Notes
Article history
The research reported in this issue of the journal was funded by the HTA programme as project number 08/67/03. The contractual start date was in March 2009. The draft report began editorial review in January 2012 and was accepted for publication in February 2013. The authors have been wholly responsible for all data collection, analysis and interpretation, and for writing up their work. The HTA editors and publisher have tried to ensure the accuracy of the authors’ report and would like to thank the reviewers for their constructive comments on the draft document. However, they do not accept liability for damages or losses arising from material published in this report.
Permissions
Copyright statement
© Queen’s Printer and Controller of HMSO 2014. This work was produced by Farmer et al. under the terms of a commissioning contract issued by the Secretary of State for Health. This issue may be freely reproduced for the purposes of private research and study and extracts (or indeed, the full report) may be included in professional journals provided that suitable acknowledgement is made and the reproduction is not associated with any form of advertising. Applications for commercial reproduction should be addressed to: NIHR Journals Library, National Institute for Health Research, Evaluation, Trials and Studies Coordinating Centre, Alpha House, University of Southampton Science Park, Southampton SO16 7NS, UK.
Chapter 1 Introduction and rationale
Diabetes is one of the leading causes of death and disability. The number of people with diabetes is projected to double worldwide over the next 30 years: 85% of patients are categorised as having type 2 diabetes, with associated mortality predominantly from cardiovascular diseases (CVDs). Another major complication of diabetes is diabetic kidney disease, which affects around 35% of the diabetic population. 1 At each successive stage of diabetic kidney disease there is an increased risk of cardiovascular death. 2 With younger onset of diabetes and a focus on preventing cardiovascular events among patients with diabetes, the number of patients projected to progress to chronic kidney disease is likely to rise. Optimising the clinical strategy for identification of deteriorating renal function is therefore of major importance.
The measurement of albumin levels is widely accepted as a simple, safe and validated test for identifying patients at an early stage of disease. 3 However, there remains a number of unanswered questions about the utility of this test, including whether it meets the criteria for a screening test and, if it does meet these criteria, what the optimal interval for retesting is. A report from the National Institute for Health Research (NIHR) Health Technology Assessment (HTA) programme identified the limited evidence supporting universal screening for microalbuminuria,4 but, despite the concerns raised, yearly intervals for screening continue to be incentivised within the United Kingdom Quality Outcomes Framework. Further work in this area supported by the NIHR HTA includes a systematic review of early referral strategies for the management of people with markers of kidney disease. 5
The work described in this report, therefore, forms part of a commissioned stream of work by the NIHR HTA programme to focus on a key recommendation of previous work: to identify an evidence base for the frequency of screening tests for microalbuminuria. This work has involved utilising and developing new approaches for determining the intervals at which a disease parameter should be monitored: systematic review of the literature to establish effect size of treatment with renin–angiotensin–aldosterone system inhibitors (RAASIs); deriving parameters from repeated measurements in cohort studies to identify biological variability, measurement error and coefficients of variation in the rate of change of microalbuminuria and glomerular filtration rate (GFR) over time; and health economic modelling to establish the cost-effectiveness of different strategies for monitoring.
This introductory chapter, therefore, sets out what we know about the development and progression of diabetic kidney disease to set the clinical background for this work, and provides the rationale for the estimates for a range of the parameters used in health economic modelling.
Diabetes mellitus, microalbuminuria and kidney disease
Renal, or kidney, disease is a serious and common complication of diabetes. Kidney damage in diabetes can arise from both microvascular (classic diabetic kidney disease) and arterial (renovascular) damage. 6 Diabetic kidney disease, characterised by a sustained increase in urinary albumin excretion (UAE),7 is an increasingly common cause of end-stage kidney disease. There are nearly 350 million people worldwide with diabetes,8 and the numbers are projected to increase further. About one-third of those with type 2 diabetes9 and 35% with type 1 diabetes develop a progressive deterioration of renal function. 10 In addition, raised UAE is a marker of increased risk of CVD, with 40–50% of those with type 2 diabetes and microalbuminuria eventually dying of CVD.
Diabetic kidney disease has a major impact on patients’ lives. It is expensive to treat and kidney disease progression is a marker of subsequent morbidity and mortality. 11 In the UK in 2004, the total annual NHS costs for managing diabetic kidney disease were reported as £152M for type 1 diabetes and £614M for type 2 diabetes. 12
Kidney disease is usually categorised into five stages determined by GFR, with the first stage identified by albuminuria or other abnormal function (Table 1 and Figure 1), and the remaining four stages by deterioration below thresholds of 90, 60, 30 and 15 ml/minute/1.73 m2. In routine clinical practice, the GFR is estimated from serum creatinine, age and sex using an equation derived from the Modification of Diets in Renal Disease (MDRD) study. 14
Stage | eGFR | UAE |
---|---|---|
1 Kidney damage with no impairment | > 90 ml/minute/1.73 m2 | Normal/micro/proteinuria |
With other evidence of kidney disease | ||
2 Mild impairment | 60–90 ml/minute/1.73 m2 | Normal/micro/proteinuria |
With other evidence of kidney disease | ||
3a Moderate impairment | 45–59 ml/minute/1.73 m2 | Normal/micro/proteinuria |
3b Moderate impairment | 30–44 ml/minute/1.73 m2 | Normal/micro/proteinuria |
4 Severe impairment | 15–29 ml/minute/1.73 m2 | Normal/micro/proteinuria |
5 Renal failure | < 15 ml/minute/1.73 m2; or | Normal/micro/proteinuria |
On dialysis |
FIGURE 1.
Development of diabetic kidney disease and micro- and macroalbuminuria.
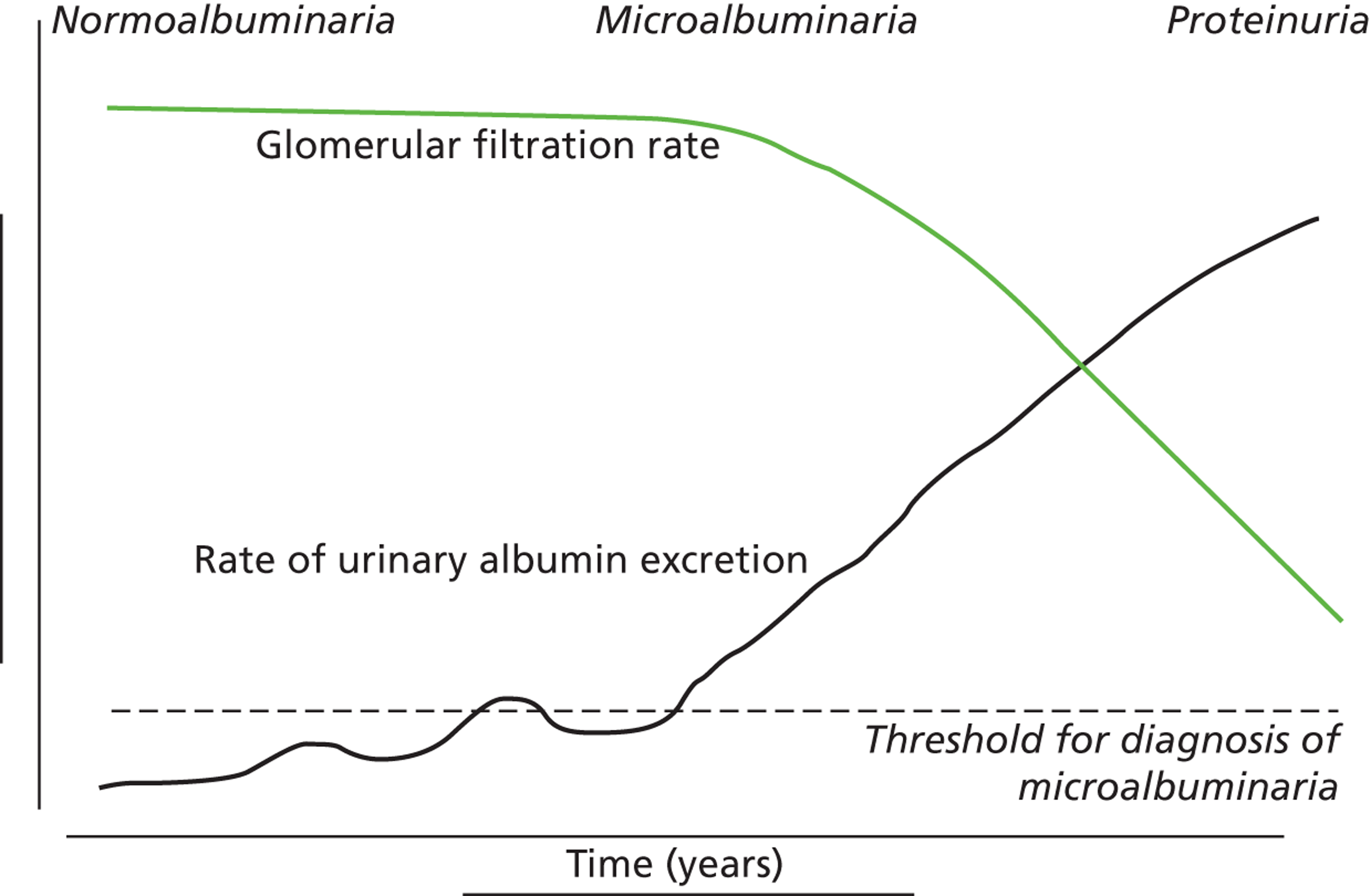
Epidemiology of microalbuminuria
Microalbuminuria is a marker of the early stages of kidney disease and reflects widespread vascular damage. Thus, it is a marker of increased risk in both type 1 and type 2 diabetes.
Definitions of microalbuminuria
Microalbuminuria is conventionally defined as levels of albumin ranging from 30 to 300 mg in a 24-hour urine collection (Table 2). 15 Overt albuminuria, macroalbuminuria or proteinuria is defined as a UAE of ≥ 300 mg/24 hours. There is increasing recognition, however, that there is not an arbitrary boundary between levels of albumin excretion and that there is a continuum of progression in which the level of albumin provides a marker of how far the disease has advanced. 3 In recognition of this, a recent position statement has recommended that the terms microalbuminuria and macroalbuminuria be replaced, respectively, by the terms ‘high albuminuria’ and ‘very high albuminuria’. 16
Measure of albumin excretion | Normal UAE | Microalbuminuria | Proteinuria |
---|---|---|---|
ACR | |||
Female | < 3.5 | 3.5–30.0 | > 30 |
Male | < 2.5 | 2.5–30.0 | > 30 |
Timed overnight UAE rate (µg/minute) | < 20 | 20–200 | > 200 |
24-hour urine collection (mg/24 hours) | < 30 | 30–300 | > 300 |
In clinical practice, albuminuria is measured by the albumin-to-creatinine ratio (ACR) in a random or first-pass morning sample of urine. Because women excrete less creatinine than men, clinicians in the UK use different ACR thresholds for diagnosing microalbuminuria in men and women. 17
Are tests to detect the onset of microalbuminuria reliable?
Detecting whether levels of urinary albumin reach the threshold for diagnosis of microalbuminuria is not straightforward. First, levels of albumin may be affected by concurrent illness including virus infections and urinary tract infections. They may also be elevated after physical activity. Second, levels vary within an individual. There is evidence, at least for type 1 diabetes, that the occurrence of intermittent tests above the threshold for microalbuminuria predicts progression, and that those with levels of urinary albumin close but below the threshold have an increased risk of progression. 18 To address these issues, a standard protocol for diagnosis of microalbuminuria requires additional tests following a positive test, with at least one of the further tests required to also be above the diagnostic threshold (Figure 2). Standard protocols recommended in guidelines vary slightly;15,19 however, they are broadly consistent in aiming to optimise the delivery of targeted intensification of therapy to high-risk patients, for whom the treatments will provide a greater absolute risk reduction than they would in those at lower risk. 3
FIGURE 2.
Flow chart for confirmation of microalbuminuria. Tests need to be carried out in the absence of proteinuria/urinary tract infection. Test abnormal if ACR > 2.5 mg/mmol for men and > 3.5 mg/mmol for women. Based on the guidance in National Institute for Health and Care Excellence CG66. 19
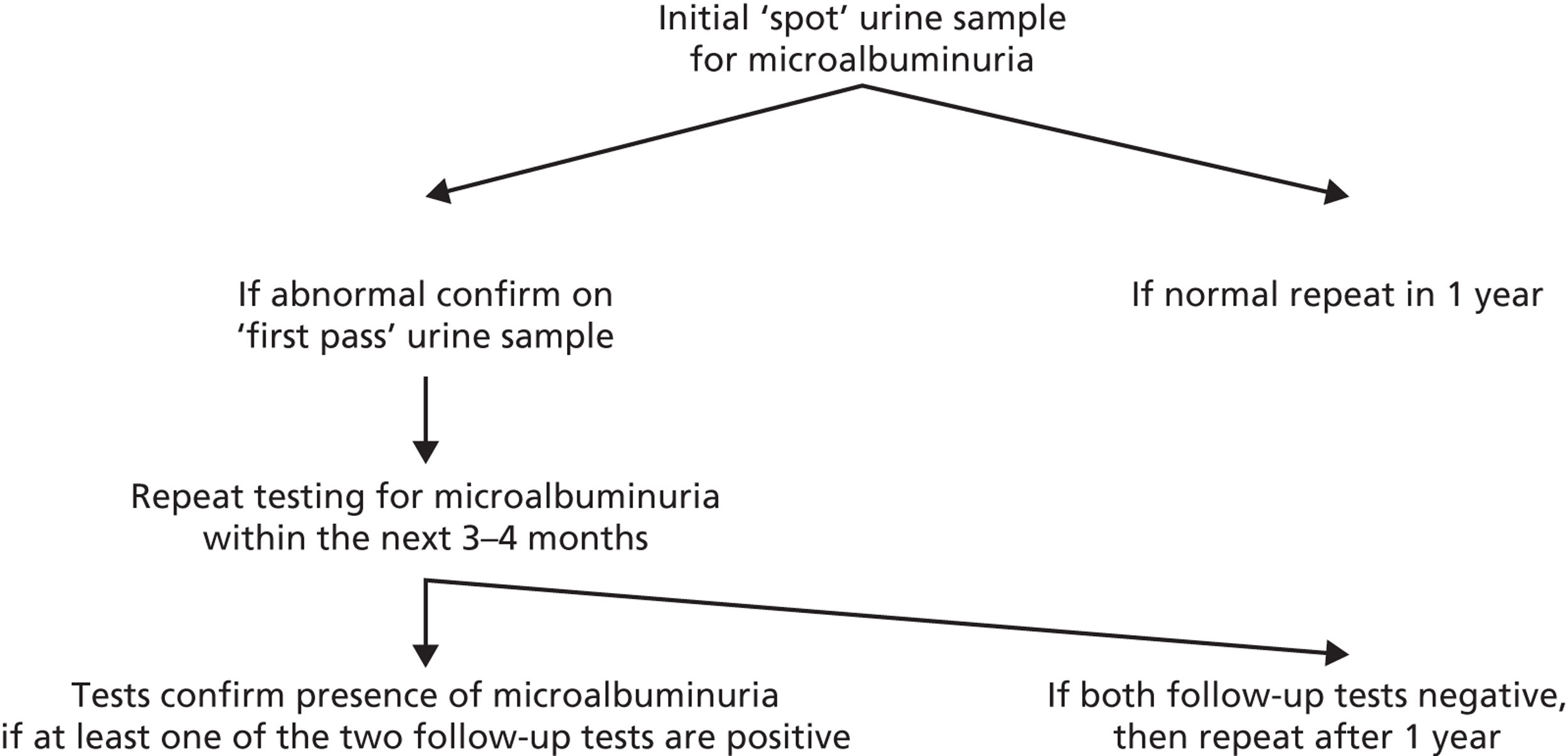
The development and progression of diabetic kidney disease
Determinants for the development and progression of diabetic kidney disease are well established and discussed in detail below. Although there are familial elements that contribute to the risk of developing kidney disease, there are, as yet, no genetic traits that have been identified as contributing a substantial risk. However, there are some differences in the risk factors for development and progression of diabetic kidney disease between type 1 and 2 diabetes.
Development and progression of diabetic kidney disease in type 1 diabetes
The epidemiology of diabetic kidney disease is most easily studied in patients with type 1 disease, as it is usually possible to identify a defined time point of onset. Around 1.5% to 2.0% of patients develop microalbuminuria annually, with one-third of patients having microalbuminuria after 15 years of diabetes, and 50% at some point in their lives, although a recent study put the figure lower, at 35%. 15,19 If untreated, the rate of increase in UAE following development of microalbuminuria is approximately 20% a year. Once identified as having microalbuminuria, less than half of the patients will progress to chronic kidney disease, and the remainder will either regress or remain stable. 1
A 25–50% elevation in GFR is seen soon after diagnosis in up to half of patients with type 1 diabetes mellitus. The significance of these changes remains uncertain. These changes in the haemodynamic of the circulation may represent early pathological changes, or may be a compensatory response to changes in tubular reabsorption as a consequence of hyperinsulinaemia and other factors. 20
At diagnosis, UAE is in the normal range for all patients with type 1 diabetes, unless there is ketosis. In the early years, microalbuminuria is not found unless there is poor glycaemic control, in which case it may be a reversible abnormality. Diabetic kidney disease results from characteristic structural and functional changes to the glomerulus. These changes include mesangial expansion, glomerular basement membrane thickening and glomerular sclerosis. Poor glycaemic control is the main reason for the development of albuminuria and diabetic kidney disease. 21 Once microalbuminuria develops, for many individuals there is an overall increase in blood pressure.
Development and progression of diabetic kidney disease in type 2 diabetes
There are now a wide range of studies that provide information about the development and progression of diabetic kidney disease in patients with type 2 diabetes. As with type 1 diabetes, the initial development of albuminuria is a consequence of poor glycaemic control, although elevated blood pressure and dyslipidaemia may already be present at diagnosis. In the United Kingdom Prospective Diabetes Study (UKPDS), approximately 7.3% of individuals were found to have microalbuminuria or worse already present at diagnosis of diabetes. 22 Modelled estimates from the study suggest that the median time that patients remain free of microalbuminuria is 18.9 years. The figures for prevalence of kidney disease vary between studies. For example, in the Wisconsin Epidemiological Study of Diabetic Retinopathy, 54.8% of participants did not have kidney disease at 15 years,23 compared with 72.0% in the UKPDS cohort,22 with a rate of progression from normoalbuminuria to microalbuminuria of 2.0% per year.
Normoalbuminuric chronic kidney disease in type 2 diabetes
An important component of monitoring patients with type 2 diabetes is the role of serum creatinine tests for identifying impaired renal function. A substantial proportion of individuals with type 2 diabetes, but without microalbuminuria, have an estimated GFR (eGFR) of < 60 ml/minute/1.73 m2. Studies using isotopic GFR (iGFR) measurements in patients with type 2 diabetes suggest that up to 24% of those with stage 3 renal disease may not have albuminuria. 24 Similar development of renal insufficiency in the absence of albuminuria and retinopathy has been demonstrated in large-scale studies using eGFR. 25 UK-based studies in primary care suggested that, in patients who had diabetes and an eGFR < 60 ml/minute/1.7 3m2, 63% had normoalbuminuria. 26 Although there is continuing uncertainty over the relationship between this impaired renal function and diabetes, the most likely aetiology is hypertensive or intrarenal vascular disease, with some degree of misclassification caused by a normal degree of declining renal function in older people.
What risk factors affect progression to microalbuminuria?
Risk factors affecting progression to microalbuminuria in type 1 diabetes
The strongest risk factor affecting progression from normal renal function to microalbuminuria is glycaemia (Tables 3–5). Data from the Diabetes Control and Complications Trial (DCCT) confirms a 39% relative risk reduction for development of microalbuminuria with intensive versus conventional treatment. 45 Smoking is also a strong predictor of the development of microalbuminuria with a hazard ratio of 1.28 quoted in one study,46 and an odds ratio of 1.40 found in another. 33 Following cessation of smoking, the rate of loss of renal function is lower than among those who continue to smoke. Elevated blood pressure is also a strong predictor of progression to microalbuminuria. Although protein intake is often cited as a risk factor for progression to microalbuminuria, the evidence is tenuous. 47
Study (first author, year) | Patients | Findings |
---|---|---|
Type 1 diabetes: normoalbuminuria | ||
Lin 200827 | 725 normoalbuminuric patients from the DCCT followed for a median of 6.5 years | In the fully adjusted mixed-effects regression models, the AER increase was 1.44 µg/minute/year in the primary prevention group |
Bilous 200928 | 710 normoalbuminuric patients without retinopathy followed for 6 years | Least squares mean of annual change in UAER = 0.54 µg/minute (95% CI 0.52 to 0.57 µg/minute) |
Type 1 diabetes: microalbuminuria | ||
Lin 200827 | 642 microalbuminuric patients from the DCCT followed for a median of 6.5 years | In the fully adjusted mixed-effects regression models, the AER increase was 8.71 µg/minute/year in the secondary prevention group |
Rossing 200529 | 181 patients followed up for a median of 7.9 years | Mean annual rate of progression (± SEM) of urinary albumin was 6.2 ± 1.7%). Annual progression rate (± SEM) without antihypertensives was 11.1 ± 2.6 |
Type 2 diabetes: normoalbuminuria | ||
Bilous 200928 | 954 normoalbuminuric patients without retinopathy or hypertension, followed for 6 years | Least squares mean of annual change in UAER = 0.69 µg/minute (95% CI 0.65 to 0.74) |
Type 2 diabetes: microalbuminuria | ||
Ravid 199330 | 45 microalbuminuric patients followed for 5 years | AER increased from a mean ± SD of 123 ± 58 mg/24 hours to a mean ± SD of 310 ± 167 mg/24 hours after 5 years |
Ahmad 199731 | 51 microalbuminuric patients followed for 5 years | AER increased from a mean ± SD of 76.3 ± 44.6 mg/24 hours to a mean ± SD of 122.4 ± 129.6 mg/24 hours after 5 years |
Christensen 198532 | 10 patients followed for 4.9 years | Annual increase in urinary albumin 19 ± 22 (mean ± SD) |
Study (first author, year) | Type of diabetes | Baseline urinary albumin | Measurement of progression | n |
---|---|---|---|---|
aVergouwe 201033 | 1 | Normoalbuminuria | Microalbuminuria defined as an AER of 20–200 g/minute, where the result is obtained as an average from two 24-hour urine collections | 1115 |
Kilpatrick 200834 | 1 | Normoalbuminuria | An increase in AER of ≥ 40 mg/24 hours (28 µg/minute) on any annual evaluation | 1441 |
aChaturvedi 200135 | 1 | Normoalbuminuria | AER between 20 and 200 µg/minute | 1134 |
Waden 200936 | 1 | Mixed | Shift to higher urinary albumin level, microalbuminuria defined as UAER ≥ 30 and < 300 mg/24 hours and macroalbuminuria defined as UAER ≥ 300 mg/24 hours in two of three consecutive timed measurements | 2107 |
RCPEDRG 200037 | 1 | Mixed | Geometric mean of two consecutive urinary albumin concentrations above 200 mg/l, or a single value above 1000 mg/l on a first void, morning, spot sample | 1164 |
Rossing 200238 | 1 | Mixed | Shift to higher urinary albumin level, microalbuminuria defined as UAER 31–299 mg/24 hours and macroalbuminuria defined as UAER ≥ 300 mg/24 hours in two of three consecutive timed measurements | 537 |
Retnakaran 200639 | 2 | Normoalbuminuria | Microalbuminuria defined as urinary albumin concentration of 50–299 mg/l | 4031 |
Amini 200740 | 2 | Normoalbuminuria | Microalbuminuria defined as 24-hour urinary albumin concentration of 30–300 mg | 505 |
Agrawal 201141 | 2 | Mixed | Normoalbuminuria defined as 0–29 mg/g, microalbuminuria 30–300 mg/g and microalbuminuria > 300 mg/g. Progression to next level after at least two measurements | 1791 |
Bruno 200342 | 2 | Mixed | 1253 | |
Xu 200843 | Not specified | Normoalbuminuria | Random morning urine sample, normoalbuminuria defined as ACR < 30 mg/g | 1330 |
Risk factor | Study (first author, year) | Type of diabetes | Baseline urinary albumin | HR/OR/RR/SERR | Effect size (95% CI) |
---|---|---|---|---|---|
HbA1c per 1% change in A1c | Kilpatrick 200834 | 1 | Normoalbuminuria | HR | 1.13 (1.05 to 1.22)a |
Chaturvedi 200135 | 1 | Normoalbuminuria | OR | 1.29 (1.14 to 1.47) | |
Rossing 200238 | 1 | Normo- and microalbuminuria | RR | 1.13 (1.04 to 1.23) | |
Waden 200936 | 1 | Normo- and microalbuminuria | HR | 1.34 (1.20 to 1.51)d | |
Retnakaran 200639 | 2 | Normoalbuminuria | HR | 1.08 (1.03 to 1.12) | |
Agrawal 201141 | 2 | Normo- and microalbuminuria | Not significant | ||
Bruno42 | 2 | Normo- and microalbuminuria | OR | 1.26 (0.78 to 2.03)g | |
Xu 200843 | NS | Normoalbuminuria | Not significant | ||
Fasting plasma glucose (per 10 mg/dl) | Xu 200843 | NS | Normoalbuminuria | OR | 1.01 (1.00 to 1.02) |
Male sex | Waden 200936 | 1 | Normo- and microalbuminuria | HR | 1.74 (1.30 to 2.33) |
Retnakaran 200639 | 2 | Normoalbuminuria | HR | 1.18 (1.01 to 1.39) | |
Xu 200843 | NS | Normoalbuminuria | Not significant | ||
Cholesterol | Chaturvedi 200135 | 1 | Normoalbuminuria | Not significant | |
Waden 200936 | 1 | Normo- and microalbuminuria | HR | 1.19 (1.04 to 1.36) | |
LDL cholesterol | Chaturvedi 200135 | 1 | Normoalbuminuria | Significantc | |
Xu 200843 | NS | Normoalbuminuria | Not significant | ||
HDL cholesterol | Chaturvedi 200135 | 1 | Normoalbuminuria | Significantc | |
Bruno 200342 | 2 | Normo- and microalbuminuria | OR | 0.54 (0.33 to 0.89)g | |
Xu 200843 | NS | Normoalbuminuria | Not significant | ||
Triglycerides per 10-fold increase | Chaturvedi 200135 | 1 | Normoalbuminuria | SERRb | 1.31 (1.05 to 1.65) |
Retnakaran 200639 | 2 | Normoalbuminuria | HR | 1.09 (1.04 to 1.14) | |
Xu 200843 | NS | Normoalbuminuria | Not significant | ||
UAE | |||||
Per microgram per minute | Chaturvedi 200135 | 1 | Normoalbuminuria | OR | 1.04 (1.01 to 1.08) |
Per 10-fold increase | Rossing 200238 | 1 | Normo- and microalbuminuria | RR | Log-UA: 2.63 (1.65 to 4.19) |
Per 20 mg/l | Retnakaran 200639 | 2 | Normoalbuminuria | HR | 1.004 (1.002 to 1.007) |
Log-ACR | Agrawal 201141 | 2 | Normo- and microalbuminuria | OR | 1.27 (1.11 to 1.45) |
Microalbuminuria | Bruno 200342 | 2 | Normo- and microalbuminuria | OR | 1.42 (0.98 to 2.06) |
ACR ≥ 10 vs. < 5 mg/g | Xu 200843 | NS | Normoalbuminuria | OR | 2.71 (1.89 to 3.90) |
Systolic blood pressure | Chaturvedi 200135 | 1 | Normoalbuminuria | Not significant | |
Waden 200936 | 1 | Normo- and microalbuminuria | HR | 1.01 (1.00 to 1.02) | |
Retnakaran 200639 | 2 | Normoalbuminuria | HR | 1.01 (1.01 to 1.02) | |
Bruno 200342 | 2 | Normo- and microalbuminuria | OR | 1.21 (0.67 to 2.18)g | |
Xu 200843 | NS | Normoalbuminuria | OR | 1.02 (1.01 to 1.04) | |
Diastolic blood pressure | Chaturvedi 200135 | 1 | Normoalbuminuria | Not significant | |
Agrawal 201141 | 2 | Normo- and microalbuminuria | Not significantf | ||
Current smoker | Chaturvedi 200135 | 1 | Normoalbuminuria | Not significante | |
Rossing 200238 | 1 | Normo- and microalbuminuria | RR | 1.61 (1.11 to 2.33) | |
Ever smoked | Waden 200936 | 1 | Normo- and microalbuminuria | HR | 1.22 (0.92 to 1.63) |
Retnakaran 200639 | 2 | Normoalbuminuria | HR | 1.20 (1.01 to 1.42) | |
Agrawal 201141 | 2 | Normo- and microalbuminuria | Not significant | ||
Xu 200843 | NS | Normoalbuminuria | OR | 1.49 (1.12 to 1.99) | |
Retinopathy | Chaturvedi 200135 | 1 | Normoalbuminuria | RR | 1.80 (1.10 to 2.80) |
Rossing 200238 | 1 | Normo- and microalbuminuria | RR | 1.90 (1.26 to 2.88) | |
Retnakaran 200639 | 2 | Normoalbuminuria | HR | 1.25 (1.05 to 1.49) | |
Agrawal 201141 | 2 | Normo- and microalbuminuria | Not significant | ||
Body mass index | Chaturvedi 200135 | 1 | Normoalbuminuria | Significante | |
Agrawal 201141 | 2 | Normo- and microalbuminuria | Not significantf | ||
Xu 200843 | NS | Normoalbuminuria | Not significant | ||
Waist-to-hip ratio | Chaturvedi 200135 | 1 | Normoalbuminuria | SERRb | 1.27 (1.02 to 1.50) |
Waist (cm) | Retnakaran 200639 | 2 | Normoalbuminuria | HR | 1.010 (1.004 to 1.016) |
HbA1C variability | Waden 200936 | 1 | Normo- and microalbuminuria | HR | 1.92 (1.49 to 2.47) |
Duration of diabetes | Chaturvedi 200135 | 1 | Normoalbuminuria | Not significant | |
Waden 200936 | 1 | Normo- and microalbuminuria | HR | 1.01 (0.99 to 1.02) | |
Agrawal 201141 | 2 | Normo- and microalbuminuria | Not significant | ||
Bruno 200342 | 2 | Normo- and microalbuminuria | OR | 1.39 (0.85 to 2.28)g | |
Xu 200843 | NS | Normoalbuminuria | Not significant | ||
Age | Chaturvedi 200135 | 1 | Normoalbuminuria | Not significant | |
At diagnosis | Retnakaran 200639 | 2 | Normoalbuminuria | HR | 1.01 (0.97 to 1.06) |
Agrawal 201141 | 2 | Normo- and microalbuminuria | Not significant | ||
Per 5 years | Xu 200843 | NS | Normoalbuminuria | Not significant | |
Ethnicity | Retnakaran 200639 | 2 | Normoalbuminuria | HR | 1.21 (0.89 to 1.65) (Afro-Caribbean); 2.02 (1.59 to 2.60) (Indian Asian) |
Agrawal 201141 | 2 | Normo- and microalbuminuria | Not significant | ||
Chandie Shaw 200644 (South Asian migrants) | 2 | OR | 3.9 (1.1 to 14) | ||
White cell count | Retnakaran 200639 | 2 | Normoalbuminuria | HR | 1.06 (1.02 to 1.10) |
Previous CVD | Retnakaran 200639 | 2 | Normoalbuminuria | HR | 1.46 (1.23 to 1.73) |
Plasma creatinine | Xu 200843 | NS | Normoalbuminuria | OR | 2.92 (1.27 to 6.69) |
History of photocoagulation | Agrawal 201141 | 2 | Normo- and microalbuminuria | Not significantf | |
History of cataract surgery | Agrawal 201141 | 2 | Normo- and microalbuminuria | Not significantf | |
Hypertension | Bruno 200342 | 2 | Normo- and microalbuminuria | OR | 0.67 (0.35 to 1.28) |
Use of antihypertensive medication | |||||
ACE inhibitors | Xu 200843 | NS | Normoalbuminuria | OR | 0.60 (0.38 to 0.95) |
Other antihypertensives | Xu 200843 | NS | Normoalbuminuria | OR | 1.15 (0.83 to 1.59) |
Type of diabetes therapy | |||||
Insulin | Xu 200843 | NS | Normoalbuminuria | OR | 2.35 (1.60 to 3.45) |
Oral medication | Xu 200843 | NS | Normoalbuminuria | OR | 2.02 (1.49 to 2.74) |
Fibrinogen | Bruno 300342 | 2 | Normo- and microalbuminuria | OR | 1.54 (0.92 to 2.58)g |
ApoB | Bruno 200342 | 2 | Normo- and microalbuminuria | OR | 1.34 (0.81 to 2.24)g |
The Oxford Regional Prospective Study of Childhood Diabetes (ORPS) has identified the importance of non-glycaemia-related factors in the development of microalbuminuria. In this young adult cohort of type 1 patients, there was no glycated haemoglobin (HbA1c) threshold below which microalbuminuria did not appear. 48
Risk factors affecting progression to microalbuminuria in type 2 diabetes
As with type 1 diabetes, glycaemia is one of the most important markers of progression to microalbuminuria in patients with type 2 diabetes. However, the effects of other adverse risk markers are more difficult to establish, as there are typically a number of coexistent risk factors in any one individual, with uncertainty about the period of exposure.
Evidence for the overall impact of glycaemia on progression to microalbuminuria is now available from a number of studies. In an epidemiological analysis of the UKPDS, the hazard ratio for a 1% increase in HbA1c was estimated as 1.08. 22 There is also strong epidemiological evidence that elevated systolic blood pressure is related to progression to microalbuminuria.
Ethnicity is a factor in determining progression to end-stage kidney disease. For example, the incidence rate of requiring renal replacement therapy for diabetic kidney disease for those of Asian ethnic origin was 486.6 [95% confidence interval (CI) 185.1 to 788.1] cases per million person-years per year, compared with 35.6 (95% CI 17 to 54.2) in white Caucasians. 49 Another survey found that people of Asian or Afro-Caribbean origin were almost six times as likely as white Caucasians to be receiving treatment for end-stage renal failure associated with diabetes. 50 In the UKPDS analysis of predictors of progression of microalbuminuria, the hazard ratio for Indian-Asian ethnicity was 2.0 in a multivariate model. 22
Other factors (with associated hazard ratios) found to predict progress to kidney disease noted in the UKPDS include previous retinopathy (1.25), previous CVD (1.46), male sex (1.18) and elevated triglycerides (1.09).
Search strategy and search results: risk factors for diabetic kidney disease
-
The PubMed database was searched to identify prospective cohort studies of ≥ 500 patients with diabetes that reported hazard ratios or odds ratios for risk factors for progression of UAE.
-
Multiple searches were conducted using medical subject heading terms and text words that included diabetes or diabetes mellitus, combined with relevant text key words such as diabetic nephropathy, urinary albumin, microalbuminuria, renal disease, renal outcomes and risk factors or progression.
-
In total, 89 potential records were identified, 11 of which were eligible for inclusion, and, of these, 6 specifically reported risk factors for progression from normoalbuminuria to microalbuminuria.
Impact of impaired renal function on long-term outcomes
Mortality in type 1 diabetes
Persistent microalbuminuria is a marker for a high risk of mortality. The importance of microalbuminuria is emphasised by data from long-term cohort studies which suggest that type 1 diabetes patients without kidney disease achieve long-term survival comparable to the general population. 51
Mortality in type 2 diabetes
Microalbuminuria is well established as a strong predictor of increased mortality in patients with type 2 diabetes compared with those with normoalbuminuria (see Tables 3–5). Since the excess risk was identified over 25 years ago,7,52 further studies have also characterised the risk of cardiovascular events and cancer. 53,54 Microalbuminuria confers an increased risk of death and cardiovascular morbidity or mortality with odds ratios of 2.4 and 2.0, respectively. 7 However, those patients who have no proteinuria 20–25 years after the onset of type 2 diabetes have a risk of developing overt kidney disease of only about 1% per year. In the UKPDS,22 cardiovascular deaths were 2.0% and 0.7%, respectively, for those with microalbuminuria and those with normoalbuminuria at 15 years after onset of type 2 diabetes.
In addition to the excess risk imposed by microalbuminuria, studies have confirmed that albuminuria and reduced eGFR are independently related to CVD event rates, cancer and non-CVD-related death in low-risk patients with type 2 diabetes. 7,52,55 Compared with those with normal renal status, these markers are predictive of a twofold increase in CVD risk in patients with an eGFR 30–59 ml/minute/1.73 m2 or macroalbuminuria.
Quantifying progression to chronic kidney disease is complicated by competing risks, particularly for patients with type 2 diabetes. CVD is the major cause of morbidity and mortality for patients with type 2 diabetes. Generally, if eGFR is < 30 ml/minute/1.73 m2, then there is a greater chance of death than progression to renal dialysis. Conversely, if eGFR is > 30 ml/minute/1.73 m2, then death from other causes is more likely than the patient reaching end-stage kidney disease.
The implications of an eGFR < 60 ml/minute/1.73 m2 for an individual aged < 75 years may differ from those for an individual > 75 years. Recent work has suggested that rates of morbidity and mortality among the older age group do not differ among those with an eGFR 45–60 ml/minute/1.73 m2 and those with an eGFR > 60 ml/minute/1.73 m2. Rates of morbidity and mortality rise only once eGFR falls below 45 ml/minute/1.73m2. 56 These findings support an approach to screening for chronic kidney disease in patients with diabetes that uses age to determine the cut-off for more intensive management, and aims to identify rapid decline in renal function rather than identify individuals > 75 years at increased risk without either microalbuminuria or an eGFR < 45 ml/minute/1.73 m2.
Interventions to prevent the development of microalbuminuria
The rationale for screening for microalbuminuria depends on the existence of an effective treatment for those identified as at increased risk, and the extent to which there is a difference in benefit between treating those identified and all patients. A series of large, interventional clinical trials has confirmed that intensive control of glycaemia and blood pressure among individuals with early diabetes reduces the occurrence of microalbuminuria. The DCCT trial demonstrated substantial reductions in the development of microalbuminuria with intensive glycaemic management. 45 Similarly, both the UKPDS57 and ADVANCE (Action in Diabetes and Vascular Disease: Preterax and Diamicron MR Controlled Evaluation)58 trials have confirmed that the treatment of patients with type 2 diabetes leads to a risk reduction with, for example, a relative reduction of 20% in onset of new microalbuminuria. 58 However, the extent to which treatment should differ between those with and without microalbuminuria is unclear.
Blood pressure, in particular, is a target for treatments that may have renal benefit. Conventional thresholds at which to initiate treatment to lower blood pressure are 130/80 and 125/75 mmHg for people with diabetes and those with diabetes and kidney disease, respectively. 59 Trials that evaluated lowering blood pressure below these thresholds, including UKPDS60 and ADVANCE,61 confirm that participants allocated to regimens targeting lower blood pressures had reduced risks of developing microalbuminuria. However, in the ACCORD (Action to Control Cardiovascular Risk in Diabetes) study, which targeted patients with more advanced diabetes and prior cardiovascular events, similar benefits were not seen when targeting a systolic blood pressure of 120 mmHg rather than 130 mmHg. 62
There is strong evidence that overactivity of the renin–angiotensin–aldosterone system (RAAS) is implicated in the deterioration of renal function in patients with diabetic kidney disease. Angiotensin-converting enzyme (ACE) and angiotensin 2 receptor blockers (A2RBs) slow deterioration of GFR and lower the rate of albumin excretion. The extent to which benefits occur at early stages of the disease is unknown. There is some evidence that suppression immediately reduces UAE rates, leading to a lower rate of progression of kidney disease,63 but, to date, a systematic review of the literature has not addressed this question.
We could not identify any studies that separately examine the impact of other specific interventions that are known predictors of the development of microalbuminuria, for example smoking cessation.
Risk reduction in microalbuminuria
Strategies for risk reduction in diabetes patients with microalbuminuria may be envisaged as falling into two broad and potentially overlapping categories. First, there may be therapies that are effective in reducing morbidity and mortality for any individual with diabetes; however, the overall absolute risk reduction may be greatest when individuals are already high risk because of their microalbuminuria. Second, there may be therapies for which the treatment effect differs in those with and without microalbuminuria.
Reducing blood pressure among those with microalbuminuria may result in a greater reduction in risk than a reduction in those without microalbuminuria. This raises issues of cost–benefit, requiring judgements about the benefit of absolute risk reduction and the adverse impacts of additional medication. Control of lipids and treatment goals are managed in the same way in the presence or absence of microalbuminuria. 64 Other strategies, such as low-protein diets, have been found to be effective only in moderate to severe kidney failure, not at early stages of kidney disease.
There is good evidence for targeting the overactivity of the RAAS with ACE inhibitors (ACEi) and A2RBs to reduce progression to kidney disease and reduce risks of cardiovascular events. However, the widespread use of ACEi in patients with type 2 diabetes (up to 50% of patients with diagnosed diabetes are treated for hypertension) indicates that, at least for some groups of patients, there may be limited opportunity for treatment optimisation. 64
Characteristics of tests for early and established kidney disease in diabetes
In routine clinical practice, two biochemical markers are widely used to screen for and monitor chronic kidney disease: urinary albumin (either 24-hour urine collection or a spot ACR) and eGFR, usually estimated from serum creatinine measurement.
The within-person variation of albumin excretion is large in people with diabetes, with the lowest within-person coefficient of variation (CV) found for the concentration of albumin in the first morning void (36%) and for the ACR (31%) (see Chapter 3 for a discussion of the implications of this biological variability). Assuming a CV of 5% for the measurement of urinary creatinine, a goal of 15% for the analytical CV for albumin is considered acceptable for estimating the ACR. 16
Test strip methods for microalbuminuria have been assessed and do not perform uniformly across all concentrations. In addition, there is a possibility of urinary dilution or other urinary proteins leading to false results. However, the routine use of low-cost, low-accuracy methods to determine whether a clinic visit might be necessary is an approach that might be explored. 65
The GFR is the volume of fluid filtered from the renal glomerular capillaries into the Bowman’s capsule per unit time. GFR predicts CVD, end-stage kidney disease and death. For routine clinical management, eGFR is calculated from serum creatinine, although these calculations are not valid for children. Serum creatinine and eGFR are continuous variables subject to biovariability and measurement error. The equations used to calculate eGFR from serum creatinine can result in the misclassification of some people as having early stage 3 chronic kidney disease.
The concentration of creatinine in peripheral blood is inversely associated with kidney function, usually measured using GFR. The rate of production of creatinine is proportional to muscle bulk, which varies among individuals, and thus age, ethnicity and body mass can also be used to estimate GFR (hence, eGFR) after measurement of serum creatinine. The two most commonly used equations to estimate GFR are the Cockcroft–Gault and MDRD formulae. The Cockcroft–Gault formula was developed in healthy individuals and is based on serum creatinine, weight and age. The MDRD equation was developed in American patients with renal insufficiency and is based on serum creatinine and age. The MDRD equation is recommended for reporting by UK laboratories. The formula is not well validated in the very old or ethnic minority groups other than African-Americans. In addition, its use is not valid for children or people with extremes of body size, muscle mass or nutritional status. As the relationship between serum creatinine and renal function is non-linear, the equations to estimate eGFR at more normal degrees of renal function are inaccurate. Laboratories reporting eGFR are encouraged not to report absolute values when eGFR is > 60 ml/minute/1.73 m2 (about half normal).
Models of care for identifying and treating kidney disease in diabetes
Strategies for surveillance of diabetic kidney disease are laid out in recent practice guidelines and recommendations. 15,19 When screening for and monitoring kidney disease, all patients with diabetes are asked to provide a first-pass morning urine specimen once a year. In the absence of proteinuria or urinary tract infection, this is sent for estimation of ACR. If a first-pass specimen is unavailable, then a spot sample should be used and, if abnormal, screening should be repeated with a first-pass specimen (although the U.S. Kidney Disease Outcomes Quality Initiative 200266 recommend routine use of a spot urine sample). A blood sample should be taken annually to measure the level of serum creatinine and estimate the GFR using the MDRD equation. 14 If an abnormal ACR is obtained, then at each of the next two clinic visits (within a maximum of 3–4 months) a further urine sample should be sent. Microalbuminuria is confirmed if either one of those repeat ACR tests is abnormal (> 2.5 mg/mmol for men or > 3.5 mg/mmol for women). 19
If early diabetic kidney disease is detected with persistent microalbuminuria, then the use of ACEi (weighing up risks with pregnant women) is recommended, with the alternative of using A2RBs if the ACEi is poorly tolerated. Blood pressure should be maintained below 130/80 mmHg (compared with the threshold of 140/80 mmHg for other diabetes patients). The British Renal Society recommends a referral threshold of eGFR of 30 ml/minute for patients with diabetes, or an average reduction in eGFR of > 4 ml/year.
In addition to the guidance offered by the National Institute for Health and Care Excellence (NICE), general practitioners (GPs) in the UK are offered incentives through the Quality Outcomes Framework to achieve targets for blood pressure measurement, albumin screening and treatment with ACEi. Despite these financial incentives for testing, the proportion of patients being tested for microalbuminuria in the last 15 months (2009/10) varies in England from 80% to 92% (www.nchod.nhs.uk).
Costs of screening for kidney disease in diabetes
The rationale for any screening programme requires that a net benefit can be achieved at a reasonable cost as a result of savings from improved outcomes. Recent guidance has noted the absence of health economic papers addressing the issue of screening for diabetic kidney disease. 19 There is, however, a basic model of the cost-effectiveness of urine albumin screening included in a report published in 2005 which compares the options of universal treatment with ACEi, annual screening and annual screening of only those not treated with ACEi. 4 The third option was marginally favoured over universal treatment without testing; however, the authors noted the extreme sensitivity of these findings to a range of assumptions and, in addition, did not address the potential of varying the interval of screening.
Modelling treatment strategies based on microalbuminuria status requires prediction and health economic models to estimate outcomes based on the presence or absence of microalbuminuria. Such models require a more detailed specification than the risk calculators used for predicting risk of cardiovascular disease or mortality. 67 To date, such models have not been available, for either type 1 or type 2 diabetes. However, some models are now available that predict the occurrence of microalbuminuria,33 drawing on data from large cohort studies and incorporating estimating equations based on renal function. However, the models available for type 1 diabetes do not incorporate estimates of outcomes by renal function, and the UKPDS outcomes model68 does not currently incorporate estimating equations derived from long-term follow-up data (see Chapter 5).
An additional difficulty of current approaches to estimating benefit from identifying people with early kidney disease is the individual variability of progression and the relatively poor precision of the tests. Longitudinal studies suggest that, although protein excretion tends, in general, to increase over time, the rate and direction of change varies between individuals. 69,70 Current approaches do not account for individual variability and thus fail to fully adjust for the possibility that more frequent testing may result in an increase in the number of false-positive tests. This may result in overestimates of the benefit of evaluated screening programmes.
Aims and objectives
The aim of the work reported in this monograph, and set out in our original research protocol (see Appendix 1), is to develop a clinical and economic model of renal function decline, and then to estimate the clinical effectiveness and cost-effectiveness of different screening models for early kidney disease in types 1 and 2 diabetes.
Research questions
There is now sufficient evidence, laid out in this introductory chapter, that treatments are available that reduce the risk of progressing from diabetic kidney disease to chronic kidney disease, in addition to modifying the associated risks of CVD. However, the differential benefit of these treatments in those identified with early kidney disease and those who do not have detectable kidney disease remains unclear.
Our objective is, therefore, to determine the clinical value and cost-effectiveness of screening programmes for early kidney disease in people with type 1 and type 2 diabetes, including current practice of annual screening. Specifically, our aim is to:
-
determine whether the renal benefit of ACEi or A2RB treatment varies by the presence or absence of early kidney disease (renal disease status) and type of diabetes (see Chapter 2);
-
quantify trends and variability in markers of kidney disease including eGFR and ACR (see Chapters 3 and 4);
-
estimate the proportion of diagnoses of diabetic kidney disease that are likely to be a result of measurement error and biovariability, rather than true change in underlying renal function (see Chapters 3 and 4); and hence
-
estimate cost-effectiveness ratios of screening programmes at various time intervals for kidney disease in patients with type 1 and type 2 diabetes (see Chapters 5 and 6).
Chapter 2 A systematic review of renin–angiotensin aldosterone inhibition using angiotensin-converting enzyme inhibitors and angiotensin-2 receptor blockers
Background
As discussed in the first chapter of this report, a successful screening programme requires evidence that treatment can improve outcomes for those detected with a problem, and provides no benefit, is not potentially harmful or is not unrealistically costly when applied to those not detected with a problem. This chapter, therefore, outlines the evidence needed to make judgements about the utility of a screening programme for detecting early kidney disease and, if beneficial, the optimal frequency for screening.
The RAASIs are of established benefit for preventing the progression of established diabetic kidney disease and the class of drugs includes ACEi and A2RBs. 71,72 This chapter looks at the evidence for the clinical effectiveness of ACEi and A2RB treatment. ACEi are currently recommended as a first-line treatment for reducing the development and progression of kidney disease, and preventing mortality and CVD in patients with type 1 and type 2 diabetes. A2RBs are recommended for patients who have an ACEi intolerance. With the detection of albuminuria in patients with diabetes, apart from reviewing the extent to which already-recommended treatments have been implemented, the use of ACEi or A2RBs to consistently lower blood pressure from below 140/80 to below 130/80 mmHg is the main therapeutic strategy. Microalbuminuria is defined as an elevated UAE (≥ 30 mg/24 hours). 71,72 This chapter, therefore, sets out the evidence for the clinical effectiveness of these medications in improving renal outcomes separately for those with and without microalbuminuria, and for those with type 1 and type 2 diabetes.
Methods
Search strategy
Trials published up to January 2005 were identified from the reference lists of two Cochrane systematic reviews on this topic. 63,64 Additional articles published between January 2005 and August 2010 were identified as follows: MEDLINE (January 2005 to August 2010), EMBASE (January 2005 to August 2010) and the Cochrane Controlled Trials Register (issue 1, 2005, to issue 8, 2010) were searched using the search strategy described in a previous Cochrane systematic review for diabetes and randomised controlled trials,63 as well as terms to identify diabetes, urinary albumin, ACEi or A2RBs (see Appendix 2). Reference lists of identified trials and relevant reviews were also searched.
Trial selection criteria
Included trials met the following criteria: (1) randomised controlled design; (2) outcomes for patients with type 1 and type 2 diabetes were reported separately; (3) the intervention used was either an ACEi or an A2RB; the comparator group was a placebo, no treatment or antihypertensive group (other than ACEi or A2RB); (4) trial duration was ≥ 6 months; and (5) at least one outcome was separately reported for patients with normoalbuminuria or micro-/macroalbuminuria and urinary albumin levels were reported, either as a timed albumin excretion rate, or as a spot test ACR. The outcomes evaluated were (1) UAE at the end of the trial; (2) progression to microalbuminuria (30–300 mg/24 hours); (3) progression to macroalbuminuria (> 300 mg/24 hours); (4) regression to normoalbuminuria (< 30 mg/24 hours); (5) change in GFR in ml/minute/1.73 m2; and (6) death.
Data extraction
Relevant publications were identified in duplicate and the data independently extracted. Disagreements were resolved by consensus with a third author. Structured forms were used for data reviewing extraction (see Appendix 3). Unpublished trial data were sought from authors of trials published since 2000. In trials that compared two doses of the same ACEi or A2RB with a comparator (placebo, no treatment or other antihypertensive), only the data from the higher dose were included in the meta-analysis to avoid double counting. 73
When multiarm trials had both placebo arms and active comparator arms (a non-ACEi/A2RB antihypertensive), we preferentially selected the antihypertensive arm as the comparator for the ACEi/A2RB arm. In a secondary analysis designed to inform our cost-effectiveness models (see Chapters 5 and 6), we also preferentially selected the active antihypertensive comparator arms from these trials for use in a metaregression of effect size on blood pressure difference. Trials that looked at both an ACEi and an A2RB in comparison with a placebo, no treatment or other antihypertensive treatment were included in the meta-analysis by splitting the data from the comparator group between the required comparisons.
Statistical methods
Statistical analysis was carried out using Stata version 11.1 (StataCorp, College Station, TX, USA). Data on progression to micro-/macroalbuminuria and regression to normoalbuminuria were analysed as dichotomous data using a Mantel–Haenszel fixed-effects model and specifying the risk ratio as the effect measure. The analysis of continuous urinary albumin data was based on an inverse variance method that also used a fixed-effects model. 73 For comparison, we report results and CIs from random-effects analyses based on the DerSimonian and Laird method. 74 A ‘ratio of means effect measure’ was used to allow us to combine the 35 trials that measured UAE using albumin excretion rate (mg/24 hours) with the five trials that reported ACR (mg/mmol or mg/g) into a single analysis. 75–77 We took the ratio of means to be the ratio of the final value in the intervention group to the final value in the comparator group, so that a ratio of means < 1 favours intervention. A comparison with standard analysis methods using mean difference and standardised mean difference verified that ratio of means was a suitable analysis method.
The following sensitivity analyses were carried out to verify that our results were not sensitive to these approximations, or to trials in which the comparator group was not an antihypertensive group; double-blinding was not clearly stated; it was not clearly stated that at least two measurements of urinary albumin were made to categorise patients with normo-, micro- and macroalbuminuria (applied both at baseline and end point); the number of participants was < 200. Statistical heterogeneity between studies was assessed with the I2 statistic. For the GFR data, we used an inverse variance, fixed-effect model and ratio of means. For a few studies, it was necessary to impute or approximate data. We undertook random-effects metaregression to evaluate the explanatory value of baseline urinary albumin (microalbuminuria vs. normoalbuminuria) and medication type (ACEi vs. A2RB) on effect size separately for type 1 and type 2 diabetes. Because a high degree of statistical heterogeneity was observed between studies in the analysis of continuous data, a post-hoc multivariate metaregression analysis was carried out to test whether the heterogeneity was explained by the following factors: study length; trial size; average participant age; country of trial; comparator type (no treatment, placebo or active comparator); medication type (ACEi or A2RB); treatment dose (categorised as high dose vs. low dose based on usual dose in the British National Formulary and excluding one study of a drug not licensed in the UK; temocapril, Acecol, Sankyo); difference in baseline UAE between the treatment and comparator groups, whether or not approximations were made; or year of trial publication. In addition, the effect of comparator type (placebo or no treatment vs. another antihypertensive) on effect size was investigated using subgroup analyses and metaregression.
Univariate and multivariate metaregression were carried out using the metareg routine in Stata version 11.1, applying the method of DerSimonian and Laird. 74 Heterogeneity was estimated from the Mantel–Haenszel model,73 and reported using the I2 statistic.
Because subgroup analyses of trials comparing ACEi/A2RB with other antihypertensives suggested an effect of ACEi/A2RB on renal outcomes beyond that attributable to blood pressure reduction, we also conducted a metaregression of effect size on between-arm blood pressure difference and used this to inform the cost-effectiveness modelling in Chapters 5 and 6.
Results
Description of studies
The reference lists of two Cochrane systematic reviews identified 65 trials, 38 of which were assessed for full eligibility (a selection of the excluded studies are listed in Appendix 4). Of these, three trials could not be included in the meta-analysis because they measured urinary protein instead of urinary albumin. 78–80 A fourth trial was excluded because consistent data could not be extracted,81 leaving 34 trials which fully met our inclusion criteria. In addition, 7849 articles were identified from the search of MEDLINE, EMBASE and The Cochrane Library from January 2005 to August 2010. Of these articles, 1450 were duplicates (Figure 3), leaving 6399 articles for review. After screening the titles and abstracts, 671 articles were examined in more detail for eligibility. A further 659 articles were excluded, leaving 12 papers (15 trials) that met our inclusion criteria.
FIGURE 3.
Flow diagram of literature search.
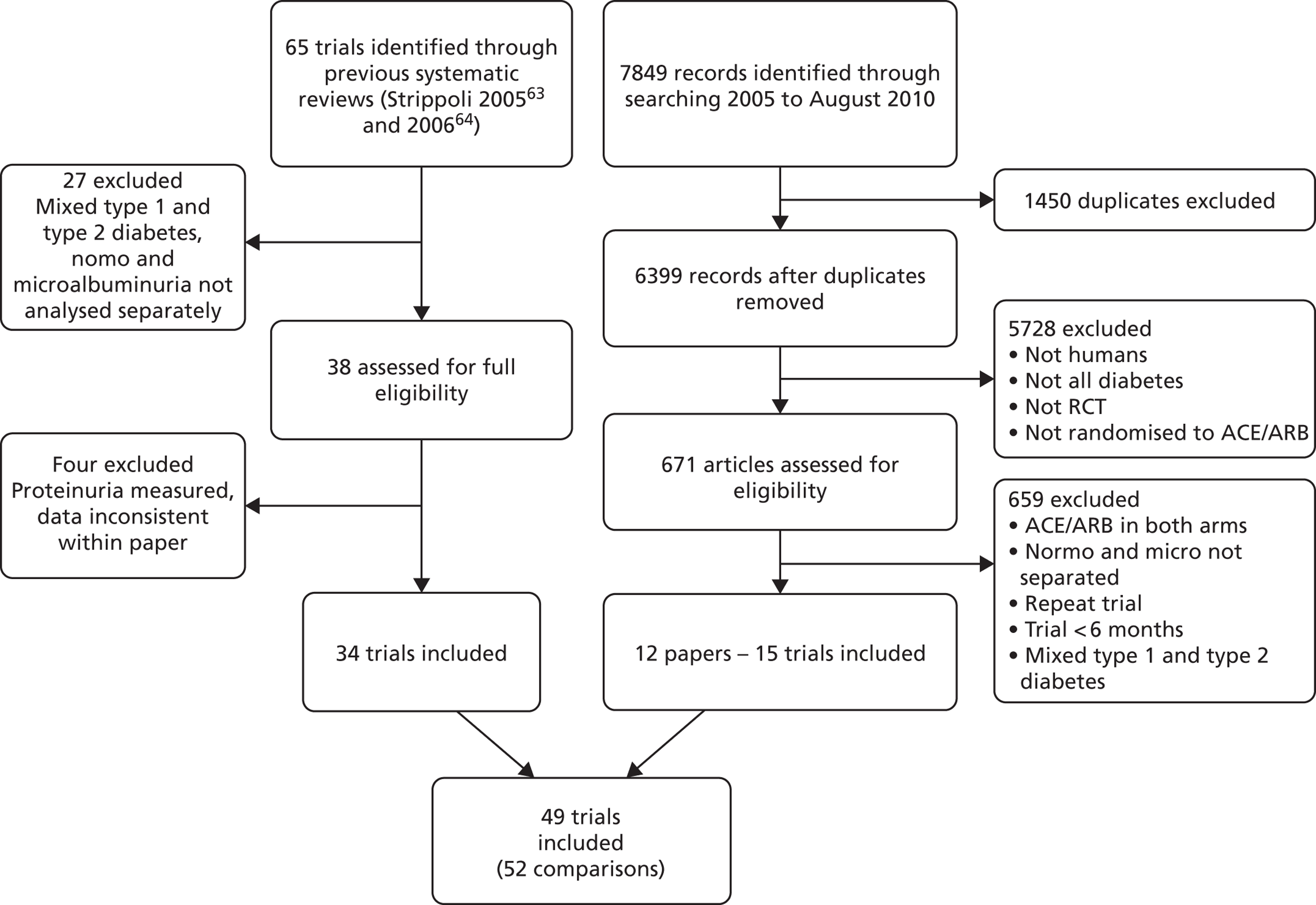
A total of 49 trials (representing 34,082 patients) were therefore included in the meta-analysis, three of which had multiple arms,82–84 which were included as separate comparisons, resulting in a total of 52 comparisons (see Figure 3). Six trials were included in the analysis of patients with type 1 diabetes and normoalbuminuria (seven comparisons). A total of 15 trials were included in the analysis of patients with type 1 diabetes and micro- or macroalbuminuria. As one trial was included for both the normoalbuminuria and the microalbuminuria groups,85 this gives a total of 20 trials of type 1 diabetes patients. In the analysis of patients with type 2 diabetes and normoalbuminuria, 10 trials were included; 22 trials were included in the analysis of patients with type 2 diabetes and micro- or macroalbuminuria (24 comparisons). As four trials were included in both the normoalbuminuria and microalbuminuria groups, this gives a total of 28 included trials for type 2 diabetes. 61,86–88 One trial could not be included in the analysis because only one patient was left in the comparator group at the end of the trial. 89
Of the 21 trials of RAASI carried out in people with type 1 diabetes, only 3 used A2RBs (Table 6),82,88 all of which were carried out on patients with normoalbuminuria. The remaining trials of type 1 diabetes patients used ACEi. As regards type 2 diabetes, three trials of normoalbuminuric patients used A2RBs, and nine trials of microalbuminuric patients used A2RBs; the remaining trials all used ACEi (see Table 6).
Study (first author, year) | Country/region | Participants (n) | Previous hypertension | Medication titration to achieve BP control | Intervention | Participants randomised to intervention (n) | Comparator | Participants randomised to comparator (n) | Patients followed up | Outcomes reported | Duration of follow-up | |
---|---|---|---|---|---|---|---|---|---|---|---|---|
Intervention (n) | Comparator (n) | |||||||||||
Type 1 diabetes – normoalbuminuria | ||||||||||||
EUCLID 199785 | Europe | 440a | No | Yes | Lisinopril (10 mg) | 213 | Placebo | 227 | 191 | 193 | UAE, progression of UAE | 2 years |
Tuominen 199890 | Finland | 26 | No | Yes | Lisinopril (15 mg) | 13 | Placebo | 13 | 11 | 13 | UAE, progression of UAE | 2 years |
Kvetny 200191 | Denmark | 89 | No | NS | Perindopril (2 mg) | 43 | Placebo | 46 | 35 | 40 | UAE, progression of UAE | 3 years |
DIRECT 1 2009 (Prevent)28 | International | 1905 | No | Yes | Candesartan (16 mg) | 951 | Placebo | 954 | 951 | 951 | UAE, death, progression of UAE | 1–5 years |
1421 | No | Yes | Candesartan (16 mg) | 711 | Placebo | 710 | 710 | 710 | UAE, death, progression of UAE | 1–5 years | ||
Mauer 2009 (ACE)82 | USA/Canada | 189 | No | NS | Enalapril (50 mg) | 94 | Placebo | 95 | UAE, death, progression of UA | 5 years | ||
Mauer 2009 (A2RB)82 | USA/Canada | 191 | No | NS | Losartan (10 mg) | 96 | Placebo | 95 | UAE, death, progression of UAE | 5 years | ||
Type 1 diabetes – microalbuminuria | ||||||||||||
Garg 199892 | USA | 11 | No | NS | Ramipril (5 mg) | 7 | Placebo | 4 | 7 | 4 | UAE | 1 year |
Bakris 199493 | USA | 15 | No | Yes | Lisinopril | 8 | Placebo | 7 | UAE | 18 months | ||
ATLANTIS 200094 | UK | 93 | No | No | Ramipril (5 mg) | 45 | Placebo | 48 | 28 | 37 | UAE, GFR | 2 years |
Bojestig 200195 | Sweden | 36 | No | No | Ramipril (5 mg) | 18 | Placebo | 18 | 16 | 18 | UAE | 2 years |
Chase 199396 | USA | 16 | No | No | Captopril (100 mg) | 7 | Placebo | 9 | 6 | 9 | UAE, progression/regression of UAE | 2 years |
EUCLID 199785 | Europe | 85a | No | Yes | Lisinopril (10 mg) | 49 | Placebo | 36 | UAE | 2 years | ||
Laffel 199597 | USA | 143 | No | Yes | Captopril (100 mg) | 70 | Placebo | 73 | 67 | 70 | UAE | 2 years |
Poulsen 200198 | Denmark | 21 | No | NS | Lisinopril (20 mg) | 11 | Placebo | 10 | UAE | 2 years | ||
Viberti 199499 | Europe/Asia | 92 | No | Yes | Captopril (100 mg) | 46 | Placebo | 46 | UAE, progression of UAE, GFR | 2 years | ||
Jerums 2001100 | Australia | 23 | No | Yes | Perindopril (8 mg) | 13 | Nifedipine (20–80 mg) | 10 | 13 | 10 | UAE, progression/regression of UAE, GFR | 2–3 years |
Lewis 1993101 | USA | 409 | Some | Yes | Captopril (75 mg/day) | 207 | Placebo | 202 | 207 | 202 | Death | 3 years |
Crepaldi 1998102 | Italy | 58 | No | Yes | Lisinopril (20 mg) | 47 | Nifedipine (20 mg) | 41 | 32 | 26 | UAE, progression/regression of UAE | 3 years |
Mathiesen 1999103 | Denmark | 44 | No | Yes | Captopril (100 mg) | 21 | No treatment | 23 | 21 | 23 | UAE, progression of UAE, GFR | 4 years |
Ahmad 2003104 | India | 73 | No | Yes | Enalapril (10 mg) | 37 | Placebo | 36 | UAE, progression of UAE, GFR | 5 years | ||
Parving 2001105 | Denmark | 33 | No | Yes | Captopril (74 mg) | 16 | No treatment | 17 | 15 | 17 | UAE, GFR | 8 years |
Type 2 diabetes – normoalbuminuria | ||||||||||||
aSawaki 200888 | Japan | 20 | NS | No | Losartan (25 mg) | 8 | No treatment | 12 | UAE | 1 year | ||
aBaba 200186 | Japan | 201 | Yes | Yes | Enalapril (5 mg) | 95 | Nifedipine (20 mg) | 106 | 65 | 70 | UAE, progression of UAE | 2 years |
aLebovitz 199480 | USA | 39 | Yes | Yes | Enalapril (5–40 mg) | 19 | Placebo | 20 | 18 | 19 | UAE, progression of UAE, GFR | 3 years |
BENEDICT 2004106 | Italy | 534 | Yes | Yes | Trandolapril (2 mg) | 301 | Verapamil | 303 | 272 | 262 | UAE, progression of UAE, death | 3 years |
ROADMAP 2011107 | Europe | 4440 | Yes – 90% | Yes | Olmesartan (40 mg) | 2220 | Placebo | 2220 | 2220 | 2220 | Progression of UAE, death | 3.2 years |
aADVANCE 2007, 200961,108,109 | International | 7986 | No BP criteria | Yes | Perindopril-(2–4 mg) | 3995 | Placebo | 3991 | UAE, progression of UAE | 4.3 years | ||
DIRECT Protect 2 200928 | International | 1905 | Normotensive or treated | Yes | Candesartan (16–32 mg) | 951 | Placebo | 954 | 951 | 954 | UAE, progression of UAE, death | 4.7 years |
aChan 200087 | China | 43 | Yes | Yes | Enalapril (10–20 mg) | 18 | Nifedipine (40–80 mg) | 25 | 18 | 25 | UAE, progression of UAE | 5.5 years |
Ravid 1998110 | Israel | 194 | No | Yes | Enalapril (10 mg) | 97 | Placebo | 97 | 77 | 79 | UAE | 6 years |
aUKPDS 1998111 | UK | 299 | Yes | Yes | Captopril | 153 | Atenolol | 146 | Progression of UAE | 9 years | ||
Type 2 diabetes – microalbuminuria | ||||||||||||
Romero 1993112 | Spain | 26 | No | Yes | Captopril (12.5 mg) | 13 | Placebo | 13 | 13 | 13 | UAE, GFR | 6 months |
SMART 2007113 | Japan | 150 | Yes | Yes | Valsartan (80–160 mg/day) | 73 | Amlodipine (5–10 mg/day) | 77 | UAE | 6 months | ||
Tan 2002114 | China | 80 | No + controlled hypertension | Yes | Losartan (50 mg) | 40 | Placebo | 40 | 40 | 40 | UAE | 6 months |
Trevisan 1995115 | Italy | 122 | ≤ 180 mmHg | No | Ramipril (1.25 mg) | 60 | Placebo | 62 | 54 | 54 | UAE | 6 months |
Rodríguez-Morán 2005116 | Mexico | 130 | No | ns | Captopril (25 mg) | 65 | Pentoxifylline (400 mg) | 65 | 61 | 62 | UAE | 6 months |
de Mello 2008117 | Brazil | 32 | Some | Yes | Enalapril (10 mg) | 16 | Diet | 16 | 15 | 13 | UAE, GFR | 1 year |
Muirhead 1999 (ACE)83 | Canada | 60 | ≤ 160 mmHg | Yes | Captopril (75 mg) | 29 | Placebo | 31 | 29 | 28 | UAE, GFR | 1 year |
Muirhead 1999 (A2RB)83 | Canada | 62 | ≤ 160 mmHg | Yes | Valsartan (160 mg) | 31 | Placebo | 31 | 31 | 28 | UAE, GFR | 1 year |
Puig 2007118 | International | 187 | Some | Yes | Enalapril (10 mg) | 92 | Indapamide (1.5 mg) | 95 | 92 | 95 | UAE | 1 year |
Sawaki 200888 | Japan | 9a | Some | No | Losartan (25 mg) | 6 | No treatment | 3 | UAE, GFR | 1 year | ||
Makino 2007119 | Japan | 342 | Some | Yes | Telmisartan (80 mg) | 168 | Placebo | 174 | 168 | 174 | UAE | 1.3 years |
Tong 2006120 | Hong Kong | 38 | Some | Yes | Fosinopril (10–20 mg) | 18 | Placebo | 20 | 11 | 10 | UAE | 1.4 years |
Ogawa 2007 (ACE)84 | Japan | 52 | Yes | Yes | Temocapril (2–4 mg) | 34 | Nifedipine (20–40 mg) | 18 | UAE, progression/regression of UAE | 1.8 years | ||
Ogawa 2007 (A2RB)84 | Japan | 58 | Yes | Yes | Candesartan (4–8 mg) | 40 | Nifedipine (20–40 mg) | 18 | UAE, progression/regression of UAE | 1.8 years | ||
Baba 200186 | Japan | 117 | Yes | Yes | Enalapril (5 mg) | 53 | Nifedipine (20 mg) | 64 | 35 | 41 | UAE, progression/regression of UAE, death | 2 years |
Parving 2001121 | International | 395 | Yes | Yes | Irbesartan (300 mg) | 194 | Placebo | 201 | 194 | 201 | UAE, progression/regression of UAE | Median 2 years |
Berl 2003122 | USA | 1148 | Yes | Yes | Irbesartan (300 mg) | 579 | Placebo | 569 | Death | 2.6 years | ||
RENAAL 2001123 | International | 1513 | Some | Yes | Losartan (50–100 mg) | 751 | Placebo | 762 | 306 | 269 | UAE, death | 3.4 years |
Marre 2004124 | International | 4912 | Some | Yes (including ACE/A2RB) | Ramipril (1.25 mg) | 2443 | Placebo | 2469 | 2443 | 2469 | Death | 3.9 years |
Sano 1996125 | Japan | 56 | No | NS | Enalapril (5 mg) | 28 | Control | 28 | 28 | 28 | UAE, GFR | 4 years |
ADVANCE 2007, 200961,108,109 | International | 3263 | No BP requirements | Yes | Perindopril (2–4 mg) + Indapamide | 1638 | Placebo | 1625 | UAE, progression/regression of UAE | 4.3 years | ||
Ahmad 199731 | India | 103 | No | Yes | Enalapril (10 mg) | 52 | Placebo | 51 | 52 | 51 | UAE, progression of UAE | 5 years |
Ravid 199330 | Israel | 94 | No | Yes | Enalapril (10 mg) | 49 | Placebo | 45 | 49 | 45 | UAE | 5 years |
Chan 200087 | China | 59 | Yes | Yes | Enalapril (10 mg) | 32 | Nifedipine (40 mg) | 27 | 21 | 13 | UAE, progression/regression of UAE, death | 5.5 years |
All trials of type 1 diabetes included normotensive or controlled hypertensive patients, and the majority of trials allowed patients to take antihypertensive medications (other than RAASI) (see Table 6). In contrast to this, nearly all trials in type 2 diabetes and normoalbuminuria included hypertensive patients, whereas 6 of the 21 trials in type 2 diabetes patients with microalbuminuria specifically selected hypertensive patients (see Table 6). Only one trial in type 1 diabetes,85 and two trials in type 2 diabetes,86,88 directly compared patients with normo- and microalbuminuria.
Trials of type 1 diabetes
The ratio of mean UAE in treatment versus comparator groups at the end of the trial for type 1 diabetes is shown in Figure 4. In patients normoalbuminuric at baseline, the ratio of means was 0.96 (95% CI 0.94 to 0.99) in the fixed-effects model (inverse variance) and 0.94 (95% CI 0.79 to 1.12) in the random-effects model (DerSimonian and Laird). This equated to a 4% and 6% lower UAE in the treatment group versus the comparator groups, respectively. In microalbuminuric patients, the ratio of means was 0.40 (95% CI 0.36 to 0.44) using a fixed-effects model (60% lower) and 0.33 (95% CI 0.23 to 0.46) using a random-effects model (67% lower).
FIGURE 4.
Ratio of mean UAE in treatment and comparator arms of trials. Ratio of mean UAE at the end of trials of RAASI treatment versus comparator (boxes) and pooled estimates across trials (diamonds) calculated by the fixed-effects inverse variance (I-V) method and by the DerSimonian and Laird random-effects (D+L) method, in patients with (a) type 1 and (b) type 2 diabetes, stratified by baseline urine albumin status. Horizontal bars and diamond widths denote 95% CIs and box sizes indicate relative weight in the I-V analysis. ATLANTIS, ace-inhibitor trial to lower albuminuria in normotensive insulin-dependent subjects; BENEDICT, Bergamo nephrologic diabetes complications trial; CCB, calcium channel blocker; DIRECT, DIabetic REtinopathy Candesartan Trials; EUCLID, a study comparing cardiovascular effects of ticagrelor and clopidogrel in patients with peripheral artery disease; NT, no treatment; RENAAL, reduction in end points in noninsulin-dependent diabetes mellitus with the angiotensin II antagonist losartan; ROADMAP, randomized olmesartan and diabetes microalbuminuria prevention; SMART, shiga microalbuminuria reduction trial.
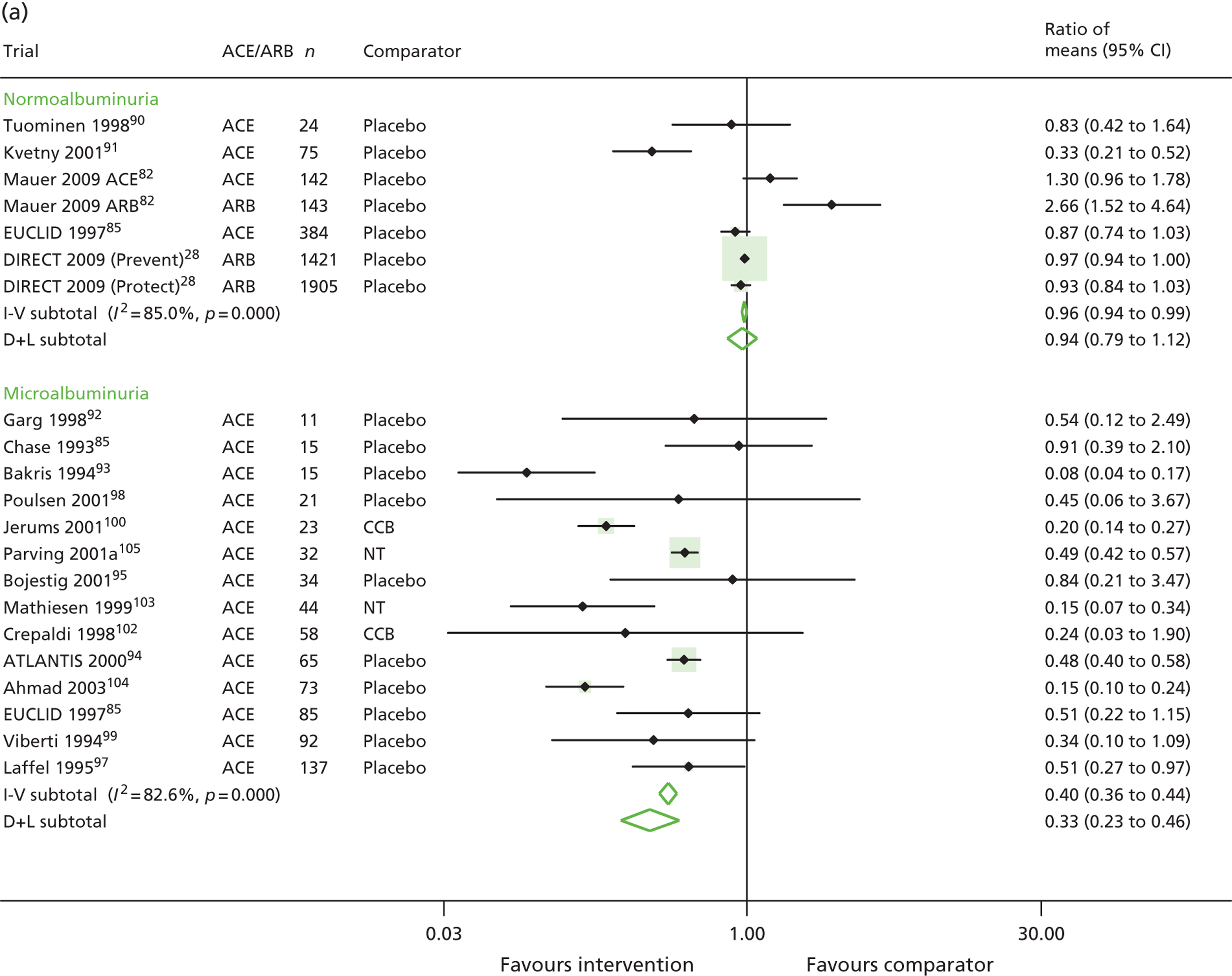
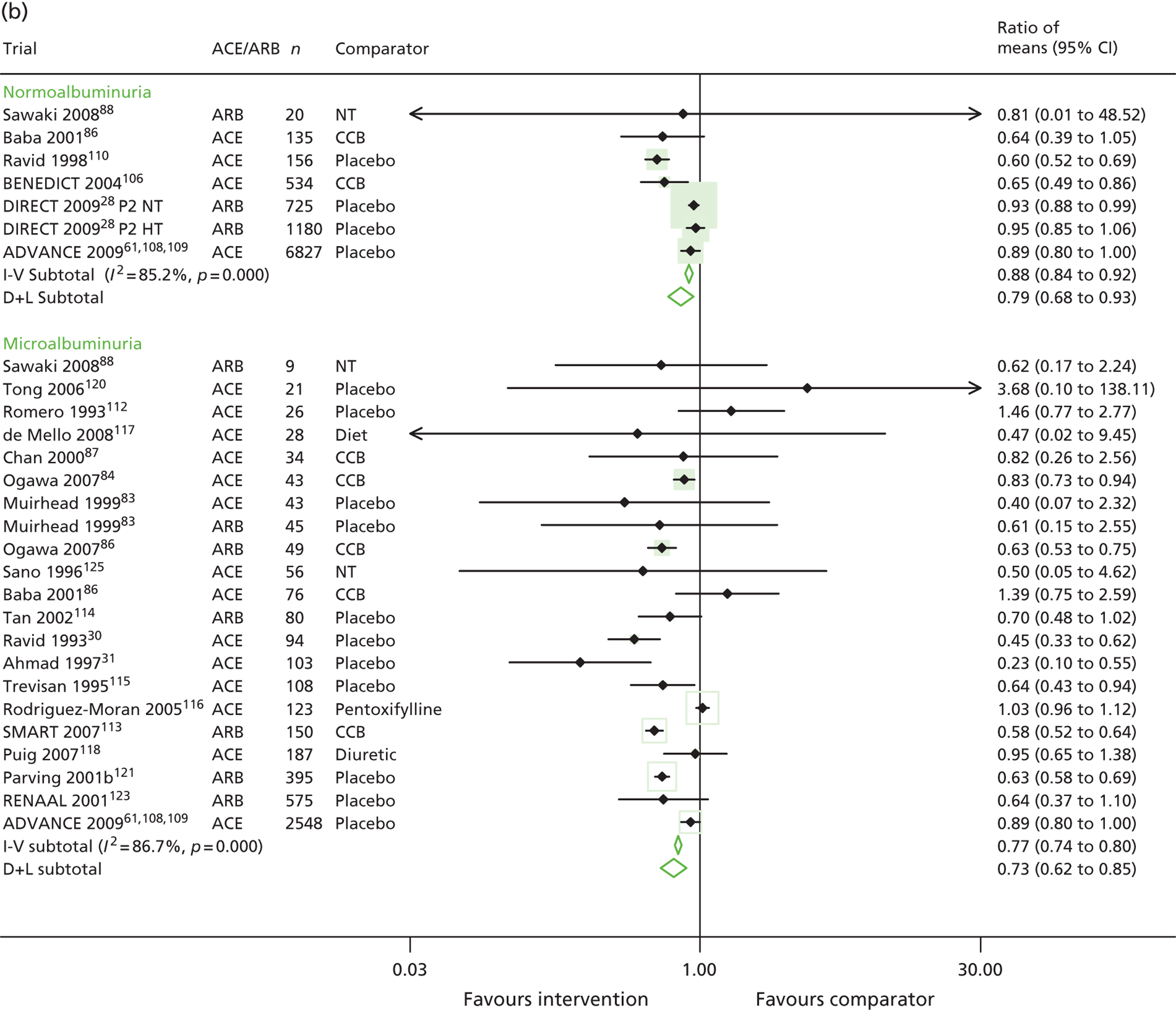
A subgroup analysis by comparator type was conducted to explore the difference between RAASI and an antihypertensive comparator, and RAASI and placebo versus no treatment. These subgroup analyses were not possible in patients with normoalbuminuria, as all trials used placebo as the comparator. In trials of microalbuminuric patients, subgroup analyses gave a ratio of means of 0.44 (95% CI 0.39 to 0.48) in 12 placebo or no treatment trials, and a ratio of means of 0.20 (95% CI 0.14 to 0.27) in two active comparator trials. This and other sensitivity analyses are listed in Table 7.
Analysis | Type 1 normoalbuminuria | Type 1 microalbuminuria | Type 2 normoalbuminuria | Type 2 microalbuminuria | ||||
---|---|---|---|---|---|---|---|---|
Number of trials | Ratio of mean UAE levels (95% CI)a | Number of trials | Ratio of mean UAE levels (95% CI)a | Number of trials | Ratio of mean UAE levels (95% CI)a | Number of trials | Ratio of mean UAE levels (95% CI)a | |
Main analysis (Figure 2) | 7 | 0.96 (0.94 to 0.99) | 14 | 0.40 (0.36 to 0.44) | 7 | 0.88 (0.84 to 0.92) | 20 | 0.77 (0.74 to 0.80) |
0.94 (0.79 to 1.12) | 0.33 (0.23 to 0.46) | 0.79 (0.68 to 0.93) | 0.73 (0.62 to 0.86) | |||||
Trials with antihypertensive comparator group | – | – | 0.20 (0.14 to 0.27) | 0.65 (0.51 to 0.83) | 0.82 (0.78 to 0.86) | |||
– | – | 2 | 0.20 (0.14 to 0.27) | 2 | 0.65 (0.51 to 0.83) | 7 | 0.82 (0.64 to 1.06) | |
Trials with duplicate urinary albumin measurements for categorising urinary albumin status of patients | 0.96 (0.94 to 0.99) | 0.36 (0.31 to 0.41) | 0.88 (0.84 to 0.92) | 0.69 (0.65 to 0.72) | ||||
7 | 0.94 (0.79 to 1.12) | 12 | 0.35 (0.23 to 0.53) | 5 | 0.81 (0.69 to 0.95) | 15 | 0.69 (0.60 to 0.79) | |
Trials with double-blinding | 0.97 (0.94 to 0.99) | 0.43 (0.37 to 0.50) | 0.88 (0.84 to 0.92) | 0.71 (0.66 to 0.75) | ||||
6 | 0.95 (0.79 to 1.14) | 10 | 0.43 (0.28 to 0.65) | 5 | 0.81 (0.69 to 0.95) | 11 | 0.69 (0.57 to 0.83) | |
Trials n ≥ 200 | 0.96 (0.94 to 0.99) | – | – | 0.92 (0.88 to 0.96) | 0.72 (0.67 to 0.77) | |||
3 | 0.96 (0.94 to 0.99) | – | – | 4 | 0.90 (0.83 to 0.98) | 3 | 0.73 (0.54 to 0.98) |
Trials of type 2 diabetes
The ratio of mean UAE in treatment versus comparator groups at the end of the trial for type 2 diabetes is shown in Figure 4. In patients normoalbuminuric at baseline, the ratio of means was 0.88 (95% CI 0.84 to 0.92) using a fixed-effects model (inverse variance) and 0.79 (95% CI 0.68 to 0.93) using a random-effects model (DerSimonian and Laird). This equated to a 12% and 21% lower UAE in the treatment group than in the comparator group, respectively. In microalbuminuric patients, the ratio of means was 0.77 (95% CI 0.74 to 0.80) using a fixed-effects model and 0.73 (95% CI 0.62 to 0.85) using a random-effects model (23% and 27% lower, respectively).
For patients with normoalbuminuria, a subgroup analysis by comparator type gave a ratio of means of 0.89 (95% CI 0.85 to 0.93) in five placebo or no treatment trials, and a ratio of means of 0.65 (95% CI 0.51 to 0.83) in two active comparator trials. The subgroup analysis of trials of microalbuminuric patients gave a ratio of means of 0.70 (95% CI 0.66 to 0.75) in 13 placebo or no treatment trials, and a ratio of means of 0.82 (95% CI 0.78 to 0.86) in 7 active comparator trials. A sensitivity analysis, restricted to trials of at least 200 patients, showed that effects remained statistically significant in trials of normoalbuminuric patients, but were smaller in magnitude (summary ratio of means 0.92, 95% CI 0.88 to 0.96). These sensitivity analyses are listed in Table 7.
Other outcomes
Type 1 diabetes
Results for progression and regression of albuminuria are presented in Figure 5. RAASI treatment of normoalbuminuric patients led to no significant difference in the number of patients who progressed to microalbuminuria, with a relative risk of 0.96 (95% CI 0.76 to 1.23, p = 0.25, I2 = 24%). All trials using ACEi favoured the treatment group, although the effect was not significant. In contrast, all trials using A2RBs favoured the comparator group, although, again, not significantly. ACEi treatment of patients with microalbuminuria resulted in fewer progressing to macroalbuminuria (UAE > 300 mg/24 hours), with a relative risk of 0.39 (95% CI 0.23 to 0.64, p = 0.0005, I2 = 0%). Treatment with ACEi also resulted in more patients regressing from microalbuminuria to normoalbuminuria, with a relative risk of 5.81 (95% CI 2.05 to 16.43, p = 0.001, I2 = 0%). In those studies in which it was assessed, there was no significant effect of treatment on mortality (see Figure 5) or GFR (data not shown).
FIGURE 5.
Relative risk of progression and regression of albuminuria in treatment and comparator arms of trials in type 1 diabetes. Relative risk of progression and regression of albuminuria during trials of RAASI treatment vs. comparator (boxes) and pooled estimates across trials (diamonds) calculated by the fixed-effects inverse variance (I-V) method and by the DerSimonian and Laird random-effects (D+L) method, in patients with type 1 diabetes, stratified by baseline urine albumin status. Horizontal bars and diamond widths denote 95% CIs and box sizes indicate relative weight in the I-V analysis. (a) Progression from normo- to microalbuminuria; (b) progression from micro- to macroalbuminuria; and (c) regression from micro- to normoalbuminuria. ATLANTIS, ace-inhibitor trial to lower albuminuria in normotensive insulin-dependent subjects; DIRECT, DIabetic REtinopathy Candesartan Trials; EUCLID, a study comparing cardiovascular effects of ticagrelor and clopidogrel in patients with peripheral artery disease.
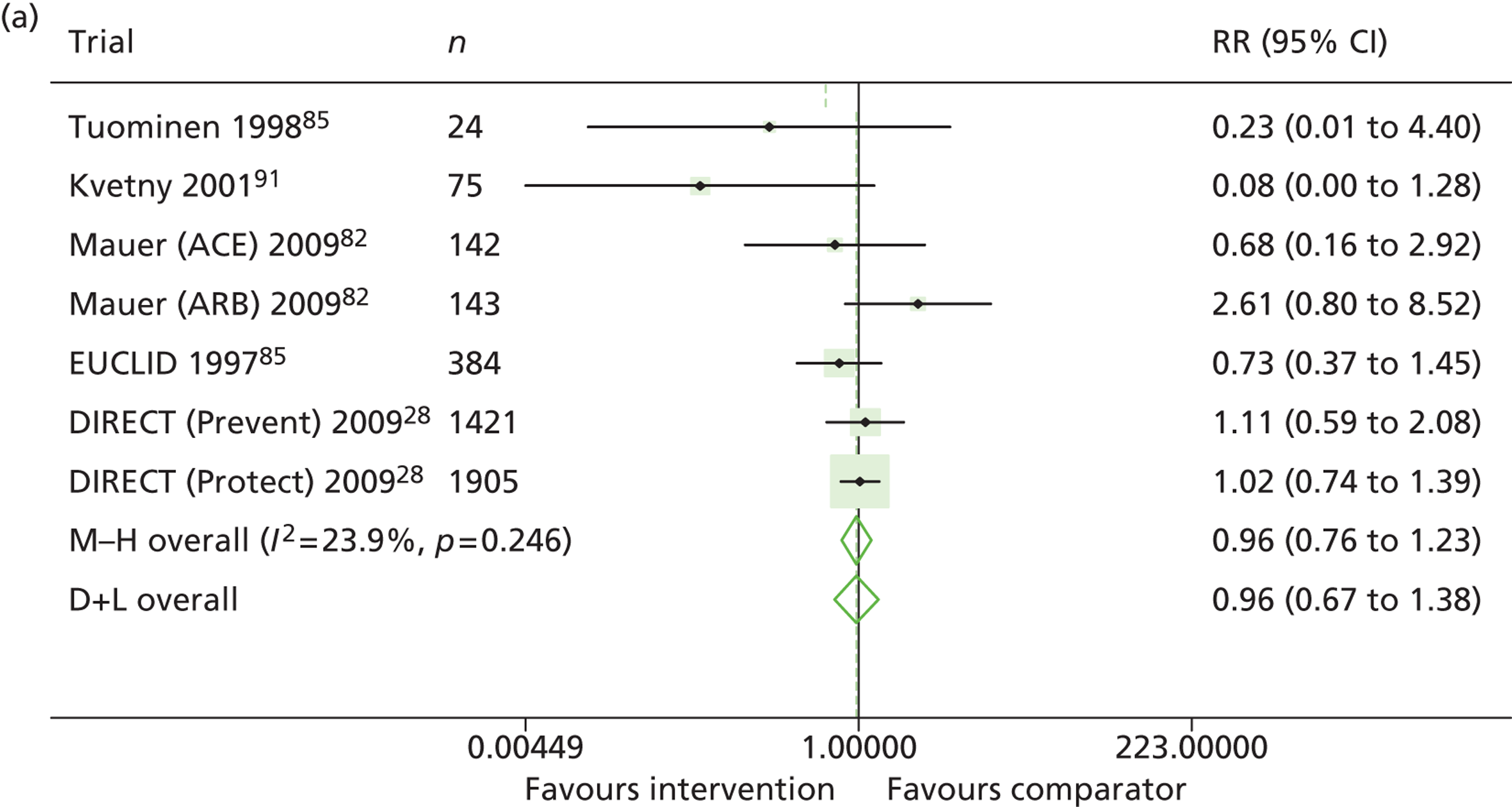
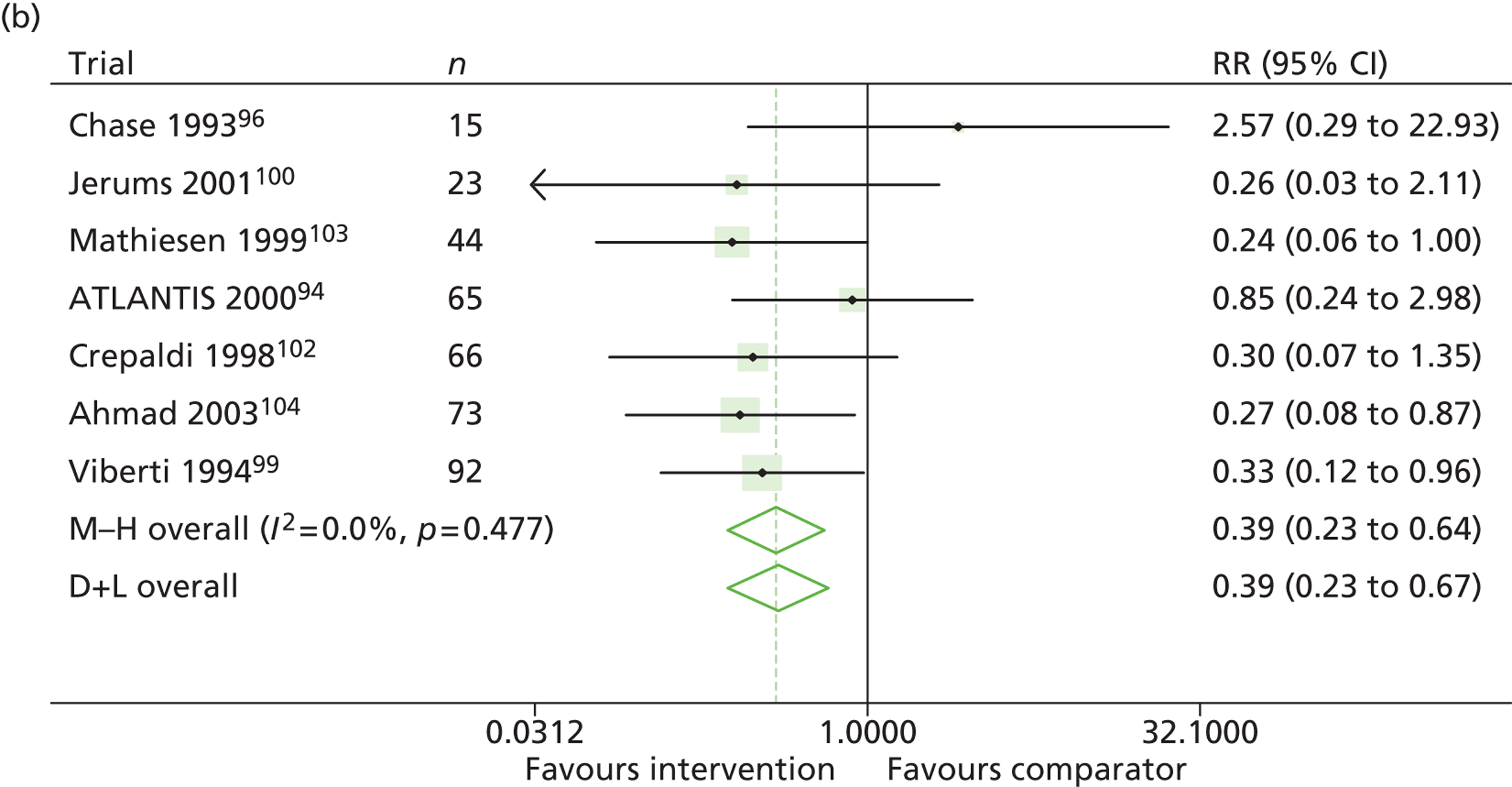
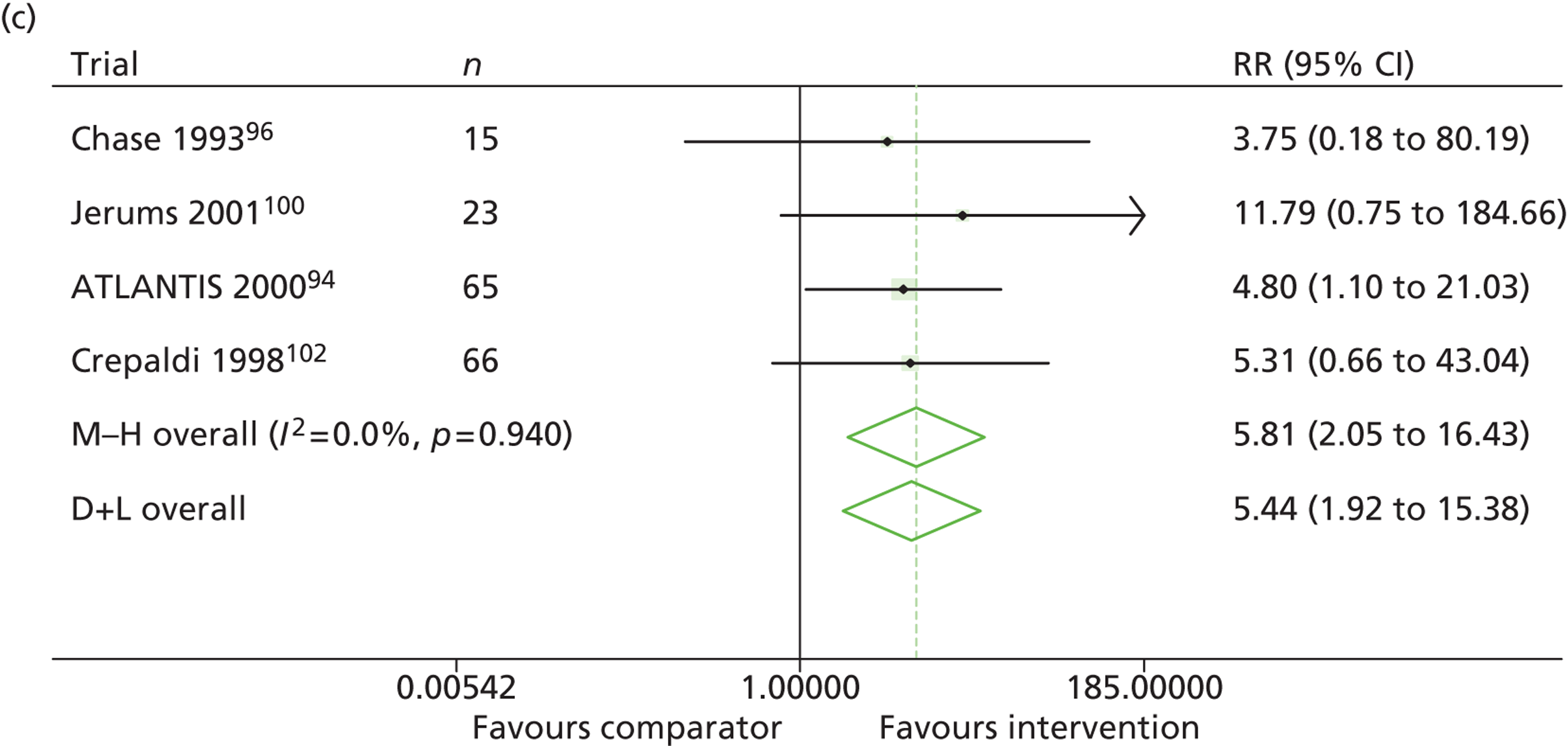
Type 2 diabetes
Results for progression and regression of albuminuria are presented in Figure 6. Patients with both normo- and microalbuminuria benefited from treatment with RAASI in terms of progression or regression of urinary albumin, but the effect was larger for patients with microalbuminuria. Fewer patients with normoalbuminuria treated with RAASI progressed to microalbuminuria, with a relative risk of 0.84 (95% CI 0.79 to 0.89, p = 0.002, I2 = 19%). RAASI treatment resulted in fewer patients progressing from micro- to macroalbuminuria, with a relative risk of 0.52 (95% CI 0.43 to 0.63, p = 0.00001, I2 = 48%). More patients regressed from micro- to normoalbuminuria in the treated group than in comparator groups, with a relative risk of 1.20 (95% CI 1.12 to 1.29, p = 0.03, I2 = 75%). In those studies in which it was assessed, there was no significant effect of treatment on mortality (Figure 7) or GFR.
FIGURE 6.
Relative risk of progression and regression of albuminuria in treatment and comparator arms of trials in type 2 diabetes. Relative risk of progression and regression of albuminuria during trials of RAASI treatment versus comparator (boxes) and pooled estimates across trials (diamonds) calculated by the fixed-effects inverse variance (I-V) method and by the DerSimonian and Laird random-effects (D+L) method, in patients type 2 diabetes, stratified by baseline urine albumin status. Horizontal bars and diamond widths denote 95% CIs and box sizes indicate relative weight in the I-V analysis. (a) Progression from normo- to microalbuminuria; (b) progression from micro- to macroalbuminuria; and (c) regression from micro- to normoalbuminuria. BENEDICT, Bergamo nephrologic diabetes complications trial; DIRECT, DIabetic REtinopathy Candesartan Trials; ROADMAP, randomized olmesartan and diabetes microalbuminuria prevention; SMART, shiga microalbuminuria reduction trial.
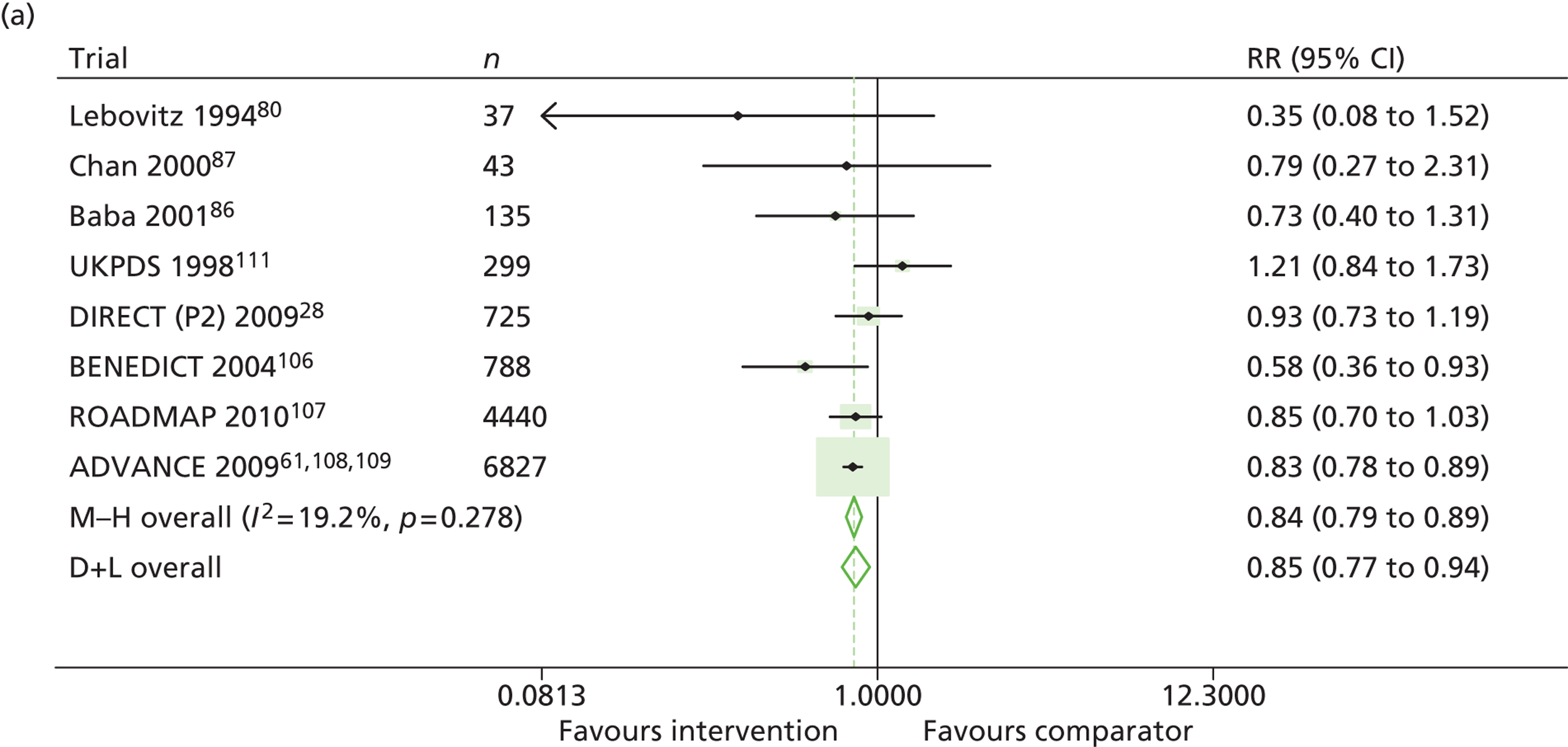
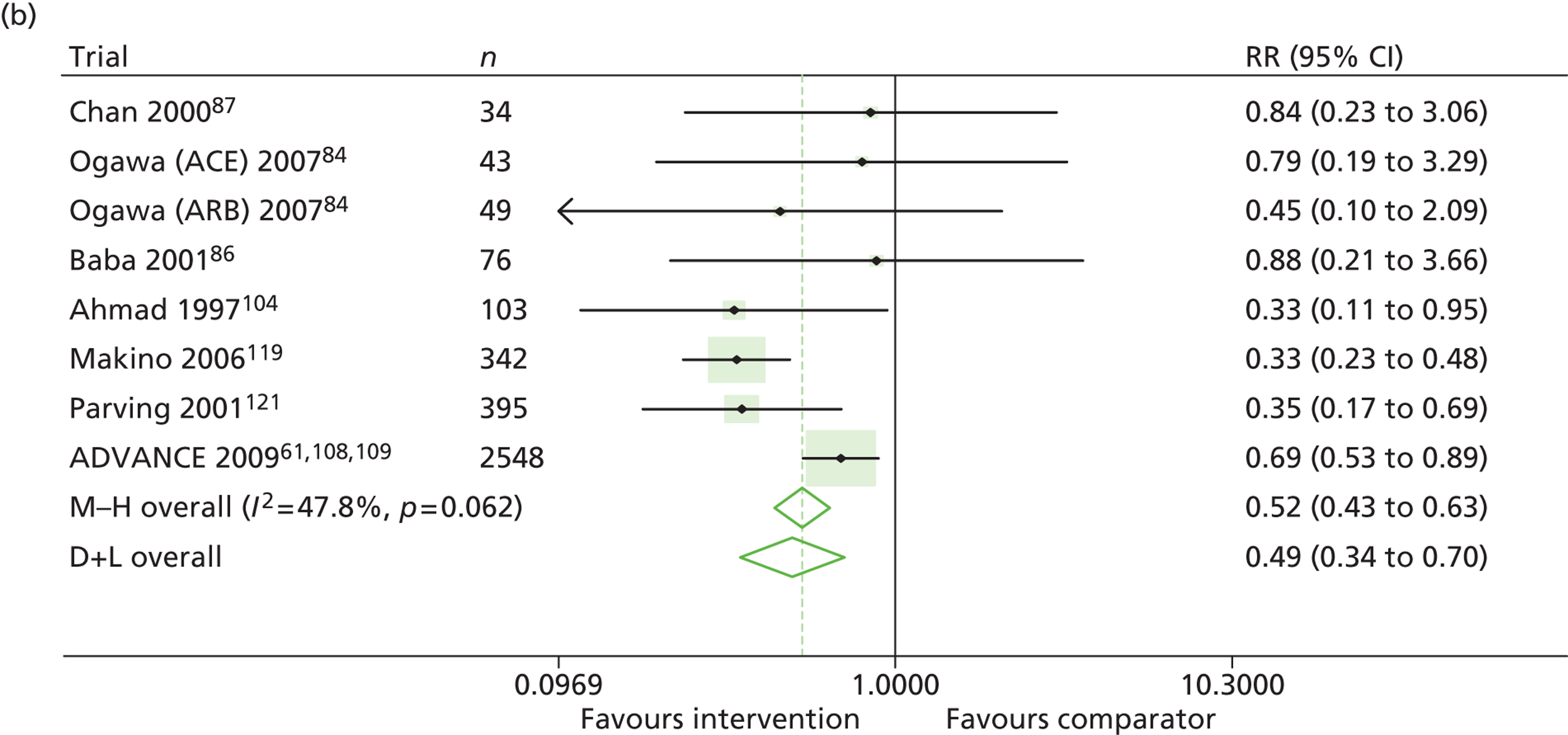
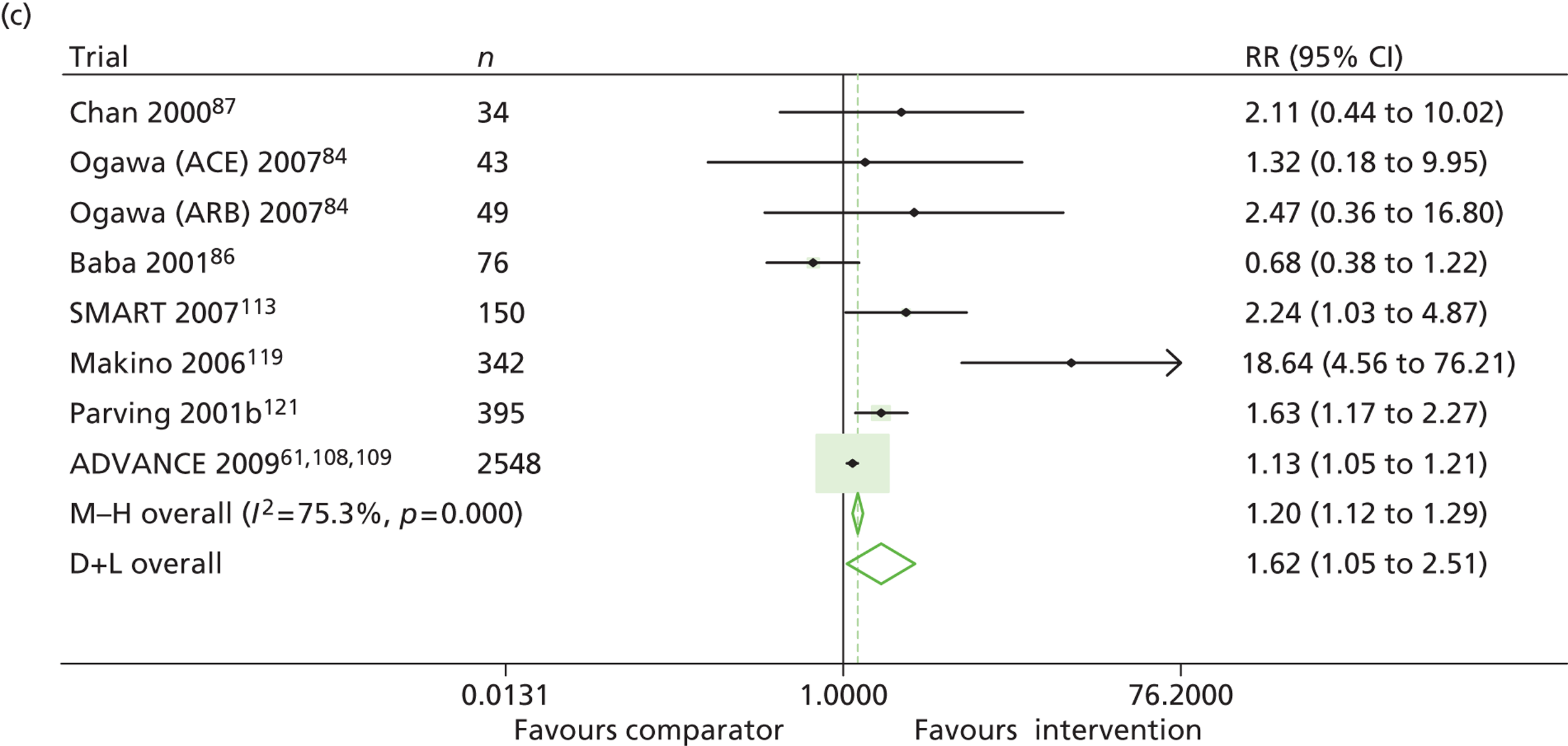
FIGURE 7.
Relative risk of all-cause mortality in treatment and comparator arms of trials. Relative risk of all-cause mortality during trials of RAASI treatment versus comparator (boxes) and pooled estimates across trials (diamonds) calculated by the fixed-effects inverse variance (I-V) method in patients with (a, b) type 1 and (c, d) type 2 diabetes, stratified by baseline urine albumin status. Horizontal bars and diamond widths denote 95% CIs and box sizes indicate relative weight in the I-V analysis. (a) Normoalbuminuria; (b) microalbuminuria; (c) normoalbuminuria; and (d) microalbuminuria. ATLANTIS, ace-inhibitor trial to lower albuminuria in normotensive insulin-dependent subjects; BENEDICT, Bergamo nephrologic diabetes complications trial; DIRECT, DIabetic REtinopathy Candesartan Trials; RENAAL, reduction in end points in noninsulin-dependent diabetes mellitus with the angiotensin II antagonist losartan; ROADMAP, randomized olmesartan and diabetes microalbuminuria prevention.
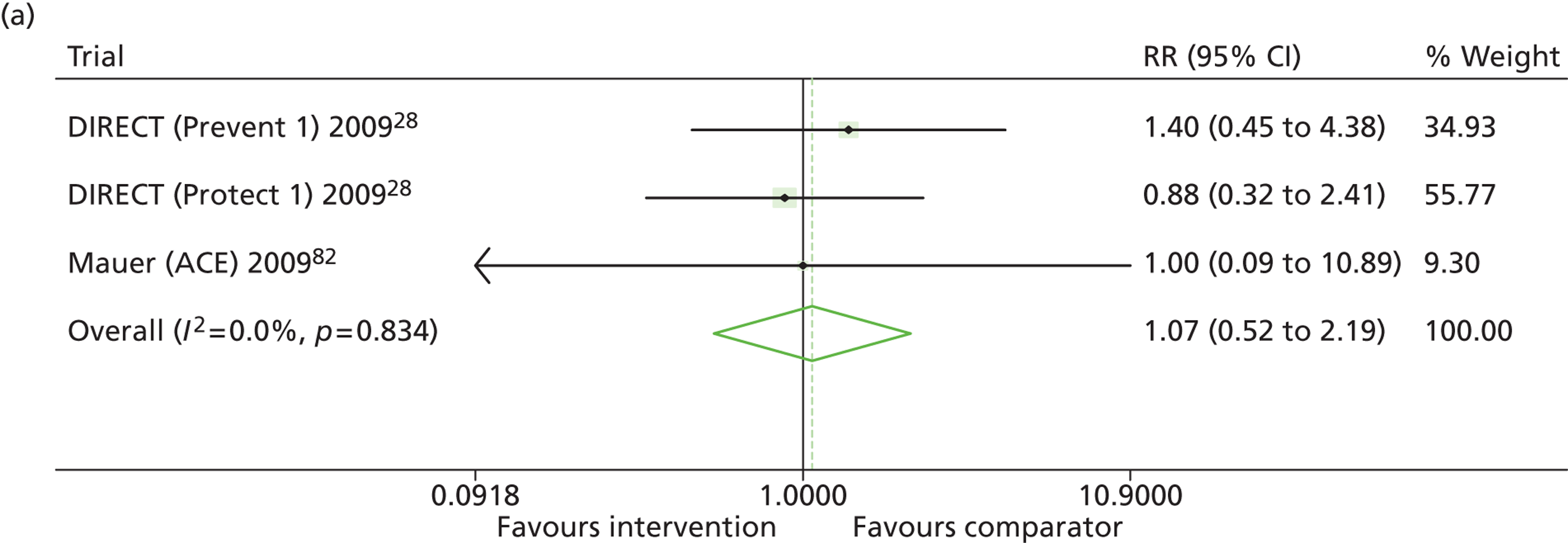
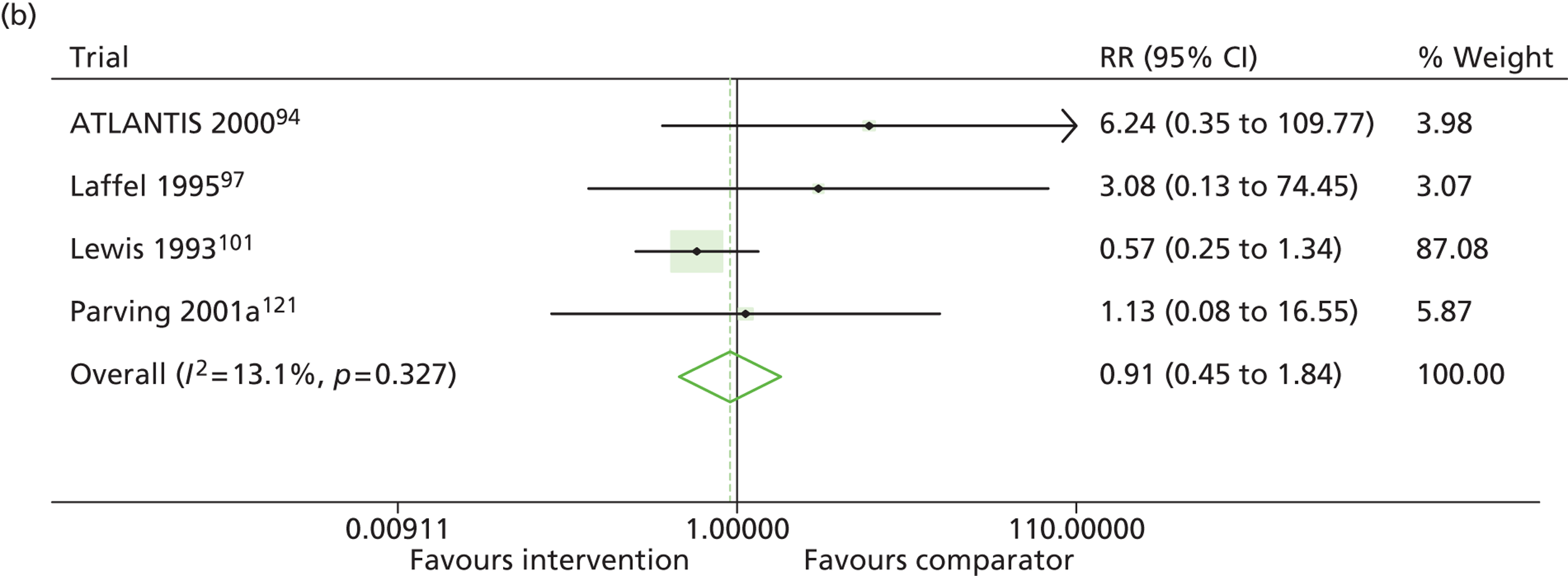
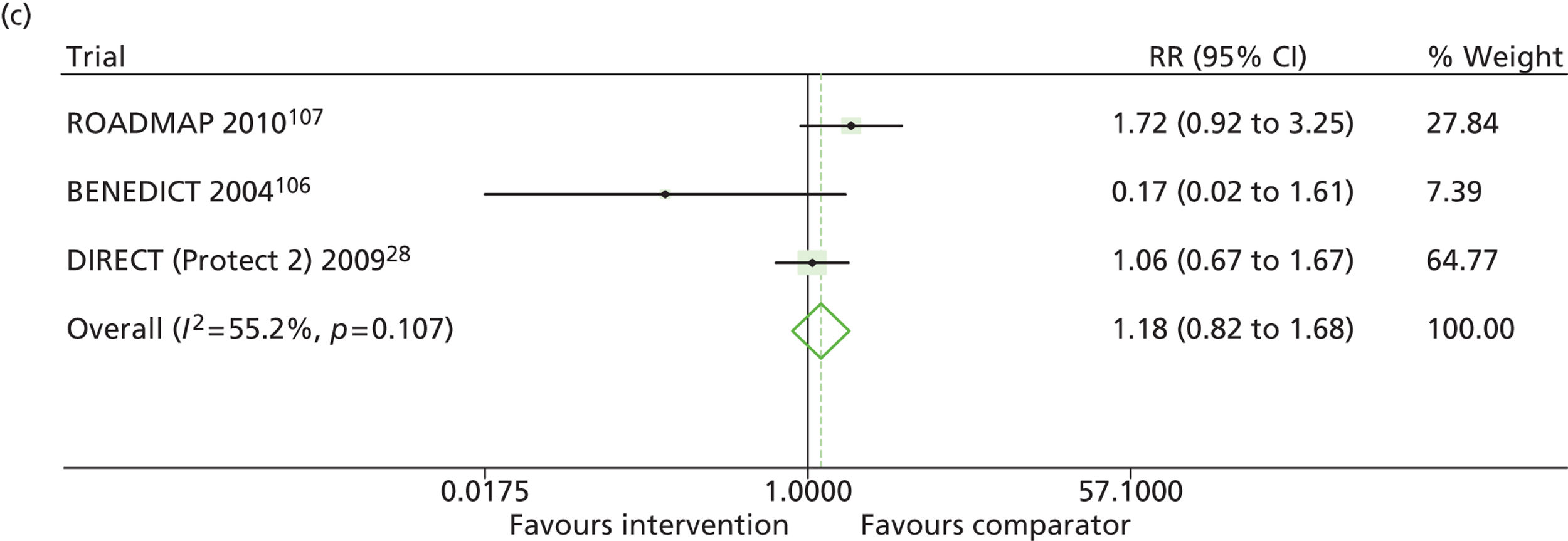
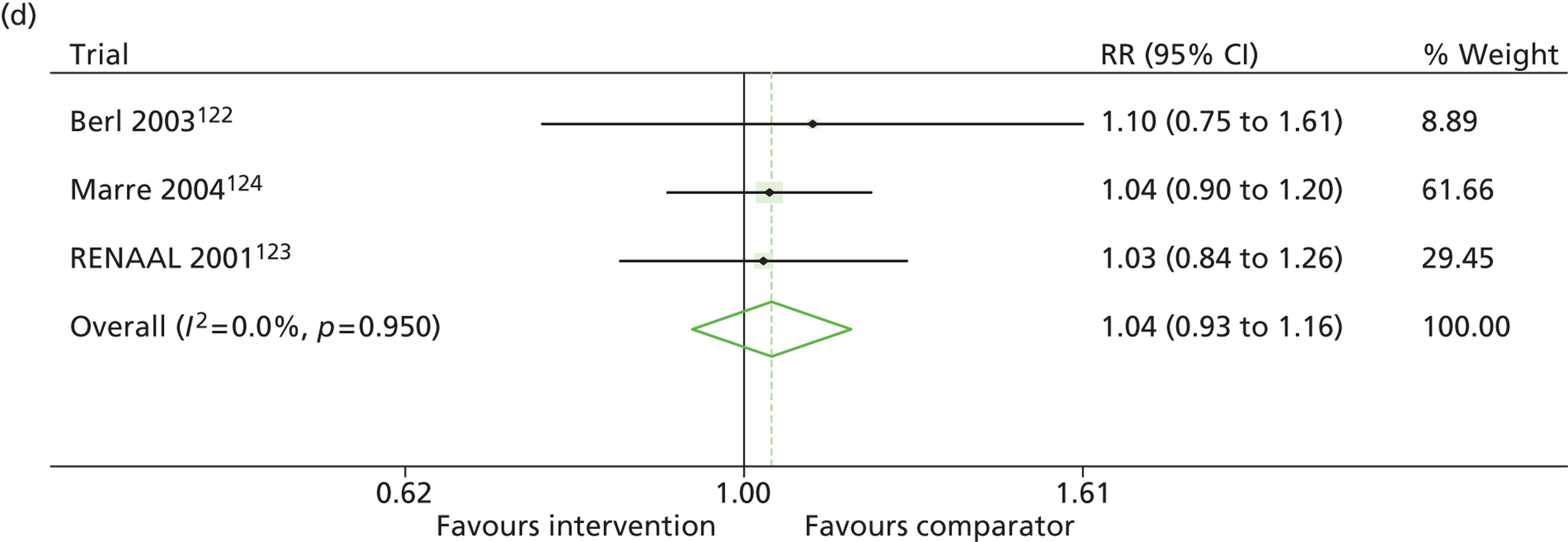
Metaregression
In a univariate metaregression of type 1 diabetes studies, it was found that treatment effect in trials of microalbuminuric patients was significantly greater than in trials of normoalbuminuric patients (p = 0.006). In studies of type 2 diabetes, differences in treatment effect between microalbuminuria and normoalbuminuria were not significant (p = 0.65). Metaregression of the type of medication (ACEi or A2RB) could not be performed for type 1 diabetes, as too few trials used A2RB; however, medication type was not found to significantly affect urinary albumin levels in patients with type 2 diabetes (p = 0.43). Comparator type (antihypertensive medication vs. no antihypertensive medication) did not significantly alter treatment effect either for type 1 diabetes (p = 0.34) or for type 2 diabetes (p = 0.86). In multivariate metaregression, the effect of the following factors on heterogeneity were explored: baseline urinary albumin, medication type, trial country, age, differences in baseline urinary albumin levels, trial size, data extraction method and dose of RAASI. None of these factors individually reduced the I2 statistic by > 20%, with trial country having the biggest impact on the heterogeneity in both type 1 and type 2 diabetes trials.
Discussion
This is the first systematic review of RAASI designed to look for possible differences in benefits between type 1 and type 2 diabetes. Previous studies of microalbuminuric patients found that RAASI reduced progression of proteinuria. 82,126 We have confirmed this and, further, shown that there is benefit in both type 1 and type 2 diabetes. However, the benefit of RAASI in primary microalbuminuria prevention found in a previous review in patients with normoalbuminuria63 was only confirmed in our review for patients with type 2 diabetes. These separate analyses by type of diabetes have been made possible by a number of new trials, the largest being ADVANCE,61 which were not included in the previous systematic reviews. In addition, we found no evidence of a difference in response between A2RB and ACEi in patients with type 2 diabetes, but this result should be treated with caution, as it is largely based on comparisons across trials of either treatment against placebo, rather than head-to-head trials of ACEi versus A2RB. We were unable to make a similar comparison for type 1 diabetes because of insufficient A2RB trial numbers.
We used a systematic search strategy to ensure that all relevant published trials were included in the review. Confining the search to those trials that separately reported outcomes for type 1 and type 2 patients allowed us to explore potential differences between the conditions. In the analysis of continuous outcomes, heterogeneity between the trials is large, even within subgroups defined by type of diabetes and baseline urinary albumin levels. The metaregression suggested that no single factor could explain the heterogeneity between the trials and that it was attributable to multiple factors, for example geographic setting, treatment type, treatment dose, trial size, type of comparator or baseline imbalance between randomisation groups. However, the greater impact of baseline urinary albumin on treatment effect size in type 1 diabetes than in type 2 diabetes was confirmed by the analysis of dichotomous outcomes, which showed relatively little heterogeneity.
The appropriate use of fixed or random effects in meta-analysis continues to be discussed. In fixed-effects analyses, CIs do not reflect heterogeneity between studies. 127 Thus, in Figure 4, the narrow CIs for fixed-effects analyses reflect the large quantity of data available rather than the degree of heterogeneity between the trials. Random-effects analyses incorporate heterogeneity when calculating CIs, but arguably give too much weight to small studies. For example, in the random-effects analysis in Figure 4, the largest study is > 100 times the size of the smallest, but has < 10 times the weight in the analysis. In presenting results from both analyses, we demonstrate that our broad clinical conclusions are not sensitive to the choice of method. Regardless of the method of analysis, RAASI protects against the onset and progression of microalbuminuria in type 2 diabetes, but in type 1 diabetes is beneficial only to patients with established microalbuminuria.
No significant effect of treatment on GFR or mortality was found. Few trials reported these outcomes, and, of those that did, the largest trials had a duration of 5 years. In the absence of longer-term outcome data, any conclusions drawn about the benefits of RAASI from our results are dependent on the acceptance of UAE as a surrogate measure. 128–130 The evidence to date for and against the use of urinary albumin as a surrogate for long-term outcomes has recently been summarised elsewhere. 131
There are some remaining concerns that the benefits of RAASI may be mediated solely by their antihypertensive effect. Our data (see Table 7) include a subgroup analysis that separates the placebo-controlled trials from the active comparator trials. The renal benefits of ACEi and A2RB are evident when the comparator is active (another antihypertensive), suggesting that there is an effect over and above that from blood pressure lowering. Another study, published in 2005,128 found that the effect of RAASI was dependent on blood pressure lowering. In two of three analyses that stratified trials by comparator type, the effect was larger in the trials that compared RAASI to active antihypertensive treatment. The difference between our findings and those of the 2005 paper may be a result of the additional trials included. However, a recent paper has carried out a network meta-analysis that confirms our findings. 132 The authors also conclude that, when two agents bring about the same drop in blood pressure, the ACEi/A2RB will have an additional effect. However, they also caution that, for a definitive analysis of this issue, an individual patient data meta-analysis or a randomised trial is required.
The randomisation method was not reported in the majority of the included trials, which is a limitation of our review in that it could be a potential source of bias in the meta-analysis. In a sensitivity analysis restricted to the largest trials (n ≥ 200), type 2 diabetes patients who were normoalbuminuric at baseline showed a lower treatment effect size than those in the main analysis (see Table 7); however, the effect was not large compared with the uncertainty in the CIs. In other analyses, there was little or no evidence of publication bias.
This review does not provide evidence to support routine use of RAASI for type 1 normoalbuminuric diabetes patients. Our conclusions are based on an analysis that includes two trials intended to prevent microalbuminuria in this group, with neither providing evidence of slowing progression to microalbuminuria. 28,82 The mechanisms for the difference in response to ACEi in type 1 and 2 diabetes are unclear, although there may be differences in response to interstitial expansion. 79,82 Although changes in albumin excretion are associated with differences in CVD, it is possible that the lack of impact of ACEi demonstrated on progression to albuminuria in type 1 diabetes does not extend to a lack of effect on development of CVD. The high rate of comorbidity among people with diabetes may mean that, in practice, many people without microalbuminuria have other indications for RAASI use. A recent study among diabetic individuals > 55 years of age suggested that virtually all of this group have additional indications for treatment with RAASI,133 including hypertension and other cardiovascular risk factors. However, in otherwise healthy younger people with type 1 diabetes, there is no indication for treatment with RAASI. A recent trial of losartan and lisinopril versus placebo suggested that treatment of this group of patients may even be potentially harmful,82 although this may be a drug-specific effect or a chance finding.
Current practice is to monitor urinary albumin in all adults with diabetes in order to initiate RAASI treatment when kidney disease is detected. In type 1 diabetes, our results support this strategy of targeting RAASI at those with kidney disease. However, in type 2 diabetes, we find that RAASI has similar albumin-lowering effects in those with and without kidney disease. Our findings suggest that RAASI treatment of type 2 diabetes patients with normoalbuminuria may be beneficial in terms of progression to microalbuminuria. As a high proportion of those with type 2 diabetes is already treated with ACEi for raised blood pressure, the impact of screening may be lessened. This even raises the possibility of using ACEi to treat larger numbers of normoalbuminuric type 2 diabetes patients as a means of preventing progression to microalbuminuria.
The findings of this systematic review inform the health economic analysis reported in Chapter 6, in addition to the information provided about the impact of ACEi and A2RBs in type 1 and type 2 diabetes.
Chapter 3 Modelling progression of kidney disease for type 1 diabetes
Background
Previous work to estimate optimal screening intervals at which to test for the occurrence of kidney disease in patients with diabetes has focused on rates of progression of albuminuria and GFR. 4 However, this approach does not fully account for variability, including the within-test biological and assay variability that cause false-positive identifications of microalbuminuria. Current estimates of test accuracy are modelled from reports of cross-sectional study data that give the proportions of individuals identified with combinations of albumin and eGFR screening. 19
To take into account variability in rates of progression and test accuracy when determining the optimal test intervals for kidney disease screening, we used an approach that was initially developed in cholesterol and blood pressure monitoring134,135 but that has since been extended to other areas of monitoring, including diabetes. 136,137 Using these methods, annual monitoring of cholesterol and blood pressure has been shown to lead to more false-positive tests than true-positive tests: that is, a positive test for high cholesterol (or high blood pressure) is more likely to arise from measurement error than from ‘true change’. 134 For many clinical monitoring tests, the variability within each measurement is greater than the likely change over 1 year, and regular cycles of testing create repeated opportunities for the occurrence of false-positive tests. In type 1 diabetes, the implication of a false-positive urine albumin test is unnecessary, and possibly harmful, pharmacological treatment in individuals with normal urine albumin levels.
We applied these methods to data from ORPS to examine the optimal interval for microalbuminuria screening in patients with type 1 diabetes. We evaluated annual urine albumin testing using data drawn from this longitudinal study in which regular testing of renal function was carried out. In addition to providing information about the properties of repeated testing, presented as the ‘signal-to-noise’ ratio, it also provided evidence regarding the impact of different schedules of testing on the extent of false-positive diagnoses. These data were used to inform the development of health economic models for progression of type 1 diabetes, presented in Chapter 5 of this report.
Methods
Data sources
We evaluated progression of ACR, eGFR and associated parameters in a cohort of newly diagnosed type 1 diabetes patients drawn from ORPS. A comprehensive description of the population and the research design is provided in a previous study report. 48 Briefly, between 1986 and 1996 young people, living in Oxfordshire, UK, and diagnosed with type 1 diabetes before the age of 16, were invited to participate in a longitudinal study. Children with diabetes secondary to another condition were excluded. Annual assessment of clinical and biochemical measures included three consecutive early morning urine specimens. Urine samples were assayed for albumin and creatinine in a central laboratory and the urinary ACR was calculated for each urine sample. Albumin was measured by an enzyme-linked immunosorbent assay, and creatinine using the modified Jaffe method. Serum creatinine samples were used to calculate eGFR using the Schwartz formula in patients < 18 years of age,138 and the MDRD formula for those ≥ 18 years. 14 Annual assessments continued for up to 20 years. Written consent was obtained from parents and children were asked to give assent before commencement of the study. Ethical approval was obtained from the local ethics committees.
Statistical methods
The parameters describing the progression of urine ACR, its variability and its test performance were derived from the repeated measurement in the ORPS data set. We used WinBUGS 1.4 (MRC Biostatistics Unit, Cambridge, UK)139 to fit the linear random-effects model described below, and report model parameters with 95% credible intervals as approximations to 95% CIs. We considered the parameters describing the progression of ACR under three main headings: (1) the between-subject variation in baseline ACR (often referred to as the intercept), for which the tendency for patients’ ACR to systematically differ from other patients’ was modelled as a mean and standard deviation of log-ACR; (2) the average change in ACR over time, which was modelled as the change in log-ACR per year; and (3) the difference between the observed ACR and the underlying ACR for a single individual, conceptualised as the within-person variability in any individual ACR measurement arising from the analytic variability in the assay and short-term biological variability. The first two of these components use random-effects models with normal distributions to describe the underlying log-ACR for each patient over time, whereas the third component uses a t-distribution to summarise the within-measurement variability. An inverse t-distribution with three degrees of freedom was used to describe the within-measurement variability of log-ACR. The t-distribution was used because the variation in individual measurements was too great to be described by a normal distribution; in particular, some measurements were much further from the mean than the standard deviation. The model included effects of age and sex on underlying ACR. A previous report from ORPS suggested that the age effect could be partly attributable to puberty, but, after adjustment for age, we found no significant effect on ACR (p = 0.4) and, therefore, did not include it in the final model.
We also fitted models for trends and variation in eGFR using the same methods. Residual plots indicated an approximate normal distribution for eGFR and, therefore, no log transformation was required. We allowed mean eGFR and mean rate of change to vary between men and women, but held other parameters the same for both men and women.
Results are reported as mean and standard deviation for log-ACR scale and eGFR and geometric mean of ACR with CIs. The average signal-to-noise ratio is also reported, with the signal being the average change over time, and the noise being the standard deviation of the t-distribution for component within-person variability in any individual ACR measurement.
As previously described,140 these estimates of between- and within-person trends and variability in log-ACR allow us to estimate the proportions of diagnoses of microalbuminuria attributable to the onset of kidney disease (true-positive results), and the proportion attributable to within-measurement variability (false-positive results). Likewise, we are able to estimate the number of people correctly or incorrectly classified with normoalbuminuria (true negative and false negative, respectively). These estimates can be derived only by modelling methods such as simulation. The high variability in any measurement of urine ACR makes it impossible to classify any individual patient in a real data set as a ‘true’ or ‘false’ positive for microalbuminuria. However, simulation allows us to classify individual patients in the simulated data set and, hence, estimate the proportion of patients who would be thus classified in a real cohort. The statistical validity and clinical utility of this approach have been established previously. 134,140
A simulated population of 75,000 individuals generated with the same distribution of age, sex, ACR and duration of diabetes was created. In each patient, the simulated ‘true ACR’ value at each year is calculated from his or her initial ACR value and annual rate of change. The true ACR value is assumed to be unobservable to patient or clinician. The measured ACR value in any given urine sample is simulated from the true value at that time and the model estimate of the within-measurement variability of ACR. We assumed that the screening process would follow the NICE guidance: (i) microalbuminuria defined by ACR > 2.5 mg/mmol for men or 3.5 mg/mmol for women; (ii) testing follows a sequence of tests following on from an initial positive test; (iii) screening starts at 12 years of age. 19 Therefore, an individual of age ≥ 12 years in the simulated population was assumed to be diagnosed with microalbuminuria if his or her first measured ACR was above the threshold, and at least one of two further ACR tests were also above the threshold that year. We then classified a diagnosis of microalbuminuria as ‘true positive’ if the true ACR was above the threshold or ‘false positive’ if the true ACR was below the threshold. Similarly, when a patient was not diagnosed with microalbuminuria – either because the first measured ACR was below the threshold or because the first measured ACR was above the threshold but not confirmed by subsequent measured ACR in the same year – we classified this as ‘true negative’ if the true ACR was below the threshold and ‘false negative’ if the true ACR was above the threshold. We assumed that patients not diagnosed with microalbuminuria continue to be monitored in subsequent years and hence may subsequently be diagnosed with microalbuminuria.
We carried out simulation modelling for annual, biennial (every 2 years) and triennial (every 3 years) screening. We also compared long-term predictions from the model with previously published reports on the prevalence of albuminuria at different ages from large studies of type 1 diabetes. There were insufficient data to study eGFR monitoring in type 1 diabetes because there were no cases of eGFR < 60 ml/minute during ORPS follow-up.
Results
The characteristics of the participants in the ORPS included in this analysis are shown in Table 8. From a total of 543 adolescents, data from 535 were eligible for inclusion in the analysis. They were followed up for a median of 10.6 years (interquartile range 6.9 to 13.4 years).
Variable | Number of patients (n = 542) | Value [median (Q1, Q3)] |
---|---|---|
Gender | ||
Male | 296 | 54.6% |
Female | 246 | 45.4% |
Age at diagnosis (years) | 535 | 9.6 (5.6, 14.8) |
Duration of diabetes (years) | 535 | 1.05 (0.87, 5.47) |
HbA1c (mmol/mol) | 520 | 83 (68, 125) |
Body mass index (kg/m2) | 535 | 18 (17, 24) |
Systolic blood pressure (mmHg) | 537 | 100 (92, 120) |
Diastolic blood pressure (mmHg) | 537 | 65 (58, 82) |
eGFR (ml/minute) | 320 | 159 (134, 225) |
ACR | 542 | 0.95 (0.61, 4.14) |
Microalbuminuriaa | ||
Yes | 30 | 5.5% |
No | 512 | 94.5% |
Albumin-to-creatinine ratio
Table 9 reports the parameters used in defining the model that estimates trends and variation in log-ACR. The mean log-ACR of 0.031 (95% CI –0.053 to 0.110) in males and 0.20 (95% CI 0.10 to 0.29) in females is equivalent to geometric mean ACR of 1.03 (95% CI 0.95 to 1.12) and 1.22 (95% CI 1.11 to 1.34), respectively. The average changes in log-ACR per year of 0.035 (95% CI 0.02 to 0.049) in males and 0.047 (95% CI 0.031 to 0.063) in females correspond to average increases in ACR of 4% and 5% per year, respectively. However, the standard deviation around this average, 0.11 on the log-ACR scale, corresponds to wide variation in this rate of change: the estimated 25th and 75th centiles of the change in ACR are a 4% per year decrease in males (3% in females) and 12% per year increase in males (13% in females), respectively. The estimated standard deviation of within-measurement variability in log-ACR is 0.79 (95% CI 0.73 to 0.86), which corresponds to a within-measurement coefficient of variation in ACR of > 100%. The average signal-to-noise ratio is 0.044 in males and 0.059 in females for annual screening, 0.088 in males and 0.120 in females for biennial screening, and 0.13 in males and 0.18 in females for triennial screening.
Parameter | Interpretation | Parameter estimate |
---|---|---|
αm | Mean log-ACR at 7.4 years’ duration of diabetes in males | 0.031 (−0.053 to 0.110) |
αw | Mean log-ACR at 7.4 years’ duration of diabetes in females | 0.20 (0.10 to 0.29) |
λ | Effect of age at diagnosis on mean log-ACR | −0.0059 (−0.018 to 0.0069) |
σa | Standard deviation of log-ACR | 0.68 (0.63 to 0.73) |
βm | Mean annual change in log-ACR in males | 0.035 (0.020 to 0.049) |
βw | Mean annual change in log-ACR in females | 0.047 (0.031 to 0.063) |
σb | Standard deviation of annual change in log-ACR | 0.110 (0.099 to 0.120) |
ρ | Correlation between actual value of log-ACR and rate of change | 0.57 (0.49 to 0.64) |
σw | Standard deviation of within-measurement variability of log-ACRa | 0.79 (0.73 to 0.86) |
The results of modelling the rate of identification of microalbuminuria for males and females separately are shown in Tables 10 and 11. Each section of the tables shows the cumulative rate of positive test results, the percentage of those tests that are ‘false positive’ and the percentage of those repeatedly testing negative that are ‘false negative’. Each of these outcomes is shown under assumptions of annual, biennial and triennial screening. Initially assuming annual screening, Table 10 (males) shows the modelled cumulative rate of identified microalbuminuria for the first year after diagnosis of diabetes, and the cumulative diagnoses of microalbuminuria over subsequent years. Over the first 6 years after diagnosis of diabetes, annual, biennial and triennial screening would identify 327, 270, and 244 cases of microalbuminuria per 1000 males with type 1 diabetes, respectively. Over 18 years, annual, biennial and triennial screening would identify 575, 529 and 503 cases of microalbuminuria per 1000 males with type 1 diabetes.
Years since first test | Cumulative number of positive tests per 1000 patients (95% CI) | Percentage of those testing positive who have a false-positive result (95% CI) | Percentage of those with repeated negative tests who have a false-negative result (95% CI) | ||||||
---|---|---|---|---|---|---|---|---|---|
Annual testing | Biennial testing | Triennial testing | Annual testing | Biennial testing | Triennial testing | Annual testing | Biennial testing | Triennial testing | |
0 | 89 (76 to 104) | 89 (76 to 104) | 89 (76 to 104) | 47 (41 to 52) | 47 (41 to 52) | 47 (41 to 52) | 3.1 (2 to 4) | 3.1 (2 to 4) | 3.1 (2 to 4) |
1 | 143 (125 to 165) | 52 (46 to 57) | 1.9 (2 to 2) | ||||||
2 | 186 (164 to 213) | 152 (131 to 174) | 53 (48 to 59) | 48 (43 to 54) | 1.5 (1 to 2) | 2.4 (2 to 3) | |||
3 | 224 (198 to 254) | 164 (142 to 187) | 53 (48 to 59) | 45 (40 to 50) | 1.3 (1 to 2) | 3 (2 to 4) | |||
6 | 327 (292 to 364) | 270 (240 to 301) | 244 (214 to 274) | 48 (43 to 53) | 41 (37 to 47) | 38 (33 to 44) | 1.5 (1 to 2) | 3.1 (2 to 4) | 4 (3 to 5) |
12 | 490 (446 to 537) | 434 (391 to 476) | 403 (360 to 446) | 34 (30 to 40) | 28 (25 to 33) | 25 (22 to 30) | 1.5 (1 to 2) | 3.5 (3 to 4) | 5 (4 to 6) |
18 | 575 (531 to 624) | 529 (486 to 576) | 503 (456 to 551) | 27 (24 to 32) | 22 (19 to 26) | 20 (17 to 23) | 0.7 (1 to 1) | 2.1 (2 to 3) | 3.4 (3 to 4) |
Years since first test | Cumulative number of positive tests per 1000 patients (95% CI) | Percentage of those testing positive who have a false-positive result (95% CI) | Percentage (%) of those with repeated negative tests who have a false-negative result (95% CI) | ||||||
---|---|---|---|---|---|---|---|---|---|
Annual testing | Biennial testing | Triennial testing | Annual testing | Biennial testing | Triennial testing | Annual testing | Biennial testing | Triennial testing | |
0 | 72 (58 to 88) | 72 (58 to 88) | 72 (58 to 88) | 52 (46 to 58) | 52 (46 to 58) | 52 (46 to 58) | 2.3 (2 to 3) | 2.3 (2 to 3) | 2.3 (2 to 3) |
1 | 116 (97 to 138) | 58 (52 to 64) | 1.4 (1 to 2) | ||||||
2 | 151 (128 to 177) | 121 (101 to 144) | 60 (54 to 66) | 56 (49 to 62) | 1 (1 to 1) | 1.7 (1 to 2) | |||
3 | 182 (156 to 210) | 129 (109 to 152) | 60 (54 to 66) | 52 (45 to 60) | 0.9 (1 to 1) | 2.1 (2 to 3) | |||
6 | 265 (231 to 301) | 214 (183 to 247) | 191 (163 to 222) | 55 (48 to 61) | 49 (41 to 56) | 45 (38 to 53) | 1.0 (1 to 1) | 2.1 (2 to 3) | 2.8 (2 to 4) |
12 | 403 (356 to 452) | 348 (303 to 397) | 320 (276 to 371) | 41 (34 to 48) | 34 (28 to 42) | 31 (25 to 38) | 1.1 (1 to 1) | 2.5 (2 to 3) | 3.6 (3 to 5) |
18 | 484 (430 to 539) | 436 (383 to 495) | 411 (357 to 473) | 33 (27 to 39) | 27 (21 to 34) | 24 (18 to 30) | 0.5 (0 to 1) | 1.7 (1 to 2) | 2.7 (2 to 3) |
Table 10 (columns 5–7) shows the percentage of those inaccurately identified with microalbuminuria, that is the number of false positives as a percentage of the total number of positive tests. For an initial test, an estimated 53% of microalbuminuria diagnoses would be true positive, and 47% would be false positive, with the inaccuracy attributable to the variability in ACR measurements. We estimate that, by year 6, with a regimen of annual, biennial and triennial screening, 48%, 41% and 38% of individuals identified with microalbuminuria would be false positive, and, by year 18, 27%, 22% and 20%, respectively. The estimated false-negative rates, again with a regimen of annual, biennial and triennial screening, are, respectively, 0.7%, 2.1% and 3.4% by year 18.
Table 11 shows the corresponding estimates for females with type 1 diabetes. Over 18 years, a regimen of annual, biennial and triennial screening would identify 484, 436 and 411 cases of microalbuminuria per 1000 females with type 1 diabetes, 33%, 27% and 24% of which would be false-positive diagnoses. Of those repeatedly testing negative for microalbuminuria, 0.5%, 1.7% and 2.7% would be false negatives.
In summary, in males and females with type 1 diabetes, 48% (95% CI 43% to 53%) and 55% (95% CI 48% to 61%) of patients, respectively, tested annually for microalbuminuria over 6 years would be false positive, that is inaccurately identified with microalbuminuria.
The estimated true- and false-positive test rates reported in Tables 10 and 11 are based on the parameter estimates shown in Table 9. Figure 8 compares estimates from the fitted model with actual data by overlaying the predicted mean log-ACR, adjusted for duration of diabetes and gender, with the actual data derived from the ORPS. Figure 9 also shows the performance of the simulation model in predicting the development of microalbuminuria, macroalbuminuria and regression to microalbuminuria in comparison with the original data on which the model is based.
FIGURE 8.
Mean (dots), interquartile range (vertical bars) and 95th centiles (+) of ACR in male (top) and female (bottom) patients with type 1 diabetes in the ORPS compared with model estimates (dashed lines).
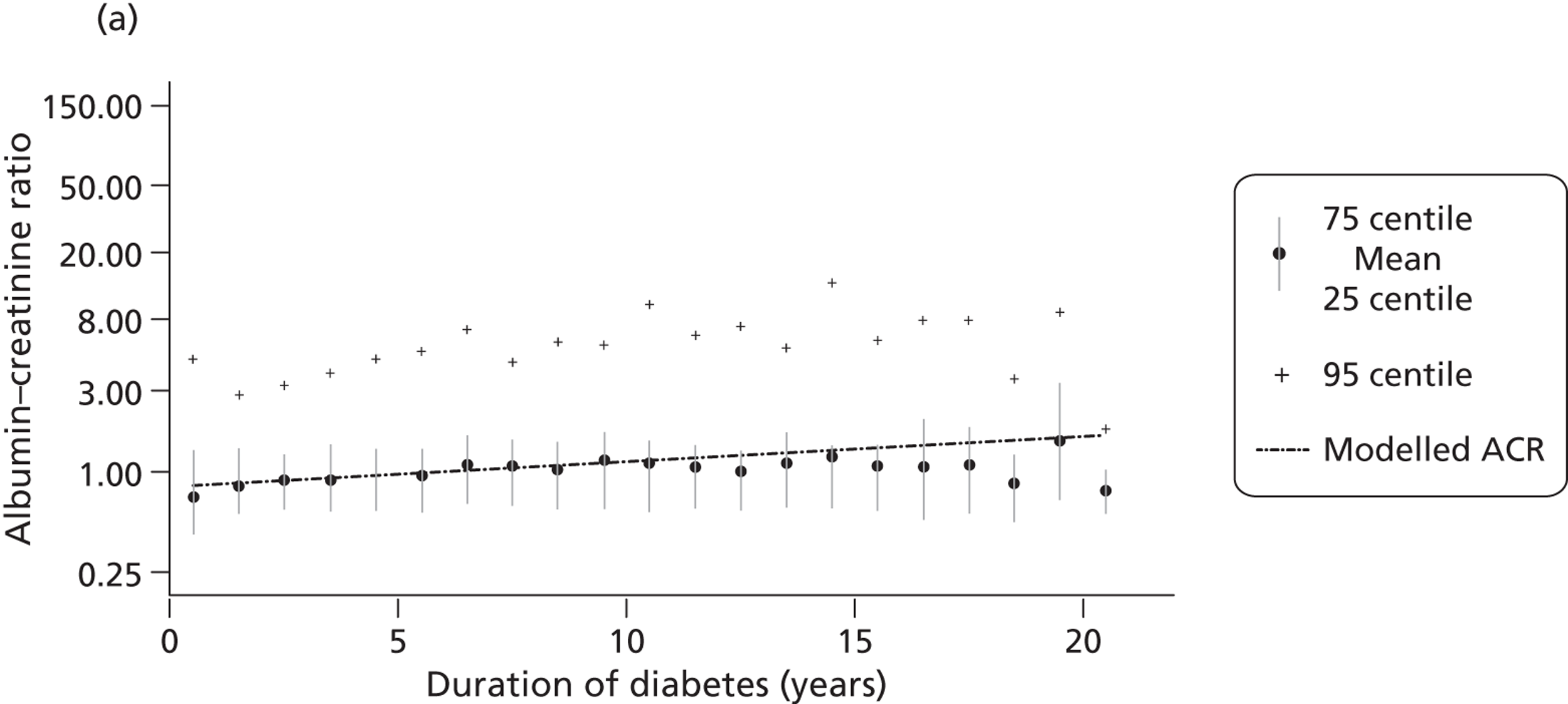
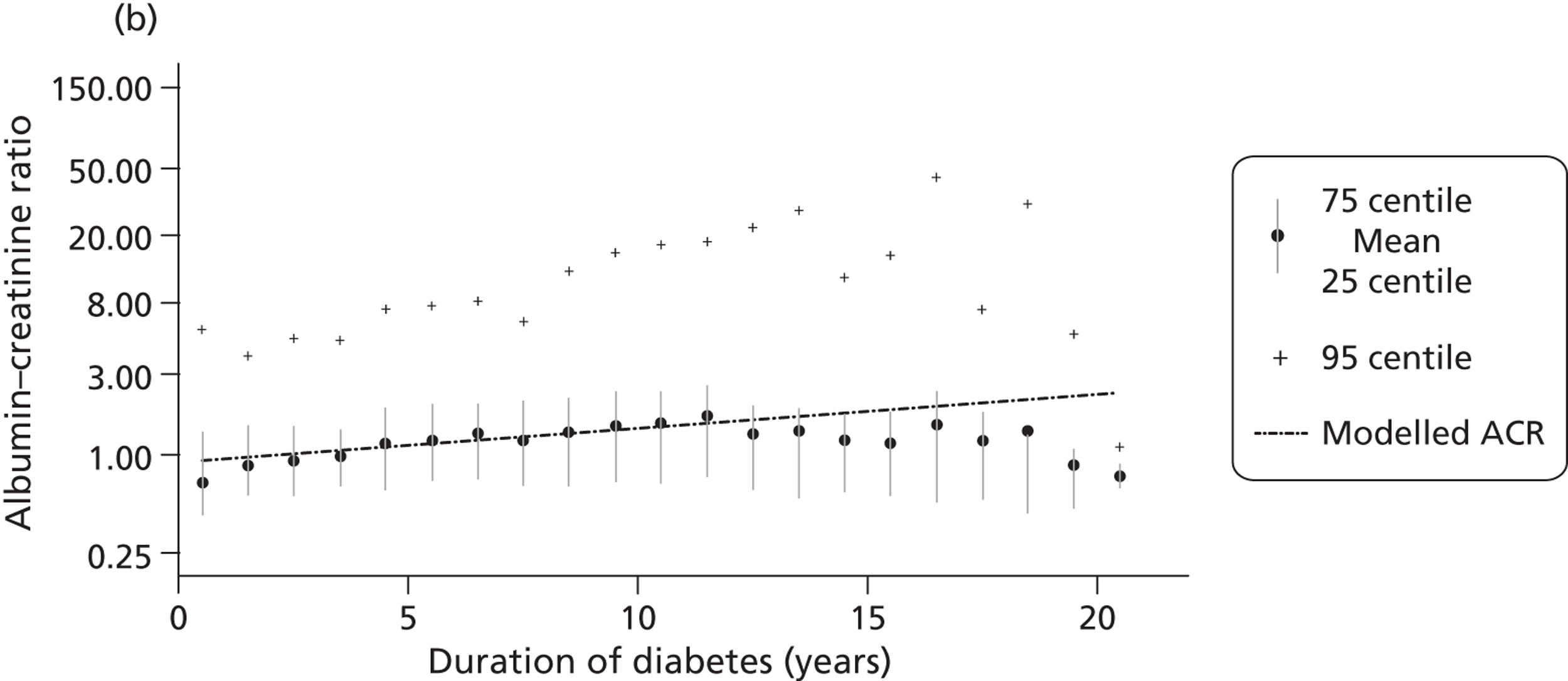
FIGURE 9.
Estimated prevalence of normo-, micro- and macroalbuminuria, and estimated incidence of apparent regression from microalbuminuria to normoalbuminuria (dots), with 95% CIs (vertical lines), in the ORPS compared with model estimates (dashed lines). (a) Normoalbuminuria; (b) microalbuminuria; (c) macroalbuminuria; and (d) regression to normoalbuminuria.
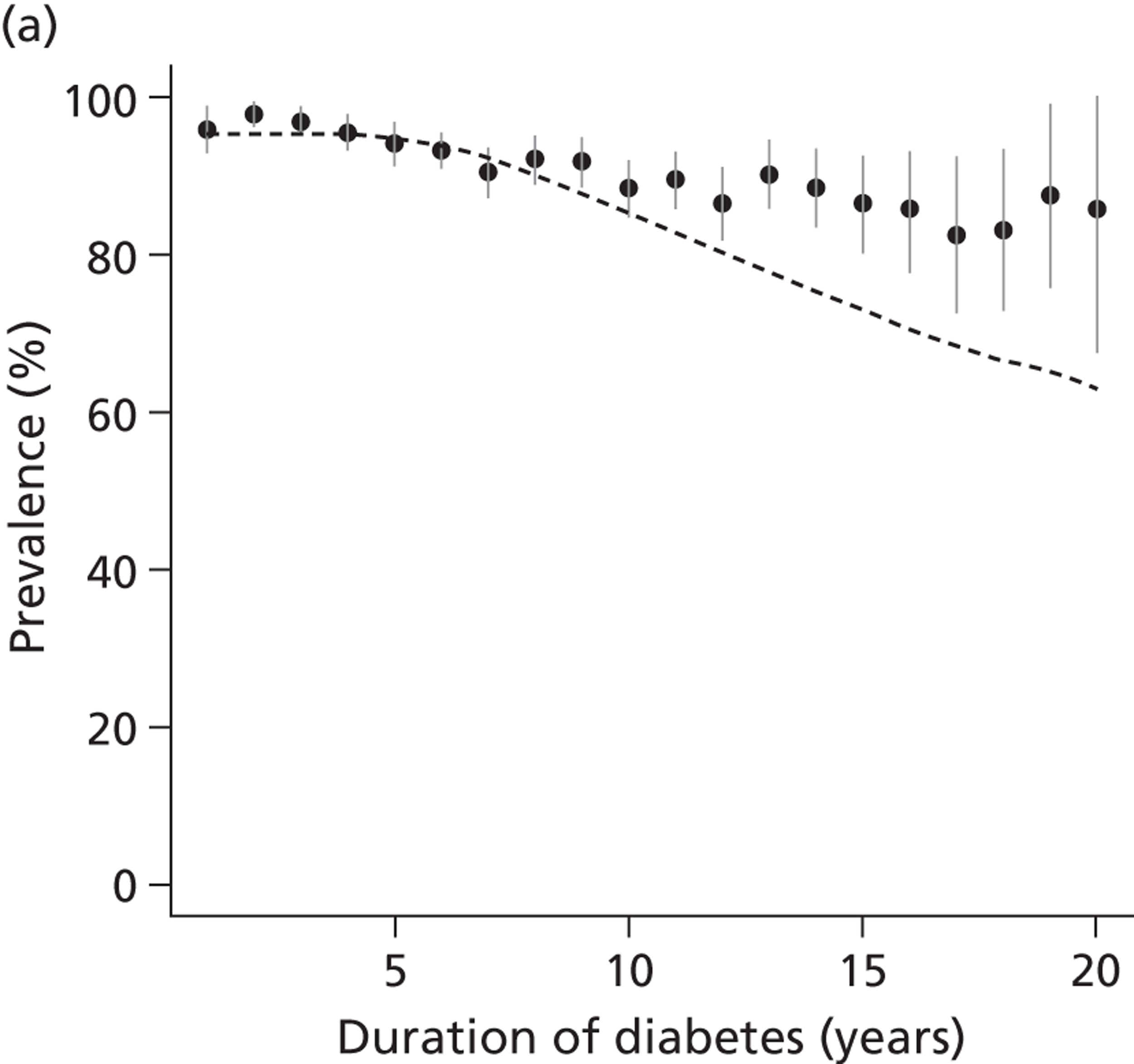
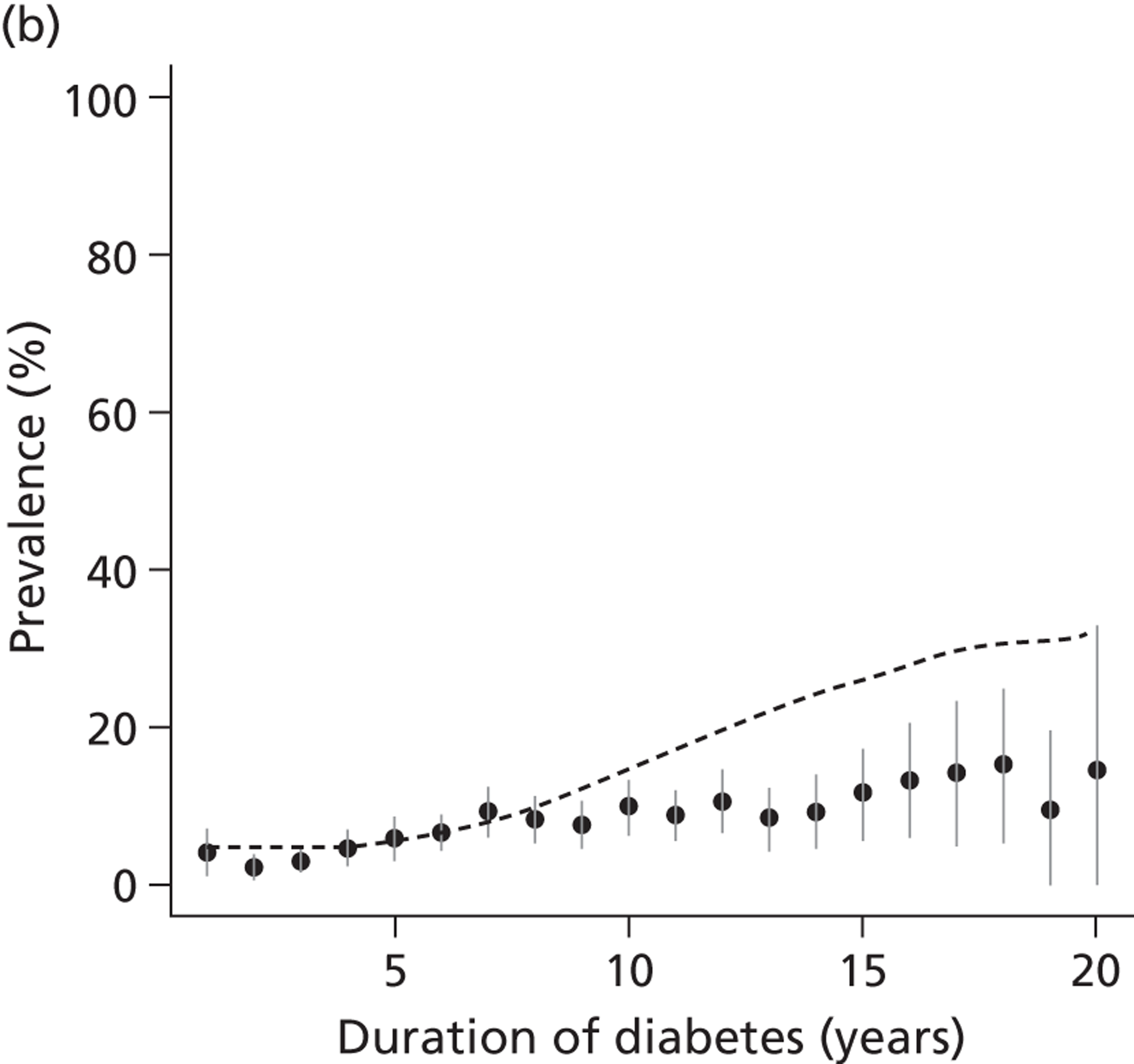
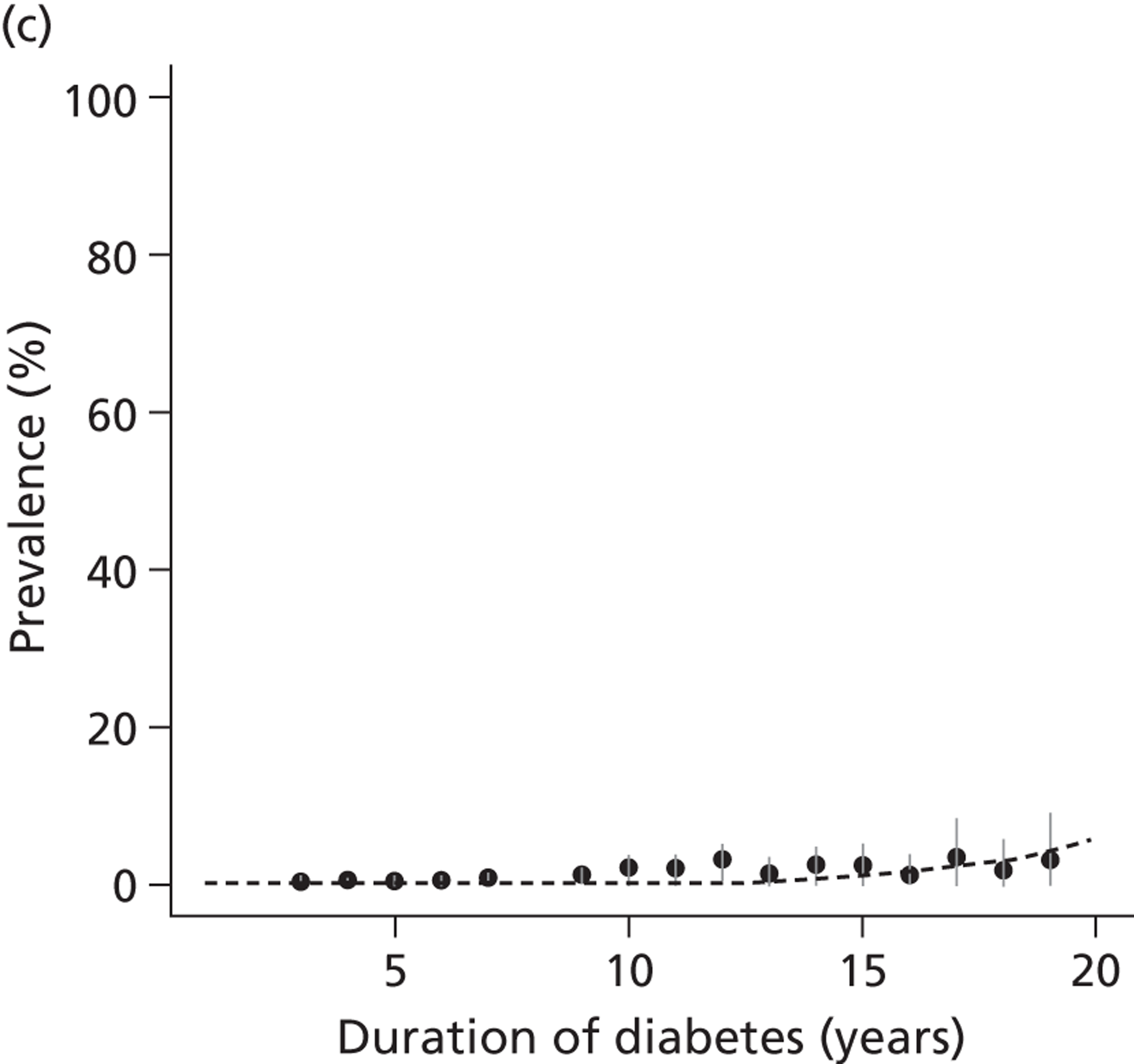
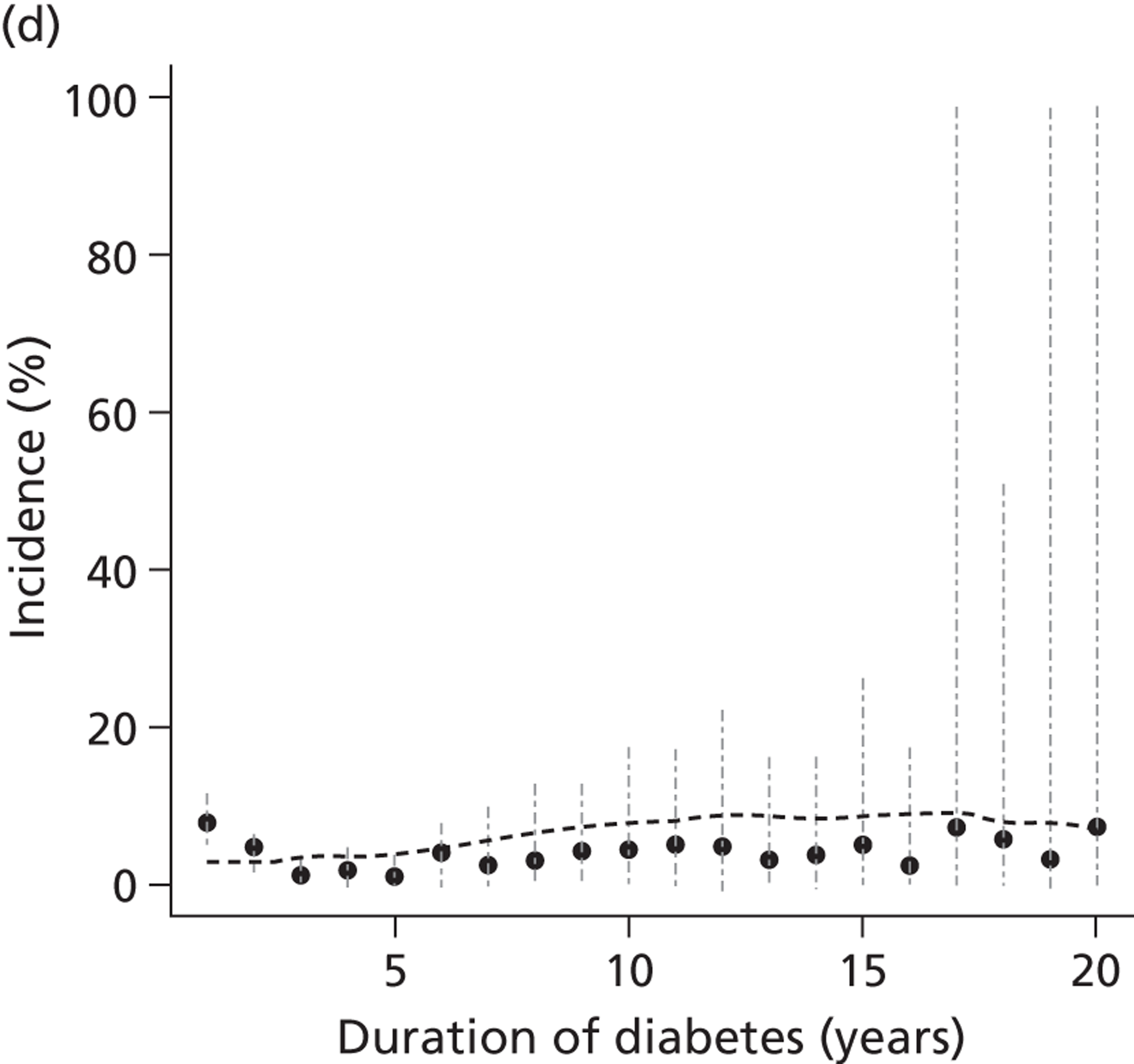
Because ORPS was primarily a study of young people with type 1 diabetes (maximum age 35 by end of follow-up), we compared model predictions to the prevalences of microalbuminuria and macroalbuminuria after diagnosis reported by other large studies of people up to 40 years (Figure 10). 1,51,141–147 The studies used different criteria (e.g. number of confirmatory tests) for diagnosing microalbuminuria.
FIGURE 10.
Proportion of patients who test positive for microalbuminuria or macroalbuminuria by duration of diabetes estimated from parameters obtained from the ORPS (simulation model) compared with studies providing comparator data.
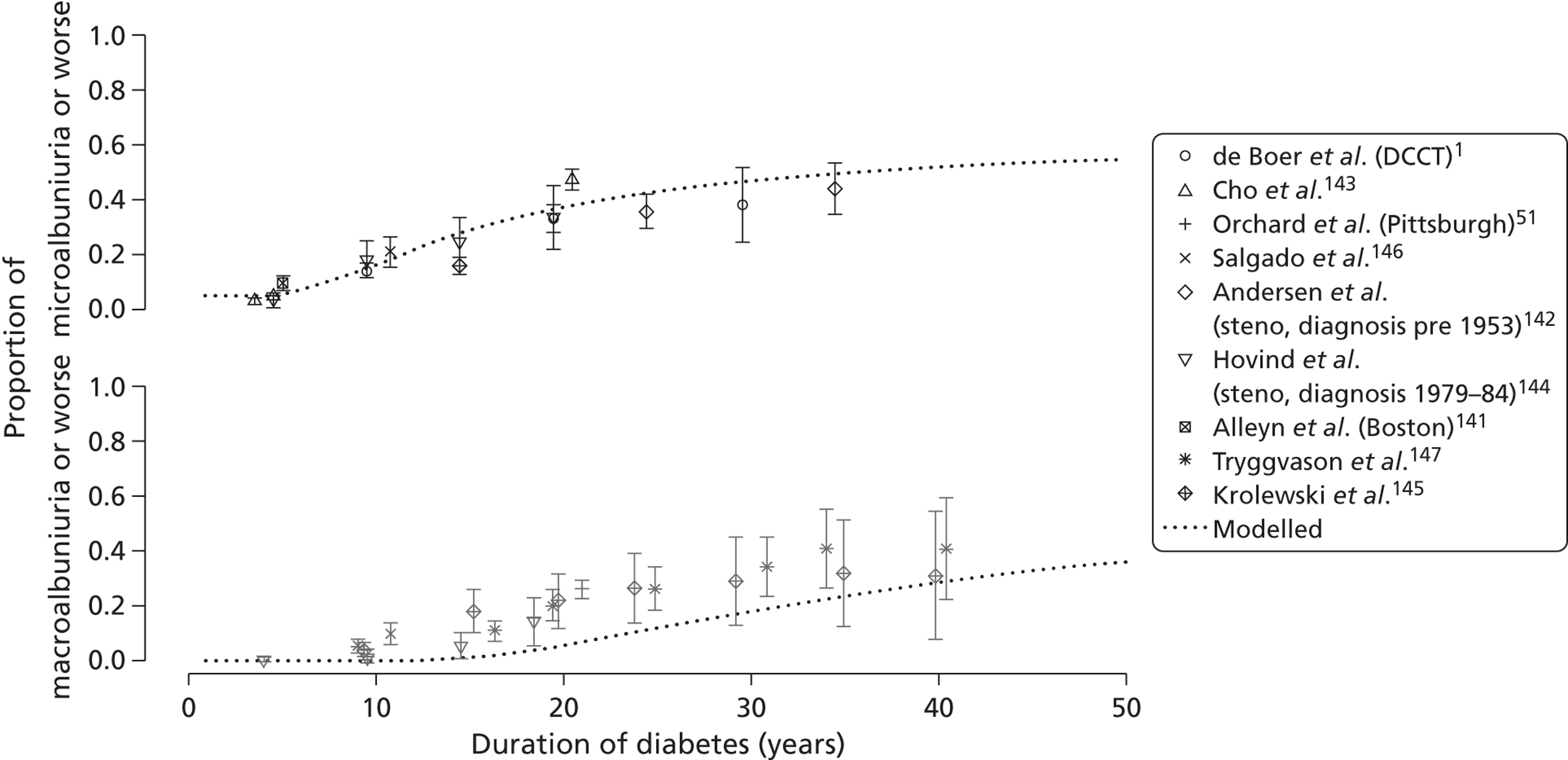
To illustrate the sensitivity of our conclusions to the fitted parameter values, Figure 11 shows how the estimated false-positive rate would vary in hypothetical populations with higher rates of progression of albuminuria. This illustrates the change in the number of false-positive tests when the average rate of change in ACR per year is increased above that observed in our cohort.
FIGURE 11.
Percentage of microalbuminuria diagnoses in male patients with type 1 diabetes that would be false positive under an annual monitoring scheme, when the average increase is as estimated from ORPS (circles; corresponding to columns 5–7 of Tables 10 and 11), or in hypothetical populations in which the average change is 50% (triangles), 100% (pluses) or 200% (crosses) higher than estimated from ORPS. Illustration of the sensitivity of results to key parameters: the results of Table 10, recalculated for four different values of the rate of change parameter. Note that the circles correspond to the analysis in Tables 10 and 11 and other lines correspond to hypothetical subpopulations with increased rates of change.
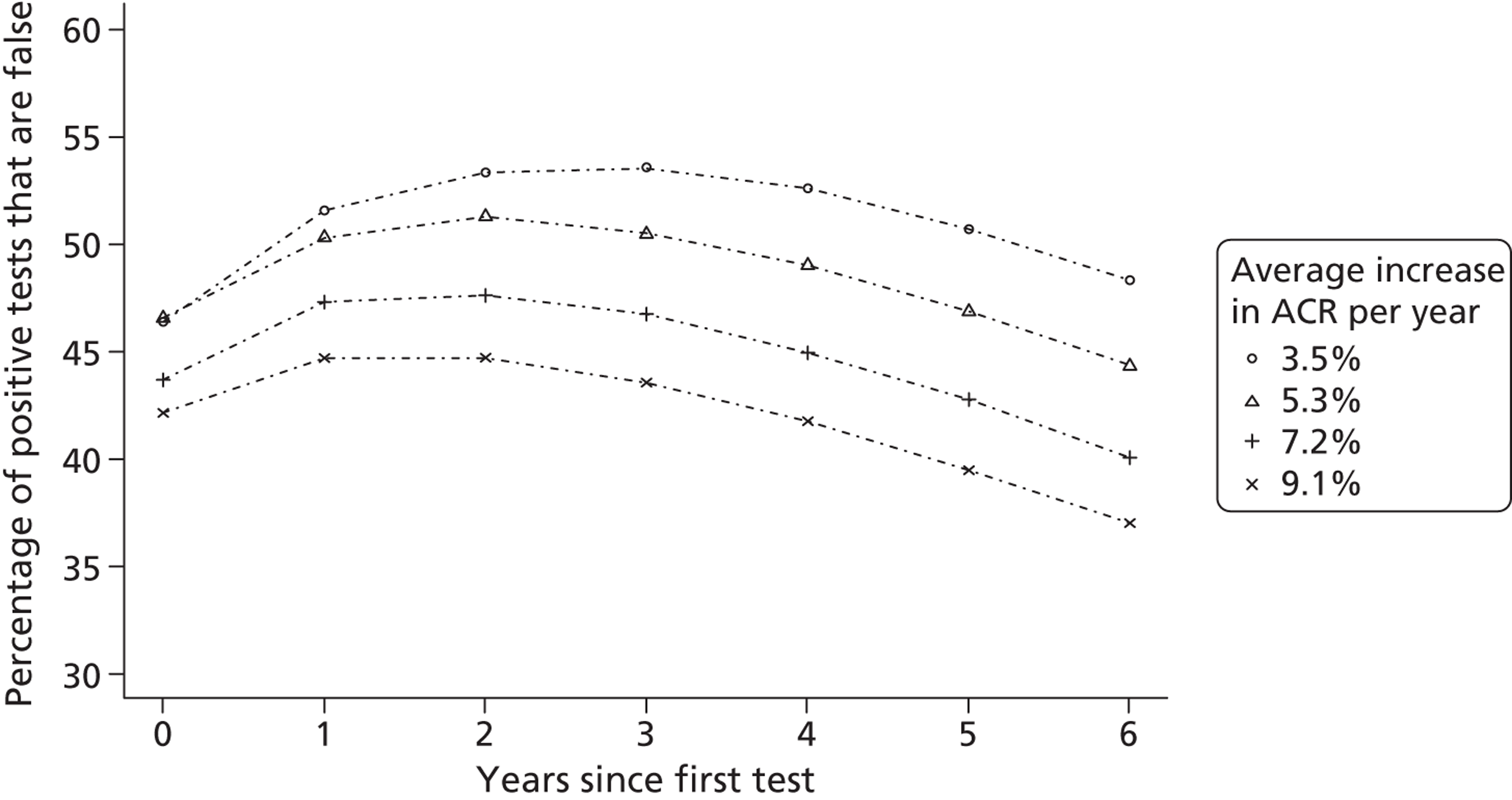
Glomerular filtration rate
During 463 person-years of follow-up on 386 patients (aged 5–27 years) with a total of 752 eGFR measurements, there were no cases of eGFR < 60 ml/minute. Table 12 reports the parameters for change in eGFR in the cohort. The estimated average decrease in eGFR was 2.1 (95% CI 0.39 to 3.7) ml/minute per year in males and 3.0 (95% CI 1.8 to 4.3) ml/minute per year in females. The estimated between-person standard deviation of the decrease was 3.3 (95% CI 1.8 to 4.7) ml/minute per year, so 74% of male patients and 82% of female patients will have a decreasing eGFR. The within-measurement variability of eGFR has an estimated standard deviation of 22 (95% CI 20 to 23) ml/minute, giving average signal-to-noise ratios for annual, biennial and triennial screening, respectively, of 0.095, 0.19 and 0.29 in males and 0.14, 0.27 and 0.41 in females.
Parameter | Interpretation | Parameter estimate (95% CIs) |
---|---|---|
αm | Mean eGFR at 6 years’ duration of diabetes in males | 165 (159 to 170) |
αw | Mean eGFR at 6 years’ duration of diabetes in females | 160 (155 to 165) |
λ | Effect of age at diagnosis of diabetes on mean eGFR (per year of age) | −2.0 (−2.9 to −1.1) |
σa | Between-person standard deviation of eGFR | 28 (25 to 31) |
βm | Mean annual increase in eGFR in men | −2.1 (−3.7 to −0.39) |
βw | Mean annual increase in eGFR in women | −3.0 (−4.3 to −1.8) |
σb | Standard deviation of annual increase in eGFR | 3.3 (1.8 to 4.7) |
ρ | Correlation between mean eGFR and annual rate of change | 0.14 (−0.22 to 0.44) |
σw | Standard deviation of within-measurement variability of eGFR | 22 (20 to 23) |
Discussion
The high short-term variability of ACR measurements results in a high number of false-positive diagnoses of microalbuminuria. This is consistent with previous studies of the long-term monitoring of other chronic conditions such as hypertension and hypercholesterolaemia. 134,135 However, in the case of ACR monitoring, the within-measurement variability is so high that even the practice of confirming a high result with further tests does not prevent a high rate of false-positive diagnoses. By comparison, the rate of false-negative diagnoses is small (Tables 10 and 11).
Limitations of the analysis include the use of a relatively young cohort collected in the UK around 20 years ago. Nevertheless, our model appears to make predictions for rates of microalbuminuria much later in the course of type 1 diabetes that match other studies (see Figure 10), despite the difficulties in modelling a measurement (ACR) that is far from normally, or even log-normally, distributed (see Figures 8 and 9). Our model predicts a levelling off of the prevalence of diabetic kidney disease, which corresponds with the clinical observation that not all patients will inevitably develop kidney disease, and with the reported course of comparator studies charted in Figure 4b.
Guidelines from the American Diabetes Association (ADA) and NICE15,148 agree in recommending annual screening for microalbuminuria, but differ on when it should begin: from 10 years of age and 5 years’ duration (ADA) and from 12 years of age (NICE). However, whereas the ADA guidelines recommend ACEi for young people with persistent elevated ACR, no such recommendation exists in the NICE guidelines for young people with type 1 diabetes and the recommendation is made only for adults. The potential benefit of this treatment for adolescents at high risk is being explored in an ongoing randomised trial,149 which includes adolescents with persistently elevated albumin levels within the normal range. This trial may indicate whether those detected with intermittent microalbuminuria are at increased risk of a wider range of adverse outcomes, regardless of whether they subsequently develop microalbuminuria. If ACEi does prove beneficial in a young population, then alternative strategies for screening would be needed to better target those who might benefit.
Our estimates of trend and variation for eGFR are limited by the known difficulties with eGFR formulae in young age groups. Further, it was not appropriate to model the rate of false-positive tests for low eGFR because of a lack of data: there were no cases of low eGFR in the cohort. This may partly reflect the relatively young cohort and, in addition, the difficulties in estimating GFR in this age group. However, data from the DCCT/EDIC (Epidemiology of Diabetes Interventions and Complications) cohort suggest that, even much later in the course of type 1 diabetes, decline in eGFR is almost exclusively a feature of patients with elevated urine albumin. 150 The same study also found that, at the time of first diagnosis of microalbuminuria, only 7% of people with type 1 diabetes are already being treated with ACEi or A2RB treatment. 1 Therefore, in type 1 diabetes, urine albumin testing is more likely to have an important role than eGFR in identifying people requiring ACEi or A2RB treatment.
In Chapter 2 we found evidence that ACEi or A2RB is highly beneficial in patients with type 1 diabetes and microalbuminuria, but not in patients with type 1 diabetes and normoalbuminuria. The clinical implication of our results for annual ACR screening is that approximately one in every three patients for whom ACEi or A2RB is recommended because of a diagnosis of microalbuminuria is in fact normoalbuminuric, and has much less to gain from the treatment. Less frequent screening (biennial or triennial) results in fewer false-positive tests and, therefore, better targeting of treatment – but rates of treatment overall are slightly lower (see Tables 10 and 11). In Chapters 5 and 6, we extend the work of this chapter to estimate the relative cost-effectiveness of annual, biennial and triennial monitoring of renal function with ACR in patients with type 1 diabetes.
Chapter 4 Modelling progression of kidney disease in type 2 diabetes
Background
In this chapter, we have applied the methods described in Chapter 3 to data from the Collaborative Atorvastatin Diabetes Study (CARDS) to examine the impact of screening interval on detection of kidney disease in a UK population with type 2 diabetes. As in Chapter 3, we use statistical estimation to quantify between-patient variation in both renal function and rate of change of renal function, as well as the natural variability of biological measures that leads to error in diagnosis. We use simulation models to estimate whether screening annually, or at 2- or 3-yearly intervals, is likely to produce more false- than true-positive diagnoses of microalbuminuria: that is, more likely to detect ‘measurement error’ than ‘true change’ in albuminuria status.
Methods
Data
The randomised trial CARDS was a trial of atorvastatin versus placebo in people with type 2 diabetes. The protocol and the characteristics of the study cohort have been described in full elsewhere. 151–153 Briefly, 132 clinical centres in the UK and Ireland recruited 2832 people with type 2 diabetes aged 40–75 years with low-density lipoprotein cholesterol ≤ 4.14 mmol/l, fasting triglycerides ≤ 6.78 mmol/l and at least one of the following conditions: hypertension, retinopathy, micro- or macroalbuminuria and current smoking status. Exclusion criteria included previous coronary heart disease or stroke, previous surgery for peripheral vascular disease, plasma creatinine ≥ 150 µmol/l, and HbA1c ≥ 12%. Patients were recruited between 1997 and 2001. Safety parameters, including urine albumin and urine creatinine, were measured 1, 2, 3 and 6 months after randomisation, and at 6-monthly intervals thereafter. Albumin and creatinine were measured using a Hitachi 747 autoanalyser (Boehringer Mannheim Corp, Indianapolis, IN) on urine samples that were confirmed free of infection by dipstick testing. At the recommendation of the data monitoring board, the study was stopped in June 2003 following an interim analysis of the primary cardiovascular end point, which showed a highly significant (p < 0.001) benefit of atorvastatin.
Statistical analysis
As described in Chapter 3, we fitted longitudinal models for trends and variation in log-ACR. Log-ACR at diagnosis of diabetes was modelled as a patient-level, normally distributed random effect. Change over time in log-ACR was modelled as a patient-level, normally distributed random effect, correlated with actual value of ACR. Within-measurement variability in log-ACR was modelled using a t-distribution with three degrees of freedom, uncorrelated with actual level of ACR. The model parameters were estimated using WinBUGS (MRC Biostatistics Unit, Cambridge, UK). 139 We also fitted models for trends and variation in eGFR using the same methods as described above, except for the modelling of the within-measurement variability, for which we used a normal distribution. Residual plots indicated an approximate normal distribution for eGFR and, therefore, a log transformation was not required. We allowed mean eGFR and mean rate of change to vary between men and women, but held other parameters the same for men and women. Models were stratified by baseline urine albumin status. We report means and standard deviations of log- and eGFR, geometric means of ACR and the average signal-to-noise ratio as defined in Chapter 3.
Although we did not have data with which to validate individual tests for microalbuminuria in the CARDS database, the model estimates of within-person variability allowed us to estimate the proportion of positive tests that were false positive – that is, attributable to measurement variability rather than to true change in underlying renal function. With the same methodology as described in Chapter 3, we used simulation modelling to calculate the proportion of those identified with true- and false-positive tests in a population similar to that of CARDS, under the assumption of annual screening according to NICE guidelines. Our primary analysis models an annual screening programme as described above, and compares this to 2-yearly screening (biennial) and 3-yearly screening (triennial), while maintaining the NICE protocol for use of confirmatory samples when the first sample measures above threshold. In sensitivity analyses, we assess the degree of change in model parameters that would be required to reach different clinical conclusions. We also used simulation to estimate rates and accuracy of diagnoses of stage 3b kidney disease, defined by eGFR < 45 ml/minute in normoalbuminuric patients.
Results
The characteristics of the participants in the CARDS included in this analysis are shown in Table 13. Data from 2562 participants were eligible for inclusion in this analysis.
Variable | Number of participants | Value [median (Q1, Q3)] |
---|---|---|
Gender | ||
Male | 1780 | 69.5% |
Female | 782 | 30.5% |
Age at diagnosis (years) | 2562 | 53 (46, 60) |
Duration of diabetes (in years) | 2562 | 7 (3, 11) |
HbA1c (mmol/mol) | 2562 | 61 (51, 72) |
Body mass index (kg/m2) | 2562 | 31 (28, 34) |
Systolic blood pressure (mmHg) | 2562 | 143 (134, 153) |
Diastolic blood pressure (mmHg) | 2562 | 83 (78, 88) |
eGFR (ml/minute) | 2562 | 64 (58, 71) |
ACR | 2562 | 1.10 (0.58, 2.78) |
Microalbuminuriab | ||
Yes | 550 | 21.5% |
No | 2012 | 78.5% |
Albumin-to-creatinine ratio
Table 14 reports the parameters used in defining the model that estimates trends and variation in log-ACR. The mean log-ACR of 0.41 (95% CI 0.28 to 0.53) in men and 0.29 (95% CI 0.11 to 0.48) in women are equivalent to geometric mean ACR at diagnosis of diabetes of 1.51 (95% CI 1.32 to 1.70) mg/mol and 1.34 (95% CI 1.12 to 1.62) mg/mol, respectively. The average change in log-ACR per year of 0.018 corresponds to a 2% per year increase in ACR in both sexes (Figure 12). The standard deviation around this average, 0.022 on the log-ACR scale, corresponds to variation in the rate of change: the estimated 25th and 75th centiles of the annual increase in ACR are a 0.3% and 3.3% increase per year, respectively. The estimated standard deviation of within-measurement variability in log-ACR is 0.85 (95% CI 0.74 to 1.00), which corresponds to a within-measurement coefficient of variation in ACR > 100%. The average signal-to-noise ratios, defined as average change divided by within-measurement standard deviation, are therefore 0.021, 0.042 and 0.063 for annual, biennial and triennial screening, respectively.
Parameter | Interpretation | Parameter estimate |
---|---|---|
αm | Mean log-ACR at 10 years’ duration of diabetes in men | 0.41 (0.28 to 0.53) |
αw | Mean log-ACR at 10 years’ duration of diabetes in women | 0.29 (0.11 to 0.48) |
λ | Effect of age at diagnosis on mean log-ACR | 0.00092 (−0.01 to 0.012) |
σa | Standard deviation of log-ACR | 1.1 (1.0 to 1.2) |
βm | Mean annual change in log-ACR in men | 0.018 (−0.00094 to 0.035) |
βw | Mean annual change in log-ACR in women | 0.018 (−0.0075 to 0.038) |
σb | Standard deviation of annual change in log-ACR | 0.022 (0.0052 to 0.042) |
ρ | Correlation between actual value of log-ACR and rate of change | 0.66 (0.09 to 0.96) |
σw | Standard deviation of within-measurement variability of log-ACRa | 0.85 (0.74 to 1.00) |
FIGURE 12.
Estimated cumulative proportion of patients with microalbuminuria and macroalbuminuria drawn from a cohort based on CARDS using parameters obtained from CARDS (modelled ACR) compared with data obtained directly from the CARDS cohort. The circles and lines are mean (95% CI) of ACR by duration (0–1, 1–2, 2–3, etc.) stratified by gender (men top, women bottom). The numbers are based on the same 535 who made up the model.
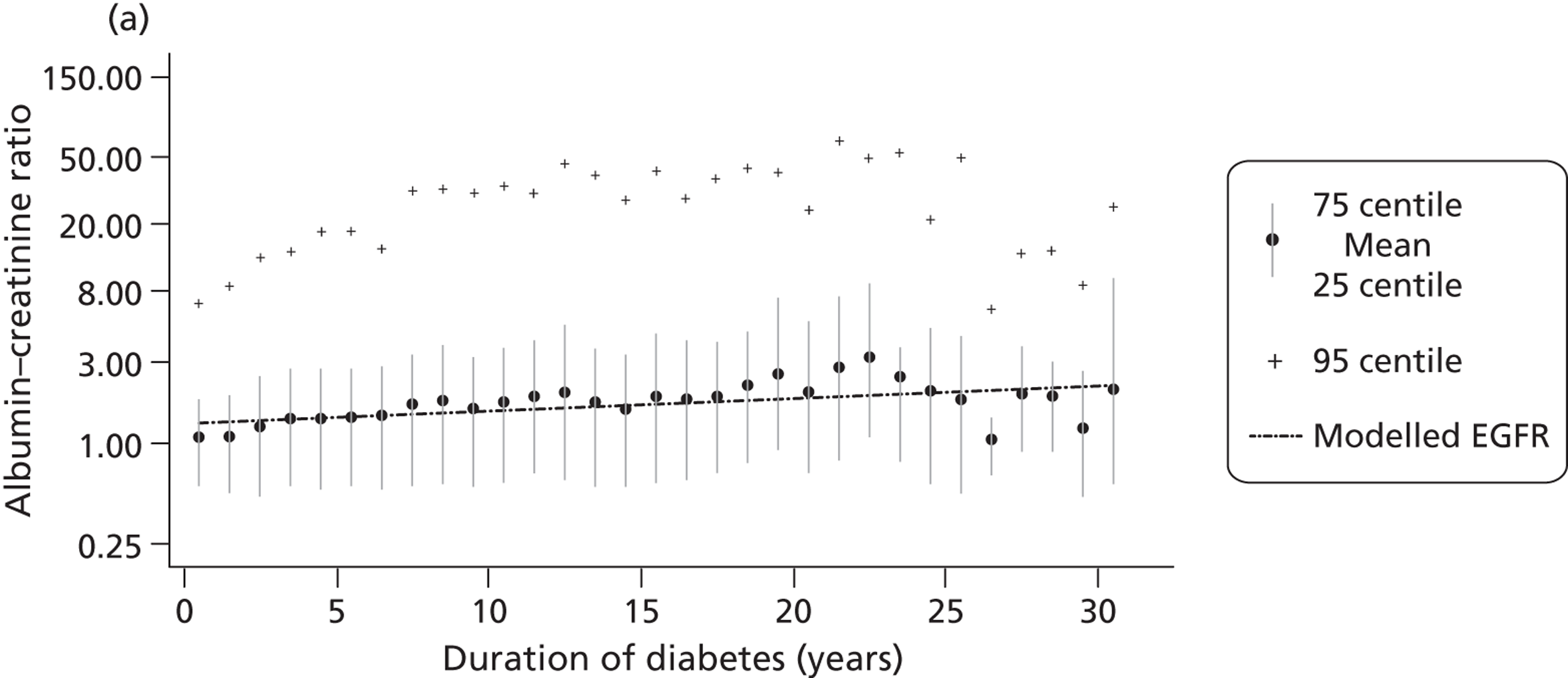
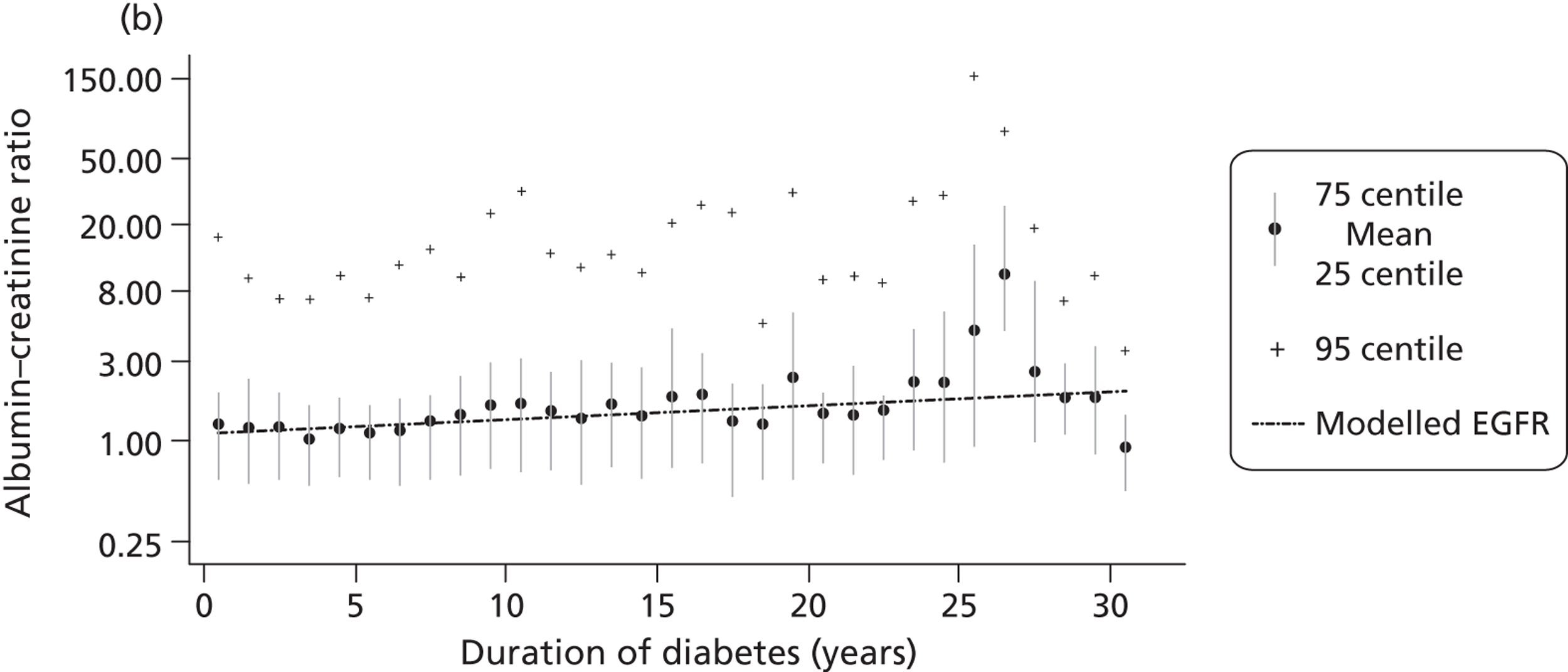
Tables 15 and 16 report the estimated rates of identification of microalbuminuria modelled from these estimates. Results for men and women are shown separately (see Tables 15 and 16). The tables show the cumulative rate of positive test results, the percentage of those that are false positive and the percentage of those repeatedly testing negative that are false negative. Each of these outcomes is shown under assumptions of annual, biennial and triennial screening.
Years since first test | Cumulative number of positive tests per 1000 patients (95% CI) | Percentage of those testing positive who have a false-positive result (95% CI) | Percentage of those with repeated negative tests who have a false-negative result (95% CI) | ||||||
---|---|---|---|---|---|---|---|---|---|
Annual testing | Biennial testing | Triennial testing | Annual testing | Biennial testing | Triennial testing | Annual testing | Biennial testing | Triennial testing | |
0 | 220 (159 to 289) | 220 (159 to 289) | 220 (159 to 289) | 22 (16 to 30) | 22 (16 to 30) | 22 (16 to 30) | 8.7 (6 to 11) | 8.7 (6 to 11) | 8.7 (6 to 11) |
1 | 298 (231 to 371) | 27 (21 to 36) | 4.5 (3 to 6) | ||||||
2 | 347 (279 to 420) | 302 (239 to 370) | 31 (25 to 38) | 26 (21 to 33) | 2.6 (2 to 3) | 4.8 (4 to 6) | |||
3 | 382 (315 to 453) | 307 (247 to 373) | 33 (28 to 40) | 25 (21 to 31) | 1.6 (1 to 2) | 5.1 (4 to 6) | |||
6 | 451 (391 to 514) | 394 (338 to 455) | 365 (309 to 425) | 36 (32 to 42) | 30 (26 to 35) | 27 (23 to 31) | 0.5 (0 to 1) | 2.1 (1 to 3) | 3.5 (3 to 4) |
12 | 530 (481 to 578) | 477 (430 to 524) | 446 (400 to 493) | 37 (30 to 44) | 30 (25 to 37) | 27 (22 to 34) | 0.2 (0 to 1) | 1.1 (0 to 2) | 2.2 (1 to 4) |
18 | 582 (536 to 627) | 534 (487 to 579) | 505 (458 to 550) | 35 (26 to 44) | 29 (22 to 39) | 26 (19 to 36) | 0.1 (0 to 0) | 0.8 (0 to 2) | 1.7 (0 to 3) |
Years since first test | Cumulative number of positive tests per 1000 patients (95% CI) | Percentage of those testing positive who have a false-positive result (95% CI) | Percentage of those with repeated negative tests who have a false-negative result (95% CI) | ||||||
---|---|---|---|---|---|---|---|---|---|
Annual testing | Biennial testing | Triennial testing | Annual testing | Biennial testing | Triennial testing | Annual testing | Biennial testing | Triennial testing | |
0 | 151 (83 to 228) | 151 (83 to 228) | 151 (83 to 228) | 28 (19 to 41) | 28 (19 to 41) | 28 (19 to 41) | 5.7 (3 to 9) | 5.7 (3 to 9) | 5.7 (3 to 9) |
1 | 212 (130 to 297) | 35 (26 to 46) | 2.8 (2 to 4) | ||||||
2 | 249 (164 to 337) | 213 (136 to 296) | 39 (32 to 49) | 34 (27 to 43) | 1.5 (1 to 2) | 2.9 (2 to 4) | |||
3 | 277 (192 to 364) | 213 (139 to 289) | 43 (35 to 50) | 33 (27 to 41) | 0.9 (1 to 1) | 2.9 (2 to 4) | |||
6 | 331 (253 to 410) | 282 (208 to 358) | 255 (182 to 327) | 48 (41 to 55) | 40 (34 to 47) | 37 (30 to 44) | 0.2 (0 to 0) | 1.0 (1 to 2) | 1.8 (1 to 3) |
12 | 392 (326 to 456) | 342 (280 to 406) | 312 (251 to 373) | 50 (39 to 62) | 44 (32 to 56) | 39 (28 to 53) | 0.1 (0 to 0) | 0.5 (0 to 1) | 1.0 (0 to 2) |
18 | 431 (369 to 497) | 382 (322 to 448) | 354 (292 to 420) | 50 (35 to 68) | 44 (29 to 63) | 40 (25 to 59) | 0.1 (0 to 0) | 0.3 (0 to 1) | 0.7 (0 to 2) |
Over the first 6 years after diagnosis of diabetes, annual, biennial and triennial screening would, respectively, identify 451, 394 and 365 cases of microalbuminuria per 1000 men with type 2 diabetes. Over 18 years, annual, biennial and triennial screening would identify 582, 534 and 505 cases, respectively.
In an initial screening test, an estimated 78% of those identified with microalbuminuria would be true positive, and 22% would be false positive. By year 6, with assumptions of annual, biennial and triennial screening, respectively, 36%, 30% and 27% of diagnoses of microalbuminuria in men would be false positive, and, by year 18, 35%, 29% and 26%. By year 18, estimated false-negative rates for men were 0.1%, 0.8% and 1.7% for annual, biennial and triennial screening, respectively.
Table 16 shows the corresponding estimates for women with type 2 diabetes. Over 18 years, we estimate that annual, biennial and triennial screening, respectively, would identify 431, 382 and 354 cases of microalbuminuria per 1000 women with type 2 diabetes, 50%, 44% and 40% of which would be false positive. Of those testing negative for microalbuminuria, 0.1%, 0.3% and 0.7% would be false negative.
In summary, in men and women with type 2 diabetes, 36% (95% CI 32% to 42%) and 48% (95% CI 41% to 55%), respectively, of patients tested annually for microalbuminuria over 6 years would be inaccurately identified as having microalbuminuria. If screening intervals were extended to 3-yearly, the corresponding figures would be 27% (95% CI 23% to 31%) and 37% (95% CI 30% to 44%).
Figure 13 shows the degree to which the model reproduces the CARDS data by comparing model predictions to observed data. Figure 14 examines the extent to which the resulting model can be generalised by comparing model-predicted rates of microalbuminuria identification to observed prevalence of microalbuminuria in other cohort studies, with the limitation that different studies have used different criteria (e.g. number of confirmatory tests) for diagnosing microalbuminuria.
FIGURE 13.
Estimated prevalence of normo-, micro- and macroalbuminuria, and estimated incidence of apparent regression from microalbuminuria to normoalbuminuria (dots), with 95% CIs (vertical lines), in the CARDS compared with model estimates using parameters obtained from CARDS (modelled ACR) (dashed lines). (a) Normoalbuminuria; (b) microalbuminuria; (c) macroalbuminuria; and (d) regression to normoalbuminuria.
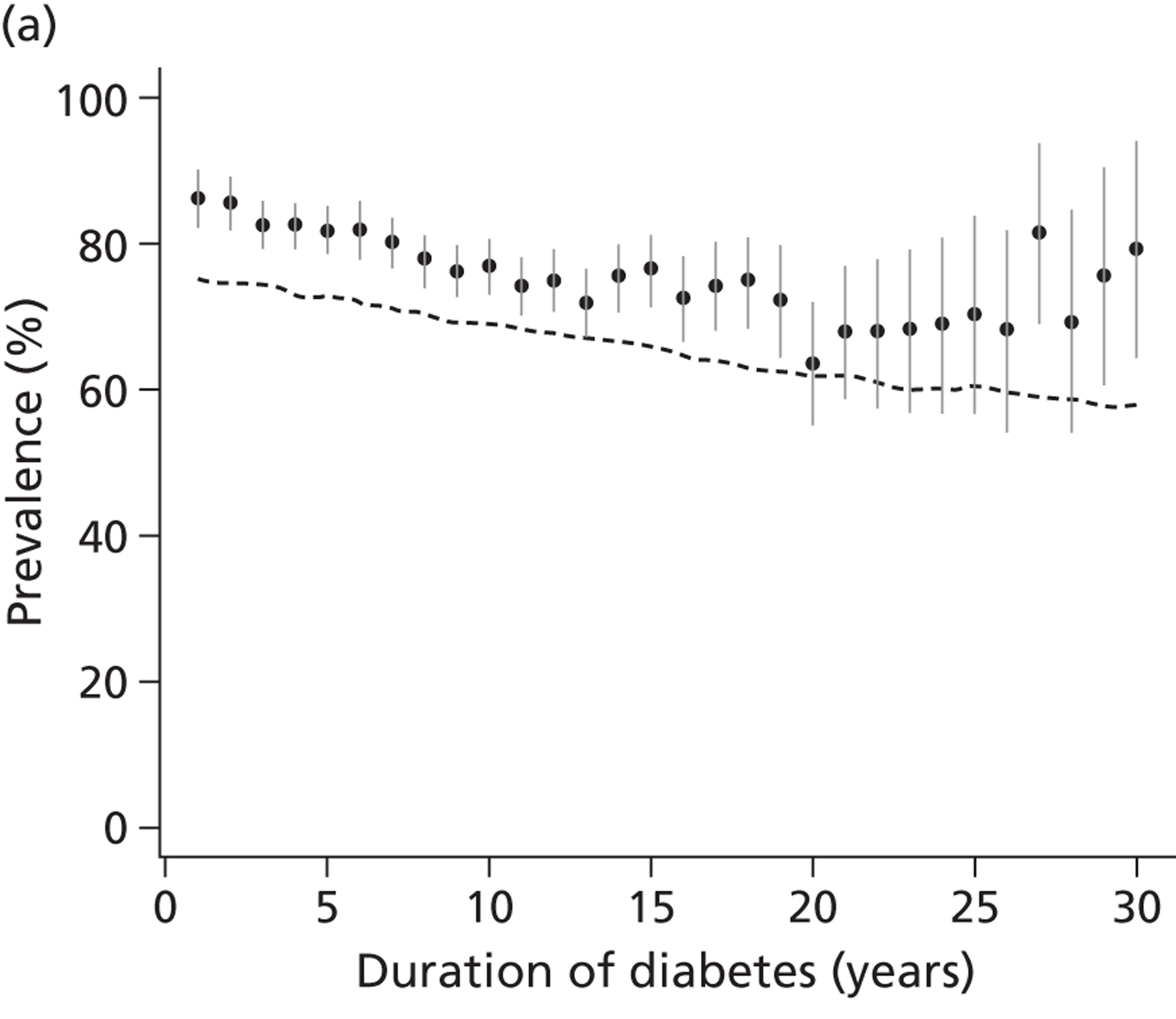
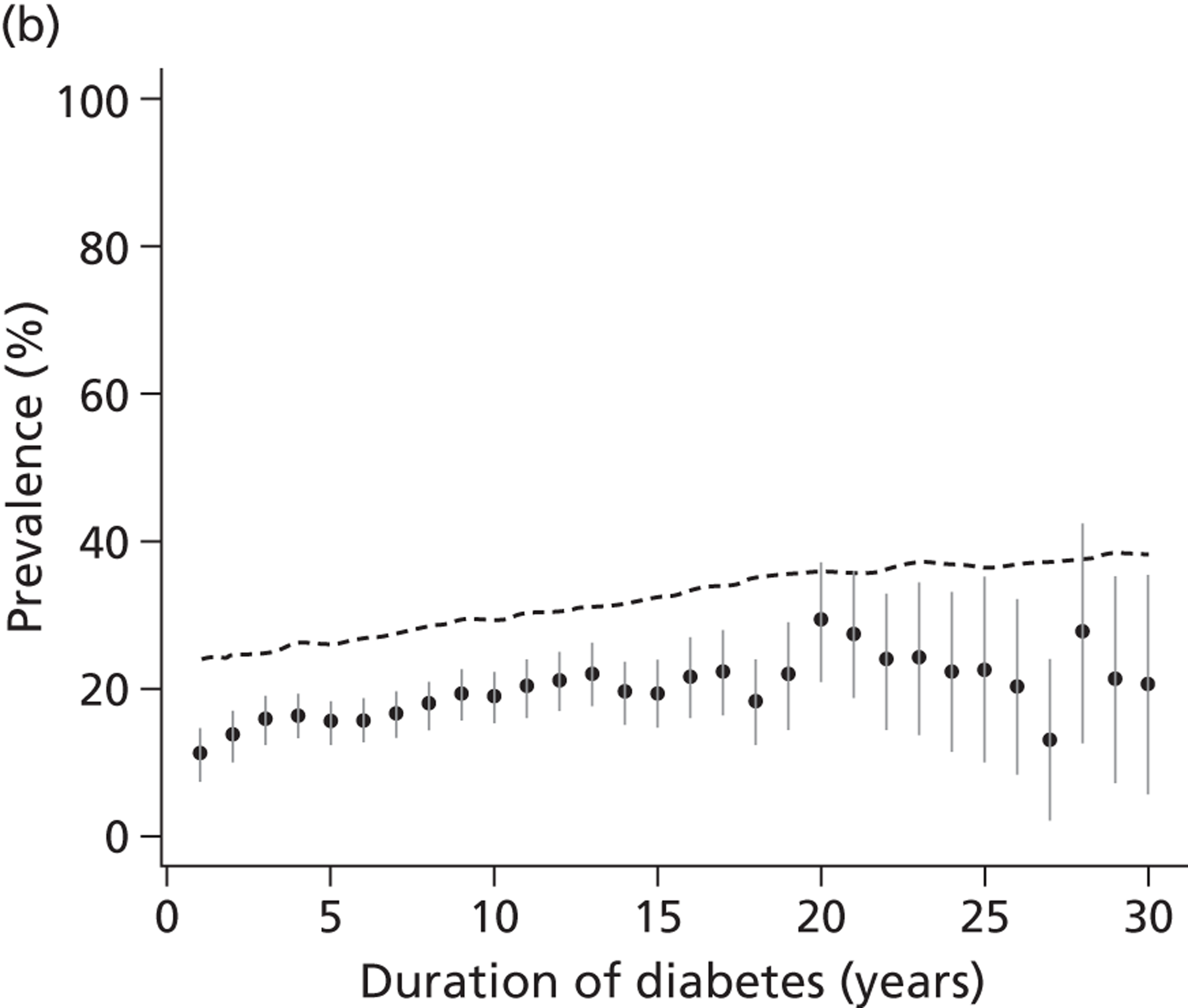
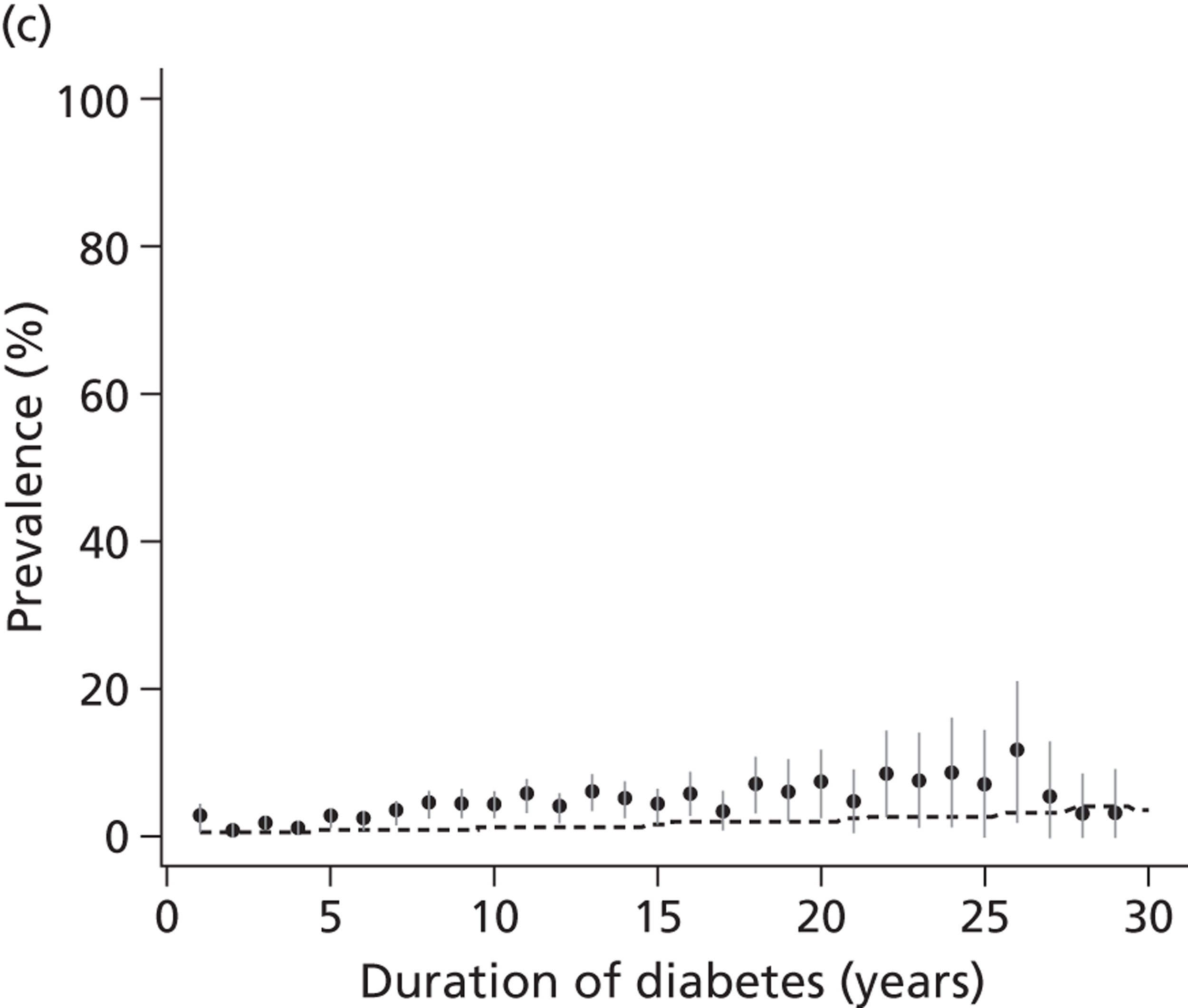
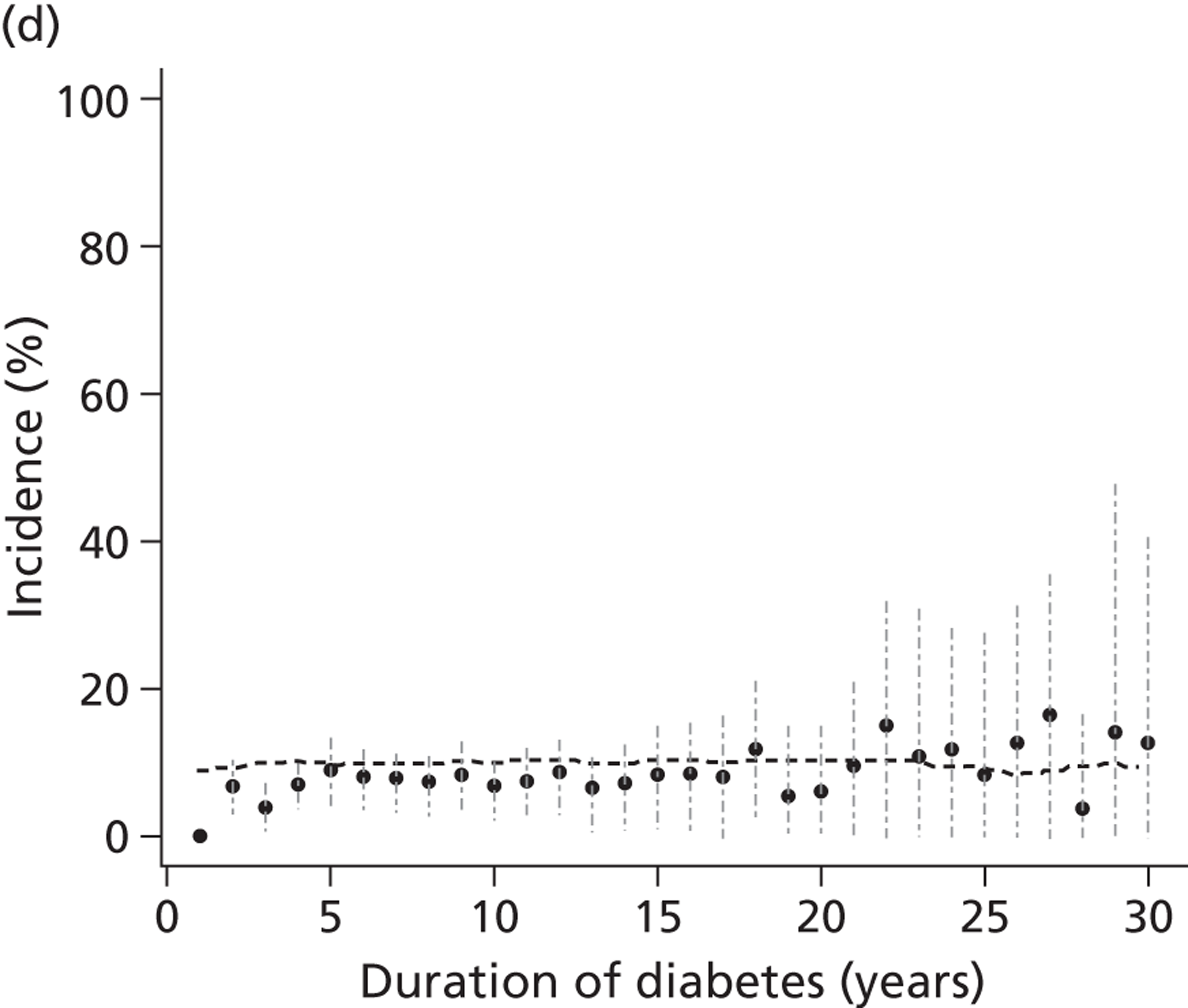
FIGURE 14.
Proportion of patients who test positive for microalbuminuria or macroalbuminuria by duration of diabetes estimated from parameters obtained from the CARDS (simulation model) compared with other cohort studies in type 2 diabetes. Bars and whiskers denote 95% CIs.
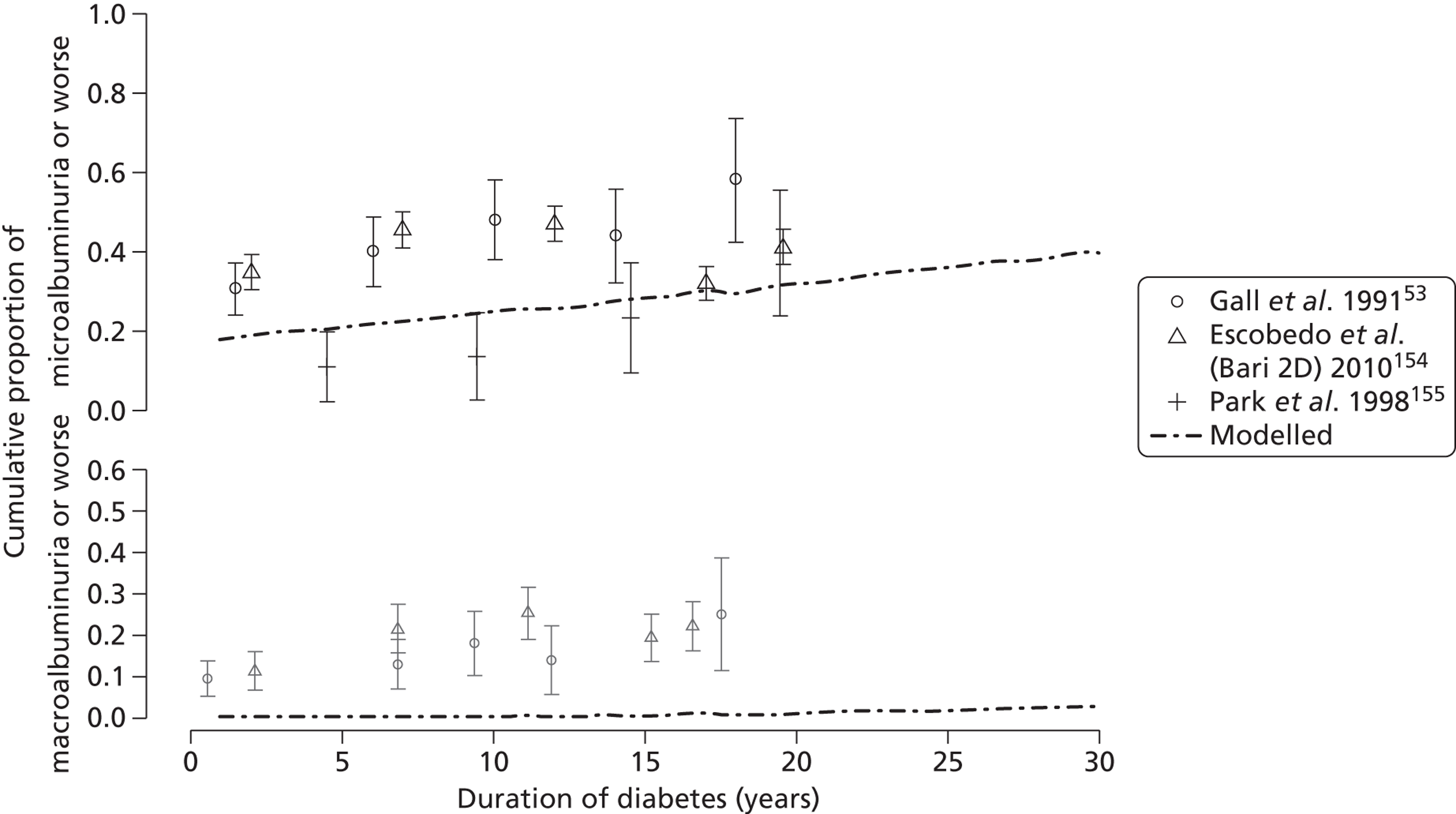
To illustrate the sensitivity of our conclusions to the exact parameter values, Figure 15 shows how the estimated false-positive rate would vary under different assumptions about the average rate of progression of log-ACR. This illustrates the change in the number of false-positive tests when the average rate of change in ACR per year used within the model is increased above that observed in our cohort.
FIGURE 15.
Sensitivity of model predictions to variation in parameter estimates. The lower line of the graph plots the percentage of positive tests that are false (numbers in the fifth column of Table 15) for males with type 2 diabetes with annual monitoring. Other lines represent the percentage of positive tests that are false when the average slope (1.8%) is multiplied by factors of 1.5, 2 and 2.5.
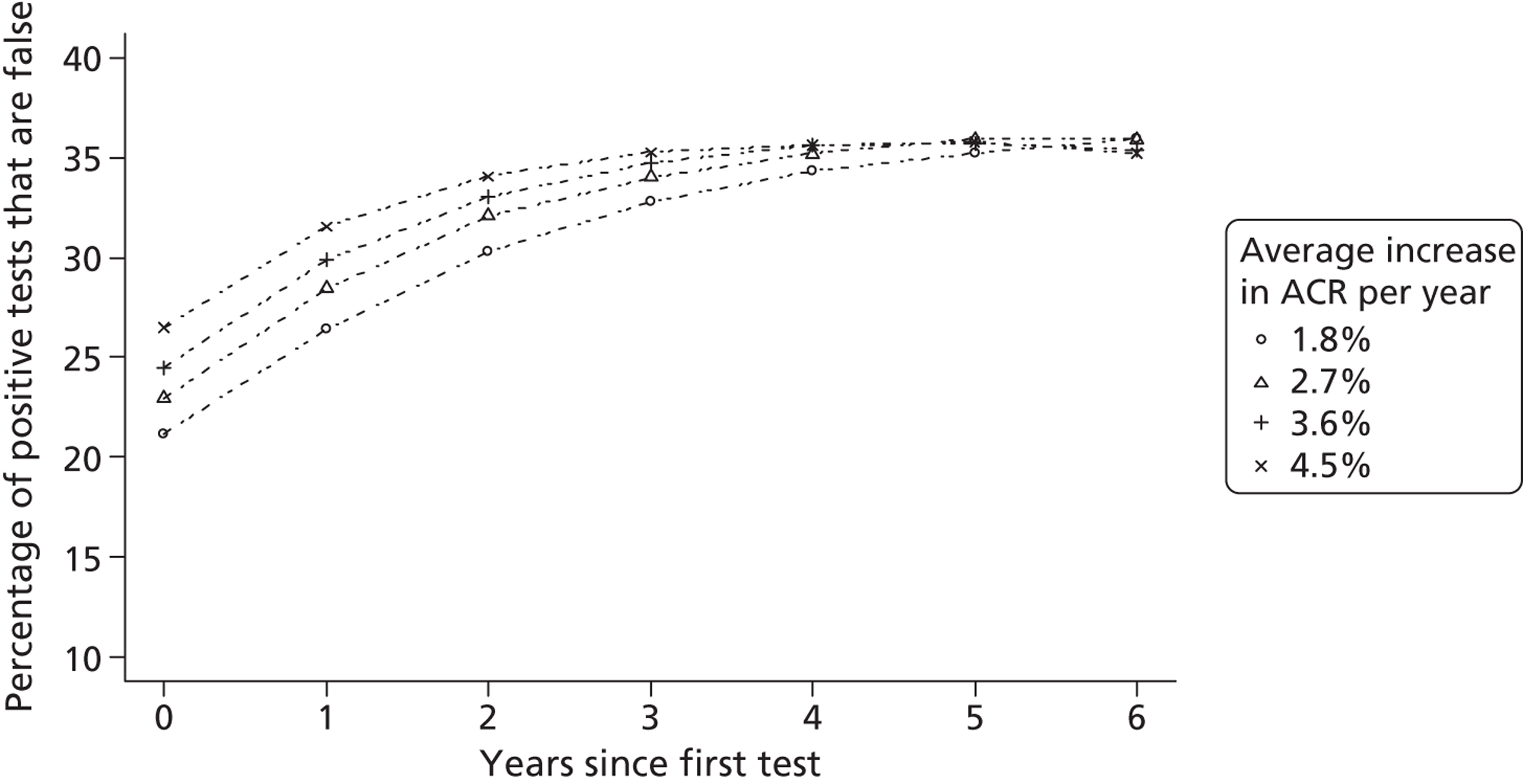
Estimated glomerular filtration rate
Data on 2012 CARDS participants with normoalbuminuria at baseline and 550 CARDS participants with microalbuminuria at baseline were used in obtaining model estimates. Table 17 reports the parameters used in defining the model that estimates trends and variation in eGFR in people with type 2 diabetes and normoalbuminuria. The estimated mean eGFR at 10 years since diagnosis of diabetes is 69 (95% CI 69 to 70) ml/minute in men and 62 (95% CI 61 to 64) ml/minute in women, and the between-person standard deviation is 8.7 (95% CI 8.1 to 9.3) ml/minute. The estimated average change in eGFR per year is 0.26 (95% CI 0.13 to 0.40) ml/minute in men, and 0.24 (95% CI 0.061 to 0.42) ml/minute in women. The estimated between-person standard deviation of the reduction per year is 0.50 (95% CI 0.28 to 0.69) ml/minute. The estimated standard deviation of within-measurement variation in eGFR is 4.9 (95% CI 4.8 to 5.1) ml/minute. The signal-to-noise ratio is therefore 0.053 for annual monitoring, 0.11 for biennial monitoring and 0.16 for triennial monitoring. Table 17 also shows the corresponding parameter estimates for participants with normoalbuminuria and microalbuminuria at baseline. Figure 16 illustrates the observed mean eGFR by duration of diabetes compared with the modelled estimates of progression and shows how the modelled rate of change matches the original cohort data.
Parameter | Interpretation | With type 2 diabetes and normoalbuminuria (95% CI) | With type 2 diabetes and microalbuminuria (95% CI) |
---|---|---|---|
αm | Mean eGFR at 10 years’ duration of diabetes in men | 69 (69 to 70) | 67 (66 to 68) |
αw | Mean eGFR at 10 years’ duration of diabetes in women | 62 (61 to 64) | 60 (58 to 62) |
λ | Effect of age at diagnosis on mean eGFR (per year) | −0.58 (−0.66 to −0.50) | −0.61 (−0.72 to −0.50) |
σa | Between-person standard deviation | 8.7 (8.1 to 9.3) | 9.6 (8.9 to 10.0) |
βm | Mean annual increase in eGFR in men | 0.26 (0.13 to 0.40) | −0.13 (−0.27 to 0.014) |
βw | Mean annual increase in eGFR in women | 0.24 (0.061 to 0.42) | −0.048 (−0.35 to 0.23) |
σb | Standard deviation of annual increase in eGFR | 0.50 (0.28 to 0.69) | 0.72 (0.45 to 0.95) |
ρ | Correlation between mean eGFR and annual rate of change | 0.46 (0.25 to 0.82) | 0.42 (0.23 to 0.62) |
σw | Standard deviation of within-measurement variability of eGFR | 4.9 (4.8 to 5.1) | 5.0 (4.8 to 5.2) |
FIGURE 16.
Observed mean (dot) and 25th and 75th centiles (vertical lines) of eGFR in men (top) and women (bottom) with type 2 diabetes and normoalbuminuria, and model estimate of trends (dashed line).
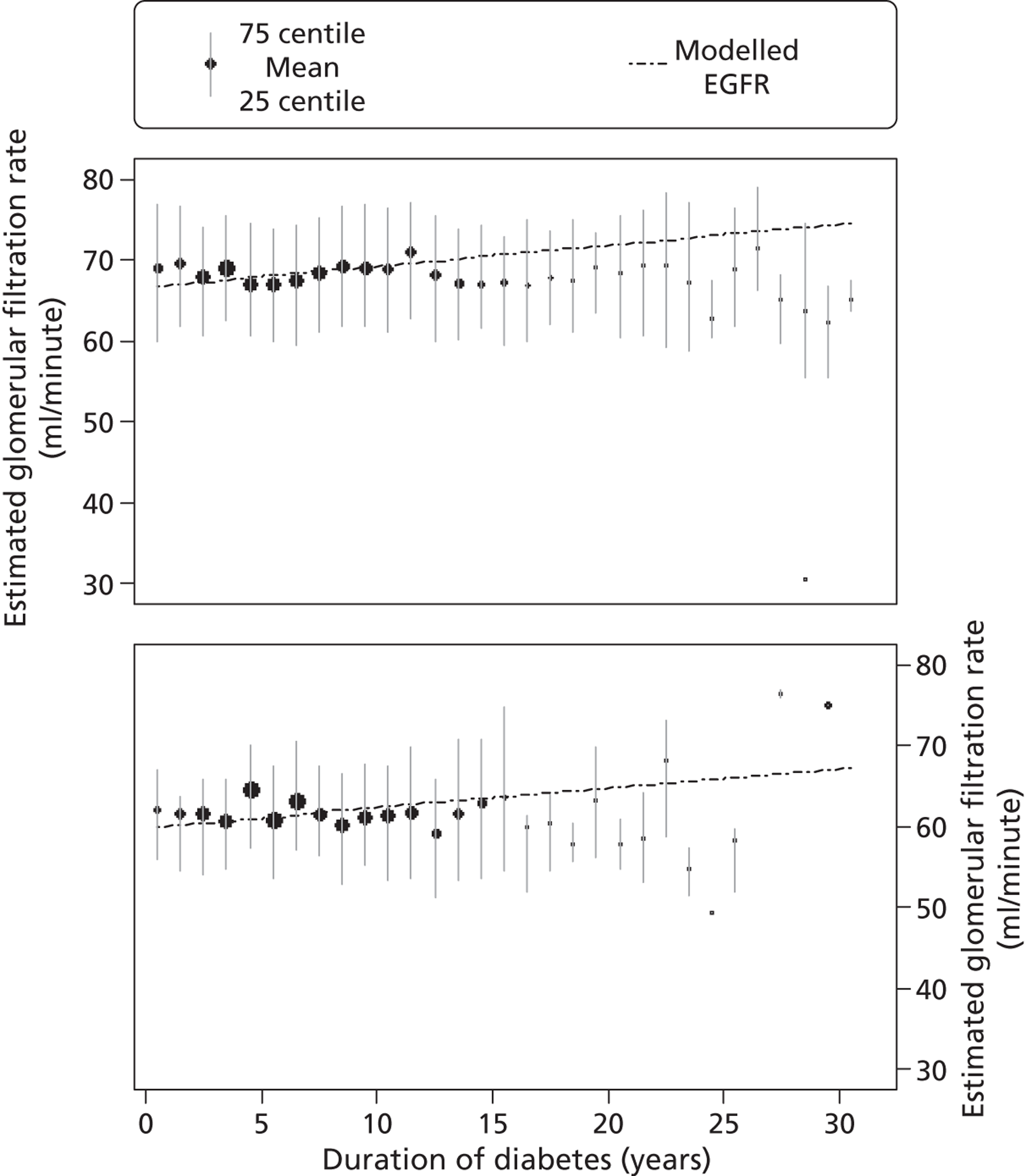
Table 18 shows the modelled estimates of the proportion of normoalbuminuric men classified as having stage 3b kidney disease (eGFR < 45 ml/minute) over time for annual, biennial or triennial eGFR screening. In annual screening, for example, there would be 20 men identified with stage 3b kidney disease (95% CI 16 to 26) per 1000 after 18 years, and 67% (95% CI 53% to 83%) of these would be false positive. Similar estimates were obtained for women (data not shown).
Years since first test | Cumulative number of positive tests per 1000 patients (95% CI) | Percentage of those testing positive who have a false-positive result (95% CI) | Percentage of those with repeated negative tests who have a false-negative result (95% CI) | ||||||
---|---|---|---|---|---|---|---|---|---|
Annual testing | Biennial testing | Triennial testing | Annual testing | Biennial testing | Triennial testing | Annual testing | Biennial testing | Triennial testing | |
0 | 4 (1 to 8) | 4 (1 to 8) | 4 (1 to 8) | 53 (41 to 68) | 53 (41 to 68) | 53 (41 to 68) | 0.1 (0.03 to 0.24) | 0.1 (0.03 to 0.24) | 0.1 (0.03 to 0.24) |
1 | 6 (2 to 12) | 63 (54 to 72) | 0 (0.02 to 0.08) | ||||||
2 | 7 (3 to 13) | 5 (2 to 10) | 70 (63 to 77) | 66 (58 to 74) | 0 (0.01 to 0.03) | 0 (0.02 to 0.07) | |||
3 | 8 (4 to 14) | 5 (2 to 10) | 75 (69 to 81) | 67 (60 to 75) | 0 (0.00 to 0.02) | 0 (0.02 to 0.06) | |||
6 | 10 (5 to 16) | 7 (4 to 13) | 6 (3 to 11) | 81 (72 to 88) | 76 (66 to 85) | 73 (62 to 83) | 0 (0.00 to 0.01) | 0 (0.01 to 0.03) | 0 (0.01 to 0.04) |
12 | 14 (9 to 21) | 11 (7 to 16) | 9 (6 to 14) | 78 (66 to 89) | 73 (58 to 87) | 70 (54 to 84) | 0 (0.00 to 0.03) | 0 (0.01 to 0.06) | 0 (0.01 to 0.09) |
18 | 20 (14 to 30) | 16 (11 to 25) | 14 (9 to 22) | 68 (53 to 83) | 62 (45 to 79) | 59 (42 to 76) | 0 (0.00 to 0.07) | 0.1 (0.01 to 0.17) | 0.1 (0.02 to 0.23) |
Discussion
As for type 1 diabetes (Chapter 3), we found that the short-term variability of ACR measurement is so great that the variability itself accounts for a high proportion of microalbuminuria diagnoses, even though these diagnoses are based on repeated testing of an individual. Replacing current practice with biennial or triennial screening would reduce the total number of false-positive diagnoses. Under all schemes the false-negative rate – people with underlying microalbuminuria incorrectly classified as normoalbuminuria – is small compared with the false-positive rate. We also found that, in the majority of patients, eGFR does not decline until after the onset of microalbuminuria, which implies that urine albumin testing has a more important role to play than serum creatinine in the monitoring of renal function in type 2 diabetes.
Our model is subject to limitations such as the degree to which the CARDS cohort is typical of the general UK type 2 diabetic population. For example, the extent to which ACEi and A2RB treatment rates within a randomised trial of HME-CoA reductase inhibitors (statins) are representative of the general diabetic population is not known. However, Figure 14 suggests that microalbuminuria rates predicted by our model are not atypical of type 2 diabetes. The microalbuminuria results in Figure 14 also show good model fit over a wide timescale despite the relatively short follow-up time in CARDS. Although our exact numerical results are subject to limitations, the general principle that high within-measurement variability brings about repeated opportunity for false-positive results is not in doubt (Box 1), and Figure 15 illustrates that our broad conclusions can be quite robust even to large changes in a key model parameter.
This classification error of a screening programme with repeated testing (equivalent to a monitoring programme) is methodologically different from a diagnostic problem. At any given time point, classification error may occur in either direction, as in a diagnostic problem – either falsely making a positive diagnosis as microalbuminuria or falsely excluding the diagnosis of microalbuminuria. However, those classified as not having microalbuminuria are subsequently invited for further monitoring, with the potential for reclassification, whereas those identified as having microalbuminuria will never be reclassified as having normoalbuminuria under current practice. This creates an asymmetrical situation in which the risk of a false-positive ‘label’ accumulates with every recall for monitoring, with the result that more frequent monitoring increases the false-positive rate.
The clinical implication of the high number of false-positive diagnoses of microalbuminuria is overtreatment, with ACEi and A2RB, of patients whose diagnosis of microalbuminuria is in fact a result of measurement error rather than underlying renal dysfunction. The systematic review in Chapter 2 found that, in type 2 diabetes, even patients with normal renal function can benefit from ACEi and A2RB. This contrasts with the situation in type 1 diabetes, in which there is little evidence of benefit from treatment in normoalbuminuric people. It follows that the cost-effectiveness of monitoring people with type 2 diabetes for onset of microalbuminuria is difficult to predict. This, and the relative value of different monitoring intervals, is therefore the subject of a full cost-effectiveness modelling exercise in Chapter 6.
Chapter 5 Simulation models for type 1 and type 2 diabetes
This chapter outlines two health economic patient-level simulation models that we have used to integrate the information provided in the previous two chapters. These models were used to conduct the economic evaluation of the various options for monitoring renal function that are reported in the next chapter.
Patient-level simulation models are valuable tools in studying diabetes. The development of clinical outcomes for an individual patient, for example progression to renal failure, can take years and sometimes decades to become apparent. It is therefore both costly and potentially unethical to undertake clinical trials of sufficient duration to obtain long-term outcomes on all patients.
Individual-level simulation models were chosen to conduct the analyses required for this study because they can model both the progression of diabetes-related complications and quantify outcomes in terms of mean quality-adjusted life expectancy. 68 This approach to simulation modelling is more commonly used, instead of the more traditional Markov outcomes models, in diabetes156 because it accounts for heterogeneity in the characteristics of individuals and the interdependence of different diabetes-related complications. We have, wherever possible, conformed to the ADA guidelines for diabetes health economic modelling. 157
The analysis of screening programmes for diabetes reported in the next chapter is informed by a simulation method developed specifically for this project, and an adaption of an existing simulation model – the UKPDS outcomes model – for use in type 2 diabetes. The methods used to develop these models and their underlying assumptions are reported in this chapter.
A simulation model for type 1 diabetes
Patient-level simulation modelling is required to examine the cost-effectiveness of various monitoring strategies because there are no long-term randomised controlled trials of different screening strategies for type 1 diabetes. Patient-level simulation modelling, which projects outcomes and costs over long periods of time, can be used to determine the outcomes of the different strategies and thereby inform cost-effectiveness analysis of various screening strategies.
There are currently no published patient-level simulation models developed specifically to examine the impact of screening for diminished renal function in type 1 diabetes. Appendix 5 includes a summary of current diabetes health economic models. Patient-level simulation models are common in type 2 diabetes; there are Markov models of the progression of type 1 diabetes,158–160 and more general simulation models have been used for economic evaluation. 161 Most of the existing simulation models for use in type 1 diabetes have been developed using information from other patient populations to capture transitions between important health states. For example, the risk of CVD has been estimated from the Framingham study,162 or using equations developed with data obtained from type 2 diabetes patients in the UKPDS. 163 The validity of using Framingham- and UKPDS-derived risk equations to predict risk of CVD in a cohort of type 1 diabetes patients was recently tested and it concluded that these equations ‘poorly predict events in type 1 diabetes’. 164
We have, therefore, developed a patient-level simulation model for type 1 diabetes that has been specifically designed to evaluate options for monitoring renal function. The model draws on results from several recent studies published after the previous type 1 diabetes models were developed. The new model uses data from a wide variety of sources, with the majority of parameters derived from data of studies involving type 1 diabetes patients. When, for specific parameters, there were few or no type-1-specific data available, we supplemented the model by including studies of type 2 diabetes patients.
Model structure
The type 1 diabetes simulation model is built around a series of ‘key health states’ and ‘interlinking dependencies’. Figure 17 shows the different states of the model and how a type 1 diabetes patient can progress through its different states over their lifetime. The aim of this model is to estimate the first occurrence of a cardiovascular event (including stroke or a myocardial infarction) for type 1 diabetes patients, either with or without end-stage kidney disease and death, in order to estimate lifetime outcomes and quality-adjusted life expectancy associated with different screening programmes. The arrows indicate dependencies linking key health states. For example, the occurrence of kidney disease elevates the risk of myocardial infarction and death.
FIGURE 17.
Diagram depicting the structure and relationship among equations, disease progression, screening and treatment steps used in the type 1 diabetes simulation model.
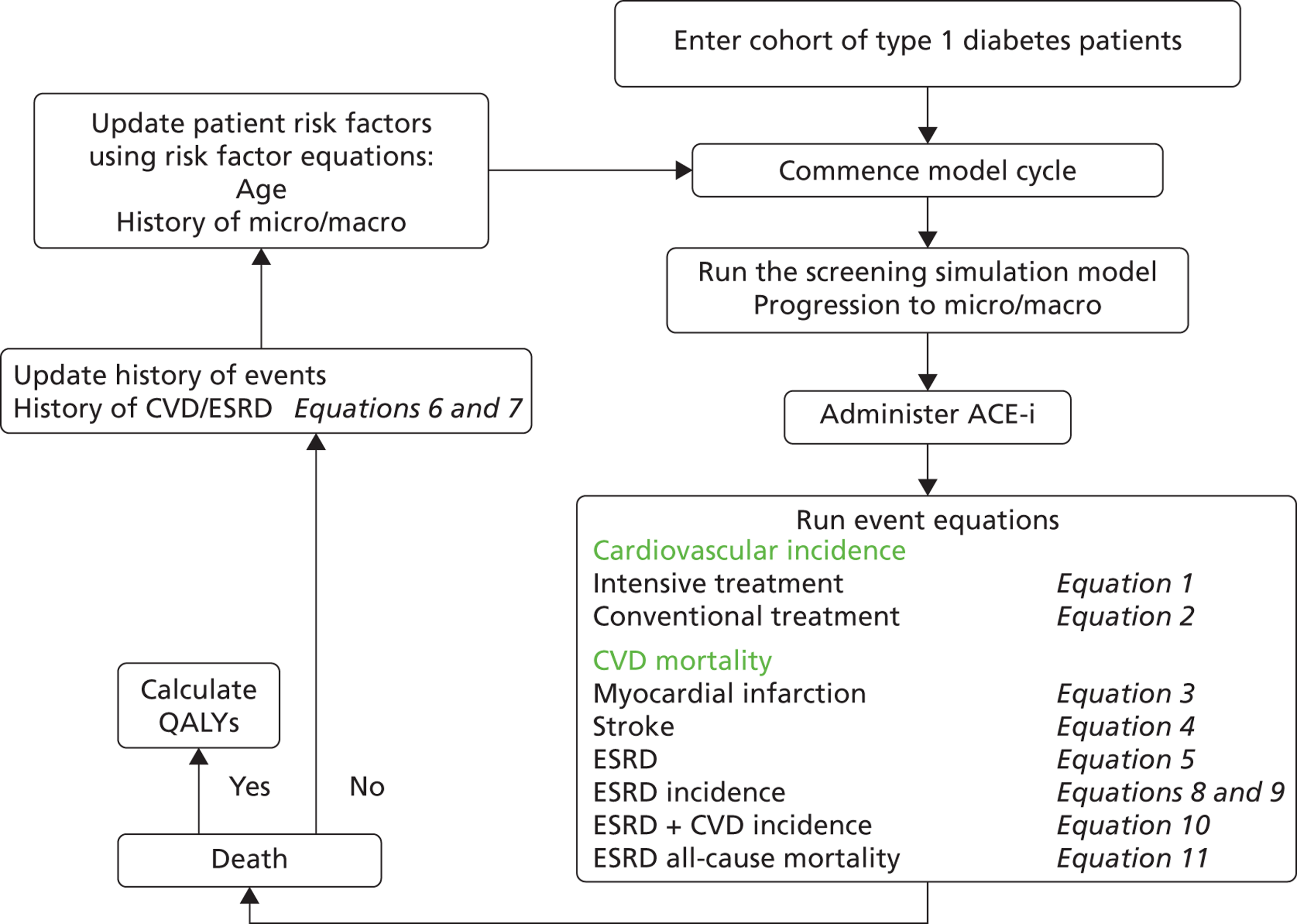
Data sources
The data sources used in the model construction are summarised in Table 19 and the functional form and coefficients used in each numbered equation are described in Table 20. Two equations for CVD-related death are used to capture both the acute and long-term impact on mortality of this disease. The key studies used to construct this model report time to first event and, therefore, the model focuses on these states (i.e. there are no equations to estimate second or subsequent events). 45,167
Publication | Data source | Section of the model | Number of patients |
---|---|---|---|
Oke et al. 2010165 | ORPS | Progression to kidney disease | 483 type 1 patients |
Nathan et al. 200545 | DCCT | Incidence of CVD event | 1441 type 1 patients |
Hayes et al. 2011166 | Western Australia | Mortality post CVD event, and history of CVD event | 12,792 (603) type 1 patients |
Finne et al. 2005167 | Finnish Diabetes Register | Incidence of ESRD | 20,005 |
Zoccali et al. 2002168 | Italian | ESRD patients’ progression to CVD, mortality or all-cause death | 228 ESRD patients (30 type 1) |
UKPDS 6868 | UKPDS | Hazard ratios for age and sex | All type 2 patients |
Kelly et al. 2011169 | Swedish Diabetes Registry | Hazard ratios for micro- and macroalbuminuric diabetes patients progressing to ESRD | |
Lung et al. 2011170 | Meta-analysis | Utility values | Type 1 and type 2 |
Functional form, data source, parameters, coefficients | Equation 1 | Equation 2 | Equation 3 | Equation 4 | Equation 5 | Equation 6 | Equation 7 | Equation 8 | Equation 9 | Equation 10 | Equation 11 |
---|---|---|---|---|---|---|---|---|---|---|---|
Weibull, DCCT (int.) | Weibull, DCCT (conv.) | Logistic, WA (MI) | Logistic, WA (stroke) | Logistic, WA (ESRD) | Gompertz, WA (male) | Gompertz, WA (female) | Gompertz, Finland, ESRD (male) | Gompertz, Finland, ESRD (female) | Gompertz, Italy, CVD | Gompertz, Italy, all-cause | |
γ/ρ (shape parameter of model) | 1.73 (0.51) | 2.22 (0.38) | 0.06 (0.003) | 0.05 (0.003) | 0.05 (0.01) | 0.01 (0.01) | 0.06 (0.01) | 0.02 (0.01) | |||
Constant | −8.87 (1.48) | −9.32 (1.13) | −5.20 (0.23) | −6.62 (0.3) | −7.70 (1.13) | −6.74 (0.22) | −5.57 (0.30) | −6.23 (0.19) | −5.86 (0.19) | −6.67 (0.33) | −5.61 (0.29) |
Microalbuminuria | 1.08 (0.24) | 1.08 (0.24) | |||||||||
Macroalbuminuria | 0.94 (0.33) | 0.948 (0.33) | |||||||||
Age | 0.08a | 0.08a | 0.03 (0.01) | 0.08 (0.01) | 0.09 (0.02) | 1.21 (0.20) | 1.21 (0.20) | 1.02 (0.01) | 1.04 (0.15) | ||
Male | 0.28a | 0.28a | −0.35 (0.09) | 0.12 (0.08) | 2.24 (0.23) | ||||||
Female | −0.45a | −0.45a | |||||||||
Diabetes | 2.53 (0.81) | 0.35 (0.10) | 1.64 (0.27) | 2.24 (0.26) | |||||||
Stroke at age ≤ 50 years | 0.56 (0.26) | ||||||||||
Stroke at age > 50 years | 0.16 (0.07) | ||||||||||
ESRD at age ≤ 50 years | 1.71 (0.33) | ||||||||||
50 < age ≤ 65 years | 1.33 (0.22) | 0.59 (0.30) | |||||||||
65 < age ≤ 75 years | 0.88 (0.23) | 0.69 (0.28) | |||||||||
Age > 75 years | 1.18 (0.30) | 1.12 (0.34) | |||||||||
CVD event | 0.58 (0.05) | 0.51 (0.06) |
Progression to kidney disease
The progression of ACR from normoalbuminuria to microalbuminuria, and the diagnostic error in testing for microalbuminuria, was modelled using the simulation model for type 1 diabetes reported in Chapter 3. Patients with positive tests were assumed to be treated with ACEi to slow the progression of kidney disease, with further blood pressure lowering if not controlled. The treatment effect of ACEi was modelled as an absolute risk reduction in the progression of kidney disease as described in the systematic review reported in Chapter 2.
Cardiovascular disease incidence and related mortality
A key outcome of the model is the occurrence of the first cardiovascular event (stroke or myocardial infarction). We used information from the DCCT to estimate the incidence of CVD by degree of renal function. The DCCT was a clinical study conducted from 1983 to 1993 to compare the effects of standard blood glucose control versus intensive control on the complications of diabetes. 45 The DCCT randomised 1441 type 1 diabetes patients, who were aged 13–39 years and had no history of CVD or hypertension at entry into conventional or intensive blood glucose treatment. Intensive control consisted of maintaining HbA1c levels as close as possible to a normal level of ≤ 6%. 45 Patients were followed up for a mean of 6.5 years with annual, central laboratory measurements of fasting lipid levels, serum creatinine values, HbA1c (quarterly measurements) and other risk factors of CVD. In total, 90% of the patients from the DCCT were followed up in a cohort study known as the EDIC to assess the incidence and predictors of CVD events (including heart attack, stroke, interventional procedures) and diabetic complications related to the eye, kidney and nerves. 45 We obtained survival estimates from the DCCT for patients who had a first occurrence of non-fatal myocardial infarction, stroke or death from CVD with microalbuminuria, macroalbuminuria and kidney disease as time-dependent covariates, using data in published reports. 45
Estimates of mortality following the occurrence of CVD events were based on a recent study involving linked administrative data on people with diabetes over the age of 35 from Western Australia, which were collected between 1990 and 1999 to estimate equations forecasting mortality. 166 The observational cohort consisted of 12,792 patients (630 of whom had type 1 diabetes) with one of the following complications of diabetes: myocardial infarction, stroke, heart failure, amputation or renal failure. The study reported a logistic regression to estimate mortality within the first month post event and a Gompertz proportional hazards model to estimate mortality due to CVD after 1 month. Type 1 diabetes was identified through diagnostic codes indicating insulin-dependent diabetes on one or more hospital admissions and where there was no evidence of these patients ever having used oral antidiabetic medications.
End-stage kidney disease
To estimate the incidence of end-stage kidney disease in our model, we used data from a cohort study that comprised 20,005 type 1 diabetes patients in Finland (data from 1965 to 1999). 167 All patients were diagnosed when < 30 years of age and were identified from the Finnish Diabetes Register; the maximum follow-up length was 37 years (median 16.7 years). Cumulative incidence estimates among male and female patients with type 1 diabetes according to age at diagnosis of diabetes were reported.
Cardiovascular events and all-cause death in patients with end-stage kidney disease were estimated from a study that reported survival curves for the duration of the study (53 months). 168 The study was based on a cohort of 228 haemodialysis patients (15% of whom were diabetes patients) who had been undergoing regular dialysis treatment for at least 6 months (median duration 43 months).
Mortality from other causes
The final transition in the model is death from non-CVD causes. Owing to the lack of information from a type 1 diabetes population, we derived information about these probabilities from age- and sex-specific mortality rates from all other causes from cause-specific US life tables for the general population. 171 Non-CVD mortality rates for 5-year age intervals were estimated as the difference between all-cause mortality and the reported CVD cause-specific mortality.
Quality of life
Utility values associated with the major health states were derived from a meta-analysis of preference-based quality-of-life [quality-adjusted life-year (QALY)] measures for people with diabetes. 170 This study was a systematic review of studies that reported QALY measures elicited from people with diabetes, including those with a history of complications. Meta-analysis was used to obtain a mean utility value for diabetes patients overall, and for patients with no complications, stroke, myocardial infarction, ulcer, amputation and end-stage kidney disease Appendix 6.
Translation of reported results
When published equations to estimate transition rates were not available (e.g. equations describing the transition to CVD and kidney disease), we obtained data about the underlying hazard by estimating survival times from published graphs. This involved using a program called Dagra 2.05 (Blue Leaf Software, Hamilton, New Zealand) to trace out the reported survival curves and export them as time-to-event data. 172 Using this approach we were able to replicate the cumulative hazard of a CVD event for both the conventional and the intensive treatment groups in the DCCT. 45 This involved fitting a parametric proportional hazards model. Tests were consistent with intensive therapy having a proportional effect on the hazard, reducing the risk of a CVD event when compared with the conventional treatment group.
A Weibull proportional hazards regression model (equations 1 and 2) was used to build the simulation model for type 1 diabetes, as this had previously been shown to fit the data well for people with type 2 diabetes. Coefficients used in the Weibull model included the following: the hazard ratios of microalbuminuria and albuminuria based on reported estimates involving a Cox model. 45 As parameters for the impact of age and sex are not reported in the literature, we used the parameters estimated from the UKPDS 68. 68 Patients were assumed to have a 70% probability of having a myocardial infarction and a 30% probability of having a stroke when patients had a cardiovascular event, based on two large diabetes trials. 68,173
For myocardial infarction and stroke, we used Hayes and colleagues’ logistic regression (equations 3–5) to estimate the higher risk of mortality 1 month post event and their Gompertz proportional hazards regression model (equations 6 and 7) to estimate the risk of mortality for surviving patients. 166 A Gompertz parametric form produces an exponential rise in death rates in older patients and is widely used to model human survival. 174
Proportional hazard ratios for age and type 1 diabetes and age and gender were used in the myocardial infarction and stroke logistic regression model, respectively. A sex-specific Gompertz proportional hazards model was used to represent patients with history of a CVD event and estimate the probability of survival post event.
Patients were at risk of progressing to the end-stage renal disease (ESRD) state in the model only if they had micro- or macroalbuminuria. We estimated a Gompertz proportional hazards model (equations 8 and 9) to determine the incidence of patients progressing to ESRD from published age- and sex-specific cumulative incidence graphs,167 using Dagra to estimate the data. These techniques were also applied to the reported Kaplan–Meier survival curves for cardiovascular events and all-cause death for type 1 patients with ESRD to determine rates of progress to a CVD event or death, respectively (equations 10 and 11). 168
Model testing and validation
All estimated proportional hazards models were tested for accuracy by comparing the estimated survival curves with the published survival curves, ensuring that the model provided the best fit. We carried out a validation exercise comparing simulation estimates of life expectancy among type 1 diabetes patients with reported all-cause mortality rates among a general non-diabetic population from an analysis of the UK General Practice Research Database (GPRD). 175
To carry out this validation exercise, life expectancy estimates were derived using a hypothetical group of 10,000 patients, who were simulated for 85 years, with a mean age at entry into the type 1 simulation model of 15 years.
Estimated mean life expectancy separated by sex and treatment group is shown in Figure 18. Men have a mean life expectancy of 66.0 (95% CI 60.7 to 71.3) years in the intensive treatment group and 55.4 (95% CI 47.8 to 63.0) years in the conventional treatment group. Life expectancy for women was estimated at 63.6 (95% CI 56.4 to 70.7) years for the conventional treatment arm and 72.2 (95% CI 68.7 to 75.6) years for the intensive treatment arm.
FIGURE 18.
Type 1 simulation outcomes for life expectancy separated by sex. Data extracted from Soedamah-Muthu 2006. 175
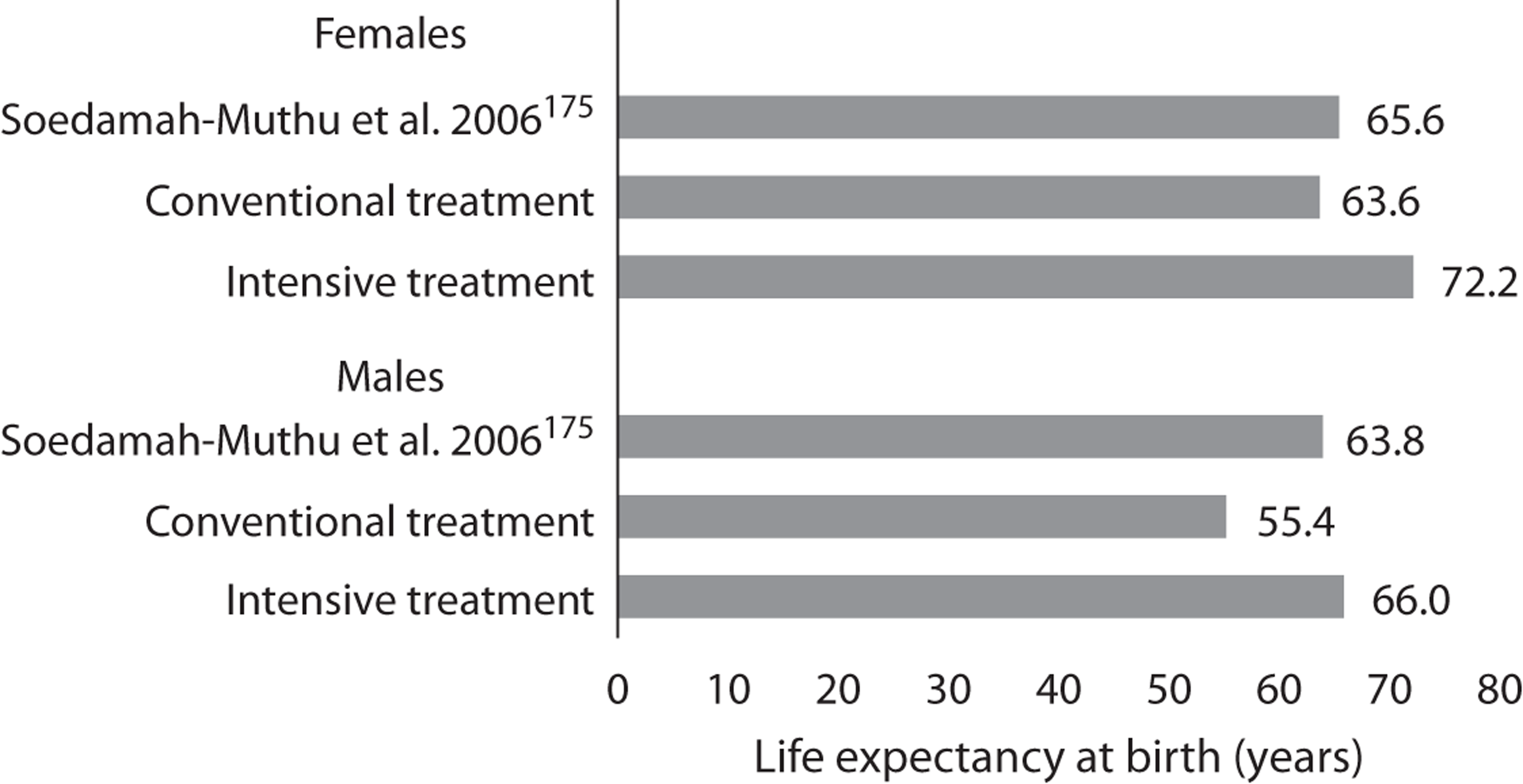
These results were compared to those of the UK type 1 diabetes GPRD study: life expectancies lay within one standard deviation of those obtained by the external study. 175 Women have a higher life expectancy across all treatment groups, which is reflected in the life expectancy of the general population. 176 Women with type 1 diabetes have a higher risk of mortality, and this is reflected in the literature. 175,177–181
To examine the validity of these model estimates, life expectancy was calculated using life table methods by adjusting the UK life table to take into account the higher relative risk of mortality among people with type 1 diabetes. The relative risks for this analysis were taken from a study that selected 7713 type 1 diabetes patients and compared them to 38,518 non-diabetic patients. 175 The reported overall hazard ratios of 3.3 for men and 4.5 for women were applied to UK life tables for 2007–9176 by multiplying the age-specific mortality rates of the general population by the appropriate hazard ratios at 5-yearly intervals. This gave figures for life expectancy at birth of 63.8 years for males and 65.6 years for females. Compared with the mean life expectancy of 77.7 years in the general population of the United Kingdom,176 our simulation model, and the external study,175 suggests a loss of approximately 14 years of life attributable to type 1 diabetes.
Uncertainty
There is uncertainty around the estimates of health outcomes and costs derived from any simulation model. Two forms of uncertainty are addressed within this modelling exercise: uncertainty in the individual patient predictions generated by the system of model equations and uncertainty in the estimated parameters of those equations. We addressed the former – the random variation between simulations – by carrying out large numbers of Monte Carlo replications until the estimates of the outcome of interest were stable. To model CIs around estimates we sampled from normal distributions using bootstrapping methods with different sets of regression coefficients around key parameters, based on the standard errors of coefficients reported in the literature. These analyses allow us to report CIs for the mean difference in QALYs and costs.
To examine the degree to which different elements of the model contribute to uncertainty, 1000 draws from normal distributions for the major parameters of the model were taken and used to estimate 95% CIs around life expectancy. In each draw, the mean value was used in the model and the variance calculated using reported measures of standard error. The same model simulations were repeatedly run with an increasing number of equations subject to parametric uncertainty.
Figure 19 shows the increased uncertainty in the life expectancy estimates in the form of 95% CIs as uncertainty for each parameter is included incrementally. This comprises, reading from left to right:
-
point estimates
-
CIs when just the CVD incidence parameters were assigned distributions
-
CIs when CVD and ESRD incidence parameters were adjusted
-
all parameters were assigned distributions around their point estimates.
FIGURE 19.
Uncertainty in the life expectancy estimates (95% CIs) when parameters were assigned distributions incrementally (see text for a detailed explanation).
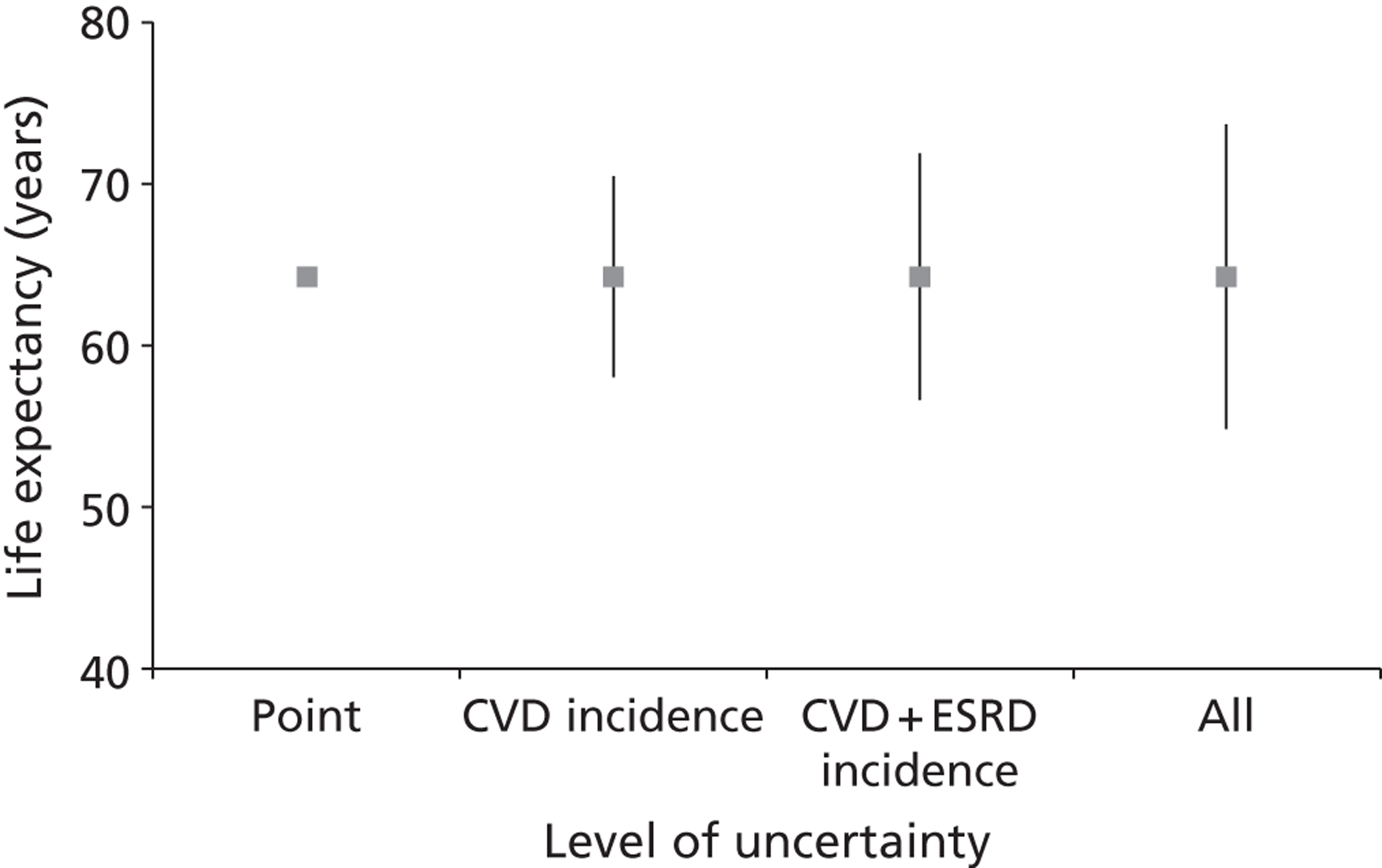
This was calculated for the life expectancy for the whole population, which included both conventional and intensive treatment arms. Figure 18 shows that the CVD incidence parameters contributed the most uncertainty in the current version of the model.
Limitations
There are a number of limitations that require acknowledgement in our model. Multiple CVD events are not included in the model, as the source equation from the DCCT reports only the first occurrence of a CVD event. Second, although the DCCT/EDIC study has a comparatively long follow-up of around 17 years, this is a relatively short period when compared with a patient’s overall lifetime. Hence, there is considerable uncertainty surrounding the lifetime extrapolation relating to the data. This is reflected in the reported uncertainty surrounding the life expectancy estimates when allowing for parameter uncertainty. When accounting for uncertainty in our model, we assumed independence between: (1) variables in the same risk equation; and (2) different risk equations. If the covariance between parameters is significant, it could impact on the level of uncertainty.
Our model also does not take into account recently published studies of risk in type 1 diabetes patients, such as two recently published equations for CVD risk from Sweden182 and the United States. 183 Furthermore, the transition probabilities for ESRD patients progressing to CVD and death have been estimated using non-type-1 diabetes populations168 because of the lack of published studies in the literature.
A simulation model for type 2 diabetes
The simulation model for type 2 diabetes was based on the UKPDS outcomes model, which has been widely used for economic evaluations of a wide variety of different interventions. The standard model required adaption, as it does not include parameters relating to markers of renal progression such as albuminuria and eGFR.
Model structure
The UKPDS outcomes model is a computer simulation model for forecasting quality-adjusted life expectancy and other outcomes for people with type 2 diabetes. It was developed using patient-level data from the UKPDS and was primarily intended as a tool to assist in the evaluation of new interventions to manage patients with type 2 diabetes. 68
The outcomes model involves probabilistic discrete-time computer simulation and is based on an integrated system of parametric proportional hazards risk equations. These were estimated over a median period of 11 years from diagnosis of diabetes using individual patient data. 68 The model includes both macrovascular (e.g. myocardial infarction, other ischaemic heart disease, congestive heart failure, stroke) and selected microvascular (e.g. blindness) complications. It can be used to assess the impact of the disease on morbidity and mortality, and can also be used to estimate health-care costs associated with the disease. The existing risk factors and complications covered by the model are summarised in Figure 20. These equations are used to estimate the probability of occurrence of different complications given risk factors such as a patient’s age, sex, duration of diabetes, systolic blood pressure, HbA1c, lipid levels and smoking status. The relative risk for each of these factors by type of complication is also reported in Figure 21.
FIGURE 20.
Summary of model equations for the type 2 diabetes simulation model (based on the UKPDS outcomes model)68 showing event-related dependencies and hazard-to-odds ratio for each risk factor.
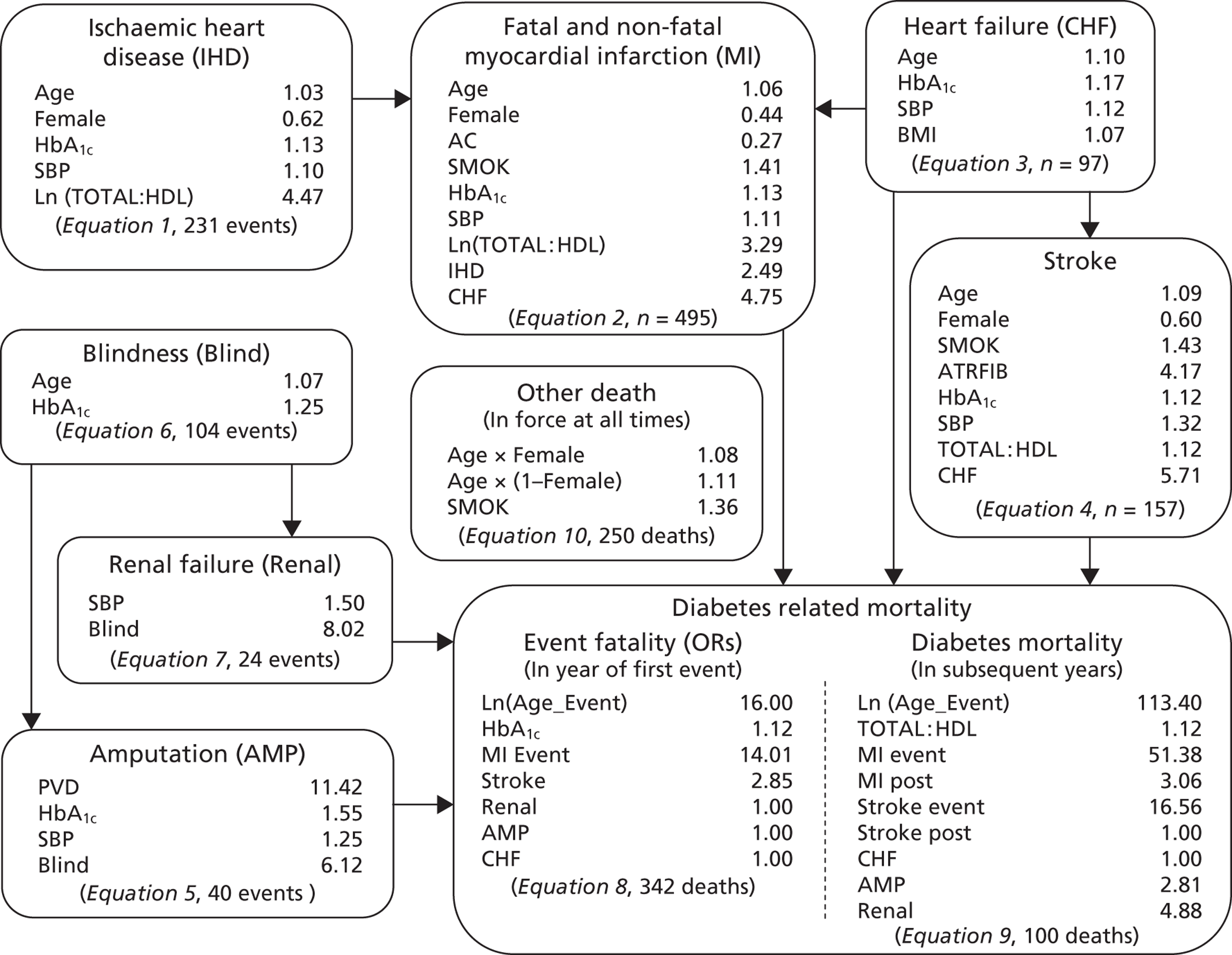
Simulation algorithm
Figure 21 comprises an algorithm that illustrates the sequence of modelling events for the existing model. In each cycle, the probability of experiencing each of the diabetes-related complications and death is calculated using the estimated risk equations. At the beginning of each cycle, the order of the equations is randomised to account for competing risks (i.e. if a patient dies in any cycle of the model, he or she cannot experience an event defined by equations lower in the order). The probability is compared with a random number ranging from zero to one from a uniform distribution to determine whether an event occurs. If the model predicts that a death has occurred, the years lived and quality-adjusted years lived are calculated. If the patient is predicted to have survived that cycle, the risk factor equations are used to update the current risk factor values (e.g. systolic blood pressure) and these are carried forward to the next cycle of the model along with the updated event history.
Although the model can estimate the risk over time of each complication and death in this study, it will primarily be used to estimate life expectancy and QALYs.
Reduced rates of complications may reduce health-care costs, producing savings that may offset some of the costs of improving treatment. The sources of costs and utility values are discussed in Chapter 6. To capture these benefits, the simulation model was also used to estimate lifetime health-care costs of diabetes-related complications. Non-diabetic morbidities are not specifically included in the simulation model, although non-diabetic mortality is included.
The methods described above to deal with uncertainty in the type 1 simulation model were also used to address the uncertainty in the type 2 model. The aim, as before, was to facilitate reporting of the CIs for the mean difference in QALYs and costs.
How the model has been adapted for this project
The population parameters of the UKPDS outcomes model were adjusted to more accurately reflect the type 2 diabetes population in the UK, including ethnic distribution. The data used to carry out this adjustment were extracted from the UKPDS,184 including data that compared intensive glucose therapy to conventional dietary therapy among 4209 newly diagnosed type 2 patients. Patient characteristics used were mean age, proportion of males, body mass index, HbA1c, blood pressure and cholesterol, and ethnic distribution.
The simulation model reported in Chapter 445 was integrated into the UKPDS outcomes model for the purposes of this project. This involved integrating programming codes in Stata version 11 that classify patients according to whether they have either hypertension or kidney disease. Increased risk of kidney disease or risk of CVD and mortality was incorporated into the UKPDS outcomes model. 169,185 Patients detected as having kidney disease were assumed to be treated with ACEi and A2RB (see Chapter 2 for further discussion) and benefit from the mortality reductions was quantified for these treatments.
The utilities derived from a meta-analysis for diabetes patients with no complications, myocardial infarction, stroke and end-stage kidney disease were integrated into the model,170 whereas for all other health utilities (ischaemic heart disease, congestive heart failure, amputation and blindness) the original values as reported in the original UKPDS outcomes model were used. 68 Costs for the analysis of renal screening were also integrated into the model, and these will be reported in Chapter 6.
Chapter 6 Cost-effectiveness of monitoring renal function for type 1 and type 2 diabetes
This section presents the methods and results of a series of cost-effectiveness analyses that evaluate alternative screening programmes for renal function in people with diabetes. The models described in Chapter 5 were used to estimate both costs and effects. The simulation models outlined in Chapters 3 and 4 for the progression and screening of ACR levels in type 1 and type 2 diabetes patients were incorporated into the models presented in Chapter 5. The results for screening people with type 1 and type 2 diabetes are presented first, followed by interpretation and discussion of the results to facilitate decisions regarding the implementation of renal function monitoring programmes by the NHS.
Methods
Economic evaluation
Incremental cost-effectiveness analyses were carried out for each of the various screening strategies. The incremental net cost and net cost-effectiveness were calculated in relation to the comparator, and expressed as a ratio for each strategy. As the economic evaluation perspective was that of the health-care purchaser, only direct health service costs were included. Both costs and effects were discounted at 3.5% per year for the first 30 years and 3% subsequently, in line with current guidelines. 186
All outcomes are expressed as QALYs, as this measure captures both increases in life expectancy and improved quality of life resulting from prevention of complications, providing a composite outcomes measure of fatal and non-fatal events that permits comparisons among a wide range of interventions.
In the main analyses, we vary the screening interval between 1 and 10 years, initially using 5-year and then 1-year increments. Where possible, results are reported as mean values and standard deviations, or mean differences with CIs, and as ‘cost-to-clinical-effectiveness’ ratios. The effect of uncertainty surrounding costs and the utility values used in the study were examined using sensitivity analyses.
Model simulations
Using the models outlined in Chapter 5, simulations were undertaken at a patient level. The simulations include (as treatment effects) estimates of the proportion of true-positive/true-negative/false-positive/false-negative tests and the consequences of these outcomes in terms of additional benefit and cost of treatment. These models were used to examine the impact of various monitoring policies on the incidence of important health states (e.g. CVD). The results presented are the average of 1000 simulations, which stabilise the expectation of different individual Monte Carlo simulations.
Resource data and costs (type 1 and the United Kingdom Prospective Diabetes Study outcomes model)
The economic evaluation was carried out from the perspective of the health-care provider and, therefore, the following costs were included in the analysis: cost of monitoring; cost of further investigations; cost of treating diagnosed kidney disease in people with diabetes; and the cost of subsequent complications.
All costs included were obtained from published UK sources (individually referenced below) and the values and sources of cost data are described in the following sections. Costs are expressed in 2011 pounds sterling and data from previous years have been adjusted to 2011 value using the Hospital and Community Services pay and price index. 186
Table 21 summarises the main sources of information on therapy unit costs, implementation unit costs and immediate (and subsequent) costs of CVD complications. As described in previous chapters, a maximum of three urinary ACR tests are taken during each year of monitoring. To determine whether a patient with normoalbuminuria has progressed to microalbuminuria or macroalbuminuria, two positive tests are required before patients are confirmed to have progression of kidney disease requiring intensification of treatment. The cost of the ACR test is estimated at £2.16 according to the Chronic Kidney Disease Costing Report, published by the National Institute for Health and Care Excellence in 2008. 187 ACR tests are administered by the practice nurse, and we approximate each test to take 10 minutes of the nurse’s time. According to the Unit Costs for Health and Social Care 2010,188 a 10-minute clinic visit costs £13.50. Once a patient is confirmed to have progressed from normoalbuminuria to microalbuminuria, a GP is consulted to discuss what action to take and the administering of an ACEi, which we estimate to take around 5 minutes of a GP’s time (£15.41). 188
Item | Unit cost (£) | Source |
---|---|---|
Therapy costs | ||
ACEi | 27.74 (per year) | Prescription Cost Analysis England (2010)189 |
Angiotensin receptor blockers | 191.12 (per year) | |
Other drugs | ||
Implementation unit costs | ||
GP (clinic visit) | 15.41 (per visit) | Unit Costs of Health and Social Care (2010),188 Chronic Kidney Disease Costing Report (2008)187 |
Practice nurse (clinic visit) | 13.50 (per visit) | |
ACR test | 2.16 (per test) | |
Immediate (subsequent years) costs of selected complications | ||
Hospital costs | Clarke et al. (2002),190 adjusted for inflation using the Hospital and Community Services pay and price index | |
Fatal MI | 1832 (1497–2221) | |
Fatal stroke | 5382 (3079–8461) | |
Non-fatal MI | 6475 (5696–7513) | |
Non-fatal stroke | 3766 (2544–5209) | |
No complications | 243 (230–257) | Clarke et al. (2002),190 adjusted for inflation using the Hospital and Community Services pay and price index |
ESRD costs | ||
Dialysis | 29,800 | Chronic Kidney Disease Costing Report (2008)187 and Klebe et al. (2007)191 |
First year costs | 11,479.11 |
If patients are confirmed to require treatment intensification through use of the ACR test, they are recommended to start an ACEi, or A2RB if they cannot tolerate ACEi. Pursuant to Prescription Cost Analysis England 2010,189 ACEi and A2RB cost £27.74 and £191.12 per year, respectively.
Furthermore, UKPDS 65 provides estimates of inpatient and outpatient costs for complications related to type 2 diabetes in the UK for fatal/non-fatal myocardial infarction, fatal/non-fatal stroke, no complications and a history of cardiovascular events. 190 These were included in the model to estimate potential cost offsets from lower rates of CVD.
End-stage kidney disease costs were divided into two parts. The National Costing Report estimated a mean annual cost of £29,800 per patient for haemodialysis, which is the main mode of renal replacement therapy in the UK. 192 A previous study, using UK guidelines, estimated the additional costs associated with diagnosis to be £11,479,191 which takes into account the cost of initial investigations, total referral cost and follow-up. For patients who move to end-stage kidney disease, the model includes a cost of around £40,000 in the first year (i.e. combining both diagnosis and dialysis costs), and £29,800 for every subsequent year they survive in that state.
Utility values
The economic evaluation for screening people with either type 1 or type 2 diabetes uses QALYs to adjust length of life for quality of life. A QALY value of 1 is equivalent to being in full health and 0 is equivalent to death. The values used are based on a recent meta-analysis. 170 Patients without complications were assumed to have a QALY of 0.81, reflecting that people with diabetes are on average in less than full health. In the type 1 simulation model, which uses a composite cardiovascular event, we assumed two-thirds had a myocardial infarction (reducing their utility to 0.75) and one-third of patients had a stroke (reducing their utility to 0.59) over their remaining lifetime. ESRD patients are assumed to have a utility of 0.48. We also incorporated these utility values into the UKPDS outcomes model, and used the original utility values for all other diabetes complications (Table 22).
Diabetes complication | Health utility value | Source |
---|---|---|
No complications | 0.81 | Lung et al. 2011170 |
Myocardial infarction | 0.75 | Lung et al. 2011170 |
Stroke | 0.59 | Lung et al. 2011170 |
ESRD | 0.48 | Lung et al. 2011170 |
Ischaemic heart disease | 0.80 | UKPDS 6868 |
Congestive heart failure | 0.70 | UKPDS 6868 |
Blindness | 0.53 | UKPDS 6868 |
Amputation | 0.56 | UKPDS 6868 |
Model baseline parameters
For the type 1 diabetes evaluation, a cohort of 10,000 patients was progressed through the type 1 simulation model (see Chapter 5). Screening for albuminuria commenced when patients were aged ≥ 12 years, in keeping with UK guidelines. The frequency of the screening was varied from annual to 10-yearly using the model described in Chapter 3.
A similar evaluation was undertaken for screening in patients with type 2 diabetes using the adapted version of the UKPDS outcomes model described in Chapter 5. For these simulations, the mean age of patients was assumed to be 62 years and the baseline characteristics were based on the patients in the post-study monitoring phase of UKPDS. Following UK guidelines, screening occurred annually beginning from time of diagnosis for type 2 diabetes patients. Again, the incremental cost-effectiveness ratios (ICERs) are calculated for different screening intervals from annual to 10-yearly using the model described in Chapter 4.
Analysis
Results are reported as mean values and standard deviations, or mean differences with CIs, and as cost-to-clinical-effectiveness ratios. To provide a visual representation of the results, the costs and health outcomes were mapped onto the cost-effectiveness plane and reported as acceptability curves. 193 The effect of uncertainty surrounding some aspects of cost and the utility values used in the study were examined using sensitivity analyses.
A series of sensitivity analyses were carried out to examine whether the results in the main analysis were robust to a series of assumptions:
-
the effect of costs: higher ACR test cost
-
the effect of changing progression values of ACR in the simulation models outlined in Chapters 3 and 4 to fixed values at the upper and lower end of the reported 95% CIs
-
the impact of assumptions about glycaemic control: estimating ICERs if all patients were simulated over a lifetime in two scenarios using each of the conventional and intensive treatment groups of the DCCT.
Results
Screening people with type 1 diabetes: results of the type 1 model
The characteristics of the patients in the type 1 cohort are summarised in Table 23. For the base-case scenario over a lifetime, around 40% of patients are estimated to have a cardiovascular event, and 25% progress to end-stage kidney disease. Life expectancy is estimated at 63.1 years, which is similar to the figure obtained in Soedamah-Muthu and colleagues’ study on type 1 diabetes patients in the UK. Females have a higher risk of mortality, and this is reflected in the literature. 175,177–181
Variable | Data |
---|---|
Mean age (years) | 27 ± 7 |
Males | 49% |
Body mass index (kg/m2) | 23.3 ± 2.7 |
Blood pressure (mmHg) | |
Systolic | 113 ± 12 |
Diastolic | 72 ± 9 |
Cholesterol (mg/dl) | |
Total | 177 ± 33 |
Low-density lipoprotein | 110 ± 29 |
High-density lipoprotein | 51 ± 12 |
The base-case scenario examined was annual screening of newly diagnosed type 1 diabetes patients ≥ 12 years old; the model determined total cost and QALYs gained over the duration of the simulation for the base case and for screening at greater intervals. Results of the simulation using the type 1 model are shown in Table 24. The columns of the table represent an incremental comparison of reducing the screening interval from 5 years progressively to 1 year. The costs associated with screening increase in proportion to its frequency, with the cost of annual screening being around five times greater than 5-yearly screening. The benefit in terms of incremental QALYs gained increases at a slower rate and hence the ICER increases from £3612 (£6586) to £9601 (£34,112), but all of these ratios are well within NICE’s QALY threshold of £30,000 per QALY.
Estimated outcomes | Screening interval | |||
---|---|---|---|---|
2-year vs. 1-year screening | 3-year vs. 2-year screening | 4-year vs. 3-year screening | 5-year vs. 4-year screening | |
Average increase in difference in cost (£) | 2431 (3567) | 1904 (3452) | 576 (1626) | 289 (330) |
Average increase in difference in QALYs | 0.26 (0.33) | 0.39 (0.342) | 0.15 (0.327) | 0.08 (0.67) |
Increase in cost per increase in QALY | 9601 (34,112) | 4941 (17,818) | 2522 (27,249) | 3612 (6586) |
The probabilistic uncertainties surrounding these estimates are shown in Figure 22, which illustrates a cost-effectiveness plane comparing biennial with annual screening intervals, and Figure 23 shows the corresponding cost-effectiveness acceptability curve. Figure 23 shows the probability that annual screening is cost-effective based on different thresholds for a QALY. The probability the intervention is cost-saving is around 25%, and it has around an 80% chance of being below the cost-effectiveness threshold of £30,000.
FIGURE 22.
Cost-effectiveness plane when comparing biennial screening intervals with annual screening intervals for patients with type 1 diabetes.
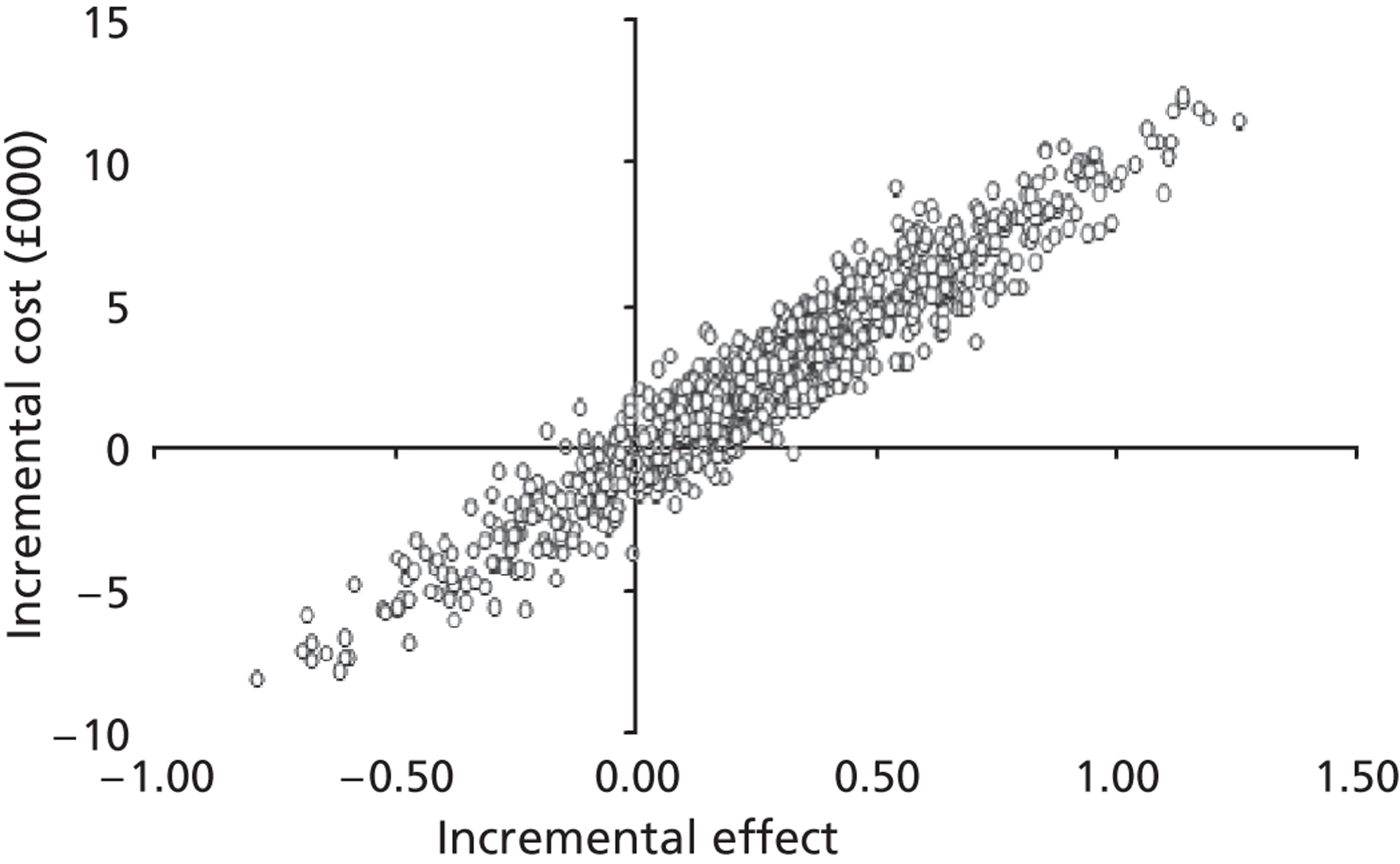
FIGURE 23.
Cost-effectiveness acceptability curve for biennial screening intervals vs. annual screening intervals for patients with type 1 diabetes.
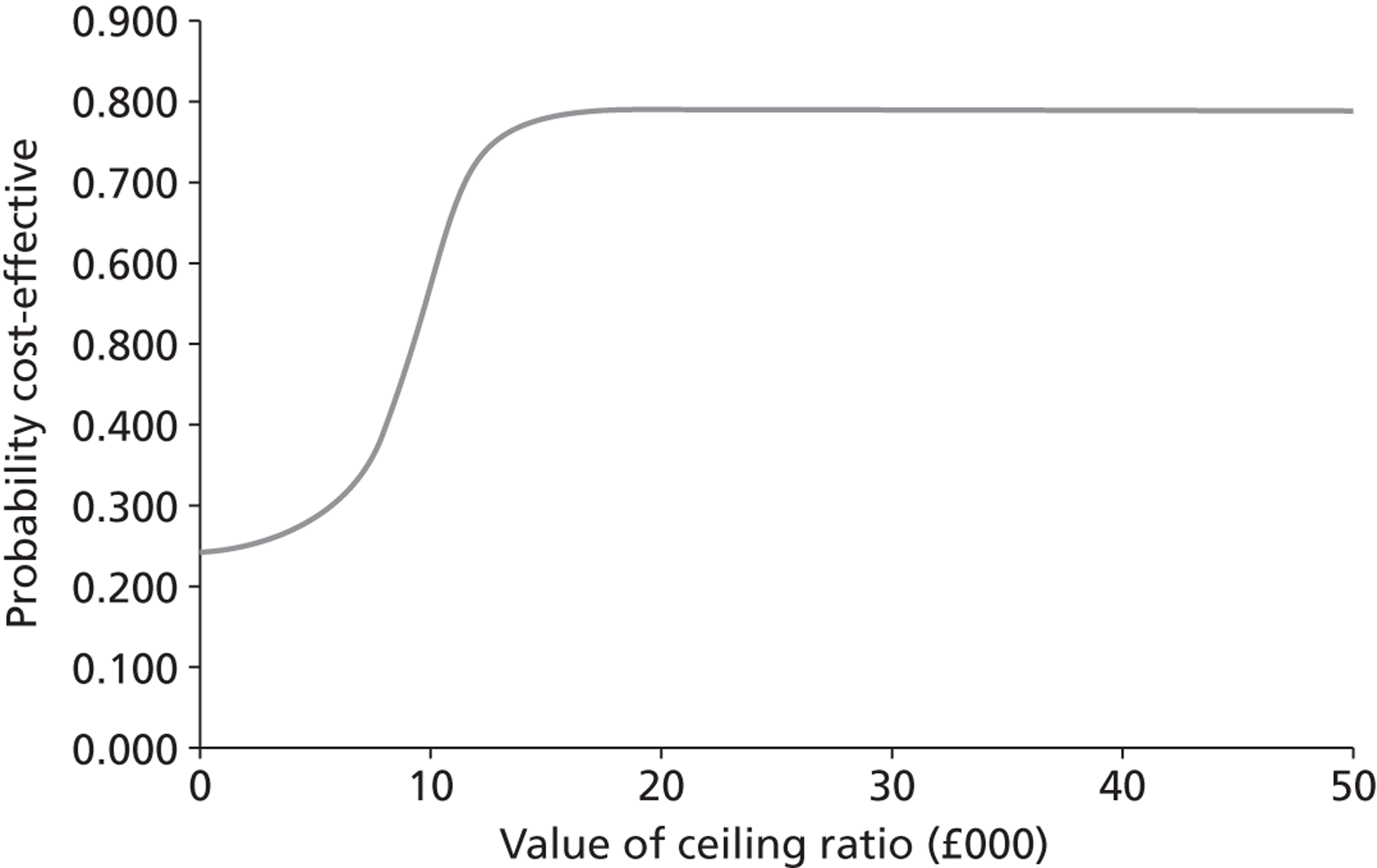
Screening people with type 2 diabetes: results of the type 2 model
Using this model, 1000 patients were simulated for 30 years; the mean age of patients newly diagnosed with type 2 diabetes was 62 years. Baseline characteristics are reported in Table 25. According to UK guidelines, screening occurred annually beginning from time of diagnosis for type 2 diabetes patients.
Variable | Data |
---|---|
Mean age (years) | 62 ± 8 |
Males | 58.5% |
Body mass index (kg/m2) | 29.4 ± 5.5 |
Blood pressure (mmHg) | |
Systolic | 137 ± 19 |
Diastolic | 77 ± 10 |
HbA1c | 8% |
Cholesterol (mg/dl) | |
Total | 198 ± 39 |
Low-density lipoprotein | 127 ± 34 |
High-density lipoprotein | 42 ± 12 |
Results of the cost-effectiveness analysis are shown in Table 26. The differences in costs and QALYs between biennial and annual screening were reported as £209 (standard deviation £309) and 0.42 (standard deviation 0.12), resulting in an overall ratio of £606 (standard deviation £1782), which makes the intervention highly cost-effective. When the screening interval is increased beyond 5 years, there is a minimal difference between QALYs, suggesting that increasing the screening interval further has little impact on QALYs and that, potentially, a ‘sojourn period’ is reached.
Estimated outcomes | Screening interval | |||
---|---|---|---|---|
2-year vs. 1-year screening | 3-year vs. 2-year screening | 4-year vs. 3-year screening | 5-year vs. 4-year screening | |
Average difference in cost (£) | 209 (309) | 112 (131) | 70 (129) | 71 (130) |
Average difference in QALYs | 0.42 (0.12) | 0.11 (0.26) | 0.24 (0.10) | 0.09 (0.11) |
Incremental cost per QALY | 606 (1782) | 493 (834) | 331 (275) | 763 (9031) |
Similarly, the differences in costs reflect the decreasing costs of ACR tests, but the increasing number of patients being treated for hypertension and kidney disease. Figures 24 and 25 represent the uncertainty surrounding the cost-effectiveness of renal screening in patients with type 2 diabetes. The cost-effectiveness plane is illustrated in Figure 24, showing a positive incremental benefit and incremental cost for all observations. This suggests that, although annual screening provides added benefits in terms of QALYs, it comes at a higher cost. Figure 25 shows a very high probability (97%) of annual screening being below the cost-effectiveness threshold of £30,000 per QALY. Based on these results, annual screening appears to be a cost-effective option.
FIGURE 24.
Cost-effectiveness plane when comparing biennial screening intervals with annual screening intervals for patients with type 2 diabetes.
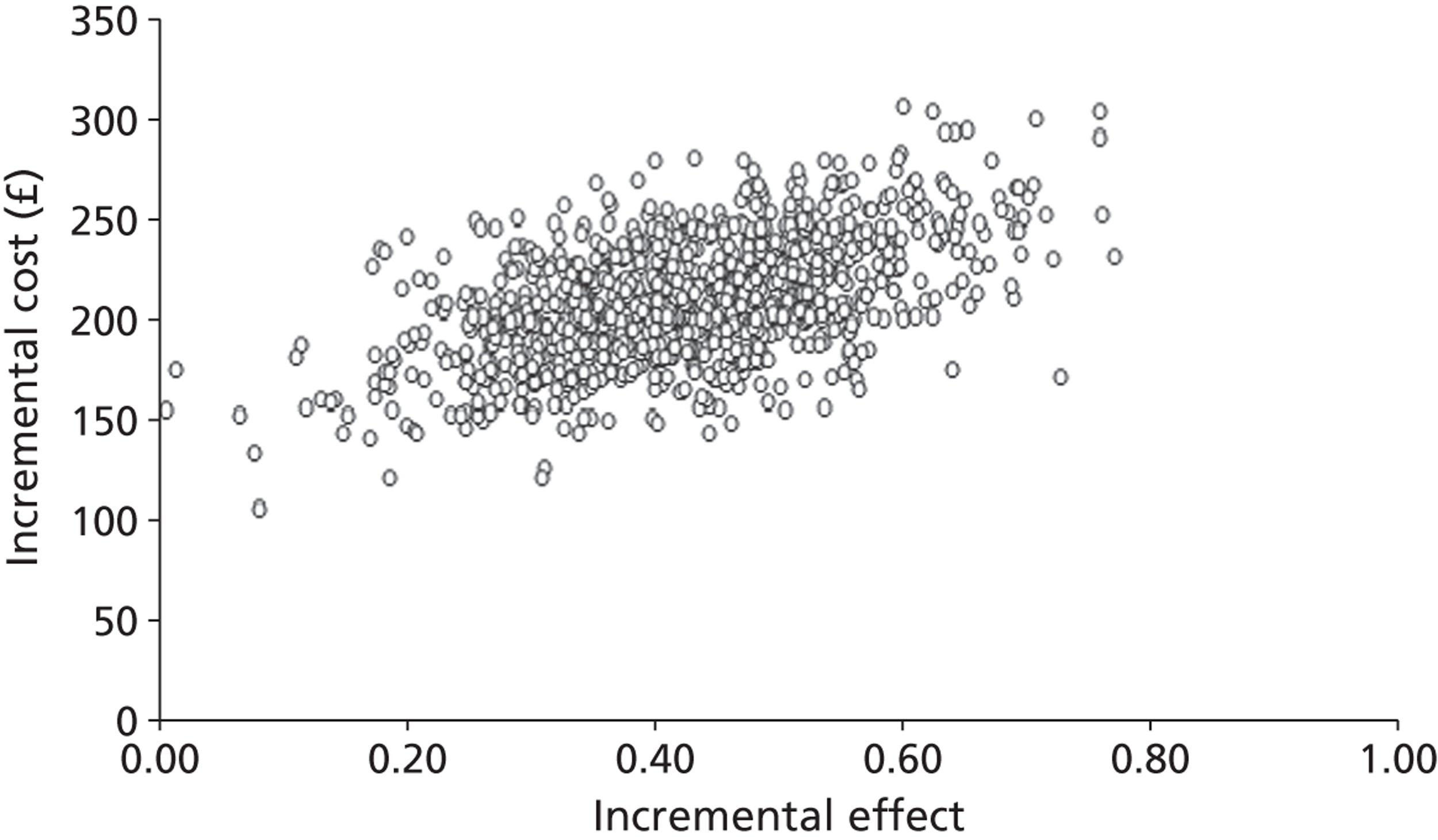
FIGURE 25.
Cost-effectiveness acceptability curve for biennial screening intervals vs. annual screening intervals for patients with type 2 diabetes.
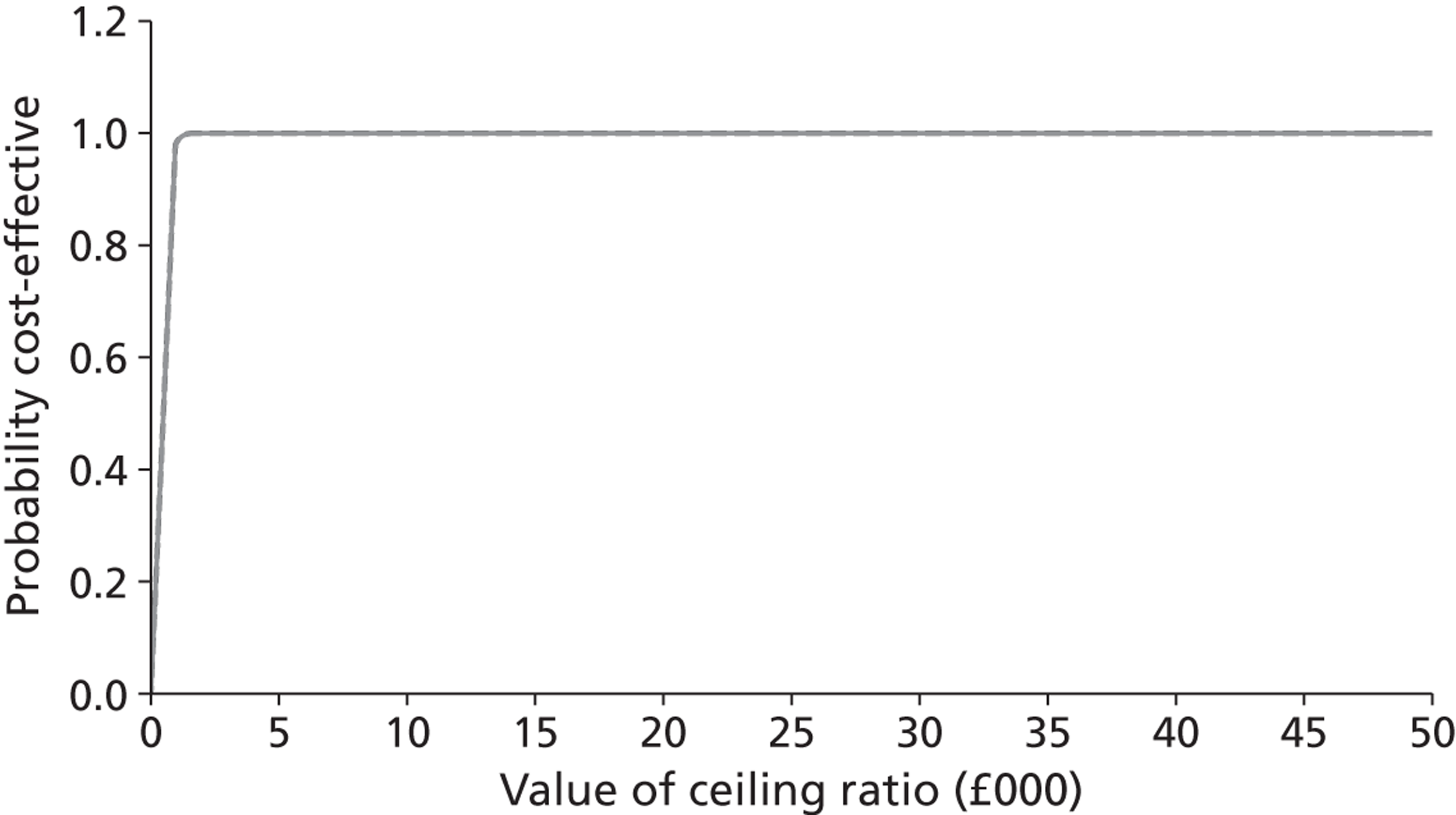
Sensitivity analysis results
The sensitivity analysis results for both type 1 and type 2 models are shown in Tables 27–29. We compared biennial screening intervals to annual screening intervals in all our analyses to help determine what factors drive our model. The results of these analyses are reported below.
Variable | Changes | Difference in cost (£) | Difference in QALYs | ICERs (£) |
---|---|---|---|---|
ACR test costs | Increased to £15 each | 3639 | 0.26 | 13,997 |
ACR progression | Lower 95% CI value | 816 | 0.02 | 40,801 |
Upper 95% CI value | 1077 | 0.04 | 26,945 | |
CVD (equations 1 and 2) | Conventional treatment only | 3193 | 0.22 | 14,517 |
Intensive treatment only | 1547 | 0.31 | 5079 | |
Utility | Lower 95% CI value | 2431 | 0.25 | 9839 |
Upper 95% CI value | 2431 | 0.26 | 9193 |
Variable | Changes | Difference in cost (£) | Difference in QALYs | ICERs (£) |
---|---|---|---|---|
ACR test costs | Increased to £15 each | 811 | 0.42 | 1932 |
ACR progression | Lower 95% CI value | 793 | 0.02 | 51,324 |
Upper 95% CI value | 1059 | 0.04 | 25,447 | |
Utility | Lower 95% CI value | 209 | 0.36 | 580 |
Upper 95% CI value | 209 | 0.55 | 380 |
Costs | Type 1 diabetes | Type 2 diabetes | ||||
---|---|---|---|---|---|---|
Screening interval | Screening interval | |||||
Annual | Biennial | Mean difference | Annual | Biennial | Mean difference | |
Screening costs (£) | 5121 | 2937 | 2184 | 1275 | 808 | 467 |
Treatment and hospitalisation costs (£) | 12,978 | 7787 | 5191 | 4225 | 4050 | 175 |
Total costs, undiscounted (£) | 18,099 | 10,724 | 7375 | 5499 | 4858 | 641 |
Total costs, 3.5% discount rate (£) | 9314 | 6883 | 2431 | 2867 | 2658 | 209 |
Increasing cost of albumin-to-creatinine ratio test
For the type 1 model, the difference in cost between annual and biennial screening intervals was calculated to be £3639, with no change in the difference in QALYs of 0.26, resulting in an increased ICER of £13,997 per QALY when compared with our initial analysis. Similarly, in the UKPDS model, differences in costs increased to £811, resulting in an increased ICER of £1932 per QALY.
Albumin-to-creatinine ratio progression
Changing the values for progression of ACR in the simulation models outlined in Chapters 3 and 4 had a large impact on the results for both diabetes models. Changing the ACR progression variables to their lower 95% CI value saw a small proportion of patients progress to requiring treatment and thus ESRD, resulting in a reduction in the difference in costs and QALYs. The ICER calculated of £40,801 lies outside NICE’s recommendation, suggesting that annual screening is not cost-effective. The UKPDS model shows a similar result of £51,324 per QALY, rendering this particular scenario cost-ineffective.
When adjusting the variables to the upper 95% CI limit, annual screening was very effective in identifying patients because of their quick progression to requiring treatment, and thus ESRD. Hence, the ICERs for both models were lower when using the higher CI limit than when using the lower CI limit: £26,945 and £25,447 per QALY for type 1 diabetes and type 2 diabetes patients, respectively.
Figures 26 and 27 show the results of modelling progression of the ACR over time from diagnosis. The modelling integrates the equations that estimate the true progression of renal function in the simulation models for type 1 and type 2 diabetes patients. The simulation uses estimates of the upper and lower 95% CI limits of coefficients for the observed mean rate of ACR progression to present two alternative scenarios for progression. The figures show that, for the upper 95% CI values, progression occurs very quickly and, therefore, annual screening would identify more patients with microalbuminuria than biennial screening. However, it might be too late to treat some of these patients before they develop end-stage kidney disease.
FIGURE 26.
Sensitivity analysis for rate of ACR progression based on running simulations for the 95% CI of the average rate of progression for type 1 diabetes.
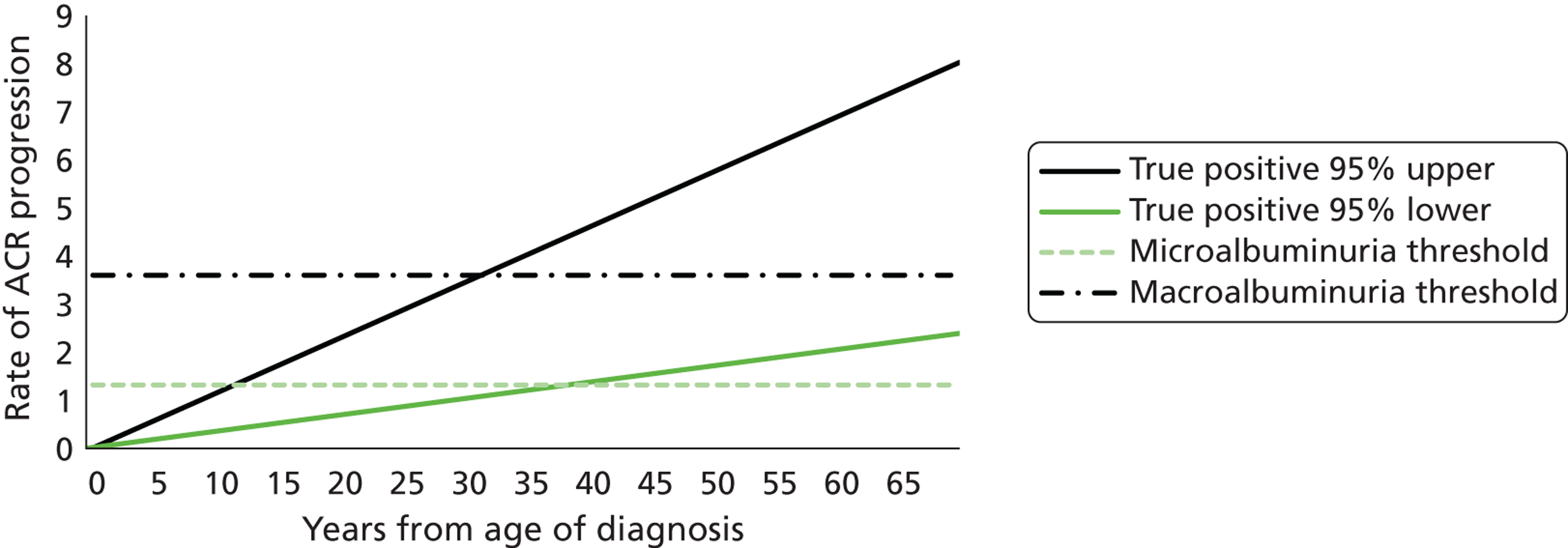
FIGURE 27.
Sensitivity analysis for rate of ACR progression based on running simulations for the 95% CI of the average rate of progression for type 2 diabetes.
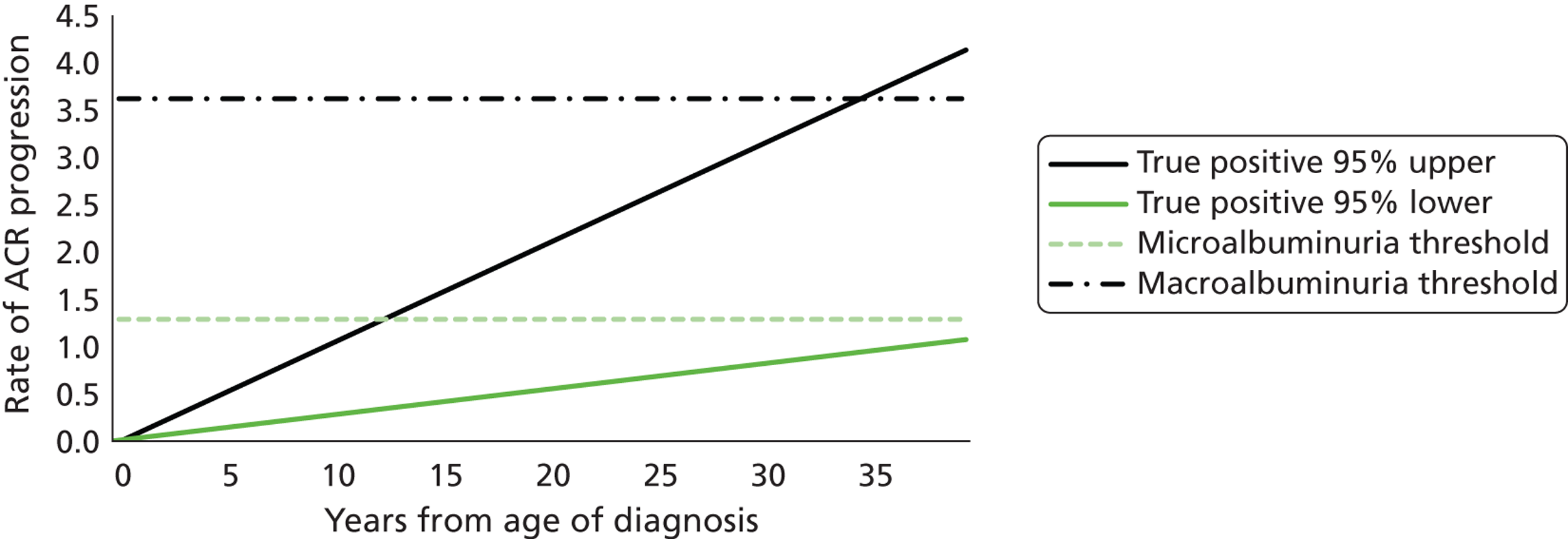
In comparison, modelling with mean ACR progression estimates based on the lower limit of the 95% CI shows that the progression of kidney disease is very slow, and thus neither annual nor biennial screening intervals would reliably identify the small number of patients progressing to microalbuminuria. Therefore, it is likely to be less cost-effective than the upper 95% CI limit scenario, and possibly cost-ineffective.
Adjusting cardiovascular equations (type 1 model only)
If all patients in the economic evaluation were assumed to have conventional treatment as stipulated by the DCCT, the ICER is calculated at £9711.71 per QALY. 45 However, if all patients were assumed to have intensive treatment, the ICER would be smaller, at £5079 per QALY. The latter scenario would see an increase in QALYs, and a decrease in cardiovascular events and thus costs.
Utility values
Adjusting utility values to the upper and lower 95% CI values for all diabetes events was shown to have little impact on the cost-effectiveness of screening, as the type 1 diabetes model and the UKPDS model found both scenarios to be very cost-effective.
Comparison of discounted and undiscounted costs
Table 29 reports the costs separately in two major categories: screening costs (i.e. tests and follow-up) and treatment and hospitalisations costs. Doubling the frequency of screening substantially increases the costs (e.g. from £2937 to £5121 in the case of type 1 diabetes). Screening and treatment costs are much higher for the type 1 diabetes patients because of the extended nature of the screening (i.e., for patients with type 1 diabetes, screening and treatment continue over their lifetime of around 50 years compared with roughly 15 years for the average person with type 2 diabetes).
Discussion and interpretation of the results
Main conclusion
Annual screening appears to be a cost-effective option for both type 1 and type 2 diabetes compared with biennial screening and other health-care interventions. For type 1 diabetes, screening produces benefit at a cost, but this cost is well below accepted thresholds used for other types of health care. For type 2 diabetes screening, the cost-effectiveness ratio is highly favourable. The data on which our analysis was based did not provide information about more frequent screening intervals than 1 year; however, the results did indicate that the benefit might be greater if the underlying rate of progression of ACR is higher than the average in the population.
Comparisons with previous literature
We have found renal screening for people with type 1 and type 2 diabetes to be cost-effective in a UK context with cost-effectiveness ratios that would compare favourably with many other funded health interventions. With regard to the benefits, it would appear that screening produces comparable or greater outcomes to that observed in a general population screening. For example, it has been previously estimated that biennial mammography for women aged 50 produces less than 1 additional month of survival and Pap smears less than 3 months. 194 Here, particularly with type 2 diabetes, the gains in QALYs range from a few months to over half a year.
The findings of this study are also consistent with two studies of the cost-effectiveness of blood pressure treatment in type 2 diabetes. A study based on an analysis alongside the UKPDS indicated that tight blood pressure control policies (including use of ACE inhibitors) produces several months’ increase in QALYs at a cost of only £300 per QALY. 195 Similarly, an analysis of the ADVANCE study showed treatment with a fixed combination of blood pressure therapies (again, including ACE inhibitors) produced significant increases in life expectancy and was cost-effective. 196
The higher cost-effectiveness ratio in screening type 1 diabetes patients is a result of a number of factors impacting on relative outcomes and costs of screening among these groups. First, when benefits are not discounted, the gains in QALYs of screening are similar in type 1 diabetes and type 2 diabetes, but the long duration between the commencement of screening and when the majority of patient experience cardiovascular events significantly reduces discounted QALYs. Another reason for the differences across these patients is the effect of treatment on ACR. In the case of type 2 diabetes, we have assumed an average 52% reduction in ACR (see Chapter 2), whereas in type 1 diabetes the effect is 32% (see also Chapter 2). The greater incremental effect in type 2 diabetes is a result of screening older patients who are at a higher risk during the entire screening period and the treatment being more effective in slowing progression to renal failure.
The average difference in lifetime screening costs is also much higher for type 1 patients, as, currently, the guidelines for screening recommend annual screening from diagnosis with either type of diabetes. Assuming that the age at diagnosis in type 1 diabetes is around 15 years, whereas in type 2 diabetes it is 62 years, patients with type 1 diabetes will have many more tests over their lifetime.
Further, these screening costs will be occurred at a constant rate, whereas any cost offsets associated with CVD or kidney disease are likely to occur after the age of 40 years. In contrast, type 2 diabetes patients are generally already at risk of complications at diagnosis and, therefore, the cost offsets will occur much sooner.
Clinical and research implications
While these simulations provide useful insights relating, in particular, to the additional benefits of universal treatment over screening, it is difficult to draw firm policy conclusions regarding the merits of their adoption in practice because of the limited number of studies reporting continued long-term ACE inhibitor use. For example, although the ADVANCE study randomly allocated all patients with ACE inhibitors in combination with another blood pressure medication, it involved screening patients’ toleration for this treatment through an active run-in. 61 Around 14% of patients withdrew during the 6-week active run-in period and the study had a mean follow-up duration of only 4.3 years. Therefore, it is necessary to collect more evidence surrounding the benefits and compliance associated with much longer periods of use before universal treatment can be considered as a practical policy option. This should be examined in future work.
A screening interval of 1 year appears optimal based on evidence of progression to renal failure of typical diabetic patients in the UK. The sensitivity analysis suggests that rates of progression would have to be substantially higher or lower than the average to have an important effect on our results. For example, within some ethnic groups (e.g. the south Asian population; see Table 5) rates of progression towards diabetic kidney disease may be substantially higher. 44,197 Further empirical work could help to establish whether more frequent monitoring of ACR might be justified, or whether alternative technologies might be required to identify those at risk. Similarly, if individuals at low risk of progression could be prospectively identified, then for future implementation of protocols for personalised care it might be possible to consider screening at less frequent intervals.
Strengths and limitations
Our type 1 diabetes model is subject to limitations, such as the exclusion of other major diabetes-related complications (peripheral vascular disease, blindness and neuropathy), because of the timeframe and available secondary data sources. Nevertheless, our model appears to make predictions for type 1 diabetes patients’ life expectancy that match another large type 1 diabetes mortality study in the UK. 175
The type 1 model includes important sources of major costs, including renal replacement therapy for patients who have either true-positive or false-negative test results but progress to end-stage kidney disease. Decrement of quality of life through occurrence of an ACE-related cough is not included, as the model assumes that these individuals are treated with A2RB. We have also, based on the results in Chapter 2, not added a disutility for treating patients with a false-positive test. We did not carry out a sensitivity analysis to explore the impact of the reduction in costs of A2RB with a move to generic prescribing, as this is a relatively small part of the overall costs and, in any case, would further increase the cost-effectiveness of annual screening. The impact of non-adherence to therapy is not included in this model.
The type 1 diabetes model is a synthesis of multiple data sources from a variety of different countries. Although the majority of sources are based on type 1 diabetes populations, some include type 2 diabetes patients and non-diabetes-related patients as well, which is a limitation when trying to inform NHS clinical practice for a UK-based screening programme. However, owing to the lack of published data specifically regarding type 1 diabetes populations, this was unavoidable. Further validation does, however, need to be conducted to reduce the uncertainty surrounding our estimates.
Chapter 7 Discussion and conclusions
Summary of findings
The results of the studies reported in this monograph provide support for the current policies of annual screening for the occurrence of albuminuria in people with diabetes, and subsequent treatment with ACEi or A2RB if identified. These treatments are effective in reducing progression of renal albumin excretion for those type 1 or type 2 diabetes patients identified as having albuminuria, and there is additional evidence of a benefit even for patients with type 2 diabetes and no albuminuria. The current screening tests available for detecting albuminuria, and the confirmatory testing strategy in use, lead to a substantial overdiagnosis of people at risk. However, the consequences, in terms of both the balance of harms and benefits and the costs, do not have a substantial impact. Therefore, less frequent testing intervals would save relatively small amounts of money at the expense of failing to identify small numbers of individuals at risk. For individuals with type 2 diabetes, screening to detect the presence of kidney disease (impaired eGFR) in the absence of albuminuria is also important, but the numbers affected are small.
This work provides an evidence base to underpin current policy for microalbuminuria screening in an area that has, until now, used consensus practice as a guide to action. To some extent the findings are surprising in that, of those identified as being at risk, more individuals are misclassified than actually at risk. However, although the costs and cost-effectiveness of this testing strategy are sensitive to the costs of testing, a more expensive test with similar performance to the current test, used at annual intervals, would still be cost-effective compared to use at 2-yearly intervals.
The sensitivity analyses suggest that, at current levels of annual screening costs, even if groups progressing more slowly towards renal failure were identified, the cost-effectiveness would remain within acceptable parameters.
Strengths and limitations
Our analyses are based on statistical modelling rather than trials directly comparing different monitoring strategies. The inefficiency and potential invalidity of randomised trials for diagnostic strategies have been discussed elsewhere198 and apply equally to screening and monitoring strategies, making modelling studies a frequent necessity. To overcome the issues associated with modelling, we have used external validation (such as Figures 10 and 14) and sensitivity analyses (such as Tables 26 and 27). Compared with the original protocol, these sensitivity analyses have been limited by the nature of the available data sets, but we have established robustness of the models over time and to key sources of uncertainty as far as possible (discussed in more detail below).
For our analyses in type 1 diabetes, we benefited from an observational cohort study, the ORPS, with up to 20 years of follow-up; however, this included only children under 16 years at diagnosis of diabetes, and hence to ages no higher than 35 years during follow-up. Our analyses for type 2 diabetes benefited from a middle-aged, adult population, with a variety of durations of diabetes at recruitment, but the CARDS data extended to relatively few years of follow-up, and was collected in a clinical trial setting rather than routine care. In both cases, we addressed these limitations by comparing projections from our models with observations from other large studies with a wide range of ages and durations of type 1 and type 2 diabetes. Figures 10 and 14 show our models performing well against these external data; there is generally greater variation among different studies than there is discrepancy between our models and the observed data. The interpretation of these figures is complicated by the use of different protocols for classifying microalbuminuria in different studies, which is likely to exaggerate the discrepancies between studies and the model predictions. In Chapter 5, further external validation is carried out when these models are extended to use long time horizons, to consider multiple morbidities and mortality risks, and to allow for interactions between different complications of diabetes, as recommended by the ADA Consensus Panel on computer modelling. 199 As further information from trials becomes available about the extent to which these assumptions are valid, the results of this analysis will need to be revisited.
We have closely followed the ADA guidelines for diabetes modelling whenever relevant. 199 In particular, we have described our model with transparency; we have carried out external validations of the models in Chapters 3 and 4 and based our cost-effectiveness modelling of type 2 diabetes on a model with previous external validation; we have used multiple simulation runs to reduce Monte Carlo uncertainty and to quantify statistical variability; we have taken into consideration the long time horizons, multiple organ systems and multiple therapies relevant to lifetime modelling of diabetes and their impact on quality and length of life; and we have stated the cost perspective of our analyses.
We have not addressed the health economics of eGFR measurement in this report. The low prevalence of impaired eGFR in our type 1 data set limits to the degree to which such analysis is possible, but, more importantly, it also shows that eGFR measurement has only a secondary role to urine albumin measurement in screening for impaired renal function in type 1 diabetes. Although we base this on a relatively young cohort with type 1 diabetes, it appears to be confirmed by follow-up of the DCCT/EDIC cohort, in which declining eGFR is seen only in those with macroalbuminuria. 150 In type 2 diabetes, we also find that declining eGFR is a feature of patients who already have microalbuminuria or worse (see Table 16).
We could not extend our modelling to look at testing intervals of < 1 year. Both our data sets collected data at annual intervals, and studies that collect markers of diabetic kidney disease more frequently are likely to have shorter follow-up. Owing to the low signal-to-noise ratio and the difficulty in distinguishing change from underlying variation in the measure of both eGFR and ACR with a short interval between measurements (see Chapters 3 and 4), we deemed that evaluating intervals shorter than 1 year would not be justified. Future work might be focused on identifying individuals at greater risk of progression and draw data from such groups to re-estimate parameters for rates of progression and interindividual variation.
Interpretation
The cost-effectiveness results reported in Chapter 6 can be viewed as quantifying overall impressions from the systematic review and analyses of cohort data. The simulation modelling (see Chapters 3 and 4) found that false-positive diagnoses of microalbuminuria are frequent and false-negative classification of normoalbuminuria comparatively rare. The former would become less frequent, and the latter more frequent, if biennial or triennial screening were adopted in place of annual screening. The cost-effectiveness models of Chapter 5 consider how these two types of error – resulting, respectively, in overtreatment or in missed or delayed opportunities to treat – might translate into financial costs for providers and health costs for patients. In type 1 diabetes, the benefit of ACEi and A2RB treatment is strongly evident only in patients who have microalbuminuria (see Chapter 2). Our cost-effectiveness model for type 1 diabetes reports estimates and surrounding uncertainty for annual screening that are well within accepted thresholds of cost-effectiveness, and comparable to similar procedures.
We have not, because data remain unclear, included any estimates of treatment at even earlier stages of renal impairment, for example with hyperfiltration or with levels of albumin excretion in the upper tertiles of normoalbuminuria. However, in type 2 diabetes, treatment with ACEi and A2RB appears to have renal benefit even in patients who do not yet have microalbuminuria and, therefore, the overtreatment resulting from false-positive diagnoses in annual screening results in benefits as well as costs. Screening for microalbuminuria in type 2 diabetes appears, therefore, highly cost-effective.
Similarly, the findings from the statistical modelling in Chapters 3 and 4 are consistent with the overall findings of the research. For example, the short-term variability of ACR and eGFR measurements is high compared with the average annual change (see Tables 9 and 14). As a result, many diagnoses of microalbuminuria or decreased eGFR are a result of measurement error rather than true change (see Tables 10, 15 and 17). This is consistent with the previous findings, for other chronic conditions, that annual screening leads to high rates of false-positive diagnoses. 134,135 Further, because of the extreme variability of ACR measurements (despite log transformation; illustrated by the 95th centiles in Figures 8 and 13), even the practice of confirming microalbuminuria across multiple tests does not fully prevent high rates of false-positive diagnoses. Our models predict that the prevalence of microalbuminuria does not increase indefinitely with age until almost the whole population has diabetic kidney disease, but ‘levels off’ with about 50% affected (see Figures 10 and 15).
Future research
This research has identified a number of issues into which further research is needed. These include areas in which we have been unable to identify research that addresses our research questions, new issues arising from our findings and issues relating to the translation of our findings into clinical practice.
The simulation models used in this work have been developed to enable further data to be added as they become available. The type 2 model, drawing on data from UKPDS, is already in the process of revision by the UKPDS group to take account of the data from long-term follow-up of the cohort. When the UKPDS model becomes available, it will allow our findings to be reviewed and, if necessary, revised.
The type 1 diabetes simulation model is constructed from a number of sources, and as further data become available from larger type 1 studies it can also be expanded, with new equations incorporated into a revised model. It therefore offers a new tool for a wide range of cost–benefit studies which have previously been carried out with models that draw heavily on data from studies of patients with type 2 diabetes.
The uncertainty around the estimates for cost-effectiveness are largely driven by the rate at which new microalbuminuria occurs. There are a number of potential ways in which these findings might be taken forward. Our estimates of cost-effectiveness may be improved by establishing cohorts of individuals at increased risk of developing microalbuminuria and diabetic kidney disease. For example, with increasing capacity to personalise protocols for individual screening, those identified at increased or low risk for progression could be screened more, or less, frequently. Another approach might be to establish whether proactive treatment of those identified at risk might lead to longer-term benefits. However, recent evidence suggests that rates of progression of diabetic kidney disease are falling. 200 In establishing cohorts, estimates of sample size may need to be adjusted to take account of these trends.
An alternative approach to screening for microalbuminuria may be to look for other markers that can be used to identify risk of diabetic kidney disease or to monitor the impact of treatment intended to reduce risk. Among the candidates for new markers is cystatin C, following studies that have shown accurate identification of individuals with diabetes who are undergoing decline in renal function. 201 Cystatin C has been suggested as a more proximal marker of renal damage than microalbuminuria. 202 Initial studies have suggested that cystatin C may identify deterioration in renal function more accurately than eGFR in patients with type 1 diabetes,203 but cost-effectiveness studies are needed to establish whether the improved detection is of sufficient clinical importance to change treatment and, in particular, whether it adds to management, based on occurrence of microalbuminuria. Other strategies that might facilitate an assessment of risk include the measurement of uric acid, tumour necrosis factor receptors, certain advanced glycation end products and chemokines. However, further research to establish the utility of these measures and potentially further trials would be required to change clinical practice; the horizon for such developments is some way into the future.
Attempts have been made to characterise groups at increased risk of developing microalbuminuria. For example, data from a large cohort study have been used to develop a prediction rule for progression to microalbuminuria in type 1 diabetes. 33 However, it is not clear from our data whether more frequent measurement of albuminuria would be helpful. Further research is required to establish whether some of those who have false-positive screening test results are unlikely to develop true microalbuminuria, or whether the increased variability that leads to false-positive test results is a precursor of established microalbuminuria and, therefore, treatment may be justified. This strategy is currently being tested in a randomised trial. 18
Another strategy that might be explored for managing cardiovascular risk in association with screening for microalbuminuria is to investigate whether the occurrence of microalbuminuria, or increasing risk of it developing, might justify other treatments in addition to ACEi/A2RB. Chapter 1 lists a number of possibilities. One further possibility is whether aspirin might have an effect. Aspirin was previously recommended for individuals with diabetes, as the condition was viewed as a cardiovascular risk equivalent. More recent research has thrown doubt on this assumption, and a large-scale trial is currently under way to identify benefit from aspirin in diabetes. 204 Subgroup analysis of this trial based on the occurrence of microalbuminuria, where data are available, might provide information to inform assumptions around the benefits of screening included in our models. It is possible, for example, that, for many individuals with diabetes, aspirin treatment may not be beneficial, but, among those at high risk and identified with microalbuminuria, there might be a benefit from adding insulin to treatment regimens.
Qualitative work with subsequent surveys, and, if appropriate, trials, may also be helpful in establishing whether patient knowledge of renal albumin status provides an additional motivational factor sufficient to increase adherence to medication, without causing an adverse impact on well-being.
Acknowledgements
In addition to the authors and members of the study group, we also wish to thank Neil Dalton for giving his permission for use of the ORPS data.
ORPS has received funding from Diabetes UK and NIHR Cambridge Comprehensive Biomedical Research Centre. CARDS was funded by Pfizer. AF receives funding from the NIHR Oxford Comprehensive Biomedical Research Consortium.
Chapter 2 is adapted from the version published in Kidney International [Hirst JA, Taylor KS, Stevens RJ, Blacklock CL, Roberts NW, Pugh CW, et al. The impact of renin–angiotensin–aldosterone system inhibitors on type 1 and type 2 diabetic patients with and without early diabetic nephropathy. Kidney Int 2012;81(7):674–83].
Chapter 5 is an adapted version of a paper submitted for publication.
AF receives funding from NIHR Oxford Biomedical Research Centre. He is also an NIHR senior investigator.
Contributions of authors
Andrew J Farmer (Professor and General Practitioner) led the programme of work, contributed to the design of all the studies, wrote the first draft of the first and last chapters, contributed to all the work packages and the drafting of all sections, and edited the final report.
Richard Stevens (Senior Research Fellow) contributed to the design and management of the study, and contributed to all work packages and the drafting of the report.
Jennifer Hirst (Research Assistant) carried out the systematic review work and wrote the chapter that reports its results, in addition to carrying out additional reviews and contributing extensively to the writing.
Tom Lung (Research Fellow) wrote the first draft of Chapters 5 and 6 and carried out the health economic modelling work.
Jason Oke (Research Assistant) carried out the interval modelling and contributed to drafts of the work.
Philip Clarke (Professor and Health Economist) led the health economic modelling studies.
Paul Glasziou (Professor of Clinical Epidemiology) contributed to the design of the programme of work, commenting on it as it progressed, and reviewed and commented on the final report.
Andrew Neil (Professor of Clinical Epidemiology) contributed to the study design and reviewed and commented on the final report.
David Dunger (Professor of Paediatrics) provided individual patient data and commented on the final report.
Helen M Colhoun (Professor of Public Health) provided individual patient data for modelling in type 2 diabetes and reviewed and commented on the final report.
Christopher Pugh (Professor of Renal Medicine) contributed to the design of the programme of work, commenting on it as it progressed, and reviewed and commented on the final report.
Germain Wong (Senior Research Fellow) contributed to the design, work and interpretation of health economic modelling.
Rafael Perera (University Lecturer in Medical Statistics) contributed to the design of the study and commented on the final report.
Brian Shine (Consultant Chemical Pathologist) contributed to the design of the programme of work, commenting on it as it progressed, and reviewed and commented on the final report.
Disclaimers
This report presents independent research funded by the National Institute for Health Research (NIHR). The views and opinions expressed by authors in this publication are those of the authors and do not necessarily reflect those of the NHS, the NIHR, NETSCC, the HTA programme or the Department of Health. If there are verbatim quotations included in this publication the views and opinions expressed by the interviewees are those of the interviewees and do not necessarily reflect those of the authors, those of the NHS, the NIHR, NETSCC, the HTA programme or the Department of Health.
References
- de Boer IH, Rue TC, Cleary PA, Lachin JM, Molitch ME, Steffes MW, et al. Long-term renal outcomes of patients with type 1 diabetes mellitus and microalbuminuria: an analysis of the Diabetes Control and Complications Trial/Epidemiology of Diabetes Interventions and Complications cohort. Arch Intern Med 2011;171:412-20. http://dx.doi.org/10.1001/archinternmed.2011.16.
- Gerstein HC, Mann JF, Yi Q, Zinman B, Dinneen SF, Hoogwerf B, et al. Albuminuria and risk of cardiovascular events, death, and heart failure in diabetic and nondiabetic individuals. JAMA 2001;286:421-6. http://dx.doi.org/10.1001/jama.286.4.421.
- Thomas MC, Viberti G, Groop PH. Screening for chronic kidney disease in patients with diabetes: are we missing the point?. Nat Clin Pract Nephrol 2008;4:2-3. http://dx.doi.org/10.1038/ncpneph0679.
- Newman DJ, Mattock MB, Dawnay ABS, Kerry S, McGuire A, Yaqoob M, et al. Systematic review on urine albumin testing for early detection of diabetic complications. Health Technol Assess 2005;9.
- Black C, Sharma P, Scotland G, McCullough K, McGurn D, Robertson L, et al. Early referral strategies for management of people with markers of renal disease: a systematic review of the evidence of clinical effectiveness, cost-effectiveness and economic analysis. Health Technol Assess 2010;14.
- Drury PL, Ting R, Zannio D, Ehnholm C, Flack J, Whiting M, et al. Estimated glomerular filtration rate and albuminuria are independent predictors of cardiovascular events and death in type 2 diabetes mellitus: the Fenofibrate Intervention and Event Lowering in Diabetes (FIELD) study. Diabetologia 2011;54:32-43. http://dx.doi.org/10.1007/s00125-010-1854-1.
- Dinneen SF, Gerstein HC. The association of microalbuminuria and mortality in non-insulin-dependent diabetes mellitus. A systematic overview of the literature. Arch Intern Med 1997;157:1413-18. http://dx.doi.org/10.1001/archinte.157.13.1413.
- Danaei G, Finucane MM, Lu Y, Singh GM, Cowan MJ, Paciorek CJ, et al. National, regional, and global trends in fasting plasma glucose and diabetes prevalence since 1980: systematic analysis of health examination surveys and epidemiological studies with 370 country-years and 2.7 million participants. Lancet 2011;378:31-40. http://dx.doi.org/10.1016/S0140-6736(11)60679-X.
- Remuzzi G, Schieppati A, Ruggenenti P. Clinical practice. Nephropathy in patients with type 2 diabetes. N Engl J Med 2002;346:1145-51.
- Raile K, Galler A, Hofer S, Herbst A, Dunstheimer D, Busch P, et al. Diabetic nephropathy in 27,805 children, adolescents, and adults with type 1 diabetes: effect of diabetes duration, A1C, hypertension, dyslipidemia, diabetes onset, and sex. Diabetes Care 2007;30:2523-8. http://dx.doi.org/10.2337/dc07-0282.
- Groop PH, Thomas MC, Moran JL, Waden J, Thorn LM, Makinen VP, et al. The presence and severity of chronic kidney disease predicts all-cause mortality in type 1 diabetes. Diabetes 2009;58:1651-8. http://dx.doi.org/10.2337/db08-1543.
- Gordois A, Scuffham P, Shearer A, Oglesby A. The health care costs of diabetic nephropathy in the United States and the United Kingdom. J Diabetes Complications 2004;18:18-26. http://dx.doi.org/10.1016/S1056-8727(03)00035-7.
- Levey AS, Bosch JP, Lewis JB, Greene T, Rogers N, Roth D, et al. A more accurate method to estimate glomerular filtration rate from serum creatinine: a new prediction equation. Ann Intern Med 1999;130:461-70. http://dx.doi.org/10.7326/0003-4819-130-6-199903160-00002.
- American Diabetes Association . Clinical Practice Recommendations 2009. Diabetes Care 2009;32.
- Hogg RJ, Furth S, Lemley KV, Portman R, Schwartz GJ, Coresh J, et al. National Kidney Foundation’s kidney disease outcomes quality initiative clinical practice guidelines for chronic kidney disease in children and adolescents: evaluation, classification, and stratification. Pediatrics 2003;111:1416-21. http://dx.doi.org/10.1542/peds.111.6.1416.
- Sacks DB, Arnold M, Bakris GL, Bruns DE, Horvath AR, Kirkman MS, et al. Guidelines and recommendations for laboratory analysis in the diagnosis and management of diabetes mellitus. Diabetes Care 2011;34:e61-99. http://dx.doi.org/10.2337/dc11-9998.
- Connell SJ, Hollis S, Tieszen KL, McMurray JR, Dornan TL. Gender and the clinical usefulness of the albumin : creatinine ratio. Diabet Med 1994;11:32-6. http://dx.doi.org/10.1111/j.1464-5491.1994.tb00226.x.
- Amin R, Widmer B, Prevost AT, Schwarze P, Cooper J, Edge J, et al. Risk of microalbuminuria and progression to macroalbuminuria in a cohort with childhood onset type 1 diabetes: prospective observational study. BMJ 2008;336:697-701. http://dx.doi.org/10.1136/bmj.39478.378241.BE.
- Type 2 Diabetes: National Clinical Guideline for Management in Primary and Secondary Care (update). London: Royal College of Physicians; 2008.
- Bank N. Mechanisms of diabetic hyperfiltration. Kidney Int 1991;40:792-807. http://dx.doi.org/10.1038/ki.1991.277.
- Mogensen CE, Poulsen PL. Microalbuminuria, glycemic control, and blood pressure predicting outcome in diabetes type 1 and type 2. Kidney Int Suppl 2004;92:S40-1. http://dx.doi.org/10.1111/j.1523-1755.2004.09210.x.
- Adler AI, Stevens RJ, Manley SE, Bilous RW, Cull CA, Holman RR, et al. Development and progression of nephropathy in type 2 diabetes: the United Kingdom Prospective Diabetes Study (UKPDS 64). Kidney Int 2003;63:225-32. http://dx.doi.org/10.1046/j.1523-1755.2003.00712.x.
- Valmadrid CT, Klein R, Moss SE, Klein BE. The risk of cardiovascular disease mortality associated with microalbuminuria and gross proteinuria in persons with older-onset diabetes mellitus. Arch Intern Med 2000;160:1093-100. http://dx.doi.org/10.1001/archinte.160.8.1093.
- MacIsaac RJ, Panagiotopoulos S, McNeil KJ, Smith TJ, Tsalamandris C, Hao H, et al. Is nonalbuminuric renal insufficiency in type 2 diabetes related to an increase in intrarenal vascular disease?. Diabetes Care 2006;29:1560-6. http://dx.doi.org/10.2337/dc05-1788.
- Kramer HJ, Nguyen QD, Curhan G, Hsu CY. Renal insufficiency in the absence of albuminuria and retinopathy among adults with type 2 diabetes mellitus. JAMA 2003;289:3273-7. http://dx.doi.org/10.1001/jama.289.24.3273.
- New JP, Middleton RJ, Klebe B, Farmer CK, de Lusignan S, Stevens PE, et al. Assessing the prevalence, monitoring and management of chronic kidney disease in patients with diabetes compared with those without diabetes in general practice. Diabet Med 2007;24:364-9. http://dx.doi.org/10.1111/j.1464-5491.2007.02075.x.
- Lin J, Glynn RJ, Rifai N, Manson JE, Ridker PM, Nathan DM, et al. Inflammation and progressive nephropathy in type 1 diabetes in the diabetes control and complications trial. Diabetes Care 2008;31:2338-43. http://dx.doi.org/10.2337/dc08-0277.
- Bilous R, Chaturvedi N, Sjolie AK, Fuller J, Klein R, Orchard T, et al. Effect of candesartan on microalbuminuria and albumin excretion rate in diabetes: three randomized trials. Ann Intern Med 2009;151:11-20. http://dx.doi.org/10.7326/0003-4819-151-1-200907070-00120.
- Rossing P, Hougaard P, Parving H. Progression of microalbuminuria in type 1 diabetes: ten-year prospective observational study. Kidney Int 2005;68:1446-50. http://dx.doi.org/10.1111/j.1523-1755.2005.00556.x.
- Ravid M, Savin H, Jutrin I, Bental T, Katz B, Lishner M. Long-term stabilizing effect of angiotensin-converting enzyme inhibition on plasma creatinine and on proteinuria in normotensive type II diabetic patients. Ann Intern Med 1993;118:577-81. http://dx.doi.org/10.7326/0003-4819-118-8-199304150-00001.
- Ahmad J, Siddiqui MA, Ahmad H. Effective postponement of diabetic nephropathy with enalapril in normotensive type 2 diabetic patients with microalbuminuria. Diabetes Care 1997;20:1576-81. http://dx.doi.org/10.2337/diacare.20.10.1576.
- Christensen CK, Mogensen CE. The course of incipient diabetic nephropathy: studies of albumin excretion and blood pressure. Diabet Med 1985;2:97-102. http://dx.doi.org/10.1111/j.1464-5491.1985.tb00608.x.
- Vergouwe Y, Soedamah-Muthu SS, Zgibor J, Chaturvedi N, Forsblom C, Snell-Bergeon JK, et al. Progression to microalbuminuria in type 1 diabetes: development and validation of a prediction rule. Diabetologia 2010;53:254-62. http://dx.doi.org/10.1007/s00125-009-1585-3.
- Kilpatrick ES, Rigby AS, Atkin SL. A1C variability and the risk of microvascular complications in type 1 diabetes: data from the Diabetes Control and Complications Trial. Diabetes Care 2008;31:2198-202. http://dx.doi.org/10.2337/dc08-0864.
- Chaturvedi N, Bandinelli S, Mangili R, Penno G, Rottiers RE, Fuller JH. Microalbuminuria in type 1 diabetes: rates, risk factors and glycemic threshold. Kidney Int 2001;60:219-27. http://dx.doi.org/10.1046/j.1523-1755.2001.00789.x.
- Waden J, Forsblom C, Thorn LM, Gordin D, Saraheimo M, Groop PH, et al. A1C variability predicts incident cardiovascular events, microalbuminuria, and overt diabetic nephropathy in patients with type 1 diabetes. Diabetes 2009;58:2649-55. http://dx.doi.org/10.2337/db09-0693.
- Royal College of Physicians of Edinburgh Diabetes Register Group . Near-normal urinary albumin concentrations predict progression to diabetic nephropathy in Type 1 diabetes mellitus. Diabetic Med 2000;17:782-91.
- Rossing P, Hougaard P, Parving HH. Risk factors for development of incipient and overt diabetic nephropathy in type 1 diabetic patients: a 10-year prospective observational study. Diabetes Care 2002;25:859-64. http://dx.doi.org/10.2337/diacare.25.5.859.
- Retnakaran R, Cull CA, Thorne KI, Adler AI, Holman RR. UKPDS Study Group . Risk factors for renal dysfunction in type 2 diabetes: U.K. Prospective Diabetes Study 74. Diabetes 2006;55:1832-9. http://dx.doi.org/10.2337/db05-1620.
- Amini M, Safaei H, Aminorroaya A. The incidence of microalbuminuria and its associated risk factors in type 2 diabetic patients in Isfahan, Iran. Rev Diabet Stud 2007;4. http://dx.doi.org/10.1900/RDS.2007.4.242.
- Agrawal L, Azad N, Emanuele NV, Bahn GD, Kaufman DG, Moritz TE, et al. Observation on renal outcomes in the Veterans Affairs Diabetes Trial. Diabetes Care 2011;34:2090-4. http://dx.doi.org/10.2337/dc11-0175.
- Bruno G, Merletti F, Biggeri A, Bargero G, Ferrero S, Pagano G, et al. Progression to overt nephropathy in type 2 diabetes: the Casale Monferrato study. Diabetes Care 2003;26:2150-5. http://dx.doi.org/10.2337/diacare.26.7.2150.
- Xu J, Lee ET, Devereux RB, Umans JG, Bella JN, Shara NM, et al. A longitudinal study of risk factors for incident albuminuria in diabetic American Indians: the Strong Heart Study. Am J Kidney Dis 2008;51:415-24. http://dx.doi.org/10.1053/j.ajkd.2007.11.010.
- Chandie Shaw PK, Baboe F, van Es LA, van der Vijver JC, van de Ree MA, de Jonge N, et al. South-Asian type 2 diabetic patients have higher incidence and faster progression of renal disease compared with Dutch-European diabetic patients. Diabetes Care 2006;29:1383-5. http://dx.doi.org/10.2337/dc06-0003.
- Nathan DM, Cleary PA, Backlund JY, Genuth SM, Lachin JM, Orchard TJ, et al. Intensive diabetes treatment and cardiovascular disease in patients with type 1 diabetes. N Engl J Med 2005;353:2643-53.
- Sawicki PT, Didjurgeit U, Muhlhauser I, Bender R, Heinemann L, Berger M. Smoking is associated with progression of diabetic nephropathy. Diabetes Care 1994;17:126-31. http://dx.doi.org/10.2337/diacare.17.2.126.
- Ritz E, Orth SR. Nephropathy in patients with type 2 diabetes mellitus. N Engl J Med 1999;341:1127-33. http://dx.doi.org/10.1056/NEJM199910073411506.
- Schultz CJ, Konopelska-Bahu T, Dalton RN, Carroll TA, Stratton I, Gale EA, et al. Microalbuminuria prevalence varies with age, sex, and puberty in children with type 1 diabetes followed from diagnosis in a longitudinal study. Oxford Regional Prospective Study Group. Diabetes Care 1999;22:495-502. http://dx.doi.org/10.2337/diacare.22.3.495.
- Burden AC, McNally PG, Feehally J, Walls J. Increased incidence of end-stage renal failure secondary to diabetes mellitus in Asian ethnic groups in the United Kingdom. Diabet Med 1992;9:641-5. http://dx.doi.org/10.1111/j.1464-5491.1992.tb01860.x.
- Roderick PJ, Raleigh VS, Hallam L, Mallick NP. The need and demand for renal replacement therapy in ethnic minorities in England. J Epidemiol Community Health 1996;50:334-9. http://dx.doi.org/10.1136/jech.50.3.334.
- Orchard TJ, Secrest AM, Miller RG, Costacou T. In the absence of renal disease, 20 year mortality risk in type 1 diabetes is comparable to that of the general population: a report from the Pittsburgh Epidemiology of Diabetes Complications Study. Diabetologia 2010;53:2312-19. http://dx.doi.org/10.1007/s00125-010-1860-3.
- Mogensen CE, Christensen CK, Vittinghus E. The stages in diabetic renal disease. With emphasis on the stage of incipient diabetic nephropathy. Diabetes 1983;32:64-78. http://dx.doi.org/10.2337/diab.32.2.S64.
- Gall MA, Rossing P, Skott P, Damsbo P, Vaag A, Bech K, et al. Prevalence of micro- and macroalbuminuria, arterial hypertension, retinopathy and large vessel disease in European type 2 (non-insulin-dependent) diabetic patients. Diabetologia 1991;34:655-61. http://dx.doi.org/10.1007/BF00400995.
- Jørgensen L, Heuch I, Jenssen T, Jacobsen BK. Association of albuminuria and cancer incidence. J Am Soc Nephrol 2008;19:992-8. http://dx.doi.org/10.1681/ASN.2007060712.
- Wong G, Hayen A, Chapman JR, Webster AC, Wang JJ, Mitchell P, et al. Association of CKD and cancer risk in older people. J Am Soc Nephrol 2009;20:1341-50. http://dx.doi.org/10.1681/ASN.2008090998.
- Drion I, van Hateren KJ, Joosten H, Alkhalaf A, Groenier KH, Kleefstra N, et al. Chronic kidney disease and mortality risk among older patients with type 2 diabetes mellitus (ZODIAC-24). Age Ageing 2012;41:345-50. http://dx.doi.org/10.1093/ageing/afs002.
- UK Prospective Diabetes Study Group . Intensive blood glucose control with sulphonylureas or insulin compared with conventional treatment and risk of complications in patients with type 2 diabetes (UKPDS 33). UK Prospective Diabetes Study (UKPDS) Group. Lancet 1998;352:837-53.
- Patel A, MacMahon S, Chalmers J, Neal B, Billot L, Woodward M, et al. Intensive blood glucose control and vascular outcomes in patients with type 2 diabetes. N Engl J Med 2008;358:2560-72.
- Bakris GL, Williams M, Dworkin L, Elliott WJ, Epstein M, Toto R, et al. Preserving renal function in adults with hypertension and diabetes: a consensus approach. National Kidney Foundation Hypertension and Diabetes Executive Committees Working Group. Am J Kidney Dis 2000;36:646-61.
- UK Prospective Diabetes Study Group . Tight blood pressure control and risk of macrovascular and microvascular complications in type 2 diabetes (UKPDS 38). UK Prospective Diabetes Study (UKPDS) Group. BMJ 1998;317:703-13.
- de Galan BE, Perkovic V, Ninomiya T, Pillai A, Patel A, Cass A, et al. Lowering blood pressure reduces renal events in type 2 diabetes. J Am Soc Nephrol 2009;20:883-92. http://dx.doi.org/10.1681/ASN.2008070667.
- Cushman WC, Evans GW, Byington RP, Goff DC, Grimm RH, . ACCORD Study Group . Effects of intensive blood-pressure control in type 2 diabetes mellitus. N Engl J Med 2010;362:1575-85.
- Strippoli GF, Craig M, Craig JC. Antihypertensive agents for preventing diabetic kidney disease. Cochrane Database Syst Rev 2005;4. http://dx.doi.org/10.1002/14651858.CD004136.
- Strippoli GF, Navaneethan SD, Johnson DW, Perkovic V, Pellegrini F, Nicolucci A, et al. Effects of statins in patients with chronic kidney disease: meta-analysis and meta-regression of randomised controlled trials. BMJ 2008;336:645-51. http://dx.doi.org/10.1136/bmj.39472.580984.AE.
- Nagrebetsky A, Jin J, Stevens R, James T, Adler A, Park P, et al. Diagnostic accuracy of urine dipstick testing in screening for microalbuminuria in type 2 diabetes: a cohort study in primary care. Fam Pract 2013;30:142-52. http://dx.doi.org/10.1093/fampra/cms057.
- National Kidney Foundation . K/DOQI clinical practice guidelines for chronic kidney disease: evaluation, classification, and stratification. Am J Kidney Dis 2002;39:S1-266.
- Noble D, Mathur R, Dent T, Meads C, Greenhalgh T. Risk models and scores for type 2 diabetes: systematic review. BMJ 2011;343. http://dx.doi.org/10.1136/bmj.d7163.
- Clarke PM, Gray AM, Briggs A, Farmer A, Fenn P, Stevens R, et al. A model to estimate the lifetime health outcomes of patients with type 2 diabetes: the United Kingdom Prospective Diabetes Study (UKPDS) Outcomes Model (UKPDS no. 68). Diabetologia 2004;47:1747-59. http://dx.doi.org/10.1007/s00125-004-1527-z.
- Wirta OR, Pasternack AI, Mustonen JT, Koivula TA, Harmoinen A. Urinary albumin excretion rate and its determinants after 6 years in non-insulin-dependent diabetic patients. Nephrol Dial Transplant 1996;11:449-56. http://dx.doi.org/10.1093/oxfordjournals.ndt.a027310.
- Niskanen L, Voutilainen R, Terasvirta M, Lehtinen J, Teppo AM, Groop L, et al. A prospective study of clinical and metabolic associates of proteinuria in patients with type 2 diabetes mellitus. Diabet Med 1993;10:543-9. http://dx.doi.org/10.1111/j.1464-5491.1993.tb00118.x.
- Gerstein HC, Yusuf S, Mann JFE, Hoogwerf B, Zinman B, . HOPE Study Investigators . Effects of ramipril on cardiovascular and microvascular outcomes in people with diabetes mellitus: results of the HOPE study and MICRO-HOPE substudy. Lancet 2000;355:253-9.
- Lewis EJ, Hunsicker LG, Clarke WR, Berl T, Pohl MA, Lewis JB, et al. Renoprotective effect of the angiotensin-receptor antagonist irbesartan in patients with nephropathy due to type 2 diabetes. N Engl J Med 2001;345:851-60. http://dx.doi.org/10.1056/NEJMoa011303.
- Higgins JP, Green S. Cochrane Handbook for Systematic Reviews of Interventions. Oxford: Wiley; 2008.
- DerSimonian R, Laird N. Meta-analysis in clinical trials. Control Clin Trials 1986;7:177-88. http://dx.doi.org/10.1016/0197-2456(86)90046-2.
- Friedrich JO, Adhikari NK, Beyene J. Ratio of means for analyzing continuous outcomes in meta-analysis performed as well as mean difference methods. J Clin Epidemiol 2011;64:556-64. http://dx.doi.org/10.1016/j.jclinepi.2010.09.016.
- Friedrich J, Adhikari N, Beyene J. The ratio of means method as an alternative to mean differences for analysing continuous outcome variables in meta-analysis: a simulation study. BMC Med Res Methodol 2008;8. http://dx.doi.org/10.1186/1471-2288-8-32.
- Kunz R, Friedrich C, Wolbers M, Mann JF. Meta-analysis: effect of monotherapy and combination therapy with inhibitors of the renin angiotensin system on proteinuria in renal disease. Ann Intern Med 2008;148:30-48. http://dx.doi.org/10.7326/0003-4819-148-1-200801010-00190.
- Bakris GL, Barnhill BW, Sadler R. Treatment of arterial hypertension in diabetic humans: Importance of therapeutic selection. Kidney Int 1992;41:912-9. http://dx.doi.org/10.1038/ki.1992.139.
- Cordonnier DJ, Pinel N, Barro C, Maynard C, Zaoui P, Halimi S, et al. Expansion of cortical interstitium is limited by converting enzyme inhibition in type 2 diabetic patients with glomerulosclerosis. J Am Soc Nephrol 1999;10:1253-63.
- Lebovitz HE, Wiegmann TB, Cnaan A, Shahinfar S, Sica DA, Broadstone V, et al. Renal protective effects of enalapril in hypertensive NIDDM: role of baseline albuminuria. Kidney Int Suppl 1994;45:S150-5.
- Crepaldi G, Carraro A, Brocco E, Adezati L, Andreani D, Bompiani G, et al. Hypertension and non-insulin-dependent diabetes – a comparison between an angiotensin-converting enzyme inhibitor and a calcium antagonist. Acta Diabetol 1995;32:203-8. http://dx.doi.org/10.1007/BF00838494.
- Mauer M, Zinman B, Gardiner R, Suissa S, Sinaiko A, Strand T, et al. Renal and retinal effects of enalapril and losartan in type 1 diabetes. N Engl J Med 2009;361:40-51. http://dx.doi.org/10.1056/NEJMoa0808400.
- Muirhead N, Feagan BF, Mahon J, Lewanczuk RZ, Rodger NW, Botteri F, et al. The effects of valsartan and captopril on reducing microalbuminuria in patients with type 2 diabetes mellitus: a placebo-controlled trial. Curr Therapeutic Res 1999;60:650-60. http://dx.doi.org/10.1016/S0011-393X(99)90003-6.
- Ogawa S, Takeuchi K, Mori T, Nako K, Tsubono Y, Ito S, et al. Effects of monotherapy of temocapril or candesartan with dose increments or combination therapy with both drugs on the suppression of diabetic nephropathy. Hypertens Res 2007;30:325-34. http://dx.doi.org/10.1291/hypres.30.325.
- EUCLID study group . Randomised placebo-controlled trial of lisinopril in normotensive patients with insulin-dependent diabetes and normoalbuminuria or microalbuminuria. The EUCLID study group. Lancet 1997;349:1787-92.
- Baba S, Koike T, Makino I, Maruhama Y, Sasaki H, Goto Y, et al. Nifedipine and enalapril equally reduce the progression of nephropathy in hypertensive type 2 diabetics. Diabetes Res Clin Pract 2001;54:191-20. http://dx.doi.org/10.1016/S0168-8227(01)00288-1.
- Chan JCN, Ko GTC, Leung DHY, Cheung RCK, Cheung MYF, So WY, et al. Long-term effects of angiotensin-converting enzyme inhibition and metabolic control in hypertensive type 2 diabetic patients. Kidney Int 2000;57:590-60. http://dx.doi.org/10.1046/j.1523-1755.2000.00879.x.
- Sawaki H, Terasaki J, Fujita A, Nakagawa S, Kanatsuna N, Sadahiro K, et al. A renoprotective effect of low dose losartan in patients with type 2 diabetes. Diabetes Res Clin Pract 2008;79:86-90. http://dx.doi.org/10.1016/j.diabres.2007.08.004.
- Perrin NESS, Jaremko GA, Berg UB. The effects of candesartan on diabetes glomerulopathy: a double-blind, placebo-controlled trial. Pediatr Nephrol 2008;23:947-54. http://dx.doi.org/10.1007/s00467-008-0745-x.
- Tuominen JA, Ebeling P, Koivisto VA. Long-term lisinopril therapy reduces exercise-induced albuminuria in normoalbuminuric normotensive IDDM patients. Diabetes Care 1998;21:1345-8. http://dx.doi.org/10.2337/diacare.21.8.1345.
- Kvetny J, Gregersen G, Pedersen RS. Randomized placebo-controlled trial of perindopril in normotensive, normoalbuminuric patients with type 1 diabetes mellitus. QJM 2001;94:89-94. http://dx.doi.org/10.1093/qjmed/94.2.89.
- Garg SK, Chase HP, Jackson WE, Harris S, Carmain JA, Hansen MH, et al. Renal and retinal changes after treatment with ramipril and pentoxifylline in subjects with IDDM. Ann Ophthalmol Glaucoma 1998;30:33-7.
- Bakris GL, Slataper R, Vicknair N, Sadler R. ACE inhibitor mediated reductions in renal size and microalbuminuria in normotensive, diabetic subjects. J Diabetes Complications 1994;8:2-6. http://dx.doi.org/10.1016/1056-8727(94)90003-5.
- O’Hare P, Bilbous R, Mitchell T, O’Callaghan CJ, Viberti GC. Ace-inhibitor Trial to Lower Albuminuria in Normotensive Insulin-Dependent Subjects Study Group . Low-dose ramipril reduces microalbuminuria in type 1 diabetic patients without hypertension: results of a randomized controlled trial. Diabetes Care 2000;23:1823-9.
- Bojestig M, Karlberg BE, Lindström T, Nystrom FH. Reduction of ACE activity is insufficient to decrease microalbuminuria in normotensive patients with type 1 diabetes. Diabetes Care 2001;24:919-24. http://dx.doi.org/10.2337/diacare.24.5.919.
- Chase H, Garg S, Harris S, Hoops S, Jackson W, Holmes D. Angiotensin-converting enzyme inhibitor treatment for young normotensive diabetic subjects: a two-year trial. Ann Ophthalmol 1993;25.
- Laffel LM, McGill JB, Gans DJ. The beneficial effect of angiotensin-converting enzyme inhibition with captopril on diabetic nephropathy in normotensive IDDM patients with microalbuminuria. Am J Med 1995;99:497-504. http://dx.doi.org/10.1016/S0002-9343(99)80226-5.
- Poulsen P, Ebbehøj E, Mogensen CE. Lisinopril reduces albuminuria during exercise in low grade microalbuminuric type 1 diabetic patients: a double blind randomized study. J Intern Med 2001;249:433-40. http://dx.doi.org/10.1046/j.1365-2796.2001.00821.x.
- Viberti G, Mogensen CE, Groop LC, Pauls JF, Boner G, van Dyk D, et al. Effect of captopril on progression to clinical proteinuria in patients with insulin-dependent diabetes mellitus and microalbuminuria. JAMA 1994;271:275-9. http://dx.doi.org/10.1001/jama.1994.03510280037029.
- Jerums G, Allen TJ, Campbell DJ, Cooper ME, Gilbert RE, Hammond JJ, et al. Long-term comparison between perindopril and nifedipine in normotensive patients with type 1 diabetes and microalbuminuria. Am J Kidney Dis 2001;37:890-9. http://dx.doi.org/10.1016/S0272-6386(05)80003-4.
- Lewis EJ, Hunsicker LG, Bain RP, Rohde RD. The effect of angiotensin-converting-enzyme inhibition on diabetic nephropathy. N Engl J Med 1993;329:1456-62. http://dx.doi.org/10.1056/NEJM199311113292004.
- Crepaldi G, Carta Q, Deferrari G, Mangili R, Navalesi R, Santeusanio F, et al. Effects of lisinopril and nifedipine on the progression to overt albuminuria in IDDM patients with incipient nephropathy and normal blood pressure. Diabetes Care 1998;21:104-10. http://dx.doi.org/10.2337/diacare.21.1.104.
- Mathiesen ER, Hommel E, Hansen HP, Smidt UM, Parving H. Randomised controlled trial of long term efficacy of captopril on preservation of kidney function in normotensive patients with insulin dependent diabetes and microalbuminuria. BMJ 1999;319:24-5. http://dx.doi.org/10.1136/bmj.319.7201.24.
- Ahmad J, Shafique S, Abidi S, Parwez I. Effect of 5-year enalapril therapy on progression of microalbuminuria and glomerular structural changes in type 1 diabetic subjects. Diabetes Res Clin Pract 2003;60. http://dx.doi.org/10.1016/S0168-8227(03)00016-0.
- Parving H, Hommel E, Jensen BR, Hansen HP. Long-term beneficial effect of ACE inhibition on diabetic nephropathy in normotensive type 1 diabetic patients. Kidney Int 2001;60:228-34. http://dx.doi.org/10.1046/j.1523-1755.2001.00790.x.
- Ruggenenti P, Fassi A, Ilieva AP, Bruno S, Iliev IP, Brusegan V, et al. Preventing microalbuminuria in type 2 diabetes. N Engl J Med 2004;351:1941-51. http://dx.doi.org/10.1056/NEJMoa042167.
- Haller H, Ito S, Izzo JL, Januszewicz A, Katayama S, Menne J, et al. Olmesartan for the delay or prevention of microalbuminuria in type 2 diabetes. N Engl J Med 2011;364:907-17. http://dx.doi.org/10.1056/NEJMoa1007994.
- Zoungas S, de Galan BE, Ninomiya T, Grobbee D, Hamet P, Heller S, et al. Combined effects of routine blood pressure lowering and intensive glucose control on macrovascular and microvascular outcomes in patients with type 2 diabetes: new results from the ADVANCE trial. Diabetes Care 2009;32:2068-74. http://dx.doi.org/10.2337/dc09-0959.
- Patel A, MacMahon S, Chalmers J, Neal B, Woodward M, . ADVANCE Collaborative Group . Effects of a fixed combination of perindopril and indapamide on macrovascular and microvascular outcomes in patients with type 2 diabetes mellitus (the ADVANCE trial): a randomised controlled trial. Lancet 2007;370:829-40. http://dx.doi.org/10.1016/S0140-6736(07)61303-8.
- Ravid M, Brosh D, Levi Z, Bar-Dayan Y, Ravid D, Rachmani R. Use of enalapril to attenuate decline in renal function in normotensive, normoalbuminuric patients with type 2 diabetes mellitus. A randomized, controlled trial. Ann Intern Med 1998;128:982-8. http://dx.doi.org/10.7326/0003-4819-128-12_Part_1-199806150-00004.
- UK Prospective Diabetes Study Group . Efficacy of atenolol and captopril in reducing risk of macrovascular and microvascular complications in type 2 diabetes: UKPDS 39. UK Prospective Diabetes Study Group. BMJ 1998;317:713-20.
- Romero R, Salinas I, Lucas A, Abad E, Reverter JL, Johnston S, et al. Renal function changes in microalbuminuric normotensive type II diabetic patients treated with angiotensin-converting enzyme inhibitors. Diabetes Care 1993;16:597-600. http://dx.doi.org/10.2337/diacare.16.4.597.
- Uzu T, Sawaguchi M, Maegawa H, Kashiwagi A. Reduction of microalbuminuria in patients with type 2 diabetes mellitus: the Shiga Microalbuminuria Reduction Trial (SMART). Diabetes Care 2007;30:1581-3.
- Tan KC, Chow W, Ai VH, Lam KS. Effects of angiotensin II receptor antagonist on endothelial vasomotor function and urinary albumin excretion in type 2 diabetic patients with microalbuminuria. Diabetes Metab Res 2002;18:71-6. http://dx.doi.org/10.1002/dmrr.255.
- Trevisan R, Tiengo A. Effect of low-dose ramipril on microalbuminuria in normotensive or mild hypertensive non-insulin-dependent diabetic patients. Am J Hypertens 1995;8:876-83. http://dx.doi.org/10.1016/0895-7061(95)00162-I.
- Rodríguez-Morán M, Guerrero-Romero F. Pentoxifylline is as effective as captopril in the reduction of microalbuminuria in non-hypertensive type 2 diabetic patients-a randomized, equivalent trial. Clin Nephrol 2005;64:91-7.
- de Mello VD, Zelmanovitz T, Azevedo MJ, de Paula TP, Gross JL. Long-term effect of a chicken-based diet versus enalapril on albuminuria in type 2 diabetic patients with microalbuminuria. J Ren Nutr 2008;18:440-7. http://dx.doi.org/10.1053/j.jrn.2008.04.010.
- Puig JG, Marre M, Kokot F, Fernandez M, Jermendy G, Opie L, et al. Efficacy of indapamide SR compared with enalapril in elderly hypertensive patients with type 2 diabetes. Am J Hypertens 2007;20:90-7. http://dx.doi.org/10.1016/j.amjhyper.2006.05.018.
- Makino H, Haneda M, Babazono T, Moriya T, Ito S, Iwamoto Y, et al. Prevention of transition from incipient to overt nephropathy with telmisartan in patients with type 2 diabetes. Diabetes Care 2007;30:1577-8. http://dx.doi.org/10.2337/dc06-1998.
- Tong P, Ko G, Chan W, Ma R, So W, Lo M, et al. The efficacy and tolerability of fosinopril in Chinese type 2 diabetic patients with moderate renal insufficiency. Diabetes Obes Metab 2006;8:342-7. http://dx.doi.org/10.1111/j.1463-1326.2005.00514.x.
- Parving H, Lehnert H, Brochner-Mortensen JF, Gomis R, Andersen S, Arner P, et al. The effect of irbesartan on the development of diabetic nephropathy in patients with type 2 diabetes. N Engl J Med 2001;345:870-8. http://dx.doi.org/10.1056/NEJMoa011489.
- Berl T, Hunsicker LG, Lewis JB, Pfeffer MA, Porush JG, Rouleau J, et al. Cardiovascular outcomes in the Irbesartan Diabetic Nephropathy Trial of patients with type 2 diabetes and overt nephropathy. Ann Intern Med 2003;138:542-9. http://dx.doi.org/10.7326/0003-4819-138-7-200304010-00010.
- de Zeeuw D, Remuzzi G, Parving H, Keane WF, Zhang Z, Shahinfar S, et al. Proteinuria, a target for renoprotection in patients with type 2 diabetic nephropathy: lessons from RENAAL. Kidney Int 2004;65:2309-20. http://dx.doi.org/10.1111/j.1523-1755.2004.00653.x.
- Marre M, Lievre M, Chatellier G, Mann JF, Passa P, Ménard J. Effects of low dose ramipril on cardiovascular and renal outcomes in patients with type 2 diabetes and raised excretion of urinary albumin: randomised, double blind, placebo controlled trial (the DIABHYCAR study). BMJ 2004;328. http://dx.doi.org/10.1136/bmj.37970.629537.0D.
- Sano T, Hotta N, Kawamura T, Matsumae H, Chaya S, Sasaki H, et al. Effects of long-term enalapril treatment on persistent microalbuminuria in normotensive type 2 diabetic patients: results of a 4-year, prospective, randomized study. Diabetic Med 1996;13:120-4. http://dx.doi.org/10.1002/(SICI)1096-9136(199602)13:2<120::AID-DIA6>3.3.CO;2-6.
- Strippoli GFM, Bonifati C, Craig M, Navaneethan SD, Craig JC. Angiotensin converting enzyme inhibitors and angiotensin II receptor antagonists for preventing the progression of diabetic kidney disease. Cochrane Database Syst Rev 2006. http://dx.doi.org/10.1002/14651858.CD006257.
- Riley RD, Gates S, Neilson J, Alfirevic Z. Statistical methods can be improved within Cochrane pregnancy and childbirth reviews. J Clin Epidemiol 2011;64:608-18. http://dx.doi.org/10.1016/j.jclinepi.2010.08.002.
- Casas JP, Chua W, Loukogeorgakis S, Vallance P, Smeeth L, Hingorani AD, et al. Effect of inhibitors of the renin-angiotensin system and other antihypertensive drugs on renal outcomes: systematic review and meta-analysis. Lancet 2005;366:2026-33. http://dx.doi.org/10.1016/S0140-6736(05)67814-2.
- Gerstein HC. Diabetes and the HOPE study: implications for macrovascular and microvascular disease. Int J Clin Prac Suppl 2001;117:8-12.
- Lambers Heerspink HJ, Gansevoort RT, Brenner BM, Cooper ME, Parving HH, Shahinfar S, et al. Comparison of different measures of urinary protein excretion for prediction of renal events. J Am Soc Nephrol 2010;21:1355-60. http://dx.doi.org/10.1681/ASN.2010010063.
- Yudkin JS, Lehman R. Olmesartan, microalbuminuria, and type 2 diabetes. N Engl J Med 2011;364.
- Vejakama P, Thakkinstian A, Lertrattananon D, Ingsathit A, Ngarmukos C, Attia J. Reno-protective effects of renin-angiotensin system blockade in type 2 diabetic patients: a systematic review and network meta-analysis. Diabetologia 2012;55:566-78. http://dx.doi.org/10.1007/s00125-011-2398-8.
- Rosen AB. Indications for and utilization of ACE inhibitors in older individuals with diabetes: findings from the national health and nutrition examination survey 1999 to 2002. J Gen Intern Med 2006;21:315-9. http://dx.doi.org/10.1111/j.1525-1497.2006.00351.x.
- Glasziou PP, Irwig L, Heritier S, Simes RJ, Tonkin A. LIPID Study Investigators . Monitoring cholesterol levels: measurement error or true change?. Ann Intern Med 2008;148:656-61. http://dx.doi.org/10.7326/0003-4819-148-9-200805060-00005.
- Keenan K, Hayen A, Neal BC, Irwig L. Long term monitoring in patients receiving treatment to lower blood pressure: analysis of data from placebo controlled randomised controlled trial. BMJ 2009;338. http://dx.doi.org/10.1136/bmj.b1492.
- Takahashi O, Farmer AJ, Shimbo T, Fukui T, Glasziou PP. A1C to detect diabetes in healthy adults: when should we recheck?. Diabetes Care 2010;33:2016-17. http://dx.doi.org/10.2337/dc10-0588.
- Oke JL, Stevens R, Gaitskell K, Farmer A. Establishing an evidence base for frequency of monitoring glycated haemoglobin levels in patients with type 2 diabetes: projections of effectiveness from a regression model. Diabet Med 2012;29:266-71. http://dx.doi.org/10.1111/j.1464-5491.2011.03412.x.
- Schwartz GJ, Gauthier B. A simple estimate of glomerular filtration rate in adolescent boys. J Pediatr 1985;106:522-6. http://dx.doi.org/10.1016/S0022-3476(85)80697-1.
- Lunn DJ, Thomas A, Best N, Spiegelhalter D. WinBUGS – A Bayesian modelling framework: concepts, structure and extensibility. Stat Comput 2000;10:325-37.
- Stevens RJ, Oke J, Perera R. Statistical models for the control phase of clinical monitoring. Stat Methods Med Res 2010;19:394-41. http://dx.doi.org/10.1177/0962280209359886.
- Alleyn CR, Volkening LK, Wolfson J, Rodriguez-Ventura A, Wood JR, Laffel LM. Occurrence of microalbuminuria in young people with type 1 diabetes: importance of age and diabetes duration. Diabet Med 2010;27:532-7. http://dx.doi.org/10.1111/j.1464-5491.2010.02983.x.
- Andersen AR, Christiansen JS, Andersen JK, Kreiner S, Deckert T. Diabetic nephropathy in type 1 (insulin-dependent) diabetes: an epidemiological study. Diabetologia 1983;25:496-501. http://dx.doi.org/10.1007/BF00284458.
- Cho YH, Craig ME, Hing S, Gallego PH, Poon M, Chan A, et al. Microvascular complications assessment in adolescents with 2- to 5-yr duration of type 1 diabetes from 1990 to 2006. Pediatr Diabetes 2011;12:682-9. http://dx.doi.org/10.1111/j.1399-5448.2011.00762.x.
- Hovind P, Tarnow L, Rossing P, Jensen BR, Graae M, Torp I, et al. Predictors for the development of microalbuminuria and macroalbuminuria in patients with type 1 diabetes: inception cohort study. BMJ 2004;328. http://dx.doi.org/10.1136/bmj.38070.450891.FE.
- Krolewski AS, Warram JH, Christlieb AR, Busick EJ, Kahn CR. The changing natural history of nephropathy in type I diabetes. Am J Med 1985;78:785-94. http://dx.doi.org/10.1016/0002-9343(85)90284-0.
- Salgado PP, Silva IN, Vieira EC, Simões e Silva AC. Risk factors for early onset of diabetic nephropathy in pediatric type 1 diabetes. J Pediatr Endocrinol Metab 2010;23:1311-20.
- Tryggvason G, Indridason OS, Thorsson AV, Hreidarsson AB, Palsson R. Unchanged incidence of diabetic nephropathy in type 1 diabetes: a nation-wide study in Iceland. Diabet Med 2005;22:182-7. http://dx.doi.org/10.1111/j.1464-5491.2004.01390.x.
- Clinical guideline 15: type 1 diabetes: diagnosis and management of type 1 diabetes in children, young people and adults. Manchester: NICE; 2004.
- Adolescent Type 1 Diabetes Cardio-renal Intervention Trial Research Group . Adolescent type 1 diabetes cardio-renal intervention trial (AdDIT). BMC Pediatr 2009;9.
- Molitch ME, Steffes M, Sun W, Rutledge B, Cleary P, De Boer IH, et al. Development and progression of renal insufficiency with and without albuminuria in adults with type 1 diabetes in the diabetes control and complications trial and the epidemiology of diabetes interventions and complications study. Diabetes Care 2010;33:1536-43. http://dx.doi.org/10.2337/dc09-1098.
- Colhoun HM, Thomason MJ, Mackness MI, Maton SM, Betteridge DJ, Durrington PN, et al. Design of the Collaborative AtoRvastatin Diabetes Study (CARDS) in patients with type 2 diabetes. Diabet Med 2002;19:201-11. http://dx.doi.org/10.1046/j.1464-5491.2002.00643.x.
- Thomason MJ, Colhoun HM, Livingstone SJ, Mackness MI, Betteridge DJ, Durrington PN, et al. Baseline characteristics in the Collaborative AtoRvastatin Diabetes Study (CARDS) in patients with type 2 diabetes. Diabetic Med 2004;21:901-5. http://dx.doi.org/10.1111/j.1464-5491.2004.01401.x.
- Colhoun HM, Betteridge DJ, Durrington PN, Hitman GA, Neil HA, Livingstone SJ, et al. Primary prevention of cardiovascular disease with atorvastatin in type 2 diabetes in the Collaborative AtoRvastatin Diabetes Study (CARDS): multicentre randomised placebo-controlled trial. Lancet 2004;364:685-96. http://dx.doi.org/10.1016/S0140-6736(04)16895-5.
- Escobedo J, Rana JS, Lombardero MS, Albert SG, Davis AM, Kennedy FP, et al. Association between albuminuria and duration of diabetes and myocardial dysfunction and peripheral arterial disease among patients with stable coronary artery disease in the BARI 2D study. Mayo Clin Proc 2010;85:41-6. http://dx.doi.org/10.4065/mcp.2009.0265.
- Park JY, Kim HK, Chung YE, Kim SW, Hong SK, Lee KU. Incidence and determinants of microalbuminuria in Koreans with type 2 diabetes. Diabetes Care 1998;21:530-4. http://dx.doi.org/10.2337/diacare.21.4.530.
- Mount Hood 4 Modeling Group . Computer modeling of diabetes and its complications: a report on the Fourth Mount Hood Challenge Meeting. Diabetes Care 2007;30:1638-46.
- American Diabetes Association . Guidelines for computer modeling of diabetes and its complications. Diabetes Care 2004;27:2262-5.
- Wu SY, Sainfort F, Tomar RH, Tollios JL, Fryback DG, Klein R, et al. Development and application of a model to estimate the impact of type 1 diabetes on health-related quality of life. Diabetes Care 1998;21:725-31. http://dx.doi.org/10.2337/diacare.21.5.725.
- Meltzer D, Egleston B, Stoffel D, Dasbach E. Effect of future costs on cost-effectiveness of medical interventions among young adults: the example of intensive therapy for type 1 diabetes mellitus. Med Care 2000;38:679-85. http://dx.doi.org/10.1097/00005650-200006000-00009.
- Tomar RH, Lee S, Wu SY, Klein R, Klein BE, Moss SE, et al. Disease progression and cost of insulin dependent diabetes mellitus: development and application of a simulation model. J Soc Health Systems 1998;5:24-37.
- Palmer AJ, Roze S, Valentine WJ, Smith I, Wittrup-Jensen KU. Cost-effectiveness of detemir-based basal/bolus therapy versus NPH-based basal/bolus therapy for type 1 diabetes in a UK setting: an economic analysis based on meta-analysis results of four clinical trials. Curr Med Res Opin 2004;20:1729-46. http://dx.doi.org/10.1185/030079904X5661.
- Sheridan S, Pignone M, Mulrow C. Framingham-based tools to calculate the global risk of coronary heart disease – a systematic review of tools for clinicians. J Gen Intern Med 2003;18:1039-52. http://dx.doi.org/10.1111/j.1525-1497.2003.30107.x.
- Stevens RJ, Kothari V, Adler AI, Stratton IM, Holman RR. The UKPDS risk engine: a model for the risk of coronary heart disease in type II diabetes (UKPDS 56). Clin Sci 2001;101:671-9. http://dx.doi.org/10.1042/CS20000335.
- Zgibor JC, Piatt GA, Ruppert K, Orchard TJ, Roberts MS. Deficiencies of cardiovascular risk prediction models for type 1 diabetes. Diabetes Care 2006;29:1860-5.
- Oke J, Stevens RJ, Perera R, Dunger D, Dalton N, Neil A, et al. Current and alternative programmes for monitoring renal function in type 1 diabetes: modelling study based on the Oxford Regional Prospective Study. Prim Health Care Res Dev 2010;11.
- Hayes AJ, Leal J, Kelman CW, Clarke PM. Risk equations to predict life expectancy of people with type 2 diabetes mellitus following major complications: a study from Western Australia. Diabet Med 2011;28:428-35. http://dx.doi.org/10.1111/j.1464-5491.2010.03189.x.
- Finne P, Reunanen A, Stenman S, Groop PH, Gronhagen-Riska C. Incidence of end-stage renal disease in patients with type 1 diabetes. JAMA 2005;294:1782-7. http://dx.doi.org/10.1001/jama.294.14.1782.
- Zoccali C, Mallamaci F, Parlongo S, Cutrupi S, Benedetto FA, Tripepi G, et al. Plasma norepinephrine predicts survival and incident cardiovascular events in patients with end-stage renal disease. Circulation 2002;105:1354-9. http://dx.doi.org/10.1161/hc1102.105261.
- Kelly PK, Clarke PM, Hayes AJ, Gerdtham UG, Nilsson PM, Eliasson B, et al. Predicting the mortality of people with type 2 diabetes mellitus following a major complication: a study using Swedish Nation Diabetes Register Data. Diabetologia 2011;54:S1-542.
- Lung TW, Hayes AJ, Hayen A, Farmer A, Clarke PM. A meta-analysis of health state valuations for people with diabetes: explaining the variation across methods and implications for economic evaluation. Qual Life Res 2011;20:1669-78. http://dx.doi.org/10.1007/s11136-011-9902-y.
- Vital Statistics of the United States, 1991: Life Tables. Hyattsville, MD: Center for Disease Control and Prevention; 1995.
- Tierney JF, Stewart LA, Ghersi D, Burdett S, Sydes MR. Practical methods for incorporating summary time-to-event data into meta-analysis. Trials 2007;8. http://dx.doi.org/10.1186/1745-6215-8-16.
- Keech A, Simes RJ, Barter P, Best J, Scott R, Taskinen MR, et al. Effects of long-term fenofibrate therapy on cardiovascular events in 9795 people with type 2 diabetes mellitus (the FIELD study): randomised controlled trial. Lancet 2006;368.
- Olshansky SJ, Carnes BA. Limits on lifetimes: ever since Gompertz. Demography 1997;34:1-15.
- Soedamah-Muthu SS, Fuller JH, Mulnier HE, Raleigh VS, Lawrenson RA, Colhoun HM. All-cause mortality rates in patients with type 1 diabetes mellitus compared with a non-diabetic population from the UK general practice research database, 1992–1999. Diabetologia 2006;49:660-6. http://dx.doi.org/10.1007/s00125-005-0120-4.
- Office for National Statistics . 2009 Population Estimates: Quinary Age Groups for Primary Care Organisations in England; Estimated Resident Population 2011. http://www.statistics.gov.uk (accessed 9 October 2011).
- Podar T, Solntsev A, Reunanen A, Urbonaite B, Zalinkevicius R, Karvonen M, et al. Mortality in patients with childhood-onset type 1 diabetes in Finland, Estonia, and Lithuania – follow-up of nationwide cohorts. Diabetes Care 2000;23:290-4.
- McNally PC, Raymond NT, Burden ML, Burton PR, Botha JL, Swift PGF, et al. Trends in mortality of childhood-onset insulin-dependent diabetes-mellitus in Leicestershire 1940–1991. Diabet Med 1995;12:961-6. http://dx.doi.org/10.1111/j.1464-5491.1995.tb00406.x.
- Nishimura R, LaPorte RE, Dorman JS, Tajima N, Becker D, Orchard TJ. Mortality trends in type 1 diabetes – the Allegheny County (Pennsylvania) Registry 1965–1999. Diabetes Care 2001;24:823-7. http://dx.doi.org/10.2337/diacare.24.5.823.
- Laing SP, Swerdlow AJ, Slater SD, Burden AC, Morris A, Waugh NR, et al. Mortality from heart disease in a cohort of 23,000 patients with insulin-treated diabetes. Diabetologia 2003;46:760-5. http://dx.doi.org/10.1007/s00125-003-1116-6.
- Secrest AM, Becker DJ, Kelsey SF, LaPorte RE, Orchard TJ. All-cause mortality trends in a large population-based cohort with long-standing childhood-onset type 1 diabetes: the Allegheny County Type 1 Diabetes Registry. Diabetes Care 2010;33:2573-9. http://dx.doi.org/10.2337/dc10-1170.
- Cederholm J, Eeg-Olofsson K, Eliasson B, Zethelius B, Gudbjornsdottir S. Swedish National Diabetes Register . A new model for 5-year risk of cardiovascular disease in type 1 diabetes; from the Swedish National Diabetes Register (NDR). Diabet Med 2011;28:1213-20. http://dx.doi.org/10.1111/j.1464-5491.2011.03342.x.
- Zgibor JC, Ruppert K, Orchard TJ, Soedamah-Muthu SS, Fuller J, Chaturvedi N, et al. Development of a coronary heart disease risk prediction model for type 1 diabetes: the Pittsburgh CHD in type 1 diabetes risk model. Diabetes Res Clin Prac 2010;88:314-21. http://dx.doi.org/10.1016/j.diabres.2010.02.009.
- Holman RR, Paul SK, Bethel MA, Matthews DR, Neil HA. 10-year follow-up of intensive glucose control in type 2 diabetes. N Engl J Med 2008;359:1577-89. http://dx.doi.org/10.1056/NEJMoa0806470.
- Garg AX, Kiberd BA, Clark WF, Haynes RB, Clase CM. Albuminuria and renal insufficiency prevalence guides population screening: results from the NHANES III. Kidney Int 2002;61:2165-75. http://dx.doi.org/10.1046/j.1523-1755.2002.00356.x.
- The Green Book: Appraisal and Evaluation in Central Government. London: Stationery Office; 2011.
- Chronic kidney disease costing report: implementing NICE guidance. London: National Institute for Health and Care Excellence; 2008.
- Curtis L. Unit costs of health and social care 2010. Canterbury: Personal Social Services Research Unit, University of Kent; 2010.
- Prescription cost analysis England 2010. London: Department of Health; 2010.
- Clarke P, Gray A, Legood R, Briggs A, Holman R. The impact of diabetes-related complications on healthcare costs: results from the United Kingdom Prospective Diabetes Study (UKPDS Study No. 65). Diabet Med 2003;20:442-50. http://dx.doi.org/10.1046/j.1464-5491.2003.00972.x.
- Klebe B, Irving J, Stevens PE, O’Donoghue DJ, de Lusignan S, Cooley R, et al. The cost of implementing UK guidelines for the management of chronic kidney disease. Nephrol Dial Transplant 2007;22:2504-12. http://dx.doi.org/10.1093/ndt/gfm248.
- Chronic kidney disease in adults: UK guidelines for identification, management and referral. Leeds: National Institute for Health and Care Excellence; 2011.
- Fenwick E, Marshall DA, Levy AR, Nichol G. Using and interpreting cost-effectiveness acceptability curves: an example using data from a trial of management strategies for atrial fibrillation. BMC Health Serv Res 2006;6.
- Wright JC, Weinstein MC. Gains in life expectancy from medical interventions – standardizing data on outcomes. N Engl J Med 1998;339:380-6. http://dx.doi.org/10.1056/NEJM199808063390606.
- Clarke PM, Gray AM, Briggs A, Stevens RJ, Matthews DR, Holman RR. Cost–utility analyses of intensive blood glucose and tight blood pressure control in type 2 diabetes (UKPDS 72). Diabetologia 2005;48:868-77. http://dx.doi.org/10.1007/s00125-005-1717-3.
- Glasziou PP, Clarke P, Alexander J, Rajmokan M, Beller E, Woodward M, et al. Cost-effectiveness of lowering blood pressure with a fixed combination of perindopril and indapamide in type 2 diabetes mellitus: an ADVANCE trial-based analysis. Med J Aust 2010;193:320-4.
- Yang XL, So WY, Kong AP, Clarke P, Ho CS, Lam CW, et al. End-stage renal disease risk equations for Hong Kong Chinese patients with type 2 diabetes: Hong Kong Diabetes Registry. Diabetologia 2006;49:2299-308. http://dx.doi.org/10.1007/s00125-006-0376-3.
- Bossuyt PM, Lijmer JG, Mol BW. Randomised comparisons of medical tests: sometimes invalid, not always efficient. Lancet 2000;356:1844-7. http://dx.doi.org/10.1016/S0140-6736(00)03246-3.
- American Diabetes Association Consensus Panel . Guidelines for computer modeling of diabetes and its complications. Diabetes Care 2004;27:2262-5.
- Marshall SM. Diabetic nephropathy in type 1 diabetes: has the outlook improved since the 1980s?. Diabetologia 2012;55:2301-6. http://dx.doi.org/10.1007/s00125-012-2606-1.
- Perkins BA, Nelson RG, Ostrander BE, Blouch KL, Krolewski AS, Myers BD, et al. Detection of renal function decline in patients with diabetes and normal or elevated GFR by serial measurements of serum cystatin C concentration: results of a 4-year follow-up study. J Am Soc Nephrol 2005;16:1404-12. http://dx.doi.org/10.1681/ASN.2004100854.
- Perkins BA, Krolewski AS. Early nephropathy in type 1 diabetes: a new perspective on who will and who will not progress. Curr Diab Rep 2005;5:455-63. http://dx.doi.org/10.1007/s11892-005-0055-7.
- Premaratne E, MacIsaac RJ, Finch S, Panagiotopoulos S, Ekinci E, Jerums G. Serial measurements of cystatin C are more accurate than creatinine-based methods in detecting declining renal function in type 1 diabetes. Diabetes Care 2008;31:971-3. http://dx.doi.org/10.2337/dc07-1588.
- Aung T, Cowan H, Haynes R, Bowman L, Armitage J. Recruiting patients cost-effectively by mail. Trials 2011;12. http://dx.doi.org/10.1186/1745-6215-12-S1-A117.
- Scognamiglio R, Nosadini R, Marin M, Nisti S, Fasoli G, Palisi M, et al. Evaluation of the efficacy and tolerability of nitrendipine in reducing both pressure and left ventricular mass in hypertensive type 2 diabetic patients. Diabetes Care 1997;20:1290-2. http://dx.doi.org/10.2337/diacare.20.8.1290.
- Parving H, Hommel E, Nielsen MD, Giese J. Effect of captopril on blood pressure and kidney function in normotensive insulin dependent diabetics with nephropathy. BMJ 1989;299. http://dx.doi.org/10.1136/bmj.299.6698.533.
- Capek M, Schnack C, Ludvik B, Kautzky-Willer A, Banyai M, Prager R. Effects of captopril treatment versus placebo on renal function in type 2 diabetic patients with microalbuminuria: a long-term study. Clin Investig 1994;72:961-6. http://dx.doi.org/10.1007/BF00577736.
- Tütüncü N, Gürlek A, Gedik O. Efficacy of ACE inhibitors and ATII receptor blockers in patients with microalbuminuria: a prospective study. Acta Diabetol 2001;38:157-61.
- Stornello M, Valvo E, Scapellato L. Hemodynamic, renal, and humoral effects of the calcium entry blocker nicardipine and converting enzyme inhibitor captopril in hypertensive type II diabetic patients with nephropathy. J Cardiovasc Pharmacol 1989;14:851-5. http://dx.doi.org/10.1097/00005344-198912000-00009.
- Stornello M, Valvo EV, Scapellato L. Persistent albuminuria in normotensive non-insulin-dependent (type II) diabetic patients: comparative effects of angiotensin-converting enzyme inhibitors and beta-adrenoceptor blockers. Clin Sci 1992;82:19-23.
- Velussi M, Brocco E, Frigato F, Zolli M, Muollo B, Maioli M, et al. Effects of cilazapril and amlodipine on kidney function in hypertensive NIDDM patients. Diabetes 1996;45:216-22. http://dx.doi.org/10.2337/diabetes.45.2.216.
- Rizzoni D, Porteri E, De Ciuceis C, Sleiman I, Rodella L, Rezzani R, et al. Effect of treatment with candesartan or enalapril on subcutaneous small artery structure in hypertensive patients with noninsulin-dependent diabetes mellitus. Hypertension 2005;45:659-65. http://dx.doi.org/10.1161/01.HYP.0000153308.91043.97.
- Ko GT, Tsang C, Chan HC. Stabilization and regression of albuminuria in Chinese patients with type 2 diabetes: a one-year randomized study of valsartan versus enalapril. Adv Ther 2005;22:155-62. http://dx.doi.org/10.1007/BF02849886.
- Lacourcière Y, Bélanger A, Godin C, Hallé J, Ross S, Wright N, et al. Long-term comparison of losartan and enalapril on kidney function in hypertensive type 2 diabetics with early nephropathy. Kidney Int 2000;58:762-9. http://dx.doi.org/10.1046/j.1523-1755.2000.00224.x.
- Barnett AH, Bain SC, Bouter P, Karlberg B, Madsbad S, Jervell J, et al. Angiotensin-receptor blockade versus converting-enzyme inhibition in type 2 diabetes and nephropathy. N Engl J Med 2004;351:1952-61. http://dx.doi.org/10.1056/NEJMoa042274.
- Schrier R, Estacio R, Jeffers B. Appropriate blood pressure control in NIDDM (ABCD) trial. Diabetologia 1996;39:1646-54. http://dx.doi.org/10.1007/s001250050629.
- Joglekar SJ, Nanivadekar AS. A randomized, controlled, multicenter study to compare prazosin GITS with enalapril in hypertensive patients with diabetes mellitus. Bombay Hypertension Study Group. J Assoc Physicians India 1998:52-6.
- Lin M, Yang YF, Chiang HT, Lee D, Wang SP, Chang MS, et al. Beneficial effects of angiotensin-converting enzyme inhibitors on cardiovascular and renal functions in patients with hypertension and diabetes. Acta Cardiol Sin 1995;11:30-8.
- Tatti P, Pahor M, Byington RP, Di Mauro P, Guarisco R, Strollo G, et al. Outcome results of the Fosinopril versus Amlodipine Cardiovascular Events randomized Trial (FACET) in patients with hypertension and NIDDM. Diabetes Care 1998;21:597-603. http://dx.doi.org/10.2337/diacare.21.4.597.
- Heart Outcomes Prevention Evaluation Study Investigators . Effects of ramipril on cardiovascular and microvascular outcomes in people with diabetes mellitus: results of the HOPE study and MICRO-HOPE substudy. Heart Outcomes Prevention Evaluation Study Investigators. Lancet 2000;355:253-9.
- Bakris GL, Barnhill BW, Sadler R. Treatment of arterial hypertension in diabetic humans: importance of therapeutic selection. Kidney Int 1992;41:912-19. http://dx.doi.org/10.1038/ki.1992.139.
- Marre M, Leblanc H, Suarez L, Guyenne TT, Menard J, Passa P. Converting enzyme inhibition and kidney function in normotensive diabetic patients with persistent microalbuminuria. Br Med J 1987;294. http://dx.doi.org/10.1136/bmj.294.6585.1448.
- Nankervis A, Nicholls K, Kilmartin G, Allen P, Ratnaike S, Martin F. Effects of perindopril on renal histomorphometry in diabetic subjects with microalbuminuria: a 3-year placebo-controlled biopsy study. Metab Clin Exp 1998;47:12-5. http://dx.doi.org/10.1016/S0026-0495(98)90364-X.
- O’Donnell MJ, Rowe BR, Lawson N, Horton A, Gyde OH, Barnett AH. Placebo-controlled trial of lisinopril in normotensive diabetic patients with incipient nephropathy. J Hum Hypertens 1993;7:327-32.
- Phillips PJ, Phillipou G, Bowen KM, Lowe J, Yue DK, Wischusen J, et al. Diabetic microalbuminuria and cilazapril. Am J Med 1993;94:58S-60S.
- Sato A, Tabata M, Hayashi K, Saruta T. Effects of the angiotensin II type 1 receptor antagonist candesartan, compared with angiotensin-converting enzyme inhibitors, on the urinary excretion of albumin and type IV collagen in patients with diabetic nephropathy. J Clin Experi Nephrol 2003;7:215-20. http://dx.doi.org/10.1007/s10157-003-0227-1.
- Maschio G, Alberti D, Janin G, Locatelli F, Mann JF, Motolese M, et al. Effect of the angiotensin-converting-enzyme inhibitor benazepril on the progression of chronic renal insufficiency. The Angiotensin-Converting-Enzyme Inhibition in Progressive Renal Insufficiency Study Group. N Engl J Med 1996;334:939-45.
- Diabetes Control and Complications Trial Research Group . Effect of intensive therapy on the development and progression of diabetic nephropathy in the Diabetes Control and Complications Trial. The Diabetes Control and Complications Trial (DCCT) Research Group. Kidney Int 1995;47:1703-20.
Appendix 1 Original protocol and modifications
This monograph was commissioned by the NIHR Health Technology Programme to provide an evidence base for decisions about the optimal interval for testing for onset of diabetic kidney disease. During the course of the work a number of minor amendments or modifications to this proposal were proposed and agreed by the steering group.
Minor amendments and modifications included:
-
Of the literature reviews proposed in the original grant application, one, on the properties of test characteristics, was superseded by the availability of patient-level data through a cohort study for which we obtained separate funding: we undertook the work looking at qualitative methods of detecting microalbuminuria in a parallel project in which two standard stick tests for albumin were compared with laboratory albumin-creatinine testing. The protocol for the full systematic review and other study components follow.
-
Our eventual selection of datasets with which to model progression of microalbuminuria and estimated glomerular filtration rate was, in the event, dictated by the very limited number of studies in which the relevant data had been collected at frequent enough intervals and could be made available for analysis. The two data sets included were drawn from those initially proposed, but we could not obtain further data sets for validation. The data sets were not sufficiently large for us to be able to examine predictors of progression in the individual data sets. We therefore relied on estimates derived from the literature to inform the sensitivity analyses carried out on our models of progression.
-
We used the methods for modelling progression of monitoring parameters specified in the original grant proposal, except that the ‘variogram’ estimation method has been superseded by a random effects modelling method; we therefore used the more recent method45
-
In our original application, the role of monitoring estimated glomerular filtration rate was given emphasis alongside the role of monitoring the urine albumin-to-creatinine ratio. As our work progressed it became clear that this was of less importance in clinical practice from the perspective of initial detection of impaired renal function (see Chapters 3 and 4 for details).
-
In response to the comments of the Commissioning Board we agreed that we would try to model patient relevant outcomes. We were able to do this through development of a type 1 simulation model with newly available data, and adapt the UKPDS Health Economic Model using the additional data obtained in this study.
We therefore undertook the following work:
-
Prepared a narrative review of the epidemiology of diabetic renal disease, treatment and monitoring as an introductory chapter
-
Systematic reviews
-
– Impact of ACEi on outcomes
-
– Dose and outcomes in type 2 diabetes (published separately)
-
-
Limited systematic reviews
-
Currently available models for examining impact of varying intervals for monitoring
-
Outcomes (progression) from other studies of type 2 diabetes and rates of progression of albuminuria
-
Factors affecting progression of albuminuria
-
Factors affecting impact of treatments for microalbuminuria
-
-
Modelling of progression of measures of renal function.
-
– Type 1 from ORPS – ACR
-
– Type 2 from CARDS – ACR and eGFR
-
-
Health economic outcomes simulation models
-
– Type 1 model constructed from newly available data
-
– Type 1 model used to evaluate intervals for monitoring
-
– Existing type 2 model adapted and used to evaluate intervals for monitoring
-
This monograph presents this work and discusses the implications of these findings for the work
Systematic review protocol: Impact of antihypertensive agents on outcomes of diabetic patients with and without kidney disease
Aims and objectives
Aim
To evaluate the benefits and harms of ACE inhibitors and ARB in comparison with placebo, other antihypertensive agents or no treatment in patients with diabetes with and without kidney disease. The review will focus on impact on overall mortality, renal and cardiovascular outcomes.
Objectives
To evaluate the effects of interventions according to:
-
Impact of ACEi and ARB in comparison to placebo, other antihypertensive agents or no treatment on mortality, cardiovascular events and progression of renal disease in type 1 and type 2 diabetes
-
Interaction of interventions above with type of diabetes, age, gender, stage of renal disease, ethnicity, type of treatment (ACEi, ARB and other antihypertensive agent) and stage of renal disease on mortality, cardiovascular events and progression of renal disease
Methods
Criteria for considering studies for this review
Types of studies will include randomised control trials (RCTs) of at least 6 months duration in which ACEi or ARB will be compared with placebo, other antihypertensive treatment or no treatment. We will only include studies in which there is specific data available for the outcomes by type of diabetes and by renal status defined by presence or absence of albuminuria.
Types of participants will include type 1 or type 2 diabetes patients with or without kidney disease.
Types of interventions (trial designs) included will be (i) ACEi or ARB versus placebo; (ii) ACEi or ARB versus no treatment; (iii) ACEi or ARB versus other antihypertensive agents; (iv) ACE vs. ARB; and (v) ACE vs. ACE/ARB at different doses.
Trial exclusion criteria are trials where no results are separately presented for type 1 or type 2 diabetes; trials where urinary albumin status is not specified or results are not separately presented by urinary albumin status; and trials in which there is no randomised comparison of ACE/ARB with either a placebo, no treatment or another antihypertensive agent.
Variables used to define subgroups for analysis and/or moderators of effect
-
Type of diabetes
-
Gender
-
Ethnicity
-
eGFR > 90, 60 to 90, 30 to 60, 15 to 30, < 15
-
Albumin excretion (macro-, > 300 mg/24 h, micro-, 30–300 mg/24 h, normo-, < 30 mg/24 h)
-
Is the trial designed to match blood pressure control between groups?
Types of outcome measures
-
Death (any cause)
-
Death (cardiovascular)
-
Non-fatal CVD (MI, stroke)
-
End-stage renal disease (defined as dialysis or transplant)
-
Doubling of creatinine
-
Number of patients who develop microalbuminuria (i.e. progression from normo-to microalbuminuria)
-
Progression from micro- to macroalbuminuria
-
Regression from macro- to microalbuminuria
-
Regression to normoalbuminuria
-
Urinary albumin/creatinine ratio
(*Dichotomous or categorical variable)
Search methods for identification of studies
Searches of the following electronic biomedical databases will be conducted to identify relevant trials:
-
The Cochrane Library (Cochrane Central Register of Controlled Trials, Database of Systematic Reviews and Database of Abstracts and Reviews of Effectiveness),
-
MEDLINE (1950–2009)
-
EMBASE (1980–2009)
Hand searches of cross -references from original articles, reviews and conference abstracts.
The overall search strategy will combine searches for type 1 and type 2 diabetes, antihypertensive agents and kidney disease with randomised controlled trials.
Data Collection and Analysis
The search strategy will be performed to obtain lists of titles and abstracts of studies that may be relevant to the review. The title and abstracts will be screened independently by two reviewers to discard studies that do not meet the inclusion criteria. Studies and reviews that might include relevant data or information will be retained initially. Where necessary the full text of articles will be obtained to determine which studies satisfied the inclusion criteria. Data extraction will be carried out independently using standard data extraction forms. Studies reported in non-English language journals will be translated. Where more than one publication of one trial exists, only the publication with the most complete data will be included. Any further information required from the original author will be requested by written correspondence. If any additional information is acquired in this manner it will be included in the review.
Study quality
The quality of studies to be included will be assessed by allocation concealment, intention-to-treat analysis, blinding of investigators, participants and outcome assessors and completeness of follow-up.
Quality checklist
Sequence generation and allocation concealment:
-
Adequate: Randomisation method described that would not allow investigator/participant to know or influence intervention group before eligible participant entered in the study.
-
Unclear: Randomisation stated but no information on method used is available.
-
Inadequate: Method of randomisation used such as alternate medical record numbers or unsealed envelopes: any information in the study that indicated that investigators or participants could influence intervention group.
Blinding and allocation concealment (Cochrane handbook):
-
Blinding of investigators: Yes/no/not stated
-
Blinding of participants: Yes/no/not stated
-
Blinding of outcome assessor: Yes/no/not stated
-
Blinding of data analysis: Yes/no/not stated
Risk of incomplete outcome data:
-
Low risk: participants included in the analysis are exactly those who were randomised into the trial, missing data is clearly reported to be an acceptable reason or if outcome data is missing in both intervention groups, but reasons for these are both reported and balanced across groups.
-
Unclear risk: the numbers randomised into each intervention group or the reasons for missing data are not clearly reported.
-
High risk: There is a difference in the proportion of incomplete outcome data across groups, or if incomplete outcome data is balanced in numbers across groups, but the reasons for missing outcomes differ.
Completeness of follow-up:
-
Per cent of patients excluded or lost to follow-up.
Statistical assessment
Data will be pooled for outcome, biochemical and safety data for the six categories of type 1 and type 2 diabetes, and normo, micro and macroalbuminuria. Comparisons will be (in order), ACE/ARB vs. placebo or no treatment; ACE vs. other antihypertensive agent.
A separate comparison of ACE vs. ARB and ACE vs. ACE of a different type or dose will be carried out.
The effect of ACE, ARB or other antihypertensive agents will be summarised using risk ratios with 95% CIs for mortality or morbidity data.
Continuous data will be pooled where possible using mean, standard deviation and sample size per group. If unavailable then percentage change will be pooled with a random effects model, or if possible data will be dichotomised.
Data comparing the same intervention will be pooled in a meta-analysis. Heterogeneity of treatment effects between studies will be examined using the i-squared statistic.
Sub-group analysis (baseline albumin, ethnicity, baseline blood pressure if available.
If sufficient data is available we will use metaregression techniques to account for heterogeneity.
Modelling
Data will be provided in the form of risk ratios for differing levels of renal function, and where possible, other modifiers of treatment will be identified, to inform the estimates used in the decision modelling for optimal albumin testing.
Individual patient data modelling protocol
Research aims and objectives
Our aim is to develop a clinical and economic model of renal function decline, and then to estimate the effectiveness and cost-effectiveness of different models for chronic kidney disease (CKD) monitoring in type 1 and 2 diabetes.
Research methods
Design
We will model eGFR and microalbumin measurements to (a) identify biological variability and measurement error and determine coefficients of variation and (b) rates of change using individual patient data cohorts of both type 1 and type 2 diabetes patients. This will allow us to model true- and false-positive rates of testing at different intervals and in different patient groups, for reach specific treatment-decision thresholds.
Individual patient datasets
We will use individual patient data sets to build a model of rates progression of chronic kidney disease by predictors in diabetes, provide data on the microalbumin screening test for CKD thresholds, and monitoring data for the modelling of false- and true-positive rates of the eGFR at different thresholds. In addition we intend to explore their potential for providing quantitative data about the change in albumin excretion in response to treatment.
Identified data sets
We have provisional agreement from the investigators for collaboration and sharing of anonymised data from the following large, long-term population based studies: ADVANCE, FIELD, CARDS, ORPS and EUCLID. In addition, we also have agreement for collaboration from the 1,000-patient Family Nephropathy study. These include trials encompassing type 1 and type 2 diabetes with sufficient patient numbers, patients not taking ACE or AR2B at cohort inception to allow sufficient patients for analysis. These studies have included detailed base-line characterisation of patients, collected serial data about treatment and disease characteristics, and have periodically measured renal function, including urinary albumin : creatinine ratios and serum creatinine.
Trial | Type of diabetes | Age (y) at cohort inception | Proportion on ACE/AR2RB at baseline† | Period of follow up (years) | Trial intervention | Frequency of measurement | |
---|---|---|---|---|---|---|---|
Repeated albumin measurements | Repeated creatinine measurements | ||||||
ADVANCE | Type 2 diabetes | 66 | 48% | 4.3 years | Blood pressure and glycaemic control | Two-yearly (urine alb/cr) | Yearly |
FIELD | Type 2 diabetes | 62 | 40% | 5 years | Fenofibrate | Two-yearly (urine alb/cr) | Yearly |
CARDS | Type 2 diabetes | 62 | 44% | 4.5 years | Atorvastatin | Yearly (urine alb/cr) | Yearly |
ORPS | Type 1 diabetes | 9 | < 1% | 22 years | Observational | Yearly | Yearly |
EUCLID | Type 1 diabetes | 33 | 0% | 2 years | Lisinopril | 6 monthly | 6 monthly |
The patient cohorts used for this analysis will be chosen to maximise the available data. If feasible we will use a second cohort for both type 1 and type 2 diabetes to validate the findings from the first cohort. The three type 2 diabetes trials have 4 to 5 year follow up of cohorts of between 3,000 to 11,000 patients. The ORPS17 data set is an inception cohort of 22 years duration with measurements over a protracted period, and will therefore provide high quality data relevant to the majority of the type 1 population.
Comparison of published estimates and data from individual patent datasets
Predictors of progression and outcome identified from the review of the literature will, where possible, be examined in the individual patient datasets. Predictive equations will be applied to the available data for each individual within the dataset. We will omit variables from the predictive scores where unavailable, use imputation where possible for missing data and adjust for the anticipated effects of treatments using estimates from systematic reviews.
Determining rates of progression and biological variability and measurement error
Using individual patient data cohorts with both type 1 and type 2 diabetes patients we will model long-term GFR and microalbumin measurement changes and errors. We will use this model to determine the impact of different monitoring intervals on the false- and true-positive rates for renal changes, the onset of microalbuminuria and clinically important change in eGFR. This modelling is based on estimating three elements (i) within-person variability, (ii) the average rate of progression of renal impairment and (iii) the variation in this average rate.
Based on this model we will estimate the utility of repeated measurements of urinary albumin to monitor intensification of treatment and of using creatinine rather than eGFR to monitor renal function. The model will include treatment effects as well as variability due to intercurrent infection and short term use of medication. We will base our model on a single data-set for type 1 and type 2 diabetes, using the most detailed, largest and longest data set.
We will check the assumptions of the model and in particular determine whether the rate of progression of the monitored variables is linear or non-linear and transform the model if needed. Previous work concluded that progression of albuminuria was non-linear. The average change in eGFR and microalbumin : creatinine ratio can be estimated from the group averages at the available time-points. We will examine the data to establish the optimal time-point for starting measurement, since the course of measures from baseline may be initially affected by trial entry and initial therapy. Short-term variability will be assessed by a ‘variogram’: a linear extrapolation backwards from the long-term measures to estimate variance at time 0. In addition we will use the above data in a mixed-longitudinal model where we assume that the rate of increase varies between individuals. Bland-Altman plots will be used to test for variability between and within persons at differing levels of initial eGFR and microalbumin excretion levels. We will also extend this analysis to a non-linear approach.
We will use the above data to model true- and false-positive rates of tests at different intervals and in different patient groups for monitoring for microalbuminuria and for identifying progression of eGFR to levels at which referral might be indicated. We will therefore identify true and false-positive rates of attainment under specific treatment-decision thresholds.
Health economics outcomes models protocol
Research methods
Design
We will use data about predictors of outcome, test characteristics, and baseline patient characteristics together with diagnostic and treatment costs, to build and populate an individual sampling model to identify cost per additional true-positive test for different frequencies of monitoring at different thresholds in different groups of patients. The results of this study will inform clinical practice and lead to evidence based recommendations for intervals for eGFR and microalbumin testing in patients with diabetes.
Modelling of cost-and effectiveness
We will develop an individual sampling model including a ‘trend’ module, a ‘variability’ module and a ‘costs’ module. The analysis algorithm will draw on these modules to estimate both true and false-positive rates, and cost of any given screening strategy. The trend module for GFR, creatinine and urine albumin will be based on the results of the work on rate of progression; and the variability module on the results of the variability of each of the measures. We will populate the model with a baseline data-set typical of the general diabetes population. The costs module will be developed from previous trial results, literature and NHS sources. This modular approach will allow flexibility depending on the extent of the data that we are able to draw from each of the individual patient data-sets.
The model will allow us to identify, for each of (a) urinary albumin : creatinine ratio and (b) eGFR and (c) hybrid strategies, a shortlist of monitoring strategies. These strategies will include more effective schemes with similar costs, lower cost schemes with similar effectiveness and strategies that improve on both cost and cost-effectiveness. The key decision points will be (i) identification of diabetic nephropathy through microalbuminuria screening with initiation of ACE therapy and intensification of blood pressure targets, and (ii) progression of disease through stages of CKD. Impact of potential modifiers of progression including blood pressure treatment and patient characteristics (including ethnicity) will be incorporated. The model will handle competing risks (e.g. death rates at differing levels of nephropathy), and if necessary we will also address competing risks in the trend models.
The model will use cost data derived from consultations with NHS providers. In addition we will use standard PSSRU reference costs for health care interventions. We will use an NHS perspective for the analysis. Total costs will be discounted at 3.5%.
Development of the individual simulation model will be supervised by PC and RS using similar processes to those used in development of the UKPDS Outcomes Model and the UKPDS Risk Engine. Programming will be carried out in the statistical modelling language R, using a modular design as described above. The American Diabetes Association guidelines on computer modelling will be followed wherever relevant: in particular, we will use internal validation to verify the accuracy of the program and cross-validation to estimate its external validity. We will handle both Monte-Carlo uncertainty (induced by simulation and handled by averaging over multiple runs) and statistical uncertainty (represented as CIs, calculated by a parametric bootstrap method). Other areas of uncertainty will be explored with a sensitivity analysis.
Different testing and screening policies will have differing associated costs, both in terms of the testing, but also in terms of associated interventions (e.g. commencement and intensification of therapy). There are also potential harms, such as incidence of hypoglycaemia with intensified glycaemic control. Costs and benefits will be formally assessed by modelling the cost-effectiveness of different screening and testing strategies. The initial focus of the analysis will be to examine cost-effectiveness of alternative screening policies using cost per true positive (incremental cost per additional true positive detected compared to the existing monitoring programme). We will also consider number of false negatives per false positive as a secondary measure of cost-effectiveness. We will also examine the feasibility of extending this analysis to final outcome measure such as a Quality Adjusted Life Expectancy, which would form a secondary analysis if there is sufficient evidence to extend the model to capture this long-term outcome.
We will also explore the extent to which the uncertainties in the model might be informed by further research, and the extent to which the likely costs of this research would reduce the uncertainty to a level where additional benefit to patients and the NHS might be obtained.
Appendix 2 Summary of searches used for systematic reviews (Chapter 2)
MEDLINE
MEDLINE search terms
-
exp antihypertensive agents/
-
(antihypertensive$ adj (agent$ or drug)).tw.
-
chlorothiazide.tw.
-
chlorthalidone.tw.
-
hydralazine.tw.
-
hydrochlorothiazide.tw.
-
indapamide.tw.
-
minoxidil.tw.
-
exp angiotensin converting enzyme inhibitors/
-
captopril.tw.
-
enalapril.tw.
-
cilazapril.tw.
-
enalaprilat.tw.
-
fosinopril.tw.
-
lisinopril.tw.
-
perindopril.tw.
-
ramipril.tw.
-
saralasin.tw.
-
teprotide.tw.
-
exp losartan/
-
losartan.tw.
-
imidazole$.tw.
-
irbesartan.tw.
-
candesartan.tw.
-
eprosartan.tw.
-
valsartan.tw.
-
olmesartan.tw.
-
telmisartan.tw.
-
(ace adj2 inhibitor$).tw.
-
(angiotensin adj2 receptor antagonist$).tw.
-
exp calcium channel blockers/
-
amlodipine.tw.
-
diltiazem.tw.
-
felodipine.tw.
-
nicardipine.tw.
-
nifedipine.tw.
-
nimodipine.tw.
-
nisoldipine.tw.
-
nitrendipine.tw.
-
verapamil.tw.
-
exp adrenergic beta-antagonists/
-
alprenolol.tw.
-
atenolol.tw.
-
metoprolol.tw.
-
nadolol.tw.
-
oxprenolol.tw.
-
pindolol.tw.
-
propranolol.tw.
-
exp adrenergic alpha-antagonists/
-
labetalol.tw.
-
prazosin.tw.
-
beta block$.tw.
-
exp diuretics/
-
spironolactone.tw.
-
triamterene.tw.
-
bumetanide.tw.
-
chlorthalidone.tw.
-
furosemide.tw.
-
indapamide.tw.
-
chlorothiazide.tw.
-
hydrochlorothiazide.tw.
-
or/1-61
-
exp diabetes mellitus/
-
diabetic nephropathies/
-
diabetic nephropath$.tw.
-
diabetic glomerulo$.tw.
-
((diabetic or diabetes) and (kidney disease$ or renal disease$)).tw.
-
or/63-67
-
62 and 68
-
randomized controlled trial.pt.
-
controlled clinical trial.pt.
-
randomized.ab.
-
placebo.ab.
-
clinical trials as topic.sh.
-
randomly.ab.
-
trial.ti.
-
74 or 75 or 72 or 71 or 70 or 73 or 76
-
exp animals/ not humans.sh.
-
77 not 78
-
69 and 79
-
limit 80 to yr="2005 -Current"
EMBASE search terms
-
exp antihypertensive agents
-
(antihypertensive$ adj (agent$ or drug$)).tw.
-
(anti-hypertensive$ adj (agent$ or drug$)).tw.
-
chlorothiazide.tw.
-
chlorthalidone.tw.
-
hydralazine.tw.
-
hydrochlorothiazide.tw.
-
indapamide.tw.
-
minoxidil.tw.
-
losartan.tw.
-
imidazole$.tw.
-
irbesartan.tw.
-
candesartan.tw.
-
eprosartan.tw.
-
valsartan.tw.
-
olmesartan.tw.
-
telmisartan.tw.
-
exp angiotensin converting enzyme inhibitors
-
(ace adj2 inhibitor$).tw.
-
(acei or ace-i).tw.
-
captopril.tw.
-
enalapril.tw.
-
fosinopril.tw.
-
lisinopril.tw.
-
perindopril.tw.
-
ramipril.tw.
-
saralasin.tw.
-
teprotide.tw.
-
exp Angiotensin 2 Receptor Antagonist
-
exp Angiotensin Receptor Antagonist
-
Angiotensin II Antagonist
-
angiotensin II receptor antagonist$.tw.
-
angiotensin 2 receptor antagonist$.tw.
-
angiotensin II receptor block$.tw.
-
angiotensin 2 receptor block$.tw.
-
AT 2 receptor block$.tw.
-
AT 2 receptor antagon$.tw.
-
angiotensin receptor antagonist$.tw.
-
exp Calcium Channel Blockers/
-
amlodipine.tw.
-
diltiazem.tw.
-
felodipine.tw.
-
nicardipine.tw.
-
nifedipine.tw.
-
nimodipine.tw.
-
nisoldipine.tw.
-
nitrendipine.tw.
-
verapamil.tw.
-
exp adrenergic beta-antagonists/
-
alprenolol.tw.
-
atenolol.tw.
-
metoprolol.tw.
-
nadolol.tw.
-
oxprenolol.tw.
-
pindolol.tw.
-
propranolol.tw.
-
exp adrenergic alpha-antagonists/
-
labetalol.tw.
-
prazosin.tw.
-
beta block$.tw.
-
exp diuretics/
-
spironolactone.tw.
-
triamterene.tw.
-
bumetanide.tw.
-
furosemide.tw.
-
indapamide.tw.
-
or/1-66
-
exp Diabetes Mellitus/
-
diabetes mellitus.tw.
-
(IDDM or NIDDM).tw.
-
Diabetic Nephropathies/
-
diabetic nephrop$.tw.
-
diabetic glomerulo$.tw.
-
((diabetic or diabetes) and (kidney disease$ or renal disease$ or nephro$ or nephrit$ or glomerulo$)).tw.
-
or/68-74
-
and/67,75
-
random$.mp. [mp=title, abstract, subject headings, heading word, drug trade name, original title, device manufacturer, drug manufacturer, device trade name, keyword]
-
factorial$.mp. [mp=title, abstract, subject headings, heading word, drug trade name, original title, device manufacturer, drug manufacturer, device trade name, keyword]
-
crossover$.mp. [mp=title, abstract, subject headings, heading word, drug trade name, original title, device manufacturer, drug manufacturer, device trade name, keyword]
-
cross over$.mp. [mp=title, abstract, subject headings, heading word, drug trade name, original title, device manufacturer, drug manufacturer, device trade name, keyword]
-
cross-over$.mp. [mp=title, abstract, subject headings, heading word, drug trade name, original title, device manufacturer, drug manufacturer, device trade name, keyword]
-
placebo$.mp. [mp=title, abstract, subject headings, heading word, drug trade name, original title, device manufacturer, drug manufacturer, device trade name, keyword]
-
(doubl$ adj blind$).mp. [mp=title, abstract, subject headings, heading word, drug trade name, original title, device manufacturer, drug manufacturer, device trade name, keyword]
-
(singl$ adj blind$).mp. [mp=title, abstract, subject headings, heading word, drug trade name, original title, device manufacturer, drug manufacturer, device trade name, keyword]
-
assign$.mp. [mp=title, abstract, subject headings, heading word, drug trade name, original title, device manufacturer, drug manufacturer, device trade name, keyword]
-
allocat$.mp. [mp=title, abstract, subject headings, heading word, drug trade name, original title, device manufacturer, drug manufacturer, device trade name, keyword]
-
volunteer$.mp. [mp=title, abstract, subject headings, heading word, drug trade name, original title, device manufacturer, drug manufacturer, device trade name, keyword]
-
84 or 85 or 83 or 80 or 78 or 79 or 81 or 87 or 77 or 86 or 82
-
88 and 76
The Cochrane Library search terms
#1 (ANTIHYPERTENSIVE AGENTS explode all trees (MeSH))
#2 chlorothiazide
#3 chlorthalidone
#4 hydralazine
#5 hydrochlorothiazide
#6 indapamide
#7 minoxidil
#8 (#1 OR #2 OR #3 OR #4 OR #5 OR #6 OR #7)
#9 captopril
#10 enalpril
#11 cilazapril
#12 enalaprilat
#13 fosinopril
#14 lisinopril
#15 perindopril
#16 ramipril
#17 saralasin
#18 teprotide
#19 (#9 OR #10 OR #11 OR #12 OR #13 OR #14 OR #15 OR #16 OR #17 OR #18)
#20 LOSARTAN explode all trees (MeSH)
#21 losartan
#22 imidazole
#23 irbesartan
#24 candesartan
#25 eprosartan
#26 valsartan
#27 olmesartan
#28 telmisartan
#29 (ace near inhibitor*)
#30 (#20 OR #21 OR #22 OR #23 OR #24 OR #25 OR #26 OR #27 OR #28 OR #29)
#31 CALCIUM CHANNEL BLOCKERS explode all trees (MeSH)
#32 amlodipine
#33 diltiazem
#34 felodipine
#35 nicardipine
#36 nifedipine
#37 nimodipine
#38 nisoldipine
#39 nitrendipine
#40 verapamil
#41 (#31 OR #32 OR #33 OR #34 OR #35 OR #36 OR #37 OR #38 OR #39 OR #40)
#42 ADRENERGIC AGONISTS explode all trees (MeSH)
#43 alprenolol
#44 atenolol
#45 metoprolol
#46 nadolol
#47 oxprenolol
#48 pindolol
#49 propranolol
#50 labetalol
#51 prazosin
#52 (#42 OR #43 OR #44 OR #45 OR #46 OR #47 OR #48 OR #49 OR #50 OR #51)
#53 DIURETICS explode all trees (MeSH)
#54 spironolactone
#55 triamterene
#56 bumetanide
#57 chlorthalidone
#58 furosemide
#59 indapamide
#60 chlorothiazide
#61 hydrochlorothiazide
#62 (#53 OR #54 OR #55 OR #56 OR #57 OR #58 OR #59 OR #60 OR #61)
#63 RECEPTORS ANGIOTENSIN single term (MeSH)
#64 ANGIOTENSINS explode tree 1 (MeSH)
#65 (angiotensin near inhibit*)
#66 (angiotensin near antagonist*)
#67 (angiotensin near blocker*)
#68 antihypertensive*
#69 (#64 OR #65 OR #66 OR #67 OR #68)
#70 (#8 OR #19 OR #30 OR #41 OR #52 OR #62 OR #69)
#71 DIABETES MELLITUS explode tree 1 (MeSH)
#72 (diabetes next mellitus)
#73 (iddm or niddm)
#74 DIABETIC NEPHROPATHIES single term (MeSH)
#75 (diabetic next nephrop*)
#76 (diabetic next glomerul*)
#77 (diabetic and (kidney next disease*))
#78 (diabetic and (renal next disease*))
#79 (diabetes and (renal next disease*))
#80 (diabetes and (kidney next disease*))
#81 (diabetes and nephro*)
#82 (diabetes and nephri*)
#83 (diabetes and glomerulo*)
#84 (diabetic and glomerulo*)
#85 (diabetic and nephrit*)
#86 (diabetic and nephro*)
#87 (#71 OR #72 OR #73 OR #74 OR #75 OR #76 OR #77 OR #78 OR #79 OR #80 OR #81 OR #82 OR #83 OR #84 OR #85 OR #86)
#88 (#70 AND #87), from 2005 to 2009
Appendix 3 Data extraction form (Chapter 2 systematic review)
Study data extraction form
Study | Author/journal/year |
Number in trial | |
Intervention drug | |
Comparator drug | |
Trial details | Country of trial |
Length of trial | |
Intervention dose | |
Number in intervention | |
Comparator dose | |
Number in comparator | |
Existing hypertension | |
Blood pressure-lowering allowed | |
Participant characteristics | Type of diabetes |
Normoalbuminuria/microalbuminuria | |
Age | |
Trial quality | Randomisation |
Blinding | |
Informed consent | |
Intention to treat |
Study results
Urinary albumin | |
---|---|
AER/ACR | |
Units | |
Intervention group | Baseline |
End point | |
Change | |
Comparator group | Baseline |
End point | |
Change | |
Progression/regression | |
Normo to micro | Intervention |
Comparator | |
Micro to macro | Intervention |
Comparator | |
Micro to normo | Intervention |
Comparator | |
Adverse events | Intervention group |
Comparator group | |
Mortality | Intervention group |
Comparator group |
Appendix 4 Excluded studies (Chapter 2 systematic review)
Study (first author, year) | Type of diabetes | Urinary albumin | Reasons for excluding |
---|---|---|---|
Scognamiglio 1997205 | 1 | Normoalbuminuria | Not all normoalbuminuric at baseline |
Bakris 199493 | 1 | NS | Normoalbuminuria and microalbuminuria not analysed separately |
Parving 1989206 | ‘IDDM’ | Macroalbuminuria | Interim report of trial reported in Parving 2001105 |
Capek 1994207 | 2 | Microalbuminuria | Results reported are not consistent throughout the paper |
Tütüncü 2001208 | 2 | Microalbuminuria | ACEi vs. AR2B |
Stornello 1989, 1992209,210 | 2 | Macroalbuminuria | All patients received both treatments |
Crepaldi 199581 | 2 | Normo- and microalbuminuria | Could not extract consistent data |
Velussi 1996211 | 2 | Normo- and microalbuminuria | Not clearly a RCT |
Rizzoni 2005212 | 2 | Normo- and microalbuminuria | Normo- and microalbuminuric patients could not be separately analysed |
Ko 2005213 | 2 | Normo- or microalbuminuria | Normo- and microalbuminuric patients could not be separately analysed |
Cordonnier 199979 | 2 | Micro- and macroalbuminuria | Outcome urinary protein not urinary albumin |
Lacourcière 2000214 | 2 | 20–350 µg/minute | ACEi vs. AR2B |
DETAIL 2004215 | 2 | Mixed | Normo- and microalbuminuric patients could not be separately analysed |
ABCD study216 | 2 | NS | Normo- and microalbuminuric patients not identified |
Baba 200186 | 2 | NS | Normo- and microalbuminuric patients not identified |
Joglekar 1998217 | 2 | NS | Normo- and microalbuminuric patients not identified |
Lin 1995218 | 2 | NS | Normo- and microalbuminuric patients not identified |
FACET 1998219 | Mixed | Normo- and microalbuminuria | Mixed type 1 and type 2 |
HOPE 2000220 | Mixed | Normo- and microalbuminuria | Mixed type 1 and type 2 |
Bakris 1992221 | Mixed | Macroalbuminuria | Mixed type 1 and type 2 |
Marre 1987222 | Mixed | Microalbuminuria | Mixed type 1 and type 2 |
Nankervis 1998223 | Mixed | Microalbuminuria | Mixed type 1 and type 2 |
O’Donnell 1993224 | Mixed | Microalbuminuria | Mixed type 1 and type 2 |
Phillips 1993225 | NS | Microalbuminuria | Mixed type 1 and type 2 |
Sato226 | NS | Micro- and macroalbuminuria | Mixed type 1 and type 2 |
AIPRI 1996227 | – | Normo- and microalbuminuria | Not all diabetes |
Appendix 5 Summary of current diabetes health economic models
Do current models allow the impact of treatment based on renal status to be evaluated?
The following table lists is a detailed list of health economic models for diabetes including a brief comment about the extent to which the models include parameters relating to the presence of and severity of nephropathy in modelling costs or outcomes.
Model | Reference | Extent to which nephropathy addressed | Type of diabetes |
---|---|---|---|
Type 1 diabetes models | |||
DCCT 1996 | Diabetes Control and Complications Trial Research Group. Lifetime benefits and costs of intensive therapy as practiced in the Diabetes Control and Complications Trial. The Diabetes Control and Complications Trial Research Group. JAMA 1996;276:1409–15 | Includes Markov submodel for nephropathy | Type 1 |
Palmer et al. 2000 | Palmer AJ, Weiss C, Sendi PP, Neeser K, Brandt A, Singh G, et al. The cost-effectiveness of different management strategies for type I diabetes: a Swiss perspective. Diabetologia 2000;43:13–26 | Includes modelling as a progression through disease states to ESRD mortality | Type 1 |
Type 2 diabetes models | |||
Eastman et al. 1997 | Eastman RC, Javitt JC, Herman WH, Dasbach EJ, Zbrozek AS, Dong F, et al. Model of complications of NIDDM: I. model construction and assumptions. Diabetes Care 1997;20:725–34. URL: http://care.diabetesjournals.org/content/20/5/725.short | Includes four submodels for nephropathy | Type 2 |
Global diabetes model | Brown JB, Russell A, Chan W, Pedula K, Aickin M. The global diabetes model: user friendly version 3.0. Diabetes Res Clin Pract 2000;50:S15–46 | Makes use of the Eastman et al. 1997 model | Type 2 |
Cardiff diabetes model156 | No publication found – reported in: http://care.diabetesjournals.org/content/30/6/1638.full.pdf | Includes data on renal events from DCCT45 and UKPDS 6422 | Type 2 |
Sheffield diabetes model156 | No publication found – reported in: http://care.diabetesjournals.org/content/30/6/1638.full.pdf | Includes a submodel for nephropathy. Nephropathy model based on Eastman et al. 1997 model of diabetes progression and the DCCT research group228 | Type 2 |
UKPDS outcomes model | Clarke PM, Gray AM, Briggs A, Farmer A, Fenn P, Stevens R, et al. A model to estimate the lifetime health outcomes of patients with type 2 diabetes: the United Kingdom Prospective Diabetes Study (UKPDS) outcomes model. Diabetologia 2004;47:1747–59. URL: www.springerlink.com/content/29qrwyvccp0yjeje/fulltext.pdf | Model contains a single Weibull equation to predict renal failure | Type 2 |
UKPDS risk engine | Stevens RJ, Kothari V, Adler AI, Stratton IM, Holman RR. The UKPDS risk engine: a model for the risk of coronary heart disease in type II diabetes (UKPDS 56). Clin Science 2001;101:671–9. URL: http://www.clinsci.org/cs/101/0671/1010671.pdf | No renal component | Type 2 |
CDC/RTI type 2 diabetes progression model | Hoerger TJ, Harris R, Hicks KA, Donahue K, Sorensen S, Engelgau M. Screening for type 2 diabetes mellitus: a cost-effectiveness analysis. Ann Intern Med 2004;140:689–99. URL: www.annals.org/content/140/9/689.full.pdf | Includes simulation for nephropathy and ESRD | Type 2 |
Caro et al. 2000 | Caro JJ, Klittich WS, Raggio G, Kavanagh PL, O’Brien JA, Shomphe LA, et al. Economic assessment of troglitazone as an adjunct to sulfonylurea therapy in the treatment of type 2 diabetes. Clin Ther 2000;22:116–27 | Includes five states of nephropathy | Type 2 |
Palmer et al. 2000 | Palmer A, Sendi P, Spinas G. Applying some UK Prospective Diabetes Study results to Switzerland: the cost-effectiveness of intensive glycaemic control with metformin versus conventional control in overweight patients with type-2 diabetes. Schweiz Med Wochenschr 2000;130:1034 | Includes renal failure and death from renal disease | Type 2 |
Bagust et al. 2001 | Bagust A, Hopkinson P, Maier W, Currie C. An economic model of the long-term health care burden of type II diabetes. Diabetologia 2001;44:2140–55 | Includes three independent modules governing nephropathy | Type 2 |
US Centre for Disease Control 2002 | Hoerger T, Bethke A, Richter A, Sorensen S, Engelgau M, Thompson T, et al. Cost-effectiveness of intensive glycemic control, intensified hypertension control, and serum cholesterol level reduction for type 2 diabetes. JAMA 2002;287:2542–51 | Renal outcomes modelled as progression through to death by ESRD | Type 2 |
Zhou et al. 2005 | Zhou H, Isaman DJ, Messinger S, Brown MB, Klein R, Brandle M, et al. A computer simulation model of diabetes progression, quality of life, and cost. Diabetes Care 2005;28:2856–63 | Includes separate Markov submodel corresponding to three states of nephropathy | Type 2 |
Tilden et al. 2007 | Tilden DP, Mariz S, O’Bryan-Tear G, Bottomley J, Diamantopoulos A. A lifetime modelled economic evaluation comparing pioglitazone and rosiglitazone for the treatment of type 2 diabetes mellitus in the UK. Pharmacoeconomics 2007;25:39–54 | Includes renal failure | Type 2 |
Types 1 and 2 diabetes models | |||
IMIB model | Palmer AJ, Brandt A, Gozzoli V, Weiss C, Stock H, Wenzel H. Outline of a diabetes disease management model: principles and applications. Diabetes Res Clin Pract 2000;50:S47–56. URL: www.sciencedirect.com/science/article/pii/S0168822700002163 | Includes renal submodel of eight states | Type 1 and type 2 |
EAGLE model | Mueller E, Maxion-Bergemann S, Gultyaev D, Walzer S, Freemantle N, Mathieu C, et al. Development and validation of the economic assessment of glycemic control and long-term effects of diabetes (EAGLE) model. Diabetes Technol Ther 2006;8:219–36. URL: www.liebertonline.com/doi/pdfplus/10.1089/dia.2006.8.219 | Can simulate kidney system (microalbuminuria, macroalbuminuria, ESRD) | Type 1 and type 2 |
Core diabetes model | Palmer AJ, Roze S, Valentine WJ, Minshall ME, Foos V, Lurati FM, et al. The CORE diabetes model: projecting long-term clinical outcomes, costs and cost-effectiveness of interventions in diabetes mellitus (types 1 and 2) to support clinical and reimbursement decision-making. Curr Med Res Op 2004;20(Suppl. 1):S5–26 | Includes simulations for nephropathy | Type 1 and type 2 |
Archimedes model | Eddy DM, Schlessinger L. Archimedes: a trial-validated model of diabetes. Diabetes Care 2003;26:3093–101. URL: http://care.diabetesjournals.org/content/26/11/3093.full.pdf | Not clear – briefly discusses nephropathy and causes | Type 1 and type 2 |
Grima et al. 2007 | Grima DT, Thompson MF, Sauriol L. Modelling cost effectiveness of insulin glargine for the treatment of type 1 and 2 diabetes in Canada. Pharmacoeconomics 2007;25:253–66 | Includes ESRD as a state | Type 1 and type 2 |
Appendix 6 Health state valuations in diabetes
Abstract of a research paper, the research for which was carried out in parallel with the research programme establishing health state values that was subsequently incorporated into the health outcome simulation models (Chapters 5 and 6)
A meta-analysis of health state valuations for people with diabetes: explaining the variation across methods and implications for economic evaluation
Lung TW, Hayes AJ, Hayen A, Farmer A, Clarke PM. Qual Life Res 2011;20:1669–78.
Purpose
To review published studies on the effect of diabetes and its complications on utility scores to establish whether there is systematic variation across studies and to examine the implications for the estimation of QALYs.
Methods
A systematic review was performed using studies reporting QALY measures elicited from people with diabetes including those with a history of complications. Meta-analysis was used to obtain the average utility, and metaregression was employed to examine the impact of study characteristics and elicitation methods on these values. The effect of different utility scores on QALYs was examined using diabetes simulation models.
Results
In the meta-analysis based on 45 studies reporting 66 values, the average utility score was 0.76 (95% CI 0.75–0.77). A metaregression showed significant variation due to age, method of elicitation and the proportion of males. The average utility score for individual complications ranged from 0.48 (95% CI 0.25, 0.71) for chronic renal disease to 0.75 (95% CI 0.73, 0.78) for myocardial infarction, and these differences produced meaningful changes in simulated QALYs. There was significant heterogeneity between studies.
Conclusions
We provide summary utility scores for diabetes and its major complications that could help inform economic evaluation and policy analysis.
List of abbreviations
- A2RB
- angiotensin 2 receptor blocker
- ACE
- angiotensin-converting enzyme
- ACEi
- angiotensin-converting enzyme inhibitor
- ACR
- albumin-to-creatinine ratio
- ADA
- American Diabetes Association
- ADVANCE
- Action in Diabetes and Vascular Disease: Preterax and Diamicron MR Controlled
- CARDS
- Collaborative Atorvastatin Diabetes
- CI
- confidence interval
- CV
- coefficient of variation
- CVD
- cardiovascular disease
- DCCT
- Diabetes Control and Complications Trial
- EDIC
- Epidemiology of Diabetes Interventions and Complications
- eGFR
- estimated glomerular filtration rate
- ESRD
- end-stage renal disease
- GFR
- glomerular filtration rate
- GP
- general practitioner
- GPRD
- General Practice Research Database
- HbA1c
- glycated haemoglobin
- HTA
- Health Technology Assessment
- ICER
- incremental cost-effectiveness ratio
- iGFR
- isotopic glomerular filtration rate
- MDRD
- Modification of Diets in Renal Disease study
- NICE
- National Institute for Health and Care Excellence
- NIHR
- National Institute for Health Research
- ORPS
- Oxford Regional Prospective Diabetes Study
- QALY
- quality-adjusted life-year
- RAAS
- renin–angiotensin–aldosterone system
- RAASI
- renin–angiotensin–aldosterone system inhibitor
- SD
- standard deviation
- statin
- HMG-CoA reductase inhibitor
- UAE
- urinary albumin excretion
- UKPDS
- United Kingdom Prospective Diabetes Study