 365 days. This was imposed to allow for the observation of baseline characteristics and patterns of health-care utilisation.
The end date of the observation period for each patient was defined as the earliest of:
-
the end of the patient’s registration at the practice
-
31 March 2014
-
the patient’s date of death (as documented in primary care records).
The following additional conditions must be met, depending on the specific analysis.
In Chapter 4:
-
The sample was limited to patients with a start date after 31 March 2007, as the CP and AR indicators (two of the key explanatory variables) were introduced after financial year 2006/7 and data on A&E department presentations (one of the outcomes in Chapter 4) were only available from 1 April 2007.
-
The start date of observation for each patient was moved later, if necessary, so that no patients had an A&E department presentation or a hospital admission for at least 1 year prior to the start date, as hospital care could influence primary care activity.
In Chapter 5:
-
The sample was limited to those patients for whom at least one prescription of an antipsychotic was documented in CPRD records during the observation period.
-
The start date was moved forward for patients who were hospitalised within the last 90 days, as patients recently discharged from hospital were at higher risk of readmission. Some of these patients had a new start date later than the last observation date and were therefore dropped from the sample.
-
As A&E data were available beginning in financial year 2007/8 only, the analysis of A&E department visits was limited to patients with an entry date after 31 March 2007. This restriction was not imposed for the analysis of all other outcomes.
In Chapter 6:
-
The sample was limited to patients with a start date after 31 March 2011 because of the use of the MHMDS data.
-
Owing to examining re-entry to specialist mental health services, represented by activity in mental health services (such as an outpatient visit, or start of a hospital admission), we had to ensure a period of at least 12 months with no such activity (i.e. a ‘stable’ SMI patient).
In Chapter 7:
-
Owing to the use of MHMDS data, the sample was limited to patients with a start date after 31 March 2011.
-
Only patients who were observed for a full financial quarter were included.
This resulted in five samples of different sizes:
-
Chapter 4 analysis sample – 19,324 patients
-
Chapter 5 analysis sample (excluding A&E analysis sample) – 17,255 patients
-
Chapter 5 A&E analysis sample – 13,247 patients
-
Chapter 6 analysis sample – 9907 patients
-
Chapter 7 analysis sample – 16,485 patients.
Figure 3 provides an overview of how each sample was formulated.
FIGURE 3.
Sample construction for each study in the report.
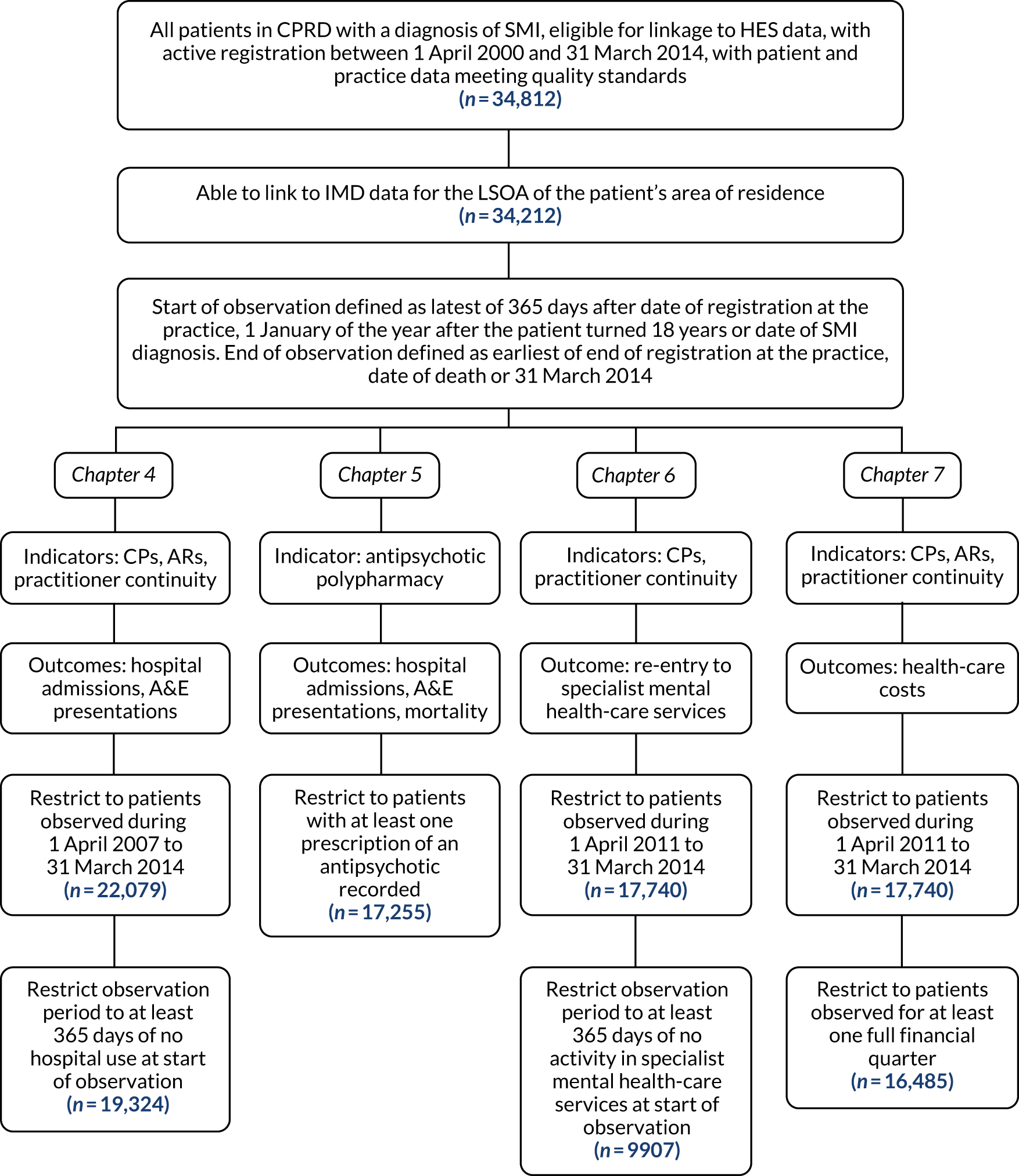
For all four analytical chapters (see Chapters 4–7), we imposed a 12-month registration period at the practice prior to the start of the observation period, to allow us to capture medical history and utilisation patterns, which further restricted the analysis period (see Covariates).
Testing for linkage bias
We compared the set of patients eligible for linkage to HES and MHMDS with a random sample of 3000 patients with SMI attending practices that participated in CPRD but had not permitted the linkages, to ensure that our practice populations were not biased in observable ways. Figure 4 shows that the linked and non-linked samples were similar in age and sex distribution.
FIGURE 4.
Clinical Practice Research Datalink samples of patients based on eligibility for linkage to other data sets. (a) Not linked; and (b) linked.
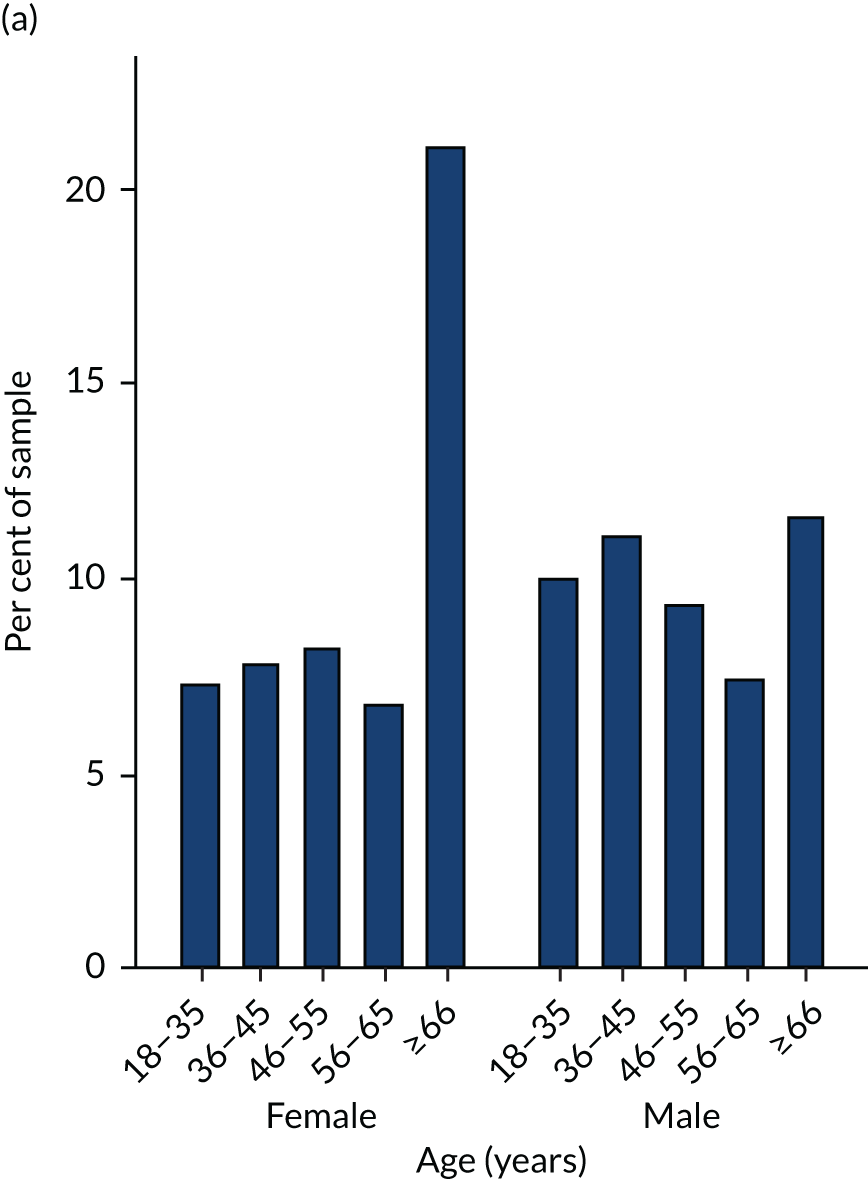
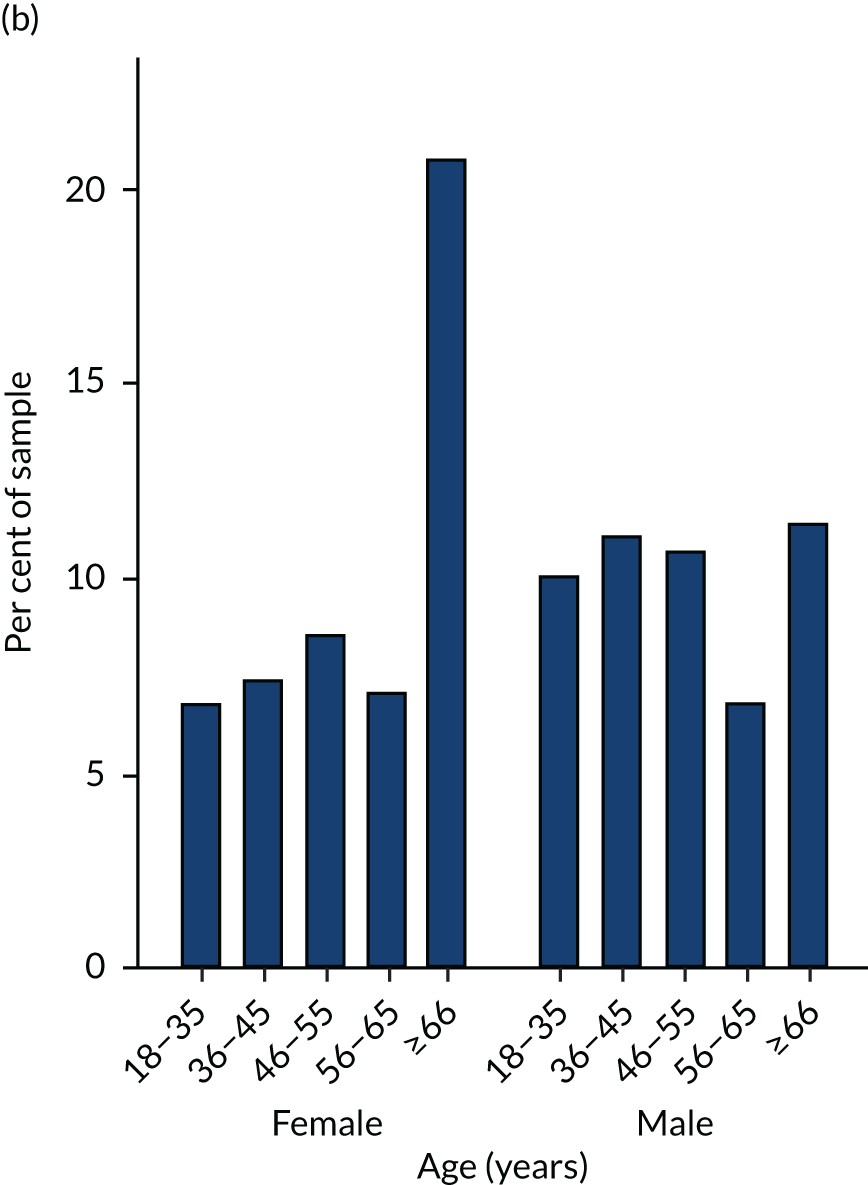
Covariates
We measured patient and practice characteristics at or before the start of the observation period by using data from the 12-month period of registration at the practice prior to the start of the study observation period.
We used the same set of control variables in all analyses. This includes age, sex, age–sex interactions, ethnicity, the number of comorbid conditions (as defined by Charlson et al. 99), alcohol and smoking status, a diagnosis of depression, the number of GP contacts (face-to-face visits and telephone calls) in the last year and small area deprivation profile based on patients’ residence. 100 We sought to make the data as complete as possible by utilising information from different sources (e.g. cross-checking ethnicity status from HES with that in CPRD), although any remaining missingness could be not at random.
We approximated ability to access secondary care by the distance from the patients’ general practice to the nearest psychiatric inpatient hospital and general hospital and by whether or not the practice is in a rural area. Finally, we controlled for the year in which the patient entered the sample and the time since first diagnosis.
We used Read codes recorded over the entire patient’s history and our own clinical expertise to define three diagnostic categories: (1) schizophrenia and other psychoses, (2) bipolar disorder and affective psychoses and (3) those who had a diagnosis from each group (codes are provided in Appendix 2).
Descriptions of covariates and their sources are described in detail in Table 4.
Variable | Type | Details |
---|---|---|
Age | Categorical (19–35,36–45, 46–55, 56–65, > 65 years) | Based on year of event and year of birth as recorded in primary care |
Male | Binary | Based on sex as recorded in primary care |
Ethnicity | Binary (white/other) | Based on HES records and CPRD Read codes |
IMD (deprivation level associated with patient’s residence area) | Categorical (quintiles) | For practices that have consented to participate in the linkage scheme, the patient postcode of residence is mapped to LSOA using a postcode look-up file. The LSOA of residence then allows linkage to the 2010 English IMD |
Number of Charlson Comorbidity Index comorbidities | Categorical (none, one, two, three or more) | CPRD Read codes recorded any time before the date of entry to the study sample |
Depression diagnosis | Binary | CPRD Read codes |
Alcohol status/smoking status | Binary | Diagnostic and management codes, and additional information entered in the structured data areas in the Vision software |
Number of GP contacts in the preceding 12 months (face-to-face visits and telephone calls) | Categorical (0–4, 5–9, 10–14, 15–19, ≥ 20) | From CPRD consultation file |
Distance from GP to nearest acute hospital provider/distance from GP to nearest mental health provider | Categorical (0–3, 3–6, 6–9, > 9 km) | We provided CPRD with a look-up table of all postcodes in England, the estimated straight line distance from the postcode to the nearest mental health and acute hospital provider. CPRD carried out the linkage using contributing practices’ postcode information. Once linked, distances (km) were categorised into distance bands by CPRD (e.g. 0–3, 3–6 km, etc.) |
General practice in rural area | Binary | To construct a practice-level rurality flag we provided CPRD with a look-up table of all LSOA levels (small areas) in England and rurality information derived from the RUC2011. This information was used to link contributing practices to their corresponding rurality flag using the practice LSOA |
Calendar year | Binary | |
Schizophrenia diagnosis/bipolar diagnosis/both schizophrenia and bipolar diagnosis | Binary | CPRD Read codes (provided in Appendix 2) |
Time since first diagnosis | Categorical (1, 1–5, > 5 years) |
Chapter 4 The association between primary care quality and hospital care utilisation
This chapter is partly based on Ride et al. 40 This is an Open Access article distributed in accordance with the terms of the Creative Commons Attribution (CC BY 4.0) license, which permits others to distribute, remix, adapt and build upon this work, for commercial use, provided the original work is properly cited. See: http://creativecommons.org/licenses/by/4.0/. The text includes minor additions and formatting changes to the original text.
This chapter is also partly based on Ride et al. 41 This is an Open Access article distributed in accordance with the terms of the Creative Commons Attribution (CC BY 4.0) license, which permits others to distribute, remix, adapt and build upon this work, for commercial use, provided the original work is properly cited. See: http://creativecommons.org/licenses/by/4.0/. The text includes minor additions and formatting changes to the original text.
Introduction
This chapter examines if three primary care activities that are framed as indicators of primary care quality (AR of patients’ physical health, creation or maintenance of a CP and continuity of GP care) influenced unplanned utilisation of hospital services for people with SMI. Our previous study1 using aggregate practice-level data for the period of April 2006 to March 2010 found a positive association between achievement rates for the QOF AR indicator and rates of SMI admission, but no association with achievement rates for CPs43 (see Appendix 3 for an extension of this study to cover the additional years up to 2014). However, the previous study used annual practice-level data and so could not ascertain whether the care preceded or followed admission. Understanding the sequence and timing of events is crucial; a prerequisite for establishing causal inference. In contrast, the current study analyses event-level data with precise timing information for a large patient cohort. By avoiding problems inherent in aggregation across individuals, these data allowed us to investigate more precisely the relationship between quality of care and outcomes using survival analysis modelling techniques.
The three potential indicators of care quality analysed in this chapter were chosen because they were either incentivised under the QOF (AR, CP) or identified as part of the literature review reported in Chapter 2 (practitioner continuity of care). For each indicator, we expected that achievement resulted in better information flow between patients and their GPs, and a higher likelihood that deteriorations in patients’ mental or physical health were identified and addressed early, thus avoiding the need for hospital care. The association between a fourth potential indicator of care quality, polypharmacy, and hospital utilisation is considered separately in Chapter 5. This is due to the use of different inclusion criteria for the study sample and a different analytical approach.
Methods
Sample
Patients were included in the sample if the conditions listed in Chapter 3, Study samples were met. Continuity is measured over defined periods of time, so the observation period for each patient was divided into periods of 3 months, dating from their first date of observation. Patients were followed until outcome or censoring [with censoring due to the patient changing general practice, death or the end of the study period (31 March 2014)].
Outcome measures
We separately examined time to three types of unplanned hospital use: (1) A&E department presentations, (2) unplanned admissions for SMI and (3) unplanned admissions for ACSC (as outlined in Chapter 3, Outcomes). For each type of outcome, we considered only the first observed instance (presentation or admission), as this could have influenced subsequent care quality in unobserved ways. For example, CPs may be updated in secondary care at the end of an admission period and this may not be reflected appropriately in the GP electronic records.
The occurrence of the outcome was measured in the 3-month period, t, and continuity of care was measured over the 12 months prior to the outcome period, t-1 to t-4. The outcome variable was a binary variable for each 3-month period, indicating whether or not the event occurred in that period.
Quality and Outcomes Framework indicators of quality
The analysis included time-varying care quality indicator variables for CPs and ARs. These binary variables indicated if a CP or AR had been recorded within the 12 months prior to the current 3-month period. The choice of the 12-month window to determine expiration status was based on the QOF guidance that a CP or AR should be undertaken and reviewed annually.
From 2006/7 to 2010/11 the QOF annual review indicator entailed the patient being given appropriate health promotion and prevention advice. As outlined in Chapter 3, The annual review indicator and Table 3, in 2011/12 this broad indicator was split into more specific indicators (a record of alcohol consumption, checks of blood pressure, BMI, blood glucose or glycated haemoglobin, ratio of total to high-density lipoprotein cholesterol and, when appropriate, cervical screening). To explore ARs for the full period of our analysis, we formulated an aggregate indicator. This signified that the patient had at least three of the four ‘health risk’ checks (blood pressure, BMI, cholesterol and glucose) documented within a 3-month period. 101 The date of the aggregate indicator was the date of the final check. The AR variable in the analysis was equal to 1 for the current 3-month period if there was an AR (using the original Read coding) or an aggregate indicator date within the 12 prior months. Appendix 3 provides an overview of how the rates of these indicators changed over the period of analysis.
Non-Quality and Outcomes Framework measures of quality: practitioner continuity
We used three indices measuring different dimensions of GP continuity: the COC, UPC and SECON. 102
The COC captures dispersion of visits across GPs as follows:
in which N is total number of visits and nj is number of visits with GP j.
The UPC is the proportion of a patient’s visits that are with the GP most frequently seen by the patient in that year:
The SECON is the proportion of consecutive pairs of visits which are to the same physician:
in which i is the visit number, N is the number of visits, N – 1 is the number of sequential pairs of visits. si = 1 if visit i and visit i + 1 are to the same provider, and si = 0 if otherwise.
Each index ranges from 0 (lowest continuity) to 1 (perfect continuity). Illustrative examples are shown in Table 5.
Scenario | Visit pattern | Number of visits | Number of practitioners | COC | UPC | SECON |
---|---|---|---|---|---|---|
A | All visits with same GP | 8 | 1 | 1 | 1 | 1 |
B | Each visit with a different GP | 8 | 8 | 0 | 0.13 | 0 |
C | Four visits with one GP, then four visits with another | 8 | 2 | 0.43 | 0.50 | 0.86 |
D | Five visits with one GP, then three visits with another | 8 | 2 | 0.46 | 0.63 | 0.86 |
E | Alternating between two GPs | 8 | 2 | 0.43 | 0.50 | 0 |
F | As for scenario E but five visits with the first GP and three visits with the second | 8 | 2 | 0.46 | 0.63 | 0.29 |
We measured continuity over 12 months (four 3-month periods), considering only face-to-face visits with GPs. There is no standard level for ‘high’ and ‘low’ continuity, so we classified practitioner continuity as ‘high’ if the level of continuity was above the median for the index and ‘low’ if at or below the median level.
A minimum of two visits is required to calculate COC and SECON, but to improve index stability we set the minimum to three visits and set continuity as ‘undefined’ below this level. We constructed a set of categorical variables based on visit frequency and on whether continuity was low or high. This allowed for different effects of continuity for frequent and less frequent users of family practice, as suggested by previous research. 103 Visit frequency was classified into low (zero to two visits), moderate (three to five visits) and high (six or more visits). These categories correspond to tertiles of the full visit distribution. Continuity indices were defined as low or high based on the median value of each index: COC low (0–0.35) and COC high (> 0.35); UPC low (0–0.67) and UPC high (> 0.67); SECON low (0–0.17) and SECON high (> 0.17).
Periods were then classified into five categories according to continuity level and visit frequency in the prior 12 months: low visit frequency (with continuity undefined – the base category); moderate visit frequency with low continuity; moderate visit frequency with high continuity; high visit frequency with low continuity; and high visit frequency with high continuity.
Statistical analysis
The necessity of creating periods for continuity measurement led us to employ discrete-time survival analysis to model all three care quality indicators (continuity, CP and AR) simultaneously. Although the outcomes of interest were (effectively) continuous measures (as we had day-level data on when the events occur), these were converted into discrete outcomes for each period, to match the measurement of continuity. The model evaluated the association between risk of the outcome in a particular 3-month period and each of the three care quality indicators in the prior 12 months.
The hazard rate at period t is the probability of observing the outcome for individual i in period t, conditional on the individual ‘surviving’ in the sample to period t (i.e. no censoring and the outcome was not observed in prior periods for that individual). A complementary log–log (cloglog) model, which is the discrete-time analogue of the continuous-time proportional hazards model, was estimated for each outcome. This model is useful when the occurrence of the outcome is rare.
A flexible piecewise constant baseline hazard function was applied by specifying dummy variables for each 3-month period. This assumes that the hazard function is constant within each period, but can vary across periods and avoids imposing a more restrictive functional form on the model. The resulting exponentiated coefficients can be interpreted as HRs, the discrete-time counterpart of the hazard from a continuous-time proportional hazards model. 81 The HR is equal to the proportional change in the hazard of the outcome for a unit change in the variable.
The hazard rate (h) is specified as:
in which Ti is the discrete survival time variable for individual i; t represents the time period of interest, Xit is the vector of time-varying factors, including a constant term; Zi is a vector of time-invariant factors; D is a series of time period dummy variables representative of the baseline hazard; and vi∼N(0,σv2) is normally distributed individual unobserved heterogeneity.
Our main modelling approach accounted for individual unobserved heterogeneity, vi. Owing to the incidental parameter problem104 that arises through specifying individual fixed effects to represent such heterogeneity in non-linear models, we instead assume unobserved heterogeneity is normally distributed and specify this as a function of the means of time-varying variables. This is often termed a correlated random-effects model. Following Mundlak,105 we assume:
in which X¯i is the mean of the time-varying variables, D¯ the mean of the time dummies, a is a constant and ci represents the remaining unobserved heterogeneity assumed to be normally distributed. The mean variables (X¯i,D¯) capture confounding by unobserved time-invariant patient factors (e.g. long-standing illness, health-seeking behaviour) that drive both primary care quality and use of hospital services. The coefficients of the period-specific levels (Xit, Dt) can be interpreted as the effect of the quality indicator specific to that 3-month period.
To allow comparison of our results to previous studies examining the effect of continuity of care, we also estimated models that did not specify individual heterogeneity (vi) as a function of the means of the time-varying variables (i.e. by estimating Equation 4 directly). These models allow for normally distributed individual heterogeneity, but it is assumed to be uncorrelated with the explanatory variables contained in the model.
All models included observed patient characteristics as explanatory variables and adjusted standard errors (SEs) for clustering at the practice level. We estimated separate models for each of the three continuity indices.
Robustness checks
We tested the sensitivity of the results to the level of visit frequency at which continuity was classified as ‘undefined’. The minimum level for measuring continuity (and corresponding categories for low vs. moderate visit frequency) were set to two or four visits rather than three visits as in our main analysis.
Results
Sample
The sample consisted of 19,324 patients attending 215 practices, observed for 15.8 3-month periods on average (range 1–28 periods). Table 6 presents the characteristics of patients in the sample. Half of the sample (50.3%) had an A&E department presentation at some point during the observation period, 13.1% had an admission for SMI and 12.8% had an ACSC admission. Using a three-visit minimum to define continuity, median (mean) values for each continuity index were 0.35 (mean 0.46) for COC, 0.67 (mean 0.65) for UPC and 0.17 (mean 0.26) for SECON. A CP had been documented in the previous 12 months for 40% of the patient-periods observed and an AR (or the alternate aggregate indicator) in 69% of periods. The Spearman’s rank-order correlation between COC and UPC was 0.94 (p < 0.001), between COC and SECON it was 0.55 (p < 0.001) and between UPC and SECON it was 0.47 (p < 0.001). Mean COC in periods with a CP in the previous 12 months was 0.47, compared with 0.45 in periods without a CP in the previous 12 months (two-sample t-test of difference in means: p < 0.001). The equivalent for UPC was 0.67, compared with 0.66 (p < 0.001), and for SECON it was 0.24 compared with 0.23 (p < 0.001).
Characteristic | n (%) | Mean (SD) |
---|---|---|
Fixed at baseline | ||
Age (years) | ||
19–35 | 5328 (27.6) | |
36–45 | 4407 (22.8) | |
46–55 | 3571 (18.5) | |
56–65 | 2678 (13.9) | |
≥ 66 | 3340 (17.3) | |
Sex | ||
Female | 9705 (50.2) | |
Male | 9619 (49.8) | |
IMD | ||
Quintile 1 (least disadvantaged) | 3113 (16.1) | |
Quintile 2 | 3546 (18.4) | |
Quintile 3 | 3605 (18.7) | |
Quintile 4 | 4484 (23.2) | |
Quintile 5 (most disadvantaged) | 4576 (23.7) | |
Ethnicity | ||
Black and minority ethnicities | 5609 (29.0) | |
White | 13,715 (71.0) | |
Diagnosis category grouping | ||
Bipolar disorder and affective psychoses | 6846 (35.4) | |
Schizophrenia and other psychoses | 10,254 (53.1) | |
Both categories | 2224 (11.5) | |
Years since diagnosis | ||
0–1 | 5779 (29.9) | |
2–5 | 3953 (20.5) | |
> 5 | 9592 (49.6) | |
Number of Charlson Comorbidity Index comorbidities | ||
None | 13,246 (68.5) | |
One | 4726 (24.5) | |
Two or more | 1352 (7.0) | |
History of depression | ||
No history of depression | 8382 (43.4) | |
Comorbid depression | 10,942 (56.6) | |
History of smoking | ||
Non-smoker | 5436 (28.1) | |
Current or ex-smoker | 13,888 (71.9) | |
During the observation period | ||
Number of 3-month periods observed | 15.8 (10.0) | |
COC | 0.46 (0.32) | |
UPC | 0.65 (0.24) | |
SECON | 0.26 (0.30) | |
CP in prior 12 months | 0.40 (0.49) | |
Annual review in prior 12 months | 0.69 (0.46) | |
At least one A&E department presentation | 9719 (50.3) | |
At least one SMI admission | 2525 (13.1) | |
At least one ACSC admission | 2475 (12.8) |
Association between quality of care and outcomes
Table 7 presents the association between the quality measures and each outcome from the discrete-time survival analyses, with practitioner continuity measured by the COC. The results in the second column are HRs for the key variables of interest from our preferred specification, the correlated random-effects model, which accounts for unobserved confounding. Results from a model that does not account for unobserved confounding, the standard random-effects model, are presented in the third column for comparison. Full results from the correlated random-effects models are presented in Table 8.
Indicator | Correlated random-effects model, HR (95% CI)a | Random-effects model, HR (95% CI)b |
---|---|---|
A&E department presentation | ||
QOF quality indicators | ||
CP vs. none | 0.74*** (0.69 to 0.80) | 0.95* (0.90 to 0.99) |
AR vs. none | 0.80*** (0.76 to 0.85) | 0.95 (0.91 to 1.00) |
Practitioner continuity | ||
Moderate visit frequency (three to five visits): high COC vs. low COC | 0.89** (0.83 to 0.97) | 0.84*** (0.77 to 0.91) |
High visit frequency (six or more visits): high COC vs. low COC | 0.92 (0.84 to 1.00) | 0.86*** (0.80 to 0.92) |
SMI admission | ||
QOF quality indicators | ||
CP vs. none | 0.67*** (0.59 to 0.75) | 1.36*** (1.23 to 1.50) |
AR vs. none | 0.75*** (0.67 to 0.84) | 0.97 (0.86 to 1.09) |
Practitioner continuity | ||
Moderate visit frequency (three to five visits): high COC vs. low COC | 0.99 (0.82 to 1.18) | 0.98 (0.83 to 1.17) |
High visit frequency (six or more visits): high COC vs. low COC | 0.91 (0.76 to 1.09) | 0.94 (0.82 to 1.08) |
ACSC admission | ||
QOF quality indicators | ||
CP vs. none | 0.73*** (0.64 to 0.83) | 0.98 (0.89 to 1.07) |
AR vs. none | 0.76*** (0.67 to 0.87) | 1.03 (0.92 to 1.16) |
Practitioner continuity | ||
Moderate visit frequency (three to five visits): high COC vs. low COC | 0.77** (0.65 to 0.92) | 0.74*** (0.62 to 0.88) |
High visit frequency (six or more visits): high COC vs. low COC | 0.74*** (0.62 to 0.87) | 0.71*** (0.61 to 0.82) |
A&E department presentation | SMI admission | ACSC admission | ||||
---|---|---|---|---|---|---|
HRa | SEb | HRa | SEb | HRa | SEb | |
Time-varying variable: period level | ||||||
CP | 0.744*** | 0.026 | 0.667*** | 0.038 | 0.726*** | 0.048 |
AR | 0.805*** | 0.023 | 0.748*** | 0.044 | 0.763*** | 0.050 |
Continuity/visit frequency (base: low visit frequency, continuity undefined) | ||||||
Moderate frequency, low continuity | 1.091* | 0.042 | 1.217** | 0.082 | 1.526*** | 0.130 |
Moderate frequency, high continuity | 0.976 | 0.041 | 1.200* | 0.110 | 1.181 | 0.101 |
High frequency, low continuity | 1.136* | 0.058 | 1.628*** | 0.141 | 2.080*** | 0.213 |
High frequency, high continuity | 1.046 | 0.054 | 1.476*** | 0.149 | 1.531*** | 0.157 |
Time-varying variables: mean level | ||||||
CP | 1.781*** | 0.134 | 6.718*** | 1.010 | 2.186*** | 0.336 |
AR | 1.466*** | 0.092 | 1.812*** | 0.315 | 2.627*** | 0.475 |
Continuity/visit frequency (low visit frequency, continuity undefined) | ||||||
Moderate frequency, low continuity | 1.448*** | 0.125 | 0.580** | 0.112 | 1.031 | 0.227 |
Moderate frequency, high continuity | 1.073 | 0.102 | 0.486** | 0.118 | 0.747 | 0.170 |
High frequency, low continuity | 2.029*** | 0.169 | 0.598** | 0.096 | 1.231 | 0.200 |
High frequency, high continuity | 1.797*** | 0.148 | 0.675* | 0.113 | 1.140 | 0.200 |
Time-invariant variables (at start of observation) | ||||||
Index of disadvantage (base: quintile 1, least disadvantaged) | ||||||
Quintile 2 | 1.105* | 0.047 | 0.987 | 0.081 | 0.933 | 0.064 |
Quintile 3 | 1.177*** | 0.051 | 1.030 | 0.083 | 1.081 | 0.073 |
Quintile 4 | 1.244*** | 0.059 | 1.009 | 0.084 | 1.192* | 0.083 |
Quintile 5 (most disadvantaged) | 1.409*** | 0.074 | 1.112 | 0.102 | 1.261** | 0.101 |
Ethnicity (base: black and minority ethnicities) | ||||||
White | 1.478*** | 0.043 | 1.802*** | 0.139 | 2.090*** | 0.156 |
History of smoking (base: non-smoker) | ||||||
Current or ex-smoker | 1.067* | 0.030 | 0.956 | 0.0483 | 1.019 | 0.057 |
Age (years) (base: 18–35 years) | ||||||
36–45 | 0.840*** | 0.026 | 1.000 | 0.0623 | 1.075 | 0.085 |
46–55 | 0.770*** | 0.026 | 0.750*** | 0.0493 | 1.383*** | 0.117 |
56–65 | 0.819*** | 0.030 | 0.651*** | 0.0449 | 1.957*** | 0.169 |
≥ 66 | 1.165** | 0.041 | 0.481*** | 0.0449 | 3.592*** | 0.324 |
Years since SMI diagnosis (base: ≤ 1 year) | ||||||
2–5 | 1.039 | 0.034 | 1.071 | 0.0740 | 1.158 | 0.091 |
> 5 | 0.943* | 0.026 | 1.067 | 0.0665 | 1.159* | 0.078 |
Sex (base: female) | ||||||
Male | 1.045 | 0.024 | 1.000 | 0.0451 | 1.076 | 0.055 |
SMI diagnosis category (base: bipolar disorder, affective psychosis) | ||||||
Schizophrenia or other psychosis | 0.954 | 0.026 | 1.267*** | 0.0672 | 1.058 | 0.058 |
Both categories | 0.961 | 0.033 | 2.046*** | 0.133 | 0.971 | 0.074 |
Comorbidity | ||||||
Number of Charlson Comorbidity Index comorbidities | 1.104*** | 0.018 | 0.913* | 0.0383 | 1.351*** | 0.042 |
Comorbid depression | 1.019 | 0.024 | 0.791*** | 0.0383 | 1.047 | 0.054 |
Observations | 203,534 | 281,017 | 286,940 | |||
Number of individuals | 19,324 | 19,324 | 19,324 |
Having a CP or AR documented in the previous 12 months was associated with lower hazard of all three outcomes. The relative magnitude of the hazard reduction associated with CPs was 26% for A&E department presentation [HR 0.74, 95% confidence interval (CI) 0.69 to 0.80], 33% for SMI admission (HR 0.67, 95% CI 0.59 to 0.75) and 27% for ACSC admission (HR 0.73, 95% CI 0.64 to 0.83). The reduction associated with ARs was 20% for A&E department presentation (HR 0.80, 95% CI 0.76 to 0.85), 25% for SMI admission (HR 0.75, 95% CI 0.67 to 0.84) and 24% for ACSC admission (HR 0.76, 95% CI 0.67 to 0.87).
For those with moderate visit frequency (three to five visits in the previous 12 months), higher GP continuity was associated with 11% lower hazard of A&E department presentation (HR 0.89, 95% CI 0.83 to 0.97) and 23% lower hazard of ACSC admission (HR 0.77, 95% CI 0.65 to 0.92), but not with hazard of SMI admission. For frequent attenders (six or more visits in the previous 12 months), higher GP continuity was associated with 26% lower hazard of ACSC admission (HR 0.74, 95% CI 0.62 to 0.87), but not with risk of A&E department presentation or SMI admission.
The standard approach (random effects) to modelling continuity, which does not account for unobserved confounding and so is not our preferred specification, produced different results (see final column of Table 7). Compared with the correlated random-effects model, the standard approach found larger risk reductions associated with higher GP continuity, none with annual reviews and CPs was associated with higher (rather than lower) risk of SMI admissions.
Some patient characteristics were also associated with risk of unplanned hospital utilisation. Living in a more deprived area was associated with a higher hazard of all three types of hospital care, as was white ethnicity (vs. black or minority ethnicities). Age had different patterns of association with the three types of hospital care: those in the middle range (aged 36–65 years) had lower risk of A&E department presentation than younger or older patients, whereas younger patients (aged < 45 years) had the highest risk of SMI admission, and the risk of ACSC admission steadily increased from the age of 46 years. Physical comorbidities were associated with higher risk of A&E department presentation and ACSC admissions, but lower risk of SMI admission. Those with a diagnosis grouped with bipolar disorder (and not a diagnosis grouped with schizophrenia) were at lower risk of SMI admission.
Table 9 shows that using a different measure of GP continuity, either UPC (measuring density of visits to a single provider) or SECON (measuring sequential continuity), produced a similar pattern of results to those obtained using the COC (measuring dispersion), although with some differences. There was an association between higher continuity and lower risk of SMI admission for frequent attenders when using the UPC, and for those with moderate visit frequency using the SECON index. In addition, there was an association between higher continuity for frequent attenders and lower risk of A&E department presentations when using the SECON. The use of different continuity indices made no difference to the results for CPs or ARs.
UPC, HR (95% CI) | SECON, HR (95% CI) | |
---|---|---|
A&E department presentation | ||
QOF quality indicator | ||
CP vs. none | 0.74*** (0.69 to 0.80) | 0.74*** (0.69 to 0.80) |
AR vs. none | 0.80*** (0.76 to 0.85) | 0.80*** (0.76 to 0.85) |
Practitioner continuity | ||
Moderate visit frequency (three to five visits): high continuity index vs. low continuity index | 0.90* (0.84 to 0.98) | 0.84*** (0.76 to 0.91) |
High visit frequency (six or more visits): high continuity index vs. low continuity index | 0.97 (0.89 to 1.05) | 0.90** (0.84 to 0.97) |
SMI admission | ||
QOF quality indicator | ||
CP vs. none | 0.67*** (0.60 to 0.75) | 0.67*** (0.60 to 0.75) |
AR vs. none | 0.75*** (0.67 to 0.84) | 0.75*** (0.67 to 0.84) |
Practitioner continuity | ||
Moderate visit frequency (three to five visits): high continuity index vs. low continuity index | 0.91 (0.75 to 1.09) | 0.81* (0.67 to 0.98) |
High visit frequency (six or more visits): high continuity index vs. low continuity index | 0.79* (0.66 to 0.95) | 0.94 (0.78 to 1.15) |
ACSC admission | ||
QOF quality indicator | ||
CP vs. none | 0.73*** (0.64 to 0.83) | 0.72*** (0.64 to 0.82) |
AR vs. none | 0.76*** (0.67 to 0.87) | 0.76*** (0.67 to 0.87) |
Practitioner continuity | ||
Moderate visit frequency (three to five visits): high continuity index vs. low continuity index | 0.83* (0.70 to 0.99) | 0.83* (0.69 to 0.99) |
High visit frequency (six or more visits): high continuity index vs. low continuity index | 0.79** (0.66 to 0.93) | 0.83* (0.70 to 0.99) |
Robustness results
Varying the minimum number of visits deemed necessary to measure continuity, from three visits in the main analysis to two or four visits, did not substantially change the overall findings. These results are presented in Table 10.
High COC vs. low COC | Two visits, HR (95% CI) | Four visits, HR (95% CI) |
---|---|---|
A&E department presentation | ||
Moderate visit frequency | 0.90** (0.84 to 0.96) | 0.86** (0.78 to 0.95) |
High visit frequency | 0.92 (0.84 to 1.00) | 0.92 (0.84 to 1.00) |
SMI admission | ||
Moderate visit frequency | 0.90 (0.77 to 1.05) | 0.89 (0.72 to 1.11) |
High visit frequency | 0.89 (0.74 to 1.07) | 0.90 (0.75 to 1.07) |
ACSC admission | ||
Moderate visit frequency | 0.79** (0.67 to 0.93) | 0.76* (0.61 to 0.95) |
High visit frequency | 0.73*** (0.62 to 0.87) | 0.74*** (0.62 to 0.88) |
Discussion
Summary of findings
The two QOF indicators, CPs and ARs, were both associated with substantial reduction in the risk of unplanned hospitalisation for patients with SMI. Among those who had a CP documented in primary care in the previous 12 months, the hazard of an A&E department presentation, ACSC admission or SMI admission were 26%, 27% and 33% lower, respectively, than for patients without a recent CP. The corresponding reductions associated with ARs were 20%, 24% and 25% lower, respectively. All of these effects were statistically significantly different from 1 when compared against a critical value of alpha = 0.05.
The association of continuity of care and unplanned hospitalisations varied with the underlying frequency of patients’ visits to their GP. For those with moderate visit frequency (three to five visits in the previous 12 months), higher GP continuity was associated with 11% lower hazard of A&E department presentation and 23% lower hazard of ACSC admission, but not with risk of SMI admission. For frequent attenders (six or more visits in the previous 12 months), higher GP continuity was associated with 26% lower hazard of ACSC admission, but not with risk of A&E department presentation or SMI admission.
Strengths and limitations
The primary strength of this analysis was its use of detailed information on the timing of care events, which allowed us to study the association between time-varying measures of care quality and hospital utilisation, and to ascertain the action–outcome ordering necessary for meaningful causal inference. Furthermore, by using statistical modelling techniques that separate within- and between-patient variation, we controlled for time-invariant patient characteristics, when possible, and reduced the scope for unobserved confounding. To our knowledge, this is the first time this modelling approach has been used to analyse the effect of care quality indicators on outcomes for patients with SMI.
There are a number of limitations to our analysis. First, although we attempted to account for time-invariant unobserved patient characteristics, we cannot rule out time-varying confounding bias that remains uncontrolled, which may have contributed to our findings. For instance, during periods of deterioration leading to admission, GPs may have less opportunity, or put less emphasis on, spending time on preventative measures, such as CPs. This would induce a negative correlation between having a current CP and risk of unplanned hospitalisation. Our results should therefore be interpreted as showing association with many sources of confounding avoided, but not strictly causation.
Second, we restricted the sample to patients registered with the same practice for the year preceding diagnosis, to allow us to include measures of historical health-care utilisation and medical history. However, the restriction may have excluded individuals with a more severe form of SMI if this led them to move practice. In addition, the sample may have included some patients who were not newly diagnosed if their diagnostic information had not been transferred to a new practice or to a new electronic recording system.
Third, patients in England can register with only one general practice and may face greater administrative barriers to changing practices than in other countries. This may result in less apparent benefit of continuity of care in our results than would be observed in other health-care systems. When patients are free to choose their provider, high practitioner continuity may reflect a strong, valued therapeutic relationship, which may in turn improve outcomes. In England, patients may have a constrained choice of general practice, so that higher continuity could, in principle, be less beneficial for some individuals.
Fourth, the collection of HES A&E data commenced on an experimental basis in April 2007 and captured 62% of national A&E department attendances in the first financial year. The experimental label was lifted by April 2013 after capture had increased to > 80%. However, there is no reason to consider that the capture of attendances was related to if a patient had a CP or AR, meaning that this should not impact adversely on our comparative analysis.
Finally, the clinical outcomes we examined are important, as they represent some of the excess health risks for people with SMI and carry substantial health-care costs. However, they are not the only outcomes that matter. Both people with SMI and GPs value continuity of care in itself, as part of how they experience giving and receiving care. 106,107 Regular physical reviews may uncover health issues that require treatment, but which would not necessarily have led to an ACSC admission. Broader outcomes important to people with SMI may also be affected by the different measures of care quality, including social functioning and health-related quality of life. 108
Implications for research and practice
The association of the QOF indicators with reduced hazard of unplanned hospital use might suggest that these activities help patients avoid hospital for conditions that can be effectively managed in primary care. As hypothesised, proactive reviews of physical health (ARs) may lead to earlier detection of physical health problems, whereas documentation of patients’ current health status, early warning signs, triggers, social support needs, co-ordination arrangements with secondary care and preferred course of action in the event of a clinical relapse (CPs) could improve the management of the patient’s health overall. Both of these indicators could thereby prevent deterioration, reducing the need for urgent care, with CPs also having the potential to direct patients into appropriate community-based services during periods of deterioration, and thereby avert hospital use.
Similarly, the finding that practitioner continuity is associated with lower hazard of A&E department presentations and ACSC admissions supports the hypothesis that seeing the same GP over time could improve the doctor–patient relationship, facilitate communication and trust, reduce fragmentation of care, and allow for earlier detection of problems and/or more effective and appropriate management of problems as they arise.
The potential for better-quality care by GPs to reduce A&E department attendances and hospital admissions suggests that improving quality may help reduce demand on secondary care services and hence possibly reduce NHS expenditure, a question we explore in Chapter 7.
From a methodological point of view, the results presented in this analysis differed substantially from a previous study addressing similar research questions, but using practice-level aggregate data of hospitalisation rates and QOF achievement rates. 43 Although the practice-level analysis identified a positive association between AR and the risk of unplanned SMI hospitalisation (i.e. high-quality care increases unplanned hospital use), the current analysis found an opposite effect. The current results may be more plausible given the hypothesised mechanism by which high-quality care affects patients’ health and health-care needs. Although the studies are not directly comparable – as they are based on different samples – it is reasonable to assume that the more aggregate-level study suffers from aggregation bias and reverse causality bias.
Our results were also informative about trends in practitioner continuity in English primary care over time and across patient groups. We found slightly lower levels of continuity than those in an earlier study of family physician continuity for patients with long-term mental illness in the UK,109 but much lower than those found in studies looking only at specialist mental health care. 110,111 Higher, and rising,112 rates of consultation in family practice may contribute to these differences. Practitioner continuity in English family practices may be affected by reductions in full-time working and increasing practice size in future. Average UPC scores for all patients in 2011–13 were 0.61. 103 Comparison with our results suggests that this dimension of family physician continuity is not lower for patients with SMI than for patients overall. Based on our results, we predict that further decreases in practitioner continuity in primary care may have negative spillover effects onto the secondary care sector.
Chapter 5 The association between antipsychotic polypharmacy and hospital care utilisation and mortality
This chapter is based on Kasteridis et al. 42 Reprinted with permission from Psychiatric Services (Copyright © 2019). American Psychiatric Association. All Rights Reserved.
Introduction
Antipsychotic drugs are a common component of the therapeutic strategy for patients with SMI. Although UK and international guidelines recommend antipsychotic monotherapy,36,113 antipsychotic polypharmacy (hereafter polypharmacy) – defined as the concurrent use of two or more different antipsychotic agents – is common in clinical practice. 114,115
The most common rationale for polypharmacy is to improve therapeutic response when the response to monotherapy is considered inadequate. 36 However, there is little empirical evidence that polypharmacy has higher efficacy than monotherapy. A Cochrane systematic review91 of RCTs concluded that, although polypharmacy might be superior to monotherapy in certain clinical situations, the evidence was too heterogeneous to derive firm conclusions. Significant risks associated with polypharmacy have been reported, particularly excessive dosing,116 which can in turn result in adverse effects, such as metabolic syndrome,117 cognitive impairment, extrapyramidal side effects118 and cardiovascular disorders. 119 Polypharmacy efficacy and adverse effects contribute to changes in broader patient outcomes reflecting overall polypharmacy effectiveness. Whether polypharmacy is a valid therapeutic option or a ‘dirty little secret’,120 it remains prevalent, and empirical evidence on its effectiveness and associated outcomes is needed.
Methods
Study design
The study employs an observational cohort design. We constructed the antipsychotic prescribing profile of patients from primary care records, which we linked to hospital and mortality data.
The argument underpinning a cohort study design is that effectiveness is assessed under the usual circumstances of health-care practice rather than the possibly atypical circumstances of a RCT, and in many situations it is not possible to randomise to evaluate an intervention. As with all observational studies, validity relies on rigorous design and adjustment of confounding factors to minimise selection bias. Significant progress towards this direction has been made by studies from Denmark121 and Finland,122 which focused on the effect of polypharmacy on mortality. However, studies that explore associations between polypharmacy and inpatient hospitalisations123,124 and A&E department attendances125 suffer from important weaknesses that stem from failure (or inability due to lack of data) to model the timing of polypharmacy episodes and outcomes. The analysis presented in this chapter42 improves on the fundamental issue of confoundedness, by employing a Cox survival analysis model that analysed time to each outcome, adjusting for both time-invariant confounders and time-dependent polypharmacy and monotherapy. 126
Sample
Patients were included in the sample if they had at least one antipsychotic drug record during the observation period and all conditions listed in Chapter 3, Study samples, were met. For reasons explained in Chapter 3, Study samples, we use two samples: one for the analysis of A&E department attendances and one for the analysis of all other outcomes.
Patient outcomes
We investigated the association between polypharmacy and the occurrence of three outcomes: (1) unplanned hospital admissions (all cause), (2) A&E department attendances and (3) mortality.
Definition of antipsychotic polypharmacy
There is no consistent definition of polypharmacy in the literature. We defined polypharmacy as the concurrent use of two or more antipsychotic substances for at least 30 days. The overlap period allowed for cross-tapering between substances. A longer overlap period has a higher risk of misclassifying polypharmacy as monotherapy, whereas a shorter overlap may misclassify switching between substances as polypharmacy.
We considered 33 antipsychotic substances covering first-generation antipsychotic drugs or typical antipsychotics, second-generation antipsychotics or atypical antipsychotics, and depot antipsychotics127,128 (see Appendix 4 for substance product codes used).
The CPRD data provide the date a prescription was issued, but the duration of prescriptions is not always recorded. We therefore inferred treatment duration from the total quantity (number of units) prescribed and the numeric daily dose (ndd) (number of units per day) when duration was absent. Furthermore, the daily dose was missing for 23% of prescriptions. For these prescriptions, we imputed the dose using an imputation strategy explained in Appendix 4. Less than 0.02% of prescription records were dropped from the analysis, as they had implausibly large estimated duration. From the prescription dates and durations, we constructed the patient’s medication profile (times at which the patient was on any antipsychotic medication and on polypharmacy).
We calculated two measures of polypharmacy prevalence. First, we calculated the annual prevalence of polypharmacy as the number of patients with at least one polypharmacy episode in a year divided by the total number of patients observed during that year. Second, we calculated the rate of polypharmacy, defined as the sum of all patients’ polypharmacy days in a year over the sum of all patients’ days at risk of polypharmacy in that year. The latter measure is an improvement over the commonly reported point estimates of polypharmacy prevalence, which measure the proportion of eligible individuals on polypharmacy on a given day (see Appendix 4 for a proof).
Statistical analysis
Semi-parametric Cox hazard models129 were applied to estimate the effect of polypharmacy on the time to the first occurrence of each of the three outcomes. The model adjusts for censoring, which may occur because (1) a patient dies, (2) registration with the practice ends or (3) the study period ends, prior to the outcome of interest occurring. The follow-up period – time from entry to the sample until the outcome occurs or censoring – was different from the observation period for outcomes other than death.
An individual could have multiple polypharmacy episodes. On each day during the study period the patient was in one of three states: (1) received no antipsychotic medication, (2) monotherapy (on one antipsychotic or on more than one, but for < 30 days) and (3) polypharmacy. To model this, we introduced two time-varying binary variables: (1) ‘no antipsychotic substance’, which took a value of 1 during periods the patient was not on an antipsychotic drug and zero otherwise, and (2) ‘polypharmacy’, which took a value of 1 during periods the patient was on two or more antipsychotic substances for > 30 days and zero otherwise. The results were interpreted with regard to monotherapy, which was the reference category.
Robustness checks
We performed two checks. First, to assess the impact on the results of a shorter or longer overlap period, we estimated our models assuming overlap periods of 14, 60 and 90 days. Second, we explored whether or not SMI admissions, which are a subset of all unplanned admissions, were affected differently by polypharmacy.
Results
All patients were prescribed an antipsychotic substance at some point during the observation period. Table 11 provides descriptive statistics. Unplanned admissions and mortality outcomes were studied using the same sample of 17,255 patients from 215 practices. These patients were observed for 5.7 years, on average, and 12.9% of them had at least one polypharmacy episode during the observation period. The average number of polypharmacy episodes per patient on polypharmacy was 5.5 and the mean polypharmacy episode length was 66 days (range 2–2340 days).
Analysis | |||
---|---|---|---|
Unplanned admissions | Death | A&E department presentations | |
Full sample | |||
Individuals, n | 17,255 | 17,255 | 13,247 |
During observation perioda | |||
Mean years | 5.7 | 5.7 | 4.1 |
Patients with at least one polypharmacy episode, n | 2228 | 2228 | 1548 |
Patients with at least one polypharmacy episode, % | 12.9 | 12.9 | 11.7 |
Polypharmacy episodes per patient on polypharmacy | 5.5 | 5.5 | 4.8 |
Number of switches on/off polypharmacy per year | 0.96 | 0.97 | 1.15 |
Mean polypharmacy length (days) | 66 | 66 | 69 |
During follow-up periodb | |||
Mean years | 3.6 | 5.7 | 2.5 |
Patients with at least one polypharmacy episode, n | 1515 | 2228 | 1068 |
Patients with at least one polypharmacy episode, % | 8.8 | 12.9 | 8.1 |
Sample of patients experiencing outcome | |||
Individuals, n | 8916 | 604 | 7523 |
During follow-up period | |||
Mean years | 2.6 | 2.8 | 1.8 |
Patients with at least one polypharmacy episode, n | 704 | 52 | 511 |
Patients with at least one polypharmacy episode, % | 7.9 | 8.6 | 6.8 |
For the unplanned admissions analysis, the average follow-up period was shorter than the observation period (3.6 years), with 8.8% of patients having at least one polypharmacy episode during this period. Almost 52% of the patients (n = 8916) had an unplanned admission and, of those 52%, 7.9% had at least one polypharmacy episode before the admission.
For the mortality analysis, the average time to death or censoring was 5.7 years. Among the 604 patients who died (3.5%), 52 (8.6%) had received polypharmacy.
The smaller sample for A&E department attendances consists of 13,247 patients from 215 practices. Of the 7523 patients with an A&E department attendance, 511 (6.8%) had received polypharmacy.
Figure 5 shows that the annual prevalence of polypharmacy fluctuated between 5% and 6%, whereas the polypharmacy rate was around 2%. Polypharmacy rate estimates were lower than the annual polypharmacy prevalence because the former reflected both if a patient was on polypharmacy during the year and the total duration of polypharmacy episodes. Figures of the annual prevalence of (antipsychotic) polypharmacy (APP) and the polypharmacy rate for different overlap periods are provided in Appendix 4.
FIGURE 5.
Polypharmacy prevalence. Kasteridis et al. 42 Reprinted with permission from Psychiatric Services (Copyright © 2019). American Psychiatric Association. All Rights Reserved.
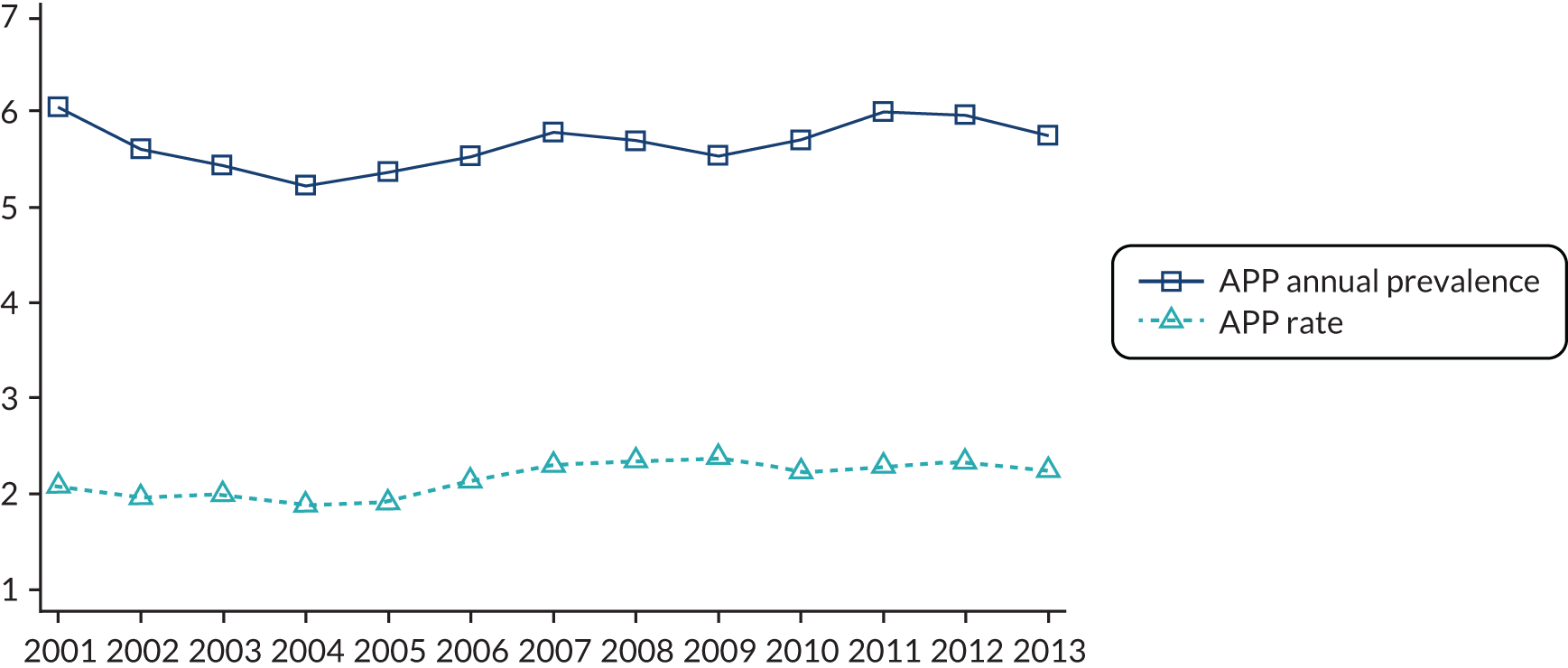
Summary statistics for the explanatory variables are presented in Table 12. Approximately 35% of patients had at least one of the Charlson Comorbidity Index morbidities and 13% were diagnosed with both schizophrenia and bipolar disorder during the observation period.
Variable | Patients, n | % |
---|---|---|
Age at entry date (years) | ||
19–35 | 4484 | 26 |
36–45 | 3718 | 22 |
46–55 | 3017 | 17 |
56–65 | 2341 | 14 |
> 65 | 3695 | 21 |
IMD | ||
Quintile 1 | 2618 | 15 |
Quintile 2 | 3093 | 18 |
Quintile 3 | 3238 | 19 |
Quintile 4 | 4064 | 24 |
Quintile 5 | 4242 | 25 |
Male | 8171 | 47 |
White | 12,521 | 73 |
General practice in rural area | 1921 | 11 |
Number of primary care contacts in year preceding entry into the sample | ||
0–4 | 3798 | 22 |
5–9 | 4496 | 26 |
10–14 | 3271 | 19 |
15–19 | 2158 | 13 |
≥ 20 | 3532 | 20 |
Distance from GP to nearest acute provider (km) | ||
0–3 | 7489 | 43 |
3–6 | 4925 | 29 |
6–9 | 2160 | 13 |
> 9 | 2681 | 16 |
Distance from GP to nearest mental health provider (km) | ||
0–3 | 3441 | 20 |
3–6 | 4330 | 25 |
6–9 | 3178 | 18 |
> 9 | 6306 | 37 |
Number of Charlson Comorbidity Index comorbidities at entry into the sample | ||
None | 11,273 | 65 |
One | 4441 | 26 |
Two | 1079 | 6 |
Three or more | 462 | 3 |
History of depression at entry into the sample | 9746 | 56 |
Schizophrenia | 9653 | 56 |
Bipolar | 5342 | 31 |
Both schizophrenia and bipolar | 2260 | 13 |
Table 13 presents the survival analysis estimates for the two time-varying variables for our main specification, assuming an overlap period of 30 days. Being on polypharmacy (relative to monotherapy) was not statistically significantly associated with the risk of unplanned admission, death or A&E department presentation. Not being prescribed any antipsychotic substance increased the hazard (relative to monotherapy) of an unplanned admission to hospital by 8.2% (95% CI 3% to 13.6%) and the hazard of an A&E department presentation by 18.6% (95% CI 13.5% to 23.9%), but had no effect on mortality risk. For estimates of the impact of other explanatory variables on outcomes, see Appendix 4. Having both diagnoses of schizophrenia and bipolar disorder increased the hazard of an unplanned admission by 20% (HR 1.20, 95% CI 1.12 to 1.29). Living in a more deprived area was associated with a higher hazard of unplanned admissions and A&E department attendances, but not mortality, as was white ethnicity compared with black or minority ethnicities.
Unplanned admissions | Death | A&E department presentations | ||||
---|---|---|---|---|---|---|
HR | 95% CI | HR | 95% CI | HR | 95% CI | |
Polypharmacy | 1.14 | 0.98 to 1.32 | 1.02 | 0.76 to 1.37 | 0.95 | 0.80 to 1.14 |
No antipsychotic substance | 1.08** | 1.03 to 1.14 | 1.02 | 0.94 to 1.10 | 1.19*** | 1.14 to 1.24 |
Robustness check results
Table 14 shows the results of sensitivity analyses that explored the impact of changing the length of overlap in the definition of polypharmacy. The estimated relationships were generally insensitive to the length of overlap. The only exception is unplanned admissions: when the lower boundary of the overlap duration was reduced to 14 days, polypharmacy was associated with an increased hazard of unplanned admission of about 21%.
Days | Unplanned admissions | Death | A&E presentations | |||
---|---|---|---|---|---|---|
HR | 95% CI | HR | 95% CI | HR | 95% CI | |
14 | 1.21** | 1.08 to 1.37 | 1.11 | 0.87 to 1.40 | 0.94 | 0.80 to 1.11 |
30 | 1.14 | 0.98 to 1.32 | 1.02 | 0.76 to 1.37 | 0.95 | 0.80 to 1.14 |
60 | 1.08 | 0.90 to 1.30 | 0.90 | 0.63 to 1.28 | 0.98 | 0.79 to 1.21 |
90 | 1.02 | 0.80 to 1.29 | 0.83 | 0.54 to 1.28 | 0.80 | 0.62 to 1.03 |
The results for SMI admissions are reported in Appendix 4. The results show no association between SMI admissions and polypharmacy.
Discussion
Summary of findings
The principal findings of the study are threefold. Annual polypharmacy prevalence fluctuates over time by between 5% and 6%. Relative to those on monotherapy, patients who are not on antipsychotic medication have an 8.2% (18.6%) higher hazard of an unplanned hospital admission (A&E department presentation). Relative to monotherapy, polypharmacy is not significantly associated with mortality, unplanned hospital admissions or A&E department presentations.
Strengths and limitations
This study is a step forward towards understanding the links between polypharmacy and health-care utilisation and mortality. As with all observational studies, validity relies on rigorous design and adjustment of confounding factors to minimise selection bias. We addressed this fundamental issue employing a three-step strategy.
First, we constructed the antipsychotic prescribing profile of patients from primary care records. In the UK, family practices provide the majority of care for patients with SMI,24 including the management of long-term prescribing. Therefore, unlike previous studies that used solely hospital data to investigate polypharmacy,130 we defined polypharmacy and monotherapy from primary care data. Second, we linked primary care data with hospital and mortality data at patient level, to determine the sequence of polypharmacy episodes, hospital utilisation and mortality. Third, we employed a Cox survival analysis model that analysed time to each outcome, adjusting for both time-invariant confounders and time-dependent polypharmacy and monotherapy. 126 By specifying polypharmacy as a time-dependent variable, we addressed the statistical challenge arising in cases in which the exposure is not present throughout the entire time of observation. The use of a large, linked data set, coupled with a suitable survival analysis model, provided more robust estimates of the effects of polypharmacy on outcomes than would be possible with aggregate data or a cross-sectional design.
There are three main limitations to the study. First, the measures of health status and health-care utilisation prior to diagnosis of SMI may not fully depict the complexities of health status, including severity of the condition. Second, imputing the treatment duration for a number of prescriptions may introduce measurement error in the calculation of polypharmacy. Last, we explored the effect of polypharmacy on broadly defined outcomes. Future research could investigate whether effects vary by reason for admission or for particular combinations of antipsychotic medication.
UK guidelines36 recommend against combining antipsychotic drugs, except as a last resort. These recommendations are based on limited supportive evidence for superior efficacy of polypharmacy over monotherapy, as well as concerns that combined antipsychotics are associated with an increased risk of side effects. Our study cannot draw conclusions on the polypharmacy effect in terms of efficacy and tolerability and, bearing in mind the limitations of an observational study (despite its advanced design), cannot substitute for RCTs. Its contribution lies in providing real-world evidence on the effectiveness of polypharmacy.
Implications for research and practice
Our study examined the overall effectiveness of polypharmacy relative to monotherapy by investigating associations between polypharmacy and three patient outcomes. We found no evidence of a positive or negative effect of polypharmacy on mortality, inpatient hospitalisations or A&E department presentations. At a policy level, these findings do not rule out polypharmacy options, but highlight the need for further research on the appropriateness of polypharmacy.
Current UK guidance36 recommends antipsychotic monotherapy as a treatment option, and our results provide further supportive evidence by establishing a negative association between antipsychotic monotherapy and hospitalisations. This may be because drug therapy helps to stabilise the patients’ condition and allows better management of their physical health. Being prescribed an antipsychotic may be associated with closer or more regular clinical monitoring in the primary care setting, as set out in the guidelines, which recommend that prescription of an antipsychotic should be considered as ‘an explicit individual therapeutic trial’,117 accompanied by detailed requirements for monitoring. The latter may facilitate timely diagnosis and treatment of health problems, avoiding the need for hospital care.
The lack of significant association between polypharmacy and any of the three outcomes provides some reassurance that the safety profile of polypharmacy may be comparable to that of monotherapy. For a shorter overlap period (14 days or longer), which captured more cross-tapering in the definition of polypharmacy, we observed an increase in the risk of unplanned admission. One explanation is that patients who changed drugs might have had more unstable disease profiles and/or that changing drugs further destabilised their condition. This suggests a need for close monitoring in the first few weeks of cross-tapering, when the risk of unplanned hospitalisation is higher.
Chapter 6 The association between primary care quality and (re-)entry to specialist mental health-care services
Introduction
This chapter examines the association between the quality of primary care and the time to (re-)entry into secondary specialist mental health-care services for people with SMI.
We examined the effects of two care quality indicators on time to re-entry to secondary care mental health care: one QOF indicator (CPs developed in primary care) and one non-QOF indicator (the continuity of primary care, which included the number of GP visits within the previous 12 months). Details of these two measures are provided in Chapter 3, The care plan indicator, and Chapter 3, The annual review indicator. The reason for choosing these two quality indicators was that they have potential to prevent, or delay the onset of, acute episodes of SMI by regular reviews of medication and other types of care, and by offering ongoing primary care support. We also considered polypharmacy and ARs as potential indicators of care quality, but rejected these in the context of re-entry to specialist mental health care. The focus of ARs is on patients’ physical health, rather than their mental health, and avoidance of polypharmacy would not be expected to reduce the need for specialist mental health care.
If patients are not currently being treated in secondary care and receive most of their care from their general practice, it is plausible that the quality of that care could affect the time to (re)-entry to secondary care. For example, if a patient’s mental health is deteriorating – and their risk of re-entry to secondary mental health care increases – then the GP could see the patient more frequently and/or ensure that they see the patient themselves each time (i.e. increase the continuity of care) to help stabilise them and so prevent a re-entry.
Methods
Study design
We restricted our sample to individuals whose mental health had been managed fully within primary care for at least 12 months (described as ‘stable’) (see Chapter 3, Study samples). This observational cohort analysis used discrete-time survival analysis to explore the effect of primary care quality on the risk that a ‘stable’ patient with SMI would re-enter specialist mental health care. The outcome was measured in 3-month periods and the primary care quality indicators were measured over the prior 12 months.
Sample
We limited the sample to people who had not been treated in specialist mental health care for at least 12 months prior to the current quarter, as captured in the MHMDS, to try to isolate the effect of primary care quality. The MHMDS includes specialist inpatient and community care. We hypothesised that if the patient was seeing both specialist mental health services and their GP within a 12-month period, the specialist care would be more influential on their subsequent mental health and use of specialist services.
Care quality indicators
The two primary care quality indicators examined in this analysis were CPs and GP continuity, as outlined in Chapter 3, The care plan indicator, for CPs and Chapter 3, Continuity of general practitioner consultations, and Chapter 4, Non-Quality and Outcomes Framework measures of quality: practitioner continuity, for GP continuity. These were hypothesised to influence patient mental health and the probability that a patient would remain stable in the care of the GP rather than requiring specialist care. This analysis uses the UPC to measure GP continuity, as re-entry to specialist mental health-care services reflects deterioration of mental health and being seen by the same GP who knows the patient could help stabilise the patient and prevent re-entry. As in Chapter 4, periods were classified according to both number of visits in primary care and level of GP continuity in the 12 months prior to the start of the period. The classifications were low visit frequency (with continuity undefined – the base category); moderate visit frequency with low continuity; moderate visit frequency with high continuity; high visit frequency with low continuity; and high visit frequency with high continuity (as outlined in Chapter 4, Non-Quality and Outcomes Framework measures of quality: practitioner continuity).
Outcomes
Re-entry to specialist care, although a measure of health-care utilisation rather than health itself, was used as a potential proxy for deterioration in the patient’s mental health. We did not examine first entry to specialist care, as most patients are treated in a specialist context when first diagnosed with SMI. Based on clinical advice, we based our approach on the assumption that only patients whose SMI was stable and well managed would be discharged from specialist services back to the care of their GP for ongoing management. If a patient then re-entered specialist mental health care, this was considered likely to indicate a deterioration in their mental health and a need for escalated care beyond that provided by the GP.
The outcome variable was a binary variable, indicating that within a 3-month period, the patient had some recorded activity in either inpatient- or community-based specialist services, having had no secondary care activity recorded in the previous 12 months. The MHMDS classifies periods of patient engagement with services into cluster episodes (CEs), intended to depict the dates during which the patient receives treatment while allocated to a particular category of mental health problem or needs (termed a ‘cluster’). However, we found that it was common for activities recorded in MHMDS to be outside the bounds of CEs, suggesting recording of errors either of the CE dates or of activities that did not actually occur. We assumed that the former was more likely and based our classification of periods of specialist mental health care on the dates of activity, rather than CE dates.
Statistical analysis
The analysis applied discrete-time survival analysis, with 3-month periods as the unit of time, following essentially the same approach as that outlined in Chapter 4, but with a different outcome variable (see Chapter 4, Statistical analysis for additional detail on this method). Note that, as the outcome indicator is re-entry to secondary care, a HR < 1.0 on a quality measure implies that the measure is associated with a better outcome (a reduced probability of re-entry to secondary care). This approach accounted for the different timing of entry and exit to the observation period for individuals in the sample, and different timing of the outcome. It captured the sequence of events (primary care quality preceding re-entry to specialist care), as well as the duration of time spent in each state. We used a panel-complementary log–log (cloglog) model with a correlated random-effects specification (Mundlak correction),105 to account for unobserved patient-specific effects.
The hazard of re-entering specialist care in a 3-month period – either in the community or as an inpatient – was defined as a function of primary care quality in the previous 12 months, and of patient and practice characteristics as common to all the analyses presented in this report. SEs were adjusted to account for clustering of multiple patients within each practice.
Results
The resulting sample consisted of 70,774 quarterly observations on 9907 patients treated at 214 primary care practices in England.
Table 15 outlines the characteristics of the sample. Most of those who re-entered specialist care during the period of observation (702 individuals, 7.09% of the sample) did so by re-entering community-based mental health care (695 individuals), rather than entering inpatient mental health care directly (seven individuals). The median (mean) value for the UPC in the observed periods was 0.67 (mean 0.66), with 34.8% of periods classified as ‘high’ continuity. A CP had been documented in the previous 12 months for 24% of the patient-periods observed. Mean UPC in periods with a CP in the previous 12 months was 0.68 with 43% of periods classified as ‘high UPC’, compared with a mean of 0.65 and 32% of periods classified as ‘high UPC’ in periods without a CP, in the previous 12 months.
Characteristic | n (%) | Mean (SD) |
---|---|---|
Fixed at baseline | ||
Age (years) | ||
19–35 | 1766 (17.8) | |
36–45 | 1898 (19.2) | |
46–55 | 1975 (19.9) | |
56–65 | 1711 (17.3) | |
≥ 66 | 2557 (25.8) | |
Sex | ||
Female | 5161 (52.1) | |
Male | 4746 (47.9) | |
IMD | ||
Quintile 1 (least disadvantaged) | 1715 (17.3) | |
Quintile 2 | 1931 (19.5) | |
Quintile 3 | 1907 (19.2) | |
Quintile 4 | 2214 (22.3) | |
Quintile 5 (most disadvantaged) | 2140 (21.6) | |
Ethnicity | ||
Black and minority ethnicities | 3118 (31.5) | |
White | 6789 (68.5) | |
Diagnosis category grouping | ||
Bipolar disorder and affective psychoses | 4000 (40.4) | |
Schizophrenia and other psychoses | 4893 (49.4) | |
Both categories | 1014 (10.2) | |
Years since diagnosis | ||
0–1 | 1409 (14.2) | |
2–5 | 2044 (20.6) | |
> 5 | 6454 (65.1) | |
Number of Charlson Comorbidity Index comorbidities | ||
None | 6759 (68.2) | |
One | 2401 (24.2) | |
Two or more | 747 (7.5) | |
History of depression | ||
No history of depression | 4151 (41.9) | |
Comorbid depression | 5756 (58.1) | |
During the observation period | ||
Number of 3-month periods observed | 9.7 (3.2) | |
UPC | 0.66 (0.24) | |
CP in prior 12 months | 0.24 (0.43) | |
Re-entered secondary mental health care | 702 (7.1) |
As Table 16 shows, for periods preceding re-entry to specialist care, on average, in the previous 12 months, patients had more visits to their GP, were more likely to have a CP and had higher GP visit continuity than in the 12 months preceding periods in which they did not re-enter specialist care.
Re-entry | In the current period | In the prior 12 months | |||
---|---|---|---|---|---|
% of periods | % of patients | Mean (median) number of GP visits | % with CP | % with UPC above median | |
Re-enters mental health care | 3.8 | 27.2 | 5.51 (4) | 27.9 | 38.2 |
Does not re-enter mental health care | 96.2 | 72.8 | 4.17 (3) | 23.4 | 34.7 |
The coefficients from the regression model of the discrete-time survival analysis are presented in Table 17. In these results, each level of GP visit continuity is compared with the base category (i.e. two or fewer visits in the prior 12 months). A more informative comparison is that between high and low continuity, which was obtained by taking the ratio of exponentiated coefficients, presented in Table 18. For example, for patients with moderate visit frequency (three to five visits in the prior 12 months), the coefficients on the high and low UPC were exponentiated, with the ratio of these two scores taken to obtain the association of high continuity with the hazard of the outcome compared with low continuity, for that visit level. These results showed that there was no statistically significant association between level of GP visit continuity or the presence of CPs and the hazard of re-entering specialist mental health care.
HR | SE | |
---|---|---|
Time-varying variables: period level | ||
Continuity/visit frequency (base: low visit frequency, continuity undefined) | ||
Moderate frequency, low continuity | 1.353** | 0.129 |
Moderate frequency, high continuity | 1.210* | 0.095 |
High frequency, low continuity | 1.695*** | 0.186 |
High frequency, high continuity | 1.558*** | 0.165 |
CP | 1.079 | 0.073 |
Time-varying variables: mean level | ||
Continuity/visit frequency (base: low visit frequency, continuity undefined) | ||
Moderate frequency, low continuity | 0.733 | 0.150 |
Moderate frequency, high continuity | 1.420* | 0.192 |
High frequency, low continuity | 1.219 | 0.169 |
High frequency, high continuity | 1.133 | 0.151 |
CP | 1.100 | 0.140 |
Time-invariant variables (at start of observation) | ||
IMD (base: quintile 1, least disadvantaged) | ||
Quintile 2 | 1.161 | 0.102 |
Quintile 3 | 1.270* | 0.122 |
Quintile 4 | 1.556*** | 0.153 |
Quintile 5 (most disadvantaged) | 1.599*** | 0.165 |
Ethnicity (base: black and minority ethnicities) | ||
White | 1.296*** | 0.072 |
Age (years) (base: 18–35) | ||
36–45 | 0.979 | 0.075 |
46–55 | 0.857* | 0.066 |
56–65 | 0.777** | 0.072 |
≥ 66 | 0.894 | 0.071 |
Years since SMI diagnosis (base: ≤ 1 year) | ||
2–5 | 0.782** | 0.057 |
> 5 | 0.724*** | 0.059 |
Sex (base: female) | ||
Male | 1.037 | 0.052 |
SMI diagnosis category (base: bipolar disorder, affective psychosis) | ||
Schizophrenia or other psychosis | 1.044 | 0.057 |
Both categories | 1.404*** | 0.100 |
Number of Charlson Comorbidity Index comorbidities (base: none) | ||
One | 1.041 | 0.057 |
Two or more | 0.732** | 0.073 |
Comorbid depression | 1.012 | 0.052 |
Constant | 0.022*** | 0.007 |
HR (95% CI) | |
---|---|
CP vs. none | 1.08 (0.93 to 1.22) |
GP visit continuity | |
Moderate visit frequency (three to five visits): high UPC vs. low UPC | 0.89 (0.76 to 1.06) |
High visit frequency (six or more visits): high UPC vs. low UPC | 0.92 (0.78 to 1.09) |
Factors associated with a higher hazard of re-entering specialist care were greater socioeconomic disadvantage, white ethnicity (vs. black or minority ethnicities) and having diagnoses from both schizophrenia- and bipolar-type classifications (vs. bipolar-type classification alone). Factors associated with a lower hazard of re-entering specialist care were middle age (46–65 years vs. 18–35 years), longer time since diagnosis of SMI (> 2 years vs. 0–1 years) and number of physical comorbidities.
Discussion
Summary of findings
We explored whether or not better quality of primary care was associated with reduced risk of re-entering specialist mental health-care services, either in the community or as an inpatient. Our results indicate no statistically significant associations between either of the two primary care quality measures and the risk of re-entry to specialist care. This suggests that neither the continuity of GP visits to the same doctor nor the presence of a CP in the previous 12 months reduced the chance of deterioration of mental health status, implied by the need for re-entry to specialist care.
However, a lower risk of re-entry was significantly associated with other explanatory variables: being aged between 45 and 65 years (compared with a younger age group), having had a SMI diagnosis for > 2 years and having two or more physical comorbidities. It is possible that individuals who have had a diagnosis for a longer period may potentially have a more stabilised condition than newly diagnosed patients, which may reduce their risk of re-entry to services. Those with a larger number of physical comorbidities may have been at lower risk if management of physical health took precedence over referral to secondary mental health services for the patient’s mental health condition.
A higher risk of re-entry was associated with greater socioeconomic deprivation, being white and having a diagnosis that included schizophrenia-type classifications, in addition to bipolar-type classifications. The latter may suggest that patients with more complex mental health conditions are more at risk of re-entry and the link with deprivation may reflect the commonly observed greater utilisation of many types of health-care services by socially disadvantaged populations experiencing higher levels of need. 131
Overall, only 7% of the sample categorised as being ‘stable’ re-entered specialist secondary care services over a 12-month period, with very few of these patients re-entering inpatient care directly (seven patients). Despite the lack of association with the indicators of primary care quality, re-entry is therefore an uncommon occurrence and suggests that the management of stable patients in primary care is, by and large, successful.
Strengths and limitations
The main strengths of the analysis relate to the longitudinal nature of the data and the methods employed, which improve on standard cross-sectional analyses based on aggregate data. First, by linking primary care and secondary care data together at the patient level we can observe the timing and sequence of events. This allowed us to identify a sample of patients who were categorised as having been ‘stable’ for a period of time and then observe their utilisation of specialised secondary care from that time onwards. As with the other analyses presented in this report, the use of survival analysis techniques allowed us to exploit fully this data linkage to investigate the relationship between primary care quality and utilisation of secondary care. Owing to the rarity of such data linkages, there has been very little previous research on this topic that uses the richness of individual-level data available to this study.
One of the main limitations of the analysis relates to our inability to use the HoNOS scores as a measure of severity of mental health condition, as originally planned (as described in Chapter 1, Deviations from study protocol). This also means that our results may be confounded, given that severity is likely to be an important factor in determining the degree to which the condition can be controlled solely in primary care. Some patients who were deteriorating (and, therefore, went on to appropriately re-enter secondary care) may have been offered more continuity of care and care planning as a priority by their GPs (indicating good-quality care), rather than re-entry indicating a failure of primary care quality.
Re-entry to specialised services may not be a reliable indicator of deterioration of SMI condition, as we do not know from these routine data the reason for and circumstances leading to re-entry. Most patients re-entering the secondary care sector in our sample entered community care services rather than going directly into inpatient care. Depending on the nature of the community services received, they may not be targeted at addressing deterioration, but more at enhancing the quality of general support received. Again, it is feasible that re-entry into secondary care is not always a negative outcome that signals poor management. Last, we had limited measures of primary care quality and it is feasible that there were other aspects of that care that influenced the risk of deterioration of condition, as well as re-entry into secondary care.
Implications for research and practice
The absence of a statistically significant positive association between aspects of quality of primary care and re-entry to specialist secondary care services suggests that prevention of a deterioration of SMI is not solely within the control of GPs. This is not surprising, given the complexity of SMI conditions and the range of services and settings appropriate to meet these needs. The main implication for research arises from the challenges arising from the HoNOS data, which are detailed in Chapter 1, Deviations from study protocol. Good measures of health outcome for people with SMI are central to the ability to undertake research that goes beyond consideration of process measures. Improved recording of HoNOS scores in secondary care would facilitate this and further allow more generalisable conclusions to be drawn from research. Further research to investigate the underlying reasons for re-entry to specialist secondary care services would provide greater insight into the role of primary care in supporting patients to avoid deterioration of SMI conditions.
Chapter 7 The association between primary care quality and multisector costs
This chapter is partly based on Ride et al. 132 This is an Open Access article distributed in accordance with the terms of the Creative Commons Attribution (CC BY 4.0) license, which permits others to distribute, remix, adapt and build upon this work, for commercial use, provided the original work is properly cited. See: http://creativecommons.org/licenses/by/4.0/. The text includes minor additions and formatting changes to the original text.
Introduction
We found CPs and ARs for patients with SMI to be associated with lower risk of hospital SMI-related admissions, ACSC admissions and reduced A&E department attendances. In addition, higher GP continuity was associated with lower risk of hospital ACSC admissions and A&E department attendances. It might therefore be expected that CPs, ARs and GP continuity were successful at reducing health-care costs. There is an absence of evidence on primary care quality and subsequent SMI expenditures. In part, this is due to the inherent complexity of estimating health-care costs for people with SMI, as services are accessed through a number of routes (primary care, secondary care, A&E department and community mental health services). This makes the tracking of costs for people with SMI difficult and requires access to multiple sources of administrative data. However, the question of if and, if so, to what extent, better-quality primary care impacts on health-care costs for people with SMI is important. It is known that patients with SMI have high levels of utilisation of health services and often have comorbidities that require further appropriate and timely treatment (as outlined in Chapter 1, Serious mental illness). Better management in primary care may have cost implications across a number of service settings. This chapter seeks to address this gap in the literature by directly examining the relationship between primary care quality and health-care expenditure for people with SMI.
In this chapter, we examine the association of CPs, ARs and GP continuity with health service costs for patients with SMI. Previous chapters showed these quality measures to be associated with use of hospital care, but their cost implications are unclear. We did not investigate the association between NHS costs and our fourth measure of quality – polypharmacy – because of the greater difficulty in measuring it and because we found no association of polypharmacy with unplanned hospital admissions, A&E department attendance or mortality.
Methods
Study design
Quarterly costs of health-care utilisation for primary care, inpatient admission, A&E department attendances and community mental health services were estimated using a bottom-up costing approach for 3 financial years (12 quarters), from 1 April to 31 June 2011 (quarter 1 of 2011/12) through to 1 January to 31 March 2014 (quarter 4 of 2013/14), applying unit costs for the 2013/14 financial year. 132 We examined the association of costs in a financial quarter with primary care quality in the previous 12 months, accounting for a range of individual and practice characteristics, and unobserved time-invariant heterogeneity.
Sample
The sample consisted of 150,748 quarter observations across 16,485 adults with SMI for the financial years 2011/12 to 2013/14, as outlined in Chapter 3, Study samples.
Table 19 shows the distribution of characteristics across the sample. Just over half of the patients in the sample (56%) were observed for all 12 quarters. The mean age in the sample was 52 (median 50) years. The majority (71%) of patients were white. Around one-third of patients had at least one physical comorbidity and two-thirds had comorbid depression. Three-quarters of patients were current or ex-smokers and 20% had been recently diagnosed with SMI. People in the sample were more likely to live in urban areas than rural areas and in more deprived areas.
Characteristic | n | % |
---|---|---|
Age (years) | ||
18–35 | 3410 | 20.7 |
36–45 | 3390 | 20.6 |
46–55 | 3397 | 20.6 |
56–65 | 2616 | 15.9 |
> 65 | 3672 | 22.3 |
Sex | ||
Female | 8313 | 50.4 |
Male | 8172 | 49.6 |
IMD | ||
Quintile 1 (least deprived) | 2630 | 16.0 |
Quintile 2 | 3033 | 18.4 |
Quintile 3 | 3038 | 18.4 |
Quintile 4 | 3825 | 23.2 |
Quintile 5 (most deprived) | 3959 | 24.0 |
Ethnicity | ||
Black, minority ethnicities | 4690 | 28.5 |
White | 11,795 | 71.5 |
Rurality of general practice | ||
Urban area | 14,592 | 88.5 |
Rural area | 1893 | 11.5 |
Distance from general practice to nearest general hospital (km) | ||
0–3 | 7227 | 43.8 |
> 3–6 | 4607 | 27.9 |
> 6–9 | 2085 | 12.6 |
> 9 | 2566 | 15.6 |
Distance from general practice to nearest inpatient mental health service (km) | ||
0–3 | 3246 | 19.7 |
> 3–6 | 4228 | 25.6 |
> 6–9 | 3007 | 18.2 |
> 9 | 6004 | 36.4 |
Diagnostic grouping | ||
Bipolar disorder and affective psychosis | 6076 | 36.9 |
Schizophrenia and other psychoses | 8575 | 52.0 |
Both categories | 1834 | 11.1 |
Number of Charlson Comorbidity Index comorbidities | ||
None | 10,523 | 63.8 |
One | 4413 | 26.8 |
Two | 1108 | 6.7 |
Three or more | 441 | 2.7 |
Comorbid depression | ||
No history of depression | 6347 | 38.5 |
History of depression | 10,138 | 61.5 |
History of smoking | ||
Non-smoker | 3818 | 23.2 |
Current or ex-smoker | 12,667 | 76.8 |
Years since diagnosis | ||
0–1 | 3228 | 19.6 |
2–5 | 3148 | 19.1 |
> 5 | 10,109 | 61.3 |
Number of quarters observed | ||
1 | 896 | 5.4 |
2 | 829 | 5.0 |
3 | 756 | 4.6 |
4 | 672 | 4.1 |
5 | 623 | 3.8 |
6 | 622 | 3.8 |
7 | 602 | 3.6 |
8 | 573 | 3.5 |
9 | 574 | 3.5 |
10 | 516 | 3.1 |
11 | 597 | 3.6 |
12 | 9225 | 56.0 |
Care quality indicators
As noted in the Introduction, we focus in this chapter on the two QOF indicators (described in Chapter 3, Quality and Outcomes Framework indicators) as measures of primary care quality, as practices were financially incentivised on these measures. We also include GP visit continuity (described in Chapter 3, Continuity of general practitioner consultations).
Costing methods for primary care data
Table 20 provides an overview of all the sources of utilisation data and unit costs (not just primary care). Primary care utilisation data were extracted from CPRD records based on Read codes recorded by practice staff, as part of routine data entry. Three types of primary care activity were included in the analysis: (1) consultations, (2) drugs prescribed and (3) diagnostic tests.
Unit of utilisation | Source of utilisation data | Source of unit cost |
---|---|---|
Primary care | ||
GP consultations: per minute of face-to-face contact | CPRD | Curtis133 |
Practice nurse consultations: per minute of face-to-face contact | CPRD | Curtis133 |
Drug prescriptions: per prescription | CPRD therapy section, BNF codes | Prescribing and Medicines Team134 |
Diagnostic tests: per test | CPRD test section, Read terms | DHSC135 |
Hospital | ||
A&E department presentations: per presentation | HES | DHSC135 |
Inpatient admissions: episode of care | HES | DHSC135 |
Community mental health services | ||
Consultations: per minute of contact | MHMDS | Curtis133 |
ECT: per episode | MHMDS | Greenhalgh et al.136 |
CP approach reviews: per review | MHMDS | Curtis133 |
Day attendances: per day | MHMDS | Curtis133 |
Mental health admissions: per day | MHMDS | DHSC135 |
Unit costs for consultations were obtained from national estimates of the cost per minute of staff time for GPs and practice nurses for 2014. 133 We used individual visit duration data to calculate visit costs rather than a standard unit cost because of evidence suggesting that consultations for patients with mental health problems are likely to be longer than average consultations. 137–140 We considered multiple visits in a day for a patient to a single staff member as duplicates, but included visits to different staff members on the same day.
The CPRD visit duration variable was based on the time that a patient’s electronic record was open for use by a member of practice staff, and our use of this variable assumed that GPs and nurses would open the electronic file when they were about to see the patient and close it at the end of the consultation. However, files may also be opened for review or other purposes at times that do not correlate to patient care, or may not be closed at the end of a consultation. We therefore applied restrictions when visit duration was recorded as zero minutes or > 120 minutes. A recording of zero minutes indicates that the file was opened for < 1 minute. The CPRD data set included two date variables: (1) the date of data entry and (2) the date associated with the consultation, as entered by the practice staff member. If the duration was zero minutes and if the date of data entry was later than the visit date, we assumed that this reflected retrospective recording of a visit that should be costed and applied the sample median duration for that type of visit (see Table 20). Otherwise, we assumed that the entry did not reflect an episode of patient care and dropped these observations from the data. We capped the maximum duration at 120 minutes, assuming that this reflected a file being left open but without ongoing patient care.
A cost of £3.60 per minute of patient contact for GPs included travel and qualification costs, but excluded nursing care staff costs, as we were accounting for these by counting nurse visits separately. A cost of £0.88 per minute of patient contact for practice nurses included qualification costs.
Table 21 outlines the average visit duration for different types of primary care visits observed in the sample.
Visit | Mean (minutes) | Median (minutes) |
---|---|---|
Primary care | ||
GP: face to face, clinic | 10.8 | 9 |
GP: telephone | 6.4 | 4 |
GP: face to face, home visit, other | 11.8 | 5 |
Nurse: face to face, clinic | 11.4 | 9 |
Nurse: telephone | 5.8 | 4 |
Nurse: face to face, home visit, other | 7.1 | 4 |
Community mental health services | ||
Doctor | 53.5 | 30 |
Nurse | 49.1 | 35 |
Other health-care professional | 55.3 | 50 |
The costs of drug prescriptions were derived from the prescription costs analysis for 2014,134 which applied the British National Formulary (BNF) edition 66141 classification system. This provided the net ingredient cost per item according to the drug tariff. It did not include any discounts, dispensing fees or adjustment for income, and was based on the usual quantity dispensed for that drug. Numbers of drug prescriptions were taken from the therapy section of the CPRD data set. We used data at the BNF subparagraph level when available or otherwise at the most specific BNF level available (from paragraph, section or chapter level). This approach did not account for other items listed in CPRD as ‘therapy’ that were not included in the BNF, such as dressings or glucose test strips.
National average unit costs for diagnostic tests were taken from the NHS reference costs for the financial year 2013/14,135 covering diagnostic imaging, diagnostic services, pathology services and outpatient procedures. The reference costs listed the average cost across the NHS of providing a particular health-care service. Utilisation volume of each test was taken from Read terms (descriptive terms attached to Read codes) recorded in the test section of the CPRD data set. These were classified, following clinicians’ advice, into groups that corresponded to the NHS reference cost categories. For example, Read terms for blood tests recorded in CPRD data included ‘liver function test’, and ‘serum creatinine’, which were both classified as ‘clinical biochemistry’ and allocated to that unit cost from the reference costs. A supplementary file is available online and lists the Read terms included in the CPRD data and how they were classified into reference cost categories (see Report Supplementary Material 1).
Costing methods for community mental health care
We used a subset of the activity recorded in MHMDS as measures of utilisation of community mental health services. Specifically, we counted health-care professional contacts (consultations), CPA reviews, electroconvulsive therapy (ECT) sessions and day attendances, and assigned units costs reported by the Personal Social Services Research Unit (PSSRU)133 and the Electronic Staff Record (ESR). 142 The cost of consultations was dependent on the staff type and the duration of the contact.
We used staff groups defined in the PSSRU unit costs 2014,133 namely hospital-based scientific and professional staff, nurses and doctors. Each of these groups was divided into subcategories (called bands) with different costs per hour. Other types of staff (ambulance staff, administration and estates staff, health-care science, general payments) had cost equal to zero. When the occupation code was not recorded in MHMDS, we used the job role to assign a consultation to one of the three staff groups. The PSSRU unit costs reported costs per hour for these groups and bands, which included not only the staff members’ salary but also cost of training and overheads, and were therefore a measure of the total cost of 1 hour of work by the different staff groups.
However, the occupation codes available in MHMDS identified the three staff groups defined in the PSSRU unit costs 2014,133 but not the bands within them. So, we needed a way to calculate an average cost for each of the three staff groups, which we could then match to MHMDS.
We used the ESR to allocate each staff type to a staff group and a band, and then calculated the (weighted) average cost of each staff group (which took into account the composition, in terms of bands, within the staff group).
The ESR had two parts, one containing information regarding the number of workers of different types (measured as full-time equivalents, rather than as head counts) and one containing information regarding the salary for those types of staff. The data for staff numbers were available for each month, so we calculated the average over the financial year (1 April–31 March). The salary data were available by financial year. We used data for 2013/14. ESR contained information for different types of organisations; we focused only on providers that have submitted data to MHMDS (mental health trusts). We used the occupation code and the salary to allocate each staff type to a staff group and a band (the occupation code identified the staff group and the salary was used to identify the band). We then attached the PSSRU unit cost for each staff group and band, and calculated the average cost per hour for the three staff groups (hospital-based scientific and professional staff, nurses and doctors) as a weighted average of the different types of staff in the mental health trusts (i.e. the average cost per hour of, for example, a nurse reflected the number of nurses in the different bands across all mental health trusts). We also calculated an overall average (including staff groups with zero cost), which we used as a cost for consultations in MHMDS without staff type.
As the duration of the contact was an important part of the calculation of the cost of a consultation, we needed to make sure that there were no observations with zero or missing duration. If the duration was zero, we used the information regarding attendance to adjust this: if the patient had attended we initially recoded the duration to missing (which we later replaced) and if the patient had not attended (or the information regarding attendance was missing) we recoded the duration to 1, a minimum amount of staff time that would be required to record that the patient did not attend. When the duration was missing, we replaced it with the average for those from the same staff group for that consultation medium.
Table 21 shows the mean duration of outpatient mental health visits observed in the data. We matched the occupation code of each consultation to the staff groups in PSSRU and assigned to them the cost calculated using ESR and PSSRU. For observations without staff type, we used the overall average cost per hour.
We restricted the number of consultations per day to a maximum of two for each type of staff member. Regarding CPA reviews, MHMDS provided the date only and not the staff involved or duration. We assumed, following clinical advice, that reviews (which were supposed to be multidisciplinary) involved a psychiatrist and a nurse and lasted 30 minutes, a lower-bound estimate. Cost per ECT session was based on past costings136 updated to 2013/14 values using the hospital and community health services pay and price inflation series. 143 Day attendance cost was based on the cost of day care provided by local authority social services for people with mental health problems. 133
Costing methods for hospital data
Admissions to specialist mental health inpatient facilities were recorded in MHMDS and admissions to general hospitals (including admissions to non-specialist mental health providers) were recorded in HES. We costed these separately.
Admissions to specialist mental health inpatient facilities
The cost of mental health admissions depended on the duration of the admission (days) and the category of mental health problem or needs (termed the ‘cluster’) to which the patient was assigned (the hospital-reported reference costs of admitted days varied by mental health cluster). 135 The admitted periods (called ward stays in MHMDS) did not record the cluster, so we needed to get this information from either a CE or a mental health clustering tool (MHCT), as both these types of activities recorded a cluster. A CE identified a continuous period in which the patient was receiving care under the banner of a particular cluster. The MHCT is an algorithm used by a treating clinician or team to allocate patients to a particular cluster based on the patient’s symptoms and functioning. We merged these data to the admitted periods (all fields taken from MHMDS) and compared the start date of the admitted period with the start date of the CEs, and to the dates of MHCTs in the same spell. This allowed us to identify the closest, in absolute value (i.e. before or after the start of the admitted period), start of a CE and the closest MHCT. If the CE start was closest to the start of the admitted period, we used the cluster recorded in the CE as the cluster of the admitted period. If the MHCT was closest, we used that cluster, and if both the start of the CE and the MHCT were equally close to the start of the admitted period, we used the cluster recorded in the CE. This is because the MHCT can be overridden by clinicians. We excluded admissions with the same start and end dates, and for the 14% of admissions without an assigned cluster we used a weighted average cost across all clusters.
General hospital costs
We identified inpatient hospital admissions and A&E department presentations from HES records. The classification system for hospital activity in England is Healthcare Resource Groups (HRGs), which are similar to Diagnosis-Related Groups used in other health-care systems. Inpatient episodes of care and A&E department attendances were grouped into HRGs, reflecting type of activity (inpatient or A&E department HRGs), additional high cost inpatient procedures and long inpatient stays. Costs for these activities were taken from the hospital-reported reference costs.
First, all episodes of care were assigned to one of more than 2000 core HRGs based on the clinical and demographic information of the patient. Second, separate unbundled HRGs were assigned to episodes with high-cost elements of treatment, such as high-cost procedures, chemotherapy, diagnostic imaging and high-cost drugs. Third, HRGs may have contained episodes with much higher length of stay than the average episode in the group. To deal with these long-stay outliers, an upper trimming point was calculated by adding 1.5 times the interquartile range to the third quartile. For admissions exceeding the upper trim point, days above that point are reimbursed at the excess bed-day rate.
Accident and emergency activity was grouped similarly, with the exception that treatment and investigation codes were used to capture clinical information instead of procedure and diagnosis codes. All NHS hospitals reported reference costs for each HRG, broken down by setting (e.g. general surgery) and type of activity (e.g. day case, elective, non-elective). As our research did not relate to the variation in costs across hospitals or settings, we assigned reference costs by HRGs and type of activity only (unbundled costs were aggregated on the HRG level only).
The allocation of episodes to HRGs was carried out using the costing grouper software available on the NHS Digital website. 144 Data were cleaned and prepared according to the grouper guidance. We grouped all the activity using the 2012/13 version of the HRGs design and the corresponding 2012/13 reference costs to obtain costs for each activity, then used the Hospital and Community Health Services Pay and Price Inflation index143 to express costs in 2013/14 prices. For episodes with missing or invalid essential data fields, the grouper returned an error HRG code. After minor adjustments and imputation of some non-essential fields, the error rate for our sample was reduced to about 7% for inpatient episodes and 10% for A&E department presentations. Although HES includes admissions to specialist mental health facilities, these were not costed, because these were captured through MHMDS, but mental health conditions treated by non-specialist mental health providers (HRGs starting with ‘WD’) were included in general hospital costs. Using the inpatient episode start and end dates, costs were apportioned into the financial years 2011/12–2013/14. When an episode of care extended over two quarters, we attributed costs to each quarter based on the proportion of episode days in each of the two quarters.
Statistical analysis
We calculated costs for each patient for each quarter for each sector – primary care, general hospital costs, specialist mental health care (including inpatient- and community-based care) – and for total costs (as the sum of the three sector costs). To provide some indication of the proportion of health-care costs that are related to mental health as distinct from physical health for this population, we identified a subset of costs that could be clearly labelled as mental health ‘related’, comprising all specialist mental health-care costs (inpatient and outpatient), and mental health drug costs in primary care.
The aim of the empirical analysis was to relate the quality of care for a patient to the costs the patient incurs in primary and secondary care. Individual patient health-care cost data are usually highly skewed, with a long right tail. They are also usually characterised by a large mass at zero (patients who do not incur health-care costs in a particular period). These features need to be accounted for in the empirical modelling. This was undertaken by the use of two-part models. 145 The first part used a probit model to estimate the probability of incurring a positive cost. The second part used a generalised linear model (GLM)146 to estimate the level of cost for those patients who incurred a positive cost. The GLM allowed for flexible forms for the distribution of costs to take into account its skewed nature. Based on the results of the modified Park’s test147 and the Pregibon link test,148 we selected a gamma distribution and log-link function for the second part of the model.
The probit model to estimate the probability of incurring costs can be written as:
The GLM to estimate the level of costs for individuals who incur costs can be represented as:
For both Equations 6 and 7, yit was the cost for individual i in quarter t (and follows a gamma distribution in Equation 7), Qualitq are the three quality indicators (CP, AR and GP visit continuity), Xit is a vector of observed time-varying explanatory variables and Zi is a vector of observed time-invariant explanatory variables. ci and mi represent unobserved individual-specific random effects. In Equation 6, Φ() is the standard normal cumulative density function required to estimate a Probit model.
Equations 6 and 7 are non-linear models, which do not allow for the unobserved individual-specific effects to be specified as fixed effects via the use of a set of dummy variables to represent individuals (often referred to as the incidental parameter problem). Instead, we accounted for the individual-specific effects, ci and mi, using the Mundlak approach105 (as outlined in Chapter 4, Statistical analysis), by modelling these as functions of the within-individual means of the observed time-varying variables, as follows:
in which X¯ is the mean of Xit for each individual and ai is a random error term assumed to be normally distributed and uncorrelated with X¯i. We adopted an equivalent specification for mi in Equation 7.
Marginal effects of each explanatory variable on overall estimated cost were calculated by obtaining predictions from the first and second parts of the model for each individual at the base level of the variable and at an alternative level, and then taking the mean of these effects over all individuals. For example, for a binary variable, such as a CP, the predicted probability [Pi] of non-zero costs for individual i was obtained from the first part of the model with the CP variable set at zero and at 1 (P^i0 and P^i1), with all other variables at their observed levels. The equivalent predictions of the level of cost with CP set at zero and 1 were obtained from the second part of the model (y^i0 and y^i1). The point estimate of the average marginal effect of a CP on overall cost was then estimated as:
Standard errors were calculated by accounting for clustering of patients within practices. For the average marginal effects SEs (seAME¯) were obtained by bootstrapping using 1000 replications,149 for which:
and R is the number of replications, AMEr is the estimated marginal effect in replication r and is the mean of the bootstrapped estimates.
Results
Resource use and costs
The mean quarterly health-care cost per patient was £1309.96, with £653.38 (50%) identifiable as related to SMI. Figure 6 shows that total costs decreased with age until middle age and then increased for patients aged > 65 years, whereas the proportion of costs related to SMI steadily decreased with age. For patients aged 19–35 years, 64% of total costs were related to SMI, whereas for patients aged > 65 years the proportion was 34%, reflecting a greater emphasis on management of physical comorbidities.
FIGURE 6.
Costs per quarter for people with SMI by age group: total and SMI related.
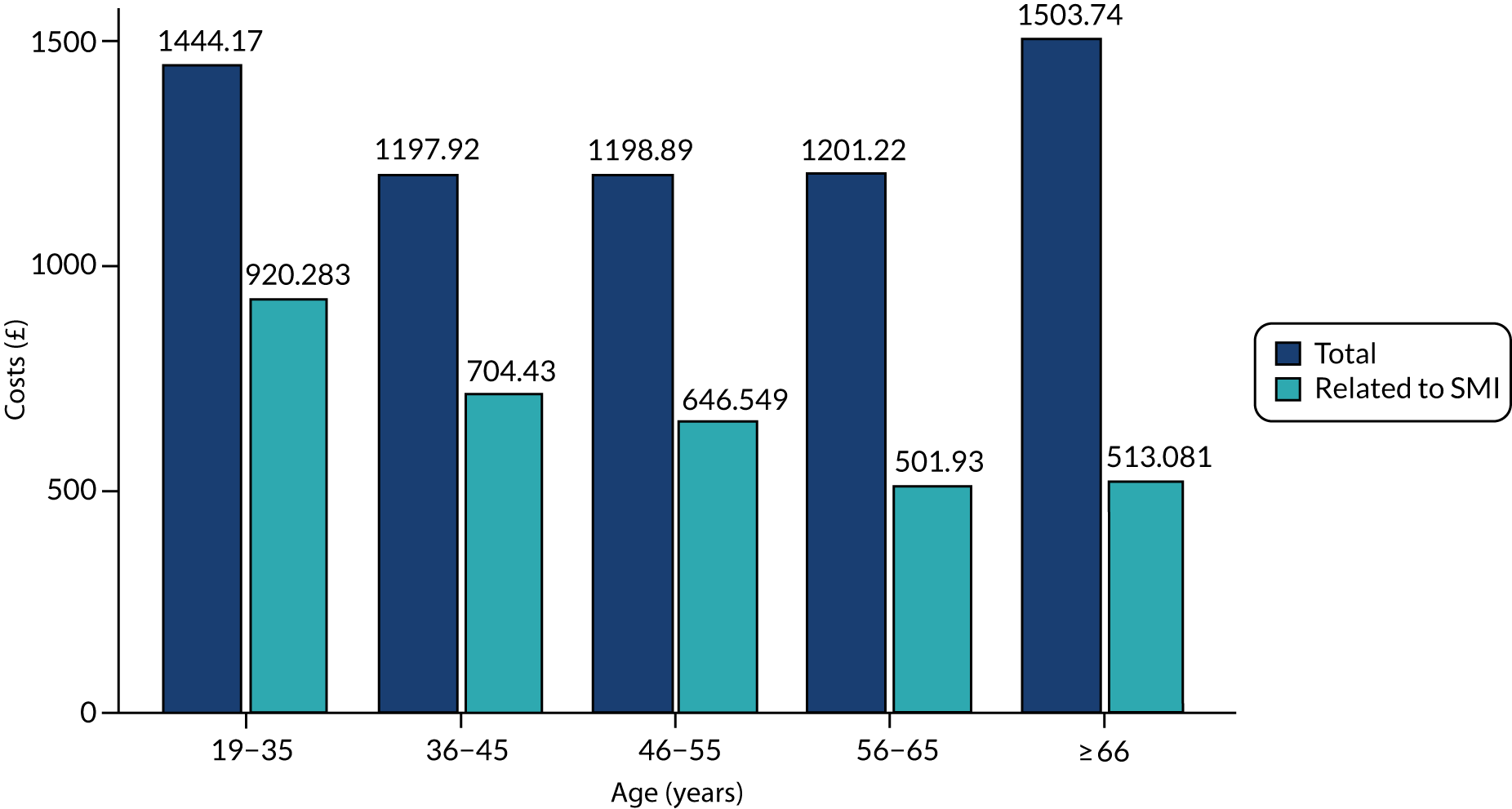
Table 22 shows that primary care costs (£235) made up approximately 18% of total mean quarterly costs, general hospital costs (£481) constituted 37% and specialist mental health-care costs (£594) accounted for around 45%.
Total cost (£) | Primary care cost (£) | General hospital cost (£) | Mental health service cost (£) | |||||
---|---|---|---|---|---|---|---|---|
Mean | SD | Mean | SD | Mean | SD | Mean | SD | |
Overall cost | 1310 | 4721 | 235 | 369 | 481 | 2325 | 594 | 3248 |
Age (years) | ||||||||
19–35 | 1444 | 5491 | 140 | 265 | 423 | 2102 | 882 | 4123 |
36–45 | 1198 | 4650 | 197 | 336 | 354 | 1907 | 647 | 3365 |
46–55 | 1199 | 4366 | 245 | 380 | 377 | 1940 | 577 | 3072 |
56–65 | 1201 | 4149 | 275 | 419 | 491 | 2384 | 435 | 2595 |
≥ 66 | 1504 | 4865 | 306 | 395 | 747 | 3037 | 450 | 2928 |
Sex | ||||||||
Female | 1297 | 4409 | 275 | 410 | 492 | 2184 | 530 | 2979 |
Male | 1323 | 5024 | 194 | 315 | 470 | 2462 | 660 | 3505 |
Ethnicity | ||||||||
Black, minority ethnicities | 865 | 4071 | 165 | 264 | 286 | 2146 | 414 | 2749 |
White | 1491 | 4950 | 264 | 400 | 561 | 2389 | 667 | 3428 |
Diagnostic grouping | ||||||||
Bipolar disorder and affective psychosis | 1099 | 3958 | 250 | 354 | 447 | 2130 | 402 | 2539 |
Schizophrenia and other psychoses | 1363 | 4982 | 215 | 362 | 493 | 2477 | 654 | 3480 |
Both categories | 1722 | 5590 | 275 | 429 | 532 | 2222 | 915 | 4012 |
Years since diagnosis | ||||||||
0–1 | 1558 | 5305 | 224 | 360 | 566 | 2513 | 768 | 3652 |
2–5 | 1249 | 4570 | 226 | 375 | 448 | 2171 | 574 | 3205 |
> 5 | 1276 | 4632 | 240 | 369 | 473 | 2327 | 563 | 3168 |
IMD | ||||||||
Quintile 1 (least deprived) | 1130 | 4306 | 220 | 343 | 430 | 2206 | 481 | 2890 |
Quintile 2 | 1195 | 4348 | 227 | 339 | 459 | 2197 | 510 | 2935 |
Quintile 3 | 1388 | 4967 | 238 | 375 | 526 | 2394 | 625 | 3492 |
Quintile 4 | 1345 | 4741 | 244 | 379 | 476 | 2267 | 625 | 3280 |
Quintile 5 (most deprived) | 1425 | 5038 | 241 | 391 | 504 | 2495 | 680 | 3473 |
Number of Charlson Comorbidity Index comorbidities | ||||||||
None | 1198 | 4654 | 183 | 299 | 390 | 2015 | 625 | 3340 |
One | 1407 | 4718 | 290 | 410 | 549 | 2474 | 568 | 3128 |
Two | 1813 | 5332 | 442 | 557 | 887 | 3600 | 483 | 3007 |
Three or more | 1944 | 4694 | 495 | 530 | 1132 | 3599 | 316 | 2553 |
Comorbid depression | ||||||||
No history of depression | 1319 | 4955 | 184 | 297 | 483 | 2525 | 651 | 3464 |
History of depression | 1304 | 4572 | 266 | 403 | 480 | 2194 | 558 | 3109 |
History of smoking | ||||||||
Non-smoker | 1235 | 4747 | 193 | 275 | 468 | 2446 | 574 | 3221 |
Current or ex-smoker | 1332 | 4713 | 247 | 391 | 485 | 2288 | 600 | 3356 |
Rurality of general practice | ||||||||
Urban area | 1336 | 4808 | 231 | 360 | 490 | 2370 | 615 | 3310 |
Rural area | 1107 | 3983 | 264 | 427 | 413 | 1941 | 430 | 2721 |
Distance from general practice to nearest acute hospital (km) | ||||||||
0–3 | 1369 | 4948 | 232 | 374 | 516 | 2486 | 621 | 3329 |
3–6 | 1270 | 4563 | 221 | 321 | 458 | 2168 | 592 | 3194 |
6–9 | 1244 | 4550 | 258 | 408 | 460 | 2256 | 526 | 3172 |
> 9 | 1268 | 4471 | 252 | 396 | 441 | 2177 | 575 | 3174 |
Distance from general practice to nearest inpatient mental health service (km) | ||||||||
0–3 | 1385 | 5037 | 228 | 349 | 515 | 2464 | 643 | 3400 |
3–6 | 1341 | 4774 | 230 | 355 | 520 | 2587 | 590 | 3233 |
6–9 | 1384 | 4993 | 221 | 335 | 503 | 2378 | 659 | 3440 |
> 9 | 1211 | 4353 | 249 | 402 | 425 | 2004 | 537 | 3071 |
Per cent observations with zero cost | 8.4 | 12.0 | 85.5 | 62.8 |
Table 22 also provides mean cost per quarter for subgroups of patients. The mean total costs increased with age, level of deprivation and number of comorbidities and were higher for white patients (vs. black or minority ethnicities) and for patients diagnosed with SMI more recently. Patients with both schizophrenia- and bipolar-type disorders had higher costs than those with schizophrenia-type disorders alone, with bipolar-type disorders associated with the lowest costs.
Of the quarterly observations, 8.4% had zero total cost, 12.0% had zero primary care cost, 62.8% had zero community mental health cost and 85.5% had zero hospital cost. Approximately one-third (33.3%) of the quarterly observations had no CP or AR recorded in the prior 12 months, 5.4% had a CP but no AR recorded, 40.3% had an AR but no CP recorded and 20.9% had both recorded. Overall, just over one-quarter of observations (26.3%) had a CP recorded in the prior 12 months and nearly two-thirds (61.3%) had an AR recorded.
Table 23 outlines the mean and median quarterly utilisation of the different types of service making up these costs across all individuals in the sample.
Sector | Resource unit | Quarterly utilisation | Mean quarterly cost (£) | |
---|---|---|---|---|
Mean | Median | |||
Primary care | GP: face-to-face clinic visit | 1.2 | 1 | 58.99 (all primary care visits) |
GP: telephone visit | 0.2 | 0 | ||
GP: face-to-face home visit, other | 0.06 | 0 | ||
Nurse: face-to-face clinic visit | 0.4 | 0 | ||
Nurse: telephone visit | 0.02 | 0 | ||
Nurse: face-to-face home visit, other | 0.01 | 0 | ||
Tests (pathology, radiology, etc.) | 2.1 | 0 | 13.09 | |
Drug prescriptions | 16.0 | 8 | 162.99 | |
Specialist mental health | Visits | 3.4 | 0 | 183.73 (outpatient care) |
Day attendances | 0.1 | 0 | ||
ECT sessions | 0.005 | 0 | ||
Reviews | 0.2 | 0 | ||
Mental health inpatient bed-days | 1.2 | 0 | 409.95 | |
General hospital | Inpatient bed-days in general hospital | 1.9 | 0 | 481.20 |
A&E department presentations | 0.1 | 0 | 16.66 |
Regression results
The average marginal effects of quality on cost estimated from the two-part models are presented in Table 24. CPs in the 12 months prior to the start of the current 3-month period were associated with lower health-care costs in the current 3-month period, in terms of overall costs and in primary care and hospital costs, but not mental health-care costs. ARs were associated with lower primary care, mental health care and total costs, but not with hospital costs. High GP visit continuity in the prior 12 months was associated with lower primary care costs in the current 3-month period, but not with total costs or any of the other sectors of costs.
Total costs (£), ME (95% CI) | Primary care (£), ME (95% CI) | Hospital (£), ME (95% CI) | Mental health (£), ME (95% CI) | |
---|---|---|---|---|
Primary care quality indicators in 12 months prior | ||||
CP | –53.40*** (–66.51 to –40.29) | –9.47*** (–11.56 to –7.37) | –25.53*** (–39.83 to –11.24) | –12.50*** (–17.91 to –7.08) |
AR | –33.32*** (–46.92 to –19.73) | –9.21*** (–11.44 to –6.99) | –6.44 (–19.00 to 6.11) | –14.97*** (–21.17 to –8.76) |
High UPC (GP visit continuity) | –1.46 (–12.77 to 9.85) | –2.81** (–4.67 to –0.95) | 1.52 (–10.40 to 13.43) | 3.42 (–1.37 to 8.21) |
Patient characteristics (at start of observation) | ||||
Index of disadvantage of patient’s neighbourhood (base: quintile 1, least disadvantaged) | ||||
Quintile 2 | 24.27 (–1.85 to 50.39) | 1.75 (–4.16 to 7.66) | 17.70 (–1.25 to 36.65) | 14.50 (–0.20 to 29.20) |
Quintile 3 | 96.02*** (43.82 to 148.23) | 5.63 (–5.04 to 16.30) | 82.53*** (42.80 to 122.25) | 31.58* (4.00 to 59.16) |
Quintile 4 | 207.90*** (122.89 to 292.91) | 18.85* (2.96 to 34.75) | 155.83*** (85.52 to 226.15) | 61.41** (18.34 to 104.48) |
Quintile 5 (most disadvantaged) | 399.19*** (259.83 to 538.55) | 39.40** (16.67 to 62.14) | 249.84*** (137.30 to 362.37) | 123.58*** (56.00 to 191.16) |
Ethnicity (base: black and minority ethnicities) | ||||
White | 288.90*** (272.78 to 305.02) | 43.49*** (39.50 to 47.48) | 175.86*** (166.06 to 185.66) | 84.97*** (76.15 to 93.78) |
Age (years) (base: 18–35 years) | ||||
36–45 | –21.24 (–47.56 to 5.09) | 27.57*** (22.46 to 32.67) | –6.39 (–20.48 to 7.69) | –34.54*** (–52.44 to –16.63) |
46–55 | –8.67 (–56.42 to 39.08) | 82.68*** (71.45 to 93.90) | 6.73 (–20.26 to 33.73) | –72.18*** (–99.08 to –45.27) |
56–65 | 30.09 (–42.88 to 103.05) | 183.32*** (159.58 to 207.06) | 79.16** (24.35 to 133.98) | –124.36*** (–151.17 to –97.55) |
≥ 66 | 147.31* (–32.06 to 262.57) | 352.02*** (303.52 to 400.52) | 324.15*** (177.41 to 470.90) | –158.90*** (–179.39 to –138.41) |
Years since SMI diagnosis (base: ≤ 1 year) | ||||
2–5 | –73.62*** (–108.60 to –38.64) | –5.92 (–12.70 to 0.87) | –12.54 (–37.08 to 12.01) | –44.84*** (–62.80 to –26.87) |
> 5 | –168.22*** (–220.89 to –115.54) | –17.05** (–28.20 to –5.90) | –43.52* (–82.01 to –5.03) | –93.39*** (–118.08 to –68.69) |
Sex (base: female) | ||||
Male | –50.66*** (–68.62 to –32.69) | –33.88*** (–37.82 to –29.94) | –31.85*** (–43.26 to –20.45) | 4.45 (–5.13 to 14.03) |
SMI diagnosis category (base: bipolar disorder, affective psychosis) | ||||
Schizophrenia or other psychosis | 66.64*** (48.64 to 84.64) | –15.67*** (–19.83 to –11.51) | 4.23 (–7.26 to 15.71) | 60.72*** (51.95 to 69.48) |
Both categories | 335.36*** (278.60 to 392.12) | –15.76*** (–23.92 to –7.60) | 81.74*** (48.74 to 114.73) | 339.61*** (289.28 to 389.94) |
Number of Charlson Comorbidity Index comorbidities (base: none) | ||||
One | 152.69*** (131.96 to 173.42) | 62.19*** (57.71 to 66.68) | 60.71*** (45.94 to 75.49) | 7.46 (–3.63 to 18.56) |
Two | 535.88*** (458.49 to 613.26) | 239.33*** (222.23 to 258.43) | 267.40*** (208.86 to 325.95) | –13.88 (–36.38 to 8.62) |
Three or more | 1362.61*** (1210.37 to 1604.84) | 667.03*** (595.50 to 738.56) | 719.38*** (540.28 to 898.48) | –65.18*** (–88.15 to –42.20) |
Depression (base: no history of depression) | ||||
Comorbid depression | –0.32 (–19.54 to 18.90) | 32.96*** (29.23 to 36.68) | 2.56 (–10.62 to 15.73) | –25.30*** (–35.97 to –14.63) |
Smoking (base: no history of smoking) | ||||
Current or ex-smoker | 40.76*** (20.96 to 60.56) | 17.32*** (13.10 to 21.53) | 4.40 (–10.60 to 19.41) | 11.83* (1.34 to 22.33) |
Practice characteristics (time invariant) | ||||
Rurality (base: urban area) | ||||
Rural area | –16.34 (–45.15 to 12.47) | 10.47** (3.63 to 17.31) | –5.32 (–25.78 to 15.13) | –36.30*** (–49.50 to –23.09) |
Distance (km) from GP to nearest acute provider (base: 0–3 km) | ||||
3–6 | –18.21 (–39.33 to –2.90) | –5.80* (–10.23 to –1.38) | –27.17*** (–41.22 to –13.12) | –5.41 (–17.13 to 6.31) |
6–9 | –52.52** (–91.95 to –13.10) | 1.23 (–7.63 to 10.09) | –48.03*** (–73.73 to –22.33) | –45.85*** (–63.65 to –28.05) |
> 9 | –35.15 (–94.73 to 24.44) | 3.82 (–9.49 to 17.13) | –55.06** (–91.25 to –18.88) | –33.05* (–60.78 to –5.31) |
Distance (km) from GP to nearest mental health provider (base: 0–3 km) | ||||
3–6 | 6.78 (–20.37 to 33.93) | 5.27 (–0.62 to 11.16) | 2.68 (–16.41 to 21.77) | –10.37 (–24.30 to 3.56) |
6–9 | –1.07 (–50.63 to 48.50) | 1.57 (–9.12 to 12.25) | –6.39 (–43.99 to 31.22) | –1.38 (–27.74 to 24.98) |
> 9 | –29.75 (–97.17 to 37.67) | 12.33 (–3.51 to 28.18) | –39.93 (–88.92 to 9.06) | –15.99 (–49.80 to 17.83) |
Other factors were also associated with costs. Greater socioeconomic disadvantage was associated with higher costs across sectors, as were white ethnicity (vs. black or minority ethnicities) and physical comorbidities. Older age was associated with higher primary care and hospital costs, but with lower mental health-care costs. Patients whose general practice was further from the nearest acute facility had lower hospital costs. Men had lower costs overall and in primary and hospital care. Smoking was associated with higher costs overall and in primary care. SMI diagnosis of a bipolar-type disorder was associated with lower costs than diagnosis of a schizophrenia-type disorder or both categories, and longer duration of SMI was associated with lower costs also.
Table 25 shows how the two parts of the model contributed to the estimated marginal effects for total costs. It reports the coefficients from the estimated models for having positive cost (see Equation 6) and for costs given that they were positive (see Equation 7). In the first part of the model, a negative coefficient implied that the probability of incurring any cost is reduced. In the second part of the model, the coefficients were the proportional change in cost (β = (∂y/∂x)/y). CPs were associated both with a higher probability of having positive costs and with a lower level of cost if a cost was incurred, whereas ARs showed no statistically significant association with the probability of having non-zero costs, but were associated with a lower level of cost if a cost was incurred. Overall, both CPs and ARs were associated with lower costs (as shown in Table 24). High GP visit continuity was associated only with a lower probability that a non-zero cost would be incurred, but overall is not associated with a statistically significant difference in cost.
First part, β (SE) | Second part, β (SE) | |
---|---|---|
Period-specific quality indicators | ||
CP | –0.078* (0.032) | –0.101*** (0.014) |
AR | –0.001 (0.025) | –0.062*** (0.013) |
High UPC (GP visit continuity) | –0.048* (0.019) | –0.002 (0.011) |
Mean of quality indicators | ||
CP | 1.641*** (0.129) | 0.638*** (0.062) |
AR | 2.058*** (0.084) | 0.282*** (0.045) |
High UPC (practitioner continuity) | 0.245** (0.083) | –0.284*** (0.040) |
Patient characteristics (at start of observation) | ||
Index of disadvantage of patient’s neighbourhood (base: quintile 1, least disadvantaged) | ||
Quintile 2 | –0.021 (0.077) | 0.052 (0.042) |
Quintile 3 | 0.014 (0.085) | 0.138** (0.043) |
Quintile 4 | 0.078 (0.082) | 0.182*** (0.040) |
Quintile 5 (most disadvantaged) | 0.243** (0.089) | 0.247*** (0.040) |
Ethnicity (base: black and minority ethnicities) | ||
White | 0.497*** (0.042) | 0.633*** (0.031) |
Age (years) (base: 18–35 years) | ||
36–45 | 0.141** (0.052) | –0.048 (0.038) |
46–55 | 0.316*** (0.068) | –0.019 (0.036) |
56–65 | 0.508*** (0.072) | 0.070 (0.037) |
≥ 66 | 1.068*** (0.075) | 0.199*** (0.038) |
Years since SMI diagnosis (base: ≤ 1 year) | ||
2–5 | –0.205** (0.068) | –0.124** (0.039) |
> 5 | –0.221** (0.064) | –0.187*** (0.037) |
Sex (base: female) | ||
Male | –0.477*** (0.043) | –0.085*** (0.022) |
SMI diagnosis category (base: bipolar disorder, affective psychosis) | ||
Schizophrenia or other psychosis | –0.059 (0.044) | 0.135*** (0.025) |
Both categories | 0.292*** (0.072) | 0.402*** (0.039) |
Number of Charlson Comorbidity Index comorbidities (base: none) | ||
One | 0.452*** (0.048) | 0.281*** (0.027) |
Two | 1.259*** (0.141) | 0.482*** (0.040) |
Three or more | 1.711*** (0.295) | 0.607*** (0.064) |
Depression (base: no history of depression) | ||
Comorbid depression | 0.202*** (0.047) | –0.005 (0.024) |
Smoking (base: no history of smoking) | ||
Current or ex-smoker | 0.197*** (0.046) | 0.074* (0.031) |
Practice characteristics (time invariant) | ||
Rurality (base: urban area) | ||
Rural area | 0.113 (0.095) | –0.028 (0.050) |
Distance (km) from GP to nearest acute provider (base: 0–3 km) | ||
3–6 | 0.106 (0.081) | –0.037 (0.035) |
6–9 | 0.023 (0.115) | –0.069 (0.062) |
> 9 | 0.198* (0.092) | 0.032 (0.048) |
Distance (km) from GP to nearest mental health provider (base: 0–3 km) | ||
3–6 | 0.016 (0.110) | 0.012 (0.045) |
6–9 | –0.088 (0.121 | –0.012 (0.052) |
> 9 | 0.099 (0.105) | –0.057 (0.052) |
Observations, n | 150,748 | 138,091 |
Individuals, n | 16,485 | 16,139 |
Older age was associated with a higher probability of incurring non-zero costs and age > 65 years was associated with a higher cost, if a cost was incurred. White ethnicity was associated with both a higher probability of incurring cost and with a higher level of cost if any cost was incurred, and the same pattern was seen for physical comorbidities. The reverse pattern (lower probability and lower cost) was observed for men.
Robustness check
The robustness check supports the findings from the core analyses, assuming the average duration for each type of consultation, as applied in Curtis133 (instead of using the CPRD duration data), did not make a substantial difference to the estimated marginal effects of the quality indicators, as shown in Table 26 (compare with the results in Table 24).
Primary care quality indicators in 12 months prior | Main analysis (from Table 24), ME (£) (95% CI) | Average duration for primary care visits, ME (£) (95% CI) |
---|---|---|
CP | –53.40*** (–66.51 to –40.29) | –52.90*** (–66.24 to –39.57) |
AR | –33.32*** (–46.92 to –19.73) | –31.67*** (–45.39 to –17.96) |
High UPC (GP visit continuity) | –1.46 (–12.77 to 9.85) | –0.54 (–12.07 to 11.00) |
Discussion
Summary of findings
We calculated the costs of health-care utilisation in each quarter for people with SMI using a bottom-up costing approach for 16,485 patients and a total of 150,748 patient-quarter observations. Using 2013/14 unit costs, the mean quarterly health-care cost per patient was £1309.96, with £653.38 (50%) identifiable as related to SMI (rather than to physical health). Converted to 2018 terms (using the health domain of the Consumer Price Index151), mean quarterly health-care cost per patient was £1432.81, with £714.65 related to SMI. Total costs decreased with age until middle age, and then increased for patients aged > 65 years, whereas the proportion of costs related to SMI steadily decreased with age. For patients aged 19–35 years, 64% of total costs were related to SMI, whereas for patients aged > 65 years the proportion was 33%, reflecting a greater emphasis on management of physical comorbidities. Primary care costs (£235) made up approximately 18% of total mean quarterly costs, general hospital costs (£481) constituted 37% and specialist mental health-care costs (£594) accounted for around 45%.
We estimated two-part models of costs, examining the effect of patient characteristics and quality of care on (1) the probability that they had any (i.e. non-zero) NHS cost in a quarter and (2) the level of cost for those with positive cost. We estimated that having a CP in the previous 12 months was associated with lower health-care costs in the current quarter. On average, the overall cost per quarter per patient was reduced by £53.40, primary care cost by £9.47, hospital costs (excluding those due to SMI) by £25.53 and specialist mental health costs (including inpatient care) by £12.50. Having an AR in the previous 12 months was associated with lower primary care costs (£9.21), mental health-care costs (£14.97) and total costs (£33.32), but not with hospital costs in the current quarter. High practitioner continuity in the prior 12 months was associated with lower primary care costs (£2.81) in the current 3-month period, but not with total costs or with hospital or mental health service costs.
Cost implications
We found in previous chapters that CPs and ARs for people with SMI were associated with reduced risk of hospital SMI-related admissions, ACSC admissions and A&E department attendances, and that greater continuity reduced the risk of hospital ACSC admissions and A&E department attendances. It was therefore to be expected that CPs, ARs and greater GP continuity were associated with reduced hospital costs.
General practices were financially incentivised via the QOF to provide CPs and ARs to people with SMI and these payments had an opportunity cost in terms of forgone care elsewhere in the NHS. It was beyond the scope of our study to attempt a comparison of the value of the gains to people with SMI from CPs and ARs with the opportunity costs of providing them. However, we could compare the QOF payments to practices for providing CPs and ARs with the reductions in costs for people with SMI.
Having a CP in the previous year reduced total costs in a quarter for a person with SMI by £53.40, or £213.60 per year (Table 27). There were 353,695 CPs in all practices in England in 2012/13, so that if the cost saving estimates were valid for all 8020 practices in England taking part in the QOF, and not just those in our sample, the total annual cost saving from CPs for patients with SMI would be £75.5M. Practices were awarded 47,965 points under QOF indicator MH10 for achievement of CPs and, at a national average price per point of £133.76, the total QOF payment for CPs was £6.4M. The total reduction in costs for people with SMI was thus around 11 times as large as the total QOF payments to practices for CPs and the net saving of NHS expenditure was £69.1M. As the other columns in Table 27 suggest, the reductions in NHS costs for people with SMI in each of the three sectors were considerably smaller than the total QOF payments for CPs.
Sector | Mental health | |||
---|---|---|---|---|
Total | Primary care | Hospital (not SMI related) | ||
CPs | ||||
Cost saving per quarter per patient per CP (£) | 53.40 | 9.47 | 25.53 | 12.50 |
Effect per year (£) | 213.60 | 37.88 | 102.12 | 50.00 |
CPs England 2012/13, n | 353,695 | 353,695 | 353,695 | 353,695 |
Cost saving (England) (£) | 75,549,252 | 13,397,967 | 36,119,333 | 17,684,750 |
Total points for MH10 2012/13 | 47,965 | 47,965 | 47,965 | 47,965 |
Price per point (£) | 133.76 | 133.76 | 133.76 | 133.76 |
Total QOF payment for MH10 (£) | 6,415,798 | 6,415,798 | 6,415,798 | 6,415,798 |
Net cost saving (£) | 69,133,454 | 6,982,168 | 29,703,535 | 11,268,952 |
Cost saving per QOF payment | 10.77 | 1.09 | 4.63 | 1.76 |
ARs | ||||
Effect per quarter per AR (£) | 33.32 | 9.21 | 6.44 | 14.97 |
Effect per year per AR (£) | 133.28 | 36.84 | 25.76 | 59.88 |
ARs in England 2010/11, n | 336,988 | 336,988 | 336,988 | 336,988 |
Cost saving (England) (£) | 44,913,761 | 12,414,638 | 8,680,811 | 20,178,841 |
Total points for MH9 2012/13 | 183,748 | 183,748 | 183,748 | 183,748 |
Price per point (£) | 125 | 125 | 125 | 125 |
Total QOF payment for MH9 (£) | 22,968,500 | 22,968,500 | 22,968,500 | 22,968,500 |
Net cost saving (£) | 21,945,261 | –10,553,862 | –14,287,689 | –2,789,659 |
Cost saving per QOF payment | 0.95 | –0.54 | –0.38 | –0.12 |
We can make similar calculations for ARs, although because of the change in the definition of ARs from 2011/12, we used data on QOF payments for the AR indicator MH9 in 2011/12, rather than in 2012/13. As ARs were associated with smaller reductions in total costs for people with SMI, the net cost implications were less dramatic and were, indeed, only positive in relation to total costs.
Although the calculations in Table 27 suggested that the cost savings for people with SMI across all three sectors for CPs and ARs were much greater than the corresponding QOF payments to practices, this does not imply that withdrawing these payments would have increased total costs for people with SMI, as we did not attempt to estimate the effects of the payments on the number of CPs. However, the magnitude of the cost saving associated with CPs suggests that even a small reduction in CPs, due to removal or reduction in the QOF incentive payment, would have had an opportunity cost in terms of care forgone in other parts of the NHS. Alternatively, to deliver the same level of care overall, the budget would have needed to increase.
Strengths and limitations
Tracking health-care resource use of people with SMI is particularly problematic because they receive NHS care in primary, secondary and community care. To assess accurately the implications of better quality of primary care on health-care expenditure, detailed information at an individual level is required over a substantial period of time. A strength of this study is in exploiting data linked across a number of administrative sources at an individual patient level. Successful linkage and management of such data is non-trivial and requires both substantial expertise and computational resources. The ability to observe health-care use in continuous time over a 3-year period provided a richness of data of sufficient detail to allow us to link resource use to practice quality accurately and robustly. Costing health-care services, particularly primary care consultations, was not straightforward and required a number of assumptions to be made, for example about the duration of a consultation when these were recorded in the data set as zero or > 2 hours. Although we believe that our assumptions were reasonable, better-quality recording in primary care would make cost estimates more reliable. However, complex data derived from different administrative systems will always require some assumptions to be applied when data are imputed and cleaned to prepare for analysis. Health-care cost data are typically highly skewed with long right-hand-side tails in their distribution, and often contain a large mass point at zero. These features were observed in our data and required non-standard regression models to estimate the link between costs and covariates. With a large mass point at zero, the two-part model applied here was the appropriate way to derive marginal effects of the impact of quality on costs.
Implications for research and practice
We found that younger patients with a SMI had a high level of need and generated additional costs for the NHS, mainly in specialist mental health care. There was also considerable variation between patients: most did not generate any hospital costs in a given year, but those who did were more likely to be admitted to general rather than mental health wards and may have had long and costly admissions. Further research to identify characteristics of patients who are more likely to develop comorbidities and to require admission may help to identify interventions that could improve outcomes for people with SMI and reduce secondary care costs. A better understanding of the characteristics of patients who attend general rather than mental health hospitals and variations in admission patterns may also help identify ways to manage costly resources more efficiently.
We found that the greatest volume of care for people with SMI was provided by specialist services, with patients having three times as many contacts as with primary care. As we used primary care records in this research, we had limited information on the quality of specialist services, and this warrants further investigation. In terms of quality of primary care, only one-fifth of patients received both a CP and an AR, and one-third received neither, despite the existence of financial incentives for practices to provide them. These low rates may have had serious implications for patient health and NHS resources: patients who were in receipt of a CP had fewer emergency contacts and generated lower primary and secondary care costs than those without, and patients who were reviewed annually generated lower primary and mental health-care costs. These interventions require further exploration, as the pathways to improved outcomes remain unclear (e.g. ARs were not, as might be expected, associated with reduced secondary care costs, but with reduced mental health-care costs).
According to the national QOF results,152 7832 patients nationally (12.7% of registered people with SMI) were ‘exception reported’ for the CP indicator in 2017/18, with wide geographical variation (from 6.6% in London North East and Central to 18.6% in Midlands Central). Another 41,784 patients (9.1%) did not receive a CP, despite not having a reason for exclusion recorded. There may therefore be potential to improve outcomes and substantially reduce costs for people with SMI by moving towards full implementation of CPs and ARs. Although most patients receive the recommended care in the context of the financial and reputational incentives offered by QOF, variation in achievement rates between regions and practices suggests that higher rates are achievable. Further research into the reasons why patients do not receive CPs and ARs could suggest potential solutions to this problem, and address the geographical variations in quality and costs of care.
Chapter 8 Conclusions and recommendations
Summary of findings
This study undertook two substantive analyses. The first was a systematic review (see Chapter 2) to identify primary care quality indicators for patients with SMI that could be assessed using routine data. In total, 1847 studies and database sources were identified using the search. A final set of 27 records were examined, which generated a set of 59 different quality indicators. Of these, 17 had already been included in the QOF. The quality of the evidence underpinning most of the indicators was relatively weak. However, we decided to construct two quality indicators identified in the review process and by PPI members as being important (continuity of GP care and polypharmacy), to enhance our subsequent analyses.
The second substantive component of analysis was applying a retrospective observational study using linked administrative data from primary care practices in England. Practices were those contributing to the CPRD GOLD database, with linkages made to hospital records in HES, A&E department attendances, mortality and community mental health service records in MHMDS. We tracked a cohort of people with SMI in primary care until they experienced an outcome or an event, using survival analysis and four different samples, depending on the outcomes examined and the inclusion and exclusion criteria.
In Chapter 4, the sample consisted of 19,324 patients attending 215 practices. Half of the sample (50.3%) had an A&E department presentation at some point during the observation period, 13.1% had an admission for SMI and 12.8% had an ACSC admission. A CP had been documented in the previous 12 months for 40% of the patient-periods observed and an AR in 69% of periods.
Having a CP documented in the previous 12 months was associated with a lower hazard of 26% for A&E department attendances (HR 0.74, 95% CI 0.69 to 0.80), 33% for SMI admissions (HR 0.67, 95% CI 0.59 to 0.75) and 27% for ACSC admissions (HR 0.73, 95% CI 0.64 to 0.83). The reduction associated with AR was 20% for A&E department presentation (HR 0.80, 95% CI 0.76 to 0.85), 25% for SMI admission (HR 0.75, 95% CI 0.67 to 0.84) and 24% for ACSC admission (HR 0.76, 95% CI 0.67 to 0.87).
An alternative way to express this is the absolute risk of each outcome with and without each care quality indicator, obtained by using the model results to predict the probability of each outcome for the individuals in the sample under two scenarios, that: (1) no-one has the indicator and (2) everyone has the indicator. Using this approach, the model estimated that the hazard of A&E department attendance was 5.4% without a CP and 4.1% with a CP, hazard of SMI admission was 0.9% without a CP and 0.6% with a CP, and hazard of ACSC admission was 0.7% without a CP and 0.5% with a CP, per 3-month period. Equally, the hazard for an A&E department attendance was 4.7% without an AR and 3.8% with an AR, for SMI admissions it was 0.8% without an AR and 0.6% with an AR, and for ACSC admissions it was 0.7% without an AR and 0.5% with an AR, per 3-month period.
For those with moderate visit frequency (three to five visits in the previous 12 months), higher GP continuity was associated with 11% lower hazard of A&E department presentation (HR 0.89, 95% CI 0.83 to 0.97) and 23% lower hazard of ACSC admission (HR 0.77, 95% CI 0.65 to 0.92), but not with risk of SMI admission. For frequent attenders (six or more visits in the previous 12 months), higher GP continuity was associated with 26% lower hazard of ACSC admission (HR 0.74, 95% CI 0.62 to 0.87), but not with risk of A&E department presentation or SMI admission.
Expressed as absolute risk, for those with moderate visit frequency, the hazard for A&E department attendances was 4.0% with low continuity and 3.6% with high continuity, whereas the hazard for ACSC admissions was 0.6% with low continuity and 0.4% with high continuity, per 3-month period.
In Chapter 5, the sample consisted of 17,255 patients for the analysis of unplanned admissions and mortality (and 13,247 for A&E department attendances) from 215 practices. On average, 12.9% of patients had at least one episode of polypharmacy during the observation period. The average number of polypharmacy episodes per patient on polypharmacy was 5.5 and the mean polypharmacy episode length was 66 days (range 2–2340 days). Annual prevalence of polypharmacy fluctuated between 5% and 6%.
Relative to monotherapy, polypharmacy was not significantly associated with higher mortality, unplanned hospital admissions or A&E department attendances. Not being prescribed any antipsychotic substance increased the hazard (relative to monotherapy) of an unplanned admission to hospital by 8.2% (95% CI 3% to 13.6%) and the hazard of an A&E department presentation by 18.6% (95% CI 13.5% to 23.9%), but had no effect on mortality risk.
In Chapter 6, the sample consisted of 70,774 quarterly observations on 9907 patients treated at 214 practices. Most of those who re-entered specialist care during the period of observation (702 individuals, 7.09% of the sample) did so by re-entering community-based mental health care (695 individuals), rather than direct into inpatient mental health care (seven individuals). For periods preceding re-entry to specialist care, on average, in the previous 12 months patients had more visits to their GP, were more likely to have a CP and had higher practitioner continuity than in the 45 months preceding periods in which they did not re-enter specialist care.
Results from the discrete-time survival analysis showed there was no statistically significant association between level of practitioner continuity or CP and hazard of re-entering specialist mental health care. Factors associated with a higher hazard of re-entering specialist care were greater socioeconomic disadvantage, white ethnicity (vs. black or minority ethnicities), and having diagnoses from both schizophrenia- and bipolar-type classifications (vs. bipolar-type classification alone). Factors associated with a lower hazard of re-entering specialist care were middle-age (aged 46–65 years vs. aged 18–35 years), longer time since diagnosis of SMI (> 2 years vs. 0–1 years) and number of physical comorbidities.
In Chapter 7, quarterly costs of health-care utilisation were estimated using a bottom-up costing approach and the sample consisted of a total of 150,748 quarter observations across 16,485 patients. The mean quarterly health-care cost per patient (at 2012/13 costs) was £1310, with £653 (50%) identifiable as related to SMI (rather than physical health). Total costs decreased with age until middle age and then increased for patients aged > 65 years, whereas the proportion of costs related to SMI steadily decreased with age. For patients aged 19–35 years, 64% of total costs were related to SMI, whereas for patients aged > 65 years the proportion was 34%, reflecting a greater emphasis on management of physical comorbidities. Primary care costs (£235) made up approximately 18% of total mean quarterly costs, general hospital costs (£481) constituted 37% and specialist mental health-care costs (£594) accounted for around 45%.
Marginal effects estimated from the two-part models suggest that CPs in the 12 months prior to the start of the current 3-month period were associated with lower health-care costs in that period, in terms of overall costs (£53 per patient), primary care costs (£9), hospital costs (£26) and mental health-care costs (£12). ARs were associated with lower primary care costs (£9), mental health-care costs (£15) and total costs (£33), but not with lower hospital costs. High practitioner continuity in the prior 12 months was associated with lower primary care costs (£3) in the current 3-month period, but not with lower mental health-care costs, hospital costs or total costs.
In terms of the cost implications of our analysis, we compared the reductions in costs for people with SMI with the costs of QOF payments to practices for providing CPs and ARs. We estimated that the annual savings from CPs across all sectors was £75.5M in 2012/13 relative to the total QOF payments for CPs of £6.4M, suggesting a net saving of NHS expenditure of £69.1M. The net savings in NHS costs for people with SMI was positive across all three sectors for CPs. The equivalent net saving figure for ARs in 2011/12 was smaller at £21.9M and was only positive in relation to total costs (not across any of the sectors).
Table 28 summarises the results for each indicator by each outcome.
Quality indicator | SMI admissions | ACSC admissions | All unplanned admissions | A&E department attendances | Mortality | Re-entry to specialist mental health services | Cost |
---|---|---|---|---|---|---|---|
QOF indicators | |||||||
CPs | Lower hazard | Lower hazard | Lower hazard | Not significant | Lower total costs, primary care costs, hospital costs, mental health costs | ||
ARs | Lower hazard | Lower hazard | Lower hazard | Lower total costs, primary care costs, mental health costs, but not hospital costs | |||
Non-QOF indicators | |||||||
Continuity of care | Not significant | Lower hazard | Lower hazard (moderate visits); not significant (high visits) | Not significant | Lower primary care costs, but not other costs | ||
Polypharmacy | Not significant | Not significant | Not significant |
Comparison with other research
Previous research into the relationship between primary care quality and unplanned hospital utilisation has often relied on aggregate-level data, such as the study by Baker et al. ,153 which found that higher performance on the QOF overall was not a predictor of area-level A&E department attendances. Other studies have shown an association between subjective assessment of higher primary care quality and lower utilisation of A&E. 154,155 Our previous study (Gutacker et al. 43) found that higher achievement on the AR indicator was associated with a higher rate of SMI admissions. Our new analysis, which was able to determine the sequence of events and found the reverse association, supports the hypothesis that the previous study’s findings may have been driven by QOF indicators being documented after a hospital admission. A study by Harrison et al. 156 found that the introduction of the QOF was associated with a decrease in unplanned admissions for incentivised ACSCs, consistent with our finding that CPs were associated with a reduced risk of ACSC admissions.
The estimated annual antipsychotic polypharmacy prevalence in our study is not directly comparable with other studies owing to diversity in the definition of polypharmacy, and differences in the sample characteristics and methodology. A large international study estimated a global median of 20%,157 but there is considerable variation between and within geographic locations. 157,158 Higher rates of polypharmacy have been estimated for the UK, but the patients included in those studies were prescribed at least one antipsychotic at the date of data collection and polypharmacy was defined as the concurrent use of more than one antipsychotic on that single date159,160 (a definition that is likely to overestimate polypharmacy). A more comparable approach by Kadra et al. ,130 using a 6-week overlap to define polypharmacy, estimated prevalence to be 11.5%. The lower estimate of polypharmacy prevalence in our study is because, although some patients are at risk of polypharmacy for the entire calendar year, others are present for only a few days and, therefore, are less likely to be on polypharmacy for that year. In Kadra et al. 130 patients were at risk for the entire study period.
It is widely believed that polypharmacy increases the risk of mortality and hospitalisations, but there is a lack of methodologically sound studies to support this assumption. To our knowledge, the only previous study using data drawn at a national level of medication prescriptions and appropriate methods to adjust for confounding factors was conducted by Tiihonen et al. 122 Tiihonen et al. 122 investigated the impact of polypharmacy on mortality using a cohort of 2588 patients from Finnish hospital data and concluded that polypharmacy was not associated with increased mortality. This conclusion is reinforced by our study using a significantly larger cohort of 17,255 patients with a record of SMI diagnosis in primary care. Our study further concludes no association between polypharmacy, inpatient hospitalisations123,124 and A&E department attendances, in contrast with the positive correlations found in previous studies. 123–125
There is a dearth of evidence on the cost implications of primary care quality for people with SMI. Previous studies have focused mostly on the costs of SMI, both direct costs to the health-care system, often emphasising hospital costs, and sometimes the wider indirect costs to society. 161–168 A systematic review169 examining the association between mental health-care hospital costs and patient characteristics identified the presence of psychotic or affective symptoms as key cost drivers of hospital costs. Other studies have used a micro-costing approach to examine differences in characteristics between high-cost users (the top decile) and the rest of the cost distribution,170,171 and show that a small proportion of mental health-care users can account for a disproportionately large share of health-care costs, the majority of those being patients with SMI.
Strengths and limitations
The primary strength of the research presented in this report was the access to detailed linked primary, secondary and community care records, covering the full patient population with a confirmed diagnosis of SMI from a representative sample of general practices in England. These linkages allowed, for the first time, analysis of the entire patient pathway from routine care provided by GPs, over acute services delivered in hospitals’ A&E departments and wards, to long-term inpatient care provided in specialised mental health hospitals and in the community. This is especially relevant in the case of chronic conditions, such as SMI, for which secondary care is not limited to acute hospitals and for which widely available data sets, such as HES in England, provide only a partial picture of the quality and quantity of care received.
In addition, the study benefited from event-level data, which allowed the chronological order of exposure to treatment (e.g. receipt of a CP) and subsequent outcome (e.g. hospitalisation) to be determined. This is a necessary condition for establishing causality. Previous studies of the effect of high-quality primary care on unnecessary hospitalisations, as incentivised in the QOF, were limited to aggregate data at the practice-year level,43,156 and, therefore, could not ascertain which patients received care or experienced outcomes, or in which order.
The use of these large linked longitudinal data sets provide more robust estimates than would be possible with smaller sample sizes in a cross-sectional design or with aggregate data. Our sample sizes are an improvement on much of the previous literature in this area, which has suffered from small sample sizes.
Another strength of this study was the use of advanced statistical methods that allowed controlling for time-invariant unobserved patient heterogeneity. Failure to observe, and thus control for, time-invariant differences across patients may lead to biased estimates of the relationships of interest if time-invariant patient characteristics correlate with outcomes. Examples of unobserved patient characteristics that can be assumed to be stable – at least over short time periods – include severity, family history and health-care-seeking behaviour.
We were able to construct measures of primary care quality in areas of importance to patients who went beyond those currently included in the QOF. As well as enriching our analysis, this is also informative for the future development of the QOF for SMI.
A significant strength of this study was costing health-care services across a number of administrative sources at an individual patient level, which required significant expertise as well as extensive computational resource. To the best of our knowledge, a bottom-up costing of the full patient care pathway has not been undertaken previously. This enabled us to determine in which health sectors savings might fall as a result of improvements in quality of primary care. It also allowed us to compare the cost of QOF indicators with the savings that are generated from use of these indicators, which enables a better understanding of relative resource use.
The main limitation of this research derives from the observational nature of the data and the consequential inability to control for confounding due to non-random allocation to treatment and reverse causality bias. For example, patients who are at low risk of complications may be less likely to have a CP written for them if health-care professionals perceive the benefit of such a CP to be low. This would result in an upwards biased estimate of the effectiveness of CPs in an unselected population. Conversely, during periods of deterioration leading to admission, GPs may have less opportunity to, or put less emphasis on, spending time on preventative measures, such as CPs. This would induce a negative correlation between having a current CP and risk of unplanned hospitalisation. The direction of bias is undetermined and our results should be interpreted as showing association, not causation, while keeping in mind that these analyses are an advance on previous studies that used aggregate rather than individual-level data, and cross-sectional rather than longitudinal data.
A further limitation of our study is the focus on newly diagnosed patients and their care patterns. It is conceivable that patients who had been diagnosed with SMI more recently would have received more attention from their GP than those who had already been living with their condition for a long time and were stable. It is therefore unclear whether or not the estimated associations would also apply to subpopulations of people with SMI that were not included in our study.
Another limitation is that we have assumed that some aspects of health-care utilisation, such as an admission or re-entry to secondary mental health services, are poor outcomes. However, it is conceivable that some of these events, including an emergency admission, may be entirely appropriate and may not always be a negative outcome that signals poor management. We are unable to tell from routine data whether or not this is the case. Equally, our definition of polypharmacy will include some patients for whom this is an appropriate management strategy and within prescribing guidelines, and does not represent a negative outcome, although polypharmacy is a well-used, macro-level indicator of poor prescribing practice.
Finally, the clinical outcomes we examined are important as they represent some of the excess health risks for people with SMI and carry substantial health-care costs. However, they are not the only outcomes that matter. For example, both people with SMI and GPs value continuity of care in itself as part of how they experience receiving and giving care. 106,107 Regular physical reviews may uncover health issues that require treatment, but which would not necessarily have led to a hospital admission. Broader outcomes, which are important to people with SMI, may also be affected by the different measures of care quality, including social functioning and health-related quality of life. 108 Patient-reported outcome measures and patient-reported experience measures would provide a way to capture broader measures of outcome and quality, but are not routinely collected and reported in primary care or hospital care.
Generalisability
All samples analysed in Chapters 4–7 used CPRD GOLD primary care data to identify patients with a diagnosis of SMI. A study conducted in collaboration between CPRD and the London School of Hygiene & Tropical Medicine96 concluded that patients in the CPRD GOLD database are broadly representative of the UK general population in terms of age, sex, ethnicity and BMI. This was in line with previous research by CPRD,98 which also demonstrated slightly lower mortality rates in younger age groups than national rates, and a larger median practice size than the national average. Owing to the geographical distribution of Vision software use, areas in the East Midlands, Yorkshire and The Humber, and the north-east of England are under-represented relative to areas in Wales, Scotland and Northern Ireland.
All samples were also restricted to those patients eligible for linkage to other data sources, including HES, which relies, in part, on the general practice providing consent. Previous research172 has shown that patient characteristics are similar between those eligible for linkage and those who were not, including in terms of age, BMI, duration of follow-up, medication use and medical history. However, there were small variations in practice consent by geographical region, with practices in London being less likely, and practices in the south-west of England being more likely, to take part in the linkage scheme.
Patients were included in the studies based on SMI diagnosis codes being recorded in their primary care record. This means that patients who were not permanently registered with a general practice would not have been included. Additionally, patients with SMI but unknown to the GP (e.g. not yet presented to a GP, or fully managed within secondary or specialist care) would not have been included. Additionally, GPs may choose not to use specific codes to record that a patient had SMI when the diagnosis was less certain, or if they preferred to use free-text comments to record the mental health status of the patient. However, it should be noted that GPs are typically closely involved in the care of patients with SMI and, hence, the reliance of this study on primary care records to identify such patients is not a major limitation.
This study used only CPRD GOLD primary care data, which led to a lack of geographical representativeness of England as a whole. Future studies should consider combining CPRD GOLD data with the newly available CPRD Aurum data, collected from practices using the EMIS (EMIS Health, Leeds, UK) software system, to improve representativeness.
Patient and public involvement
An integral aspect of this research project was the involvement of experts by experience. Both CD and LA have lived experience of SMI and their contributions to this project were invaluable. Key contributions were (1) assessing the degree to which the outcomes and quality measures we discussed throughout the project (not just those we analysed) were important and valid for service users; (2) drawing on their own experience of the entire care pathway to advise us on the interpretation of results; and (3) acting as a sense check about a whole range of aspects of the project, allowing us to stand back from the technicalities of the modelling and think about what we were really trying to do.
Both individuals formed part of the research team, which involved attending regular team meetings and contributing to discussions of the analytical approach and interpretation of the results, as well as participating on the SSC and guiding the research programme. Having two individuals involved in the PPI aspect of this project throughout allowed insights from more than one perspective. It also meant that when an individual was unable to attend meetings, the other individual was still able to contribute. The experts by experience had the support of other research team members, as and when needed.
The involvement of people with lived experience allowed the team to focus on key elements important to people who use health-care services for their mental health. CD and LA assisted with many aspects of the project: from the start in developing the research proposal, to interpreting the results, to finally disseminating the findings in a manner suitable for laypeople (e.g. supporting deriving the key messages for production of a video). Both individuals have co-authored publications, and LA is now actively engaged in research, and reports that her involvement in the project has enhanced her skills in research.
A workshop for primary care practitioners on ‘improving the quality of primary care for people with serious mental illness’ was held to gather the perspective of GPs and other health-care professionals on the practical aspects of primary care quality for people with SMI. At this workshop, we asked participants for feedback on issues relating to the care of people with SMI, including writing of CPs, liaison with specialist mental health care, management of physical compared with mental health, challenges of providing care for the patient population and system constraints. The feedback helped guide the research team’s thinking around the variation in delivery, adherence, content and quality of CPs and ARs.
Conclusions
The provision of both CPs and ARs represent indicators of good-quality primary care, in that they are associated with a lower risk of SMI admissions, ACSC admissions and A&E department attendances, and thus reductions in more expensive secondary care utilisation. Both of these quality indicators are also associated with reduced total costs across the health-care sector, although the distribution of the cost reductions varies across indicators. CPs are associated with lower primary care and hospital costs, whereas ARs are associated with lower primary care and mental health-care costs. It would therefore seem reasonable to continue to incentivise both these indicators in the QOF, and encourage their use and uptake in clinical practice.
We provided important new evidence on two additional non-QOF indicators, as we found the evidence base for many quality indicators for people with SMI in primary care lacking. The non-QOF indicators we identified also hold promise as quality indicators. High continuity of care was associated with a lower risk of ACSC admissions and A&E department attendances. It also served to reduce primary care costs, although only by a small amount. Continuity of care is, however, difficult to operationalise as a QOF indicator for two reasons: First, there are many definitions of continuity of care and there is no consensus on what constitutes the most appropriate definition. Second, a continuity of care indicator could potentially be ‘gamed’ by practitioners (e.g. by changing coding practices without a corresponding change in clinical practice). In contrast, an indicator for (absence of) polypharmacy could be operationalised for the purposes of the QOF, but polypharmacy showed no significant associations with any of the outcomes we examined. Although it would not necessarily be a good indicator to include as a metric into a P4P scheme, in the absence of clinical benefit, the analysis nevertheless provided important new evidence that when the use of polypharmacy may be deemed clinically necessary, it does not appear to increase risks of mortality or higher utilisation. Our findings give support to current guidelines encouraging antipsychotic monotherapy, rather than polypharmacy, in routine clinical practice.
Although it may not be appropriate to incentivise the two non-QOF indicators as metrics in a P4P scheme, the indicators nevertheless provide important information about primary care quality and do support the evidence base on what would constitute good-quality care for this particular patient group.
Implications for practice
Many of the indicators identified in our systematic review relate to aspects of physical care. Many people with SMI have significant needs for physical health care that may be missed or undertreated, leading to avoidable morbidity and disability, and premature mortality. 173 Consideration should therefore be given to the development of a broader set of quality indicators, including those focusing on physical care. Given the increased risks of diabetes, cardiovascular disease and respiratory disease in this population, ongoing primary care for people with SMI could improve health outcomes by focusing on disease prevention through tackling obesity and smoking. 174,175
Similarly, several quality indicators from our systematic review relate to the processes of care, including continuity of care and ongoing contact with relevant services. Targeting comprehensive primary care to people with SMI could promote their engagement with appropriate specialised mental and physical health-care services, helping them to reach their full potential.
Our results suggest that seeing the same family physician over time can improve the physical health of people with SMI. These findings are similar to those in previous studies. 103,176 Higher continuity of GP care may reduce the risk of ACSC admissions through improved management of physical health, by facilitating familiarity, communication, trust and quality of relationship between doctor and patient. 177 Our results also showed that better continuity of care reduced primary care costs.
Current changes in UK general practice mean continuity is likely to decrease rather than improve (particularly with practice closures, recruitment and retention problems, increasing use of locums and formation of practice networks). This suggests that practices should prioritise continuity of care in this patient group, despite the challenges in doing so.
We found that having a CP or AR documented in the previous 12 months was associated with a lower risk of A&E department presentations and SMI and ACSC admissions (by around one-third). Given that there is considerable scope for improvement in completion rates, support for increasing timely completion of CPs and ARs could help to improve achievement rates and reduce variation between regions and practices. Taken together with our findings that these activities are associated with lower health-care costs, this strategy could be a promising approach to reducing health-care costs.
Our finding that, relative to monotherapy, polypharmacy is not significantly associated with higher mortality or unplanned hospital admissions and A&E department presentations, and the relatively low levels of polypharmacy (prevalence rate of 2%), perhaps suggests that, in clinical practice in the UK (outside inpatient and acute care), polypharmacy may not be as large an issue as previously reported. 178 Our results also provide support for the role of antipsychotic therapy in reducing unplanned admissions and A&E department presentations, which may be markers of mental illness relapse. For a shorter overlap period (≥ 14 days), which captured more cross-tapering in the definition of polypharmacy, we observed an increase in the risk of unplanned admission. This could be because patients who changed drugs might have had more unstable disease profiles and suggests a need for close monitoring in the first few weeks of cross-tapering, when the risk of unplanned hospitalisation is higher.
Although the emphasis has in part been moving away from QOF towards local enhanced services,179 the requirement for evidence on quality indicators in primary care remains undiminished, and will be needed to develop future guidance and incentives under local improvement schemes and will inform proposed reviews of QOF in mental health. 180 Any changes in incentive structures for quality indicators to bring about improved outcomes needs to be evidence based.
Recommendations for research (in order of priority)
Better evidence is needed on the impact of primary care quality on a broader set of health outcomes for people with serious mental illness
Most indicators included in our systematic review (see Chapter 2) relate to processes of care, and although highly relevant, especially to patients, it is important to establish whether or not they lead to improved health outcomes. With the exception of mortality (which we examined only in relation to polypharmacy), all our study outcome measures were based on health-care utilisation. We had planned to examine effects on the severity of SMI using the clinician-rated HoNOS outcome measure (see Chapter 6); however, HoNOS is not recorded in primary care and the quality of reporting in secondary care is patchy. We therefore used another measure of health-care utilisation instead: re-entry to secondary care. Although these outcomes are likely to impact on health status and are also important in terms of their implications for the efficient use of NHS resources, they are indirect measures of health outcomes.
To be included in the QOF, quality indicators must be supported by NICE evidence-based clinical guideline recommendations or evidence from systematic reviews. This is why the large majority of indicators we identified are not currently part of the QOF. Better measures of health outcome are essential to determine the effectiveness of current and potential QOF indicators.
Good measures of health outcome for people with SMI are central to the ability to undertake research that goes beyond consideration of process measures, for example measures of social functioning, patient experience and health-related quality of life. Routine recording of quality-of-life measures would enable the cost-effectiveness of incentive policies and other measures of primary care quality to be determined. Looking beyond direct health outcomes to the impact of high-quality care on wider aspects of mental health and well-being would mean including data on other non-health outcomes, such as employment and housing status.
The mechanisms by which primary care affects utilisation of hospital care need to be understood
Although not all hospital care can, or should, be avoided for people with SMI, the prevention of acute episodes of the condition is a key objective of good primary care. Our study assesses ‘real-world’ primary care quality, rather than care provided in accordance with strict protocols in a clinical trial context. Our study data are therefore likely to encompass wide variations in the content and delivery of primary care (e.g. an AR). A better understanding of the nature and quality of CPs and ARs, which might be best gained through qualitative research rather than using observational data, would help understand who receives CPs and ARs, why some patients do not receive incentivised care and how that may affect their utilisation of secondary care. It is unclear which components, or combination of components, of care are most effective in preventing avoidable hospital care. The timing and setting for providing care also needs to be understood. For example, further research to investigate the underlying reasons for re-entry to specialist secondary care services would provide greater insight into the role of primary care in supporting patients to avoid the deterioration of SMI conditions.
A clear conceptual framework would be an essential first step, identifying the potential mechanisms by which aspects of primary care quality impact secondary care use among this specific patient group. Interface issues with care provided in other settings, such as informal care provided by families or friends, or social care, could also be identified within the framework. Hypotheses could then be drawn and tested empirically. If suitable data do not exist – which is highly probable – primary studies may be needed.
Understanding variations in capacity to benefit could help to deliver person-centred care
Our study found that CPs and ARs were associated with significantly lower hazards of A&E department attendances and emergency admissions and, in some cases, with lower costs. However, these are average effects and mask a range of effects at individual level. A better understanding of the variations in outcomes could enable clinicians to target efforts accordingly. For example, capacity to benefit may vary according to each individual’s clinical and sociodemographic characteristics. Equally, certain subgroups may be underserved or face greater barriers to accessing care; evidence on supply side facilitators could be used to improve the delivery of person-centred care.
A better evidence base is needed on what outcome measures and quality indicators are most highly valued by people with serious mental illness, so that efforts can be prioritised according to what matters most to patients
There is a growing literature on what matters to people with SMI and how to better incorporate patient perspectives into outcome measurement in mental health, including the development and application of patient-reported outcome measures and experience measures. However, we need to know which outcome measures and quality indicators matter most to people with SMI, so that efforts to measure these in routine data can be prioritised. Focus groups with service users could provide views on what outcomes matter and various elicitation methods, including qualitative research and discrete choice experiments, can be used to prioritise outcome and quality measures using patient preferences. However, any new indicators should be properly consulted on before implementation. An evidence base on how patients prioritise outcome and quality measures could help to focus resources and efforts towards areas that matter most to patients, for example if people with SMI value having a CP renewed when nothing has changed over time, or whether they care about continuity with the same GP or would more highly value ease of seeing any GP urgently. These preference sets may also vary by different groups (e.g. age, sex or ethnicity) and it would be important to understand better this variation to facilitate a targeted approach to service delivery.
Evidence is needed on how patient outcomes are influenced by the interfaces between health care, social care and informal care
Serious mental illness is a complex set of diseases that typically affects individuals across the life course and in all areas of their lives. Focusing on only one or two parts of the health-care system when examining patient outcomes risks ignoring wider social determinants of health and well-being and increases the chances of drawing spurious inferences about cause and effect.
To the best of our knowledge, our study was the first to cover the full patient care pathway for people with SMI, and this was facilitated by the use of MHMDS, the collection of which is a welcome effort to improve the coverage and quality of data on people with mental health problems. However, details on the type of care provided by different health and social care professionals are sketchy and are reported inconsistently. There is almost no information on informal social support networks (friends and family). Many of these types of care may be substitutes or complements. The inter-relationships between these types of care need to be clarified through further research, so that care can be integrated around the individual. This is likely to require the collection of primary data, as such factors are not recorded in routine care data.
A fuller understanding of resource implications is needed to inform resource allocation
This study has indicated the potential for better-quality primary care to reduce costs in secondary care. However, a more detailed research agenda investigating the mechanisms for identifying, releasing or transferring and reinvesting resources ‘saved’, particularly across sectors, would be welcome. This would provide valuable evidence on which to base decisions about the allocation of resources, as well as informing the integration of care, which is often hampered by financial barriers.
Data coverage and linkage, specifically for mental health data, should be improved to address further research questions
Throughout this report, we have commented on some of the limitations of our analyses that arise from constraints in the nature of the data to which we had access. Improving the scope of routine outcome measurement in mental health services and facilitating linkages from diverse data sources would enhance the ability to address further important research questions. Facilitating researcher access to relevant data sets in a timely way is also a key aspect of enhancing both the quantity and quality of mental health research undertaken.
Acknowledgements
We would particularly like to thank our colleagues María José Aragon, for her contribution to the costing of MHMDS, and Christoph Kronenberg, for his contributions to the systematic review and the early analysis of QOF indicators. We would also like to thank Ruth Helstrip and Kath Devlin for their outstanding project management and administrative support. We are very grateful to all members of our SSC for the valuable contributions and input they made throughout the life of this project and, in particular, for providing comments on an earlier draft of this report. They are David Daniel (chairperson), Jane Dunning, David Osborn, Evan Kontopantelis, Holly Paulsen, Sarah Alderson, Stephen Wright, Lauren Aylott and Ceri Dare. We would also like to thank all staff who participated in a workshop that we held for primary care practitioners in Hull on 19 January 2017, on ‘improving the quality of primary care for people with serious mental illness’ and for their candid feedback about ‘how things really work’.
Contributions of authors
Rowena Jacobs (https://orcid.org/0000-0001-5225-6321) (Professor of Health Economics) was the overall principal investigator of the project; agreed the outline structure of the report; contributed to the design of the various elements of the research project, the analytical approaches and interpretations of the findings; contributed various aspects to the writing of the report; collated and edited contributions; contributed to the systematic review; and approved the final manuscript.
Lauren Aylott (https://orcid.org/0000-0002-2261-9360) (expert by experience) contributed to the study design and research questions, the systematic review, the interpretation of results and the writing of the report; contributed to the design of the various elements of the research project, the analytical approaches and interpretations of the findings; and approved the final manuscript.
Ceri Dare (https://orcid.org/0000-0002-6762-506X) (expert by experience) contributed to the study design and research questions, the systematic review, the interpretation of results and the writing of the report; contributed to the design of the various elements of the research project, the analytical approaches and interpretations of the findings; and approved the final manuscript.
Tim Doran (https://orcid.org/0000-0001-7857-3704) (Professor of Health Policy) provided clinical expertise and contributed to the design of the study, interpretation of results and the writing of the report; contributed to the systematic review; and approved the final manuscript.
Simon Gilbody (https://orcid.org/0000-0002-8236-6983) (Professor of Psychological Medicine and Health Services Research) provided clinical expertise and contributed to the design of the study, interpretation of results and the writing of the report; contributed to the design of the various elements of the research project, the analytical approaches and interpretations of the findings; contributed to the systematic review; and approved the final manuscript.
Maria Goddard (https://orcid.org/0000-0002-1517-7461) (Professor of Health Economics) contributed to the design of the study, interpretation of the results and report writing; contributed to the design of the various elements of the research project, the analytical approaches and interpretations of the findings; contributed to the systematic review; and approved the final manuscript.
Hugh Gravelle (https://orcid.org/0000-0002-7753-4233) (Professor of Health Economics) contributed to the design and methods of analyses, interpretation of results and the writing of the report; contributed to the design of the various elements of the research project, the analytical approaches and interpretations of the findings; and approved the final manuscript.
Nils Gutacker (https://orcid.org/0000-0002-2833-0621) (Senior Research Fellow) was a main analyst; agreed the outline structure of the report; contributed to the design of the various elements of the research project, the analytical approaches and interpretations of the findings; contributed various aspects to the writing of the report; and approved the final manuscript.
Panagiotis Kasteridis (https://orcid.org/0000-0003-1623-4293) (Research Fellow) was a main analyst; agreed the outline structure of the report; produced the analyses for the various chapters; contributed to the design of the various elements of the research project, the analytical approaches and interpretations of the findings; contributed various aspects to the writing of the report; collated and edited contributions; and approved the final manuscript.
Tony Kendrick (https://orcid.org/0000-0003-1618-9381) (Professor of Primary Care) provided clinical expertise and contributed to the design of the study, interpretation of results and the writing of the report; contributed to the design of the various elements of the research project, the analytical approaches and interpretations of the findings; contributed to the systematic review; and approved the final manuscript.
Anne Mason (https://orcid.org/0000-0002-5823-3064) (Senior Research Fellow) contributed to the design of the study, interpretation of the results and report writing; contributed to the design of the various elements of the research project, the analytical approaches and interpretations of the findings; and approved the final manuscript.
Nigel Rice (https://orcid.org/0000-0003-0312-823X) (Professor of Health Economics) contributed to the design and methods of analyses, interpretation of results and the writing of the report; contributed to the design of the various elements of the research project, the analytical approaches and interpretations of the findings; and approved the final manuscript.
Jemimah Ride (https://orcid.org/0000-0002-1820-5499) (Research Fellow) was a main analyst; agreed the outline structure of the report; produced the analyses for the various chapters; contributed to the design of the various elements of the research project, the analytical approaches and interpretations of the findings; contributed various aspects to the writing of the report; collated and edited contributions; and approved the final manuscript.
Najma Siddiqi (https://orcid.org/0000-0003-1794-2152) (Clinical Senior Lecturer in Psychiatry) provided clinical expertise and contributed to the design of the study, interpretation of results and the writing of the report; contributed to the design of the various elements of the research project, the analytical approaches and interpretations of the findings; and approved the final manuscript.
Rachael Williams (https://orcid.org/0000-0001-9007-6292) (Observational Research Manager, CPRD) contributed expertise on CPRD data and the linkages, and contributed to the interpretation of results and the writing of the report; contributed to the design of the various elements of the research project, the analytical approaches and interpretations of the findings; and approved the final manuscript.
Publications
Kronenberg C, Doran T, Goddard M, Kendrick T, Gilbody S, Dare CR, et al. Identifying primary care quality indicators for people with serious mental illness: a systematic review. Br J Gen Pract 2017;67:e519–30.
Ride J, Kasteridis P, Gutacker N, Kronenberg C, Doran T, Mason A, et al. Do care plans and annual reviews of physical health influence unplanned hospital utilisation for people with serious mental illness? Analysis of linked longitudinal primary and secondary healthcare records in England. BMJ Open 2018;8:e023135.
Ride J, Kasteridis P, Gutacker N, Doran T, Rice N, Gravelle H, et al. Impact of family practice continuity of care on unplanned hospital use for people with serious mental illness. Health Serv Res 2019;54:1316–25.
Kasteridis P, Ride J, Gutacker N, Aylott L, Dare C, Doran T, et al. Association between antipsychotic polypharmacy and outcomes for people with serious mental illness in England. Psychiatr Serv 2019;70:650–6.
Ride J, Kasteridis P, Gutacker N, Aragón MJ, Jacobs R. Healthcare costs for people with serious mental illness in England: an analysis of costs across primary care, hospital care, and specialist mental healthcare. Appl Health Econ Health Policy 2020;18:177–88.
In addition, our work has benefited from feedback from participants at the following events:
Quality of Care and Outcomes for People with Serious Mental Illness (SMI) in England: What Can We Learn From Financial Incentives and Policy Initiatives? University of Toronto, Centre for Addiction and Mental Health and the Canadian Centre for Health Economics, Toronto, ON, Canada, 7 October 2016.
Improving the Quality of Primary Care for People with Serious Mental Illness Workshop for Primary Care Practitioners. Hull, UK, 19 January 2017.
Do Care Plans Influence Admissions and Mortality for People with Serious Mental Illness (SMI)? Supportive care, Early Diagnosis and Advanced Disease Research Group Seminar. Hull York Medical School, University of Hull, Hull, UK, 7 February 2017.
Does Pay-for-Performance Influence Outcomes for People with Serious Mental Illness (SMI): Analysis of Linked Longitudinal Primary and Secondary Care Data in England. International Health Policy Conference, London School of Economics, London, UK, 16–19 February 2017.
Do Care Plans Influence Admissions and Mortality for People with Serious Mental Illness (SMI)? A Survival Analysis of Linked Primary and Secondary Care Data in England. Thirteenth Workshop on Costs and Assessment in Psychiatry: Mental Health Policy and Economics, Venice, Italy, 24–26 March 2017.
Does Pay-for-Performance Influence Outcomes for People with Serious Mental Illness? Society for Academic Primary Care, University of Warwick, Coventry, UK, 12–14 July 2017.
Does Pay-for-Performance Influence Outcomes for People with Serious Mental Illness? International Conference on Pharmacoepidemiology & Therapeutic Risk Management, Montreal, QC, Canada, 26–30 August 2017.
Does Better Quality Primary Care Reduce Total Cost of Primary, Community and Secondary Health Care for Patients with Serious Mental Illness? European Health Economics Association Conference, Maastricht, the Netherlands, 11–14 July 2018.
Association Between Incentivized Primary Care and Outcomes for People with Serious Mental Illness in the UK’s Quality and Outcomes Framework: Analysis of Linked Longitudinal Primary and Secondary Care Data. European Health Economics Association Conference, Maastricht, the Netherlands, 11–14 July 2018.
Can Care Plans or Provider Continuity in Primary Care Influence Use of Specialist Mental Health Care for Patients With Serious Mental Illness? Fourth Meeting on Patient Reported Outcomes and Person Centred Care in Mental Health, International Center of Mental Health Policy and Economics, Washington, DC, USA, 21–23 September 2018.
Impact of Family Practice Continuity of Care on Unplanned Hospital Use for People with Serious Mental Illness, Academy Health 2019 Annual Research Meeting, Washington, DC, USA, 2–4 June 2019.
Data-sharing statement
Owing to the agreement in place with CPRD, we are unable to share any data. For further information please contact the corresponding author.
Patient data
This work uses data provided by patients and collected by the NHS as part of their care and support. Using patient data is vital to improve health and care for everyone. There is huge potential to make better use of information from people’s patient records, to understand more about disease, develop new treatments, monitor safety, and plan NHS services. Patient data should be kept safe and secure, to protect everyone’s privacy, and it’s important that there are safeguards to make sure that it is stored and used responsibly. Everyone should be able to find out about how patient data is used. #datasaveslives You can find out more about the background to this citation here: https://understandingpatientdata.org.uk/data-citation.
Disclaimers
This report presents independent research funded by the National Institute for Health Research (NIHR). The views and opinions expressed by authors in this publication are those of the authors and do not necessarily reflect those of the NHS, the NIHR, NETSCC, the HS&DR programme or the Department of Health and Social Care. If there are verbatim quotations included in this publication the views and opinions expressed by the interviewees are those of the interviewees and do not necessarily reflect those of the authors, those of the NHS, the NIHR, NETSCC, the HS&DR programme or the Department of Health and Social Care.
References
- Jacobs R, Gutacker N, Mason A, Goddard M, Gravelle H, Kendrick T, et al. Do higher primary care practice performance scores predict lower rates of emergency admissions for persons with serious mental illness? An analysis of secondary panel data. Health Serv Deliv Res 2015;3. https://doi.org/10.3310/hsdr03160.
- Harrison G, Hopper K, Craig T, Laska E, Siegel C, Wanderling J, et al. Recovery from psychotic illness: a 15- and 25-year international follow-up study. Br J Psychiatry 2001;178:506-17. https://doi.org/10.1192/bjp.178.6.506.
- Ochoa S, Usall J, Cobo J, Labad X, Kulkarni J. Gender differences in schizophrenia and first-episode psychosis: a comprehensive literature review. Schizophr Res Treatment 2012;2012. https://doi.org/10.1155/2012/916198.
- Saha S, Chant D, Welham J, McGrath J. A systematic review of the prevalence of schizophrenia. PLOS Med 2005;2. https://doi.org/10.1371/journal.pmed.0020141.
- Saunders KEA, Goodwin GM. The course of bipolar disorder. Adv Psychiatr Treat 2010;16:318-28. https://doi.org/10.1192/apt.bp.107.004903.
- Andrew A, Knapp M, McCrone P, Parsonage M, Trachtenberg M. Effective Interventions in Schizophrenia: The Economic Case. London: Personal Social Services Research Unit, London School of Economics and Political Science; 2012.
- McCrone PR, Dhanasiri S, Patel A, Knapp M, Lawton-Smith S. Paying the Price: The Cost of Mental Health Care in England to 2026. London: The King’s Fund; 2008.
- Miller BJ, Paschall CB, Svendsen DP. Mortality and medical comorbidity among patients with serious mental illness. Psychiatr Serv 2006;57:1482-7. https://doi.org/10.1176/ps.2006.57.10.1482.
- Wahlbeck K, Westman J, Nordentoft M, Gissler M, Laursen TM. Outcomes of Nordic mental health systems: life expectancy of patients with mental disorders. Br J Psychiatry 2011;199:453-8. https://doi.org/10.1192/bjp.bp.110.085100.
- Brown S, Inskip H, Barraclough B. Causes of the excess mortality of schizophrenia. Br J Psychiatry 2000;177:212-17. https://doi.org/10.1192/bjp.177.3.212.
- Osborn DP, Levy G, Nazareth I, Petersen I, Islam A, King MB. Relative risk of cardiovascular and cancer mortality in people with severe mental illness from the United Kingdom’s General Practice Research Database. Arch Gen Psychiatry 2007;64:242-9. https://doi.org/10.1001/archpsyc.64.2.242.
- Harris EC, Barraclough B. Excess mortality of mental disorder. Br J Psychiatry 1998;173:11-53. https://doi.org/10.1192/bjp.173.1.11.
- Olfson M, Gerhard T, Huang C, Crystal S, Stroup TS. Premature mortality among adults with schizophrenia in the United States. JAMA Psychiatry 2015;72:1172-81. https://doi.org/10.1001/jamapsychiatry.2015.1737.
- Bouza C, López-Cuadrado T, Amate JM. Hospital admissions due to physical disease in people with schizophrenia: a national population-based study. Gen Hosp Psychiatry 2010;32:156-63. https://doi.org/10.1016/j.genhosppsych.2009.11.014.
- Kendrick T. Cardiovascular and respiratory risk factors and symptoms among general practice patients with long-term mental illness. Br J Psychiatry 1996;169:733-9. https://doi.org/10.1192/bjp.169.6.733.
- Liu NH, Daumit GL, Dua T, Aquila R, Charlson F, Cuijpers P, et al. Excess mortality in persons with severe mental disorders: a multilevel intervention framework and priorities for clinical practice, policy and research agendas. World Psychiatry 2017;16:30-4. https://doi.org/10.1002/wps.20384.
- McManus S, Meltzer H, Campion J. Cigarette Smoking and Mental Health in England: Data From the Adult Psychiatric Morbidity Survey 2007. London: National Centre for Social Research; 2010.
- Connolly M, Kelly C. Lifestyle and physical health in schizophrenia. Adv Psychiatr Treat 2005;11:125-32. https://doi.org/10.1192/apt.11.2.125.
- Koskinen J, Löhönen J, Koponen H, Isohanni M, Miettunen J. Prevalence of alcohol use disorders in schizophrenia – a systematic review and meta-analysis. Acta Psychiatr Scand 2009;120:85-96. https://doi.org/10.1111/j.1600-0447.2009.01385.x.
- Murray CJ, Lopez AD. The Global Burden Of Disease: A Comprehensive Assessment of Mortality and Disability from Diseases, Injuries, and Risk Factors in 1990 and Projected to 2020: Summary. Geneva: World Health Organization; 1996.
- Nazareth I, King M, Haines A, Tai SS, Hall G. Care of schizophrenia in general practice. BMJ 1993;307. https://doi.org/10.1136/bmj.307.6909.910.
- Kai J, Crosland A, Drinkwater C. Prevalence of enduring and disabling mental illness in the inner city. Br J Gen Pract 2000;50:992-4.
- Lang FH, Johnstone EC, Murray GD. Service provision for people with schizophrenia. II. Role of the general practitioner. Br J Psychiatry 1997;171:165-8. https://doi.org/10.1192/bjp.171.2.165.
- Reilly S, Planner C, Hann M, Reeves D, Nazareth I, Lester H. The role of primary care in service provision for people with severe mental illness in the United Kingdom. PLOS ONE 2012;7. https://doi.org/10.1371/journal.pone.0036468.
- Lohr KN, Donaldson MS, Harris-Wehling J. Medicare: a strategy for quality assurance, V: quality of care in a changing health care environment. QRB Qual Rev Bull 1992;18:120-6. https://doi.org/10.1016/S0097-5990(16)30518-8.
- Richardson W, Berwick D, Bisgard J, Bristow L, Buck C, Cassel C. Institute of Medicine. Crossing the Quality Chasm: A New Health System for the 21st Century. Washington, DC: National Academy Press; 2001.
- Donabedian A. The quality of care. How can it be assessed?. JAMA 1988;260:1743-8. https://doi.org/10.1001/jama.260.12.1743.
- Heath I, Rubinstein A, Stange KC, van Driel ML. Quality in primary health care: a multidimensional approach to complexity. BMJ 2009;338. https://doi.org/10.1136/bmj.b1242.
- Collings JS. General practice in England today – a reconnaissance. Lancet 1950;255. https://doi.org/10.1016/S0140-6736(50)90473-9.
- Kennedy I. The Report of the Public Inquiry into Children’s Heart Surgery at Bristol Royal Infirmary 1984–1995 2001.
- Smith J. The Shipman Inquiry. First Report. Volume One. Death Disguised 2002.
- Doran T, Roland M. Lessons from major initiatives to improve primary care in the United Kingdom. Health Aff 2010;29:1023-9. https://doi.org/10.1377/hlthaff.2010.0069.
- Roland M. Linking physicians’ pay to the quality of care – a major experiment in the United Kingdom. N Engl J Med 2004;351:1448-54. https://doi.org/10.1056/NEJMhpr041294.
- Gillam SJ, Siriwardena AN, Steel N. Pay-for-performance in the United Kingdom: impact of the Quality and Outcomes Framework: a systematic review. Ann Fam Med 2012;10:461-8. https://doi.org/10.1370/afm.1377.
- World Health Organization (WHO) . Quality Improvement for Mental Health: Mental Health Policy and Service Guidance Package 2003.
- National Institute for Health and Care Excellence (NICE) . Psychosis and Schizophrenia in Adults: Treatment and Managment 2014.
- National Collaborating Centre for Mental Health (NCCMH) . Psychosis and Schizophrenia in Adults 2014.
- National Institute for Health Research . NIHR INVOLVE 2019. www.invo.org.uk/ (accessed 22 July 2019).
- Kronenberg C, Doran T, Goddard M, Kendrick T, Gilbody S, Owen C, et al. Quality indicators for primary care of people with serious mental illness: a systematic review. Brit J Gen Pract 2017;67:e519-30. https://doi.org/10.3399/bjgp17X691721.
- Ride J, Kasteridis P, Gutacker N, Kronenberg, C, Doran T, Mason A, et al. Do care plans and annual reviews of physical health influence unplanned hospital utilisation for people with serious mental illness? Analysis of linked longitudinal primary and secondary healthcare records in England. BMJ Open 2018;8. https://doi.org/10.1136/bmjopen-2018-023135.
- Ride J, Kasteridis P, Gutacker N, Doran T, Rice N, Gravelle H, et al. Impact of family practice continuity of care on unplanned hospital use for people with serious mental illness. Health Serv Res 2019;54:1316-325. https://doi.org/10.1111/1475-6773.13211.
- Kasteridis P, Ride J, Gutacker N, Aylott L, Dare C, Doran T, et al. Association between antipsychotic polypharmacy and outcomes for people with serious mental illness in England. Psychiatr Serv 2019;70:650-6. https://doi.org/10.1176/appi.ps.201800504.
- Gutacker N, Mason AR, Kendrick T, Goddard M, Gravelle H, Gilbody S, et al. Does the Quality and Outcomes Framework reduce psychiatric admissions in people with serious mental illness? A regression analysis. BMJ Open 2015;5. https://doi.org/10.1136/bmjopen-2014-007342.
- Smith P. On the unintended consequences of publishing performance data in the public sector. Int J Public Adm 1995;18:277-310. https://doi.org/10.1080/01900699508525011.
- Doran T, Kontopantelis E, Valderas JM, Campbell S, Roland M, Salisbury C, et al. Effect of financial incentives on incentivised and non-incentivised clinical activities: longitudinal analysis of data from the UK Quality and Outcomes Framework. BMJ 2011;342. https://doi.org/10.1136/bmj.d3590.
- Eggleston K. Multitasking and mixed systems for provider payment. J Health Econ 2005;24:211-23. https://doi.org/10.1016/j.jhealeco.2004.09.001.
- Moher D, Liberati A, Tetzlaff J, Altman DG. PRISMA Group . Preferred reporting items for systematic reviews and meta-analyses: the PRISMA statement. Ann Intern Med 2009;151:264-9. https://doi.org/10.7326/0003-4819-151-4-200908180-00135.
- Stegbauer C, Szecsenyi J, Bramesfeld A. [Studies to evaluate outpatient mental health services: are patients’ priorities considered?]. Psychiatrische Praxis 2017;44:13-20. https://doi.org/10.1055/s-0035-1552680.
- Großimlinghaus I, Falkai P, Gaebel W, Janssen B, Reich-Erkelenz D, Wobrock T, et al. [Developmental process of DGPPN quality indicators.]. Der Nervenarzt 2013;84:350-65. https://doi.org/10.1007/s00115-012-3705-4.
- Agency for Healthcare Research and Quality . AHRQ – Quality Indicators 2016. www.qualityindicators.ahrq.gov/ (accessed 23 May 2017).
- Balshem H, Helfand M, Schünemann HJ, Oxman AD, Kunz R, Brozek J, et al. GRADE guidelines: 3. Rating the quality of evidence. J Clin Epidemiol 2011;64:401-6. https://doi.org/10.1016/j.jclinepi.2010.07.015.
- Parameswaran SG, Spaeth-Rublee B, Pincus HA. Measuring the quality of mental health care: consensus perspectives from selected industrialized countries. Adm Policy Ment Health 2015;42:288-95. https://doi.org/10.1007/s10488-014-0569-x.
- Holden J. An audit of the care of 266 patients with schizophrenia in 16 general practices. Ir J Psychol Med 2014;15:61-3. https://doi.org/10.1017/S0790966700003542.
- Sweeney A, Rose D, Clement S, Jichi F, Jones IR, Burns T, et al. Understanding service user-defined continuity of care and its relationship to health and social measures: a cross-sectional study. BMC Health Serv Res 2012;12. https://doi.org/10.1186/1472-6963-12-145.
- Ware NC, Dickey B, Tugenberg T, McHorney CA. CONNECT: a measure of continuity of care in mental health services. Ment Health Serv Res 2003;5:209-21. https://doi.org/10.1023/a:1026276918081.
- Cerimele JM, Chan YF, Chwastiak LA, Avery M, Katon W, Unützer J. Bipolar disorder in primary care: clinical characteristics of 740 primary care patients with bipolar disorder. Psychiatr Serv 2014;65:1041-6. https://doi.org/10.1176/appi.ps.201300374.
- Swartz L, MacGregor H. Integrating services, marginalizing patients: psychiatric patients and primary health care in South Africa. Transcult Psychiatry 2002;39:155-72. https://doi.org/10.1177/136346150203900202.
- Lester H, Tritter JQ, Sorohan H. Managing crisis: the role of primary care for people with serious mental illness. Fam Med 2004;36:28-34.
- McCullagh M, Morley S, Dodwell D. A systematic, confidential approach to improving community care for patients with non-affective psychosis. Prim Care Psychiatr 2003;8:127-30. https://doi.org/10.1185/135525703125001578.
- Ruud T. Mental health quality and outcome measurement and improvement in Norway. Curr Opin Psychiatry 2009;22:631-5. https://doi.org/10.1097/YCO.0b013e3283330cc4.
- Lader M. Consensus statements on standards of care in schizophrenia. Prim Care Psychiatr 1997;3:145-9.
- Haro JM, Salvador-Carulla L. The SOHO (Schizophrenia Outpatient Health Outcome) study: implications for the treatment of schizophrenia. CNS Drugs 2006;20:293-301. https://doi.org/10.2165/00023210-200620040-00003.
- National Institute for Health and Care Excellence (NICE) . Bipolar Disorder: Assessment and Management 2014.
- Caughey GE, Kalisch Ellett LM, Wong TY. Development of evidence-based Australian medication-related indicators of potentially preventable hospitalisations: a modified RAND appropriateness method. BMJ Open 2014;4. https://doi.org/10.1136/bmjopen-2013-004625.
- Busch AB, Lehman AF, Goldman H, Frank RG. Changes over time and disparities in schizophrenia treatment quality. Med Care 2009;47:199-207. https://doi.org/10.1097/MLR.0b013e31818475b7.
- Young AS, Sullivan G, Burnam MA, Brook RH. Measuring the quality of outpatient treatment for schizophrenia. Arch Gen Psychiatry 1998;55:611-17. https://doi.org/10.1001/archpsyc.55.7.611.
- Nayrouz S, Ploumaki S, Farooq R, Stock D, Lim H. Physical health problems in patients with severe mental illness: relationship between antipsychotic treatment and physical health. Eur Neuropsychopharm 2011;21:S480-S1. https://doi.org/https://doi.org/10.1016/S0924-977X(11)70779-9.
- Mainz J, Hansen AM, Palshof T, Bartels PD. National quality measurement using clinical indicators: the Danish National Indicator Project. J Surg Oncol 2009;99:500-4. https://doi.org/10.1002/jso.21192.
- Rodgers J, Black G, Stobbart A, Foster J. Audit of primary care of people with schizophrenia in general practice in Lothian. Qual Prim Care 2003;11:133-40.
- Pincus HA, Spaeth-Rublee B, Watkins KE. Analysis & commentary: the case for measuring quality in mental health and substance abuse care. Health Aff 2011;30:730-6. https://doi.org/10.1377/hlthaff.2011.0268.
- Highet NJ, McNair BG, Thompson M, Davenport TA, Hickie IB. Experience with treatment services for people with bipolar disorder. Med J Aust 2004;181:S47-51. https://doi.org/10.5694/j.1326-5377.2004.tb06353.x.
- Osborn DP, Nazareth I, Wright CA, King MB. Impact of a nurse-led intervention to improve screening for cardiovascular risk factors in people with severe mental illnesses. Phase-two cluster randomised feasibility trial of community mental health teams. BMC Health Serv Res 2010;10. https://doi.org/10.1186/1472-6963-10-61.
- Yeomans D, Dale K, Beedle K. Systematic computerised cardiovascular health screening for people with severe mental illness. Psychiatr Bull 2014;38:280-4. https://doi.org/10.1192/pb.bp.113.045955.
- Mitchell AJ, Delaffon V, Lord O. Let’s get physical: improving the medical care of people with severe mental illness. Adv Psychiatr Treat 2018;18:216-25. https://doi.org/10.1192/apt.bp.111.009068.
- Roberts L, Roalfe A, Wilson S, Lester H. Physical health care of patients with schizophrenia in primary care: a comparative study. Fam Pract 2007;24:34-40. https://doi.org/10.1093/fampra/cml054.
- Cimo A, Stergiopoulos E, Cheng C, Bonato S, Dewa CS. Effective lifestyle interventions to improve type II diabetes self-management for those with schizophrenia or schizoaffective disorder: a systematic review. BMC Psychiatry 2012;12. https://doi.org/10.1186/1471-244X-12-24.
- Druss BG, Zhao L, Cummings JR, Shim RS, Rust GS, Marcus SC. Mental comorbidity and quality of diabetes care under Medicaid: a 50-state analysis. Med Care 2012;50:428-33. https://doi.org/10.1097/MLR.0b013e318245a528.
- Ryan AM, Doran T. The effect of improving processes of care on patient outcomes: evidence from the United Kingdom’s Quality and Outcomes Framework. Med Care 2012;50:191-9. https://doi.org/10.1097/MLR.0b013e318244e6b5.
- Kahn KL, Tisnado DM, Adams JL, Liu H, Chen W-P, Hu FA, et al. Does ambulatory process of care predict health-related quality of life outcomes for patients with chronic disease?. Health Serv Res 2007;42:63-8. https://doi.org/10.1111/j.1475-6773.2006.00604.x.
- Flodgren G, Eccles MP, Shepperd S, Scott A, Parmelli E, Beyer FR. An overview of reviews evaluating the effectiveness of financial incentives in changing healthcare professional behaviours and patient outcomes. Cochrane Database Syst Rev 2011;7. https://doi.org/10.1002/14651858.CD009255.
- Prentice RL, Gloeckler LA. Regression analysis of grouped survival data with application to breast cancer data. Biometrics 1978;34:57-6. https://doi.org/10.2307/2529588.
- NHS Employers . Revisions to the GMS Contract 2006 07. Delivering Investment in General Practice 2006.
- Jones DR, Macias C, Barreira PJ, Fisher WH, Hargreaves WA, Harding CM. Prevalence, severity, and co-occurrence of chronic physical health problems of persons with serious mental illness. Psychiatr Serv 2004;55:1250-7. https://doi.org/10.1176/appi.ps.55.11.1250.
- Mitchell AJ, Malone D, Doebbeling CC. Quality of medical care for people with and without comorbid mental illness and substance misuse: systematic review of comparative studies. Br J Psychiatry 2009;194:491-9. https://doi.org/10.1192/bjp.bp.107.045732.
- Cook BL, Wayne GF, Kafali EN, Liu Z, Shu C, Flores M. Trends in smoking among adults with mental illness and association between mental health treatment and smoking cessation. JAMA 2014;311:172-82. https://doi.org/10.1001/jama.2013.284985.
- Callaghan RC, Veldhuizen S, Jeysingh T, Orlan C, Graham C, Kakouris G, et al. Patterns of tobacco-related mortality among individuals diagnosed with schizophrenia, bipolar disorder, or depression. J Psychiatr Res 2014;48:102-10. https://doi.org/10.1016/j.jpsychires.2013.09.014.
- De Hert M, Dekker JM, Wood D, Kahl KG, Holt RI, Möller HJ. Cardiovascular disease and diabetes in people with severe mental illness position statement from the European Psychiatric Association (EPA), supported by the European Association for the Study of Diabetes (EASD) and the European Society of Cardiology (ESC). Eur Psychiatry 2009;24:412-24. https://doi.org/10.1016/j.eurpsy.2009.01.005.
- Osborn DP, Wright CA, Levy G, King MB, Deo R, Nazareth I. Relative risk of diabetes, dyslipidaemia, hypertension and the metabolic syndrome in people with severe mental illnesses: systematic review and metaanalysis. BMC Psychiatry 2008;8. https://doi.org/10.1186/1471-244X-8-84.
- Maarsingh OR, Henry Y, van de Ven PM, Deeg DJH. Continuity of care in primary care and association with survival in older people: a 17-year prospective cohort study. Brit J Gen Pract 2016;66. https://doi.org/10.3399/bjgp16X686101.
- Barnes TRE, Paton C. Antipsychotic polypharmacy in schizophrenia. Cns Drugs 2011;25:383-99. https://doi.org/10.2165/11587810-000000000-00000.
- Correll CU, Rummel-Kluge C, Corves C, Kane JM, Leucht S. Antipsychotic combinations vs monotherapy in schizophrenia: a meta-analysis of randomized controlled trials. Schizophr Bull 2009;35:443-57. https://doi.org/10.1093/schbul/sbn018.
- Gur S, Weizman S, Stubbs B, Matalon A, Meyerovitch J, Hermesh H, et al. Mortality, morbidity and medical resources utilization of patients with schizophrenia: a case–control community-based study. Psychiatry Res 2018;260:177-81. https://doi.org/10.1016/j.psychres.2017.11.042.
- NHS England . A&Amp;E Attendances and Emergency Admissions 2013–14 2014. www.england.nhs.uk/statistics/ae-waiting-times-andactivity/weekly-ae-sitreps-2013-14/ (accessed 22 July 2019).
- Booth N. What are the Read Codes?. Health Libr Rev 1994;11:177-82. https://doi.org/10.1046/j.1365-2532.1994.1130177.x.
- Chisholm J. The Read clinical classification. BMJ 1990;300. https://doi.org/10.1136/bmj.300.6732.1092.
- Herrett E, Gallagher AM, Bhaskaran K, Forbes H, Mathur R, van Staa T, et al. Data Resource Profile: Clinical Practice Research Datalink (CPRD). Int J Epidemiol 2015;44:827-36. https://doi.org/10.1093/ije/dyv098.
- Office for National Statistics . 2011 Census Data 2011. www.ons.gov.uk/census/2011census/2011censusdata (accessed March 2020).
- Campbell J, Dedman DJ, Eaton SC, Gallagher AM, Williams TJ. Is the CPRD Gold population comparable to the UK Population?. Pharmacoepidemiol Drug Saf 2013;22:280-1.
- Khan NF, Perera R, Harper S, Rose PW. Adaptation and validation of the Charlson Index for Read/OXMIS coded databases. BMC Fam Pract 2010;11. https://doi.org/10.1186/1471-2296-11-1.
- McLennan D, Barnes H, Noble M, Davies J, Garratt E, Dibben C. The English Indices of Deprivation 2010. London: Department for Communities and Local Government; 2011.
- Chang KC, Soljak M, Lee JT, Woringer M, Johnston D, Khunti K, et al. Coverage of a national cardiovascular risk assessment and management programme (NHS Health Check): Retrospective database study. Prev Med 2015;78:1-8. https://doi.org/10.1016/j.ypmed.2015.05.022.
- Jee SH, Cabana MD. Indices for continuity of care: a systematic review of the literature. Med Care Res Rev 2006;63:158-88. https://doi.org/10.1177/1077558705285294.
- Barker I, Steventon A, Deeny SR. Association between continuity of care in general practice and hospital admissions for ambulatory care sensitive conditions: cross sectional study of routinely collected, person level data. BMJ 2017;356. https://doi.org/10.1136/bmj.j84.
- Lancaster KJ. A new approach to consumer theory. J Polit Econ 1966;74:132-57. https://doi.org/10.1086/259131.
- Mundlak Y. On the pooling of time series and cross section data. Econometrica 1978;46:69-85. https://doi.org/10.2307/1913646.
- Dambha-Miller H, Silarova B, Irving G, Kinmonth AL, Griffin SJ. Patients’ views on interactions with practitioners for type 2 diabetes: a longitudinal qualitative study in primary care over 10 years. Br J Gen Pract 2018;68:e36-e43. https://doi.org/10.3399/bjgp17X693917.
- Freeman G, Hughes J. Continuity of Care and the Patient Experience. London: The King’s Fund; 2010.
- Mitton CR, Adair CE, McDougall GM, Marcoux G. Continuity of care and health care costs among persons with severe mental illness. Psychiatr Serv 2005;56:1070-6. https://doi.org/10.1176/appi.ps.56.9.1070.
- Kendrick T, Burns T, Freeling P. Randomised controlled trial of teaching general practitioners to carry out structured assessments of their long term mentally ill patients. BMJ 1995;311:93-8. https://doi.org/10.1136/bmj.311.6997.93.
- Hoertel N, Limosin F, Leleu H. Poor longitudinal continuity of care is associated with an increased mortality rate among patients with mental disorders: results from the French National Health Insurance Reimbursement Database. Eur Psychiatry 2014;29:358-64. https://doi.org/10.1016/j.eurpsy.2013.12.001.
- Chien CF, Steinwachs DM, Lehman A, Fahey M, Skinner EA. Provider continuity and outcomes of care for persons with schizophrenia. Ment Health Serv Res 2000;2:201-11. https://doi.org/10.1023/A:1010160419561.
- Kontopantelis E, Olier I, Planner C, Reeves D, Ashcroft DM, Gask L, et al. Primary care consultation rates among people with and without severe mental illness: a UK cohort study using the Clinical Practice Research Datalink. BMJ Open 2015;5. https://doi.org/10.1136/bmjopen-2015-008650.
- Hasan A, Falkai P, Wobrock T, Lieberman J, Glenthoj B, Gattaz WF, et al. World Federation of Societies of Biological Psychiatry (WFSBP) guidelines for biological treatment of schizophrenia, part 1: update 2012 on the acute treatment of schizophrenia and the management of treatment resistance. World J Biol Psychiatry 2012;13:318-78. https://doi.org/10.3109/15622975.2012.696143.
- Faries D, Ascher-Svanum H, Zhu B, Correll C, Kane J. Antipsychotic monotherapy and polypharmacy in the naturalistic treatment of schizophrenia with atypical antipsychotics. BMC Psychiatry 2005;5. https://doi.org/10.1186/1471-244X-5-26.
- Paton C, Barnes TR, Cavanagh MR, Taylor D, Lelliott P. POMH-UK project team . High-dose and combination antipsychotic prescribing in acute adult wards in the UK: the challenges posed by p.r.n. prescribing. Br J Psychiatry 2008;192:435-9. https://doi.org/10.1192/bjp.bp.107.042895.
- Hou CL, Ma XR, Zang Y, Jia FJ, Lin YQ, Chiu HF, et al. Antipsychotic polypharmacy and quality of life in patients with schizophrenia treated in primary care in China. Int J Clin Pharmacol Ther 2016;54:36-42. https://doi.org/10.5414/CP202413.
- Misawa F, Shimizu K, Fujii Y, Miyata R, Koshiishi F, Kobayashi M, et al. Is antipsychotic polypharmacy associated with metabolic syndrome even after adjustment for lifestyle effects?: a cross-sectional study. BMC Psychiatry 2011;11. https://doi.org/10.1186/1471-244X-11-118.
- Lochmann van Bennekom MW, Gijsman HJ, Zitman FG. Antipsychotic polypharmacy in psychotic disorders: a critical review of neurobiology, efficacy, tolerability and cost effectiveness. J Psychopharmacol 2013;27:327-36. https://doi.org/10.1177/0269881113477709.
- Fleischhacker WW, Uchida H. Critical review of antipsychotic polypharmacy in the treatment of schizophrenia. Int J Neuropsychopharmacol 2014;17:1083-93. https://doi.org/10.1017/S1461145712000399.
- Stahl SM. Antipsychotic polypharmacy, part 1: therapeutic option or dirty little secret?. J Clin Psychiatry 1999;60:425-6. https://doi.org/10.4088/jcp.v60n0701.
- Baandrup L, Gasse C, Jensen VD, Glenthoj BY, Nordentoft M, Lublin H, et al. Antipsychotic polypharmacy and risk of death from natural causes in patients with schizophrenia: a population-based nested case-control study. J Clin Psychiatry 2010;71:103-8. https://doi.org/10.4088/JCP.08m04818yel.
- Tiihonen J, Suokas JT, Suvisaari JM, Haukka J, Korhonen P. Polypharmacy with antipsychotics, antidepressants, or benzodiazepines and mortality in schizophrenia. Arch Gen Psychiatry 2012;69:476-83. https://doi.org/10.1001/archgenpsychiatry.2011.1532.
- Gilmer TP, Dolder CR, Folsom DP, Mastin W, Jeste DV. Antipsychotic polypharmacy trends among Medicaid beneficiaries with schizophrenia in San Diego County, 1999–2004. Psychiatr Serv 2007;58:1007-10. https://doi.org/10.1176/ps.2007.58.7.1007.
- Kreyenbuhl JA, Valenstein M, McCarthy JF, Ganoczy D, Blow FC. Long-term antipsychotic polypharmacy in the VA health system: patient characteristics and treatment patterns. Psychiatr Serv 2007;58:489-95. https://doi.org/10.1176/ps.2007.58.4.489.
- Velligan DI, Carroll C, Lage MJ, Fairman K. Outcomes of Medicaid beneficiaries with schizophrenia receiving clozapine only or antipsychotic combinations. Psychiatr Serv 2015;66:127-33. https://doi.org/10.1176/appi.ps.201300085.
- Crowley J, Hu M. Covariance analysis of heart transplant survival data. J Am Stat Assoc 1977;72:27-36. https://doi.org/10.1080/01621459.1977.10479903.
- Springate DA, Kontopantelis E, Ashcroft DM, Olier I, Parisi R, Chamapiwa E, et al. ClinicalCodes: an online clinical codes repository to improve the validity and reproducibility of research using electronic medical records. PLOS ONE 2014;9. https://doi.org/10.1371/journal.pone.0099825.
- Windfuhr K, While D, Kapur N, Ashcroft DM, Kontopantelis E, Carr MJ, et al. Suicide risk linked with clinical consultation frequency, psychiatric diagnoses and psychotropic medication prescribing in a national study of primary-care patients. Psychol Med 2016;46:3407-17. https://doi.org/10.1017/S0033291716001823.
- Cox DR. Regression models and life-tables. J R Stat Soc Series B Stat Methodol 1972;34:187-220. https://doi.org/10.1111/j.2517-6161.1972.tb00899.x.
- Kadra G, Stewart R, Shetty H, Jackson RG, Greenwood MA, Roberts A, et al. Extracting antipsychotic polypharmacy data from electronic health records: developing and evaluating a novel process. BMC Psychiatry 2015;15. https://doi.org/10.1186/s12888-015-0557-z.
- Cookson R, Propper C, Asaria M, Raine R. Socio-economic inequalities in health care in England. Fisc Stud 2016;37:371-403. https://doi.org/10.1111/j.1475-5890.2016.12109.
- Ride J, Kasteridis P, Gutacker N, Aragon Aragon MJ, Jacobs R. Healthcare costs for people with serious mental illness in England: an analysis of costs across primary care, hospital care, and specialist mental healthcare. Appl Health Econ Health Policy 2019. https://doi.org/10.1007/s40258-019-00530-2.
- Curtis L. Unit Costs of Health and Social Care 2014. Canterbury: PSSRU, University of Kent; 2014.
- Westcott R. The length of consultations in general practice. J R Coll Gen Pract 1977;27:552-5.
- McCabe R, Khanom H, Bailey P, Priebe S. Shared decision-making in ongoing outpatient psychiatric treatment. Patient Educ Couns 2013;91:326-8. https://doi.org/10.1016/j.pec.2012.12.020.
- Fleury MJ, Imboua A, Aubé D, Farand L, Lambert Y. General practitioners’ management of mental disorders: a rewarding practice with considerable obstacles. BMC Fam Pract 2012;13. https://doi.org/10.1186/1471-2296-13-19.
- Waterreus A, Morgan VA. Treating body, treating mind: the experiences of people with psychotic disorders and their general practitioners – findings from the Australian National Survey of High Impact Psychosis. Aust N Z J Psychiatry 2018;52:561-72. https://doi.org/10.1177/0004867417728806.
- Prescribing and Medicines Team . Prescription Cost Analysis: England 2014 2015.
- Department of Health and Social Care (DHSC) . NHS Reference Costs 2013 to 2014 2014.
- Greenhalgh J, Knight C, Hind D, Beverley C, Walters S. Clinical and cost-effectiveness of electroconvulsive therapy for depressive illness, schizophrenia, catatonia and mania: systematic reviews and economic modelling studies. Health Technol Assess 2005;9. https://doi.org/10.3310/hta9090.
- Joint Formulary Committee . British National Formulary 2013.
- The Health Informatics Service . Electronic Staff Record 2018. www.electronicstaffrecord.nhs.uk/home/ (accessed 11 December 2018).
- Financial Planning, Monitoring and Analysis Team . Hospital and Community Health Services Pay and Price Inflation 2018.
- NHS Digital . Costing Grouper 2018.
- Duan N, Manning WG, Morris CN, Newhouse JP. A comparison of alternative models for the demand for medical care. J Bus Econ Stat 1983;1:115-26. https://doi.org/10.2307/1391852.
- Mihaylova B, Briggs A, O’Hagan A, Thompson SG. Review of statistical methods for analysing healthcare resources and costs. Health Econ 2011;20:897-916. https://doi.org/10.1002/hec.1653.
- Manning WG, Mullahy J. Estimating log models: to transform or not to transform?. J Health Econ 2001;20:461-94. https://doi.org/10.1016/S0167-6296(01)00086-8.
- Pregibon D. Goodness of link tests for generalized linear models. Appl Stat 1980;29:15-4. https://doi.org/10.2307/2346405.
- Cameron AC, Gelbach JB, Miller DL. Bootstrap-based improvements for inference with clustered errors. Rev Econ Stat 2008;90:414-27. https://doi.org/10.1162/rest.90.3.414.
- Information Centre . 2006/07/General/Practice/Workload/Survey 2007.
- Office for National Statistics . Consumer Price Inflation Tables. 2019. www.ons.gov.uk/economy/inflationandpriceindices/datasets/consumerpriceinflation (accessed 17 June 2019).
- NHS Digital . Quality and Outcomes Framework 2019. https://qof.digital.nhs.uk/index.asp (accessed 16 May 2019).
- Baker R, Bankart MJ, Rashid A, Banerjee J, Conroy S, Habiba M, et al. Characteristics of general practices associated with emergency-department attendance rates: a cross-sectional study. BMJ Qual Saf 2011;20:953-8. https://doi.org/10.1136/bmjqs.2010.050864.
- Wong CY, Hall J. Does patients’ experience of general practice affect the use of emergency departments? Evidence from Australia. Health Policy 2018;122:126-33. https://doi.org/10.1016/j.healthpol.2017.11.008.
- Xin H, Kilgore ML, Sen BP, Blackburn J. Can nonurgent emergency department care costs be reduced? Empirical evidence from a U.S. nationally representative sample. J Emerg Med 2015;49:347-54. https://doi.org/10.1016/j.jemermed.2015.01.034.
- Harrison MJ, Dusheiko M, Sutton M, Gravelle H, Doran T, Roland M. Effect of a national primary care pay for performance scheme on emergency hospital admissions for ambulatory care sensitive conditions: controlled longitudinal study. BMJ 2014;349. https://doi.org/10.1136/bmj.g6423.
- Gallego JA, Bonetti J, Zhang J, Kane JM, Correll CU. Prevalence and correlates of antipsychotic polypharmacy: a systematic review and meta-regression of global and regional trends from the 1970s to 2009. Schizophr Res 2012;138:18-2. https://doi.org/10.1016/j.schres.2012.03.018.
- Malandain L, Thibaut F, Grimaldi-Bensouda L, Falissard B, Abenhaim L, Nordon C. CGS study group . Correlates and predictors of antipsychotic drug polypharmacy in real-life settings: results from a nationwide cohort study. Schizophr Res 2018;192:213-18. https://doi.org/10.1016/j.schres.2017.05.015.
- Lelliott P, Paton C, Harrington M, Konsolaki M, Sensky T, Okocha C. The influence of patient variables on polypharmacy and combined high dose of antipsychotic drugs prescribed for in-patients. Psychiatr Bull 2018;26:411-14. https://doi.org/10.1192/pb.26.11.411.
- Connolly A, Taylor D. Factors associated with non evidence-based prescribing of antipsychotics. Ther Adv Psychopharmacol 2014;4:247-56. https://doi.org/10.1177/2045125314540298.
- Marcellusi A, Fabiano G, Viti R, Francesa Morel PC, Nicolò G, Siracusano A, et al. Economic burden of schizophrenia in Italy: a probabilistic cost of illness analysis. BMJ Open 2018;8. https://doi.org/10.1136/bmjopen-2017-018359.
- Das Gupta R, Guest JF. Annual cost of bipolar disorder to UK society. Br J Psychiatry 2002;180:227-33. https://doi.org/10.1192/bjp.180.3.227.
- Goeree R, Farahati F, Burke N, Blackhouse G, O’Reilly D, Pyne J, et al. The economic burden of schizophrenia in Canada in 2004. Curr Med Res Opin 2005;21:2017-28. https://doi.org/10.1185/030079905X75087.
- Behan C, Kennelly B, O’Callaghan E. The economic cost of schizophrenia in Ireland: a cost of illness study. Ir J Psychol Med 2008;25:80-7. https://doi.org/10.1017/S079096670001106X.
- Knapp M, Mangalore R, Simon J. The global costs of schizophrenia. Schizophr Bull 2004;30:279-93. https://doi.org/10.1093/oxfordjournals.schbul.a007078.
- Evers SM, Ament AJ. Costs of schizophrenia in the Netherlands. Schizophr Bull 1995;21:141-53. https://doi.org/10.1093/schbul/21.1.141.
- Hewlett E, Moran V. Making Mental Health Count: The Social and Economic Costs of Neglecting Mental Health Care. Paris, France: Organisation for Economic Co-operation Development; 2014.
- Young AH, Rigney U, Shaw S, Emmas C, Thompson JM. Annual cost of managing bipolar disorder to the UK healthcare system. J Affect Disord 2011;133:450-6. https://doi.org/10.1016/j.jad.2011.06.016.
- Wolff J, McCrone P, Koeser L, Normann C, Patel A. Cost drivers of inpatient mental health care: a systematic review. Epidemiol Psychiatr Sci 2015;24:78-89. https://doi.org/10.1017/S204579601300067X.
- Senese F, Rucci P, Fantini MP, Gibertoni D, Semrov E, Nassisi M, et al. Measuring costs of community mental health care in Italy: a prevalence-based study. Eur Psychiatry 2018;51:34-41. https://doi.org/10.1016/j.eurpsy.2018.02.001.
- de Oliveira C, Cheng J, Vigod S, Rehm J, Kurdyak P. Patients with high mental health costs incur over 30 percent more costs than other high-cost patients. Health Aff 2016;35:36-43. https://doi.org/10.1377/hlthaff.2015.0278.
- Gallagher A, Puri S, van Staa T. Linkage of the General Practice Research Database (GPRD) with other data sources. Pharmacoepidemiol Drug Saf 2011;20:230-1.
- Vinogradova Y, Coupland C, Hippisley-Cox J, Whyte S, Penny C. Effects of severe mental illness on survival of people with diabetes. Br J Psychiatry 2010;197:272-7. https://doi.org/10.1192/bjp.bp.109.074674.
- Dickerson FB, Brown CH, Kreyenbuhl JA, Fang L, Goldberg RW, Wohlheiter K, et al. Obesity among individuals with serious mental illness. Acta Psychiatr Scand 2006;113:306-13. https://doi.org/10.1111/j.1600-0447.2005.00637.x.
- Banham L, Gilbody S. Smoking cessation in severe mental illness: what works?. Addiction 2010;105:1176-89. https://doi.org/10.1111/j.1360-0443.2010.02946.x.
- van Loenen T, van den Berg MJ, Westert GP, Faber MJ. Organizational aspects of primary care related to avoidable hospitalization: a systematic review. Fam Pract 2014;31:502-16. https://doi.org/10.1093/fampra/cmu053.
- Tammes P, Purdy S, Salisbury C, MacKichan F, Lasserson D, Morris RW. Continuity of primary care and emergency hospital admissions among older patients in England. Ann Fam Med 2017;15:515-22. https://doi.org/10.1370/afm.2136.
- Kadra G, Stewart R, Shetty H, MacCabe JH, Chang CK, Taylor D, et al. Long-term antipsychotic polypharmacy prescribing in secondary mental health care and the risk of mortality. Acta Psychiatr Scand 2018;138:123-32. https://doi.org/10.1111/acps.12906.
- NHS Commissioning Board . Enhanced Services Commissioning Fact Sheet 2012.
- NHS England . GP Contract 2019. www.england.nhs.uk/gp/gpfv/investment/gp-contract/ (accessed 17 June 2019).
- Bardsley M, Blunt I, Davies S, Dixon J. Is secondary preventive care improving? Observational study of 10-year trends in emergency admissions for conditions amenable to ambulatory care. BMJ Open 2013;3. https://doi.org/10.1136/bmjopen-2012-002007.
- Noordzij M, Leffondré K, van Stralen KJ, Zoccali C, Dekker FW, Jager KJ. When do we need competing risks methods for survival analysis in nephrology?. Nephrol Dial Transplant 2013;28:2670-7. https://doi.org/10.1093/ndt/gft355.
Appendix 1 Systematic literature review of primary care quality
Searched: February 2015
Date range: January 1990 to February 2015
-
serious mental illness*.tw. (2037)
-
serious mental disorder*.tw. (260)
-
serious psychiatric illness*.tw. (61)
-
serious psychiatric ill-health*.tw. (0)
-
serious mental ill-health*.tw. (0)
-
serious psychiatric disorder*.tw. (130)
-
severe mental illness*.tw. (2679)
-
severe mental disorder*.tw. (720)
-
severe mental ill-health*.tw. (2)
-
severe psychiatric illness*.tw. (128)
-
severe psychiatric disorder*.tw. (379)
-
severe psychiatric ill-health*.tw. (0)
-
major mental disorder*.tw. (288)
-
major mental illness*.tw. (350)
-
major psychiatric illness*.tw. (151)
-
major psychiatric ill-health*.tw. (0)
-
major psychiatric disorder*.tw. (730)
-
major mental ill-health*.tw. (0)
-
schizophrenia/or schizophrenia, catatonic/or schizophrenia, disorganized/or schizophrenia, paranoid/or shared paranoid disorder/ (86,432)
-
(Schizophrenia* or schizophrenic or dementia praecox).tw. (90,771)
-
Schizotypal Personality Disorder/ (2217)
-
(disorder* adj2 schizotypal).tw. (702)
-
(disorder* adj1 delusional).tw. (703)
-
Psychotic Disorders/ (32,708)
-
((psychotic adj2 disorder*) or (schizoaffective adj2 disorder*) or psychoses or psychosis or schizophreniform).tw. (38,127)
-
bipolar disorder/or cyclothymic disorder/ (32,171)
-
(Bipolar adj2 (disorder* or depression or depressive or psychosis or psychoses)).tw. (22,038)
-
(Manic state* or mania).tw. (8053)
-
(Manic adj2 (disorder* or depression or depressive or psychosis or psychoses)).tw. (4445)
-
(cyclothymic disorder* or cyclothymic personalities or cyclothymic personality).tw. (95)
-
or/1-30 (179,930)
Line 31 captures terms for serious mental illness.
-
exp Primary Healthcare/ (82,203)
-
general practitioners/or physicians, family/or physicians, primary care/ (18,403)
-
general practice/or family practice/ (64,455)
-
(family adj2 pract*).tw. (11,764)
-
(primary adj2 care).tw. (89,376)
-
(general adj2 pract*).tw. (69,034)
-
(family adj2 physician*).tw. (12,969)
-
Ambulatory Care/ (36,401)
-
or/32-39 (268,786)
Line 40 captures terms for primary care.
-
Quality Indicators, Healthcare/ (10,737)
-
(quality adj2 indicat*).tw. (6747)
-
(quality adj2 measure*).tw. (12,491)
-
(quality adj2 criteria).tw. (3829)
-
(performance adj2 indicat*).tw. (4837)
-
(performance adj2 measure*).tw. (14,194)
-
(performance adj2 criteria).tw. (1367)
-
(incentive* adj3 scheme*).tw. (207)
-
(incentive* adj3 assess*).tw. (96)
-
(incentive* adj3 measure*).tw. (152)
-
(incentive* adj3 outcome*).tw. (96)
-
“Standard of Care”/ (1049)
-
(standard* adj2 care).tw. (25,676)
-
(standard* adj2 healthcare).tw. (400)
-
“Quality of Healthcare”/ (58,460)
-
(quality adj2 (healthcare or care)).tw. (39,007)
-
patient outcome assessment/ (934)
-
(patient adj2 outcome assessment*).tw. (70)
-
(patient adj2 outcome measure*).tw. (2492)
-
proms.tw. (263)
-
patient satisfaction/or patient preference/ (63,756)
-
(patient* adj2 satisfaction).tw. (26,024)
-
(patient* adj2 experience*).tw. (59,692)
-
(patient* adj2 preference*).tw. (8103)
-
quality.tw. (594,390)
-
or/41-65 (782,974)
Line 66 captures terms for quality indicators.
-
31 and 40 and 66 (551)
Line 67 identifies records that contain at least one term for serious mental illness, and at least one term for primary care and at least one term for quality indicators.
-
limit 67 to yr=‘1990 -Current’ (537)
Line 68 applies the date limit.
Appendix 2 Study framework
Category | Read codes used to identify diagnostic category |
---|---|
Schizophrenia and other psychoses | E100.00 E100.11 E100000 E100100 E100200 E100300 E100400 E100500 E100z00 E101.00 E101000 E101400 E101500 E101z00 E102.00 E102000 E102100 E102500 E102z00 E103.00 E103000 E103200 E103300 E103400 E103500 E103z00 E104.00 E105.00 E105000 E105200 E105500 E105z00 E106.00 E107.00 E107.11 E107000 E107100 E107200 E107300 E107400 E107500 E107z00 E10y.00 E10y.11 E10y000 E10y100 E10yz00 E10z.00 E120.00 E121.00 E122.00 E123.00 E123.11 E12y.00 E12y000 E12yz00 E12z.00 E13..00 E13..11 E131.00 E132.00 E133.00 E133.11 E134.00 E13y.00 E13y100 E13yz00 E13z.00 E13z.11 E1z..00 E212200 Eu20.00 Eu20000 Eu20011 Eu20100 Eu20111 Eu20200 Eu20211 Eu20212 Eu20213 Eu20214 Eu20300 Eu20311 Eu20400 Eu20500 Eu20511 Eu20600 Eu20y00 Eu20y12 Eu20y13 Eu20z00 Eu21.00 Eu21.11 Eu21.12 Eu21.13 Eu21.14 Eu21.15 Eu21.16 Eu21.17 Eu21.18 Eu22.00 Eu22000 Eu22011 Eu22012 Eu22013 Eu22014 Eu22015 Eu22100 Eu22111 Eu22200 Eu22300 Eu22y00 Eu22y11 Eu22y12 Eu22y13 Eu22z00 Eu23.00 Eu23000 Eu23011 Eu23012 Eu23100 Eu23112 Eu23200 Eu23211 Eu23212 Eu23214 Eu23300 Eu23312 Eu23y00 Eu23z00 Eu23z11 Eu23z12 Eu24.00 Eu24.12 Eu24.13 Eu25.00 Eu25000 Eu25011 Eu25012 Eu25100 Eu25111 Eu25112 Eu25200 Eu25212 Eu25y00 Eu25z00 Eu25z11 Eu26.00 Eu2y.00 Eu2y.11 Eu2z.00 Eu2z.11 Eu44.14 |
Bipolar disorder and affective psychoses | E11..00 E11..12 E110.00 E110.11 E110000 E110100 E110200 E110300 E110400 E110600 E110z00 E111.00 E111000 E111100 E111200 E111300 E111400 E111500 E111600 E111z00 E112400 E113400 E114.00 E114.11 E114000 E114100 E114200 E114300 E114400 E114500 E114600 E114z00 E115.00 E115.11 E115000 E115100 E115200 E115300 E115400 E115500 E115600 E115z00 E116.00 E116000 E116100 E116200 E116300 E116400 E116500 E116600 E116z00 E117.00 E117000 E117100 E117200 E117300 E117400 E117500 E117600 E117z00 E11y.00 E11y000 E11y100 E11y300 E11yz00 E11z.00 E11z000 E11zz00 E130.00 E130.11 E13y000 Eu30.00 Eu30.11 Eu30000 Eu30100 Eu30200 Eu30211 Eu30212 Eu30y00 Eu30z00 Eu30z11 Eu31.00 Eu31.11 Eu31.12 Eu31.13 Eu31000 Eu31100 Eu31200 Eu31300 Eu31400 Eu31500 Eu31600 Eu31700 Eu31800 Eu31900 Eu31911 Eu31y00 Eu31y11 Eu31y12 Eu31z00 Eu32300 Eu32311 Eu32312 Eu32313 Eu32314 Eu32800 Eu33213 Eu33300 Eu33311 Eu33312 Eu33313 Eu33314 Eu33315 Eu33316 Eu3z.11 |
Condition | ICD-10 code(s) used to identify the conditionb |
---|---|
SMI admission codes | |
SMI | F20 F21 F22 F23 F24 F25 F26 F27 F28 F29 F30 F31 |
ACSC admission codes | |
Angina | I10 I24.0 I24.8 I24.9 |
Asthma | J45 J46 |
Chronic obstructive pulmonary disease |
J41 J42 J43 J44 J47 First diagnosis J20 + second diagnosis, one of J41 J42 J43 J44 J47 |
Congestive heart failure | I50 I11.0 J81 |
Diabetes (in any diagnosis field) | E10.0 E10.1 E10.2 E10.3 E10.4 E10.5 E10.6 E10.7 E10.8 E11.0 E10.1 E11.2 E10.3 E11.4 E10.5 E11.6 E10.7 E11.8 E12.0 E10.1 E12.2 E10.3 E12.4 E10.5 E12.6 E10.7 E12.8 E13.0 E10.1 E13.2 E10.3 E13.4 E10.5 E13.6 E10.7 E13.8 E14.0 E10.1 E14.2 E10.3 E14.4 E10.5 E14.6 E10.7 E14.8 |
Epilepsy | G40 G41 O15 R56 |
Hypertension | I10 I11.9 |
Anaemia | D50.0 D50.8 D50.9 |
Cellulitis | L03 L04 L08 L88 L98.0 L98.3 |
Dehydration | E86 |
Dental | A69.0 K02 K03 K04 K05 K06 K08 K09.8 K09.9 K12 K13 |
Ear, nose and throat infections | H66 H67 J02 J03 J06 J31.2 |
Gangrene (in any diagnosis field) | R02 |
Gastroenteritis | K52.2 K52.8 K52.9 |
Nutritional deficiencies | E40 E41 E42 E43 E55 E64.3 |
Perforated or bleeding ulcer | K25.0 K25.1 K25.2 K25.4 K25.5 K25.6 K26.0 K26.1 K26.2 K26.4 K26.5 K26.6 K27.0 K27.1 K27.2 K27.4 K27.5 K27.6 K28.0 K28.1 K28.2 K28.4 K28.5 K28.6 |
Urinary tract infection or pyelonephritis | N10 N11 N12 N13.6 N39.0 |
Influenza (in any diagnosis field, exclude secondary diagnosis of D57) | J10 J11 |
Pneumonia (in any diagnosis field, exclude secondary diagnosis of D57) | J13 J14 J15.3 J15.4 J15.7 J15.9 J16.8 J18.1 J18.8 |
Tuberculosis | A15 A16 A19 |
Other vaccine-preventable diseases (in any diagnosis field) | A35 A36 A37 A80 B05 B06 B16.1 B16.9 B18.0 B18.1 B26 G00.0 M01.4 |
Item | Item number | Recommendation | Page number |
---|---|---|---|
Title and abstract | 1 | (a) Indicate the study’s design with a commonly used term in the title or the abstract | v |
(b) Provide in the abstract an informative and balanced summary of what was done and what was found | xxiii–xxvii | ||
Introduction | |||
Background/rationale | 2 | Explain the scientific background and rationale for the investigation being reported | 1–3 |
Objectives | 3 | State specific objectives, including any prespecified hypotheses | 4 |
Methods | |||
Study design | 4 | Present key elements of study design early in the paper | 17 |
Setting | 5 | Describe the setting, locations and relevant dates, including periods of recruitment, exposure, follow-up and data collection | 24–27 |
Participants | 6 | (a) Give the eligibility criteria and the sources and methods of selection of participants. Describe methods of follow-up | 24–27 |
(b) For matched studies, give matching criteria and number of exposed and unexposed | |||
Variables | 7 | Clearly define all outcomes, exposures, predictors, potential confounders and effect modifiers. Give diagnostic criteria, if applicable | 19–21, 27–28 |
Data sources/measurement | 8a | For each variable of interest, give sources of data and details of methods of assessment (measurement). Describe comparability of assessment methods if there is more than one group | 21–24 |
Bias | 9 | Describe any efforts to address potential sources of bias | 17 |
Study size | 10 | Explain how the study size was arrived at | 26 |
Quantitative variables | 11 | Explain how quantitative variables were handled in the analyses. If applicable, describe which groupings were chosen and why | 28 |
Statistical methods | 12 | (a) Describe all statistical methods, including those used to control for confounding | 18, 31–32, 44, 52, 66–67 |
(b) Describe any methods used to examine subgroups and interactions | 27 | ||
(c) Explain how missing data were addressed | 27, 44, 64–66, 121 | ||
(d) If applicable, explain how loss to follow-up was addressed | |||
(e) Describe any sensitivity analyses | 32, 45, 67 | ||
Results | |||
Participants | 13a | (a) Report numbers of individuals at each stage of study (e.g. numbers potentially eligible, examined for eligibility, confirmed eligible, included in the study, completing follow-up and analysed) | 26 |
(b) Give reasons for non-participation at each stage | 26 | ||
(c) Consider use of a flow diagram | 26 | ||
Descriptive data | 14a | (a) Give characteristics of study participants (e.g. demographic, clinical, social) and information on exposures and potential confounders | 33, 34, 45, 53, 54, 60, 61 |
(b) Indicate number of participants with missing data for each variable of interest | |||
(c) Summarise follow-up time (e.g. average and total amount) | 33, 34, 45, 53, 54 | ||
Outcome data | 15a | Report numbers of outcome events or summary measures over time | 33, 45, 53, 54, 67–69 |
Main results | 16 | (a) Give unadjusted estimates and, if applicable, confounder-adjusted estimates and their precision (e.g. 95% CI). Make clear which confounders were adjusted for and why they were included | 35, 46, 47, 54, 70–72 |
(b) Report category boundaries when continuous variables were categorised | |||
(c) If relevant, consider translating estimates of relative risk into absolute risk for a meaningful time period | 79 | ||
Other analyses | 17 | Report other analyses done (e.g. analyses of subgroups and interactions, and sensitivity analyses) | 39, 48, 75 |
Discussion | |||
Key results | 18 | Summarise key results with reference to study objectives | 79–81 |
Limitations | 19 | Discuss limitations of the study, taking into account sources of potential bias or imprecision. Discuss both direction and magnitude of any potential bias | 82–84 |
Interpretation | 20 | Give a cautious overall interpretation of results, considering objectives, limitations, multiplicity of analyses, results from similar studies and other relevant evidence | 85, 86 |
Generalisability | 21 | Discuss the generalisability (external validity) of the study results | 84 |
Other information | |||
Funding | 22 | Give the source of funding and the role of the funders for the present study and, if applicable, for the original study on which the present article is based | iii |
Appendix 3 The association between primary care quality and hospital care utilisation
Practice-level analysis
Overview
The practice-level analysis investigated whether or not better quality of primary care is associated with unplanned hospital admissions. It was an extension of our previous project1 and a precursor of the patient-level analysis in the current project.
Quality of primary care was captured by the practice performance on the CP and AR indicators discussed in Chapter 3, Quality and Outcomes Framework indicators. Although the patient-level analysis utilised patient-level information on the two indicators, in the practice-level analysis CPs and ARs were analysed at the practice level using two measures of achievement on these indicators: (1) the population achievement rate and (2) the reported achievement rate, which we define in the next subsection. Our two research questions were formulated as follows:
-
What is the association between the CP population and reported achievement rates with emergency hospital admissions for SMI?
The analysis was carried out for the period 2006/7–2013/14.
-
What is the association between the AR reported achievement and emergency hospital admissions for ACSCs?
The analysis was performed for the period 2011/12–2013/14. The impact of the AR indicator on all physical hospital admissions during the period 2006/7–2010/11 was assessed in our previous SMI project. For reasons explained in the next subsection, the population achievement rate was not analysed.
In a previous study,1 we conducted a practice-level analysis to investigate the association between achievement rates on the CP and AR indicators and hospital admissions for SMI and physical conditions for the period of 2006/7 to 2010/11. The current practice-level analysis differs in two ways: first, we analysed ACSCs instead of all physical conditions; second, we extended the study period to include 3 more years (2011/12–2013/14).
We combined patient- and general practice-level data for the study period of April 2006 to March 2014. Specifically, we merged QOF data from around 8500 general practices in England with admissions data from HES (aggregated to practice level) for the study period of April 2006 to March 2014, using a unique general practice identifier. We linked these data to publicly available information on general practice characteristics, characteristics of their patient population, such as disease prevalence, and population characteristics, such as deprivation and other potential confounders recorded at small area level. We also controlled for measures of access to care.
Practice performance
The ‘population achievement’ rate is defined as the percentage of patients in the relevant (CP or AR) register for which the indicator was achieved. Practices can ‘exception report’ patients from the indicators according to valid exception reporting criteria, such as the patient is deemed unsuitable for treatment, is newly registered with the practice or newly diagnosed, or that the patient exercises informed dissent. The ‘reported achievement’ rate removes exception-reported patients from the achievement calculation. In this study, both measures were used to ascertain whether or not results are sensitive to the choice of achievement measure.
As explained in Chapter 3, The annual review indicator, the AR indicator was split into six subdomains after 2010/11. For each year in the period of 2011/12 to 2013/14, we calculated the AR achievement rate by weighting the achievement rate on each of the six subdomains by the associated QOF points. For instance, the AR achievement rate for 2011/12 is given by:
in which MH11–MH16 denote the achievement rates on the subdomains, the maximum of points a practice receives on an indicator is 4 or 5 and the total maximum points that can be received is 27.
For each general practice, we obtained information on the population and reported achievement rates from the QOF data set.
As shown in Figure 7, the average annual achievement rates for the CP indicator initially increased over time, but reached a plateau in 2009/10. The average population(-reported) achievement rate increased from 64% (76%) in 2006/7 to about 81% (89%) in 2009/10 and remained at about the same level until 2012/13. The drop in population achievement in 2013/14 was due to changes in the definition of excepted reported patients. Owing to this inconsistency in definition, we restricted our analysis to the 2006/7–2012/13 period, with respect to population achievement.
FIGURE 7.
Achievement on CP indicator.
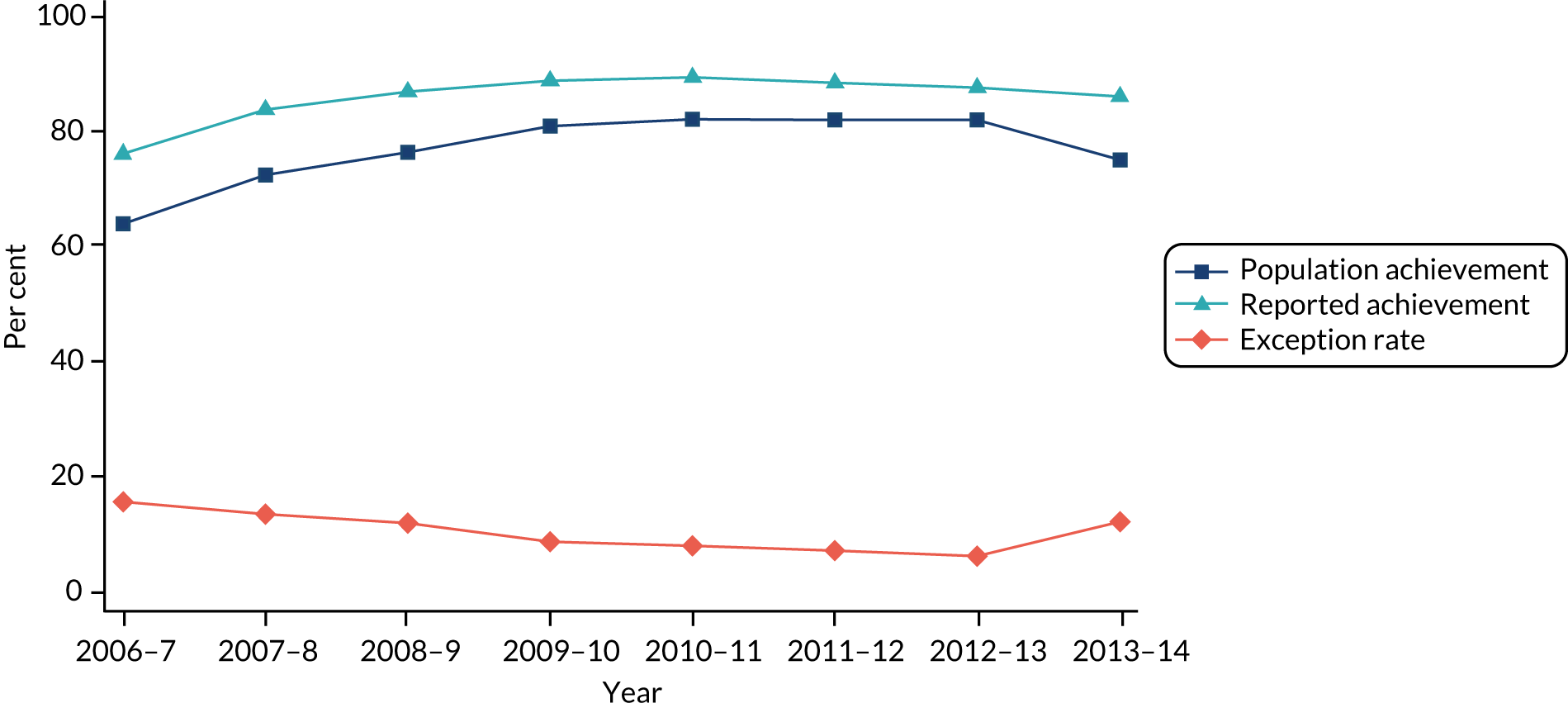
The average reported achievement rate for the AR indicator was flat through our study period (2011/12–2013/14). We did not consider the AR population achievement rate in the analysis, as the definition of exception-reported patients changed in year 2012/13, making the AR population achievement rate inconsistent across years.
Sample and outcomes
We extracted information on all NHS-funded inpatient activity for people aged ≥ 18 years who were diagnosed with SMI. To identify people who had been diagnosed with SMI, we searched all primary and secondary diagnosis fields in HES since April 2001 for the following ICD-10 diagnosis codes: F20–F25, F28 and F29, covering schizophrenia, schizotypal and delusional disorders, and F30 and F31, covering bipolar and mood affective disorders.
To capture all relevant activity, we linked patient records across time and identified all secondary care provided to the patient from April 2006 onwards. SMI admissions were defined as when the patient had a primary diagnosis of SMI (see ICD-10 codes above) in the first episode of the inpatient spell. Admissions for ACSC conditions were defined by specific ICD-10 codes. 181
Covariates
We controlled for a number of GP and practice characteristics, local population characteristics and measures of access to secondary care. To reflect differences between practices and regions in terms of supply and access to care, we recorded the catchment population prevalence of NHS community psychiatric residential beds and the catchment population providing informal care.
Finally, we used primary care trust-level fixed effects to capture all time-invariant differences between primary care trusts in terms of their resource capabilities, including the availability of crisis resolution and home treatment teams that provide alternative home care in an emergency and play a ‘gatekeeping’ role in admissions to hospital.
Table 32 summarises all dependent and independent variables in the SMI and ACSC samples. Further details about the covariates used can be found in our previous project. 1
Variable description | Source | SMI sample (2006/7–2013/14) | ACSC sample (2011/12–2013/14) | ||
---|---|---|---|---|---|
n | Mean | n | Mean | ||
Number of SMI admissions within a year | HES | 62,882 | 3.63 | ||
Population achievement (CP)a | QOF | 55,200 | 77.41 | ||
Reported achievement (CP) | QOF | 62,882 | 86.21 | ||
Number of ACSC admissions within a year | HES | 23,111 | 0.56 | ||
Population achievement (AR) | QOF | 23,111 | 74.83 | ||
Reported achievement (AR) | QOF | 23,111 | 88.35 | ||
Proportion of male GPs in general practice | GMS | 62,882 | 0.59 | 23,111 | 0.56 |
Proportion of foreign GPs in general practice | GMS | 62,882 | 0.32 | 23,111 | 0.32 |
Mean age (years) of GPs in general practice | GMS | 62,882 | 47.81 | 23,111 | 47.55 |
General practice is contracted under PMS | GMS | 62,882 | 0.42 | 23,111 | 0.41 |
General practice is alternative provider of medical services | GMS | 62,882 | 0.01 | 23,111 | 0.02 |
Practice list size | ADS | 62,882 | 6878 | 23,111 | 7135 |
Patient population: average age (years) | ADS | 62,882 | 39.96 | 23,111 | 40.26 |
Patient population: proportion of male patients | ADS | 62,882 | 0.50 | 23,111 | 0.5 |
IMD, income domain, 2010 | ONS | 62,882 | 0.16 | 23,111 | 0.16 |
Proportion of non-white, PCA | ONS | 62,882 | 0.11 | 23,111 | 0.11 |
NHS psychiatric residents/1000, PCA | ONS | 62,882 | 0.21 | 23,111 | 0.21 |
Proportion living in urban setting, PCA | ONS | 62,882 | 0.82 | 23,111 | 0.82 |
Proportion providing informal care, PCA | ONS | 62,882 | 0.10 | 23,111 | 0.1 |
Distance (miles) to closest acute hospital | HES | 62,882 | 4.89 | 23,111 | 4.89 |
Distance (miles) to closest mental health hospital | HES | 62,882 | 9.65 | 23,111 | 9.65 |
Model specification
We estimated panel data models to assess the impact of primary care quality on hospital admissions. As the response variable – number of admissions to hospital per practice per year – is a non-negative integer, we employed count data models. Specifically, we estimated both fixed- and random-effects Poisson models.
As the number of patients at risk of admission differed across practices, a crucial part of the model specification is to define the proper exposure variable (i.e. the pool of practice patients at risk of hospital admission). This is defined as the CP register (or the AR register) and enters the set of explanatory variables logarithmically.
We calculated robust SEs for the fixed-effects model and bootstrapped SEs for the random-effects model. Statistical significance was always assessed at the 5%, 1% and 0.1% levels. All models are estimated using the xtpoisson and poisson commands in Stata.
Results
We analysed the association between CPs and emergency SMI admissions and the association between ARs and emergency ACSC admissions using two separate samples. The two samples differ in the number of participating practices because practices were excluded from the samples if the number of patients on the register was below five, and these numbers are different for the CP and AR register.
The SMI sample consists of 8250 general practices that have reported treating patients with SMI during the 8-year study period. The panel is unbalanced, with only 7267 (88%) of the practices contributing observations every year. The reason for the unbalance is that for some years, some practices (1) do not report or participate in the QOF, (2) have fewer than five patients with SMI on their patient register, (3) their overall list size is < 1000 patients, or (4) are yet to be established or have ceased to exist. In total, there are 62,882 practice-year observations. The ACSC sample consists of 7856 general practices during the 3-year study period and about 96% of them contribute observations every year. In total, there were 23,111 practice-year observations.
Figure 8 shows the average annual admission rates for the SMI and ACSC samples. Despite a slight increase in the average number of SMI admissions per general practice per year (from 3.41 to 3.50) during the period 2006–13, the SMI admission rates exhibited a negative trend, falling from 8.18% in 2006 to 6.51% in 2013. The reason is that the CP register – the denominator in the admission rate – increased over the same period from 44 to 55 patients. During the period 2011–13, the ACSC admission rates exhibit a positive trend, increasing from 0.89% in 2011 to 1.07% in 2013.
FIGURE 8.
Average annual admission rates for SMI and ACSC.
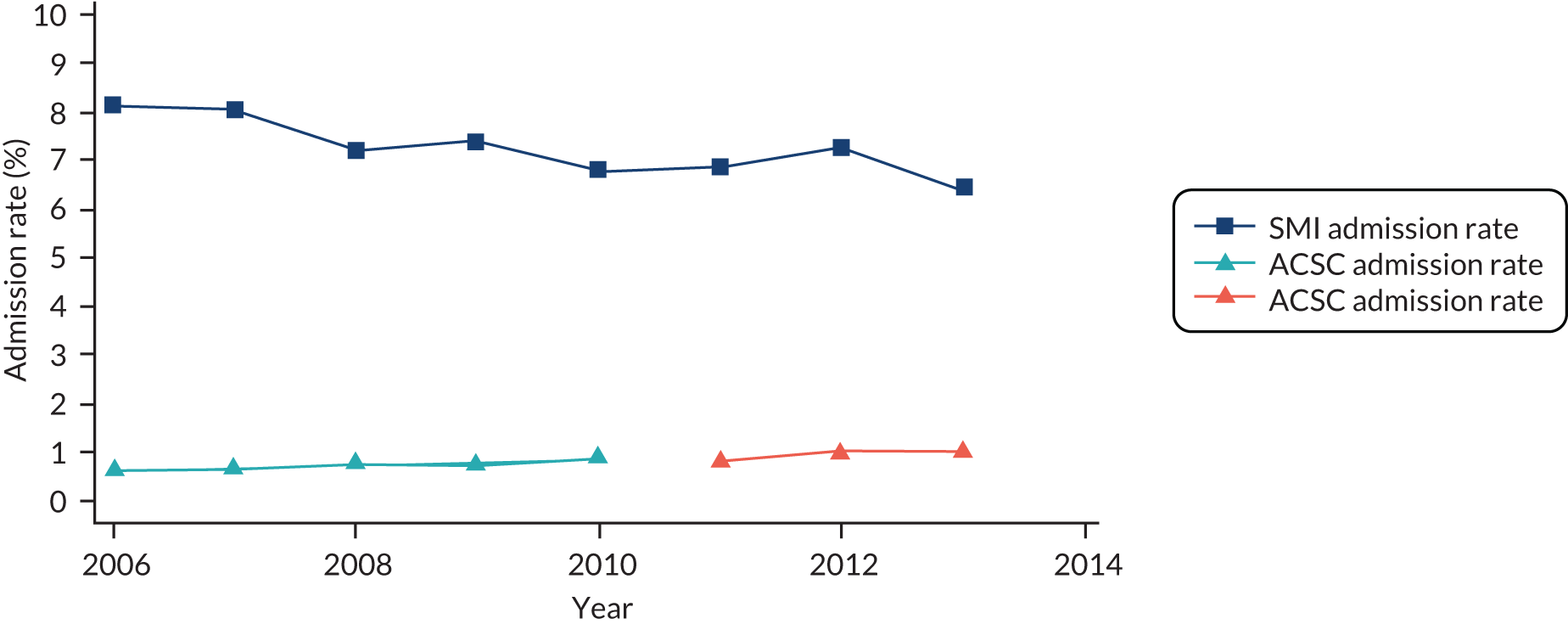
Table 33 presents the effects of a 10 percentage point change in the QOF achievement rates on (1) the number of hospital admissions (see Table 33, column 4) and (2) the percentage change in admissions (see Table 33, column 6).
Effect | Model | n | Absolute change (number of admissions) | 95% CI | Relative change, % | 95% CI |
---|---|---|---|---|---|---|
Effect of CP on SMI admissions | ||||||
Reported achievement | RE | 62,882 | –0.002 | –0.016 to 0.013 | –0.04 | –0.45 to 0.36 |
FEa | 62,437 | 0.003 | –0.021 to 0.027 | 0.09 | –0.54 to 0.72 | |
Population achievementb | RE | 55,200 | 0.025 | 0.009 to 0.042 | 0.72 | 0.26 to 1.19 |
FEa | 54,675 | 0.037 | –0.058 to 0.133 | 1.02 | 0.18 to 1.87 | |
Effect of AR on ACSC admissions | ||||||
Reported achievement | RE | 23,111 | 0.002 | –0.010 to 0.013 | 0.44 | –2.65 to 3.61 |
FEa | 12,398 | 0.037 | –0.384 to 0.458 | 3.61 | –1.74 to 9.23 |
The association between CP achievement and SMI emergency admissions is statistically significant when only population achievement is considered. Even then, it is positive and small. The random-effects model estimates that a 10 percentage point increase in the CP population achievement rate is associated with an increase of 0.025 SMI admissions by general practice (0.72%). The fixed-effects model estimates a slightly larger increase of 0.037 admissions by general practice (1.02%).
We found no association between the AR reported achievement rate and ACSC admissions.
Discussion
Our results showed a small positive association between CP achievement and emergency SMI admissions. This finding is consistent with the results from our previous SMI project. 1 A 10 percentage point change in the CP population achievement rate increases SMI admissions by 1.08% according to the previous study1 and by 0.72% according to the current study. The small difference is due to the extension of the study period by 2 years (2006/7–2010/11 vs. 2006/7–2012/13). The effects of ARs are not comparable between the two studies, as the previous study focused on all physical admissions, whereas the current focuses on ACSC admissions.
However, the practice-level analysis is subject to serious limitations. First, we cannot be sure if the specific individuals who were admitted had received QOF checks. It is possible that those who were admitted as emergencies had not received an annual QOF review. It is also possible that individuals admitted as emergencies did receive QOF reviews, but only after discharge from hospital, rather than prior to admission, which could also explain the positive association.
Second, aggregate data do not enable us to specify the nature of individual care pathways or determine causality. Although we attempted to model all known confounding factors, we were unable to capture time-variant aspects of the influence of community mental health teams, and could not account for disease severity (case mix). In addition, we cannot rule out the possibility that there are other, unknown, biases that impact our findings.
Additional material
Figure 9 shows the rate of care indicators recorded by general practices per patient-year for each financial year in our sample. This rate is the number of times an indicator is recorded in that year across all patients, divided by the number of patient-years of observation in that financial year (which takes into account partial years for patients who were not observed for the full financial year). For example, in 2007/8 the rate of annual reviews (composite measure) recorded was 1.06 per patient-year.
FIGURE 9.
Rate of care indicators per patient-year.
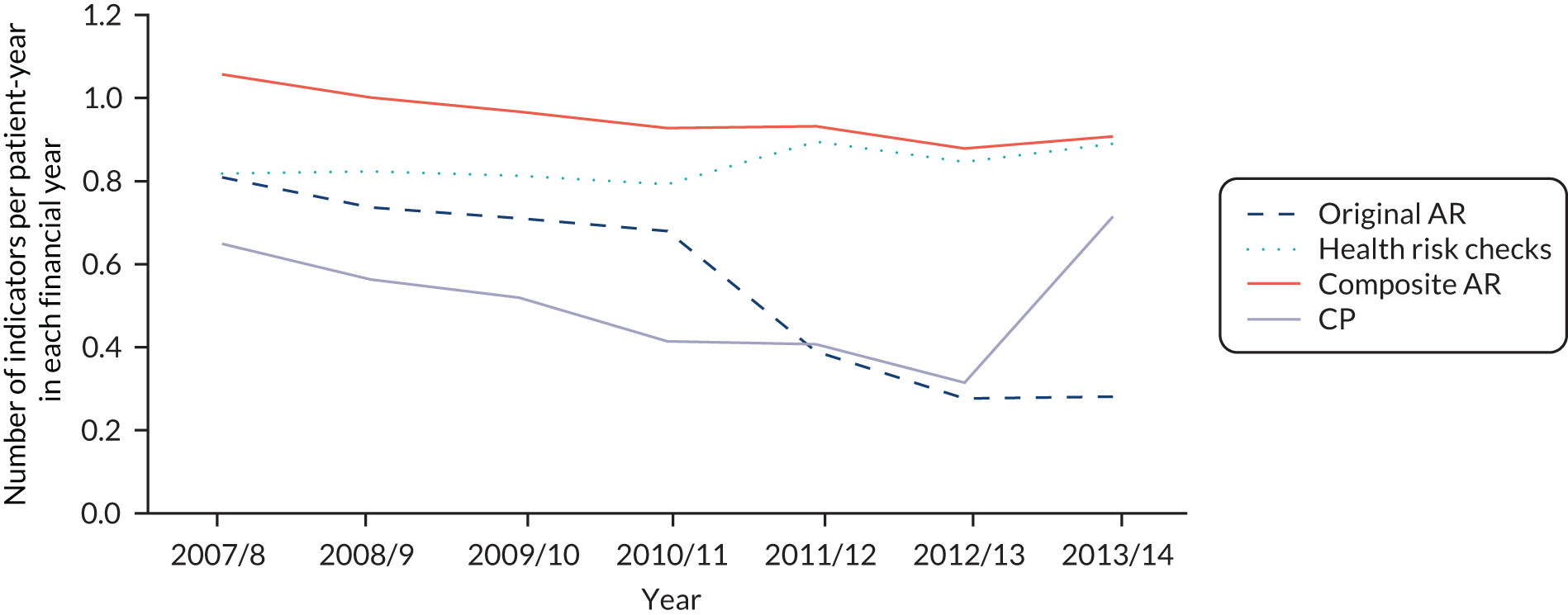
The CP indicator shows a decline over the study period until 2012/13, when there was an increase in the threshold of achievement required to attain the maximum payment. The original annual review indicator shows a marked drop-off after it ceased to be incentivised in 2010/11, whereas the aggregate indicator composed of the ‘health risk’ checks shows less marked change, but a small increase after the specific components began to be incentivised in 2011/12. The composite of these two annual review indicators shows little change in response to the QOF rule changes.
Appendix 4 The association between antipsychotic polypharmacy and hospital care utilisation and mortality
Continuous survival analysis
Complete linkage of primary, secondary and mortality data provides important information about the timing of APP episodes and the three outcomes. To exploit this rich information, we employ a time-to-event design. Our modelling choice is also supported by the following three features of the data: (1) patients enter the study at different times, (2) they may exit the study before the outcome occurs (right censoring), and (3) APP is a time-varying variable that does not occur at consistent intervals for all patients.
We follow each individual from entry date until the outcome of interest or until censoring. Censoring may occur because (1) a patient dies, (2) registration with the practice ends or (3) the study period ends (i.e. the patient is still registered on 31 March 2014). We treat death as censoring rather than a competing risk because our focus is on the relationship between quality indicators and outcomes, rather than on the prediction of the probability of an event at a given point in time. 182 Although some patients experience multiple events, we consider only the time to first event.
The hazard function for each outcome is specified as:
It is the product of the baseline hazard, λ0(t), and an exponentiated linear function of time-invariant covariates, Xi1, . . . , Xik, and the two time-varying variables APP(t) and NS(t), indicating if the patient is on APP at time t and whether or not the patient is on an antipsychotic medication at time t.
In survival analysis model without time-dependent variables, the baseline hazard cancels out and the HR between two individuals is constant over time. However, the proportionality of hazards does not hold in our case, as the time-dependent APP and average length of stay change at different times for different individuals. Estimation of the Cox model via partial likelihood is still feasible.
Antipsychotic product codes
We downloaded the antipsychotic drug codes list from the ClinicalCodes database [URL: https://clinicalcodes.rss.mhs.man.ac.uk/ (accessed 12 February 2020)], a web repository of clinical codes developed by the University of Manchester’s Institute of Population Health. 127 The repository holds a collection of peer-reviewed articles and the associated codes used irrespective of code type (e.g. Read, CPRD product/medical code, BNF code, ICD-10) and database (e.g. CPRD, The Health Improvement Network). We used the list of antipsychotic drug codes uploaded by Windfuhr et al. 128 The list includes three BNF chapter headings: (1) first-generation antipsychotic drugs or typical antipsychotics, (2) second-generation antipsychotics or atypical antipsychotics, and (3) depot antipsychotics. From the list of 33 antipsychotic substances presented in Table 34, 27 were recorded at least once in our sample.
Substancea | Product code | ||||||||||
---|---|---|---|---|---|---|---|---|---|---|---|
1 | Amisulpride | 80139020 | 68222020 | 81945020 | 35008020 | 35010020 | 85993020 | 69302020 | 68224020 | 00411021 | 89950020 |
85994020 | 85992020 | 68217020 | 80154020 | 79348020 | 86000020 | 86001020 | 85999020 | ||||
2 | Aripiprazole | 91145020 | 87950020 | 91147020 | 87952020 | 91149020 | 87954020 | 88668020 | 10469020 | 94973020 | 91139020 |
87944020 | 91141020 | 87946020 | 91143020 | 87948020 | 88666020 | 94971020 | |||||
3 | Asenapine | 00341021 | 337021 | ||||||||
4 | Benperidol | 64108020 | 98282020 | 64105020 | 84860020 | ||||||
5 | Chlorpromazine | 61251020 | 61249020 | 61250020 | 72606020 | 61237020 | 58896020 | 55203020 | 72588020 | 55710020 | 72587020 |
68531020 | 55217020 | 58894020 | 55204020 | 66353020 | 91404020 | 61244020 | 65188020 | 52087020 | 61238020 | ||
72597020 | 55228020 | 68532020 | 60250020 | 55202020 | 66356020 | 91408020 | 61243020 | 61264020 | 56481020 | ||
56450020 | 56444020 | 56445020 | 73791020 | 56446020 | 56468020 | 56456020 | |||||
6 | Chlorprothixene | 51832020 | |||||||||
7 | Clozapine | 72581020 | 97149020 | 72580020 | 97145020 | 97153020 | 72577020 | 72576020 | 88169020 | 97151020 | 88167020 |
97147020 | 97155020 | 88806020 | 88804020 | ||||||||
8 | Droperidol | 58513020 | 72300020 | 58514020 | 62878020 | 62879020 | 72297020 | 94599020 | |||
9 | Flupentixol | 48897020 | 62029020 | 48900020 | 91584020 | 53030020 | 53031020 | 89976020 | 89974020 | 57938020 | 89972020 |
62034020 | 91582020 | 68096020 | 89970020 | 62033020 | 62032020 | ||||||
10 | Fluphenazine | 62037020 | 62042020 | 50459020 | 50472020 | 92214020 | 62049020 | 92200020 | 92202020 | 61027020 | 62048020 |
62954020 | 92212020 | 92204020 | 92206020 | 92208020 | 50445020 | 92210020 | 92218020 | 53053020 | 92216020 | ||
11 | Fluspirilene | 62066020 | 51357020 | ||||||||
12 | Haloperidol | 63137020 | 63136020 | 58501020 | 58505020 | 58502020 | 58500020 | 95205020 | 58506020 | 63104020 | 55437020 |
53896020 | 54580020 | 75300020 | 63108020 | 99757020 | 60366020 | 63114020 | 511021 | 00443021 | 63115020 | ||
55438020 | 53576020 | 63110020 | 78059020 | 63109020 | 88468020 | 20065020 | 20067020 | 75301020 | 53575020 | ||
75302020 | 63113020 | 63118020 | 59312020 | 59028020 | 63105020 | 53898020 | 55351020 | 95203020 | 99755020 | ||
72226020 | 55346020 | 91764020 | 56176020 | 56181020 | 56182020 | 56183020 | 56175020 | 56177020 | 56187020 | ||
58510020 | 58509020 | 63124020 | |||||||||
13 | Levomepromazine | 87842020 | 38919020 | 64327020 | 64326020 | 32615020 | 97323020 | 87840020 | 52695020 | 3365020 | 52694020 |
14 | Methotrimeprazine | 64330020 | |||||||||
15 | Olanzapine | 00719021 | 81016020 | 00717021 | 00715021 | 87556020 | 83405020 | 00727021 | 55972020 | 00725021 | 00723021 |
59434020 | 81014020 | 03580020 | 00733021 | 90165020 | 00731021 | 00729021 | 92053020 | 00713021 | 81015020 | ||
00711021 | 00709021 | 83403020 | 83404020 | 00589021 | 87558020 | 83399020 | 78913020 | 58203020 | 79499020 | ||
81008020 | 92055020 | 90167020 | 83397020 | 78912020 | 83398020 | 98278020 | 98329020 | ||||
16 | Paliperidone | 93747020 | 93739020 | 93741020 | 93743020 | 99845020 | 99847020 | 99841020 | 99843020 | 99853020 | 99855020 |
99849020 | 99851020 | ||||||||||
17 | Penfluridol | 49952020 | 65318020 | ||||||||
18 | Pericyazine | 50678020 | 96724020 | 50677020 | 96722020 | 50679020 | 52648020 | 65580020 | 65584020 | 65579020 | 65581020 |
19 | Perphenazine | 49428020 | 49429020 | 53721020 | 65588020 | 65592020 | 65593020 | 56832020 | 65589020 | 56833020 | 65587020 |
52047020 | |||||||||||
20 | Pimozide | 58519020 | 58518020 | 65799020 | 65798020 | ||||||
21 | Pipotiazine | 53586020 | 91989020 | 91987020 | 91985020 | 91981020 | 65842020 | ||||
22 | Prochlorperazine | 68249020 | 91364020 | 54878020 | 66241020 | 66242020 | 66237020 | 54234020 | 57101020 | 69868020 | 60605020 |
55530020 | 56337020 | 66245020 | 69516020 | 69517020 | 72187020 | 56506020 | 91368020 | 58525020 | 52717020 | ||
52716020 | 51690020 | 04131020 | 4128020 | 04129020 | 96911020 | 04123020 | 51692020 | 96913020 | 52248020 | ||
52249020 | |||||||||||
23 | Promazine | 72991020 | 66279020 | 51749020 | 72987020 | 66278020 | 55705020 | 72988020 | 51751020 | 66277020 | 66283020 |
66282020 | 55706020 | 72183020 | 72184020 | 58296020 | 52959020 | 52957020 | 52958020 | 52968020 | 51649020 | ||
24 | Quetiapine | 85204020 | 97203020 | 97205020 | 00209021 | 00447021 | 98873020 | 85207020 | 94789020 | 85206020 | 85203020 |
42176020 | 33610020 | 99745020 | 94791020 | 87045020 | 94793020 | 94787020 | 36328020 | 85202020 | 85213020 | ||
85216020 | 85215020 | 85212020 | 87047020 | 98875020 | 95789020 | 95791020 | 95793020 | 95787020 | |||
25 | Remoxipride | 73439020 | 73440020 | 73441020 | 73474020 | 73475020 | 84528020 | ||||
26 | Risperidone | 49437020 | 73799020 | 49438020 | 49439020 | 73798020 | 77749020 | 73800020 | 78753020 | 77550020 | 82616020 |
13768020 | 81545020 | 77501020 | 92723020 | 92725020 | 88877020 | 36905020 | 79476020 | 53727020 | 74841020 | ||
49490020 | 86494020 | 81543020 | 53728020 | 86496020 | 92717020 | 53729020 | 92721020 | 49489020 | 88875020 | ||
77912020 | 74927020 | 74786020 | 65788020 | 49491020 | |||||||
27 | Sertindole | 82836020 | 82837020 | 82835020 | 82828020 | 82829020 | 82831020 | 82827020 | |||
28 | Sulpiride | 52921020 | 52922020 | 80696020 | 66836020 | 60498020 | 56102020 | 56529020 | 59205020 | 66837020 | 66838020 |
59150020 | 82057020 | ||||||||||
29 | Thioridazine | 54413020 | 67032020 | 75277020 | 67027020 | 67037020 | 54418020 | 54411020 | 67028020 | 67036020 | 67038020 |
54412020 | 67029020 | 75276020 | 55600020 | ||||||||
30 | Trifluoperazine | 51013020 | 51663020 | 51681020 | 51676020 | 51678020 | 51687020 | 51677020 | 87980020 | 67148020 | 67234020 |
75976020 | 55747020 | 75977020 | 67240020 | 67235020 | 55746020 | 67241020 | 69003020 | ||||
31 | Trifluperidol | 67244020 | 67245020 | 67248020 | 67249020 | ||||||
32 | Zotepine | 55209020 | 55210020 | 50782020 | 50780020 | 50781020 | |||||
33 | Zuclopenthixol | 48713020 | 48714020 | 48712020 | 90151020 | 90149020 | 72839020 | 61507020 | 61508020 | 61506020 | 90147020 |
90145020 | 72836020 | 91596020 | 53027020 | 67405020 | 91592020 | 61511020 | 61512020 |
Calculation of treatment duration
To mark the beginning and end of an APP episode we need information about the prescription date and the number of days the antipsychotic drug was prescribed. The CPRD therapy file provides the prescription date but the treatment duration is poorly populated, with 99% missing values for antipsychotic drugs. The reason is that, in most cases, GPs prescribe the quantity a patient should use until it runs out and, therefore, they are not interested in recording the duration of the prescription.
To overcome this problem, we calculated the treatment duration as the ratio of the total quantity entered by the GP for the prescribed product, over the ndd prescribed for the event (drug). As information on ndd is missing for > 23% of the therapy events, we employ the following imputation strategy: to events with missing ndd we assigned the most frequent ndd across all events with the specific product code. Approximately 1% of the therapy events involve a product for which all events have missing ndd. For these events, we imputed the ndd by assigning the most frequent ndd across all events with the specific substance.
Estimating the treatment duration as the ratio quantity : ndd was further complicated by another two issues. First, although pack size or type of the prescribed product is provided (e.g. 560 ml, 21 tablets), the unit in which ndd is recorded is not, and in some cases it is obvious that quantity and ndd are recorded in different units (e.g. quantity = 21 tablets, ndd = 300). In these cases, we divided ndd by the strength of the prescribed substance to impute the true ndd (e.g. ndd = 300/100 mg = three tablets). Second, < 0.02% of prescription records (245/1,035,856) had an implausibly large calculated duration and were dropped from the analysis.
Measures of polypharmacy prevalence
We calculated two measures of polypharmacy prevalence. First, the annual prevalence of polypharmacy as the number of patients with at least one polypharmacy episode in a year divided by the total number of patients observed during that year. Second, the rate of polypharmacy defined as the sum of all patients’ polypharmacy days in a year over the sum of all patients’ days at risk of polypharmacy in that year.
Our second measure – the rate of polypharmacy – is an improvement over the point estimates of polypharmacy prevalence often reported in the literature. Point estimates of prevalence show what fraction of eligible individuals are prescribed polypharmacy on a given day. Average point estimates of prevalence can be calculated by averaging daily point estimates.
In the following, we prove that the rate of polypharmacy is a weighted average of the point estimates of polypharmacy on each of the 365 days of the year. Instead of assigning an equal weight to each day of the year, our measure puts a different weight according to the ratio of patients at risk on that day over the patients at risk in the entire year.
Let POLnt and POPnt be defined as:
The average point estimate of polypharmacy prevalence is:
The rate of polypharmacy is:
From the Equations 15 and 16 it follows that the two measures differ only in the weights used.
Figure 10 is the same as Figure 5, with the addition of the polypharmacy prevalence calculated on 1 January of each calendar year. The trends of the rate of polypharmacy and the point estimate of polypharmacy prevalence are very similar (Figures 11 and 12, and Tables 35 and 36).
FIGURE 10.
Measures of polypharmacy prevalence.
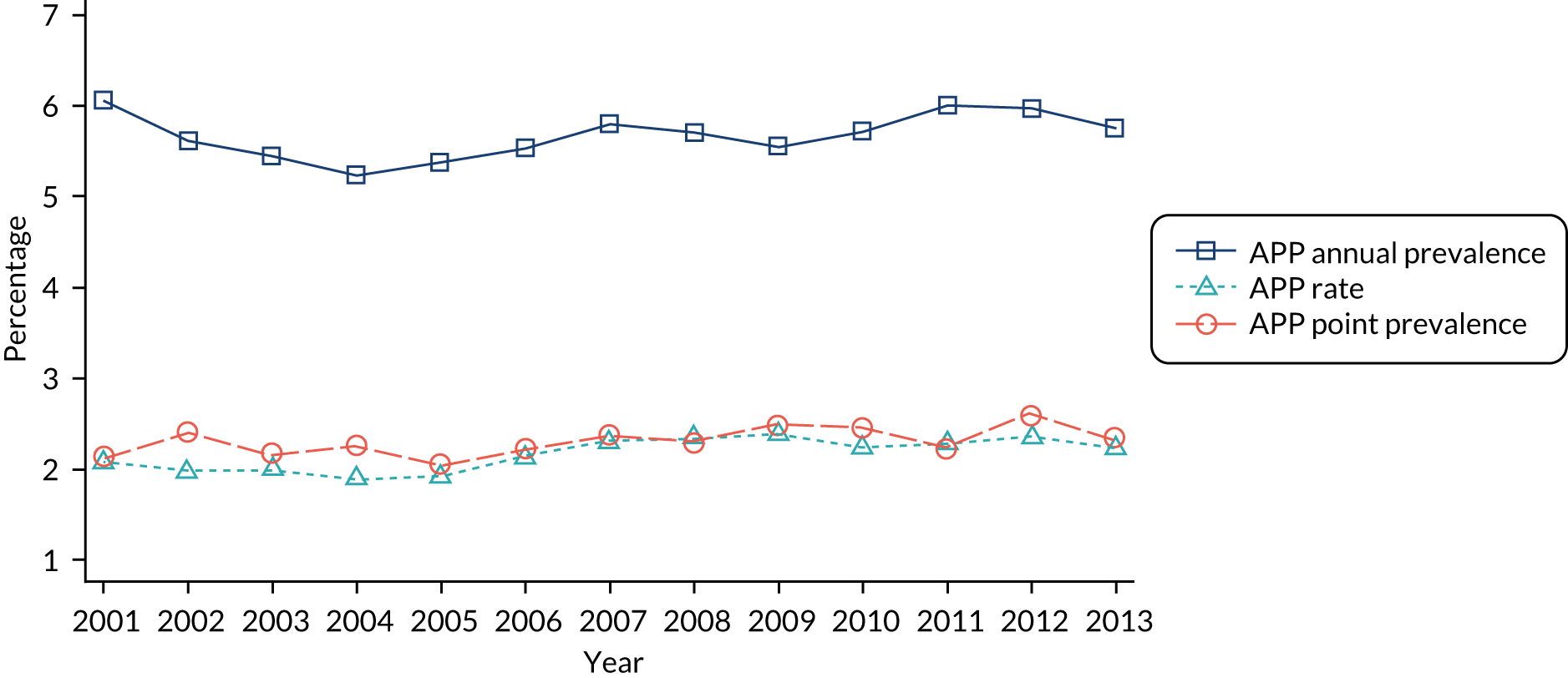
FIGURE 11.
Annual prevalence of (antipsychotic) polypharmacy.
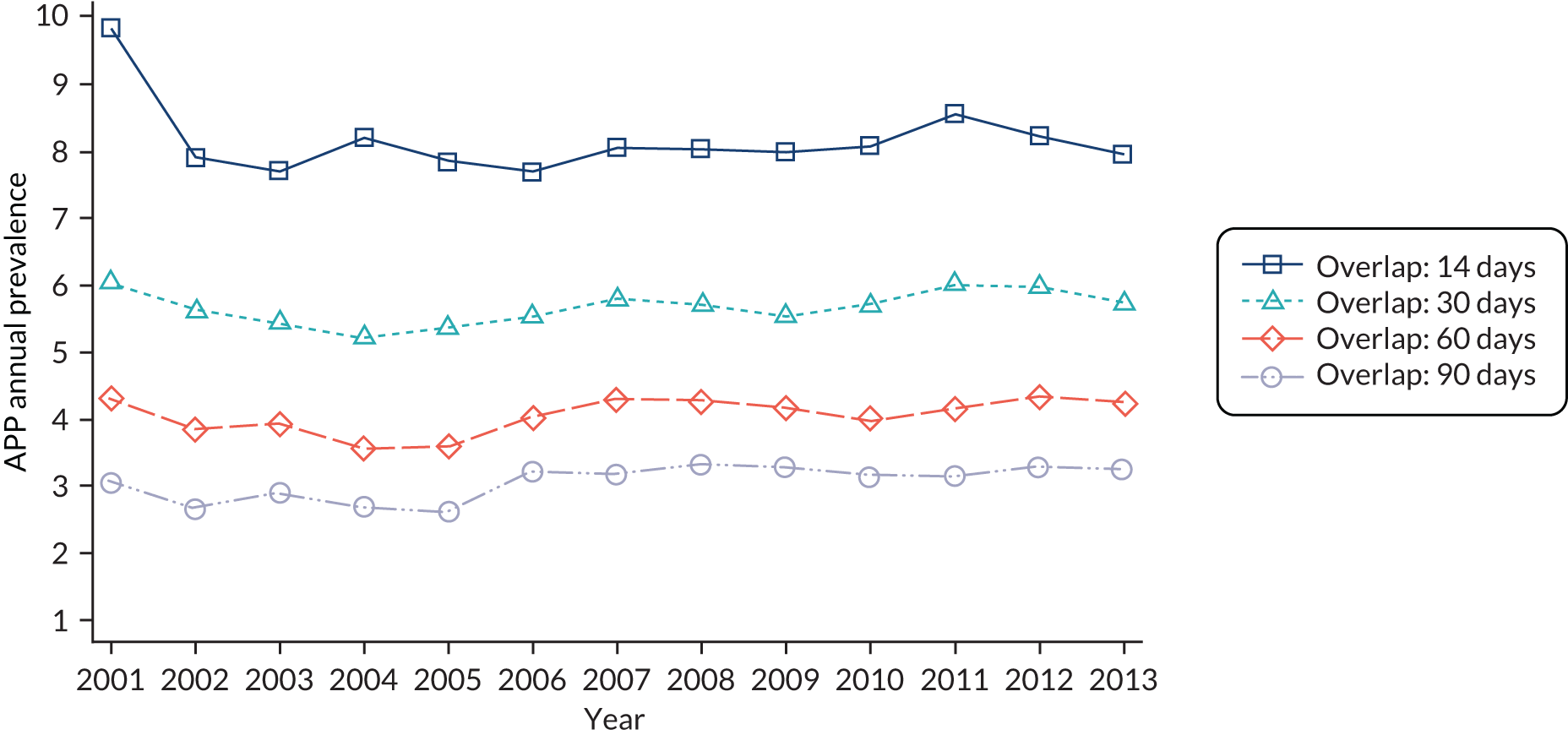
FIGURE 12.
Rate of APP.
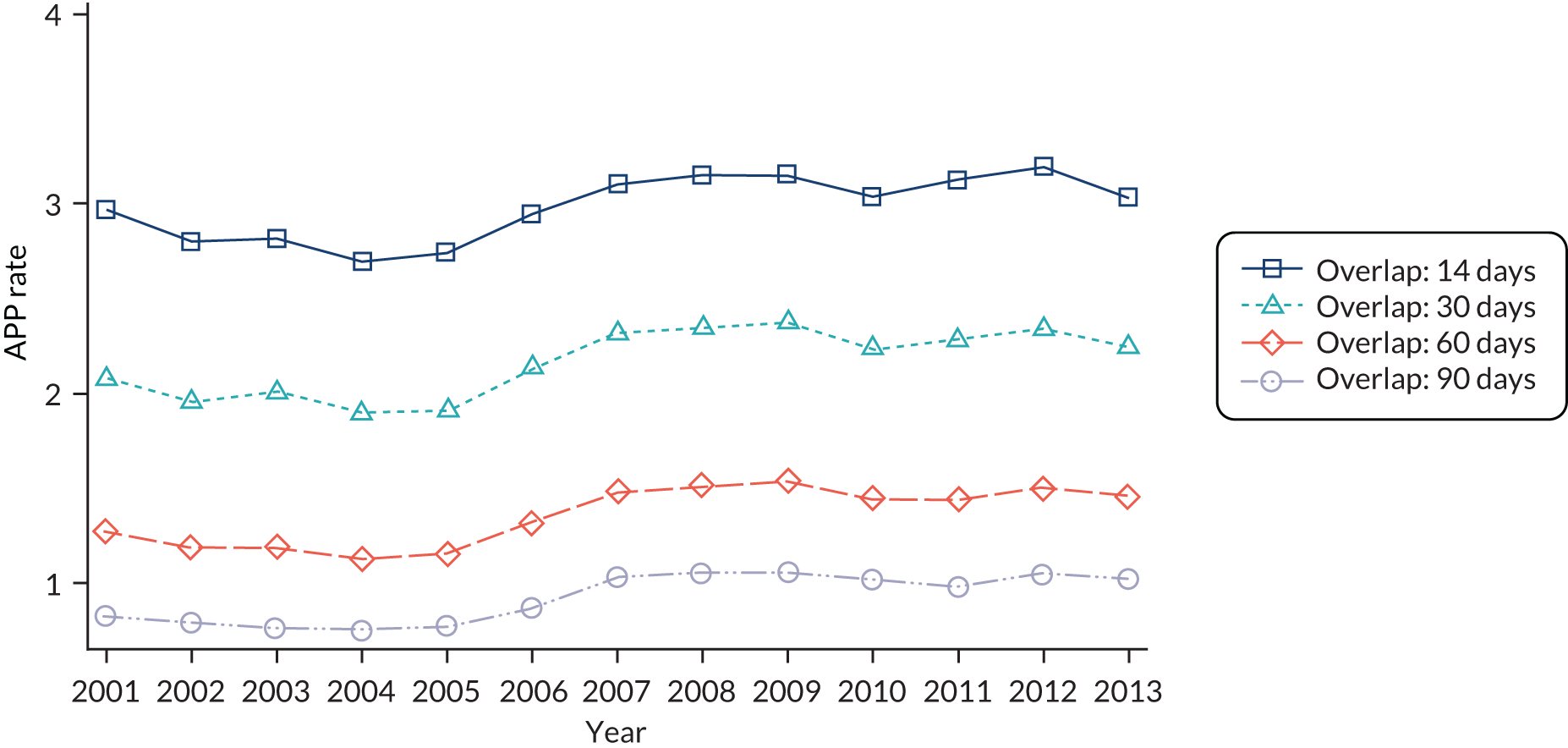
Unplanned admissions | Unplanned SMI admissions | |||
---|---|---|---|---|
HR | 95% CI | HR | 95% CI | |
APP (time varying) | 1.14 | 0.99 to 1.32 | 1.19 | 0.92 to 1.53 |
No substance (time varying) | 1.08** | 1.03 to 1.14 | 1.23*** | 1.13 to 1.35 |
Age (years) at entry into the sample (base: 19–35 years) | ||||
36–45 | 0.95 | 0.86 to 1.04 | 0.88 | 0.76 to 1.02 |
46–55 | 0.86** | 0.79 to 0.94 | 0.69*** | 0.60 to 0.79 |
56–65 | 0.98 | 0.89 to 1.09 | 0.56*** | 0.47 to 0.67 |
≥ 66 | 1.51*** | 1.37 to 1.67 | 0.42*** | 0.35 to 0.51 |
Male | 0.99 | 0.90 to 1.10 | 1.01 | 0.87 to 1.16 |
Age (years) at diagnosis*Male (base: 19–35 years*Male) | ||||
36–45*Male | 0.94 | 0.82 to 1.07 | 0.96 | 0.81 to 1.16 |
46–55*Male | 1.01 | 0.88 to 1.17 | 0.96 | 0.78 to 1.18 |
56–65*Male | 1.01 | 0.88 to 1.15 | 0.92 | 0.71 to 1.20 |
≥ 66*Male | 1.09 | 0.96 to 1.25 | 0.95 | 0.72 to 1.26 |
Time (years) since diagnosis (base: < 1 year) | ||||
1–5 | 0.97 | 0.91 to 1.03 | 1.22*** | 1.11 to 1.34 |
> 5 | 0.86*** | 0.81 to 0.90 | 1.15** | 1.04 to 1.27 |
IMD (base: quintile 1) | ||||
Quintile 2 | 1.00 | 0.93 to 1.08 | 0.98 | 0.86 to 1.12 |
Quintile 3 | 1.08 | 0.99 to 1.17 | 1.01 | 0.87 to 1.19 |
Quintile 4 | 1.11* | 1.02 to 1.20 | 1.03 | 0.90 to 1.18 |
Quintile 5 | 1.21*** | 1.11 to 1.31 | 1.16* | 1.01 to 1.33 |
Ethnicity: white | 2.26*** | 2.11 to 2.41 | 2.11*** | 1.86 to 2.34 |
Practice is in rural area | 0.96 | 0.89 to 1.04 | 0.97 | 0.82 to 1.15 |
Minimum distance (km) to acute health services (base: < 3) | ||||
3–6 | 1.03 | 0.97 to 1.09 | 1.04 | 0.91 to 1.18 |
6–9 | 0.92 | 0.84 to 1.01 | 0.97 | 0.82 to 1.16 |
> 9 | 1.02 | 0.94 to 1.11 | 0.98 | 0.82 to 1.18 |
Minimum distance (km) to mental health services (base: < 3) | ||||
3–6 | 0.99 | 0.92 to 1.07 | 0.96 | 0.82 to 1.14 |
6–9 | 1.03 | 0.95 to 1.12 | 1.00 | 0.84 to 1.20 |
> 9 | 0.98 | 0.90 to 1.06 | 0.97 | 0.81 to 1.16 |
Number of primary care contacts within 12 months prior to diagnosis (base: < 5) | ||||
5–9 | 1.05 | 0.99 to 1.12 | 0.88* | 0.80 to 0.97 |
10–14 | 1.08* | 1.01 to 1.16 | 0.84*** | 0.76 to 0.93 |
15–19 | 1.18*** | 1.10 to 1.27 | 0.85* | 0.75 to 0.96 |
≥ 20 | 1.30*** | 1.22 to 1.39 | 0.85** | 0.75 to 0.96 |
Current or ex-smoker | 1.08** | 1.02 to 1.14 | 1.04 | 0.94 to 1.15 |
Current or ex-alcohol user | 0.97 | 0.93 to 1.02 | 1.03 | 0.95 to 1.11 |
Number of Charlson Comorbidity Index comorbidities | 1.22*** | 1.18 to 1.25 | 0.94 | 0.88 to 1.00 |
Diagnosis of depression | 1.02 | 0.97 to 1.06 | 0.84*** | 0.77 to 0.90 |
SMI diagnosis group (base: bipolar disorder or affective psychosis) | ||||
Schizophrenia or other psychosis | 1.02 | 0.97 to 1.07 | 1.10* | 1.01 to 1.20 |
Both | 1.20*** | 1.12 to 1.29 | 1.77*** | 1.58 to 1.98 |
Mortality | A&E department attendance | |||
---|---|---|---|---|
HR | 95% CI | HR | 95% CI | |
APP (time varying) | 1.02 | 0.76 to 1.37 | 0.95 | 0.80 to 1.14 |
No substance (time varying) | 1.02 | 0.94 to 1.10 | 1.19*** | 1.14 to 1.24 |
Age (years) at entry into the sample (base: 19–35 years) | ||||
36–45 | 2.53*** | 1.57 to 4.09 | 0.86** | 0.78 to 0.95 |
46–55 | 4.34*** | 2.82 to 6.66 | 0.75*** | 0.68 to 0.82 |
56–65 | 9.22*** | 6.09 to 13.97 | 0.71*** | 0.64 to 0.79 |
≥ 66 | 31.72*** | 21.38 to 47.05 | 1.02 | 0.93 to 1.12 |
Male | 1.98** | 1.27 to 3.09 | 0.97 | 0.88 to 1.07 |
Age (years) at diagnosis *Male (base: 19–35 years*Male) | ||||
36–45*Male | 0.77 | 0.45 to 1.32 | 0.97 | 0.84 to 1.13 |
46–55*Male | 0.74 | 0.45 to 1.21 | 1.05 | 0.91 to 1.20 |
56–65*Male | 0.63 | 0.39 to 1.02 | 1.10 | 0.95 to 1.28 |
≥ 66*Male | 0.60* | 0.39 to 0.94 | 1.03 | 0.89 to 1.18 |
Time (years) since diagnosis (base: < 1 year) | ||||
1–5 | 1.01 | 0.91 to 1.13 | 0.97 | 0.90 to 1.05 |
> 5 | 0.76*** | 0.68 to 0.85 | 0.90** | 0.84 to 0.96 |
IMD (base: quintile 1) | ||||
Quintile 2 | 0.92 | 0.80 to 1.06 | 1.07 | 0.97 to 1.18 |
Quintile 3 | 1.06 | 0.93 to 1.20 | 1.11* | 1.00 to 1.23 |
Quintile 4 | 0.99 | 0.86 to 1.14 | 1.18** | 1.06 to 1.31 |
Quintile 5 | 1.05 | 0.91 to 1.22 | 1.31*** | 1.17 to 1.47 |
Ethnicity: white | 0.92 | 0.83 to 1.02 | 1.49*** | 1.40 to 1.58 |
Practice is in rural area | 1.03 | 0.88 to 1.20 | 0.85* | 0.75 to 0.97 |
Minimum distance (km) to acute health services (base: < 3) | ||||
3–6 | 1.07 | 0.95 to 1.20 | 0.92 | 0.83 to 1.02 |
6–9 | 1.11 | 0.96 to 1.29 | 0.90 | 0.78 to 1.05 |
> 9 | 1.17 | 0.99 to 1.34 | 0.99 | 0.85 to 1.17 |
Minimum distance (km) to mental health services (base: < 3) | ||||
3–6 | 0.95 | 0.82 to 1.10 | 1.02 | 0.90 to 1.16 |
6–9 | 0.96 | 0.82 to 1.12 | 0.97 | 0.85 to 1.10 |
> 9 | 0.97 | 0.83 to 1.14 | 0.88 | 0.77 to 1.00 |
Number of primary care contacts within 12 months prior to diagnosis (base: < 5) | ||||
5–9 | 1.04 | 0.91 to 1.18 | 1.12** | 1.04 to 1.20 |
10–14 | 1.06 | 0.93 to 1.21 | 1.22*** | 1.13 to 1.33 |
15–19 | 1.28** | 1.10 to 1.48 | 1.37*** | 1.25 to 1.50 |
≥ 20 | 1.28*** | 1.11 to 1.48 | 1.59*** | 1.46 to 1.73 |
Current or ex-smoker | 0.93 | 0.85 to 1.03 | 1.07* | 1.01 to 1.14 |
Current or ex-alcohol user | 0.83*** | 0.76 to 0.92 | 0.98 | 0.92 to 1.04 |
Number of Charlson Index comorbidities | 1.43*** | 1.37 to 1.49 | 1.14*** | 1.11 to 1.18 |
Diagnosis of depression | 0.89** | 0.81 to 0.96 | 1.07** | 1.02 to 1.13 |
SMI diagnosis group (base: bipolar disorder or affective psychosis) | ||||
Schizophrenia or other psychosis | 1.42*** | 1.29 to 1.56 | 0.94* | 0.89 to 1.00 |
Both | 1.07 | 0.93 to 1.25 | 1.02 | 0.94 to 1.09 |
List of abbreviations
- ACSC
- ambulatory care sensitive condition
- A&E
- accident and emergency
- APP
- annual prevalence of (antipsychotic) polypharmacy
- AR
- annual review of the patient’s physical health
- ASSIA
- Applied Social Sciences Index and Abstracts
- BMI
- body mass index
- BNF
- British National Formulary
- CE
- cluster episode
- CENTRAL
- Cochrane Central Register of Controlled Trials
- CI
- confidence interval
- COC
- Continuity of Care Index
- CP
- care plan
- CPA
- care programme approach
- CPCI-S
- Conference Proceedings Citation Index – Science
- CPRD
- Clinical Practice Research Datalink
- DARE
- Database of Abstracts of Reviews of Effects
- ECT
- electroconvulsive therapy
- ESR
- Electronic Staff Record
- GLM
- generalised linear model
- GP
- general practitioner
- HES
- Hospital Episodes Statistics
- HoNOS
- Health of the Nation Outcome Scale
- HR
- hazard ratio
- HRG
- Healthcare Resource Group
- ICD-10
- International Statistical Classification of Diseases and Related Health Problems, Tenth Revision
- IMD
- Index of Multiple Deprivation
- LSOA
- lower-layer super output area
- MHCT
- mental health clustering tool
- MHMDS
- Mental Health Minimum Data Set
- ndd
- numeric daily dose
- NICE
- National Institute for Health and Care Excellence
- NIHR
- National Institute for Health Research
- ONS
- Office for National Statistics
- P4P
- pay for performance
- PPI
- patient and public involvement
- PSSRU
- Personal Social Services Research Unit
- QOF
- Quality and Outcomes Framework
- RCT
- randomised controlled trial
- SE
- standard error
- SECON
- Sequential Continuity Index
- SMI
- serious mental illness
- SSC
- Scientific Steering Committee
- UPC
- Usual Provider of Care Index
Notes
Supplementary material can be found on the NIHR Journals Library report page (https://doi.org/10.3310/hsdr08250).
Supplementary material has been provided by the authors to support the report and any files provided at submission will have been seen by peer reviewers, but not extensively reviewed. Any supplementary material provided at a later stage in the process may not have been peer reviewed.