Notes
Article history
The research reported in this issue of the journal was funded by the HTA programme as project number 14/159/07. The contractual start date was in September 2015. The draft report began editorial review in September 2016 and was accepted for publication in January 2017. The authors have been wholly responsible for all data collection, analysis and interpretation, and for writing up their work. The HTA editors and publisher have tried to ensure the accuracy of the authors’ report and would like to thank the reviewers for their constructive comments on the draft document. However, they do not accept liability for damages or losses arising from material published in this report.
Declared competing interests of authors
Rupert Payne sat on the Health Technology Assessment Efficient Study Designs Board for 1 year, from 2015 to 2016.
Permissions
Copyright statement
© Queen’s Printer and Controller of HMSO 2017. This work was produced by Miani et al. under the terms of a commissioning contract issued by the Secretary of State for Health. This issue may be freely reproduced for the purposes of private research and study and extracts (or indeed, the full report) may be included in professional journals provided that suitable acknowledgement is made and the reproduction is not associated with any form of advertising. Applications for commercial reproduction should be addressed to: NIHR Journals Library, National Institute for Health Research, Evaluation, Trials and Studies Coordinating Centre, Alpha House, University of Southampton Science Park, Southampton SO16 7NS, UK.
2017 Queen’s Printer and Controller of HMSO
Chapter 1 Introduction
Background
Volume and cost of repeat prescriptions in primary care
In England, the NHS spends > £9B on prescription medicines dispensed in the community each year. 1 An increasing number of NHS patients receive prescriptions for chronic conditions, which can be generated without the need for a consultation in primary care. These are known as repeat prescriptions. 2 It is estimated that repeat prescriptions account for two-thirds of prescriptions generated in primary care. 3 Among the 20 most prescribed medicines dispensed in the community in England during 2015, all but one (amoxicillin, an antibiotic) are commonly prescribed on a repeat basis for chronic conditions (Table 1). Such conditions include diabetes, asthma and hypertension.
BNF chemical name | Items prescribed, n (millions) | NIC, £ (millions)a | Class of item/example(s) of conditions it can treat |
---|---|---|---|
Simvastatin | 34.4 | 46.5 | HMG CoA reductase inhibitor/hypercholesterolaemia, primary prevention CVD |
Omeprazole | 30.1 | 64.8 | PPI/gastro-oesophageal reflux, peptic ulceration |
Levothyroxine sodium | 29.7 | 104.5 | Thyroid hormone therapy/hypothyroidism |
Aspirin | 28.0 | 27.3 | Antiplatelet agent/secondary prevention of stroke, myocardial infarction |
Atorvastatin | 27.2 | 53.8 | HMG CoA reductase inhibitor/hypercholesterolaemia, primary prevention of CVD |
Ramipril | 26.7 | 42.7 | ACE/hypertension, heart failure |
Amlodipine | 25.4 | 31.9 | Calcium channel blocker/hypertension, angina |
Lansoprazole | 22.9 | 41.6 | PPI/gastro-oesophageal reflux, peptic ulceration |
Paracetamol | 22.9 | 87.6 | Analgesic/mild to moderate pain |
Salbutamol | 21.9 | 62.4 | Bronchodilator/asthma |
Colecalciferol | 19.9 | 90.6 | Secosteroid/osteoporosis |
Metformin hydrochloride | 19.8 | 120.4 | Antihyperglycaemic agent/diabetes mellitus |
Bisoprolol fumarate | 19.4 | 26.1 | Beta blocker/angina, heart failure |
Co-codamol | 15.7 | 97.6 | Analgesic/mild to moderate pain |
Citalopram hydrobromide | 14.4 | 17.8 | SSRI/depression, panic disorder |
Bendroflumethiazide | 13.5 | 14.8 | Thiazide diuretic/hypertension |
Furosemide | 12.5 | 13.9 | Loop diuretic/oedema |
Amitriptyline hydrochloride | 12.4 | 23.1 | Tricyclic antidepressant/neuropathic pain (unlicensed) |
Amoxicillin | 11.9 | 18.4 | Antibiotic/infection |
Warfarin sodium | 11.6 | 23.1 | Anticoagulant/prevention of stroke in atrial fibrillation |
The majority of prescription costs in primary care are for chronic conditions. For the last 9 years, drugs used in the treatment of diabetes [classified under section 6.1 of the British National Formulary (BNF)] have accounted for the largest net ingredient costs (NICs) (i.e. the cost of the drug not including dispensing costs, fees or discounts) of prescriptions dispensed in primary care in England. Costs increased for diabetes drugs by £87.6M (10.3%) since 2014 to reach £936.7M in 2015. 5
The total NIC of all prescriptions dispensed in the community has increased by 16.8% since 2005, despite a fall in the average NIC per prescription. 5 It has been estimated that between £100M and £300M is wasted in the form of unused or partially used medications each year. 6,7 Ensuring that prescriptions are issued for a duration that minimises the waste of medicines is an important factor in reducing financial loss to the NHS.
Guidance and policy on repeat prescription length
With regard to repeat prescriptions, there is some ambiguity in the Department of Health’s (DH’s) guidance on prescription length. Guidance issued by commissioners (Primary Care Trusts until 2011 and now Clinical Commissioning Groups) in some areas, as well as that from the Pharmaceutical Services Negotiating Committee (PSNC),8 has encouraged general practitioners (GPs) to issue shorter prescriptions, typically of 28 days in length. 9–11 This guidance was based on evidence that limiting prescription length to 28 days reduces medicine waste and thus results in cost savings,12,13 and on the reported success of local prescribing schemes, for example in Surrey and Grampian,13 and Brighton and Hove. 14 This was stated to be in line with the DH’s policy to strike a ‘balance between patient convenience, good medical practice and drug wastage’. 11 Shorter prescription lengths have also been shown to benefit patients by providing better signalling to GPs for treatment discontinuations due to adverse events. 15
The guidance issued by local commissioning organisations and by the PSNC8 encouraging shorter prescriptions has tended to advocate a blanket 28-day prescribing policy. In contrast, the National Institute for Health and Care Excellence (NICE), through the BNF, recommends blanket 28-day prescribing for certain classes of controlled drugs only. 16 In addition, recent evidence argues for a more informed use of 28-day intervals for repeat prescriptions,7,17,18 closer to the DH’s more general principle that prescription duration should be consistent with medically appropriate patient needs while also considering NHS resources, patient convenience and the dangers of having excess quantities of prescription medications in the home. 19 Similarly, the British Medical Association and the General Medical Council do not recommend a specific prescription duration but instead encourage safe and appropriate repeat prescription intervals adapted to the needs of individual patients. 19,20
Indeed, evidence shows that there may be some disadvantages to shorter prescriptions. Shorter prescriptions may (1) increase the costs to the health system through increased GP administrative workload and dispensing fees to pharmacists,21 (2) increase costs incurred by the patient,22 (3) have a negative impact on patient satisfaction23 and (4) have a negative impact on adherence. 24 Whether or not the most commonly used prescription length should be changed was identified as a key area for research in the DH’s 2011 roundtable, Making Best Use of Medicines. 25
Aims and objectives
Given the substantial cost that wasted medication represents, disparities in the evidence, and the ambiguity in national dispensing guidelines for GPs, the National Institute for Health Research (NIHR) Health Technology Assessment (HTA) programme has commissioned research to synthesise and assess the evidence on the clinical effectiveness and cost-effectiveness of shorter (28-day) versus longer (3-month) duration prescriptions in terms of patients’ health outcomes and health system costs.
The aim of this study is to provide a high-quality reference on the clinical effectiveness and cost-effectiveness of primary care physicians issuing longer duration versus shorter duration (3-month vs. 28-day) prescriptions in patients with stable chronic diseases. This study is intended to help inform prescribing policy. In addition, this study is directly relevant to patient groups with stable, chronic conditions who require regular repeat prescriptions. In order to provide a comprehensive and transparent assessment of the impact of different prescription lengths on a relevant set of outcomes, the following approaches have been used:
-
a systematic review of the clinical effectiveness and cost-effectiveness evidence, incorporating any relevant clinical and cost outcomes
-
a cost analysis based on available secondary data
-
disease-specific decision-analytic models.
Table 2 presents the full list of the potential outcomes of interest, as well as a brief description of each one and an indication of which approach(es) was used to examine it.
Outcome | Description | Method |
---|---|---|
Disease-specific health outcomes | Any health outcomes that measure the impact of a particular disease or condition on an individual’s health and well-being, for example disease management measures such as glycosylated haemoglobin level or cholesterol measures | Systematic review |
Generic health outcomes | Any health outcome measures that can be applied across diseases or conditions, and that could be used to estimate QALYs | Systematic review, decision models |
Adverse events | Any outcome that measures untoward medical occurrence in a patient, for example adverse drug reaction, unplanned hospitalisation including A&E attendance as well as admission for ambulatory care sensitive conditions, death | Systematic review |
Errors | Any outcome that measures preventable adverse effect of care, for example prescription error, drug monitoring error | Systematic review |
Adherence | Any outcomes that measure the extent to which a patient is dispensed the medication as prescribed and takes the prescribed medication as intended; this broad definition includes measures of compliancea | Systematic review |
Costs associated with adherence | ||
Drug wastage | Any outcome used to measure medicines issued to a patient but not consumeda | Systematic review, cost analysis |
Costs associated with wastage | ||
Professional administration time/costs | For example, time to write, renew or process the prescription and costs associated with administration time | Systematic review, cost analysis |
Pharmacists’ time/costs | For example, time to renew or process the prescription and costs associated with pharmacists’ time | Systematic review, cost analysis |
Patient experience/satisfaction | Any measure used to elicit feedback from patients on their views of care and services | Systematic review |
Patient costs | Any measure of personal expenses incurred by patients during the course of their care, for example out-of-pocket payments and travel costs | Systematic review |
Costs to the NHS | Longer-term health service costs | Decision model |
Structure of the report
Following this introductory chapter, the report is structured by method: Chapter 2 presents the systematic review, Chapter 3 reports on the cost analyses and Chapter 4 reports on the decision analyses. These three chapters can each be read as standalone documents, as they each present the method, findings and discussion of the approach indicated by their title. Chapter 5 draws overarching conclusions and recommendations from the different methods.
Patient and public involvement
Patients and members of the public, consulted through the INsPIRE (patIeNt and Public Involvement in REsearch) group based in Cambridgeshire, were involved in the drafting of the systematic review protocol specifically to help identify outcomes that were directly relevant to them. Based on their suggestions, we included three patient-centred outcomes: patient time, costs to the patient and synchronisation of prescriptions. This was in addition to the initial list of outcomes that we had already considered, which included adherence measures, disease-specific outcome measurements, drug wastage, adverse events, patient experience and satisfaction, professional administration time/costs, pharmacist costs, health outcomes and cost-effectiveness.
In addition, a copy of the draft report was sent to members of the INsPIRE group to obtain their feedback on the plain English summary. The summary was amended in light of the comments made by five patient and public involvement representatives.
Chapter 2 Systematic review
Introduction
This systematic review was conducted using rigorous methods26 and is reported in accordance with the Preferred Reporting Items for Systematic Reviews and Meta-Analyses (PRISMA) guidance. 27 The protocol for this systematic review is published on the PROSPERO database (registration number CRD42015027042). 28
This chapter is structured as follows: first, the objectives of the review are outlined (see Objectives); second, our methods are described (see Methods); third, our findings are presented (see Results); and, finally, these findings are discussed (see Discussion).
Objectives
This systematic review addresses the following research question: how do longer duration (i.e. 3-month) medication prescriptions compare with shorter duration (i.e. 28-day) medication prescriptions in terms of clinical effectiveness and cost-effectiveness in patients with stable, chronic conditions requiring one or more repeat prescriptions in primary care settings?
The objectives of this systematic review were:
-
to assess whether shorter or longer prescription lengths have positive or negative impacts on a range of health outcomes and patient experiences in patients with chronic stable diseases
-
to assess whether or not shorter or longer prescription lengths have an impact on patient adherence, wastage, GP time, dispensing costs, and costs to patients with chronic stable diseases
-
to evaluate the cost-effectiveness of different prescription lengths in patients with chronic stable diseases based on previously published economic analyses.
Methods
Inclusion and exclusion criteria
To address the above research questions, the inclusion and exclusion criteria of the populations, interventions, comparisons, outcomes and study types of interest are defined in the following sections and summarised in Table 3.
Inclusion | Exclusion |
---|---|
Population and setting | |
Studies of patients being treated in a primary care setting with a stable chronic disease or condition requiring one or more repeat prescriptions (including, but not limited to, hypothyroidism, diabetes, hypertension, CVD and depression), were eligible for inclusion | Studies of patients in low-income countries |
Studies of patients in high- and middle-income countries were eligible for inclusion | Studies conducted exclusively in secondary or tertiary care settings |
Interventions | |
Eligible studies were those that evaluated 3-month (90-day) prescriptions, or prescription lengths of around 90 days. The prescriptions could be for pharmaceutical medication, but could also include other medical prescriptions such as urostomy bags | Excessively long prescription lengths (> 12 months) and prescriptions that do not require dispensing (e.g. physical activity prescriptions) |
Comparisons | |
28-day (i.e. 1-month) prescription lengths, or prescriptions around 1 month | Prescription lengths < 28 days (i.e. 1 month) |
Outcomes | |
Eligible studies had to report on at least one of the following outcomes:
|
Studies were excluded if they:
|
Economic outcomes of interest included all of the above as well as costs, QALYs and ICERs | |
Study designs | |
RCTs, observational studies, cost comparison studies and economic evaluations were eligible for inclusion | Letters, editorials and commentaries were not eligible for inclusion unless they presented new data |
Studies published as abstracts or conference presentations were included if enough data were presented, and if the abstract was not associated with a full paper |
Populations
Studies were eligible for inclusion if they involved patients being treated in a primary care setting with a stable chronic disease or condition such as hypothyroidism, diabetes, cardiovascular disease (CVD) or depression, requiring one or more repeat prescriptions.
Studies conducted in secondary/tertiary care settings, or in low-income countries as defined by the World Bank (2016),29 were excluded from the review. When it was not clear whether or not a study was exclusively conducted in a primary care setting, or if it was not stated what the study setting was, we took an inclusive approach and considered the study to be eligible.
Interventions and comparisons
This systematic review focused on studies that had the objective of evaluating prescription lengths. Eligible studies were those that evaluated 3-month (90-day) prescriptions (or prescriptions around 90 days) in comparison with 28-day prescriptions (or prescription lengths around 1 month). The prescriptions could be for pharmaceutical medication but could also include other non-pharmaceutical prescriptions such as urostomy bags. Studies were excluded that evaluated excessively long prescription lengths (e.g. > 12 months) or evaluated prescriptions that did not require dispensing (e.g. physical activity prescriptions).
Outcomes
Eligible studies had to report on at least one of the following outcomes:
-
disease-specific health outcomes (any health outcomes that measure the impact of a particular disease or condition on an individual’s health and well-being, e.g. disease management measures such as glycosylated haemoglobin level or cholesterol measures)
-
generic health outcomes [any health outcome measures that can be applied across diseases or conditions, and that could be used to estimate quality-adjusted life-years (QALYs)]
-
adverse events [any outcome that measures untoward medical occurrence in a patient, e.g. adverse drug reaction, unplanned hospitalisation including accident and emergency (A&E) attendance as well as admission for ambulatory care sensitive conditions and death]
-
errors (any outcome that measures preventable adverse effect of care, e.g. prescription error, drug monitoring error)
-
adherence (any outcomes that measure the extent to which a patient takes the prescribed medication as intended by the prescriber; this broad definition includes established measures of compliance)
-
drug wastage (any outcome used to measure medicines issued to a patient but not consumed)
-
professional administration time/costs (e.g. time to write, renew or process the prescription and costs associated with administration time)
-
pharmacists’ time/costs (e.g. time to renew or process the prescription and costs associated with pharmacists’ time)
-
patient experience/satisfaction (any measure used to elicit feedback from patients on their views of care and services)
-
patient costs (any measure of personal expenses incurred by patients during the course of their care, e.g. out-of-pocket payments and travel costs)
-
costs to the NHS (longer-term health service costs).
Studies that reported only prescribing patterns or trends or reported on the costs of generic versus branded prescribing were excluded. Studies that evaluated adverse events without evaluating this outcome in direct association with prescription length were also excluded.
Study designs
Randomised controlled trials (RCTs), observational studies, cost analyses (e.g. cost description studies) and economic evaluations [e.g. cost-effectiveness analyses and cost–utility analyses, which may have reported incremental cost-effectiveness ratios (ICERs)] were eligible for inclusion.
Studies published as abstracts or conference presentations were included if enough outcome data were presented to interpret the findings, and if the abstract was not associated with a full paper, which we sought to confirm by contacting authors. Letters, editorials and commentaries were not eligible for inclusion unless they presented new data.
Search strategy
To identify relevant primary studies, we searched a number of databases:
-
MEDLINE (PubMed)
-
EMBASE
-
Cumulative Index to Nursing and Allied Health Literature (CINAHL)
-
Web of Science
-
Cochrane Central Register of Controlled Trials, which includes the Database of Abstracts of Reviews of Effects (DARE), the HTA database and the NHS Economic Evaluation Database (NHS EED).
We also performed searches in grey literature databases:
-
Open Archives Initiative harvester (OAIster)
-
OpenGrey
-
The New York Academy of Medicine (NYAM)’s Grey Literature Report.
Searches were conducted in all of the databases between 13 October 2015 and 21 October 2015. The search was rerun in PubMed in June 2016 to check that no additional relevant studies had been published in the interim period. Given that no additional studies of relevance were identified, further searching of other databases was deemed unnecessary.
All of the search terms used were in English, but the searches were not otherwise restricted by language. Search terms included (but were not limited to) ‘prescription length’, ‘prescription duration’, ‘medication duration’, ‘medication length’, ‘length of prescription’, ‘duration of prescription’, ‘prescribing pattern’, ‘prescription pattern’, ‘repeat dispensing’, ‘prescription interval’, ‘dispensing trends’, ‘prescription trends’, ‘prescribing trends’, ‘standardised prescribing’, ‘standardised prescription’, ‘one month prescription’, ‘one month supply’, ‘three month prescription’, ‘three month supply’, ‘90 day supply’, ‘28 day supply’, ‘30 day supply’, ‘long prescription’ and ‘short prescription’.
Details of the full search strategy are presented in Appendix 1.
Additional searching techniques to identify relevant studies were applied. These included:
-
searching for systematic reviews and health technology assessments that could yield additional primary studies. We searched the Cochrane Database of Systematic Reviews (CDSR), NIHR HTA, DARE and the NICE website between 13 October 2015 and 21 October 2015
-
checking the references within included papers and other reviews
-
searching for additional studies carried out by the first authors of relevant studies
-
carrying out citation searches of key publications to identify subsequent publications that have cited those key publications [using the ‘cited by’ option in Google Scholar™ (Google Inc., Mountain View, CA, USA)].
Study selection
The study selection involved four stages, shown in Table 4.
Stage | Study selection |
---|---|
1 | Titles and abstracts of studies identified in the searches were entered into an EndNote (Thomson Reuters, CA, USA) database, and references that were obviously not relevant (e.g. studies conducted in animals, low-income countries) were screened and excluded by the information specialist (JL). More details about this screening stage can be found in Appendix 2 |
2 | A pilot screening of 400 references was undertaken to ensure consistent agreement among the reviewers (AK, CMiani and JE) regarding the application of the inclusion/exclusion criteria. Any discrepancies were discussed among all reviewers, including a senior systematic reviewer (SK) |
3 | Two reviewers independently screened the remaining titles and abstracts for inclusion. Given the large numbers of references to screen, three reviewers (AK, CMiani and JE) were involved in the double screening process. Any discrepancies were discussed among all three reviewers, and a fourth reviewer (SK) screened studies deemed ‘unsure’ |
4 | Full papers of potentially relevant studies identified during stage 3 (and any subsequent papers identified through backward and forward searching of relevant studies) were obtained and screened independently by two reviewers (CMiani and JE), with any discrepancies discussed with a third reviewer (SK). At this stage, a table with excluded studies was created, stating reasons for exclusion (see Appendix 3) |
Data extraction
To facilitate data extraction, a form was developed in Microsoft Excel® (Microsoft Corporation, Redmond, WA, USA) and piloted using several studies. Eligible studies were extracted twice, once each by two reviewers working independently (two of AK, AM, CMiani, JE and SK). When the data were incomplete [e.g. sample sizes not reported, standard deviations (SDs) not reported], we attempted to contact the study authors.
In some cases, studies were found to be ineligible during this process. These studies were discussed among the wider research team to ensure that there was agreement regarding their ineligibility before they were added to the table of excluded studies. The two data extraction forms were then combined and compared by a third reviewer. Any discrepancies were resolved through discussion among all of the reviewers.
Risk-of-bias assessment
As no RCTs were included, to assess risk of bias in observational cohort and cross-sectional studies we used the Risk Of Bias in Non-Randomized Studies – of Interventions (ROBINS-I) assessment tool. 30 The tool assesses seven domains of bias (see Table 24, Appendix 4, for an overview of the tool). For each domain, the ‘signalling questions’ were completed by three reviewers independently (two of CMiani, JE and SK in each case) to determine the domain-level risk of bias, with any discrepancies resolved through discussion or by consulting a fourth reviewer (CMeads). The overall risk of bias was determined by all four reviewers based on the domain-level risk of bias and reviewers’ judgement of both the severity of the bias in a particular domain and the relative consequences of bias in different domains. 30 Studies were considered to be at a ‘serious risk’ of bias if one or more of the domains assessed was at serious risk of bias. When insufficient data were reported to allow a judgement, the risk of bias was classified as ‘no information’.
Grading of Recommendations Assessment, Development and Evaluation assessment
We used the Grading of Recommendations Assessment, Development and Evaluation (GRADE) criteria31 to assess the quality of the body of evidence for each outcome. As no RCTs were eligible for inclusion, only GRADE methodology applicable to non-RCTs is presented here. Using the standard four GRADE levels of quality (high, moderate, low and very low), non-RCTs were considered to have an initial rating of low. This rating was then up- or downgraded using the criteria (1) risk of bias, (2) imprecision, (3) inconsistency, (4) indirectness and (5) publication bias.
Synthesising the evidence
The evidence included in this review was largely summarised using a narrative synthesis, with data presented in tables, in the text, and in forest plots for visual purposes.
For each eligible outcome presented within a study, we calculated effect sizes [odds ratios (ORs) with 95% confidence intervals (CIs) for dichotomous outcomes, and mean difference (MD) with 95% CIs for continuous outcomes]. In some of the studies, SDs were imputed based on p-values (in cases where we could not obtain SDs from the study authors). We have noted when this was done in the tables.
The studies classified data by general therapeutic area (e.g. lipid-lowering drugs, antidiabetics) and by more specific chemical classification [e.g. angiotensin-converting enzyme inhibitors (ACEIs), statins]. We refer to these henceforth as the therapeutic class and the chemical class, respectively. We presented data for both the therapeutic class and the chemical class when these were available. When a study reported data by chemical class only,32 we combined these data using a meta-analysis in order to derive data for one of the corresponding broader therapeutic classes as evaluated in other studies (i.e. lipid-lowering agents, antidiabetics, etc.). We did not compare any effect size differences between the different therapeutic classes, as this review was not designed to consider these differences.
Exploratory meta-analyses were conducted for medication adherence and wastage. In each of these meta-analyses, we combined continuous and dichotomous data. The first step of this process was to calculate the standardised mean differences (SMDs) with 95% CIs for each dichotomous or continuous outcome as presented within each study. Dichotomous outcomes were converted to continuous data using the methods recommended in the Cochrane Handbook section 9.4.6. 33 We then calculated a standard error from these CIs. The last step was to pool the SMD and standard errors using a random-effects model in RevMan version 5.3 (RevMan, The Cochrane Collaboration, The Nordic Cochrane Centre, Copenhagen, Denmark). Evidence of the extent of statistical heterogeneity was assessed by visually examining the extent to which the CIs overlapped. Additionally, the I2 value, automatically calculated by the RevMan software, was reported, and an interpretation of the levels of heterogeneity was made based on the recommendations of Deeks et al. 34
Results
Studies identified
Our search identified a total of 24,876 records across the databases searched. After the removal of duplicates and the initial screening of titles and abstracts, we considered 47 references for full-text evaluation. Of these, nine studies were identified as eligible for inclusion in the review, along with seven additional studies retrieved through backwards and forwards citation checking (Figure 1). Appendix 3 provides reference details of excluded studies and reasons for their exclusion based on our full-text review.
FIGURE 1.
The PRISMA flow chart.
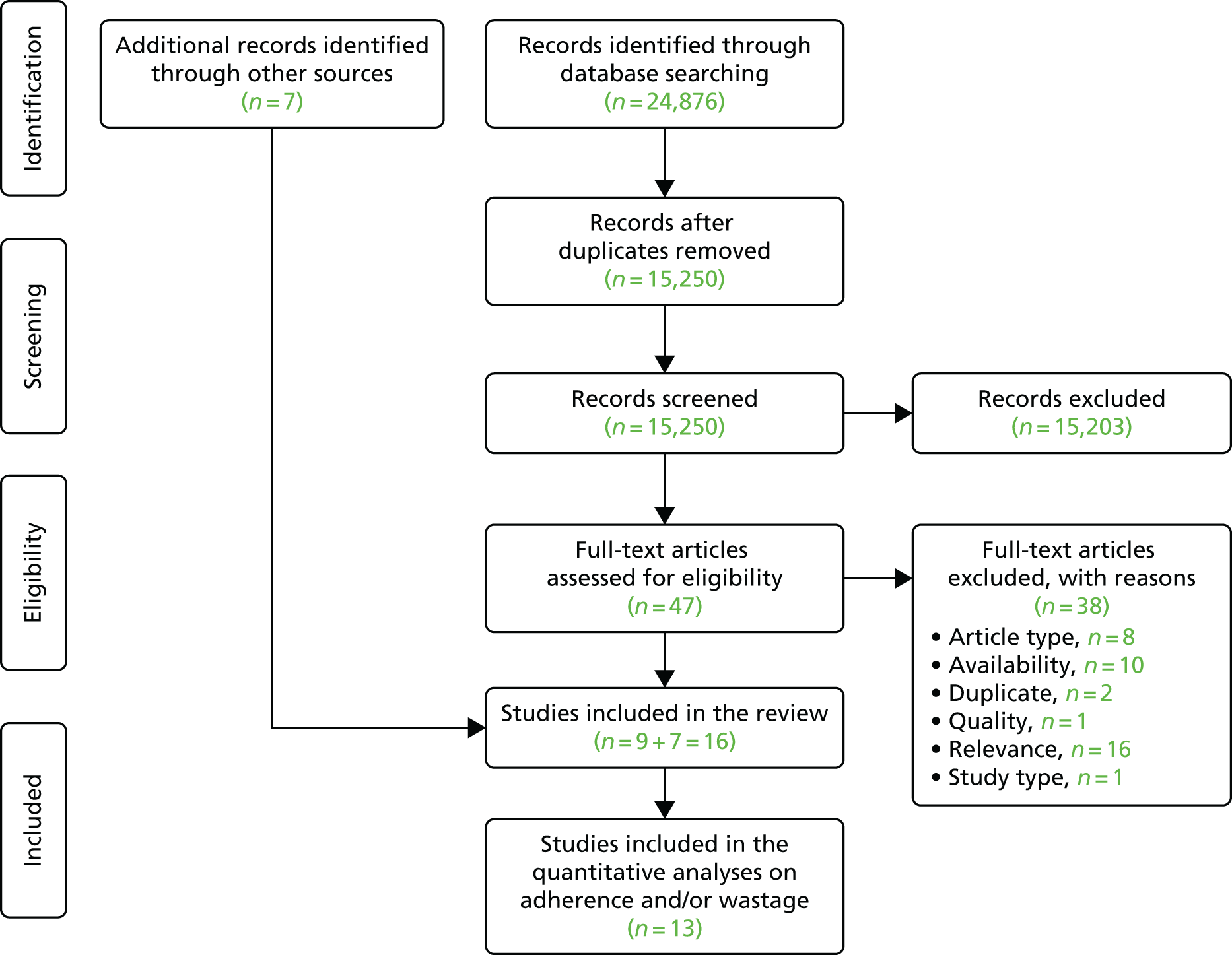
Included studies
Overview
The information presented on study populations was limited. Some study populations included those who were new to treatment,35–38 while others included those receiving ongoing care,32,39–41 and another included both those who were new to treatment and those receiving ongoing care. 42 Half of the studies specified the insurance scheme that patients were enrolled in: two (US) studies included predominantly indigent populations (adults who do not have health insurance and are not eligible for Medicaid, Medicare or private health insurance),39,43 two studies were conducted among Medicaid patients,44,45 four studies were set in Veterans Health Administrations37,40,42,46 and one study used data from Kaiser Permanente health-care delivery sites. 47
All of the studies were conducted in the USA. In the majority of studies, there was insufficient information presented to determine whether or not patients were being treated exclusively in primary care settings. For the three studies that provided details of the setting, one was conducted in a primary care clinic,39 one was conducted among patients seen in primary care, mental health clinics, inpatient services and integrated mental health primary care,37 and one was conducted in an internal medicine practice. 43 Faris et al. 48 investigated patients in four specialty therapeutic categories (multiple sclerosis, rheumatoid arthritis, oncology and growth hormone) that are not typically primarily managed in primary care in England, but insufficient information is presented to determine in which setting these patients were being treated.
Nine of the 16 studies compared a 30-day medication supply with a 90-day supply32,35,36,41,42,45,46,48,49 and three studies compared three lengths of supply: 30 days, 31–89 days and ≥ 90 days. 38,40,47 The remaining four studies considered (1) 30 days’ versus 60 days’ supply,39 (2) 100 days’ versus 34 days’ supply,44 (3) 90 days versus < 90 days’ supply37 and (4) prescription lengths of ≤ 90 days. 43
The number of medications examined in a single study ranged from one40 to ‘any medication for a chronic condition’. 49 The most common clinical classes evaluated were lipid-lowering agents,32,35,36,38,39,41–45,47,49 antihypertensives,32,35,36,38,41–45,47,49 antidiabetics32,35,41–45,47 and antidepressants. 32,36,37,42,44,45
The study periods ranged from 3 months46 to 7 years;37 only one study was conducted over a period of less than 1 year,46 six studies were conducted over a 12-month period 32,36,42,43,45,47 and eight studies were conducted over a period of more than 1 year. 35,37,39–41,44,48,49 Ryvkin et al. 38 did not report the length of their study. The most common outcomes measured were adherence,35,36,39,40,43–45,47 wastage32,36,38,41,42,45,46,48 and costs. 42,44–46,49
When the study design was not reported35,36,38,43,46 or was unclear,41,49 the design was classified by three reviewers (JE, SK and CMiani) based on the information presented, and guided by the NICE algorithm for classifying quantitative study designs. 50 No RCTs were identified. Of the 16 included studies, nine were retrospective cohorts (six described as such by the study authors32,37,39,40,45,48 and three classified as such by the reviewers35,36,38), two were retrospective pre–post studies (one so described by the authors,44 the other defined as such by the reviewers41), three were cross-sectional studies (two described as such by the authors,42,47 the other so defined by the reviewers43) and two were cost analyses (both defined as such by the reviewers). 46,49 In addition, the authors of one of the retrospective cohort studies45 and of one of the retrospective pre–post studies44 undertook a cost–consequences analysis. In the vast majority of the studies, the authors undertook a secondary data analysis of pharmacy claims data. 32,36–38,40–47,49
For five of the included studies, only an abstract was available,35,36,38,41,48 and a sixth included article was an extended conference abstract. 46 Although we attempted to contact the authors of these reports, the full papers corresponding to these abstracts could not be obtained. Given the paucity of high-quality studies identified, and in discussion with expert advisors, the decision was taken to include all six identified abstracts. An overview of the characteristics of all 16 studies is presented in Table 5. The key findings are presented by outcome measures (health, adherence, wastage, costs and other) in the Outcomes section of this chapter.
Reference, country, study design | Setting | Aim | Participants | Medication evaluated | Comparison | Total sample size (number of patients unless otherwise stated) | Outcomes measured | Study length |
---|---|---|---|---|---|---|---|---|
Batal et al. 2007,39 USA, retrospective cohort | Primary care clinic serving a predominantly minority and indigent population | To determine the effect of prescription size on patients’ adherence to hyperlipidaemia therapy | Patients receiving ongoing care and medication for hyperlipidaemia | Lipid-lowering agents (statins) | 60-day supply of medication (based on modal supply of > 45 days) compared with a 30-day supply (based on modal supply of < 45 days) | 3386 | Adherence, health | 3 years |
Domino et al. 2011,44 USA, retrospective pre–post controlled study with a cost–consequences analysis | Not explicitly reported; claims data from two centres for Medicare and Medicaid services | To estimate the effect of two separate policy changes in the North Carolina Medicaid programme: (1) reduced prescription lengths from 100 to 34 days’ supply and (2) increased co-payments for brand name medications | Adult Medicaid recipients who use medications for chronic conditions | Antidepressants, antihypertensives, antipsychotics, antidiabetics (sulfonylureas), seizure disorder medications, lipid-lowering agents (statins) | Reduced prescription length from 100 days to 34 days | 268,050 | Adherence, costs | 18 months |
Faris et al. 2010,48 USA, retrospective cohorta | Not explicitly reported | To determine the impact of days’ supply on waste. To compare medication waste rates between patients with a 90-day supply and those with a 30-day supply in four specialty therapeutic categories | Patients in one of four specialty therapeutic categories: multiple sclerosis, rheumatoid arthritis, oncology and growth hormone | Medications for multiple sclerosis, rheumatoid arthritis, oncology and growth hormone | 90-day supply compared with 30-day supply | Not reported | Wastage | 21 months (360-day study period and 270-day washout period to determine drop off) |
Hermes et al. 2010,35 USA, retrospective cohorta | Not explicitly reported | To compare adherence within three chronic medication classes by days’ supply and evaluate potential adherence predictors | Members with a first claim | Antihypertensives, antidiabetics, lipid-lowering agents | 90-day supply compared with 30-day supply | At 270 days: 183,666; at 540 days: 112,220 | Adherence | 540 days |
Jiang et al. 2007,36 USA, retrospective cohorta | Not explicitly reported; pharmacy claims data (Walgreens) | To compare adherence and wastage of 30-day retail programme, mandatory 90-day retail programme and voluntary 90-day retail programme | Patients who were new to ACEI, statins or SSRIs | Antidepressants (SSRIs), antihypertensives (ACEIs), lipid-lowering agents (statins) | 90-day supply, either mandatory or voluntarily, compared with 30-day supply | 1685 | Adherence, wastage | 1 year |
Murphy et al. 2012,32 USA, retrospective cohort | Not explicitly reported; pharmacy claims data (Walgreens) | To examine medication wastage for patients filling 90-day supplies of medication compared with those filling 30-day prescriptions | Adults with repeat prescriptions for ongoing care | Antidepressants (SSRIs, tricyclics), antidiabetics (biguanides, insulin), antihypertensives (alpha-beta blockers, ACEIs, angiotensin II receptor antagonists, calcium channel blockers, cardioselective beta-blockers, loop diuretics, thiazides), lipid-lowering agents (statins, fibric acid derivatives), thyroid hormones | 90-day supply compared with 30-day supply; 90-day supply stratified in two prescription fulfilment groups: mail and retail | 60,358 | Wastage | 1 year |
Parikh et al. 2001,46 USA, cost analysis | Not explicitly reported; hospital records from Veterans Affairs San Diego Healthcare System | To determine whether or not the cost of dispensing 90-day quantities offsets the expense of potential waste because of patients’ oversupply | Patients with a chronic disease receiving a 90-day supply of medication | Not reported | 90-day supply compared with 30-day supply (NB hypothetical comparator as all participants received a 90-day supply) | 178 | Costs | 3 months |
Pfeiffer et al. 2012,37 USA, retrospective cohortb | Not explicitly reported; pharmacy claims from Veterans Affairs National Registry for Depression. Variety of settings including primary care, mental health clinics and inpatient services | To examine whether or not receipt of an initial 90-day supply of an antidepressant was associated with better or worse longer-term antidepressant coverage compared with patients who initially received a < 90-day supply | Adult patients newly diagnosed with major depression | Antidepressants | 90-day supply compared with < 90-day supply | 383,634 | Adherence, other (clinical encounters) | 7 years |
Rabbani and Alexander 2009,49 USA, cost analysis | Not explicitly reported; nationally representative medical expenditure panel survey data | To measure the difference in out-of-pocket and total costs among patients receiving different quantities of the same prescription drug used to treat a chronic condition, and to examine patient and health system characteristics associated with the use of a 3-month supply | Non-institutionalised patients who were observed to have filled both a 3-month and a 1-month supply of a given medicine during a 12-month period | 395 medications for chronic conditions. Most commonly prescribed were antihypertensives (atenolol, hydrochlorothiazide, furosemide, amlodipine), oestrogen hormone (conjugated oestrogens), lipid-lowering agents (atorvastatin), thyroid hormones (levothyroxine) | 3-month supply compared with 1-month supply | 2971 | Costs | 2 years |
Ryvkin and Garavaglia 2009,38 USA, retrospective cohorta | Not explicitly reported; pharmacy claims data (Medco Health Solutions) | To quantify medication wastage for lipid-lowering agents, antihypertensive therapy and PPIs | Patients new to therapy | Antihypertensives, lipid-lowering agents, antiulcers (PPIs) | 30- to 90-day supply and > 90-day supply compared with 30-day supply | 43,318 | Wastage | Not reported |
Schectman et al. 2002,43 USA, cross-sectional study | Academic internal medicine practice | To evaluate the association between multiple demographic and prescription factors with the adherence behaviour of an indigent rural population to determine whether or not such factors could assist in targeting interventions | Low-income patients without prescription insurance coverage on hypertension, hypercholesterolemia or oral diabetes medication | 42 medications: antihypertensives, antidiabetics, lipid-lowering agents | NA; looking at factors associated with adherence among a population receiving standard care. Prescription length varied, maximum supply was 90 days | 1984 | Adherence | 9 months |
Schmittdiel et al. 2015,47 USA, cross-sectional study | Not explicitly reported; electronic health data from the three largest Kaiser Permanente health-care delivery sites | To examine the relationship between Medicare STAR medication adherence metrics and modifiable health system-level characteristics in a cohort of Medicare-aged diabetes patients | Adults aged ≥ 65 years with diabetes | Antihypertensives (ACEIs, ARBs), antidiabetics (oral antihyperglycaemics), lipid-lowering agents (statins) | NA; looking at four health system level factors associated with adherence. Days’ supply was derived from pharmacy electronic medication dispensing records. Categorised as: < 30-day, 31- to 60-day, 61- to 90-day and > 90-day supply | 236,025 | Adherence | ≤ 1 year |
Steiner et al. 1993,40 USA, retrospective cohort | Not explicitly reported; pharmacy records from 10 Veteran Affairs Medical centres | To determine whether large prescriptions (≥ 90 days’ supplies) enhance the acquisition of maintenance medications by patients | Patients who had received digoxin prescriptions in the previous year (ongoing care) | Digoxin | 31- to 89-day supply and ≥ 90-day supply compared with 30-day supply | 120 | Adherence | 14 months |
Taitel et al. 2012,45 USA, retrospective cohort with a cost-consequence analysis | Not explicitly reported; pharmacy claims data (Walgreens) | To determine whether or not 90-day refills at community pharmacies could improve adherence, minimise wastage and control costs | Medicaid patients | Antidepressants (SSRIs), antidiabetics (oral hypoglycaemics), antihypertensives, lipid-lowering agents (statins) | 90-day prescription (day supply ≥ 84 days) compared with 30-day prescription (day supply < 84 days) | 52,898 | Adherence, cost, wastage, other (persistency) | 1 year |
Thiebaud and Patel 2006,41 USA, retrospective pre–post controlled studya | Not explicitly reported; managed care organisation | To evaluate the effect of a new programme that allowed patients to fill 90-day prescriptions in retail pharmacies | Patients insured in a single managed care organisation receiving ongoing care | Antidiabetics, antihypertensives, lipid-lowering agents | Increased from 30-day supply to 90-day supply | 88,590 | Other (number of prescriptions, duration of supply) | 18 months |
Walton 2001,42 USA, cross-sectional study and cost analysis | Outpatient prescription data from the Veteran Administration’s Chicago Health Care System | To investigate the relative roles that fill quantity, dispensing costs and wasted medication play in the total cost of outpatient prescriptions | Outpatients who are receiving ongoing care and who are new to treatment through Veteran Administration’s Chicago Health Care System | Lipid-lowering agents (statins) | 90-day supply compared with 30-day supply | 16,990 prescriptions | Cost, wastage | 1 year |
Risk-of-bias assessment
Risk of bias was assessed in all 16 included studies based on the criteria presented in the ROBINS-I assessment tool (see Table 24, Appendix 4). 30 In addition, for the three cost analysis studies42,46,49 and the two studies with cost–consequence analysis44,45 the quality was appraised using Drummond et al. 51
The ROBINS-I is a recently published tool which, following careful consideration and pilot testing by reviewers, was considered more appropriate to this review and the nature of the studies included therein than other pre-existing tools (e.g. the Newcastle–Ottawa scale52). Not all of the signalling questions within each domain of bias presented in the ROBINS-I tool were found to be applicable to the studies included in this review. For example, signalling questions relating to participant selection bias were not well suited to retrospective cohort studies, and strictly following the users’ guidance did not appear to appropriately distinguish between the higher and lower quality studies for this criterion. Therefore, we followed the general principles of the ROBINS-I and used the accompanying guidance to critically appraise the risk of bias in each domain, but we did not always apply the full list of questions. We present a summary of the quality appraisal (Table 6) based on the ROBINS-I tool; full details of the double assessment for each study are available on request.
Reference | Bias as a result of confounding | Bias in selection of participants into the study | Bias in classification of interventions | Bias as a result of departures from intended interventions | Bias as a result of missing data | Bias in measurement of outcomes | Bias in selection of the reported result | Overall bias |
---|---|---|---|---|---|---|---|---|
Batal et al. 200739 | Low | Moderate | Low | Low | Low | Low | Low | Moderate |
Domino et al. 201144 | Low | Low | Low | Moderate | Moderate | Low | Low | Moderate |
aFaris et al. 201048 | No information | No information | Low | No information | No information | Low | No information | No information |
aHermes et al. 201035 | Moderate | Moderate | Low | No information | No information | Low | No information | No information |
aJiang et al. 200736 | No information | Moderate | Low | No information | No information | Low | No information | No information |
Murphy et al. 201232 | Low | Moderate | Low | Low | Moderate | Low | Low | Moderate |
bParikh et al. 200146 | No information | Serious | Low | Moderate | Serious | Low | No information | Serious |
Pfeiffer et al. 201237 | Low | Moderate | Low | Low | Low | Low | Low | Moderate |
Rabbani and Alexander 200949 | Low | Moderate | Low | Low | Low | Low | Low | Moderate |
aRyvkin and Garavaglia 200938 | No information | No information | Low | No information | No information | Low | No information | No information |
Schectman et al. 200243 | Moderate | No information | No information | Moderate | No information | Low | Low | Moderate/serious |
Schmittdiel et al. 201547 | Low | Moderate | Low | Moderate | Low | Low | Low | Moderate |
Steiner et al. 199340 | Serious | Moderate | Low | Low | No information | Low | Low | Serious |
Taitel et al. 201245 | Moderate/serious | Moderate | Serious | Moderate | Low | Low | Low | Serious |
aThiebaud and Patel 200641 | No information | Low | Low | No information | No information | Low | No information | No information |
Walton 200142 | Moderate/serious | Moderate/serious | Low | Low | Low | Low | Low | Moderate |
Of the 16 studies, an overall assessment of risk of bias could not be made for the five studies presented as abstracts, as insufficient information was reported on the study methodology used. 35,36,38,41,48 Three studies were considered to be at a serious risk of bias,40,45,46 and the remaining eight studies were considered to be at a moderate risk of bias. 32,37,39,42–44,47,49 In general, these eight studies were found to be sound for a non-randomised study (according to the ROBINS-I assessment tool) but cannot be considered comparable with a well performed randomised trial, so they were considered to be at a moderate risk of bias.
For the majority of studies, potential bias was observed in the selection and classification of participants into the study. For example, in all of the retrospective cohort studies, assignment to the intervention was based on the prescription length that they had received. When studies did not explicitly restrict the inclusion criteria to those patients who were new to treatment, the start of the follow-up period and the start of the intervention did not coincide, so that bias could have been introduced if the length of time that an individual had previously been on treatment varied. It is possible that patients who have been receiving treatment for a longer period of time are more likely to receive longer prescription lengths than those new to treatment.
Given that patients were not randomly allocated to intervention or control there was also the potential for bias as a result of additional underlying differences in patients who received longer or shorter prescriptions. For example, in the retrospective cohort studies, patients who had received longer prescriptions might have been those who were considered by the prescriber to be more adherent. In the majority of cases, the study authors did not explicitly report that they took measures to control for selection bias. In contrast, however, Domino et al. 44 examined the ways in which individuals with longer prescriptions differed from individuals with shorter prescriptions. They found that women, the continuously enrolled, and disabled, older and individuals with more comorbid diseases were more likely to receive longer prescriptions, while minorities and those with greater hospital use during the study period were less likely to fill a longer prescription. The authors used person-specific fixed effects to control for differences.
Two studies were classified as being at a serious risk of bias owing to a small sample size (a criterion that is not considered in the ROBINS-I tool). 40,46 In the study by Parikh et al. ,46 750 patients were randomly selected for inclusion, of whom only 178 agreed to participate. Steiner et al. 40 included only 120 patients, of whom only 27 received shorter prescriptions. The small sample sizes result in uncertainty about the reliability of findings. A third study was classified as being at a serious risk of bias based on the way in which the lengths of prescriptions were classified. Taitel et al. 45 used a cut-off point of 84 days but provided no justification for this choice. This raises concerns that the assignment of the intervention status might have been determined in a way that could have been affected by knowledge of the outcome.
For the five studies that included a cost assessment,42,44–46,49 we assessed the quality using selected aspects of the Drummond assessment criteria that were appropriate to cost analyses or cost–consequences analyses (Table 7). We concluded that, overall, the quality of evidence was low. This was primarily because most studies did not account for uncertainty in the estimates of costs using a sensitivity analysis. Although none of the studies used discounting to adjust for differential timings of costs (to reflect savings rates and the positive rate of time preference), this was justified by the short follow-up. Similarly, no study performed an incremental analysis of costs and consequences of alternative treatment, as no study was a cost-effectiveness analysis. In addition, three of the studies did not allow for uncertainty in the estimates of costs and consequences,44–46 and two others42,49 relied on limited sensitivity analyses to allow for uncertainty. Finally, the limitations of the Parikh et al. 46 study raised by the ROBINS-I tool were confirmed, as it was one of two studies42,46 that did not provide a comprehensive description of the competing alternatives, and the only study in which costs and consequences were not valued credibly and the presentation and discussion of study results did not include all issues of concern to users.
Reference | Was a well-defined question posed in an answerable form? | Was a comprehensive description of the competing alternatives given (i.e. can you tell who did what to whom, where and how often)? | Was the effectiveness of the programmes or services established? | Were all of the important and relevant costs and consequences for each alternative identified? | Were costs and consequences measured accurately in appropriate physical units (e.g. hours of nursing time, number of physician visits, lost work-days, gained life-years)? | Were costs and consequences valued credibly? | Were costs and consequences adjusted for differential timing? | Was an incremental analysis of costs and consequences of alternatives performed? | Was allowance made for uncertainty in the estimates of costs and consequences? | Did the presentation and discussion of study results include all issues of concern to users? |
---|---|---|---|---|---|---|---|---|---|---|
Domino et al. 201144 | Yes | NA | NA | Yes | Yes | Yes | No | No | No | Yes |
Parikh et al. 200146 | Yes | No | NA | Yes | Yes | No | No | No | No | No |
Rabbani and Alexander 200949 | Yes | NA | NA | Yes | Yes | NA | No | No | No (sensitivity analysis looked at patient subgroups) | Yes |
Taitel et al. 201245 | Yes | Yes | NA | Yes | Yes | Yes | No | No | Yes | Yes |
Walton 200142 | Yes | No | NA | Yes | Yes | Yes | No | No | No (sensitivity analysis looked at patient subgroups) | Yes |
Outcomes
One study presented findings of a risk factor for health outcomes,39 nine studies presented data on medication adherence,35–37,39,40,43–45,47 medication wastage was reported in six studies,32,36,38,42,45,48 costs were reported in five studies42,44–46,49 and three studies reported on ‘other’ outcomes. These ‘other’ outcomes were medication persistency,45 number of clinical encounters,37 and number of prescriptions and prescription duration. 41 No evidence was found related to other outcomes of interest. Findings for each outcome are presented in turn below.
Health outcomes and health risk factors
No studies measured health outcomes. Only one of the included studies explicitly measured a risk factor for health outcomes. 39 This retrospective cohort study compared achievement of target cholesterol levels between patients with 60-day prescriptions and those with 30-day prescriptions (overall sample size analysed 3292; sample sizes by group were not reported). Patients in the 60-day group had significantly lower mean final serum cholesterol values than those in the 30-day group [185.3 mg/dl (SD 46.2 mg/dl) vs. 191.5 mg/dl (SD 52.6 mg/dl); p = 0.003]. An attempt was made to contact the study authors to obtain sample sizes, but no data were forthcoming. As a result, we could not calculate an effect size.
Quality assessment
Based on a GRADE assessment, the overall quality of the evidence for risk factors of health outcomes (i.e. cholesterol levels) is low.
Adherence
Medication adherence was reported in nine of the included studies (six retrospective cohort studies,35–37,39,40,45 one pre–post controlled study44 and two cross-sectional studies). 43,47 All measures used to assess adherence were indirect estimates based on pharmacy claims refill data. The most common measures of adherence were the proportion of days covered (PDC) or the medication possession ratio (MPR) (Box 1). When the MPR is used, patients who routinely refill their medications early can have a MPR of > 100% (i.e. the numerator can be greater than the denominator).
PDC is calculated as:
MPR is calculated as:
Measures of adherence were presented as dichotomous (i.e. the number of patients with ≥ 80% or < 80% PDC or MPR) or continuous outcomes (i.e. mean PDC or MPR). One study reported both dichotomous and continuous outcomes. 44 It was possible to calculate effect sizes for six cohort studies based on the information reported in the papers (see Table 8 and Figures 2 and 3). 35–37,39,40,45 The findings are presented for dichotomous and continuous outcomes in turn below and summarised in Table 8.
Reference, study type | Condition(s)/medication(s) evaluated | Adherence measurement (as reported by the study authors) | Duration of study | 90-day supply (unless otherwise stated) | 30-day supply (unless otherwise stated) | Effect size |
---|---|---|---|---|---|---|
Dichotomous outcomes (≥ 80% adherence) | ||||||
Batal et al. 2007,39 retrospective cohort | Lipid-lowering agents (statins) | Pharmacy refills; each patient’s adherence score was calculated as their days of drug acquired divided by their days in the study (days from first prescription fill to last prescription fill). The primary outcome was proportion with ≥ 80% adherence | 3 years | 60-day supply: 1307 out of 2553 (51%) | 303 out of 833 (36%) | OR 0.53 (95% CI 0.45 to 0.62)a |
bDomino et al. 2011,44 pre–post controlled study with a cost–consequences analysis | Antidepressants, antipsychotics, antihypertensives, antidiabetics, seizure disorder medications, lipid-lowering agents (statins) | PDC measure; calculates daily indicators of medication used divided by the number of days in the quarter. The outcome was reported as difference-in-difference-in-differencesc in the percentage of quarters in which individuals had PDC ≥ 80% | 18 months | NR | NR | Statins: –0.132 (0.025), p < 0.01; diabetes: –0.053 (0.017), p < 0.01; antihypertensives: –0.083 (0.006), p < 0.01; seizure disorder: –0.022 (0.014), p = ns; antidepressants: –0.027 (0.021), p = ns; antipsychotics 0.004 (0.018), p = ns |
Hermes et al. 2010,35 retrospective cohortd | Antihypertensives, antidiabetics, cholesterol-lowering agents | PDC (no further details reported). The primary outcome was the proportion with a PDC ≥ 80% | 540 days | Cholesterol-lowering: 5414e out of 7219 (74.9%); antihypertensives: 7928 out of 9405 (84.3%); antidiabetics: 1221 out of 1578 (77.4%) | Cholesterol-lowering: 20,820 out of 31,982 (65.1%); antihypertensives: 41,064 out of 53,192 (77.2%); antidiabetics: 6094 out of 8844 (68.9%) | Cholesterol-lowering:f OR 0.62 (95% CI 0.59 to 0.66); antihypertensives: OR 0.63 (95% CI 0.59 to 0.67); antidiabetics: OR 0.65 (95% CI 0.57 to 0.74) |
Pfeiffer et al. 2012,37 retrospective cohort | Antidepressants | Proportion of patients who received ≥ 180 days of an antidepressant treatment during the 231-day period following the index prescription. The primary outcome was the proportion with > 80% adherence | 7 years | 67,077g out of 87,000 (77.1%) | ‘Less than a 90-day supply’: 123,993 out of 296,634 (41.8%) | OR 0.21 (95% CI 0.21 to 0.22) |
Schmittdiel et al. 2015,47 cross-sectional study | Antihypertensives (ACEIs; ARBs), antidiabetics (oral antihyperglycaemics), lipid-lowering agents (statins) | PDC: the percentage of days in the measurement period ‘covered’ by prescription fills for the same medication or medications in the same therapeutic category. The primary outcome was predictors of adherence modelled as a dichotomous outcome in a Poisson regression model | Up to 1 year | NR | NR | Estimated risk ratio of being adherent (PDC ≥ 0.8) (reference group with < 31 days’ supply):h ACEI/ARB: 61–90 days 1.35; > 90 days 1.61; oral diabetes medications: 61–90 days 1.48; > 90 days 1.61; statins: 61–90 days 1.47; > 90 days 1.61 (p < 0.001 for all) |
Continuous (mean PDC or MPR) | ||||||
bDomino et al. 2011,44 pre–post controlled study with a cost–consequences analysis | Antidepressants, antipsychotics, antihypertensives, antidiabetics, seizure disorder medications, lipid-lowering agents (statins) | PDC measure; as above | 18 months | NR | NR | Statins: –0.080 (0.012), p < 0.01; diabetes: –0.034 (0.008), p< 0.01; antihypertensives: –0.045 (0.002), p < 0.01; seizure disorder: –0.009 (0.006), p = ns; antidepressants: –0.030 (0.010), p < 0.01; antipsychotics: –0.010 (0.008), p = ns |
Jiang et al. 2007,36 retrospective cohortd | Antidepressants (SSRIs), antihypertensives (ACEIs), lipid-lowering agents (statins) | MPR (no further details reported) | 1 year | Mandatory 90 days: 0.7543 (SD not reported)i (n = 148), voluntary 90 days: 0.6895 (SD not reported) (n = 582) | 0.3999 (SD not reported) (n = 955) | MD –0.30 (95% CI –0.58 to –0.03)j |
Schectman et al. 2002,43 cross-sectional study | 42 medications: antihypertensives, antidiabetics, lipid-lowering agents | Number of days of therapy dispensed between first and last refills divided by interval between first and last refills. The primary outcome was predictors of adherence modelled in a multivariable linear regression model. No details were reported as to whether or not MPR was capped at 100% | 9 months | NR | NR | Based on multivariate analysis, each 30-day increment in prescription drug supply (maximum supply was 90 days) was associated with a 5.7% increase in mean adherence (p < 0.0001)k |
Steiner et al. 1993,40 retrospective cohort | Digoxin | The proportion of prescribed dose of maintenance medication obtained. Calculated as the total days’ supply divided by the number of days between the first and last fills | 14 months |
31 to 89 days: 103.6%l (SD 26.6) (n = 41) ≥ 90 days: 113.0%l (SD 21.4) (n = 46) |
89.7% (SD 34.9) (n = 27) | ≥ 90 days vs. ≤ 30 days: MD –0.23 (95% CI –0.38 to –0.09) |
Taitel et al. 2012,45 retrospective cohort with a cost–consequences analysis | Antidepressants (SSRIs), antidiabetics (oral hypoglycaemics), antihypertensives, lipid-lowering agents (statins) | MPR: sum of the days’ supply for each therapeutic class divided by 365, the number of days in the follow-up period | 1 year | Antihypertensives: 0.910 (SD 0.174)m (n = 5835); statins: 0.819 (SD 0.194) (n = 2162); SSRIs: 0.817 (SD 0.196) (n = 266); hypoglycaemics: 0.875 (SD 0.190) (n = 1511) | Antihypertensives: 0.774 (SD 0.292) (n = 33,009); statins: 0.671 (SD 0.278) (n = 12,136); SSRIs: 0.611 (SD 0.295) (n = 7017); hypoglycaemics: 0.775 (SD 0.289) (n = 11,842) | Antihypertensives: MD –0.14 (95% CI –0.14 to –0.13); statins: MD –0.15 (95% CI –0.16 to –0.14); SSRIs: MD –0.21 (95% CI –0.23 to –0.18); hypoglycaemics: MD –0.12 (95% CI –0.13 to –0.11) |
FIGURE 3.
Studies that assessed mean adherence using the MPR or the PDC. a, Steiner et al. 40 compared a 30-day medication supply with a ≥ 90-day supply. IV, instrumental variable.
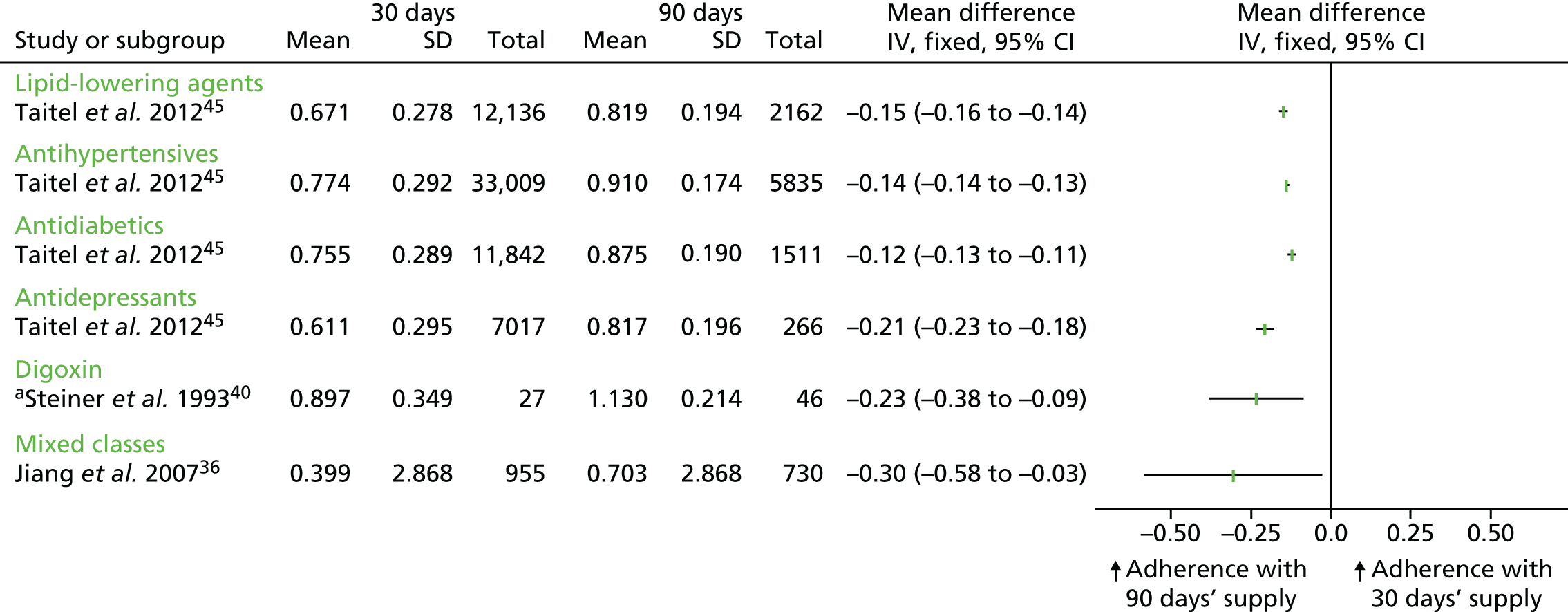
Proportion of days covered and medication possession ratio measured as a dichotomous outcome
Five studies (three cohort studies, one pre–post study and one cross-sectional study) compared the proportion of patients with ≥ 80% or < 80% medication adherence using PDC35,37,44,47 or MPR. 39
Effect sizes (ORs) could be calculated for three of the cohort studies because they reported adherence rates of individuals who received longer prescription lengths and those who received shorter prescription lengths (see Table 8). 35,37,39 These studies presented data for lipid-lowering agents, antihypertensives, antidiabetics and antidepressants. The effect sizes consistently show that longer prescriptions increase medication adherence compared with shorter prescriptions for all four clinical classes (see Figure 2).
Domino et al. 44 compared changes in adherence both before and after the introduction of a policy in one US state (North Carolina) to reduce prescription length from 100 days to 34 days, and another state (Georgia) where the maximum duration of supply remained constant at 31 days. Adherence was expressed as the proportion of quarters per year in which individuals were at least 80% adherent to their target medications. This study found that shortening the prescription length was associated with a reduction in adherence for five of the six clinical classes studied, but the difference was statistically significant only for statins, diabetes and antihypertensive medications (see Table 8). The policy change in North Carolina was stated to have increased the rate at which chronic medication users were non-adherent to their prescribed medication. 44
In a cross-sectional study by Schmittdiel et al. ,47 associations between adherence and health system characteristics, including mean days’ supply of medication (as categorical variables 31–60 days, 61–90 days and > 90 days), were analysed using regression analysis. The authors considered various cardiovascular medications including ACEIs and angiotensin II receptor blockers (ARBs), statins and oral diabetes medications. 47 Across all medications, the strongest health system predictor of achieving greater medication adherence was a longer prescription length (a mean supply of > 90 days) [the relative risk (RR) was 1.61 for each of these medications; p < 0.001] (see Table 8). 47
Proportion of days covered and medication possession ratio measured as a continuous outcome
Five studies (three cohort studies,36,40,45 one pre–post controlled study44 and one cross-sectional study43) evaluated medication adherence using mean values of PDC44 or MPR. 36,40,43,45
Effect sizes (mean differences) could be calculated for the three cohort studies that reported the mean adherence rates of individuals who received longer prescription lengths and of those who received shorter prescription lengths (see Table 8). 36,40,45 These studies presented data for lipid-lowering agents, antihypertensives, antidiabetics, antidepressants and digoxin. The effect sizes consistently show that longer prescriptions increase medication adherence compared with shorter prescriptions for all four clinical classes, and for digoxin (see Figure 3).
The pre–post controlled study by Domino et al. 3 found that shortening the supply of medication from 100 days to 34 days was associated with decreased adherence by 1.5–4.6 percentage points across the six therapeutic classes studied. However, the results for seizure medication and antipsychotics were not statistically significant (see Table 8).
Finally, a cross-sectional study by Schectman et al. 43 examined associations between demographics and prescription factors, including length of medication supply and adherence to medications for diabetes, hypertension and hypercholesterolaemia. Based on a multivariate regression model, the authors reported that each 30-day increment in prescription length (the maximum supply was 90 days) was associated with a 5.7% increase in mean adherence (p < 0.0001) (see Table 8). 43
Pooled meta-analysis
As an exploratory analysis, we combined dichotomous and continuous measures of adherence from six retrospective cohort studies35–37,39,40,45 by pooling SMDs for each therapeutic class and all classifications combined (Figure 4). As ORs or mean differences with 95% CIs (and thus SMDs) could not be calculated for three of the nine studies reporting on adherence, their results are not included in this analysis. 43,44,47 The overall effect size shows that medication adherence was significantly increased in patients who received a 90-day medication supply compared with patients who received a 30-day supply (SMD –0.45, 95% CI –0.65 to –0.26; p < 0.00001), but there was significant statistical heterogeneity between the different studies/comparisons [τ2 = 0.10; χ2 = 2611.51, degrees of freedom (df) = 10; p < 0.00001; I2 = 100%]. Given this heterogeneity, the effect size estimate may not be meaningful, and should, as intended, be considered exploratory.
FIGURE 4.
Combined meta-analysis of studies/comparisons that assessed claims-based medication adherence. The results for different therapeutic classes presented by the same author did not share the same control groups, so they could be pooled as separate studies in the meta-analysis. Batal et al. 39 compared 30 vs. 60 days’ medication supply, Pfeiffer37 compared 90 vs. < 90 days’ supply and Steiner40 compared 30 vs. ≥ 90 days’ medication supply. The analysis is based on six retrospective cohort studies. IV, instrumental variable.
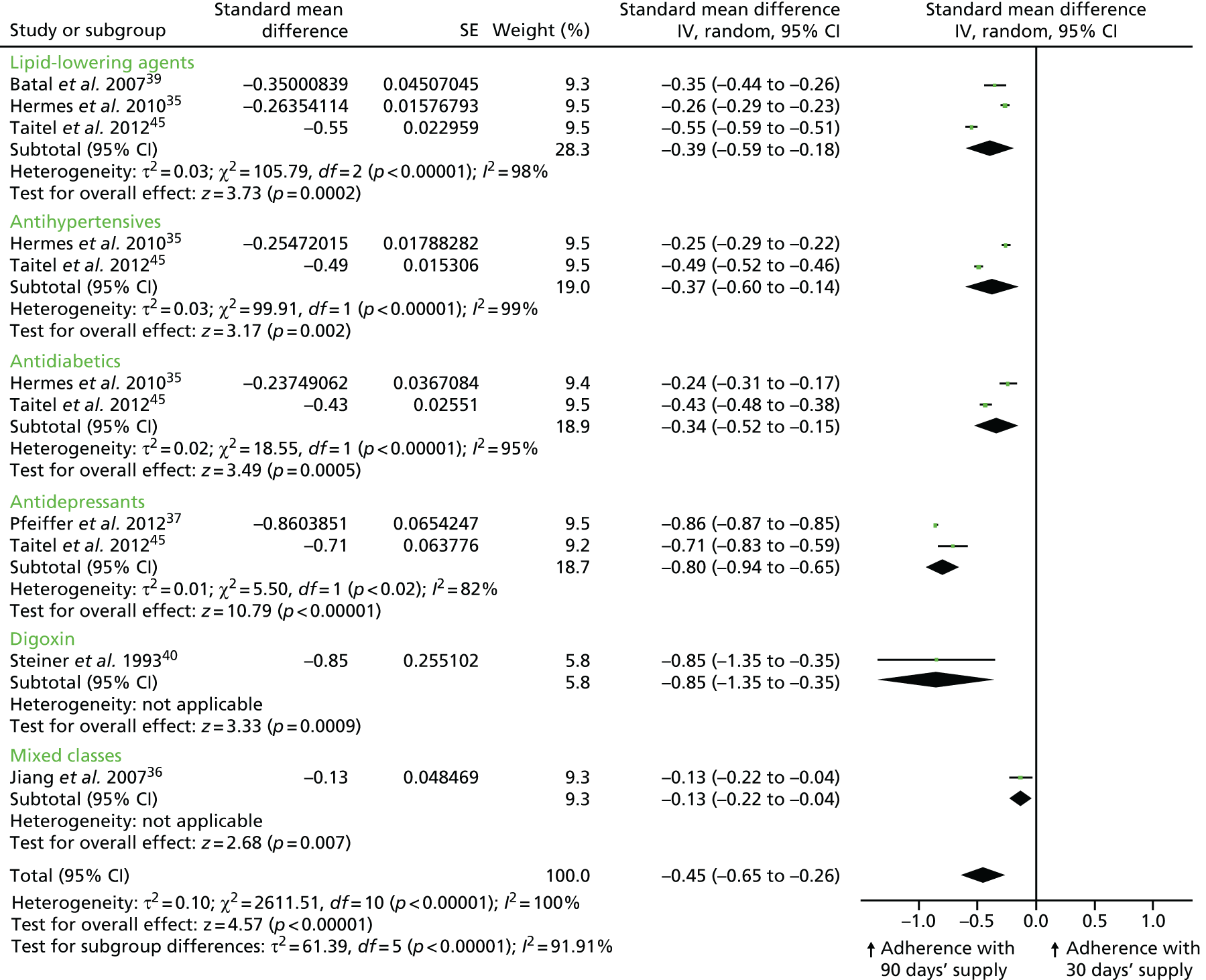
Quality assessment
Based on a GRADE assessment, the overall quality of the evidence for adherence is moderate. This assessment was made because most of the studies that evaluated this outcome were considered to be at only a moderate risk of bias, there was consistency in the direction of effect, the CIs were generally narrow and we did not find any evidence (such as small study effects) that could indicate publication bias. However, there was concern regarding the extent to which the evidence presented is applicable to the UK setting, as all of the studies were conducted in the USA, which has a very different health service structure from that of the UK.
In summary, there is consistent evidence that longer prescriptions increase medication adherence compared with shorter prescriptions. The overall quality of the evidence for this outcome is moderate.
Wastage
Medication wastage was reported in six of the included studies (five retrospective cohort studies32,36,38,45,48 and one cross-sectional cost analysis42). All measures of wastage were indirectly estimated based on pharmacy claims refill data. The majority of these studies defined wastage in a similar manner, such as a ‘switch in medication type within the same clinical class or to the same medication but with a different strength, occurring before the expected refill date’. 32 One study also included discontinuation within its definition. 48
It was possible to calculate effect sizes for four cohort studies,32,36,42,45 based either on information reported in the papers or on data obtained from the study authors. Three different ‘wastage’ outcomes were reported in the included studies: the percentage of days’ supply wasted, the mean number of days’ supply wasted and the percentage of patients who wasted medication. Two studies reported multiple outcomes. 38,42 The findings related to each of these outcomes are presented in turn below and summarised in Table 9.
Reference, study type | Condition(s)/medication(s) evaluated | Wastage definition and calculation (as reported by the study authors) | Duration of study | 90 days’ supply (unless otherwise stated) | 30 days’ supply (unless otherwise stated) | Effect size |
---|---|---|---|---|---|---|
Percentage of days’ supply wasted | ||||||
Faris et al. 2010,48 retrospective cohorta | Medications for multiple sclerosis, rheumatoid arthritis, oncology and growth hormone | Wastage occurred when patients switched medication or stopped taking therapy (drop-off waste). The outcome measured was the proportion of days’ supply wasted, calculated as the sum of switch waste and drop-off waste divided by total days supplied | 21 months | Multiple sclerosis: 2.44%; rheumatoid arthritis: 2.73%; oncology: 1.90%; growth hormone: 4.28% (sample sizes and SDs were not reported) | Multiple sclerosis: 2.55%; rheumatoid arthritis: 3.97%; oncology: 3.42% growth hormone: 3.54% (sample sizes and SDs were not reported) | An effect size was not reported and could not be calculated |
Ryvkin and Garavaglia 2009,38 retrospective cohorta | Antihypertensives, lipid-lowering agents, antiulcers (PPIs) | Defined as a switch within therapeutic class. The outcome measured was the proportion of days’ supply wasted | Not reported | Antihypertensives: 2.0% (sample sizes and SDs not reported); lipid-lowering agents: 1.2% (sample sizes and SDs not reported); PPIs: 0.7% (sample sizes and SDs not reported) | Antihypertensives: 2.1% (sample sizes and SDs not reported); lipid-lowering agents: 0.4% (sample sizes and SDs not reported); PPIs: 0.7% days (sample sizes and SDs not reported) | An effect size was not reported and could not be calculated |
Percentage of patients who wasted medication | ||||||
Ryvkin and Garavaglia 2009,38 retrospective cohorta | Antihypertensives, lipid-lowering agents, antiulcers (PPIs)b | Defined as a switch within therapeutic class. The outcome measured was the proportion of patients who wasted medication | Not reported | Antihypertensives: 5.2% (sample sizes not reported); lipid-lowering agents: 2.9% (sample sizes not reported); PPIs: 1.8% (sample sizes not reported) | Antihypertensives: 6.3% (sample sizes not reported); lipid-lowering agents: 1.2% (sample sizes not reported); PPIs: 1.9% (sample sizes not reported) | An effect size was not reported and could not be calculated |
Taitel et al. 2012,45 retrospective cohort with a cost–consequences analysis | Antidepressants (SSRIs), antidiabetics (oral hypoglycaemics), antihypertensives, lipid-lowering agents (statins) | Defined as a switch of drug type or strength within the same therapeutic class that occurred before the expected refill date. The outcome measured was the percentage of patients who wasted medication | 1 year | Antihypertensives: 712c out of 5835 (12.2%); statins: 212 out of 2162 (9.8%); SSRIs: 39 out of 266 (14.7%); hypoglycaemics: 175 out of 1511 (11.6%) | Antihypertensives: 3928 out of 33,009 (11.9%); statins: 1104 out of 12,136 (9.1%); SSRIs: 975 out of 7017 (13.9%) hypoglycaemics: 1255 out of 11,842 (10.6%) | Antihypertensives: OR 0.97 (95% CI 0.89 to 1.06); statins: OR 0.84 (95% CI 0.72 to 0.98); SSRIs: OR 0.94 (95% CI 0.66 to 1.33); hypoglycaemics: OR 0.90 (95% CI 0.76 to 1.07) |
Walton 2001,42 cross-sectional study | Lipid-lowering agents (HMG CoA reductase inhibitors) | Defined as a switch within therapeutic class. The outcome measured was the proportion of patients that switched who | 1 year | 545 out of 3635 (15.0%) | 1909 out of 13,355 (14.3%) | OR 0.95 (95% CI 0.85 to 1.05) |
Mean number of days’ supply wasted | ||||||
Jiang et al. 2007,36 retrospective cohorta | Antidepressants (SSRIs), antihypertensives (ACEIs), lipid-lowering agents (statins) | Wastage occurred either when patients switched to different medication within the same class or to similar medication of a different strength, so that the patients’ actual days’ supply was less than the dispensed days’ supply. The outcome measured was the total days’ supply wasted among a normalised 30-day period | 1 year | All classes: mandatory 90 days: 2.5 days per 30-day period (n = 148); voluntary 90 days: 2.2 days per 30-day period (n = 582) | All classes: 2.3 days per 30-day period (n = 955) | MD –0.10 (95% CI –0.20 to 0.00)b |
Murphy et al. 2012,32 retrospective cohort | Antidepressants (SSRIs, tricyclics), antidiabetics (biguanides, insulin), antihypertensives (alpha-beta blockers, ACEIs, angiotensin II receptor antagonists, calcium channel blockers, cardioselective beta-blockers, loop diuretics, thiazides), lipid-lowering agents (HMG CoA reductase inhibitors, fibric acid derivatives), thyroid hormones | Wastage was defined as an excess days’ supply of medication resulting from a switch in medication within the same therapeutic class or to the same medication but of a different strength occurring before the expected refill date. The outcome measured was the mean number of days wasted calculated as the sum of the excess days divided by the total number of fills, converted to 30-day equivalents | 1 year | Antidepressants: SSRIs: 0.142 days per 30-day period (SDs not reported) (n = 3337); tricyclics: 0.147 days per 30-day period (n = 456). Antidiabetics: insulins: 0.512 days per 30-day period (n = 546); biguanides: 0.131 days per 30-day period (n = 1938). Antihypertensives: cardioselective beta-blockers: 0.144 days per 30-day period (n = 4353); alpha-beta blockers: 0.202 days per 30-day period (n = 554); calcium channel blockers: 0.156 days per 30-day period (n = 3246); ACEIs: 0.134 days per 30-day period (n = 4786); angiotensin II receptor antagonists: 0.247 days per 30-day period (n = 2224); loop diuretics: 0.100 days per 30-day period (n = 556); thiazides: 0.062 days per 30-day period (n = 1778). Lipid-lowering agents: HMG CoA reductase inhibitors: 0.118 days per 30-day period (n = 10,674); fibric acid derivatives: 0.079 days per 30-day period (n = 1236); thyroid hormones: 0.383 days per 30-day period (n = 4846) | Antidepressants: SSRIs: 0.157 days per 30-day period (SDs not reported) (n = 7969); tricyclics: 0.131 days per 30-day period (n = 1091). Antidiabetics: insulins: 0.281 days per 30-day period (n = 1545); biguanides: 0.082 days per 30-day period (n = 3429). Antihypertensives: cardioselective beta-blockers: 0.087 days per 30-day period (n = 5458); alpha-beta blockers: 0.114 days per 30-day period (n = 934); calcium channel blockers: 0.127 days per 30-day period (n = 4249); ACEIs: 0.102 days per 30-day period (n = 6371); angiotensin II receptor antagonists: 0.117 days per 30-day period (n = 2451); loop diuretics: 0.151 days per 30-day period (n = 1179); thiazides: 0.024 days per 30-day period (n = 2335). Lipid-lowering agents: HMG CoA reductase inhibitors: 0.086 days per 30-day period (n = 10,410); fibric acid derivatives: 0.079 days per 30-day period (n = 1710); thyroid hormones: 0.252 days per 30-day period (n = 6725) | Antidepressants: SSRIs: MD 0.02 (95% CI –0.03 to 0.06);d tricyclics: MD –0.02 (95% CI –0.06 to 0.03). Antidiabetics: insulins: MD –0.23 (95% CI –0.43 to –0.04); biguanides: MD –0.02 (95% CI –0.06 to 0.03). Antihypertensives: cardioselective beta-blockers: MD –0.06 (95% CI –0.11 to –0.01); alpha-beta blockers: MD –0.09 (95% CI –0.20 to 0.03); calcium channel blockers: MD –0.03 (95% CI –0.08 to 0.02); ACEIs: MD –0.03 (95% CI –0.07 to 0.00); angiotensin II receptor antagonists: MD –0.13 (95% CI –0.24 to –0.02); loop diuretics: MD 0.05 (95% CI –0.06 to 0.16); thiazides: MD –0.04 (95% CI –0.07 to –0.00). Lipid-lowering agents: HMG CoA reductase inhibitors: MD –0.03 (95% CI –0.06 to 0.00); fibric acid derivatives: MD 0.0 (95% CI 0.00 to 0.00); thyroid hormones: MD –0.13 (95% CI –0.24 to –0.02) |
Taitel et al. 2012,45 retrospective cohort with a cost–consequences analysis | Antidepressants (SSRIs), antidiabetics (oral hypoglycaemics), antihypertensives, lipid-lowering agents (statins) | Defined as a switch of drug type or strength within the same therapeutic class that occurred before the expected refill date. The outcome measured was the average number of waste days | 1 year | Antihypertensives: 9.211 days (SD 30.284)e (n = 5835); statins: 5.757 days (SD 22.205) (n = 2162); SSRIs: 10.425 days (SD 32.463) (n = 266); hypoglycaemics: 7.899 days per 90-day period (SD 25.385) (n = 1511) | Antihypertensives: 4.037 days (SD 16.236) (n = 33,009); statins: 2.251 days (SD 10.673) (n = 12,136); SSRIs: 3.501 days per 30-day period (SD 12.941) (n = 7017); hypoglycaemics: 3.289 days per 30-day period (SD 13.441) (n = 11,842) | Antihypertensives: MD –5.17 (95% CI –5.97 to –4.38); statins: MD –3.51 (95% CI –4.46 to –2.55); SSRIs: MD –6.92 (95% CI –10.84 to –3.01); hypoglycaemics: MD –4.61 (95% CI –5.91 to –3.31) |
Walton 2001,42 cross-sectional study | Lipid-lowering agents (HMG CoA reductase inhibitors) | Defined as a switch within therapeutic class. The outcome measured was the mean number of days wasted, calculated as the difference between the average quantity dispensed and the average quantity used for each group. If a switch occurred, the quantity used was calculated as the difference between the date that the first prescription was dispensed and the date that the second, different, prescription was dispensed | 1 year | 5.33 days (SD not reported) (n = 3635) | 1.06 days (SD not reported) ‘for each 30-day fill period’ (n = 13.355) | An effect size was not reported and could not be calculated. It is not clear whether or not these results are standardised for the same time period; therefore, it is not clear whether or not the results are directly comparable |
Percentage of days’ supply wasted
Two retrospective cohort studies reported on the percentage of days’ supply wasted. 38,48 Both studies were reported in conference abstracts, and insufficient data were reported to enable an effect size to be calculated. An attempt was made to contact the authors for further information, but no additional data were obtained. Both studies found only small differences in the proportion of days’ supply wasted between patients with different prescription lengths, although neither study reported raw data or statistical comparisons (see Table 9).
Percentage of patients who wasted medication
Three studies (two retrospective cohort studies38,45 and one cross-sectional cost analysis42) reported on the percentage of patients who wasted medication. As none of these three studies stated how wastage per patient was defined, it is not clear whether these patients wasted a small or a large amount of medication.
Effect sizes (ORs) could be calculated for lipid-lowering agents, antihypertensives, antidiabetics and antidepressants based on data presented in two of the studies. 42,45 These data consistently show no statistical differences in the proportion of patients who wasted medication between longer and shorter prescriptions for each of the clinical classes evaluated (Figure 5).
FIGURE 5.
Studies that assessed the percentage of patients with wasted medication. M–H, Mantel–Haenszel.
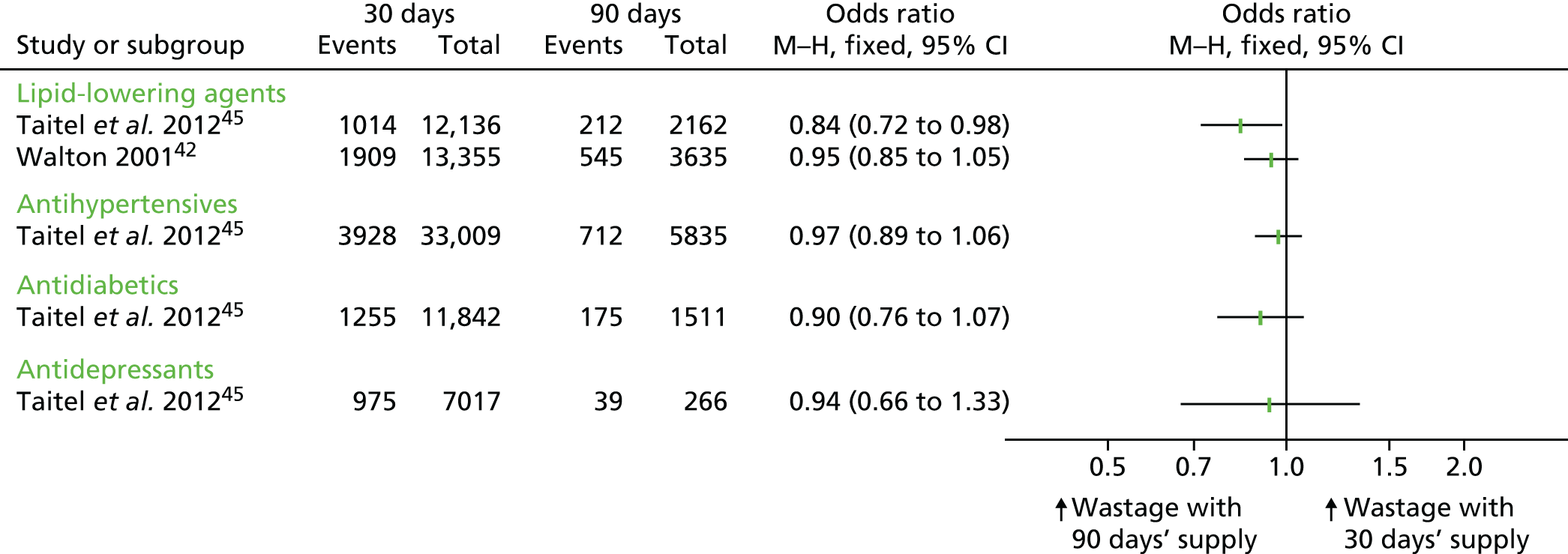
The third study38 reported insufficient data to calculate an effect size; however, similarly to the other studies, the authors found only small differences in wastage between study participants, although no statistical comparisons were reported.
Mean number of days’ supply wasted
Four studies (three retrospective cohort studies32,36,45 and one cross-sectional cost analysis42) measured the mean number of days’ supply wasted over 1 year. Two of the studies used wastage data to inform their cost analysis. 42,45 As such, these two studies appear to present unstandardised means (i.e. the actual mean number of days’ supply wasted over the study period) for each comparison group. The other two studies32,36 investigated wastage as a primary outcome and reported a standardised mean days’ supply (i.e. the mean number of days’ supply wasted per 30 days).
Effect sizes (mean difference) for lipid-lowering agents, antihypertensives, antidiabetics, antidepressants and thyroid hormones could be calculated based on data presented in three of the studies (see Table 9 and Figures 6 and 7). 32,36,45 As Murphy et al. 32 and Jiang et al. 36 report rate data per 30 days, we have presented mean differences from these studies in a separate forest plot (Figure 7) from Taitel et al. 45 (Figure 6).
FIGURE 6.
Studies that assessed mean days with wasted medication. IV, instrumental variable.
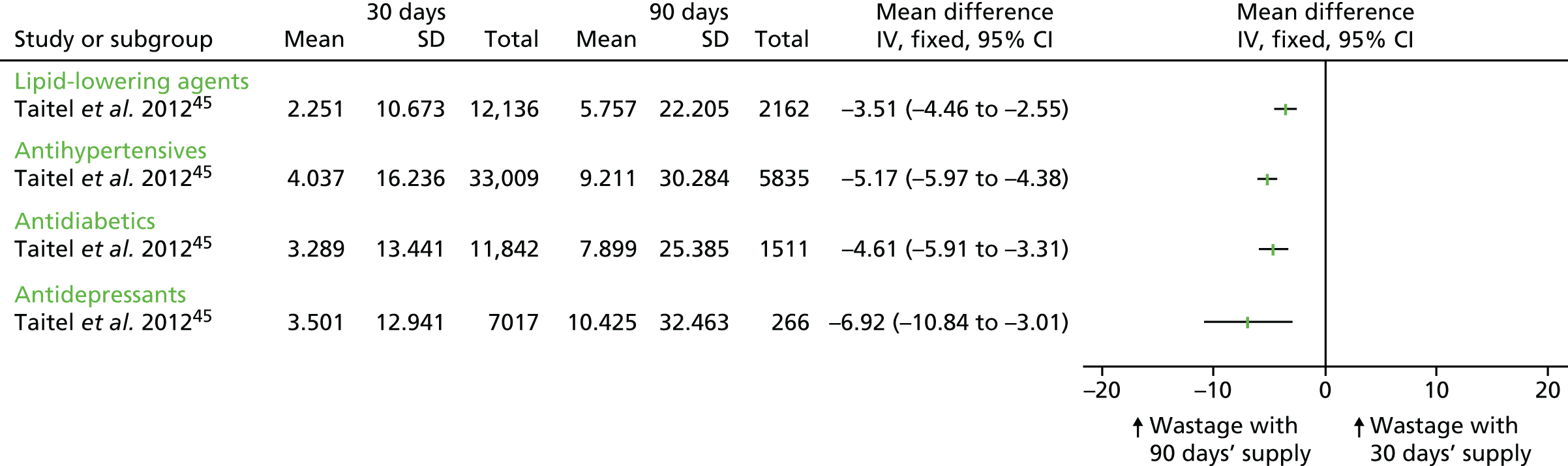
FIGURE 7.
Studies that assessed mean days with wasted medication per 30 days (rate data). For Jiang et al. ,36 the means from the mandatory and voluntary 90-day groups were combined. IV, instrumental variable.
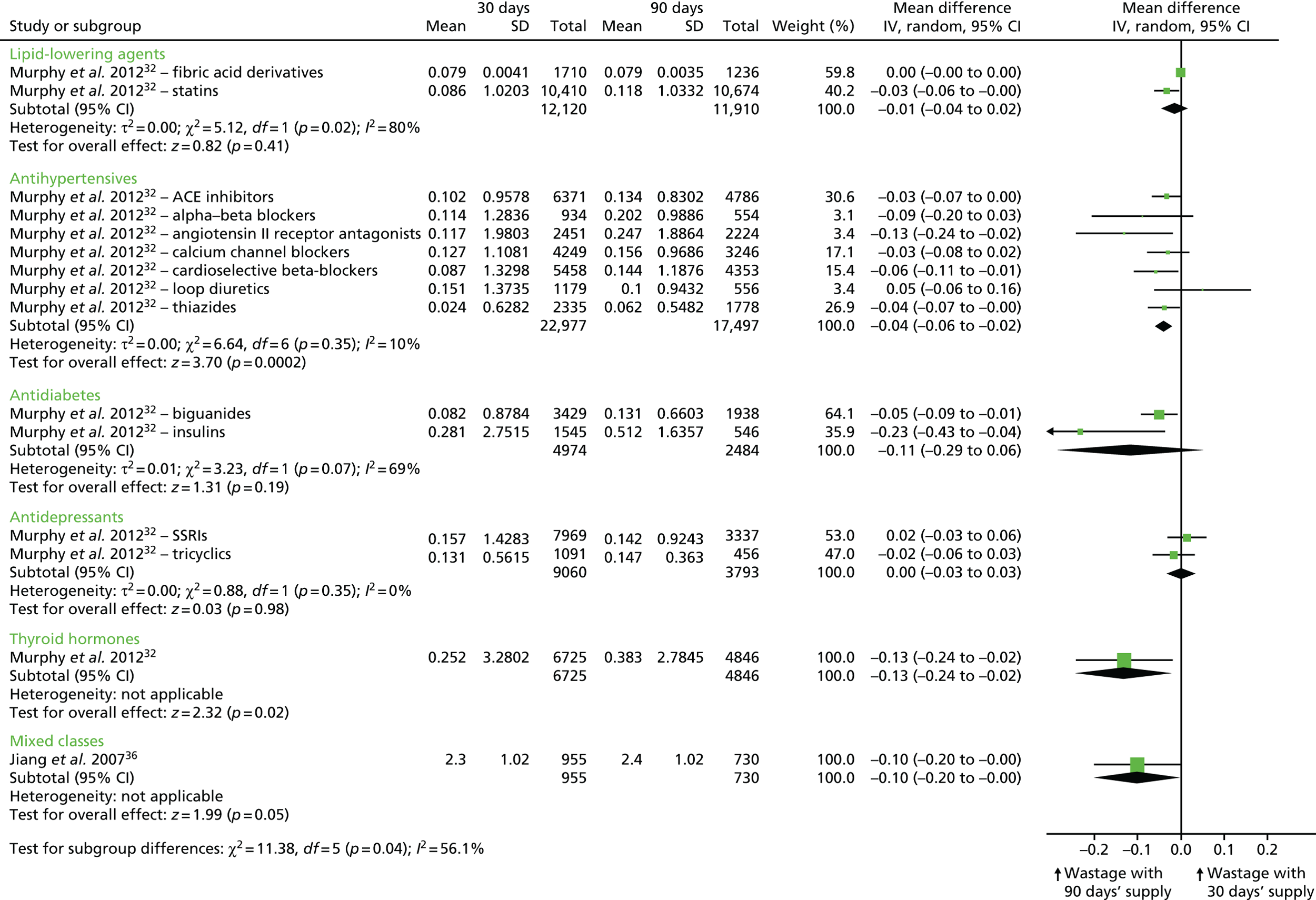
The data from Taitel et al. 45 show that longer prescriptions significantly increase medication wastage compared with shorter prescriptions for all of the clinical classes evaluated (see Figure 6). Jiang et al. 36 reported that the mean number of days with wasted medication was highest for patients who had a mandatory 90-day supply of (any) medication (2.5 days per 30-day period), followed by a 30-day supply (2.3 days per 30-day period), and that the mean number of days with wasted medication was lowest for patients who had a voluntary 90-day supply (2.2 days per 30-day period). However, there was no significant difference between the groups. 36 To estimate the effect size, the two 90-day supply groups were combined, and a SD was imputed based on a p-value of > 0.05. The data show that wastage was higher in patients who received a 90-day supply of (any) medication than in those who received a 30-day supply. 36 The results from Murphy et al. 32 are less consistent: looking across 14 therapeutic classes, the results do not significantly favour one prescription length over the other (see Figure 7). 32
The data presented by Walton42 on wastage are less clear. It appears that wastage of lipid-lowering medication was higher in the 90-day supply group than in the 30-day supply group (5.33 days vs. 1.06 days). 42 However, based on our reading of the data, it is not completely clear whether or not these two sets of numbers are standardised to the same time period and, therefore, it is unclear whether or not they are directly comparable.
Pooled analysis
We conducted an exploratory meta-analysis combining data from three retrospective cohort studies that measured days of medication supply wasted. 32,36,45 The SMDs were pooled for each therapeutic class and for all classifications (Figure 8). This first required pooling data reported by Murphy et al. 32 for each chemical class, and then pooling these results with those from the other studies that reported data by therapeutic class. Mean differences with 95% CIs (and SMDs) could not be calculated for three of the above studies,38,42,48 including two that reported dichotomous data (i.e. percentage of days that medication was wasted), and so the results of these studies are not included in this analysis.
The overall effect size demonstrates that longer prescriptions are associated with a small but significant increase in the mean number of days’ supply wasted (SMD –0.15 days, 95% CI –0.23 to –0.08 days; p < 0.0001), but there was significant statistical heterogeneity between the different studies/comparisons (τ2 = 0.01; χ2 = 382.90, df = 9; p < 0.00001; I2 = 98%). Given this heterogeneity, the effect size estimate may not be meaningful, and should, as intended by the original authors, be considered as exploratory.
Quality assessment
Based on a GRADE assessment, the overall quality of the evidence for ‘wastage’ is very low. This assessment was made because most of the studies that evaluated this outcome did not report enough information to assess quality, although there was consistency in the direction of effect (where calculable), and the CIs were generally narrow. There was some evidence of small study effects, which could be indicative of publication bias. There was also concern regarding the extent to which the evidence presented is applicable to the UK setting, as all of the studies were conducted in the USA, which has a very different health-care system from that of the UK. However, we note that two of the studies used public-funded payers (Medicaid, Veterans Affairs) as their data source. 42,45
In summary, current evidence suggests that the proportion of patients with wasted medication does not differ according to prescription length. However, a longer prescription period (90 days’ supply) shows small increases in the mean number of days’ supply wasted compared with a shorter prescription period (30 days’ supply), although the results were not always significant. The overall quality of this evidence is very low.
Costs
Costs were reported in five of the included studies. 42,44–46,49 Two of the included studies reported cost comparisons alongside changes in adherence, that is, they conducted a cost–consequences analysis. 44,45 The other three studies were cost analyses, as neither adherence nor any other health-related outcomes were reported. 42,46,49
In all of the studies, costs were reported from the perspective of third-party payers (i.e. public or private sector insurers), and in one study out-of-pocket costs paid by the patient were also reported. 49 These out-of-pocket costs were payments made by patients for their prescription drugs, depending on their insurance policy, and did not include any additional costs that might be thought of as out-of-pocket costs in the UK context, such as travel to the pharmacist. Third-party payer costs in all five studies were based on claims data, and included the cost of the medication and the dispensing (or filling) fees, although in two studies the inclusion of dispensing fees had to be assumed as they were not discussed explicitly (e.g. the study by Domino et al. 44 included all health-care expenditure for each patient). 44,49 Two studies also included the mail-out costs associated with mail order prescriptions (which are relatively common in the USA). 46,49 Three studies included the cost of drug wastage,42,45,46 and one study included the costs associated with all health-care expenditure for each patient, as recorded in their Medicaid claims file during the period of observation. 44
All of the studies reported costs in US dollars (US$), typically in the year of data collection. However, Rabbani and Alexander49 adjusted costs to 2005 US$ to account for inflation that occurred during the relatively long period of data collection (2000–5). None of the studies used discounting to adjust for differential timing of costs (in economic evaluation, to reflect savings rates and the positive rate of time preference, it is common practice to place a higher weight on costs that are paid at the present time than on costs that are to be paid some years in the future). The findings related to each of the cost outcomes are presented in turn below and summarised in Table 10.
Reference, study type, setting | Condition(s)/medication(s) evaluated | Source of costs data | Perspective of study | Costs measured | Units | 90-day supply | 30-day supply | Difference (90-day compared with 30-day) | |||
---|---|---|---|---|---|---|---|---|---|---|---|
Sample size | Mean (SD) | Sample size | Mean (SD) | Unadjusted | Adjusted | ||||||
Domino et al. 2011,44 pre–post controlled study with a cost–consequences analysis, USA | Antidepressants, antipsychotics, antihypertensives, antidiabetics, seizure disorder medications, lipid-lowering agents (statins) | Medicaid claims data (obtained from the Centres for Medicare and Medicaid Services) 2000–2 | Third-party payer (Medicaid) | Direct costs (medication costs, ‘before rebates from manufacturer’) | Costs per patient in US$ (year not reported) after 3 monthsa per 3-month period | 1.0 million prescription observations (165,000 unique individuals) | NR | 1.7 million prescription observations (102,000 unique individuals) | NR | NR | bStatins: +US$94.12**; diabetes: +US$114.86**; antihypertensives: +US$75.75**; seizure disorder: +US$155.25**; antidepressants +US$104.61**; antipsychotics +US$257.05** |
Total expenditure on health care as recorded in their Medicaid claims file during the period of observation | As above | As above | NR | As above | NR | NR | cStatins: +US$245.27**; diabetes: +US$348.81**; antihypertensives: +US$270.32**; seizure disorder: +US$288.33**; antidepressants: +US$439.98**; antipsychotics: +US$279.74** | ||||
Parikh et al. 2001,46 cost analysis, USA | Not reported | VASDHS | Third-party payer (VASDHS) | Direct costs (medication costs, dispensing fees and mail-out costs) | Total costs in US$ (assumed 1999) over a 90-day period | n = 178, prescriptions = 346 | US$1394 | n = 178, prescriptions = 346 | US$3530 | –US$2136 (total), equivalent to –US$6.17 per prescription | NA |
Rabbani and Alexander 2009,49 cost analysis, USAd | 395 medications for chronic conditions | 2000–5 Medical Expenditure Panel Survey | Third-party payers (including Medicaid, private insurance and Veterans Affairs) and individuals (out-of-pocket costs) | Direct costs (assumed included medication costs, dispensing fees and mail-out costs) | Monthly costs per patient in US$ (2005) | n = 2971 individuals, prescriptions = 15,060 | US$37.95 (95% CI US$37.26 to US$38.64) | n = 2971 individuals, prescriptions = 11,792 | US$42.72 (95% CI US$42.01 to US$43.42) | –US$4.76** | –US$7.81** |
Individuals only | Out-of-pocket costs | Monthly costs per patient in US$ (2005) | US$15.10 (95% CI US$14.68 to US$15.53) | US$20.44 (95% CI US$19.99 to US$20.89) | –US$5.33** | –US$5.91** | |||||
Taitel et al. 2012,45 retrospective cohort with a cost–consequences analysis, USA | Antidepressants (SSRIs), antidiabetics (oral hypoglycaemics), antihypertensives, lipid-lowering agents (statins) | California Medicaid claims | Third-party payer (Medicaid) | Direct costs [medication costs (including volume discounts), and dispensing fees] and indirect costs (drug wastage) | US$ (assumed 2010) per person per year | Antihypertensives: 5835; statins: 2162; SSRIs: 266; hypoglycaemics: 1511 (prescriptions) | NR | Antihypertensives: 33,009; statins: 12,136; SSRIs: 7017; hypoglycaemics: 11,841 (prescriptions) | NR | Antihypertensives: –US$10.38; statins: –US$7.40; SSRIs: –US$18.48; hypoglycaemics: –US$26.13; overall: –US$13.54 | Antihypertensives: –US$10.80; statins: –US$7.70; SSRIs: –US$18.52; hypoglycaemics: –US$26.86; overall: –US$13.95 |
Walton 2001,42 cross-sectional study; cost analysis, USA | Lipid-lowering agents | VACHCS | Third-party payer (VACHCS) | Direct costs (medication costs and dispensing fees) and indirect costs (drug wastage) | Average cost per patient over 90-day period in US$ (year not reported) | 13,355 prescriptions | US$3.17 (SD NR) | 3635 prescriptions | US$5.62 (SD NR) | –US$2.45 | NR |
Total costs
Four out of the five studies reported lower costs associated with longer prescription lengths than with shorter prescription lengths. 42,45,46,49 Rabbani and Alexander49 examined the cost savings associated with a 3-month supply by analysing individuals who had received both a 1-month and a 3-month supply within the same year for the same drug–dose combination. They reported that the total costs per patient were 18% lower when individuals were prescribed longer prescriptions (mean total monthly cost = US$37.95) than when they were prescribed shorter prescriptions (US$42.72). After controlling for observable differences between individuals (e.g. demographic, health, insurance and economic status), the mean monthly cost saving associated with longer prescriptions was US$7.81. 49
Conversely, the data presented by Domino et al. 44 showed that Medicaid expenditures in all analyses were higher for 90-day prescriptions than for 30-day prescriptions. Longer prescription lengths were associated with higher medication costs [ranging from US$25 (antihypertensives) to US$86 (antipsychotics) per patient per month] as well as with a higher total health-care expenditure [ranging from US$82 (statins) to US$147 (antidepressants)].
None of the five studies reported a breakdown of the third-party payer costs in terms of the relative contribution of the cost of the medication and the dispensing (or filling) fees to the total costs.
Only one study42 used a form of sensitivity analysis to address uncertainty in the accuracy of a model parameter. Three other studies44,45,49 reported some subgroup analyses to examine differences between certain patient groups44,45,49 or medication types. 44,45 Across these four studies42,44,45,49 there were only two specific scenarios in which the observed effect was in the opposite direction to that reported in the main analyses. 42,45 In one scenario, drug costs were based on wholesale drug prices, which were higher than those actually paid by the insurer,42 and, in the other, analysis was restricted to patients who were new to statin therapy. 45 In both cases, shorter prescriptions were associated with lower costs owing, at least in part, to less drug wastage.
Costs borne by patient
The study by Rabbani and Alexander49 reported lower out-of-pocket costs associated with 90-day prescriptions than with 30-day prescriptions. In the main analysis, the average monthly out-of-pocket costs for 90-day prescriptions were US$15.10 (95% CI US$14.68 to US$15.53), compared with US$20.44 (95% CI US$19.99 to US$20.89). These differences were statistically significant and persisted in the subgroup analyses, which examined the types of drugs that patients had been prescribed (generic or branded drugs).
Quality assessment
Based on a GRADE assessment, the overall quality of the evidence for costs is low. This assessment was made because all of the studies were observational studies and there was inconsistency in the direction of effect observed. It is probable that this inconsistency was caused, in part, by variation in the quality of studies. For example, Domino et al. 44 used a robust quasi-experimental study design, whereas Parikh et al. 46 was based on the hypothetical (rather than observed) cost of shorter prescriptions for a sample of patients who were prescribed longer prescriptions. We did not conclude that there was any evidence of publication bias. There was, however, concern regarding the extent to which the evidence presented, which is from the USA, is applicable to the UK setting, particularly as many patients with long-term conditions in the UKare likely to be exempted from prescription charges, either because they are aged > 60 years or because of the nature of their condition. 53
In summary, evidence on the relationship between prescription length and costs is inconsistent between studies. The overall quality of the evidence for the ‘cost’ outcome is low. All studies were US based and are likely to have limited validity in the UK, where many patients with long-term conditions receive free prescriptions.
Other outcomes
Three included studies reported on other outcomes. 37,41,45 Outcomes included medication persistency,45 number of clinical encounters,37 and number of prescriptions and prescription duration. 41
Taitel et al. 45 reported on medication persistency, defined as the average number of days on therapy without a 30-day gap. The authors reported that, across all four clinical classes studied [antihypertensives, statins, selective serotonin reuptake inhibitors (SSRIs) and hypoglycaemics], persistence was 23% higher in the 90-day group than in the 30-day group (p < 0.001). Participants in the 90-day group had, on average, 44 more days on therapy than participants in the 30-day group over the 12-month study period. Controlling for age, gender, number of comorbidities and new-to-therapy status (i.e. patients taking the drug for the first time) did not affect the results. 45 After adjusting for confounders, the greatest difference in medication persistency was observed for the SSRIs group: medication persistency was 32% higher in the 90-day group than in the 30-day group (p < 0.001). 45
In addition to greater adherence among patients with a longer prescription length, Pfeiffer et al. 37 reported that a marginally higher proportion of patients prescribed a longer prescription were more likely to refill their medication at least once than those prescribed shorter supplies (< 90 days group): 84% and 83%, respectively (statistical significance was not reported). In addition, a higher proportion of patients in the shorter prescription group had at least three more clinical encounters than those who received longer prescriptions in the 90 days after initial diagnosis: 24.9% and 13.7%, respectively (statistical significance was not reported).
Finally, a retrospective pre–post study (reported in a conference abstract)41 evaluated changes in the number of prescriptions and the duration of supply following the introduction of 90-day retail dispensing for patients with diabetes, dyslipidaemia or hypertension. 41 The authors reported that 90-day retail dispensing reduced the number of prescriptions patients filled by an average of 1.2 for antidiabetic drugs, 2.2 for lipid-lowering agents and 4.4 for antihypertensives over an 18-month period. Statistical significance of the comparisons was not reported for this outcome. 41 It is not clear whether this refers to a weighted average per prescription or to total numbers in the 18-month follow-up. 41 The authors did not respond to our request for further information that would enable interpretation of this finding.
Quality assessment
Based on a GRADE assessment, the overall quality of the evidence for each of the above outcomes was considered to be very low. There was also concern regarding the extent to which this evidence is applicable to the UK setting, because all of the studies were conducted in the USA, which has a very different health-care system from that of the UK.
Discussion
The systematic review examined the clinical effectiveness and cost-effectiveness of issuing longer versus shorter duration prescriptions in primary care for patients with stable chronic conditions requiring one or more repeat prescriptions in terms of patients’ health outcomes and health systems costs. We identified 16 relevant observational studies, all of which were conducted in the USA. Of the included studies, only one reported data on a risk factor for health outcomes and five reported on costs. The most commonly reported outcome was adherence (variously defined), followed by wastage, both of which were based on pharmacy refill data.
There was very limited evidence on health effects associated with different prescription lengths. One retrospective cohort study, by Batal et al. ,39 found that patients receiving a larger quantity of statins at each fill achieved greater adherence, and that higher adherence was associated with a decrease in final serum cholesterol level. 39 Previous studies have reported an association between increased adherence and improved health outcomes. For example, a cohort study of patients newly treated with statins in Quebec, Canada, found that patients with low adherence were more likely to have coronary artery disease, cerebrovascular disease, and health failure within a 3-year follow-up period. 54,55 More generally, a meta-analysis of 21 studies, involving 46,847 participants with a range of conditions, found a consistent association between increased adherence and a reduction in all-cause mortality, although good adherence was found to be associated with healthy behaviours. 56 Taken together with the moderate-quality evidence from nine studies included in this review35–37,39,40,43–45,47 that longer prescriptions increased medication adherence, this may suggest that increasing prescription lengths could potentially improve clinical outcomes through increased medication adherence.
There was suggestive evidence from one retrospective cohort study of adults newly diagnosed with depression that shorter prescriptions may be associated with higher levels of health-care utilisation. 37 The authors do not suggest a reason for this finding. However, given that they found an association between adherence and prescription length, it could be hypothesised that increased adherence resulted in fewer adverse events and hence fewer clinical encounters. There is also some evidence from the wider literature to suggest that improved medication adherence among patients with chronic conditions may reduce hospitalisation, resulting in a reduction in health-care costs. 54,57–59 Conversely, one pre–post controlled study found that decreasing prescription length was associated with decreased total health-care costs. 44 In this study, health-care costs were reduced as a result of lower adherence to medication. The authors speculated that the changes in adherence may not have been sufficiently large to result in adverse health outcomes, or that follow-up was not long enough to capture spillover to greater use of other services. An earlier simulation model, undertaken by the same authors, conducted prior to the introduction of shorter prescription lengths, predicted that if adherence was unaffected, reducing the days’ supply would result in increased medication expenditures, because the increased pharmacy dispensing fees would outweigh the estimated savings from the reduced wastage of drug products. 22 This finding is in line with the other cost studies, which found that lower costs for third-party payers were associated with longer prescription lengths. 42,45,46,49
Longer prescriptions may result in increased wastage; there was very low-quality evidence from five studies32,36,38,42,45,48 that the proportion of patients with wasted medication did not differ by prescription length, but that a longer prescription period resulted in small increases in the mean number of days’ supply wasted compared with a shorter prescription period. However, overall, longer prescriptions were associated with net savings as a result of greater decreases in pharmacy expenditures through reductions in dispensing fees and drug ingredient costs. 42,45 Despite differences in the scale of dispensing fees between the USA and the UK [US$4.00 (equivalent to £3.83 in 2016)22 vs. £0.9060], similar effects have been found in the UK; improved prescribing efficiencies may reduce the cost of medications to a level at which the dispensing fees for monthly fills are greater than the potential wastage of longer fills. 7,21 These findings are in contrast to earlier evidence that restricting prescription lengths in the UK to 28 days would result in net savings to the NHS owing to decreased medication wastage. 12,13
Only one cost analysis study considered (some of) the costs borne by the patient. 49 This study found that providing a 3-month supply of a prescription drug rather than a 1-month supply reduced patients’ out-of-pocket expenditure by an average of 29%. Changes in prescription duration may also result in savings by reducing the frequency of trips to a pharmacy, which may benefit individuals living in rural areas and those populations for whom access is harder, such as infirm elderly people and people with disabilities.
Limitations of the evidence
All of the studies were conducted in the USA, which has a distinctly different health-care system from that of the UK, with very different (generally higher) costs. Unlike in the UK, where people receiving repeat prescriptions do not pay for their medication and where many people are exempt from prescription fees, in the USA the majority of patients are required by insurance companies to make co-payments. Differences in prescribing systems could also differentially affect adherence and wastage outcomes. For example, in the USA, a 3-month supply of medication can be dispensed through mail order, which may reduce dispensing costs more significantly than in a less automated system,49 although the impact that this has on wastage is not clear. Murphy et al. 32 found less wastage among patients filling retail rather than mail-order prescriptions, although the difference was not statistically significant. Schmittdiel et al. 47 also found greater adherence among individuals who made greater use of mail order to deliver medications.
Furthermore, the study populations evaluated in these US studies may not be directly generalisable to the UK population. Two studies were conducted among Medicaid patients,44,45 who, on average, have lower socioeconomic status than the general population, and four studies were conducted among members of Veterans health-care systems,37,40,42,46 which serve a population with distinctly different demographics and health challenges from those of the general population.
Only one of the included studies39 considered the impact of different prescription lengths on clinical outcomes. Further research is needed on the impact of prescription length on clinical outcomes before any definite conclusions can be drawn.
There is a lack of evidence on patients’ perspectives of different prescription lengths to inform this review, and this aspect needs further research. In a study on patients’ perceptions of, and satisfaction with, a 28-day prescribing policy for thyroid hormone, Mitchell et al. 23 found widespread dissatisfaction. The most common reasons cited for being dissatisfied were the inconvenience of having to pick up the prescription/tablets more frequently, as it interferes with the working day. 23 A qualitative study61 that investigated patients with chronic conditions’ experiences of repeat prescriptions in the NHS found that patients often described shorter prescriptions as a hassle. 61 Respondents reported that managing a repeat prescription was a time-consuming task. In a response to a call for evidence for the DH’s review of prescription charges for those with chronic conditions, Katherine White (chair of the Addison’s Disease Self-Help Group) describes the 28-day prescription policy as disempowering, claiming that it causes anxiety for patients when they are running low on a prescription, and constrains their ability to travel. 62
None of the included studies considered whether or not changing prescription lengths may affect non-pharmacological interventions, for example a physician’s ability to monitor a condition and explain non-pharmacological management issues. The impact of prescription length on clinician–patient relationships warrants further investigation.
All of the studies used indirect measures to assess adherence. The two key measures used were PDC and MPR. These proxy measures may introduce bias in favour of longer prescriptions. For example, if one patient receives a 90-day supply of medication and another is prescribed a 30-day supply, but both stop taking their medication after 14 days, at 90 days pharmacy refill adherence may be calculated as 100% for the first patient and 33% for the second patient. It is also possible that results may differ by the type of measure used, as the PDC has been found to provide a more conservative estimate of adherence than the MPR, and thus may underestimate effectiveness compared with the MPR. 63
Furthermore, refill adherence may not reflect actual medication adherence, as it assumes that medication is being taken exactly as prescribed. 64,65 For example, a patient who takes half of the recommended daily dose of their medication for all days in a month will have the same PDC as a patient who takes the correct daily dose for only half the days in the month (PDC = 0.5 in both cases). 44 In addition, refill data do not capture instances of patients self-discontinuing by not refilling their prescriptions. A review of methods for assessing refill compliance, however, has determined that indirect measures can nevertheless be ‘a useful source of compliance information’. 66
None of the studies explored reasons why adherence may differ between prescription lengths. Reasons for medication non-adherence are often complex. Non-adherence can be either intentional (e.g. when a patient actively deviates from the treatment regimen stemming from their health beliefs) or non-intentional (e.g. passive deviation as a result of carelessness or forgetfulness). 67 Some study authors have suggested that longer prescription lengths may facilitate greater adherence, as longer prescriptions overcome barriers to non-intentional adherence such as enabling patients to follow a regular medicine regimen39,40 and reducing logistical barriers such as trips to the pharmacy. 43 However, all of the included studies were observational, and no participants were randomly assigned to the different prescription durations. As such, the majority of studies are at risk of selection bias owing to systematic differences between patients who received a longer or shorter prescription. Pfeiffer et al. 37 reported that patients who received a 90-day medication supply were more likely to be treated in primary care settings and to have fewer prior or subsequent outpatient visits, suggesting that patients who receive longer prescriptions may have more stable illnesses. Rabbani and Alexander49 also found that men were more likely to receive longer prescriptions than women, and that white patients were more likely to receive longer prescriptions than patients from other ethnic backgrounds.
It was not clear from the evidence if differences in drug item prices had an impact on the relationship between prescription lengths and costs to the health system. Parikh et al. 46 restricted their analysis to the less expensive medications (no more than US$1.50 per day). The authors comment that, for this reason, cost savings might not be generalisable to more expensive medications the costs of wastage of which would have been more substantial. This resonates with a UK commentary in which Hickey et al. 68 argue that for lower-cost medication such as levothyroxine tablets (which are commonly used to treat hypothyroidism) with a standard dispensing fee of £0.90 per item, the NHS could save > £7M per year if 28-day prescribing were replaced with 4-monthly prescriptions.
None of the studies considered time spent and cost borne by the prescriber, administrator or pharmacist to write, process and deliver the prescription, although dispensing fees, which may be seen as paying for that time/cost, were sometimes taken into account. More research is needed to understand the impact of prescribing policy on professionals’ workloads and incomes.
Six out of 16 included studies were commissioned or funded by private providers, pharmacies or insurers (i.e. Walgreens,32,36,45 Accredo Health Group,48 Prime Therapeutics LLC35 and Kaiser Permanente47). It is possible that such funding may have had an influence on the formulation of the research questions, and, to some extent, on the findings. For example, it may have introduced a publication bias and had an impact on the choice of outcomes in favour of wastage or costs over clinical outcomes.
The majority of studies were retrospective cohort studies based on secondary analysis of administrative data. The use of administrative data limits the quality of the analysis, as there is no control over the types of patients included in the dataset, and we cannot validate how the data were collected and recorded. 69 As such, underlying confounding as a result of selection bias limits the strength of the conclusions that can be drawn. The negative consequences of reliance on cohort data are highlighted by research into hormone replacement therapy, with early evidence based on cohort studies later disproved; the study population was healthier, better educated and from higher socioeconomic backgrounds and had better access to health care than the control group. 70
Limitations of the review
Although we followed rigorous systematic review methodology, it is possible that some bias may have been introduced during the review process. For example, some changes to the protocol were made, including the decision to exclude studies that did not evaluate patients with non-chronic diseases, such as patients receiving repeat prescriptions for contraception. This decision was made because of the higher than expected number of studies that evaluated patients with chronic conditions, which were directly applicable to our systematic review research questions. It is possible, however, that some valuable data may have been missed through making this decision. In addition, we stated in the protocol that we would include only patients treated in primary care settings, but then decided to include some studies in which the setting was unclear, or in which some patients may have received treatment in an outpatient setting. We made this decision to be inclusive but, as a result, some of these studies may not be directly applicable to primary care settings. We note, however, that the results from all of the studies appear to be consistent regardless of setting.
It is also possible that we may have missed evidence by restricting the inclusion/exclusion criteria to studies that evaluated 28-day versus 3-month prescriptions, or close to these prescription lengths. Studies were identified in the literature that evaluated other prescription lengths (e.g. Wong et al. 24), and data from different prescription lengths could have helped to inform a model that evaluates an optimal prescription length.
We decided to use the ROBINS-I quality assessment tool, a very recent tool that was published in 2016. This tool did not capture some biases specific to retrospective studies, such as whether or not there was bias in the selection of the study cohort, and for this reason we used the accompanying guidance to critically appraise the risk of bias in each category, but did not always apply the full list of questions. However, in our study, the results obtained using this tool were similar to those obtained with other quality assessment measures (e.g. the Newcastle–Ottawa scale52). It appears that the GRADE quality assessment, in any case, appropriately reflected the overall evidence, and we are confident in these assessments.
While conducting this review, we also found that some studies included only patients who were new to treatment, or presented subgroup results for patients who were new to treatment compared with those who had existing prescriptions. Other studies did not report this information. We did not distinguish between these population groups in our analyses because this distinction was not considered at the protocol stage, and because there were not enough studies that reported this information to carry out a post hoc subgroup analysis. It would be helpful if future studies reported this type of information to facilitate analysis.
In this review, a number of the included studies presented data by type of chemical class or by therapeutic class. We did not attempt to compare across therapeutic class, as this was not the remit of the review and direct comparisons between the effect sizes for different therapeutic classes cannot be made. It does appear that there may be some differences between therapeutic classes, and this merits further investigation.
Finally, for six35,36,38,41,46,48 out of the 16 included studies, only an abstract or extended abstract was available and, despite our attempts to contact the authors, no further publications were available. Full peer review of the quality of the studies was not possible for five of the abstracts,35,36,38,41,48 and the lack of detailed findings from these six studies limits the overall conclusions that can be drawn. The decision to include abstracts was based, in discussion with our expert advisors, on the paucity of high-quality data identified and our decision to be as inclusive as possible.
Chapter 3 Cost analysis based on available secondary data
Introduction
We undertook a cost analysis of Clinical Practice Research Datalink (CPRD) data to estimate the cost of medication wastage associated with shorter and longer prescriptions lengths for five case study conditions. 71 In order to estimate the net cost impact of shorter and longer prescriptions lengths, the costs of dispensing fees and prescribers’ time to issue a prescription were also assessed.
Clinical Practice Research Datalink is a large, longitudinal, primary care data set, comprising routinely collected, anonymised, electronic health record data from GPs within the NHS in the UK. 72 Data are collected from > 650 general practices and include > 79 million person-years of follow-up, representing approximately 7% of the UK population. Comparisons between the UK census and CPRD have shown that patients within the data set are broadly representative of the UK population in terms of age, sex and ethnicity,72,73 but may not be representative of all practices in the UK based on geography and size. 74 Information captured broadly includes demographics, clinical events (symptoms, diagnoses), specialist referrals, immunisations, laboratory tests and prescription details. Data are also quality checked for continuity and completeness at both the patient and the practice level. CPRD has been extensively used for research purposes, including numerous pharmacoeconomic studies. 75
This chapter is structured as follows: the first section sets out the objectives of the cost analysis (see Objectives), the second section describes our methods (see Methods), and our findings are presented in the third section (see Results); these are then discussed in the final section (see Discussion).
Objectives
The objective of the cost analysis was to estimate differences in the costs of medication wastage and the additional impact of assessing the costs associated with dispensing fees and prescriber time in patients receiving medications within the NHS in the UK as either short or long prescription lengths for five common chronic conditions.
Methods
This retrospective multicohort study evaluated medication wastage and the associated cost of wastage, dispensing fees, and the cost associated with the time needed to issue a prescription [i.e. how long it takes a GP to complete the technical process of producing a prescription (note that this does not include clinical decision-making time or administrative staff time)] in five, condition-specific, random samples of 50,000 patients each, obtained from CPRD. The five samples were derived from all adult patients (aged ≥ 18 years) receiving one or more prescriptions relevant to a case study condition of interest (Table 11) during the 11-year period between 1 January 2004 and 31 December 2014. The five full samples (i.e. all relevant prescriptions for each of the five conditions over the 11-year study period available in CPRD) were restricted to patients with complete data for two variables [numeric daily dose (ndd) and quantity (qty)] required for the analysis to create five limited samples. The ndd variable is derived using a CPRD algorithm on common dosage text strings, and has the potential to be equal to zero in cases where the dosage text string is non-specific or otherwise impossible to accurately quantify (e.g. ‘apply as needed’). The qty variable refers to the total quantity intended to be dispensed for that specific prescription. The analysis was, therefore, limited to patients with complete data (i.e. ndd is not missing or equal to zero and qty is not missing or equal to zero). This approach has been employed in other similar CPRD studies using these two variables. 77,78 Random samples of 50,000 patients from these limited samples were then taken to form the five main analysis samples (Table 12). Product codes (CPRD unique code for treatment selected by the GP) associated with relevant prescriptions used in the treatment of five case study conditions were used to select the patients to be included in the samples. The five conditions of interest were:
-
glucose control with oral drug therapy in type 2 diabetes mellitus (T2DM)
-
treatment of hypertension in T2DM
-
treatment with statins (lipid management) in T2DM
-
treatment for the secondary prevention of myocardial infarction
-
depression.
Condition | Relevant prescriptions |
---|---|
(1) Glucose control with oral drug therapy in T2DM | Patients receiving one or more prescriptions for any oral antidiabetic drug listed under BNF section 6.1.2, Antidiabetic drugs,76 in any year from 2004 to 2014 |
(2) Treatment of hypertension in T2DM | In addition to receiving an oral antidiabetic drug as defined in (1), patients receiving one or more prescriptions for any ACEIs, angiotensin II receptor antagonists, calcium channel blockers, beta-adrenoceptor blockers, alpha-adrenoceptor blockers, potassium-sparing diuretics and/or thiazide-like diuretics in any year from 2004 to 2014 |
(3) Treatment with statins (lipid management) in T2DM | In addition to receiving an oral antidiabetic drug as defined in (1), patients receiving one or more prescriptions for any statin in any year from 2004 to 2014 |
(4) Treatment for the secondary prevention of myocardial infarction | In addition to receiving concurrenta prescriptions for an ACEI, antiplatelet and statin for a duration of ≥ 1 year, patients receiving one or more prescriptions for any beta-adrenoceptor blockers and/or angiotensin II receptor antagonists in any year from 2004 to 2014 |
(5) Depression | Patients receiving one or more prescriptions for any antidepressant drug listed under BNF section 4.3, Antidepressant drugs,76 in any year from 2004 to 2014 |
Condition | Full sample (patients) | Full sample (obs) | ndd = 0 or qty = 0 (patients) | ndd = 0 or qty = 0 (obs) | Limited samplea (patients) | Limited samplea (obs) | Random sample (patients) | Random sample (obs) | Dropped prescription errorb (obs) | Final sample (obs) | Same day switches not wastagec (obs) |
---|---|---|---|---|---|---|---|---|---|---|---|
Glucose control with oral drug therapy in T2DM | 310,391 | 21,091,529 | 170,967 | 4,518,765 | 139,424 | 7,135,397 | 50,000 | 2,577,282 | 6483 | 2,570,799 | 548,850 |
Hypertension in T2DM | 230,760 | 23,886,597 | 63,802 | 1,446,199 | 166,958 | 16,041,452 | 50,000 | 4,803,444 | 3983 | 4,799,461 | 1,588,921 |
Lipid management in T2DM | 242,741 | 13,388,759 | 36,577 | 776,718 | 206,164 | 11,216,086 | 50,000 | 2,718,216 | 913 | 2,717,303 | NA |
Secondary prevention of myocardial infarction | 208,682 | 44,151,527 | 87,281 | 3,270,504 | 121,401 | 24,479,014 | 50,000 | 10,131,377 | 767 | 10,130,610 | 5,856,361 |
Depression | 1,207,523 | 32,744,994 | 424,446 | 4,438,319 | 783,077 | 15,712,941 | 50,000 | 1,010,463 | 3234 | 1,007,229 | 12,401 |
These conditions were selected for study based on their chronic nature, the frequency of their occurrence in the population and the potential for a variety of expected frequencies in prescription changes over the course of their treatment.
Relevant prescriptions for each of the five conditions were first identified based on a review of NHS clinical guidelines and the BNF. 79–82 Product code lists were then developed by one author (BD) using CPRD Research Applications Code Browser Version 3.0.0 (CPRD and Medicines and Healthcare products Regulatory Agency, London, UK) and subsequently assessed for accuracy, completeness and clinical relevance by one of the study authors (RP), who is a GP. The definitions of the relevant prescriptions and product code lists of the potentially prescribed medications for each of the five case study conditions are provided in Table 11 and Appendix 5, respectively.
Treatment patterns evaluated
Treatment patterns were evaluated in each of the five cohorts in a similar manner. Data for each patient were first ordered in sequence from earliest to latest prescription date. To identify treatment patterns, three main variables were used: (1) product code (CPRD unique code for treatment selected by the GP; this is unique to individual brands, formulations, strengths, etc.); (2) drug substance code (derived from ‘drugsubstance’ variable and used to identify different dosages and/or formulations of the same drug chemical substance); and (3) drug class code [derived from ‘bnfcode’ variable and used to identify drugs with different chemical compositions, but a similar mechanism of action based on their categorisation in the BNF (e.g. SSRIs)]. Next, four different types of treatment patterns that may occur in a sequence of prescriptions were identified for each patient over the course of their available follow-up, namely:
-
refills of the same product
-
product, drug substance and drug class codes are the same for two prescriptions in sequence, for example two prescriptions for metformin 500-mg tablets
-
-
substitutions between different dosages or formulations of the same drug substance
-
product codes are different, but drug substance and drug class codes are the same for two prescriptions in sequence, for example a switch in dosage for the same statin (atorvastatin 10-mg tablets to atorvastatin 40-mg tablets)
-
-
substitutions between drugs that are in the same class
-
product and drug substance codes are different, but drug class codes are the same for two prescriptions in sequence, for example a switch from one statin (atorvastatin 40-mg tablets) to another statin (simvastatin 40-mg tablets)
-
-
substitutions between drugs that have similar clinical indications from different classes (e.g. different drugs used in the secondary prevention of myocardial infarction)
-
product, drug substance and drug class codes are different for two prescriptions in sequence, for example a switch from an ACEI (captopril 25-mg tablets) to calcium channel blocker (amlodipine 10-mg tablets).
-
The volume of medication wastage from early refills and treatment switches (defined as a repeat prescription or new prescription based on the mapped substitutions outlined above, respectively, being issued prior to the expiry of the previously prescribed quantity) was estimated for prescriptions within the 11-year study period (i.e. any prescriptions not falling within this time period were excluded from the analyses). This was done by dividing the total qty entered by the GP for the prescribed product by the ndd prescribed for the event and comparing this with the difference in the two dates associated with the event and the next prescription in the sequence. Prescriptions issued on the same day for drugs in the same class and with different product codes (e.g. two different statins) were considered prescriber error and dropped from the analysis (see column 9 in Table 12). The one exception to this was for antiplatelet drugs in the secondary prevention of myocardial infarction cohort, as it was assumed that two different antiplatelet drugs could be prescribed at the same time. In addition, prescriptions for medications with similar clinical indications from different classes issued on the same day were counted not as a switch, but rather as an add-on to existing therapy or concomitant therapy (see column 11 in Table 12).
Analysis of medication wastage
As treatment patterns were assessed in sequence, there was the potential to overstate the amount of wastage that occurred. For example, counting every overlap of prescription days associated with refills of the same product as wastage (i.e. even prescriptions issued 1 day before the expiry of the previous prescription would be counted as 1 day’s worth of medication wastage, and two prescriptions issued on the same day would count as one prescription as entirely wasted) may overstate actual wastage. A threshold of 1 year after the initial prescription in a particular series was, therefore, used to estimate wastage for early refills (i.e. repeat prescriptions), in that any product prescribed over and above the expected quantity to be consumed within the 1-year time period was considered waste. This is to account for the fact that patients may fill their prescriptions before their existing supply is exhausted, but still consume all of the previous prescription before using the new supply.
In addition, although excessive switching of drugs could appear to be wastage, consistent patterns could suggest a valid, prescribed treatment regimen. To avoid the overestimation of wastage, additional effort was made to differentiate between add-ons/concomitant therapy and switches for medications with similar clinical indications from different classes (i.e. product, drug substance and drug class codes are different for two prescriptions in sequence). Generally, if the difference between the number of changes between medications with similar clinical indications from different classes and the number of unique drug classes within an annual period was ≥ 1, then any overlap in prescription dates was not considered wastage as a result of a switch, but rather as an add-on or concomitant therapy. For example, within an annual period, a patient may have six changes between clinically related drugs from different classes, but these changes are only between two different drugs from different classes (i.e. two unique drug classes). As six minus two is greater than one, these changes were considered not switches, but rather add-on/concomitant prescriptions. The rationale for this was that, if the number of changes was large, but the number of unique drugs involved in the changes was low, an add-on or concomitant therapy was being prescribed, whereas if the number of changes was large and the number of unique drugs involved in the changes was also large, switches in therapies were occurring and therefore there was the potential for wastage to occur. A similar approach was applied by Taitel et al. ,45 and theirs is the only previous study that has attempted to differentiate between add-on/concomitant prescriptions and actual switches in therapy. Additional constraints in counting overlaps in dates for two prescriptions in sequence for medications with similar clinical indications from different classes were also applied in three of the case study conditions owing to the potential for a number of the included therapies to be given concomitantly. For the glucose control in T2DM cohort, overlaps in prescription dates involving metformin with drugs from other classes were not counted as switches (and therefore wastage), as metformin is usually administered in combination with other classes of oral antidiabetics. 79 For the treatment of hypertension in T2DM cohort, overlaps in prescription dates involving ACEIs and angiotensin II receptor antagonists with either calcium-channel blockers or thiazide-like diuretics were not counted as switches, as these therapies are commonly administered together as second-line therapy. 76 Finally, for the secondary prevention of myocardial infarction cohort, only overlapping prescription dates involving beta-blockers and angiotensin II receptor antagonists were counted as switches, as patients are likely to receive the other classes of drugs included in the analysis (ACEIs, antiplatelet drugs and statins) continuously over the course of treatment. 81
Costs
To estimate the costs of wastage, defined daily doses (DDDs) associated with each drug substance code in the five cohorts were first obtained from the World Health Organization’s Anatomical Therapeutic Chemical (ATC)/DDD Index 2016. 83 The NHS Business Services Authority’s prescription cost analysis (PCA) 2015,84 which provides details of the quantity of individual doses and NICs of all the prescriptions in England, was then used to determine a NIC/quantity value of a specific strength of the medication associated with each drug substance code. This value was then standardised using the associated DDD to obtain a cost per day for each drug substance code in all five of the cohorts. Details of these calculations are provided in Appendix 6.
The dispensing fees from the Drug Tariff [£0.90 per standard prescription and 2% of the cost per prescription (cost per day multiplied by prescription length) for prescriptions over £100]84 and the estimated cost for a physician to issue a prescription based on the literature were then determined for each prescription. A targeted literature review was designed (see Appendix 7 for search strategy and study selection details) to determine the time involved for a physician to issue a prescription (i.e. how long it takes a GP to complete the technical process of producing a prescription; note that this does not include clinical decision-making time or administrative staff time). It should be noted that none of the identified studies from the targeted literature review reported the time involved for a physician to issue a prescription from a UK-specific primary care context. It was therefore necessary to prioritise the use of the available evidence for this parameter based on studies with the largest sample sizes and those studies reporting prescriber time for different types of prescriptions (e.g. new vs. renewals). Refills were assigned a shorter time than changes in dose/formulation, within drug classes and between drug classes (48.7 seconds vs. 61.2 seconds). 85 Per minute costs related to GPs’ time (£3.80/minute), derived from the Personal Social Services Research Unit’s (PSSRU’s) Unit Costs of Health and Social Care, were then applied. 86 All costs are reported in 2015 Great British pounds (GBP).
Statistical analysis
Descriptive analyses of trends in treatment switching and early refills were used to assess medication wastage. The proportion of days’ supply wasted, the mean number of days’ supply wasted and the mean costs of wastage per prescription were determined for two prescription lengths (< 60 and ≥ 60 days, used to proxy 28-day and 3-month prescriptions, respectively) over the 11-year period (2004–14). The mean cost of wastage per prescription was reported for each of the four treatment patterns individually over the 11-year study period and combined for each annual period. Two-sample t-tests using groups (prescription lengths < 60 days and ≥ 60 days) assuming unequal variance were used to compare the differences between the < 60 days and ≥ 60 days groups. This statistical test was chosen despite the potential for skewed data (i.e. costs) because our interest was in testing the arithmetic means and not other characteristics of the distribution (e.g. log transforming data tests for equality of geometric means).
To determine and compare the total unnecessary costs (TUCs) (costs of medication wastage, dispensing fees and prescriber time) associated with prescription lengths < 60 days and ≥ 60 days, a model originally used by Walton42 was adapted and applied to the prescription data from the five cohorts. The model incorporates the quantity (Q) of medication wastage (where Qdays wasted = Qdays supplied – Qdays used) and the cost (C) of medication wastage (where Cwastage = Cdrug/day × Qdays wasted) as well as the cost of dispensing a prescription (Cdispensing) and the cost of prescriber time associated with issuing a prescription (Cprescriber time) to estimate the TUC. To compare the TUCs between different prescription lengths (< 60 and ≥ 60 days), it was necessary to standardise the time period over 90 days. The TUCs for the two prescription lengths were then calculated using the two equations below:
The terms (90/Qdays used < 60) and (90/Qdays used ≥ 60) standardise the total costs over a period of days (90 days) and Cdispensing and Cprescriber time are subtracted to reflect that one dispensing fee and prescriber time costs for one prescription will be incurred for each set period of days (90 days). Each value used in the equations represents a mean calculated for the entire group of patients for each prescription length in each of the five cohorts over the 11-year study period. Mean values for the base case analysis are reported in Table 13. An example scenario is provided in Box 2 to highlight how the model compares the TUCs for prescription lengths < 60 days and ≥ 60 days over a standardised period of 90 days.
Parameters | Glucose control with oral drug therapy T2DM | Hypertension in T2DM | Lipid management in T2DM | Secondary prevention of myocardial infarction | Depression | |||||
---|---|---|---|---|---|---|---|---|---|---|
< 60 days | ≥ 60 days | < 60 days | ≥ 60 days | < 60 days | ≥ 60 days | < 60 days | ≥ 60 days | < 60 days | ≥ 60 days | |
Mean days used (Qdays used) | 31.61 | 75.72 | 32.51 | 86.91 | 32.74 | 122.71 | 31.41 | 102.28 | 27.43 | 65.03 |
Mean days wasted (Qdays wasted) | 0.86 | 4.96 | 1.23 | 6.98 | 0.63 | 16.21 | 0.96 | 6.56 | 1.87 | 2.69 |
Mean drug cost per day (£) (Cdrug/day) | 0.36 | 0.28 | 0.084 | 0.082 | 0.10 | 0.10 | 0.074 | 0.068 | 0.14 | 0.11 |
Mean dispensing fee cost (£) (Cdispensing) | 0.92 | 1.05 | 0.90 | 0.93 | 0.90 | 1.00 | 0.90 | 0.97 | 0.91 | 0.96 |
Mean prescriber time cost (£) (Cprescriber time) | 0.36 | 0.28 | 3.54 | 3.55 | 3.12 | 3.15 | 3.76 | 3.77 | 3.23 | 3.18 |
Assume that, on average, the prescription length < 60 days is 35 days and the prescription length ≥ 60 days is 120 days. Also assume that, regardless of prescription length, patients on average switch their prescription 30 days after a prescription is issued. The quantity used is, therefore, 30 days for both prescription lengths (Qdays used < 60 = Qdays used ≥ 60 = 30), but the quantity wasted is much larger for the ≥ 60 days prescription (90 days wasted compared with only 5 days wasted). Because over a 90-day period both prescription lengths will incur the same dispensing fees and prescriber time costs (three prescriptions will be issued regardless of prescription length as a switch occurs every 30 days), the ≥ 60 days prescription will be associated with higher TUCs.
Note that this example has been developed by adapting an example provided by Walton42 to the prescription lengths considered in our study.
Reproduced from Doble et al. 71
© Article author(s) (or their employer(s) unless otherwise stated in the text of the article) 2017. All rights reserved. No commercial use is permitted unless otherwise expressly granted. This is an Open Access article distributed in accordance with the terms of the Creative Commons Attribution (CC BY 4.0) license, which permits others to distribute, remix, adapt and build upon this work, for commercial use, provided the original work is properly cited. See: http://creativecommons.org/licenses/by/4.0/.
One-way sensitivity analyses were conducted to examine differences in TUC under a variety of different scenarios, including exclusion of prescriber time costs, ± 50% mean days wasted, ± 50% of the mean cost of drugs per day, dispensing fees and prescriber time.
All statistical analyses were preformed using Stata®/MP 13.1 (Stata Corp LP, College Station, TX, USA). The protocol (16_117R) for this study was approved on 21 June 2016 by the Independent Scientific Advisory Committee (ISAC), the independent body that approves the use of CPRD data.
Results
Overall cohort selection
Full samples for the five cohorts ranged in size, with four of the cohorts having a similar number of patients (range 208,682–310,391) and the depression cohort being much larger (1,207,523 patients) (see Table 12, column 1). The number of observations varied from 13,388,759 in the lipid management cohort to 44,151,527 in the secondary prevention of myocardial infarction cohort (see Table 12, column 2). The proportion of patients dropped from the full sample to create the limited sample owing to missing or observations equal to zero in either the ndd or qty variables was quite substantial, ranging from 15% for the lipid management cohort to 55% for the glucose control in T2DM cohort. The impact on the proportion of observations was, however, much smaller, ranging from 6% in both the lipid management and hypertension cohorts to 21% in the glucose control in T2DM cohort. Taking random samples from the limited samples resulted in the number of observations from each cohort ranging from 1,010,463 for the depression cohort to 10,131,377 for the secondary prevention of myocardial infarction cohort (see Table 12, column 8). The numbers of observations were slightly reduced after accounting for prescription error (i.e. prescriptions issued on the same day for medications in the same class with different product codes were dropped from the sample) (see Table 12, column 9). A number of prescriptions for medications with similar clinical indications from different classes issued on the same day were also identified, ranging from 12,401 for the depression cohort to 5,856,361 in the secondary prevention of myocardial infarction cohort; these were assumed to represent add-ons or concomitant therapy, and therefore were not counted as wastage despite overlapping dates (see Table 12, column 11).
Medication wastage
Over the 11-year study period, there was a statistically significant difference in the proportion of days’ supply wasted, the mean number of days’ supplied wasted and the mean cost of wastage per prescription between the shorter (< 60 days) and longer (≥ 60 days) prescription groups for all five of the case study conditions (Table 14). The proportion of days’ supply wasted was consistently larger for the ≥ 60 days groups across the case study conditions, with the exception of the depression cohort, in which the < 60 days group wasted 6.3% of days’ supply wasted, compared with 3.7% in the ≥ 60 days group. The mean number of days’ supply wasted was also consistently larger for the ≥ 60 days groups, but the difference between the two prescription length groups was much smaller for the depression cohort than for the other four cohorts. The mean cost of wastage per prescription was the largest for the ≥ 60 days groups for the glucose control and lipid management cohorts and largest for the < 60 days groups for the glucose control and depression cohorts. Similar mean costs of wastage per prescription were observed for the ≥ 60 days groups (range 0.43–0.51) and < 60 days groups (range 0.05–0.09) in the other cohorts.
Condition | Proportion of days’ supply wasted (%) | Mean number of days’ supply wasted (days) | Mean cost of wastage per prescription (2015 £) | ||||||
---|---|---|---|---|---|---|---|---|---|
< 60 days | ≥ 60 days | p-value | < 60 days | ≥ 60 days | p-value | < 60 days | ≥ 60 days | p-value | |
Glucose control with oral drug therapy in T2DM | 2.7 | 4.9 | < 0.001 | 0.86 | 4.96 | < 0.001 | 0.33 | 1.37 | < 0.001 |
Hypertension in T2DM | 3.8 | 5.0 | < 0.001 | 1.23 | 6.98 | < 0.001 | 0.09 | 0.44 | < 0.001 |
Lipid management in T2DM | 1.7 | 4.1 | < 0.001 | 0.63 | 16.21 | < 0.001 | 0.05 | 1.43 | < 0.001 |
Secondary prevention of myocardial infarction | 3.3 | 3.7 | < 0.001 | 0.96 | 6.56 | < 0.001 | 0.07 | 0.51 | < 0.001 |
Depression | 6.3 | 3.7 | < 0.001 | 1.87 | 2.69 | < 0.001 | 0.21 | 0.43 | < 0.001 |
Medication wastage by treatment pattern
In four out of the five case study conditions, all four treatment patterns resulted in a statistically significant difference in the mean cost of wastage per prescription between the two prescription lengths (Table 15). The one exception was for the depression cohort, in which the mean cost of wastage per prescription for both dosage/formulation and within-class treatment switches did not show statistically significant differences between the two prescription length groups. The refill treatment pattern consistently had the largest mean cost of wastage per prescription across the case study conditions, particularly for the ≥ 60 days groups. The one exception was for the depression cohort that also had similarly large mean costs of wastage per prescription for the dosage/formulation switch treatment pattern compared with the refill treatment pattern. The lowest mean cost of wastage per prescription was consistently observed for the between-class switch treatment (switches between medications with similar clinical indications from different classes) pattern across all case study conditions reporting these values. Note that the lipid management cohort did not report any between-class treatment switches, as all medications included in the analysis were from the same class of statins. Low mean costs of wastage per prescription were also reported for the within-class switch treatment pattern for the hypertension, lipid management, and depression cohorts.
Condition | Mean cost of refill wastage per prescription | Mean cost of dosage/formulation switch wastage per prescription | Mean cost of within-class treatment switch wastage per prescription | Mean cost of treatment between-class switch wastage per prescription | ||||||||
---|---|---|---|---|---|---|---|---|---|---|---|---|
< 60 days | ≥ 60 days | p-value | < 60 days | ≥ 60 days | p-value | < 60 days | ≥ 60 days | p-value | < 60 days | ≥ 60 days | p-value | |
Glucose control with oral drug therapy in T2DM | 0.23 | 1.03 | < 0.001 | 0.06 | 0.17 | < 0.001 | 0.03 | 0.10 | < 0.001 | 0.009 | 0.06 | < 0.001 |
Hypertension in T2DM | 0.05 | 0.27 | < 0.001 | 0.04 | 0.13 | < 0.001 | 0.004 | 0.013 | < 0.001 | 0.003 | 0.03 | < 0.001 |
Lipid management in T2DM | 0.02 | 1.10 | < 0.001 | 0.02 | 0.15 | < 0.001 | 0.008 | 0.17 | < 0.001 | NA | ||
Secondary prevention of myocardial infarction | 0.04 | 0.44 | < 0.001 | 0.01 | 0.04 | < 0.001 | 0.009 | 0.029 | < 0.001 | 0.00005 | 0.0006 | < 0.001 |
Depression | 0.04 | 0.21 | < 0.001 | 0.15 | 0.14 | NS | 0.01 | 0.01 | NS | 0.01 | 0.06 | < 0.001 |
Medication wastage over time
On an annual basis, a statistically significant difference in the mean cost of wastage per prescription was observed between the two prescription lengths for each of the 11 years across the case study conditions (Table 16). The one exception was for the years 2012 and 2013 for the depression cohort, in which no statistically significant differences in the mean costs between the two prescription lengths were observed, despite the magnitude of the mean costs remaining relatively consistent over the 11 years for both groups. For the most part, the magnitude of the mean costs also remained relatively consistent over the 11-year study period across the other four case study conditions. There were, however, a few trends in the mean costs that should be noted.
Year | Glucose control with oral drug therapy in T2DM | Hypertension in T2DM | Lipid management in T2DM | Secondary prevention of myocardial infarction | Depression | ||||||||||
---|---|---|---|---|---|---|---|---|---|---|---|---|---|---|---|
< 60 days | ≥ 60 days | p-value | < 60 days | ≥ 60 days | p-value | < 60 days | ≥ 60 days | p-value | < 60 days | ≥ 60 days | p-value | < 60 days | ≥ 60 days | p-value | |
2004 | 0.38 | 1.81 | 0.02 | 0.13 | 0.46 | < 0.001 | 0.07 | 1.51 | 0.002 | 0.09 | 0.38 | 0.005 | 0.25 | 0.43 | 0.003 |
2005 | 0.42 | 1.33 | < 0.001 | 0.12 | 0.52 | < 0.001 | 0.06 | 2.36 | < 0.001 | 0.08 | 0.52 | < 0.001 | 0.22 | 0.45 | 0.004 |
2006 | 0.42 | 1.05 | < 0.001 | 0.11 | 0.43 | < 0.001 | 0.06 | 2.27 | < 0.001 | 0.08 | 1.28 | 0.04 | 0.22 | 0.58 | 0.002 |
2007 | 0.39 | 1.11 | < 0.001 | 0.10 | 0.47 | < 0.001 | 0.05 | 1.53 | 0.002 | 0.07 | 0.40 | < 0.001 | 0.22 | 0.38 | 0.02 |
2008 | 0.34 | 1.08 | < 0.001 | 0.10 | 0.53 | < 0.001 | 0.05 | 1.66 | 0.003 | 0.07 | 0.46 | < 0.001 | 0.21 | 0.36 | 0.02 |
2009 | 0.30 | 1.07 | < 0.001 | 0.09 | 0.47 | < 0.001 | 0.05 | 1.72 | 0.002 | 0.06 | 0.53 | < 0.001 | 0.21 | 0.42 | 0.02 |
2010 | 0.33 | 1.40 | 0.001 | 0.08 | 0.38 | < 0.001 | 0.05 | 0.99 | 0.01 | 0.06 | 0.48 | < 0.001 | 0.18 | 0.37 | 0.02 |
2011 | 0.26 | 3.14 | 0.02 | 0.08 | 0.48 | < 0.001 | 0.04 | 0.88 | 0.02 | 0.06 | 0.41 | < 0.001 | 0.17 | 0.38 | 0.005 |
2012 | 0.25 | 0.93 | < 0.001 | 0.08 | 0.40 | 0.001 | 0.05 | 0.74 | 0.02 | 0.06 | 0.35 | < 0.001 | 0.18 | 0.35 | NS |
2013 | 0.27 | 0.87 | < 0.001 | 0.07 | 0.23 | < 0.001 | 0.04 | 0.45 | 0.04 | 0.05 | 0.20 | < 0.001 | 0.20 | 0.44 | NS |
2014 | 0.30 | 1.17 | < 0.001 | 0.08 | 0.27 | < 0.001 | 0.05 | 0.80 | 0.03 | 0.05 | 0.19 | 0.002 | 0.23 | 0.59 | 0.001 |
In the glucose control in T2DM cohort, the mean costs for the ≥ 60 days group in 2004 and 2011 were slightly higher (range £1.81–3.14) than the other nine annual means (range £0.87–1.40). In the hypertension cohort, there was a slight trend of decreasing magnitude of the mean cost over the 11 years for the < 60 days group; a decrease in mean cost was limited to the years 2013 and 2014 in the ≥ 60 days group. For both the lipid management and the secondary prevention of myocardial infarction cohorts, the magnitude of the mean costs remained relatively consistent over the 11 years for the prescription length < 60 days groups, whereas there was a slightly decreasing trend in the magnitude of the mean costs for the prescription length ≥ 60 days groups.
Differences in total unnecessary costs for short and long prescription lengths
After standardising the TUCs (wastage, dispensing fees and prescriber time) to a 90-day period, shorter (< 60 days) and longer (≥ 60 days) prescription lengths in each of the five case study conditions were compared (see Appendix 8). Using the mean values reported in Table 13 resulted in the base case TUCs and differences reported in Appendix 8. The differences in TUCs reported between the two prescription length groups resulted in the average TUC for prescriptions ≥ 60 days being lower (range £6.33–9.07 per prescription) than for prescriptions < 60 days.
The results of the sensitivity analyses for different scenarios are also shown in Appendix 8. The cost savings reported in the base case for prescriptions ≥ 60 days remained for all tested scenarios. The magnitude of the savings, however, reduced considerably across all five of the case study conditions when prescriber time costs were excluded from the models (range £0.72–2.12). The other scenarios tested had relatively little impact on the magnitude of the savings, with the exception of increases and decreases of 50% in the cost of prescriber time.
Discussion
Longer prescription lengths (≥ 60 days) are associated with more medication wastage per prescription than shorter prescription lengths (< 60 days). The largest and smallest differences in the mean cost of wastage per prescription were observed in the lipid management in T2DM and depression cohorts respectively. Generally, for both prescription lengths, the largest mean cost of wastage per prescription based on treatment pattern was observed for refills and the smallest for switches between different classes of medications with similar clinical indications. Patterns of the annual mean cost of wastage over the 11-year study period were relatively consistent, with the exception of the decreasing trend in the mean costs for prescriptions ≥ 60 days for the lipid management in T2DM cohort and the secondary prevention of myocardial infarction cohort in the last 5 years of the study (i.e. from 2009 to 2014).
When accounting for the additional dispensing fees and prescriber time required when prescriptions are issued for shorter durations (< 60 days), longer prescription lengths result in lower TUCs (e.g. cost of medication wastage, dispensing fees and prescriber time). These findings are consistent across all five case study conditions, and the magnitude of the cost savings in issuing longer prescriptions compared with multiple shorter prescriptions ranged from £6.33 to £9.07 per prescription when TUCs were standardised to a common 90-day time period. These cost savings could potentially be seen as a lower bound, as they would probably be much larger if the prescriber time associated with additional consultations, rather than just the administrative time required to issue a prescription (i.e. how long it takes a GP to complete the technical process of producing a prescription, not including clinical decision-making time) were considered. Consideration of the time associated with additional consultations would further increase the cost savings in favour of the longer prescription lengths. Alternatively, the cost savings could be considered an overestimate, as it was assumed that a GP would be involved in issuing all prescriptions. Nurses are playing an increasing role in drug therapy management of some chronic conditions (e.g. diabetes and CVD) and, as the technical process of producing a prescription is likely to be less costly for nurses, smaller cost savings would be observed. The cost savings would decrease in magnitude, but would still favour longer prescription lengths, as, even in the absence of prescriber time costs, savings were still observed for the longer prescription lengths. Cost savings were largest for the secondary prevention of myocardial infarction and smallest for glucose control in T2DM cohorts. The cost savings remained for all of the five case study conditions under a number of one-way sensitivity analyses, but decreased considerably, particularly for the glucose control and lipid management in T2DM cohorts, when the costs associated with prescriber time were removed from the models (range £0.72 to £2.12). Increases and decreases of 50% in the number of days wasted, drug costs per day and dispensing fees had relatively small impacts on the cost savings, whereas 50% increases and decreases in prescriber time costs had much larger impacts.
Based on the reported cost savings of longer prescription lengths in our study, extrapolating the mean values to the affected populations in the UK could result in substantial estimated savings to the NHS. For example, the majority of prescriptions in our CPRD analysis in the depression cohort were for < 60 days (94.76%). It should be noted that, in the depression cohort as well as the other cohorts, the majority of prescriptions were for ≤ 30 days, with relatively small proportions of patients having prescription lengths between 31 and 60 days (18%, 27%, 28%, 27% and 25% for the depression, T2DM, hypertension, lipid management and myocardial infarction cohorts, respectively). Knowing that roughly 39 million prescriptions are issued for antidepressants in the UK each year,87 if the 95% issued as supplies of < 60 days were instead issued as prescriptions for ≥ 60 days, the total savings to the NHS could be as much as £305M per year. Similarly, using the total number of simvastatin and atorvastatin prescriptions issued in England in 2015 (roughly 61.6 million),84 and knowing from our CPRD analysis that 97.05% of prescriptions for statins are for < 60 days, the total savings to the NHS just in England for issuing longer rather than shorter prescriptions for only two statins could be as much as £424M per year. Note that the majority of savings for both examples will not be cash releasing, but will be realised as savings of GP time. Using the cost savings from the models that included only dispensing fees (see Appendix 8) and not prescriber time costs can give us a rough estimate of the cash-releasing savings within the NHS that may be realised (£78M and £47M for antidepressants and the two statins, respectively). The magnitude of the savings for the other case study conditions are also likely to be similarly large given the high prevalence of the conditions and the large proportions of current prescriptions being issued as < 60-day supplies. However, these figures represent an upper bound, and should be interpreted with extreme caution, as they assume that it is clinically appropriate for all prescriptions to be issued for a longer duration, which is certainly not the case.
Comparison with previous studies
Six previous studies have estimated costs differences associated with long (3-month) and short (1-month) prescriptions. 22,42,44–46,49 All of the existing studies are from various perspectives in the USA (e.g. Veterans Affairs, Medicaid and non-institutionalised civilian populations). Only one of the studies accounted for differences in prescriber time (indirectly by assessing differences in all Medicaid expenditures, i.e. outpatient, inpatient, emergency and pharmacy costs),44 and only four of the studies accounted for the impact of differences in drug wastage. 42,44–46 Prescriptions for a number of chronic diseases were assessed,44,45,49 but some studies limited their analyses to specific drugs (e.g. simvastatin and lovastatin)42 or did not limit their analyses to any particular therapeutic class of medication. 46
Walton42 applied a similar model to the one used in our study to estimate the TUC (wastage and dispensing fees only) associated with either 30-day or 90-day supplies of two statin medications. When compared with the results of our analysis for the lipid management cohort and excluding prescriber time costs, the difference in the mean TUC between long and short prescription lengths was greater in the US study (US$2.45 vs. £0.79). It should be noted that the dispensing fee used by Walton42 was also slightly larger (US$1.79 vs. £0.90), which may partly account for the slightly larger savings reported.
Taitel et al. 45 estimated the per-patient savings of switching from 30-day prescriptions to 90-day prescriptions for four therapeutic classes of medication (statins, antihypertensives, SSRIs and oral hypoglycaemics). Similarly to our analysis, all four therapeutic classes reported a greater number of wastage days for 90-day prescriptions than for 30-day prescriptions. However, after adding dispensing fees and medication costs to the costs of wastage and standardising to per-patient per-year values, savings were reported of US$7.70 for statins, US$10.80 for antihypertensives, US$18.52 for SSRIs and US$26.86 for oral hypoglycaemics. 45 Multiplying our reported savings for longer prescriptions standardised to 90 days by four, so as to encompass a year, results in estimated savings of £3.16 per prescription per year for the lipid management cohort, £5.00 for the hypertension cohort, £8.48 for the depression cohort and £2.88 for the glucose control in T2DM cohort. Thus, in all cases, the estimated savings were somewhat larger in the Taitel et al. 45 US study than our estimates for the UK, although this is to be expected based on the estimated savings in our study being on a per-prescription per-year basis rather than on a per-patient per-year basis as reported by Taitel et al. 45 Any further differences may be attributed to the inclusion of volume discounts for medication costs in the study by Taitel et al. ,45 and the fact that the drugs included in our study were not limited to single classes of medications (with the exception of the lipid management cohort), and were selected to be representative of all of the treatments available for a particular case study condition.
Rabbani et al. 49 assessed differences in third-party expenditures for 3-month and 1-month supplies of 395 unique medications for common chronic conditions, including high cholesterol, hypertension, hypothyroidism and depression. 49 Only two of the six payers in their study achieved statistically significant savings with a 3-month supply (range US$0.34M to US$1.74M), as the majority of savings were accrued for individuals through reductions in out-of-pocket costs, which were not assessed in our analysis. 49 Another major difference between our study and that conducted by Rabbani et al. 49 is the latter’s lack of consideration of the cost of medication wastage, making it difficult to compare the results of this study with the results of our analysis in a meaningful manner.
Parikh et al. 46 estimated a theoretical saving of US$6.17 if 90-day prescriptions were issued instead of 30-day prescriptions. 46 In addition to medication and wastage costs, Parikh et al. 46 also included mailing costs for mail-order prescriptions that were not considered in our analysis. The mean cost saving in our analysis over the five case study conditions when excluding prescriber time costs was £1.30 per prescription, but it is difficult to compare our results with those presented by Parikh et al. 46 because their analysis was not specific to any particular chronic condition and included only a very small number of prescriptions (346 unique prescriptions) over a short time period (3 months). The standardisation approach used by Parikh et al. 46 was also different from the models used in our study. Costs of wastage, dispensing fees (US$1.573–4.275 vs. £0.90) and mailing costs were simply multiplied by three for the 30-day prescriptions to determine the total cost, then subtracted from the actual total costs associated with 90-day prescriptions. This difference in methodology and dispensing fees might account for the difference in the magnitude of savings reported in our study.
The first study by Domino et al. 22 simulated the effect of a policy change from a maximum of a 100-day supply for prescriptions to one in which only a 34-day supply was allowed for six specific therapeutic categories of medications. 22 Only three therapeutic categories (sulfonylureas, SSRIs and ACEIs), all of which were associated with relatively small amounts of wastage in comparison with the other categories (antiulcers, antipsychotics, non-steroidal anti-inflammatory drugs), were included as treatments for one or more of the five case study conditions analysed in our study. A comparison of our results is therefore difficult, but a specific pattern should be noted. When simulating 34-day supplies for all six categories, any savings in wastage as a result of the shorter prescription length were offset by increases in dispensing fees (US$5.60 per prescription). 22 Reducing the dispensing fee to US$2.40 resulted in the wastage savings exceeding dispensing costs for three of the categories (antiulcers, antipsychotics and non-steroidal anti-inflammatory drugs), none of which was assessed in our analysis. For the remaining three categories, the reduction in dispensing fees required to achieve savings for 34-day supplies was not noted, although Domino et al. 22 stated that it would have to be much lower, which is consistent with the findings of our analyses.
The second study by Domino et al. 44 provides the most comprehensive study to date using a pre–post controlled partial difference-in-difference-in-differences design to assess the impact of a prescription length policy change in the North Carolina Medicaid programme (from 100 to 34 days’ supply) on total Medicaid expenditures (outpatient, inpatient, emergency and pharmacy costs). 44 Total Medicaid expenditures were shown to decrease for patients initially receiving 100-day prescriptions after the implementation of the shorter prescription length policy (range US$245–440 per person per quarter in all six classes of medications assessed (antihypertensives, antidiabetic medications, lipid-lowering drugs, seizure disorder medications, antidepressants and antipsychotics). The results, however, are not stratified by expenditure category (with the exception of also reporting decreases in expenditures for the targeted prescriptions across all six medication classes); therefore, it is not clear to what extent changes in outpatient, inpatient or emergency visits are individually responsible for the decreases in expenditures. In addition to the difference in study design, a number of methodological contrasts with our study should also be noted. To be included in the analysis, medical diagnoses were required for four of the six medication classes (antihypertensives, antidepressants, antipsychotics and seizure disorder medications),44 which is in contrast to our reliance on product codes to identify our five cohorts of interest. Our analysis could therefore be capturing a broader range of patients (i.e. those receiving the medications of interest off-label or for other conditions). The study by Domino et al. 44 also accounted for differences in adherence, and indicated that reduced adherence for shorter prescriptions led to the decreases in expenditures, but this was not assessed in our study owing to differences in study design and available data. This somewhat counterintuitive finding may potentially be explained by small adverse health effects as a result of changes in adherence, patients absorbing any health effects through informal care or tolerating greater disease burden, and the 18-month post-policy period in the study being too short to capture the spillover effects of decreased medication adherence on other Medicaid services. Furthermore, NHS expenditures in the UK for the treatment of chronic conditions are likely to differ based on the organisation of primary care and the existence of a referral system to specialty care compared with those incurred in a US Medicaid patient population; therefore, the generalisability of the conclusions presented by Domino et al. 44 to the UK context are unclear. It will, however, be important to incorporate a more comprehensive measurement of the potential differences in NHS expenditures associated with different prescription lengths in any future UK study.
Limitations of the cost analysis
Although our study, to the best of the authors’ knowledge, provides the only available evidence of the unnecessary costs associated with different prescription lengths from the perspective of the NHS in the UK, and builds on existing methodological approaches available in the literature (accounts for prescriber time costs and potential wastage between medications with similar clinical indications from different classes, rather than single classes of drugs), there are a few limitations that warrant discussion. First, a limitation of CPRD prescription data is that they do not indicate whether or not a medication has been dispensed, or if patients took their prescribed medications as recommended (i.e. they indicate only when a prescription has been issued). Our estimates may, therefore, either over- or understate the amount of wastage that actually occurred, depending on patient behaviour not captured by CPRD. Assuming that all prescriptions issued were actually dispensed places an upper bound on the potential savings that would occur if drug wastage from premature medication switches could be eliminated entirely. In terms of medication adherence, longer prescription lengths (≈ 3 months) consistently show improved adherence compared with shorter prescription lengths (≈ 1 month). 39,44,45 One of these studies, however, also indicated that reduced adherence related to shorter prescription lengths led to lower total expenditure from a Medicaid perspective (outpatient, inpatient, emergency and pharmacy costs). 44 In contrast, our study was not designed to estimate the impact of different prescription lengths on total NHS expenditures, as this would require additional linkages to secondary care datasets (e.g. Hospital Episode Statistics) and more comprehensive analysis of CPRD through consideration of additional data files (e.g. clinical, consultations and laboratory test files). Chapter 5 provides a model-based analysis that attempts to capture these ‘downstream’ costs as well as health outcomes. The main objective of our study was, however, to estimate differences in TUCs (medication wastage, dispensing fees and prescriber time costs) associated with different prescription lengths, therefore it may be pertinent to conduct more comprehensive analyses using linked data in future research.
Second, the five case study conditions were purposively rather than randomly selected to represent the impact of medication refill and switching behaviour on wastage; they may not be representative of prescribing behaviour in other chronic conditions. However, those selected do represent some of the most common chronic conditions treated with prescribed medications. Nine of the top 20 prescribed medications within NHS England were included in at least one of the case study conditions in our analyses, and, combined, they accounted for around £378M drug expenditure in NHS England in 2015 (4% of the total), and are, therefore, highly policy relevant. In common with other studies,22,45 our analyses have shown that the amount of wastage, and therefore its associated cost, can differ depending on the therapeutic category of the medications being analysed. Our analysis also excluded patients in whom one or more observations were missing or had zero values for either the ndd and/or qty variables. This approach resulted in substantial proportions (15–55%) of patients from the full samples being dropped and therefore limits the generalisability of the results from our original random samples. Appropriate methods to impute these variables are, however, limited, and our approach was similar to other studies using CPRD data. 77,78
Third, the identification of patients within CPRD with the five case study conditions (see Table 11) was based solely on product codes, rather than on a specific medical diagnosis (identified using Read codes in CPRD). This was straightforward for four of the five conditions, but required additional assumptions for the secondary prevention of myocardial infarction cohort. To avoid the additional complexity of using Read codes, patients were identified according to whether or not they had prescriptions for drugs as per clinical guidelines, for example patients receiving concurrent prescriptions for an ACEI, antiplatelet and statin for a duration of ≥ 1 year. 81 As the main aim of our study was to estimate drug wastage, the possible inclusion of patients without a previous myocardial infarction event, but still receiving at least four of the prescriptions of interest for ≥ 1 year, provided our analysis with relevant information concerning drug wastage, dispensing fees and prescriber time.
Fourth, an overlap of dates between prescriptions does not necessarily mean wastage has occurred, as consumption of early refills may be delayed until the initial supply is exhausted, and treatment changes might actually be add-ons to existing prescriptions or concomitant therapy rather than switches in therapy. To ensure that wastage was not overestimated, a threshold of 1 year after the initial prescription in a particular series was used to estimate wastage for early refills and a threshold of < 1 in the difference between the number of drug changes between medications with similar clinical indications from different classes and the number of unique drug classes within an annual period was used to identify wastage from between-class treatment switches. There is, however, a possibility that our analysis approach could have overestimated the amount of medication wastage.
Fifth, for pragmatic purposes, we dichotomised prescription lengths into ‘short’ versus ‘long’, with a cut-off point of 60 days. This will have classified 56-day prescriptions as ‘short’. Although this will have resulted in a loss of sensitivity (there may be differences in TUC between 1- and 2-month prescriptions), the overall conclusions are not affected: 3-month prescriptions are associated with higher drug wastage than 1- or 2-month prescriptions, but the reduction in administration costs (e.g. dispensing fees and prescriber time) more than compensates for this, leading to overall lower TUC with the longer prescription lengths.
Finally, a number of assumptions were required to assign unit costs to the estimated proportions of wastage. Mean cost per day values derived using DDDs, NICs and quantities at the drug substance level were calculated and then applied to any prescription categorised under that particular drug substance. This approach is not ideal; given the inability to link CPRD data to individual unit costs specific to each prescription, it was necessary, but it means that the direction and magnitude of any resulting bias is difficult to predict.
Furthermore, NICs do not include any discounts that may be applied or include any adjustment for revenue received by the NHS if a prescription charge is paid at the time the prescription is dispensed, or if the patient has purchased a pre-payment certificate, and therefore may be different from the net cost incurred specifically by the NHS. Patients with T2DM are exempt from the prescription charge,88 and, overall, almost 90% of prescriptions dispensed in the NHS in England are exempt. 5 Given a current prescription charge of £8.40 (as in August 2016), and with only 10% of prescriptions attracting a patient charge, revenue to the NHS is, on average, £0.84 per prescription. If this average revenue per prescription is incorporated into our TUC models, the predicted net savings to the NHS from issuing longer prescriptions decreases on average by £1.56 (range £1.45–1.69) from the base case values across all five of the case study conditions. Applying the adjusted cost savings accounting for losses in revenue from prescription charges to the NHS from switching to longer prescriptions for antidepressants and the two statins (simvastatin and atorvastatin) would reduce projected savings from £305M to £246M and from £424M to £323M, respectively.
All of these limitations risk biasing the results. The projected savings should, therefore, be interpreted with caution, especially if they are to be extended to other types of medications that are not prescribed at relatively low unit costs to a large population of patients. Proportional savings for high-costs drugs used to treat relatively small patient groups may not be similarly observed.
Chapter 4 Disease-specific decision-analytic modelling
Introduction
We combined evidence from the systematic review (see Chapter 2) with the CPRD cost analysis estimates (see Chapter 3) and adapted existing decision models to predict the medium- and longer-term costs and health consequences of different adherence levels associated with shorter and longer prescriptions. The analysis involved three discrete case studies of three classes of commonly prescribed medications. They are presented from the perspectives of costs to the NHS, and health outcomes for patients.
Decision modelling is used extensively in the UK as a tool for assessing and accounting for uncertainty in the medium- to longer-term costs, health consequences and cost-effectiveness of new health technologies and, to a lesser extent, policy changes. 89 For example, in the field of pharmacy, a recent study examined the longer-term costs and health consequences of the New Medicine Service (NMS), a pharmacist-led intervention in the UK designed to tackle low adherence with newly prescribed medications. 90 However, our study is the first to use a decision modelling approach to examine the impact of changes in prescription length on longer-term health service costs and health consequences.
This chapter is structured as follows: the first section sets out the objectives of the decision-analytic modelling (see Objectives); in the second section, our methods are described (see Methods); and our findings are presented in the third section (see Results) and discussed in the final section (see Discussion).
Objectives
The overall objective of the decision modelling was to predict the medium- and longer-term costs and health consequences that may be associated with shorter and longer prescription lengths.
In comparison with the studies of the relationship between prescription length and adherence identified in the systematic review, the specific objectives of this chapter were to:
-
examine the longer-term costs and health consequences of different prescription lengths [in contrast with the evidence examined in the systematic review, which generally had a shorter follow-up (median follow-up of 14 months and a mean follow-up of 20.3 months)]
-
assess costs from the perspective of the NHS in the UK [in contrast with the evidence in the review, which was solely US based and rarely accounted for transaction costs (dispensing fees and prescriber time) or drug wastage costs]
-
examine the potential relationship between prescription length and health consequences [in contrast with the systematic review, which identified just one study that examined health consequences (achievement of target cholesterol levels)39].
Methods
Our approach involved developing three case study scenarios that examined the potential impact of prescription length on the costs and health consequences of three classes of commonly prescribed medications:
-
case study 1 – primary prevention of cardiovascular events in patients with recent-onset T2DM
-
case study 2 – treatment of depression in adults with SSRIs
-
case study 3 – secondary prevention of cardiovascular events in patients with hypertension.
For each case study, we adapted existing decision models developed to inform NICE guidance to determine the cost-effectiveness of commonly prescribed medications. These three case studies were chosen primarily because they represent a range of clinical situations in the UK in which variation in prescription lengths may have a significant impact on costs and/or health outcomes. They are also the disease areas examined in our systematic review and CPRD analysis, and for which many decision models already exist for our case study drugs.
The model adaptations were based on relevant parameters identified in the systematic review and CPRD analysis or, when necessary, from additional evidence identified in other published studies, for example, clinical evidence reviews produced for NICE guidance. When data were unavailable, appropriate assumptions were made. In particular, our approach relied on two key assumptions:
-
Treatment effects observed in model active and placebo/no treatment arms of clinical trials were assumed to represent the maximum effect comparing zero and perfect adherence.
-
A linear dose–response curve was assumed (e.g. it was assumed that 50% adherence would generate 50% of the treatment effect).
These simplifying assumptions were necessary in the absence of evidence to the contrary. Although the systematic review identified studies on the relationship between prescription length and adherence, these studies did not examine the relationship between those identified levels of adherence and health outcomes. The implications of these assumptions on our main findings are explored in the Discussion section of this chapter. As is common in all decision models that include a ‘no treatment’ arm, it was necessary to assume that health outcome data from the ‘placebo’ arm of clinical trials were equivalent to ‘no treatment.’ However, because the placebo effect means that being treated with a placebo is not the same as knowingly not being treated at all, this may have led to an overestimation of the QALYs associated with no treatment. As a result, we may have underestimated the health gain from increased adherence as a result of longer prescription lengths.
Our general approach is depicted in Figure 9, in which the expected costs and outcomes of the 3-month or 28-day repeat strategies are presented as weighted averages of the costs and QALYs associated with perfect and zero adherence (assumed to be the long-term costs and QALYs associated with treatment vs. placebo/no treatment, respectively). Given that our systematic review showed that 3-month repeat prescriptions are associated with higher adherence than 28-day repeat prescriptions, in the absence of evidence to the contrary, we assumed that the 3-month repeats would yield the expected costs and QALYs associated with perfect adherence, that is, p = 1 in Figure 9. The RR of being adherent varies between 0 and 1. The RR gives the full range of possible values for the incremental cost and QALYs gained from 3-month versus 28-day repeat prescriptions. The most plausible value for the RR is extracted from the systematic review.
FIGURE 9.
General approach to modelling. p, probability of perfect adherence in active treatment arm of existing model (in our modelling, we have assumed p = 1); RR, relative risk of being adherent to a 28-day prescription compared with a 3-month prescription.
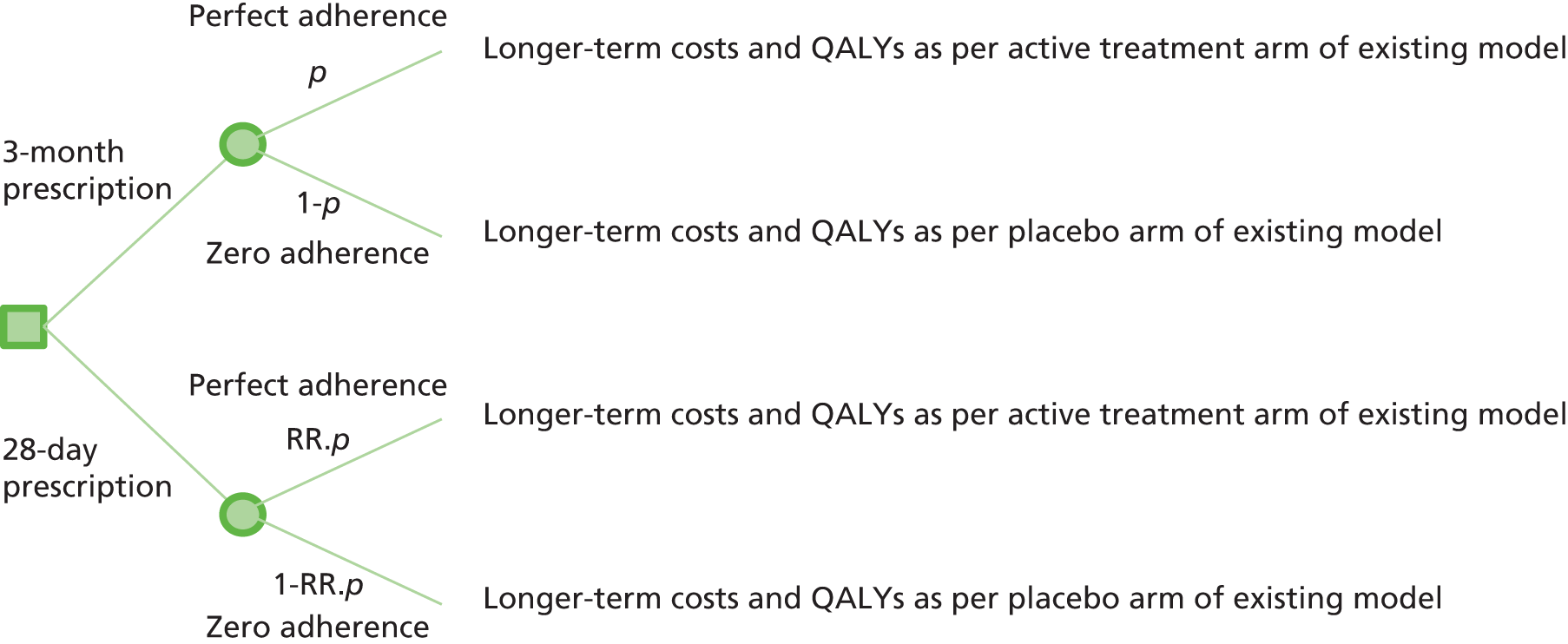
It should be noted that, prior to the inclusion of transaction costs (dispensing fees and prescriber time) and the costs of wastage, the method described in Figure 9 always yielded an identical ICER between the two strategies. As the RR changes, incremental costs and QALYs change exactly in proportion so that the ratio between the two remains constant. However, the values are associated with different incremental net benefits (INBs), an absolute measure of the net gain to society from one strategy compared with another. The INB is calculated from a simple rearrangement of the ICER (Equation 5, which is explained graphically in Figure 10):
where λ = willingness to pay for a unit of outcome and E = QALYs (NICE assigns a value of £20,000–30,000 to λ).
Changing RR represents a movement along the ICER line from point a to point b in Figure 10, but increases the INB from x to y.
FIGURE 10.
Incremental net benefit vs. ICER. ΔC, incremental costs; ΔE, health effects. The diagram shows a cost-effectiveness plane. The gradient of the line 0ab is equal to the ICER. The gradient of the lines INBλ is equal to the threshold, λ. The INB line that passes through the origin (INBλ = 0) shows the locus of points where INB = 0. The parallel lines INBλ = x and INBλ = y show the locus of points where INB = x and INB = y, respectively, where y > x > 0. Points a and b represent the incremental costs and QALYs gained with strategies a and b vs. comparator, respectively. They have the same ICERs with respect to the origin and to each other, but point b is associated with a higher INB than point a.
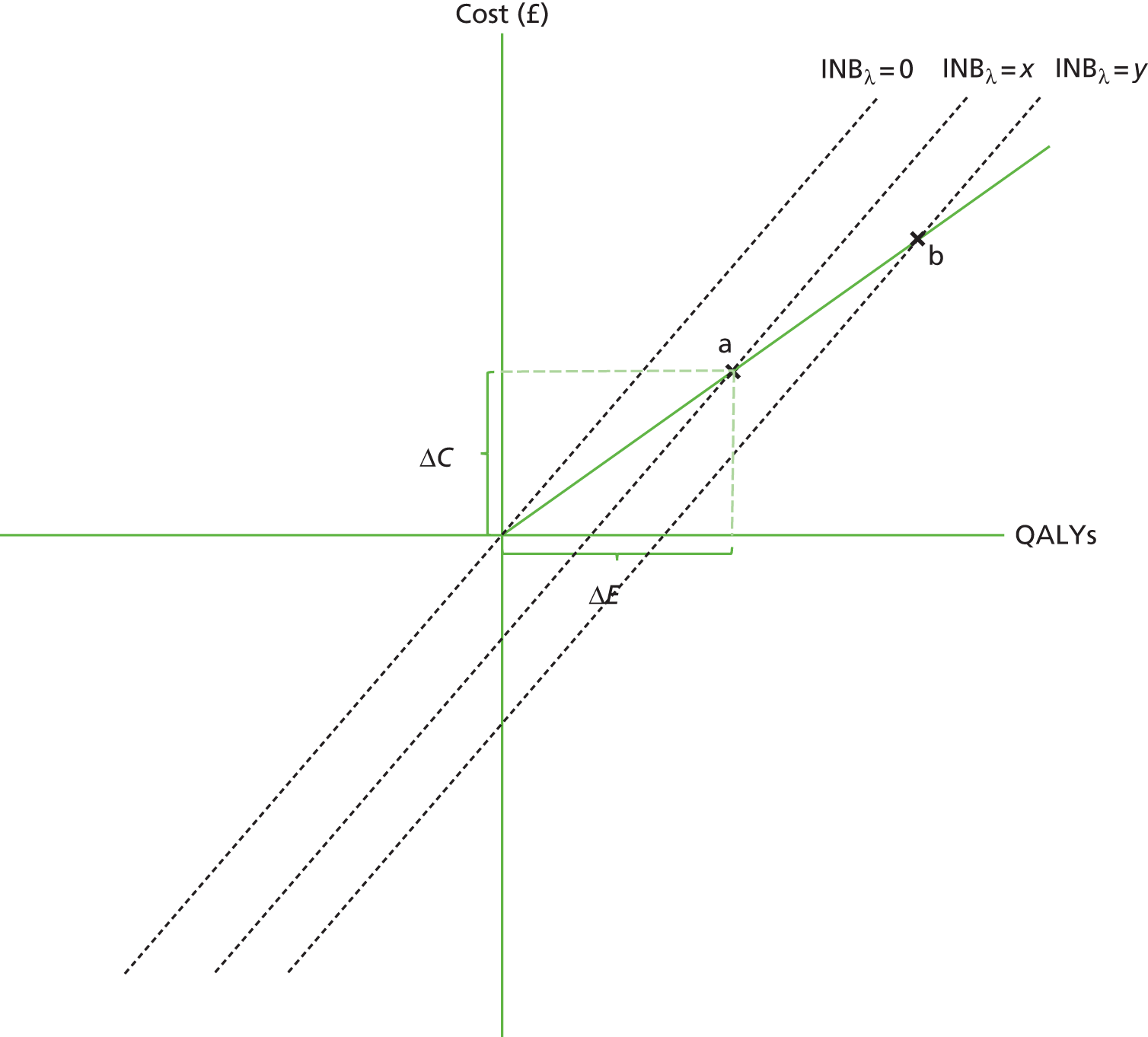
This general approach described in this section represents the ‘default’ approach to analysis in the absence of superior evidence. Where the data allowed, we made appropriate modifications and enhancements to the default as described in the specifics for each case study below. A summary of our approach is provided in Table 17, and the strengths, limitations and plausibility of the assumptions are considered in the Discussion section later in this chapter.
Stage 1: identify relevant data and decision models | |
---|---|
1(a) | Identify relevant studies from the systematic review estimating the relationship between prescription length, adherence and/or health outcomes |
1(b) | Review NICE guidelines and other literature to identify an appropriate existing decision model that has been used to assess the cost-effectiveness of relevant medications |
1(c) | Identify additional data, including
|
Stage 2: adapt the decision model | |
2(a) | Drawing on the data identified in stage 1 or, when necessary, appropriate assumptions, adjust the input parameters and/or model outputs of the identified decision model to account for different costs, QALYs and levels of adherence associated with no treatment and treatment with different prescription lengths |
2(b) | Report the health consequences, costs, ICERs and INBa for
|
2(c) | Address uncertainty in the results through sensitivity analyses based on the methods used by NICE in the original source models |
Results
The results for each case study are reported separately and structured as per the overview of our methods presented in Table 17. All cost data are reported in 2015 GBP. Costs were inflated when necessary to 2015 levels using the PSSRU Hospital and Community Health Service indices. 86
Summaries of the original source models for each of the three case studies are provided in Table 18. The three decision models chosen for this study represent the full range of modelling techniques currently used in health economic evaluation: a decision tree, a Markov model and a patient-level simulation. Further information about each model can be found in the full NICE report for each model (see Table 18 for the relevant references), where more detail can be found than was possible to include in this document. All three models were commissioned by NICE for the most recent clinical guidance in the relevant disease areas, and have thus been used to inform policy in the recent past. The choice of modelling technique is justified in the NICE guidance for the particular disease area considered. For example, the decision tree was considered appropriate for the modelling of SSRIs because the clinical data reviewed by the guideline development group related to a relatively short 9-month treatment period. In contrast, the modelling of the primary prevention of cardiovascular events in patients with recent-onset T2DM was supported by high-quality clinical studies on a range of longer-term health outcomes, and hence a patient-level simulation was most appropriate in this case. Further details on all aspects of the design of the source models can be found in the full NICE report.
Characteristic | Case study 1: primary prevention of cardiovascular events in patients with recent-onset T2DM | Case study 2: treatment of depression in adults with SSRIs | Case study 3: secondary prevention of cardiovascular events in patients with hypertension |
---|---|---|---|
Type of model | Patient-level simulation model, 12-month cycles, 1000 ‘outer loop’ iterations | Decision tree | Markov model, 6-month cycles, 2000 iterations |
Source of model | The original model is the UKPDS model. It was adapted by NICE for CG2879 | NICE CG9082 | NICE CG12791 |
Time horizon | 40 years (equivalent to lifetime, given that the average starting was between 60 and 65 years) | 14–15 months (2- to 3-month acute treatment phase, 6-month maintenance treatment phase, 6-month follow-up phase) | Lifetime, with an assumed upper age of 100 years (most have died by then) |
Comparators | The model was run separately for three discrete stages of disease progression (initial therapy, first intensification and second intensification). In each stage, at least seven comparators were modelled (e.g. for initial therapy this included placebo and metformin) | One analysis focused on pharmacological intervention (10 different antidepressants were assessed). Another analysis focused on combination therapy (CBT combined with SSRI treatment compared with SSRI treatment alone) | Four groups of alternative antihypertensive drugs (ACEIs/ARBs, beta-blockers, calcium-channel blockers, thiazide-type diuretics) and no treatment |
Patients | 50,000 patients (each simulated 100 times – ‘inner loop’ iterations) | 100 patients in each treatment group with either moderate or severe depression | 1000 patients |
Selected base case patient characteristics | For initial therapy scenario: gender = 57.1% male; mean age = 59.8 years | NA | 65-year-old male with a 2% CVD risk, 1% heart failure risk and 1.1% T2DM risk |
Perspective | NHS for costs and patients for health outcomes | NHS for costs and patients for health outcomes | NHS for costs and patients for health outcomes |
Health outcome | QALYs reflecting the impact of treatment on the first occurrence of seven diabetes-related complications (fatal or non-fatal MI, other IHD, stroke, heart failure, amputation, renal failure and eye disease measured in terms of blindness in one eye) and death. Based on data from the UKPDS RCT92 | QALYs (utility scores were based on a study by Sapin et al. 2004, a study that was identified through a systematic review)93 | QALYs reflecting prevention of cardiovascular events (non-fatal unstable angina, myocardial infarction, heart failure and stroke, and cardiovascular-related deaths) and side effects (onset of heart failure and diabetes) (various sources; see tables 92 and 93 in appendix I of NICE CG12791) |
Clinical input parameters | Treatment effects on HbA1c, weight, hypoglycaemic episodes and treatment dropouts owing to intolerance taken from a clinical review network meta-analysis | Odds and probabilities of dropout (after 2–3 months), remission (after 8–9 months) and relapse (after 14–15 months) (identified in a literature review or through expert opinion) | Baseline risks (from a range of sources reported in NICE CG12791 (see appendix I) and treatment effects |
Cost parameters | Complications and event costs (mainly from UKPDS RCT),92 and drug unit costs from the NHS Drug Tariff 2014; both are reported in table 84, appendix F of NICE guideline 2879 | Resource utilisation (identified in a literature review or through expert opinion). Unit costs: drug costs (e.g. BNF and Electronic Drug Tariff data), primary and secondary care costs (e.g. PSSRU estimates of standard GP care and specialist mental health outpatient care for patients with severe depression) | See I.3.7 in CG12791 |
Discounting | 3.5% per annum (for costs and benefits) | None (owing to a short time horizon) | 3.5% per annum (for costs and benefits) |
Sensitivity analyses | Probabilistic sensitivity analyses were reported to evaluate and combine all sources of uncertainty in the model. One-way sensitivity analyses using stochastic model runs | Deterministic one-way sensitivity analysis on the upper and lower 95% credibility intervals around the clinical input parameters | Variety of univariate and multivariate deterministic sensitivity analyses to test the impact of uncertainty in model parameters and assumptions |
Reference(s) for full description of economic model | NICE: appendix F;79 UKPDS Outcomes Model 194 | NICE: chapters 8 and 1095 | NICE: appendix I91 |
Key limitations of model | No placebo treatment group was included in the first and second intensification of treatment. Patients on initial treatments therefore moved to metformin–sulfonylurea (first intensification) then metformin–NPH insulin (second intensification) after a period of time | Treatment continued for only 9 months with follow-up for a further 6 months. Although this model was developed to inform current NICE guidance and is consistent with other SSRI studies (e.g. Cipriani et al.96), this may not reflect current clinical practice, and would not capture longer-term costs and health consequences | Although a range of multi-way sensitivity analyses were reported, the modelling did not include a probabilistic sensitivity analysis |
In this chapter, each case study is presented in order of the complexity of the adjustments made to the source model. In the first case study, only the outputs (lifetime costs and QALYs) of the source model were adapted to reflect different prescription lengths. In the second case study, the source model did not include a placebo or no treatment arm. We therefore modified the model inputs to represent this and the additional transaction and drug wastage costs associated with different prescription lengths. The model outputs were then adjusted to account for different levels of adherence associated with different prescription lengths. In the third case study, model inputs were adjusted to account for different prescription lengths. This included both the additional costs as well as the impact of different levels of adherence on relative treatment effects. Access to each economic model was provided through contact with the relevant authority.
Table 19 provides a summary of the identified data and key assumptions for each case study, and a summary of the results for each case study are provided in Tables 20–22.
Data | Case study 1: primary prevention of cardiovascular events in patients with recent onset T2DM | Case study 2: SSRIs for depression | Case study 3: secondary prevention of cardiovascular events |
---|---|---|---|
(1) Costs (UK) and health consequences of relevant treatment vs. no treatment or placebo | A placebo comparator included in the source model (NICE NG28)79 | Clinical evidence review in NICE CG90 included evidence on treatment vs. placebo82 | ‘No treatment’ included in the source model (NICE CG127)91 |
(2) Relationship between adherence and health consequences | Assumed linear | Assumed linear | Assumed linear |
(3) Relationship between prescription length and adherence | Hermes and Taitel papers identified in systematic review35,45 | Taitel and Pfeiffer papers identified in systematic review37,45 | Hermes and Taitel papers identified in systematic review35,45 |
(4) Dispensing fees | NHS Drug Tariff60 | NHS Drug Tariff60 | NHS Drug Tariff60 |
(5) Prescriber time and wastage costs | CPRD analysis (see Chapter 3) | CPRD analysis (see Chapter 3) | CPRD analysis (see Chapter 3) |
Years on initial treatmenta | Total lifetime cost (£) | The total cost includes | Total lifetime QALYs | Incremental analysis | Incremental net benefit (£) | |||||
---|---|---|---|---|---|---|---|---|---|---|
UKPDS (£)b | Treatment costs (£) | Additional costs (£)c | Costs (£) | QALYs | ICER | |||||
Placebo and treatment arms in source model | ||||||||||
Placebo | 2.30 | 20,450 | 14,036 | 5590 | NA | 8.912 | NA | NA | NA | NA |
Treatment (metformin) | 3.80 | 19,639 | 13,969 | 4950 | NA | 9.033 | –811 | 0.121 | Dominant | 3231 |
28-day and 3-month prescribing based on Hermes et al.35 (RR = 0.891) | ||||||||||
28-day | 3.64 | 19,797 | 13,977 | 5020 | 69.37 | 9.020 | NA | NA | NA | NA |
3-month | 3.80 | 19,677 | 13,969 | 4950 | 38.76 | 9.033 | –158 | 0.013 | Dominant | 423 |
28-day and 3-month prescribing based on Taitel et al.45 (RR = 0.863) | ||||||||||
28-day | 3.59 | 19,818 | 13,979 | 5038 | 68.58 | 9.016 | NA | NA | NA | NA |
3-month | 3.80 | 19,677 | 13,969 | 4950 | 38.76 | 9.033 | –179 | 0.017 | Dominant | 511 |
Sensitivity analysis | ||||||||||
28-day and 3-month prescribing based on upper bound of Hermes et al.35 (RR = 0.926) | ||||||||||
28-day | 3.64 | 19,769 | 13,974 | 4997 | 70.40 | 9.020 | NA | NA | NA | NA |
3-month | 3.80 | 19,677 | 13,969 | 4950 | 38.76 | 9.033 | –130 | 0.009 | Dominant | 308 |
28-day and 3-month prescribing based on lower bound of Taitel et al.45 (RR = 0.851) | ||||||||||
28-day | 3.59 | 19,827 | 13,979 | 5045 | 68.25 | 9.016 | NA | NA | NA | NA |
3-month | 3.80 | 19,677 | 13,969 | 4950 | 38.76 | 9.033 | –189 | 0.018 | Dominant | 548 |
Moderate depression | Severe depression | |||||||||||||
---|---|---|---|---|---|---|---|---|---|---|---|---|---|---|
Total costs (£) | Total QALYs | Net benefit (£) | Incremental analysis | Total costs (£) | Total QALYs | Net benefit (£) | Incremental analysis | |||||||
Costs (£) | QALYs | ICER | INB (£) | Costs (£) | QALYs | ICER | INB (£) | |||||||
Placebo and ‘typical treatment’ armsa | ||||||||||||||
Placebo | 201,315 | 61.13 | 1,021,249 | NA | 225,470 | 49.38 | 762,142 | NA | ||||||
Treatment | 188,274 | 62.78 | 1,067,300 | –13,041 | 1.65 | Dominant | 46,051 | 212,965 | 51.37 | 814,349 | –12,505 | 1.99 | Dominant | 52,207 |
28-day and 3-month prescribing scenarios based on Taitel et al.45 (RR = 0.748) | ||||||||||||||
28-day | 219,983 | 62.36 | 1,027,268 | NA | 247,988 | 50.87 | 769,315 | NA | ||||||
3-month | 189,445 | 62.78 | 1,066,129 | –30,538 | 0.42 | Dominant | 38,862 | 214,135 | 51.37 | 813,178 | –33,853 | 0.50 | Dominant | 43,863 |
28-day and 3-month prescribing scenarios based on Pfeiffer et al.37 (RR = 0.542) | ||||||||||||||
28-day | 223,187 | 62.02 | 1,017,273 | NA | 251,067 | 50.46 | 758,070 | NA | ||||||
3-month | 189,445 | 62.78 | 1,066,129 | –33,743 | 0.76 | Dominant | 48,856 | 214,135 | 51.37 | 813,178 | –36,931 | 0.91 | Dominant | 55,108 |
Sensitivity analyses | ||||||||||||||
28-day and 3-month prescribing scenarios based on upper bound of Taitel et al.45 RR estimate (RR = 0.780) | ||||||||||||||
28-day | 219,487 | 62.42 | 1,028,814 | NA | 247,512 | 50.93 | 771,055 | NA | ||||||
3-month | 189,445 | 62.78 | 1,066,129 | –30,043 | 0.36 | Dominant | 37,315 | 214,135 | 51.37 | 813,178 | –33,376 | 0.44 | Dominant | 42,123 |
28-day and 3-month prescribing scenarios based on lower bound of Pfeiffer et al.37 RR estimate (RR = 0.540) | ||||||||||||||
28-day | 223,221 | 62.02 | 1,017,168 | NA | 251,099 | 50.45 | 757,952 | NA | ||||||
3-month | 189,445 | 62.78 | 1,066,129 | –33,776 | 0.76 | Dominant | 48,961 | 214,135 | 51.37 | 813,178 | –36,963 | 0.91 | Dominant | 55,226 |
Total lifetime cost (£) | Total lifetime QALYs | Net benefit (£) | Incremental analysis | INB (£) | |||
---|---|---|---|---|---|---|---|
Costs (£) | QALYs | ICER | |||||
Source model: no intervention and weighted treatment comparators | |||||||
No intervention | 5117 | 9.57 | 186,283 | NA | NA | NA | NA |
Weighted treatment | 4503 | 10.16 | 198,602 | –614 | 0.59 | Dominant | 12,319 |
First approach based on Hermes et al.35 (RR = 0.916) | |||||||
28-day | 5413 | 10.12 | 196,756 | NA | NA | NA | NA |
90-day | 4795 | 10.16 | 198,310 | –618 | 0.05 | Dominant | 1554 |
First approach based on Taitel et al.45 (RR = 0.851) | |||||||
28-day | 5470 | 10.07 | 195,879 | NA | NA | NA | NA |
90-day | 4795 | 10.16 | 198,310 | –675 | 0.09 | Dominant | 2431 |
Second approach based on Hermes et al.35 (RR = 0.916) | |||||||
28-day | 5416 | 10.10 | 196,680 | NA | NA | NA | NA |
90-day | 4795 | 10.16 | 198,310 | –621 | 0.05 | Dominant | 1630 |
Second approach based on Taitel et al.45 (RR = 0.851) | |||||||
28-day | 5476 | 10.06 | 195,773 | NA | NA | NA | NA |
90-day | 4795 | 10.16 | 198,310 | –681 | 0.09 | Dominant | 2537 |
Sensitivity analysis | |||||||
First approach based on upper bound of Hermes et al.35 RR estimate (RR = 0.928) | |||||||
28-day | 5406 | 10.11 | 196,859 | NA | NA | NA | NA |
90-day | 4795 | 10.16 | 198,310 | –611 | 0.04 | Dominant | 1451 |
First approach based on lower bound of Taitel et al.45 RR estimate (RR = 0.846) | |||||||
28-day | 5474 | 10.07 | 195,831 | NA | NA | NA | NA |
90-day | 4795 | 10.16 | 198,310 | –678 | 0.09 | Dominant | 2479 |
Case study 1: primary prevention of cardiovascular events in patients with recent onset type 2 diabetes mellitus
The results for each case study are structured as per the methods overview presented in Table 17.
Stage 1: identify relevant data and decision models
1a
The systematic review (see Chapter 2) identified two studies, both of which indicated a positive relationship between prescription length and adherence in antidiabetic medications. 35,45 This positive relationship was consistent with that identified in all studies in the systematic review, regardless of disease area. No studies examined the relationship between prescription length (or adherence) and health outcomes, and no decision models were identified.
1b
Relevant NICE guidance on the management of T2DM in adults was reviewed. The most recent NICE guidance (NG28, updated July 2016) included a decision model that assessed the cost-effectiveness of a large number of pharmacological blood glucose-lowering therapies for adults with T2DM at three discrete stages of disease progression (initial therapy, first stage and second stage treatment intensification). 79 Table 18 provides an overview of the model that was developed by NICE but is itself a modified version of the UK Prospective Diabetes Study (UKPDS) Outcomes Model Version 1, which is described in detail elsewhere. 79,97 Briefly, the model is a patient-level simulation that estimates the first occurrence of each of seven diabetes-related complications (fatal or non-fatal myocardial infarction, other ischaemic heart disease, stroke, heart failure, amputation, renal failure and eye disease) and death in order to estimate lifetime QALYs for UK populations with T2DM. It is based on patient-level data from the UKPDS, a landmark, randomised, multicentre trial of glycaemic therapies in patients with newly diagnosed T2DM, which extends to 20 years of follow-up. 97 The model is run in Microsoft Excel, with a time horizon of a lifetime.
The modifications made to the model by NICE included the addition of data on the costs and treatment effects of the various interventions under examination. The model is the first to analyse the cost-effectiveness of initial therapy (i.e. monotherapy with one oral antidiabetic drug) for T2DM in a setting relevant to the UK. In this case of initial therapy, for example, lifetime costs and QALYs for seven therapies (six pharmacological interventions, e.g. metformin, and placebo) were compared on the assumption that patients would later move on to metformin–sulfonylurea and metformin–neutral protamine Hagedorn insulin as first- and second-stage intensification treatments.
1c
The original model already included a placebo comparator. Hence, it was not necessary to identify additional data on the incremental health benefits of commonly prescribed medications when compared with ‘no treatment’.
As the decision model did not include dispensing fees, we identified these from the NHS Drug Tariff. 60 The costs of prescriber time (see Table 13) and wastage (see Table 14) were identified in the CPRD analysis of initial glucose control in type 2 diabetes (see Chapter 3).
Stage 2: adapt the decision model
2a
In this case study, we focused on the impact of different prescription lengths at the initial therapy stage. The existing structure of the model and all model inputs remained unchanged, as the identified model already examined the costs and consequences of a wide range of pharmacological interventions for T2DM that are currently prescribed in general practice in the UK.
2b
Table 20 shows the lifetime costs and QALYs for metformin and placebo (two of the seven comparators in the original model), which were estimated using the original source model, with the cost outputs of the model inflated to 2015 prices. Metformin was chosen for use in this case study because it is current first-line practice, and was the most cost-effective option, dominating all other comparators, yielding expected lifetime costs and QALYs of £19,639 and 9.033, respectively. For comparison, vildagliptin was associated with lifetime costs and QALYs of £21,043 and 8.954. Unsurprisingly, the lifetime QALYs associated with the placebo were lower than those of the other six treatments. However, costs were higher for the placebo than for metformin, owing to a higher risk of diabetes-related complications and higher treatment costs as a result of more rapid progression to first- and second-stage intensification therapies (metformin–sulfonylurea and metformin–NPH). For these reasons, metformin dominated placebo (INB £3231). In a pairwise probabilistic comparison of treatment options for initial therapy reported in the NICE guidelines, there were no instances in which placebo was cost-effective at the £20,000 per QALY threshold.
We estimated the lifetime costs and QALYs associated with 3-month and 28-day prescribing of metformin. As stated previously, we assumed that the 3-month prescribing yielded equivalent costs and outcomes to the metformin treatment arm in the source model (i.e. p = 1 as per Figure 9). To this was added additional annual transactional (dispensing fees and prescriber time) and drug wastage costs {£10.34 per annum [dispensing fees (£0.90) + prescriber time (£0.28) + wastage costs (£1.37)] × [365/90]} for the period of time the average patient received initial treatment (2.3 years).
For the 28-day prescriptions, we took a weighted average of the costs and QALYs of the metformin and placebo arms according to the RR of being adherent reported by Hermes et al. 35 and Taitel et al. ,45 which were 0.891 and 0.863, respectively. These are reported as two separate scenario analyses. 35,45 The total costs and QALYs for the 28-day prescriptions in the first scenario are calculated as (0.891 × metformin arm costs or QALYs) + (0.109 × placebo arm costs or QALYs). To these calculated total costs were added the additional transactional and drug wastage costs for the period of time the average patient received initial treatment (3.64 years in the first scenario). In both scenarios, 3-month prescriptions were less costly and yielded higher QALYs than 28-day prescriptions, with expected INBs of £423 and £511, respectively.
2c
We assessed uncertainty in our estimates using the lower bound of the 95% CI in the RR estimate reported by Taitel et al. 45 (RR = 0.851) and the upper bound of the RR estimate reported by Hermes et al. 35 (RR = 0.926). In both cases, the INB was positive (see sensitivity analyses reported in Table 20).
Case study 2: selective serotonin reuptake inhibitors
Stage 1: identify relevant data and decision models
1a
The systematic review (see Chapter 2) identified two studies that had examined the relationship between prescription length and adherence in antidepressant medications. 37,45 No studies examined the relationship between prescription length (or adherence) and health outcomes, and no decision models were identified.
1b
We reviewed relevant NICE guidance on depression in adults. The most recent NICE guidance (CG90, published October 2009 and updated April 2016) included a decision model that examined the cost-effectiveness of alternative pharmacological interventions (10 different groups of antidepressants were assessed overall) with or without cognitive–behavioural therapy (CBT). 95 Table 18 provides an overview of the model, which is a decision tree with a 15-month time horizon. The model is operated in Microsoft Excel.
As Figure 11 shows, in an example with two treatment arms, there are 12 possible pathways through the model. Each pathway has probability nodes attached to three discrete treatment phases. These treatment phases are a three-month acute treatment phase, a 6-month maintenance period (which together form a 9-month ‘treatment phase’) and a 6-month follow-up phase. Probability nodes in the model reflect the likelihood of dropout (during the acute phase), no remission (during the maintenance period) and relapse (during the follow-up phase). Costs (from the NHS perspective; see Table 29, Appendix 9) and QALYs (derived from another study93) are assigned to each of the pathways through the model. The results for each analysis were reported for two separate cohorts of 100 patients with moderate and severe depression. Patients with severe depression faced higher patient monitoring costs than those with moderate depression because they had hospital outpatient mental health consultations. Deterministic sensitivity analysis was carried out on the upper and lower 95% credible intervals around some of the input parameters (e.g. response and dropout probabilities). The guideline states that probabilistic sensitivity analysis was not possible because of limited access to data from a network meta-analysis from which many of the input parameters were derived. 95
FIGURE 11.
Case study 2: adapted decision tree.
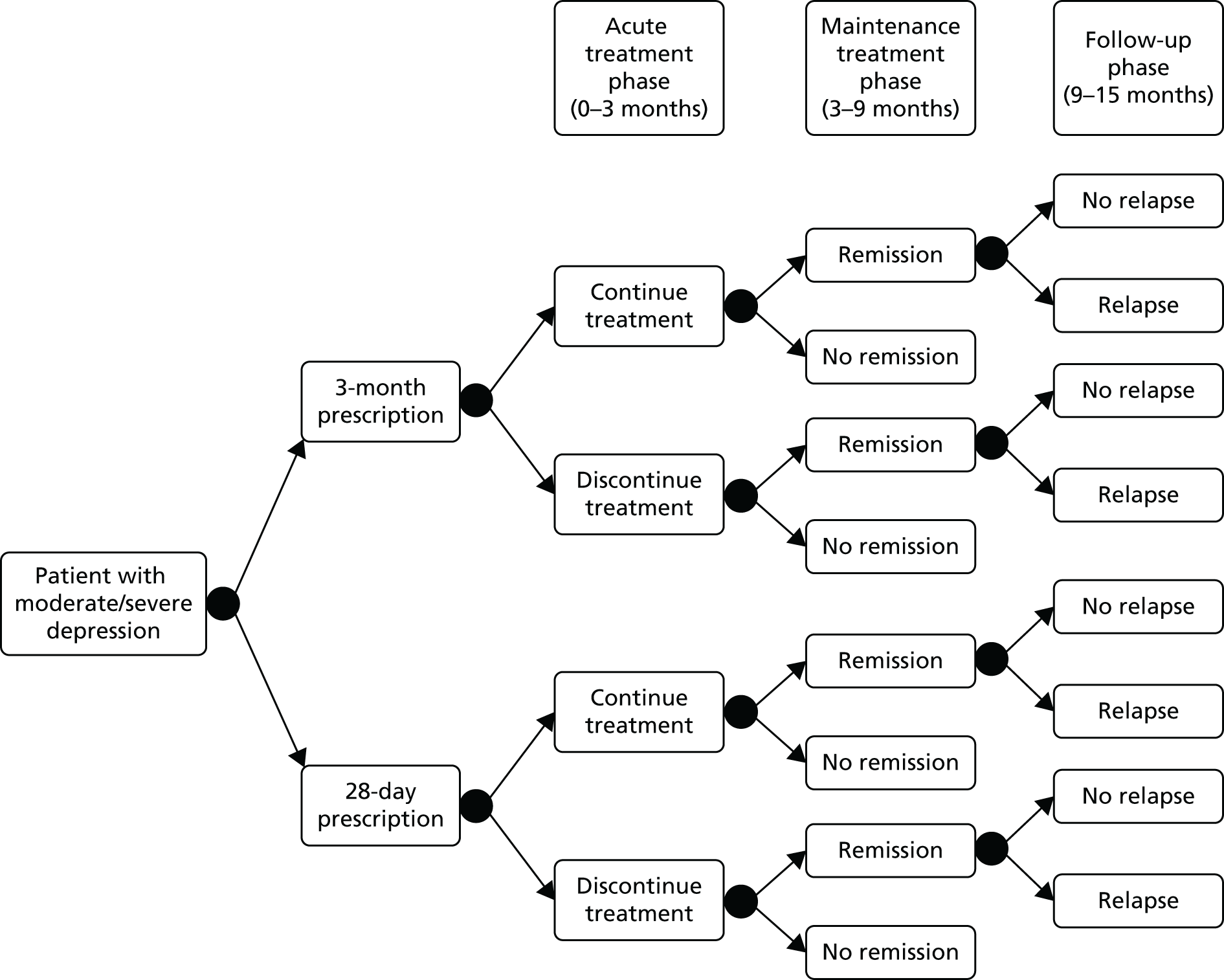
1c
Although the original decision model did not include a placebo arm, the full NICE guidance did include a separate clinical evidence review, which included data on the health consequences of specific pharmacological interventions when compared with placebo. These data were used to inform our model adaptations.
As the decision model did not include dispensing fees, we identified these from the NHS Drug Tariff. 60 The costs of prescriber time (see Table 13) and wastage (see Table 14) were identified in the CPRD analysis of depression (see Chapter 3).
Stage 2: adapt the decision model
2a
In this case study, we maintained the overall model structure since the identified model had recently been updated by NICE in 2016 and already examined the costs and consequences of antidepressants currently prescribed in general practice. However, when the original treatment arms emanating from the decision node in the model were two comparable pharamacological interventions (or a pharamacological intervention with and without CBT), in our adapted model (see Figure 11), we substituted instead 3-month and 28-day prescribing of a ‘typical’ SSRI.
The unit cost data for our ‘typical’ SSRI were based on a weighted average of the costs associated with 10 groups of antidepressant medications. The weighted average was calculated using unit cost data for each group of medications in the source model and data from the PCA (published by NHS England), which showed the total number of items dispensed in the community in 2014 for each group of medications. 84 For the 3-month and 28-day arms of the decision tree, we added the appropriate transaction and wastage costs to these SSRI unit costs.
The health consequence data for our ‘typical’ SSRI were based on data reported in the NICE clinical evidence review on the absolute risk of dropout, no remission and relapse for a placebo arm, and the RR for an escitalopram treatment arm. These data were used to calculate the probability nodes in the decision tree where the placebo arm represented our zero adherence scenario and the escitalopram treatment arm represented our perfect adherence scenario (see Table 28, Appendix 9, for an overview of the health consequence data). As the NICE guideline development group concluded that there was sufficient doubt about the clinical importance of differences between antidepressant treatments to not justify the development of recommendations for specific drugs,95 we did not examine data on the relative efficacy of other antidepressants compared with escitalopram.
2b
Replicating the approach taken by NICE using the source model, we reported the model outputs in terms of the total costs and QALYs of the two arms of the decision tree for two separate cohorts of 100 patients with moderate and severe depression (see Table 21).
We compared the costs and QALYs of SSRI of a treatment arm versus a placebo arm, prior to inclusion of the additional transaction and wastage costs associated with 28-day and 3-month prescribing. For patients with moderate and severe depression, SSRI treatment was less costly (£188,274 vs. £201,315 in the case of moderate depression) than placebo with higher QALY gains (62.78 vs. 61.13 in the case of moderate depression) (see Table 21). Comparing SSRI treatment with placebo for moderate and severe depression resulted in INBs of £46,051 and £52,207.
Our 3-month treatment arm was based on data for perfect adherence. As described in case study 1, the costs and QALYs for our 28-day treatment arm were calculated using a weighted average of the costs and QALYs associated with perfect and zero adherence based on the evidence from studies on the relationship between prescription length and adherence identified in the systematic review. These studies, by Pfeiffer et al. 37 and Taitel et al. ,45 reported a RR of 0.542 and 0.748 respectively. Where RR = 0.542, 3-month prescriptions were less costly than 28-day prescriptions (£189,445 vs. £223,221 in the case of moderate depression) and with higher QALY gains of 0.76 QALYs (62.78 vs. 62.02). In both moderate and severe depression, the INB was positive (see Table 21).
When the RR = 0.748, 3-month prescriptions were less costly than 28-day prescriptions (£189,445 vs. £219,983 in the case of moderate depression) and had higher QALY gains of 0.42 QALYs (62.78 vs. 62.36 in the case of moderate depression). Again, in both moderate and severe depression, the INB was positive (see Table 21).
2c
First, we assessed uncertainty in our estimates using the lower bound of the 95% CI in the RR estimate reported by Pfeiffer et al. 37 (RR = 0.540) and the upper bound of the RR estimate reported by Taitel et al. 45 (RR = 0.780). In both cases, for moderate and severe depression, the ICERs remained negative and the INB was positive (see the sensitivity analyses reported in Table 21).
Second, following the original source model, we also completed sensitivity analyses around maximum and minimum plausible values of the health state utilities and the RR of dropout, no remission and relapse (for SSRI when compared with placebo). These values are shown in Table 28, Appendix 9. In all cases, the ICER remained negative and the INB was positive. For example, using the lower bound estimates of the RR of dropout, no remission and relapse, the INB for moderate depression ranged from £53,346 (when based on Taitel et al. 45 data) to £76,593 (when based on Pfeiffer et al. 37 data).
Case study 3: secondary prevention of cardiovascular events
Stage 1: identify relevant data and decision models
1a
The systematic review (see Chapter 2) identified two studies, both of which indicated a positive relationship between prescription length and adherence in antihypertensive medications. 35,45 As in the other case studies, we found no studies that had examined the relationship between prescription length (or adherence) and health outcomes, and no decision models were identified.
1b
We reviewed relevant NICE guidance on pharmacological interventions for adults with hypertension. The most recent NICE guidance (CG127, updated 2011) included an economic model that assessed the cost-effectiveness of four groups of alternative antihypertensive medications (ACEIs/ARBs, beta-blockers, calcium-channel blockers, thiazide-type diuretics) and a ‘no treatment’ comparator. 91 Table 18 provides an overview of the model, which is a Markov model with seven health states (event-free/well, myocardial infarction, unstable angina, stroke, diabetes, heart failure and death). Figure 12, Appendix 9, provides a schematic representation of the model. All patients start in the event-free/well health state and, during each 6-month cycle of the model, a proportion of all patients enter each of the other health states. The likelihood of moving between states during each model cycle is determined by transition probabilities, which vary according to patient characteristics (e.g. age, sex, ethnicity and baseline health event risks) and the prescribed medication. The baseline risk for each health state in an untreated population is based on published studies, and these risks are adjusted for each prescribed medication using published data on the RRs versus no treatment (see Table 30, Appendix 10). Health state utilities are also derived from published studies, and these are used to calculate QALYs. The annual costs associated with each health state (from the NHS perspective), and the costs of medications, are used as inputs in the model drawn from a variety of published studies and the BNF (see Table 18). The model is run in Microsoft Excel for 1000 patients at a time, with a time horizon of a lifetime.
1c
It was not necessary to identify additional input data on the costs and health consequences of zero adherence, as the source model included a ‘no treatment’ comparator. As the decision model did not include dispensing fees, we identified these from the NHS Drug Tariff. 60 The costs of prescriber time (see Table 13) and wastage (see Table 14) were identified in the CPRD analysis of ‘secondary prevention of myocardial infarction.’
Stage 2: adapt existing model
2a
In this case study, we maintained the overall model structure, since the source model already examined the costs and consequences of the antihypertensive interventions currently prescribed in general practice. For all our analyses, our cohort was based on a 65-year-old male with an annual CVD risk of 2%, heart failure risk of 1% and diabetes risk of 1.1%, as was the case in the base case model reported in the NICE guidance.
2b
The source model provided estimates of the total lifetime costs and QALYs for each of the four groups of antihypertensive medications and the ‘no treatment’ comparator (these are reported in Table 31 (see Appendix 10) alongside the ICER for each treatment compared with ‘no treatment’). For example, for the ‘no treatment’ comparator, the total costs were £5117 and the total QALYs were 9.57. Using the estimates for the four groups of antihypertensive medications, we created our own ‘typical treatment’ comparator, which was a weighted average of the costs and QALYs associated with each of the four groups of antihypertensive medications. The weighted average was based on PCA figures published by NHS England, which showed the total number of items dispensed in the community in 2014 for each of the four groups of antihypertensive medications. 84 For this ‘typical treatment’ comparator, the total costs were £4503 and QALYs were 10.16. When comparing the ‘typical treatment’ with the ‘no treatment’ comparator, the INB was positive at £12,319. These results are shown in Table 22.
We estimated the lifetime costs and QALYs associated with 3-month and 28-day prescribing of antihypertensive medications using two different approaches. Both approaches involved adapting the cost inputs to the model to reflect different prescription lengths. However, the second approach differs from the first in that the relative treatment effects (vs. no treatment) for each health state (e.g. diabetes; see Table 30, Appendix 10) were also adjusted to reflect different adherence levels.
First, we created 3-month and 28-day treatment groups by adjusting the cost inputs and the QALY outputs of the source model. The cost inputs in the model for each of the four antihypertensive treatment groups were adjusted by adding the additional transactional and drug wastage costs to the annual drug costs already included in the model. These additional costs were calculated on an annual basis as the sum of the additional costs per prescription multiplied by the annual number of prescriptions.
In the 3-month treatment group, after the additional costs of £21.01 per annum {[dispensing fees (£0.90) + prescriber time (£3.77) + wastage costs (£0.51)] × [365/90]} were added to the four treatment groups in the model, the total cost and total QALY outputs were calculated as per the ‘typical treatment’ comparator described above.
In the 28-day treatment group, the total QALYs were a weighted average of the QALYs in the ‘typical treatment’ and the ‘no treatment’ comparators. The weighted average was calculated using the method described in the other case studies and based on evidence from the studies by Hermes et al. 35 and Taitel et al. 45 on the relationship between prescription length and adherence, which reported RRs of 0.92 and 0.85, respectively. 35,45 Thus, using the Hermes data as an example, the total QALYs were calculated as the sum of 0.92 multiplied by ‘typical treatment’ QALYs and 0.08 multiplied by ‘no treatment’ QALYs.
The total costs in the 28-day treatment group were a weighted average of a perfect and a zero adherence scenario using the same RRs identified in the Hermes et al. 35 and Taitel et al. 45 studies. The perfect adherence scenario was calculated as per the 3-month treatment group above, but with £61.68 of additional costs per annum {[dispensing fees (£0.90) + prescriber time (£3.76) + wastage costs (£0.07)] × [365/28]}. The zero adherence scenario was calculated as per the perfect adherence scenario, however the RRs associated with treatment (vs. no treatment) for each health state were adjusted in the model from RR < 1 (as reported in Table 30, Appendix 10) to RR = 1.
The results for this first approach are reported in Table 22. In all analyses, the 3-month prescriptions were less costly and yielded more QALYs than the 28-day prescriptions. The expected INB ranged from £1554 (using Hermes et al. ’s35 RR) to £2431 (using Taitel et al. ’s45 RR).
In our second approach, the 3-month treatment group remained unchanged. For the 28-day treatment group, the cost inputs were adjusted following the same method used in the first approach. The relative treatment effects associated with each of the four treatments (vs. no treatment) were also adjusted for each of the six health states in the model (myocardial infarction, unstable angina, stroke, diabetes, heart failure and death). These relative treatment effects (24 in total) were adjusted using a weighted average of those used in the source model and a zero adherence scenario (i.e. relative treatment effect = 1) using the data from the Hermes et al. 35 and Taitel et al. 45 studies. Thus, in an example using the Hermes data, where RR = 0.92, the relative treatment effect for unstable angina when prescribed thiazide-type diuretics was 0.901 [(0.893 × 0.92) + (1 × 0.08) where 0.893 = relative treatment effect in the source model].
The results are reported in Table 22. In all analyses, the 3-month prescriptions are less costly and yield more QALYs than 28-day prescriptions. The expected INB ranges from £1630 (using Hermes et al. ’s35 RR) to £2537 (using Taitel et al. ’s45 RR).
2c
First, we assessed uncertainty in our estimates using the lower bound of the 95% CI in the RR estimate reported by Taitel et al. 45 and the upper bound of the RR estimate reported by Hermes et al. 35 (see Table 22). Second, we replicated the four-way sensitivity analysis used in the NICE source model. This included a range of plausible estimates for age, annual risk of CVD, diabetes and heart failure. In all cases, the ICERs remained negative and the INB was positive.
Discussion
The systematic review and CPRD analyses reported in Chapters 2 and 3 provided consistent evidence that longer prescription lengths are associated with increased adherence but increased wastage. However, the CPRD analyses showed that reductions in transaction costs associated with longer prescriptions more than compensated for the increased wastage costs, at least in the case studies considered. The modelling component presented in the current chapter builds on these findings by attempting to quantify the overall costs incurred by the NHS, and the resulting outcomes from 3-month versus 28-day prescription lengths over a longer time horizon.
In all three case studies, longer prescriptions were associated with lower costs and higher QALYs than shorter prescription lengths. The underlying reason for this finding was improved adherence in the 3-month scenarios, which resulted from the evidence identified in the systematic review. However, in contrast to the studies in the systematic review, our models were able to account for the medium- and longer-term QALY gains and cost savings accrued by the NHS through reductions in health-care utilisation that arose from improved adherence. As a result, the INB for 3-month prescription lengths was always positive. Notably, this was the case despite the models having different time horizons owing to the nature of the case study treatments. Case studies 1 and 3 focus on the prevention of future cardiovascular events, so increased adherence reduces the expected costs. In contrast, case study 2 focused on SSRI treatment for moderate and severe depression, a chronic or episodic condition, and hence there are no potential ‘downstream’ savings from avoided future events. In this second case study, the lower costs in the 28-day arm are also a result of reduced drug costs for patients who discontinued their treatment during the acute treatment phase (i.e. reduced persistence; see Table 29, Appendix 9) and thus were prescribed treatment for only 28 days rather than for 3 months.
The finding of QALY gains and cost savings associated with 3-month prescription lengths was also consistently found in our sensitivity analyses, which assessed a plausible range of various inputs to the models including the RRs of being adherent. The finding would also be unaffected by any change to the two key assumptions introduced in the Methods section of this chapter on the relationship between adherence and health outcomes (namely the assumptions of a linear dose–response curve and the maximum effect being equivalent to a comparison of model active and placebo/no treatment arms of clinical trials), although the magnitude of QALY gains, cost savings and INB would be affected. Longer prescriptions would only be associated with a negative INB if they resulted in a decline in treatment effect. This appears unlikely, as our systematic review of the evidence (see Chapter 2) suggests a statistically significantly positive association with adherence, and it is reasonable to suppose improved adherence will not be associated with reduced treatment effect. The major caveat to bear in mind alongside this, however, is that the studies included in the review are rated as having a high risk of bias.
Compared with the literature review, which identified just one study that had examined the relationship between health outcomes and prescription length,39 our study has provided an indication of the magnitude of change in health outcomes (QALYs) that might be associated with 3-month prescription lengths in comparison with 28-day prescription lengths as a consequence of the impact on adherence to treatment. Unsurprisingly, owing to the finding in the systematic review of a positive relationship between prescription length and adherence, all of the results reported in this section suggested that longer prescriptions were associated with more QALYs. This was also the finding of the Batal et al. 39 study, although that study was restricted to a single health-related outcome (cholesterol level) and short-term follow-up. By examining longer-term follow-up, we have been able to include the health consequences of a reduction in longer-term complications and associated treatments.
The finding of a negative relationship between costs and prescription length in the case studies in this section was consistent with four42,45,46,49 of the five42,44–46,49 studies identified in the systematic review, but the relationship arose for different reasons. As these four studies in the systematic review had examined the costs to third-party payers over a short time horizon, cost savings arose in longer prescriptions in those studies because of reductions in some of the administrative costs of prescribing medication, for example dispensing fees. However, these studies did not account for the changes in wider health-care expenditure that would result, particularly in the longer term, from the changes in health status associated with different adherence levels. Conversely, although the fifth study identified in the systematic review44 did examine those wider health-care expenditures, it was the only study to identify a positive relationship between prescription length and costs. This is likely to be because the health-care expenditures were examined over only a short time horizon, whereas two of the case studies in this section examined the costs of health care associated with the longer-term health consequences over a lifetime. Furthermore, the five studies42,44–46,49 identified in the systematic review that examined the relationship between prescription length and costs were US based and generally from a particular perspective (e.g. Veterans Affairs or Medicaid), and thus may not be directly comparable to the UK setting.
Limitations of the decision modelling
The three case studies are based on existing, good-quality decision models, all of which have been used to inform policy in the past. However, there are a number of limitations to our analyses.
First, our assumption of a positive relationship between prescription length and adherence was based on a small number of studies (n = 2 in each case study) identified in the systematic review. The limitations of these studies are fully assessed in the systematic review section, but, crucially, they were observational, not randomised experimental studies, and so are potentially subject to bias. Other potential sources of bias include those common to all decision modelling, namely validity of all input data on cost and effectiveness, whether or not the structure of the model is plausible and whether or not the model is ‘consistent’ (Philips et al. 98). In contrast to other studies that have used decision modelling, including the evaluation of the NMS by Boyd et al. ,90 which assessed the longer-term cost and health impacts of a policy change in pharmacy to support increased adherence, our study was limited because it was not based on evidence from a robust RCT.
Second, we assumed that the accrued costs and QALYs in the treatment arms of the models equated to ‘perfect adherence’, or at least to ‘best adherence’, and that the placebo or no treatment arms represented zero adherence. Given prior belief (informed by the systematic review) that longer prescriptions are associated with better adherence, we set the 3-month prescription costs and QALYs equal to the treatment arm costs and QALYs. The 28-day prescription costs and QALYs were assumed to be a weighted average of the treatment and placebo arm according to the RR of being adherent (as described in the Methods section of this chapter, the RR of being adherent in those studies was based on two standard measures of adherence: a dichotomised measure, based on the percentage of patients with ≥ 80% medication adherence, and the MPR, i.e. days covered per measurement period). These were necessary assumptions owing to the lack of better-quality data.
There are several points to note. First, except for the additional transaction and wastage costs, the ICER is insensitive to the ‘starting point’ for the analysis. For example, the opposite extreme would have been to assume that the 28-day prescription arm costs and QALYs were equal to those in the placebo/no treatment arm, and to define the 3-month prescription arm as a weighted average of 1 – 1/RR of the treatment arm costs and QALYs and 1/RR of the no treatment arm. This would yield the same ICER as illustrated in Figure 10 and Equation 5:
where λ = willingness to pay for a unit of outcome and E = QALYs (NICE assigns a value of £20,000–30,000 to λ).
Second, as stated in the Methods section of this chapter, we have used ‘no treatment’ and ‘placebo’ interchangeably in the analysis and, as a result, we may have underestimated the health gain from increased adherence as a result of longer prescription lengths.
Other limitations were that we assumed adherence did not change over the period of the analysis, and, although we took a NHS perspective, we excluded any revenue gained by the NHS from prescription charges. Patients with diabetes are exempt from the prescription charge, and almost 90% of prescriptions dispensed in the community in England do not attract any charge; therefore, any revenue would be minimal and thus unlikely to change the results of our analysis. 88 Costs of drugs were based on the lowest price brand and pack size listed in the BNF, and we did not consider any combination preparations. If more expensive versions were used, then the cost savings through reduced wastage would be higher than those suggested here.
Chapter 5 Conclusions and recommendations for further work
The systematic review and CPRD cost analyses provided consistent evidence that longer prescription lengths were associated with modest improvements in adherence but also with increased wastage. However, despite increased waste, there was evidence that longer prescriptions result in net cost savings as a result of reductions in costs associated with dispensing fees and prescriber time, which outweigh wastage costs. Based on the findings of the CPRD analyses, the biggest impact on costs was prescribers’ time. Overall, the CPRD analyses predicted that TUC savings ranged from £6.33 to £9.07 for the five conditions studied (glucose control with oral drug therapy in T2DM, treatment of hypertension in T2DM, lipid management in T2DM, treatment for the secondary prevention of myocardial infarction, and depression) when total costs were standardised to a common 90-day time period. Switching to longer prescriptions could potentially result in substantial savings to the NHS. For example, if the 95% of prescriptions for antidepressants that are for < 60 days’ supply were issued as longer prescriptions, the total savings for the NHS could be as much as £305M per year. If the model is restricted to just dispensing fees, savings would be in the region of £78M per year. This portion could be considered cash releasing, and the remaining portion would be accounted for by freed-up GP time.
The decision modelling exercise quantified overall costs and outcomes associated with longer versus shorter duration prescriptions over a longer time horizon. In all three cases, after accounting for differences in prescribing time, dispensing fees and wastage costs, longer prescription lengths were associated with lower costs incurred by the NHS as a result of reductions in the cost of treating disease-specific health complications. In all cases, longer prescriptions were assumed to lead to better health outcomes as a result of increased medication adherence.
The quality of evidence included in this study is considered to be weak; the findings from all three research strands are based on retrospective analysis of administrative data. Despite the limitations of the study, the consistency between the results of the systematic review and CPRD analyses, and across case studies within the CPRD analyses, increases the confidence in our conclusions that longer prescriptions are associated with short-term cost savings as a result of reductions in transaction costs. Broadening our analysis to consider differences in adherence and out-of-pocket expenses incurred by the patient, such as patient time and travel costs, is likely to further increase the cost savings associated with issuing longer prescription lengths.
There is less certainty with regard to long-term costs. The validity of the finding from the decision modelling that long-term cost savings were achieved is underpinned by the assumption that improved adherence is associated with improved health outcomes. This study provides no direct evidence of the impact of differing prescription lengths on health outcomes.
Overall, the current evidence base does not support current policies promoting shorter prescription lengths over longer prescription lengths. If medication adherence is positively correlated with health outcomes, as seems to be suggested by the wider literature,54,55 there may be clinical benefits of increasing the length of repeat prescriptions for patients with chronic conditions. Based on the reported cost savings associated with longer prescription lengths in our study, extrapolating the mean values to populations with stable chronic conditions in the UK could also result in substantial savings to the NHS.
Recommendations for future research
There is a need to more reliably evaluate the impact of differing prescription lengths on adherence, patient health outcomes and impact on total costs to the NHS. This need can be addressed through a variety of means. First, a prospective cluster RCT could provide some insights into the health impacts of different prescription lengths for well-defined patient groups. For example, within a chosen disease group, one could consider comparing patients who are new to treatment with patients who have been receiving treatment for years, exploring the different parameters of long-term management and adherence. Second, observational studies focusing on patient experience, the patient–provider relationship and on the provider’s time management would complement the evidence base by looking at outcomes that have so far been rarely studied in relation to prescription length, adding a much-needed individual-centred dimension to the more widely researched cost aspect of prescribing issues. Third, more modelling studies could build on the growing accessibility, linkage possibilities and quality of large primary care databases to look at the effectiveness and cost-effectiveness of prescription length on a wider range of conditions in a fashion similar to the one used in this study. Overall, the priority for future research should be to identify conditions or groups of patients that should receive shorter or longer prescriptions. To determine the need for any further research, an expected value of perfect information (EVPI) analysis should be performed. It has not been possible to conduct an EVPI analysis based on data generated in this study, as the analysis of uncertainty in the modelling in Chapter 3 was limited to a multiway sensitivity analysis, rather than to a probabilistic sensitivity analysis.
A further strand of future research could be to explore means of quantifying the high risk of bias in the existing literature. The obvious approach would be through a well-conducted prospective randomised study as suggested above. In the absence of this, the only alternative would be to seek expert opinion. This could be elicited using a recognised tool such as the Sheffield Elicitation Framework (SHELF). 99 The results of this systematic review of the effect of longer duration of prescriptions on adherence and the associated manuscripts would form the background material, and the meta-analysis would form the prior distribution of effect. At the workshop, participants would dissect the manuscripts in detail, with a view to quantifying the bias, and revise the prior distribution to generate a posterior distribution of effect. Inputting this posterior distribution into the decision models and converting them to probabilistic analyses would allow a value of information analysis to determine whether or not a randomised trial would be of value to society.
Future studies should seek to standardise measurements of adherence and wastage to ease interpretation of findings and to increase comparability across settings. In addition, future research should shift from comparing dichotomous prescription lengths to comparing a range of different prescription lengths in order to identify the optimal prescription length for patients receiving repeat prescriptions for stable chronic conditions.
Acknowledgements
We would like to thank a number of people who contributed to the successful completion of this study. Jody Larkin (Research Librarian, Knowledge Services, RAND Corporation) contributed to the development of the systematic review protocol and conducted the literature searches for the systematic review.
Jon Sussex (RAND Europe) acted in a quality assurance role and provided valuable insight throughout the project. Rachel Elliott (Professor of Medicines and Health, School of Pharmacy, University of Nottingham) provided advice and guidance for the economic modelling. Finally, we would like to thank the patient and public involvement representatives of the INsPIRE group for their support and comments.
Contributions of authors
Céline Miani [Senior Analyst, Cambridge Centre for Health Services Research (CCHSR), RAND Europe] co-led the systematic review and co-co-ordinated the preparation of this report for publication.
Adam Martin (Analyst, CCHSR, RAND Europe) contributed to the systematic review and co-led the economic modelling.
Josephine Exley (Senior Analyst, CCHSR, RAND Europe) co-led the systematic review and co-co-ordinated the preparation of this report for publication.
Brett Doble (Research Associate, CCHSR, Institute of Public Health, University of Cambridge) co-led the CPRD analysis.
Ed Wilson (Senior Research Associate, CCHSR, Institute of Public Health, University of Cambridge) co-led the CPRD analysis and the economic modelling.
Rupert Payne (Consultant Senior Lecturer, Centre for Academic Primary Care, University of Bristol) provided expert advice and guidance for the whole study.
Anthony Avery (Professor of Primary Health Care, Dean of the School of Medicine, School of Medicine, University of Nottingham) provided advice and guidance for the whole study.
Catherine Meads (Research Leader, CCHSR, RAND Europe) provided intellectual oversight for the whole study.
Anne Kirtley (Insight Research Analyst, Strategy Division, Wellcome Trust) contributed to the systematic review.
Molly Morgan Jones (Associate Director, RAND Europe) was the principal investigator on the project from September 2015. She was involved in the reviewing of findings and provided oversight for the study.
Sarah King (Visiting Fellow, Institute of Public Health, University of Cambridge) conceived the study and co-led the systematic review.
All authors reviewed and contributed to the overall synthesis.
Publications
Doble B, Payne R, Harshfield A, Wilson ECF. Retrospective, multicohort analysis of the Clinical Practice Research Datalink (CPRD) to determine differences in the cost of medication wastage, dispensing fees and prescriber time of issuing either short (< 60 days) or long (≥ 60 days) prescription lengths in primary care for common, chronic conditions in the UK. BMJ Open 2017;7:e019382.
Data sharing statement
All available data are included as appendices to the report.
Disclaimers
This report presents independent research funded by the National Institute for Health Research (NIHR). The views and opinions expressed by authors in this publication are those of the authors and do not necessarily reflect those of the NHS, the NIHR, NETSCC, the HTA programme or the Department of Health. If there are verbatim quotations included in this publication the views and opinions expressed by the interviewees are those of the interviewees and do not necessarily reflect those of the authors, those of the NHS, the NIHR, NETSCC, the HTA programme or the Department of Health.
References
- NHS Digital . Prescription Cost Analysis, England – 2015. www.hscic.gov.uk/catalogue/PUB20200/pres-cost-anal-eng-2015-fact.pdf (accessed May 2016).
- Petty DR, Zermansky AG, Alldred DP. The scale of repeat prescribing – time for an update. BMC Health Serv Res 2014;14. http://dx.doi.org/10.1186/1472-6963-14-76.
- Guidance for the Implementation of Repeat Dispensing. London: NHS Confederation Publications Team; 2013.
- NHS Digital . Prescription Cost Analysis, England – 2015. Data Top 20 n.d. http://content.digital.nhs.uk/article/2021/Website-Search?productid=20437&q=Prescription+cost+analysis++England+2015&sort=Relevance&size=10&page=1&area=both#top (accessed May 2016).
- Prescriptions Dispensed in the Community: England 2005–2015. Leeds: NHS Digital; 2016.
- Prescribing Costs in Primary Care. London: National Audit Office; 2007.
- Trueman P, Lowson K, Blighe A, Meszaros A, Wright D, Glanville J, et al. Evaluation of the Scale, Causes and Costs of Waste Medicines: Final Report. York and London: York Health Economics Consortium and The School of Pharmacy, University of London; 2010.
- Medicines Wastage and 28 Day Prescribing Guidance. London: Pharmaceutical Services Negotiating Committee; 2007.
- Repeat Medication for 28 Days. Cambridge: Cambridgeshire Primary Care Trust; 2009.
- Prescribing Interval Policy. Essex: North East Essex PCT; 2010.
- Medicines Code Chapter 15: Policy for Repeat Prescribing and Medication Review. Dorset Clinical Commissioning Group; 2013.
- Hawksworth G, Wright D, Chrystyn H. A detailed analysis of the day to day unwanted medicinal products returned to community pharmacies for disposal. J Soc Adm Pharm 1996;13:215-22.
- PSNC Briefing 08613: Medicines Wastage and Prescription Duration. London: Pharmaceutical Services Negotiating Committee; 2013.
- All Wales Medicines Strategy Group . All Wales Review and Guidance for Prescribing Intervals 2013.
- Sun AP, Kirby B, Black C, Helms PJ, Bennie M, McLay JS. Unplanned medication discontinuation as a potential pharmacovigilance signal: a nested young person cohort study. BMC Pharmacol Toxicol 2014;15. http://dx.doi.org/10.1186/2050-6511-15-11.
- British National Formulary . Controlled Drugs and Drug Dependence n.d. www.evidence.nhs.uk/formulary/bnf/current/guidance-on-prescribing/controlled-drugs-and-drug-dependence (accessed May 2016).
- Davies JE, Taylor DG. Individualisation or standardisation: trends in National Health Service prescription durations in England 1998–2009. Prim Health Care Res Dev 2013;14:164-74. https://doi.org/10.1017/S146342361200045X.
- Duerden M, Millson D, Avery A, Smart S. The Quality of GP Prescribing. London: The King’s Fund; 2011.
- Prescribing in General Practice. London: BMA; 2013.
- Good Practice in Prescribing and Managing Medicines and Devices. London: General Medical Council; 2013.
- White KG. UK interventions to control medicines wastage: a critical review. Int J Pharm Pract 2010;18:131-40.
- Domino ME, Olinick J, Sleath B, Leinwand S, Byrns PJ, Carey T. Restricting patients’ medication supply to one month: saving or wasting money?. Am J Health Syst Pharm 2004;61:1375-9.
- Mitchell AL, Hickey B, Hickey JL, Pearce SH. Trends in thyroid hormone prescribing and consumption in the UK. BMC Public Health 2009;9. http://dx.doi.org/10.1186/1471-2458-9-132.
- Wong MC, Tam WW, Wang HH, Cheung CS, Tong EL, Cheung NT, et al. Duration of initial antihypertensive prescription and medication adherence: a cohort study among 203,259 newly diagnosed hypertensive patients. Int J Cardiol 2015;182:503-8. http://dx.doi.org/10.1016/j.ijcard.2014.12.058.
- Making Best Use of Medicines: Report of a Department of Health Roundtable Event Hosted by The King’s Fund. London: DH; 2011.
- Higgins J, Green S. Cochrane Handbook for Systematic Reviews of Interventions: Version 5.1.0 [updated March 2011] 2011. http://handbook.cochrane.org (accessed May 2016).
- Liberati A, Altman D, Tetzlaff J, Mulrow C, Gøtzsche P, Ioannidis J, et al. The PRISMA statement for reporting systematic reviews and meta-analyses of studies that evaluate health care interventions: explanation and elaboration. Ann Intern Med 2009;15:W65-94.
- King S, Miani C, Larkin J, Exley J, Martin A, Kirtley A. Clinical and Cost-Effectiveness of Issuing Longer Versus Shorter Duration (3 Month Vs. 28 Day) Prescriptions for Prescribed Medication in Patients With Stable, Chronic Conditions 2015. www.crd.york.ac.uk/PROSPERO/display_record.asp?ID=CRD42015027042 (accessed June 2016).
- The World Bank . World Bank Country and Lending Groups n.d. http://data.worldbank.org/about/country-and-lending-groups#Low_income (accessed June 2016).
- Sterne J, Hernán M, Reeves B, Savović J, Berkman N, Viswanathan M, et al. The Risk Of Bias In Non-Randomized Studies – of Interventions (ROBINS-I) Assessment Tool 2016. https://sites.google.com/site/riskofbiastool//welcome/home (accessed July 2016).
- Schünemann HJ, Oxman AD, Vist GE, Higgins JPT, Deeks JJ, Glasziou P, et al. Cochrane Handbook for Systematic Reviews of Interventions. The Cochrane Collaboration; 2011.
- Murphy P, Khandelwal N, Duncan I. Comparing medication wastage by fill quantity and fulfillment channel. Am J Pharm Benefits 2012;4:e166-71.
- Higgins J, Green S. 9.4.6 Combining Dichotomous and Continuous Outcomes 2011. http://handbook.cochrane.org/chapter_9/9_4_6_combining_dichotomous_and_continuous_outcomes.htm (accessed September 2016).
- Deeks JJ, Higgins JPT, Altman DG, Higgins JPT, Green S. Cochrane Handbook for Systematic Reviews of Interventions. The Cochrane Collaboration; 2008.
- Hermes M, Gleason PP, Starner CI. Adherence to chronic medication therapy associated with 90-day supplies compared with 30-day supplies. J Manag Care Pharm 2010;16:141-2.
- Jiang JZ, Khandelwal N, Lee KY. Comparing medication adherence and wastage among three different retail programs. Value Health 2007;10. https://doi.org/10.1016/S1098-3015(10)68621-4.
- Pfeiffer PN, Szymanski BR, Valenstein M, McCarthy JF, Zivin K. Trends in antidepressant prescribing for new episodes of depression and implications for health system quality measures. Med Care 2012;50:86-90. http://dx.doi.org/10.1097/MLR.0b013e3182294a3b.
- Ryvkin M, Garavaglia S. Wasted medication: How big is the problem?. Value Health 2009;12. https://doi.org/10.1016/S1098-3015(10)73471-9.
- Batal HA, Krantz MJ, Dale RA, Mehler PS, Steiner JF. Impact of prescription size on statin adherence and cholesterol levels. BMC Health Serv Res 2007;7. https://doi.org/10.1186/1472-6963-7-175.
- Steiner JF, Robbins LJ, Roth SC, Hammond WS. The effect of prescription size on acquisition of maintenance medications. J Gen Intern Med 1993;8:306-10. https://doi.org/10.1007/BF02600143.
- Thiebaud P, Patel BV. Does 90-day prescription supply at retail improve compliance in a managed care setting?. Value Health 2006;9. http://dx.doi.org/10.1016/s1098-3015(10)64725-0.
- Walton S. A model for comparing unnecessary costs associated with various prescription fill-quantity policies: illustration using VA data. J Managed Care Pharm 2001;7:384-90. https://doi.org/10.18553/jmcp.2001.7.5.386.
- Schectman JM, Bovbjerg VE, Voss JD. Predictors of medication-refill adherence in an indigent rural population. Med Care 2002;40:1294-300. http://dx.doi.org/10.1097/01.MLR.0000036412.26803.92.
- Domino ME, Martin BC, Wiley-Exley E, Richards S, Henson A, Carey TS, et al. Increasing time costs and copayments for prescription drugs: an analysis of policy changes in a complex environment. Health Serv Res 2011;46:900-19. http://dx.doi.org/10.1111/j.1475-6773.2010.01237.x.
- Taitel M, Fensterheim L, Kirkham H, Sekula R, Duncan I. Medication days’ supply, adherence, wastage, and cost among chronic patients in Medicaid. Medicare Medicaid Res Rev 2012;2:E1-13. http://dx.doi.org/10.5600/mmrr.002.03.a04.
- Parikh SP, Dishman BR, Smith TL. Ninety-day versus thirty-day drug-dispensing systems. Am J Health Syst Pharm 2001;58:1190-1.
- Schmittdiel JA, Nichols GA, Dyer W, Steiner JF, Karter AJ, Raebel MA. Health care system-level factors associated with performance on Medicare STAR adherence metrics in a large, integrated delivery system. Med Care 2015;53:332-7. http://dx.doi.org/10.1097/MLR.0000000000000328.
- Faris RJ, Filipek TM, Tang J, Wanta TM, Takagi MA, Steinberg SC. A retrospective comparative analysis of medication waste from day supply plan design in specialty pharmacy. J Manag Care Pharm 2010;16.
- Rabbani A, Alexander GC. Cost savings associated with filling a 3-month supply of prescription medicines. Appl Health Econ Health Policy 2009;7:255-64. http://dx.doi.org/10.2165/11313610-000000000-00000.
- Methods for the Development of NICE Public Health Guidance (third edition). Appendix E Algorithm for Classifying Quantitative (Experimental and Observational) Study Designs:. National Institute for Health and Care Excellence; 2012.
- Drummond MF, Sculpher MJ, Claxton K, Stoddart GL, Torrance GW. Methods for the Economic Evaluation of Health Care Programmes. Oxford: Oxford University Press; 2015.
- Wells G, Shea B, O’Connell D, Peterson J, Welch V, Losos M, et al. The Newcastle–Ottawa Scale (NOS) for Assessing the Quality of Nonrandomised Studies in Meta-Analyses 2014. www.ohri.ca/programs/clinical_epidemiology/oxford.asp (accessed May 2016).
- NHS Business Services Authority . Medical Exemption Certificates 2012. www.nhsbsa.nhs.uk/1126.aspx (accessed August 2016).
- Dragomir A, Côté R, White M, Lalonde L, Blais L, Bérard A, et al. Relationship between adherence level to statins, clinical issues and health-care costs in real-life clinical setting. Value Health 2010;13:87-94. http://dx.doi.org/10.1111/j.1524-4733.2009.00583.x.
- Perreault S, Ellia L, Dragomir A, Côté R, Blais L, Bérard A, et al. Effect of statin adherence on cerebrovascular disease in primary prevention. Am J Med 2009;122:647-55. http://dx.doi.org/10.1016/j.amjmed.2009.01.032.
- Simpson SH, Eurich DT, Majumdar SR, Padwal RS, Tsuyuki RT, Varney J, et al. A meta-analysis of the assocation between adherence to drug therapy and mortality. BMJ 2006;333. https://doi.org/10.1136/bmj.38875.675486.55.
- McGrady ME, Hommel KA. Medication adherence and health care utilization in pediatric chronic illness: a systematic review. Pediatrics 2013;132:730-40. http://dx.doi.org/10.1542/peds.2013-1451.
- Roebuck MC, Liberman JN, Gemmill-Toyama M, Brennan TA. Medication adherence leads to lower health care use and costs despite increased drug spending. Health Aff 2011;30:91-9. http://dx.doi.org/10.1377/hlthaff.2009.1087.
- Wong ES, Bryson CL, Hebert PL, Liu CF. Estimating the impact of oral diabetes medication adherence on medical costs in VA. Ann Pharmacother 2014;48:978-85. https://doi.org/10.1177/1060028014536981.
- NHS Business Services Authority on behalf of the Department of Health . Part IIIA: Professional Fees (Pharmacy Contractors), in NHS Electronic Drug Tariff 2016. www.drugtariff.nhsbsa.nhs.uk/#/00336026-DD_1/DD00335916/Part%20IIIA%20-%20Professional%20Fees%20(Pharmacy%20Contractors) (accessed August 2016).
- Wilson PM, Kataria N, McNeilly E. Patient and carer experience of obtaining regular prescribed medication for chronic disease in the English National Health Service: a qualitative study. BMC Health Serv Res 2013;13. http://dx.doi.org/10.1186/1472-6963-13-192.
- Addison’s Disease Self Help group . Letter to Professor Gilmore on Review of Prescription Charges for Those With Long-Term Conditions 2009. www.addisons.org.uk/forum/index.php?/files/file/124-2009-review-of-prescription-charges/ (accessed July 2016).
- Martin BC, Wiley-Exley EK, Richards S, Domino ME, Carey TS, Sleath BL. Contrasting measures of adherence with simple drug use, medication switching, and therapeutic duplication. Ann Pharmacother 2009;43:36-44. http://dx.doi.org/10.1345/aph.1K671.
- Christensen DB, Williams B, Goldberg HI, Martin DP, Engelberg R, LoGerfo JP. Assessing compliance to antihypertensive medications using computer-based pharmacy records. Med Care 1997;35:1164-70. https://doi.org/10.1097/00005650-199711000-00008.
- Lam WY, Fresco P. Medication adherence measures: an overview. Biomed Res Int 2015;2015. http://dx.doi.org/10.1155/2015/217047.
- Steiner JF, Prochazka AV. The assessment of refill compliance using pharmacy records: methods, validity, and applications. J Clin Epidemiol 1997;50:105-16. https://doi.org/10.1016/S0895-4356(96)00268-5.
- Payne R. Understanding can lead to a solution for non-adherence. Prescriber 2014;25:27-8. https://doi.org/10.1002/psb.1284.
- Hickey JL, Beckett W, Pearce S. Restriction of levothyroxine prescriptions – inconvenient for patients and economically ineffective. BMJ 2007;334.
- Schlomer BJ, Copp HL. Secondary data analysis of large data sets in urology: successes and errors to avoid. J Urol 2014;191:587-96. http://dx.doi.org/10.1016/j.juro.2013.09.091.
- Gauch RR. It’s Great! Oops, No It Isn’t: Why Clinical Research Can’t Guarantee the Right Medical Answers. Dordrecht: Springer Netherlands; 2009.
- Doble B, Payne R, Harshfield A, Wilson ECF. Retrospective, multicohort analysis of the Clinical Practice Research Datalink (CPRD) to determine differences in the cost of medication wastage, dispensing fees and prescriber time of issuing either short (< 60 days) or long (≥ 60 days) prescription lengths in primary care for common, chronic conditions in the UK. BMJ Open 2017;7. https://doi.org/10.1136/bmjopen-2017-019382.
- Herrett E, Gallagher AM, Bhaskaran K, Forbes H, Mathur R, van Staa T, et al. Data Resource Profile: Clinical Practice Research Datalink (CPRD). Int J Epidemiol 2015;44:827-36. http://dx.doi.org/10.1093/ije/dyv098.
- Mathur R, Bhaskaran K, Chaturvedi N, Leon DA, vanStaa T, Grundy E, et al. Completeness and usability of ethnicity data in UK-based primary care and hospital databases. J Public Health 2014;36:684-92. http://dx.doi.org/10.1093/pubmed/fdt116.
- Campbell J, Dedman D, Eaton S, Gallagher A, Williams T. Is the GPRD GOLD population comparable to the UK population?. Pharmacoepidemiol Drug Saf 2013;22.
- Medicines and Healthcare products Regulatory Agency (MHRA) . The Clinical Practice Research Datalink (CPRD) 2016. www.cprd.com/home/ (accessed August 2016).
- British National Formulary. London: BMJ group and Pharmaceutical Press; n.d.
- Brodie MJ, Chung S, Wade A, Quelen C, Guiraud-Diawara A, François C, et al. Clobazam and clonazepam use in epilepsy: results from a UK database incident user cohort study. Epilepsy Res 2016;123:68-74. http://dx.doi.org/10.1016/j.eplepsyres.2016.04.003.
- Francis NA, Hood K, Lyons R, Butler CC. Understanding flucloxacillin prescribing trends and treatment non-response in UK primary care: a Clinical Practice Research Datalink (CPRD) study. J Antimicrob Chemother 2016;71:2037-46. http://dx.doi.org/10.1093/jac/dkw084.
- Type 2 Diabetes in Adults: Management. London: National Institute for Health and Care Excellence; 2015.
- Cardiovascular Disease: Risk Assessment and Reduction, Including Lipid Modification. London: National Institute for Health and Care Excellence; 2015.
- Myocardial Infarction: Cardiac Rehabilitation and Prevention of Further MI. London: National Institute for Health and Care Excellence; 2013.
- Depression in Adults: Recognition and Management (CG90). London: National Institute for Health and Care Excellence; 2016.
- WHO Collaborating Centre for Drug Statistics Methodology . ATC/DDD/Index/2016 2015. www.whocc.no/atc_ddd_index/ (accessed August 2016).
- NHS Business Services Authority . Prescription Cost Analysis (PCA) Data 2015. www.nhsbsa.nhs.uk/PrescriptionServices/3494.aspx (accessed August 2016).
- Hollingworth W, Devine EB, Hansen RN, Lawless NM, Comstock BA, Wilson-Norton JL, et al. The impact of e-prescribing on prescriber and staff time in ambulatory care clinics: a time motion study. J Am Med Inform Assoc 2007;14:722-30. https://doi.org/10.1197/jamia.M2377.
- Curtis L, Burns A. Unit Costs of Health and Social Care 2015. Canterbury: PSSRU, University of Kent; 2015.
- Coupland C, Dhiman P, Morriss R, Arthur A, Barton G, Hippisley-Cox J. Antidepressant use and risk of adverse outcomes in older people: population based cohort study. BMJ 2011;343. http://dx.doi.org/10.1136/bmj.d4551.
- NHS Choices . NHS in England – Help With Health Costs 2016. www.nhs.uk/NHSEngland/Healthcosts/Pages/Prescriptioncosts.aspx (accessed August 2016).
- Guide to the Methods of Technology Appraisals 2013. London: National Institute for Health and Care Excellence; 2013.
- Boyd M, Waring J, Barber N, Mehta R, Chuter A, Avery AJ, et al. Protocol for the New Medicine Service Study: a randomized controlled trial and economic evaluation with qualitative appraisal comparing the effectiveness and cost effectiveness of the New Medicine Service in community pharmacies in England. Trials 2013;14. http://dx.doi.org/10.1186/1745-6215-14-411.
- Hypertension in Adults: Diagnosis and Management. London: National Guideline Centre; 2011.
- Clarke P, Gray A, Holman R. Estimating utility values for health states of type 2 diabetic patients using the EQ-5D (UKPDS 62). Med Decis Making 2002;22:340-9. https://doi.org/10.1177/027298902400448902.
- Sapin C, Fantino B, Nowicki ML, Kind P. Usefulness of EQ-5D in assessing health status in primary care patients with major depressive disorder. Health Qual Life Outcomes 2004;2. http://dx.doi.org/10.1186/1477-7525-2-20.
- Clarke PM, Gray AM, Briggs A, Farmer AJ, Fenn P, Stevens RJ, et al. A model to estimate the lifetime health outcomes of patients with type 2 diabetes: the United Kingdom Prospective Diabetes Study (UKPDS) Outcomes Model (UKPDS no. 68). Diabetologia 2004;47:1747-59. http://dx.doi.org/10.1007/s00125-004-1527-z.
- National Institute for Health and Clinical Excellence: Guidance. Leicester: British Psychological Society and Royal College of Psychiatrists; 2010.
- Cipriani A, Furukawa T, Salanti G, Geddes J, Higgins J, Churchill R, et al. Comparative efficacy and acceptability of 12 new-generation antidepressants: a multiple-treatments meta-analysis. Lancet 2009;373:746-58. http://dx.doi.org/10.1016/s0140-6736(09)60046-5.
- Clarke P, Gray A, Legood R, Briggs A, Holman R. The impact of diabetes-related complications on healthcare costs: results from the United Kingdom Prospective Diabetes Study (UKPDS Study No. 65). Diabet Med 2003;20:442-50. http://dx.doi.org/10.1046/j.1464-5491.2003.00972.x.
- Philips Z, Bojke L, Sculpher M, Claxton K, Golder S. Good practice guidelines for decision-analytic modelling in health technology assessment: a review and consolidation of quality assessment. Pharmacoeconomics 2006;24:355-71. https://doi.org/10.2165/00019053-200624040-00006.
- O’Hagan A, Buck CE, Daneshkhah A, Eiser JR, Garthwaite PH, Jenkinson DJ, et al. Uncertain Judgements: Eliciting Experts’ Probabilities. Chichester: John Wiley & Sons Ltd; 2006.
- Westbrook JI, Li L, Georgiou A, Paoloni R, Cullen J. Impact of an electronic medication management system on hospital doctors’ and nurses’ work: a controlled pre-post, time and motion study. J Am Med Inform Assoc 2013;20:1150-8. http://dx.doi.org/10.1136/amiajnl-2012-001414.
- Franklin BD, O’Grady K, Donyai P, Jacklin A, Barber N. The impact of a closed-loop electronic prescribing and administration system on prescribing errors, administration errors and staff time: a before-and-after study. Qual Saf Health Care 2007;16:279-84. https://doi.org/10.1136/qshc.2006.019497.
- Laurant M, Reeves D, Hermens R, Braspenning J, Grol R, Sibbald B. Substitution of doctors by nurses in primary care. Cochrane Database Syst Rev 2005;2. http://dx.doi.org/10.1002/14651858.CD001271.pub2.
- Shum C, Humphreys A, Wheeler D, Cochrane MA, Skoda S, Clement S. Nurse management of patients with minor illnesses in general practice: multicentre, randomised controlled trial. BMJ 2000;320:1038-43. https://doi.org/10.1136/bmj.320.7241.1038.
- Venning P, Durie A, Roland M, Roberts C, Leese B. Randomised controlled trial comparing cost effectiveness of general practitioners and nurse practitioners in primary care. BMJ 2000;320:1048-53. https://doi.org/10.1136/bmj.320.7241.1048.
- Murphy GE, Simons AD, Wetzel RD, Lustman PJ. Cognitive therapy and pharmacotherapy. Singly and together in the treatment of depression. Arch Gen Psychiatry 1984;41:33-41. https://doi.org/10.1001/archpsyc.1984.01790120037006.
- British National Formulary. London: BMJ Group and Pharmaceutical Press; 2008.
- Curtis L. Unit Costs of Health and Social Care 2008. Canterbury: PSSRU, University of Kent; 2009.
- The Secretary of State for Health, the NHS Commissioning Board and the Welsh Ministers . Amendments to the Drug Tariff 2016. www.nhsbsa.nhs.uk/PrescriptionServices/Documents/PPD%20Drug%20Tariff/July_2016.pdf (accessed August 2016).
Appendix 1 Search strategy
This appendix provides the full list of search terms used in each database searched. Search terms are presented first for the peer-reviewed literature and then for the grey literature searches.
Peer-reviewed literature searches
MEDLINE (PubMed)
Prescription length*[title/abstract] OR prescription duration*[title/abstract] OR medication duration*[title/abstract] OR “medication length”[title/abstract] OR “length of prescription”[title/abstract] OR “length of prescriptions”[title/abstract] OR “duration of prescription”[title/abstract] OR “duration of prescriptions”[title/abstract] OR “durations of prescriptions”[title/abstract] OR “drug prescribing”[title/abstract] OR “multiple drug prescriptions”[title/abstract] OR prescribing pattern*[title/abstract] OR prescription pattern*[title/abstract] OR prescribing behavior*[title/abstract] OR prescribing behaviour*[title/abstract] OR prescribing practice*[title/abstract] OR prescribing standard*[title/abstract] OR (installment[title/abstract] AND dispensing[title/abstract]) OR repeat prescri*[title/abstract] OR “repeat dispensing”[title/abstract] OR prescribing interval*[title/abstract] OR prescription interval*[title/abstract] OR “28 day supply”[title/abstract] OR “34 day supply”[title/abstract] OR (“28 day"[title/abstract] AND (“drug supply”[title/abstract] OR prescribing[title/abstract] OR prescription[title/abstract])) OR “56 day supply”[title/abstract] OR (“56 day"[title/abstract] AND (“drug supply"[title/abstract] OR prescribing[title/abstract] OR prescription[title/abstract])) OR “28 day drug limit”[title/abstract] OR “56 day drug limit”[title/abstract] OR “one month prescription”[title/abstract] OR “one month prescriptions”[title/abstract] OR “1 month prescription”[title/abstract] OR “1 month supply”[title/abstract] OR “one month supply”[title/abstract] OR “3 month prescriptions”[title/abstract] OR “three month prescription”[title/abstract] OR “three month prescriptions”[title/abstract] OR “3 month prescription”[title/abstract] OR “3 month supply”[title/abstract] OR “three month supply”[title/abstract] OR “90 day supply”[title/abstract] OR “30 day supply”[title/abstract] OR “60 day supply”[title/abstract] OR dosage unit*[title/abstract] OR “prescription standardization”[title/abstract] OR “prescription standardisation”[title/abstract] OR prescription restriction*[title/abstract] OR prescribing restriction*[title/abstract] OR “restricting prescriptions”[title/abstract] OR “restricting medication”[title/abstract] OR medication restriction*[title/abstract] OR dispensing restriction*[title/abstract] OR prescribing trend*[title/abstract] OR prescription trend*[title/abstract] OR dispensing trend*[title/abstract] OR “trends in dispensing”[title/abstract] OR “trends in prescribing"[title/abstract] OR prescription suppl*[title/abstract] OR medication suppl*[title/abstract] OR term prescription*[title/abstract] OR ((short course*[title/abstract] OR long course*[title/abstract]) AND (prescription*[title/abstract] OR medication*[title/abstract])) OR “short prescription”[title/abstract] OR “long prescription”[title/abstract] OR “short prescriptions”[title/abstract] OR “long prescriptions”[title/abstract] OR standardized prescri*[title/abstract] OR “standardised prescription”[title/abstract] OR “standardised prescribing”[title/abstract] OR (Standardization[title/abstract] AND (prescribing[title/abstract] OR prescription*[title/abstract])) OR (Standardisation[title/abstract] AND (prescribing[title/abstract] OR prescription*[title/abstract])) OR individualized prescri*[title/abstract] OR individualised prescri*[title/abstract] OR (individualization[title/abstract] AND prescrib*[title/abstract]) OR (individualisation[title/abstract] AND prescrib*[title/abstract]) OR Drug Prescriptions/trends OR Drug Prescriptions/supply and distribution
Results: 8242 – animal = 8207.
EMBASE
(prescription* NEAR/2 length*):ti,ab OR (prescription* NEAR/2 duration*):ti,ab OR (medication* NEAR/2 duration*):ti,ab OR (medication* NEAR/2 length*):ti,ab OR “drug prescribing”:ti,ab OR “multiple drug prescriptions”:ti,ab OR (prescri* NEXT/1 pattern*):ti,ab OR (prescribing NEXT/1 behaviour*):ab,ti (prescribing NEXT/1 behavior*):ab,ti OR (prescribing NEXT/1 practice*):ab,ti OR (prescribing NEXT/1 standard*):ab,ti OR “installment dispensing”:ti,ab OR (repeat NEXT/1 prescri*):ti,ab OR (repeat NEXT/1 dispens*):ti,ab OR (prescri* NEXT/1 interval*):ti,ab OR (prescri* NEXT/1 interval*):ti,ab OR ((28 OR 30 OR 34 OR 56 OR 60 OR 90) NEXT/1 day NEXT/1 supply):ti,ab OR ((28 OR 30 OR 34 OR 56 OR 60 OR 90) NEXT/1 day NEXT/1 drug NEXT/1 supply):ti,ab OR ((28 OR 30 OR 34 OR 56 OR 60 OR 90) NEXT/1 day NEXT/1 prescri*):ti,ab OR ((28 OR 30 OR 56 OR 60 OR 90) NEXT/1 day NEXT/1 drug NEXT/1 limit):ti,ab OR “one month prescription”:ti,ab OR “1 month prescription”:ti,ab OR “one month supply”:ti,ab OR “1 month supply”:ti,ab OR (3 NEXT/1 month NEXT/1 prescription*):ti,ab OR (three NEXT/1 month NEXT/1 prescription*):ti,ab OR “3 month supply”:ti,ab OR “three month supply”:ti,ab OR (dosage NEXT/1 unit*) OR “prescription standardization”:ti,ab OR “prescription standarisation”:ti,ab OR (prescri* NEAR/1 restrict*):ti,ab OR (medication* NEAR/1 restrict*):ti,ab OR (dispensing NEXT/1 restrict*):ti,ab OR (prescri* NEAR/2 trends):ti,ab OR (dispensing NEAR/2 trends):ti,ab OR ((prescription OR medication) NEXT/1 suppl*):ti,ab OR ((short OR long) NEXT/1 (course OR term) NEXT/1 (prescription* OR medication*)):ti,ab OR ((short OR long) NEXT/1 prescription*):ti,ab OR ((standardised OR standardized OR standardization OR standardisation) NEXT/2 prescri*):ti,ab OR ((individualized OR individualized OR individualization OR individualisation) NEXT/1 prescri*):ti,ab
Results: 6266 – duplicates/animal = 3600.
Cumulative Index to Nursing and Allied Health Literature
TI “prescription length*” OR AB “prescription length*” OR TI “prescription duration*” OR AB “prescription duration*” OR TI “length* of prescription*” OR AB “length* of prescription*” OR TI “duration* of prescription*” OR AB “duration* of prescription*” OR TI “drug prescribing” OR AB “drug prescribing” OR TI “multiple drug prescriptions” OR AB “multiple drug prescriptions” OR TI “prescri* pattern*” OR AB “prescri* pattern*” OR TI “prescribing behavior*” OR AB “prescribing behavior*” OR TI “prescribing behaviour*” OR AB “prescribing behaviour*” OR TI “prescribing practice*” OR AB “prescribing practice*” OR TI “prescribing standard*” OR AB “prescribing standard*” OR TI “installment dispensing” OR AB “installment dispensing” OR TI “repeat prescri*” OR AB “repeat prescri*” OR TI “28 day supply” OR AB “28 day supply” OR TI “30 day supply” OR AB “30 day supply” OR TI “30 day drug supply” OR AB “30 day drug supply” OR TI “28 day drug supply” OR AB “28 day drug supply” OR TI “34 day drug supply” OR AB “34 day drug supply” OR TI “34 day supply” OR AB “34 day supply OR TI “28 day prescri*” OR AB “28 day prescri*” OR TI “30 day prescri*” OR AB “30 day prescri*” OR TI “34 day prescri*” OR AB “34 day prescri*” OR TI “28 day drug limit*” OR AB “28 day drug limit*” OR TI “30 day drug limit*” OR AB “30 day drug limit*” OR TI “34 day drug limit*” OR AB “34 day drug limit*” OR TI “56 day supply” OR AB “56 day supply” OR TI “56 day drug supply” OR AB “56 day drug supply” OR TI “56 day prescri*” OR AB “56 day prescri*” OR TI “56 day drug limit*” OR AB “56 day drug limit*” OR TI “60 day supply” OR AB “60 day supply” OR TI “60 day drug supply” OR AB “60 day drug supply” OR TI “60 day prescri*” OR AB “60 day prescri*” OR TI “60 day drug limit*” OR AB “60 day drug limit*” OR TI “90 day supply” OR AB “90 day supply” OR TI “90 day drug supply” OR AB “90 day drug supply” OR TI “90 day prescri*” OR AB “90 day prescri*” OR TI “90 day drug limit*” OR AB “90 day drug limit*” TI “one month prescription*” OR AB “one month prescription*” OR TI “1 month prescription*” OR AB “1 month prescription*” OR TI “1 month supply” OR AB “1 month supply” OR TI “one month supply” OR AB “one month supply” OR TI “three month prescription*” OR AB “three month prescription*” OR TI “3 month prescription*” OR AB “3 month prescription*” OR TI “3 month supply” OR AB “3 month supply” OR TI “three month supply” OR AB “three month supply” OR TI “dosage unit*” AND AB “dosage unit*” OR TI “prescription standardization*” OR AB “prescription standardization*” OR TI “prescription standardisation*” OR AB “prescription standardisation*” OR TI “prescri* restriction*” OR AB “prescri* restriction*” OR TI “restricting prescription*” OR AB “restricting prescription*” OR TI “restricting medication*” OR AB “restricting medication*” OR TI “medication restriction*” OR AB “medication restriction*” OR TI “dispensing restriction*” OR AB “dispensing restriction*” OR TI “prescri* trend*” OR AB “prescri* trend*” OR TI “dispensing trend*” OR AB “dispensing trend*” OR TI “trends in dispensing” OR AB “trends in dispensing” OR TI “trends in prescribing” OR AB “trends in prescribing” OR TI “prescription suppl*” OR AB “prescription suppl*” OR TI “medication suppl*” OR AB “medication suppl*” OR TI “term prescription*” OR AB “term prescription*” OR TI “short course prescription*” OR AB “short course prescription*” OR TI “long course prescription*” OR AB “long course prescription*” OR TI “short course medication*” OR AB “short course medication*” OR TI “long course medication*” OR AB “long course medication*” OR TI “short prescription*” OR AB “short prescription*” OR TI “long prescription*” OR AB “long prescription*” OR TI “standardized perscri*” OR AB “standardized perscri*” OR TI “standardised perscri*” OR AB “standardised perscri*” OR TI “standarization of prescri*” OR AB “standarization of prescri*” OR TI “standarisation of prescri*” OR AB “standarisation of prescri*” OR TI “individualized prescri*” OR AB “individualized prescri*” OR TI “individualised prescri*” OR AB “individualised prescri*” OR TI “individualization prescri*” OR AB “individualization prescri*” OR TI “individualisation prescri*” OR AB “individualisation prescri*”
Results: 1737 – duplicates = 367.
Web of Science
Refined by: [excluding] DOCUMENT TYPES: ( LETTER OR NEWS ITEM OR EDITORIAL MATERIAL OR BOOK CHAPTER OR NOTE OR BOOK REVIEW OR DISCUSSION ) AND [excluding] WEB OF SCIENCE CATEGORIES: ( OPERATIONS RESEARCH MANAGEMENT SCIENCE OR VETERINARY SCIENCES OR COMPUTER SCIENCE ARTIFICIAL INTELLIGENCE OR MATHEMATICAL COMPUTATIONAL BIOLOGY OR COMPUTER SCIENCE THEORY METHODS OR METEOROLOGY ATMOSPHERIC SCIENCES OR MECHANICS OR FORESTRY OR TELECOMMUNICATIONS OR MATHEMATICS OR MATERIALS SCIENCE MULTIDISCIPLINARY OR ECOLOGY OR FOOD SCIENCE TECHNOLOGY OR AUTOMATION CONTROL SYSTEMS OR ASTRONOMY ASTROPHYSICS ) AND [excluding] WEB OF SCIENCE CATEGORIES: ( STATISTICS PROBABILITY OR POLYMER SCIENCE OR MATHEMATICS INTERDISCIPLINARY APPLICATIONS OR AGRICULTURE DAIRY ANIMAL SCIENCE OR PLANT SCIENCES OR PHYSICS PARTICLES FIELDS OR BIOCHEMISTRY MOLECULAR BIOLOGY OR OCEANOGRAPHY OR ENGINEERING MULTIDISCIPLINARY ) AND [excluding] RESEARCH AREAS: ( WATER RESOURCES OR MATERIALS SCIENCE OR MINING MINERAL PROCESSING OR METALLURGY METALLURGICAL ENGINEERING OR MATHEMATICS ) AND [excluding] WEB OF SCIENCE CATEGORIES: ( PHYSICS FLUIDS PLASMAS OR ENERGY FUELS OR AGRICULTURE MULTIDISCIPLINARY ) Indexes=SCI-EXPANDED, CPCI-S Timespan=All years
TS=(prescription* NEAR/2 length*) OR TS=(prescription* NEAR/2 duration*) OR TS=(“duration of medication*”) OR TS=(“length* of medication*”) OR TS=(“drug prescribing”) OR TS=(“multiple drug prescriptions”) OR TS=(“prescription pattern*”) OR TS=(“prescribing pattern*”) OR TS=(“prescri* pattern*”) OR TS=(“prescribing behavior*”) OR TS=(“prescribing behaviour*”) OR TS=(“prescription behavior*”) OR TS=(“prescription behaviour*”) OR TS=(“prescri* practice*”) OR TS=(“prescription standard*”) OR TS=(“prescribing standard*”) OR TS=(“installment dispensing”) OR TS=(“repeat dispens*”) OR TS=(“repeat* prescription*”) OR TS=(“repeat* prescribing*”) OR TS=(“prescribing interval*”) OR TS=(“prescription interval*”) OR TS=(“28 day supply”) OR TS=(“30 day supply”) OR TS=(“34 day supply”) OR TS=(“56 day supply”) OR TS=(“60 day supply”) OR TS=(“90 day supply”) OR TS=(“28 day drug supply”) OR TS=(“30 day drug supply”) OR TS=(“34 day drug supply”) OR TS=(“56 day drug supply”) OR TS=(“60 day drug supply”) OR TS=(“90 day drug supply”) OR TS=(“28 day prescri*”) OR TS=(“30 day prescri*”) OR TS=(“34 day prescri*”) OR TS=(“56 day prescri*”) OR TS=(“60 day prescri*”) OR TS=(“90 day prescri*”) OR TS=(“28 day drug limit*”) OR TS=(“30 day drug limit*”) OR TS=(“34 day drug limit*”) OR TS=(“56 day drug limit*”) OR TS=(“60 day drug limit*”) OR TS=(“90 day drug limit*”) OR TS=(“one month prescription”) OR TS=(“1 month prescription”) OR TS=(“one month supply”) OR TS=(“1 month supply”) OR TS=(“three month prescription*”) OR TS=(“3 month prescription*”) OR TS=(“three month supply”) OR TS=(“3 month supply”) OR TS=(“dosage unit*”) OR TS=(“prescription standardization”) OR TS=(“prescription standardisation”) OR TS=(prescri* NEAR/1 restrict*) OR TS=(medication* NEAR/1 restrict*) OR TS=(“dispensing restrict*”) OR TS=(dispensing NEAR/2 trends) OR TS=(prescri* NEAR/2 trends) OR TS=(“prescription suppl*”) OR TS=(“medication suppl*”) OR TS=(“short term prescription*”) OR TS=(“short term medication*”) OR TS=(“short course prescription*”) OR TS=(“short course medication*”) OR TS=(“long term prescription*”) OR TS=(“long term medication*”) OR TS=(“long course prescription*”) OR TS=(“long course medication*”) OR TS=(“short prescription*”) OR TS=(“long prescription*”) OR TS=((standardized OR standardised OR standardization OR standardisation) NEAR/2 prescri*) OR TS=((individualized OR indvidualised OR individualization OR individualization) NEAR/1 (prescription* OR prescribing))
8592 – duplicates/animal = 3002.
Cochrane
“length of prescription”:ti,ab OR “prescription length”:ti,ab OR “prescription duration”:ti,ab OR “drug prescribing”:ti,ab OR “multiple drug prescription*”:ti,ab OR “prescri* pattern”:ti,ab OR “prescribing behavior”:ti,ab OR “prescri practice”:ti,ab OR “prescri* standard*”:ti,ab OR “repeat dispens*”:ti,ab OR “repeat prescri*”:ti,ab OR “prescri* interval*”:ti,ab OR “28 day supply":ti,ab OR “30 day supply":ti,ab or “34 day supply":ti,ab OR “60 day supply":ti,ab or “90 day supply":ti,ab OR “28 day prescri*”:ti,ab OR “30 day prescri*”:ti,ab or “34 day prescri*":ti,ab OR “60 day prescri*”:ti,ab or “90 day prescri*”:ti,ab or “56 day prescri*”:ti,ab OR “one month prescription”:ti,ab OR “1 month prescription”:ti,ab OR “one month supply”:ti,ab OR “1 month supply”:ti,ab OR “three month prescription”:ti,ab OR “3 month prescription”:ti,ab OR “3 month supply”:ti,ab OR “three month supply”:ti,ab OR “dosage unit*”:ti,ab OR “prescription standardization”:ti,ab OR “prescri* restriction*”:ti,ab OR “medication restrict*”:ti,ab OR “dispensing restriction*”:ti,ab OR “dispensing NEAR/2 trend*”:ti,ab OR “prescription NEAR/2 trend*” OR “medication supply”:ti,ab OR “medication supplies”:ti,ab OR “short term prescription*”:ti,ab OR “long term prescription*”:ti,ab OR “prescription suppl*”:ti,ab OR ((standardized OR standardised OR standardization OR standardisation) NEAR/2 prescri*) OR ((individualized OR indvidualised OR individualization OR individualization) NEAR/1 (prescription* OR prescribing))
After duplicates: 69.
National Institute for Health and Care Excellence
“length of prescription” OR “prescription length” OR “medication length” OR “prescription trends” OR “medication trends” OR “multiple prescriptions” OR “30 day supply” OR “60 day supply” OR “90 day supply” OR “one month supply” OR “three month supply” OR “prescription supply” OR “medication supply” OR “short term prescription” OR “long term prescription” OR “standardised prescription” OR “individualised prescription” OR “prescribing behaviour”
**added 5 records.
Total
15,250 (No year limits).
(Limiting to 1995 ≈ 13,470/Limiting to 2000 ≈ 12,150/Limiting to 2005 ≈ 9950).
Grey literature searches
The New York Academy of Medicine
prescribing patterns; prescription length; one month supply; 28 day supply; length of prescription; multiple drug prescription; dispensing restriction; prescribing trends; prescribing behavior; individualized prescribing; individualized prescription; month supply;
Open Archives Initiative harvester
Ti: Prescribing patterns; ti: length of prescription; ti: prescription standardization; ti: dispensing regulation; ti: 30 day supply; ti: repeat dispensing; ti: medication prescription; ti: individualized prescri*; ti: multiple drug prescri*; ti: short term prescri*; ti: long term prescri*; ti: prescription trends; ti: prescribing trends;
OpenGrey
“prescribing patterns”; “prescription length”; “prescription standardization”; “dispensing regulations”; “prescription trends”; “prescription patterns”; “individualized prescri*”; “prescribing trends”; “28 day supply”; “multiple prescriptions”;
Total
14.
Appendix 2 Detail of stage 1 of the screening
Titles and abstracts of studies identified in the searches were entered into an EndNote database. The senior systematic reviewer (SK) sifted through the database to identify clusters of articles that were obviously not relevant. She suggested a list of terms that would allow for identification of such articles. JE and CMiani reviewed and validated this list. References that were obviously not relevant were tagged by the information specialist (JL). JL searched for and tagged citations with at least one of the following terms in the title:
Conditions, populations and settings
Helicobacter pylori, allergic, haemorrhage, contact lens, chemotherapy, dentist, dental, malaria, hormone therapy, acne, obesity, antifungal, antibiotic, vitamin D, HIV, human immunodeficiency virus, tuberculosis, leprosy, eye, contraceptive, hypnotics, fracture, menopause, palliative, catheter, DNA, suicide, counselling, education, biomarker, nutrition, animals, pregnancy, preterm, foetal, fetal, neonatal, surgery, postoperative, acute (TITLE with ‘acute’ AND ‘chronic’ were not excluded), outpatient, hospital, inpatient, vaccine, vaccination, meningitis, MRSA, anesthesia, anaesthesia, stroke, infection, birth, transplant, MRI, emergency department, prison, influenza, critical care.
Low-income economies as listed by the World Bank29
Afghanistan (when not referring to US/British soldiers), Benin, Burkina Faso, Burundi, Cambodia, Central African Republic, Chad, Comoros, Congo, Ethiopia, Gambia, Guinea, Guinea-Bissau, Haiti, North Korea OR Democratic People’s Republic of North Korea, Liberia, Malawi, Mali, Mozambique, Nepal, Niger, Rwanda, Sierra Leone, Somalia, South Sudan, Tanzania, Togo, Uganda, Zimbabwe.
Two reviewers (JE and CMiani) then screened the articles tagged in the outpatient, hospital and inpatient categories to make sure that no studies considering primary care AND secondary/tertiary care were excluded. Upon the recommendation of the wider team, they also screened the articles tagged in the vitamin D and hormone therapy categories to make sure that no relevant studies were excluded.
Appendix 3 Studies excluded at full-text review stage
Reference | Reason for exclusion |
---|---|
Adherence improves with 90-day medication supply. Drug Benefit Trends, 7 April 2010, p. 66 | Article type: news piece |
Beattie, J. People with CVD identified 5 areas of medication-related problems that influenced adherence and informed decision making. Evid Based Nurs 2007;10:127 | Article type: commentary |
Bobat N, Cubbin I, Lowe DA, Vickers S. A review of the prescribed medicines wastage audit for the NHS community pharmacies of Western Cheshire. Int J Pharm Pract 2011;19:57–8 | Quality: not enough information in the abstract |
Bond C, Matheson C, Williams S, Williams P, Donnan P. Repeat prescribing: a role for community pharmacists in controlling and monitoring repeat prescriptions. Br J Gen Pract 2000;50:271–5 | Relevance: the article does not compare different prescription lengths |
Bradley F, Elvey R, Ashcroft DM, Noyce P. Influence of prescription charges on repeat prescribing in primary care. J Clin Pharm Ther 2007;32:269–75 | Relevance: the article does not compare different prescription lengths |
Cubitt T, De Quincey M. Repeat prescribing management – a cause for concern? Br J Gen Pract 1999;49:580 | Article type: letter |
Cuiper N. Faster and safer delivery of repeat prescriptions. Pharmaceutisch Weekblad 2006;141:68–9 | Not available |
Darlath W. Asthma: long term medication. Med Monatsschr fur Pharm 1989;12:74–9 | Not available |
De Leeuw M. Doctor little focused on discontinuation of long-term medication: it is worthwhile to stop more often. Pharmaceutisch Weekblad 2009;144:16–21 | Not available |
De Smet PA, Dautzenberg M. Repeat prescribing: scale, problems and quality management in ambulatory care patients. Drugs 2004;64:1779–800 | Relevance: the article does not compare different prescription lengths |
Del Mar C. Improving prescribing practices in primary care. A randomised trial and economic analysis of a multicomponent intervention showed small, but important, gains. PLOS Med 2006;3:e229 | Article type: commentary |
Domino ME, Olinick J, Sleath B, Leinwand S, Byrns PJ, Carey T. Restricting patients’ medication supply to one month: saving or wasting money? Am J Health Syst Pharm 2004;61:1375–9 | Study type: simulation. Moreover, this article has been updated by Domino et al.,44 included in this review |
Drury VW. Repeat prescribing – a review. J R Coll Gen Pract 1982;32:42–5 | Relevance: the article does not compare different prescription lengths |
Gaziano T, Cho S, Sy S, Pandya A, Levitt NS, Steyn K. Increasing prescription length could cut cardiovascular disease burden and produce savings in South Africa. Health Aff (Millwood) 2015;34:1578–85 | Relevance: setting too different from the UK context (underserved areas in poor neighbourhoods in South Africa) |
Hawksworth G, Wright D, Chrystyn H. A detailed analysis of the day to day unwanted medicinal products returned to community pharmacies for disposal. J Soc Adm Pharm 1996;13:215–22 | Relevance: the article does not compare two prescriptions length for the same drug and it is not possible to identify which conditions the article covers |
Helling DK, Yesalis CE, Norwood GI, Burmeister LF, Lipson DP, Fisher WP, et al. Effects of capitation payment for pharmacy services on pharmacist-dispensing and physician-prescribing behavior: I. Prescription quantity and dose analysis. Drug Intell Clin Pharm 1981;15:581–9 | Not available |
Ivers N, Schwalm JR, Kingsbury K, Guo H, Tu J, Grimshaw J, et al. Long-term statin adherence in secondary prevention: a provincial retrospective cohort study. Can J Cardiol 2012;28:S225 | Relevance: population is not a stable, chronically ill population |
Kawazoe H, Iihara N, Doi C, Morita S. Impact of prescription-term deregulation with revised medical service fees on drug therapy management. Yakugaku Zasshi 2005;125:959–69 | Relevance: the article does not compare different prescription lengths |
Kazerooni R, Nguyen JB, Bounthavong M, Tran MH, Madkour N. New start versus continuing users on aripiprazole: implications for policy. Am J Manag Care 2015;21:e43–50 | Relevance: the article does not compare different prescription lengths |
Keenan W. Reforming drug prescribing policies could save NHS millions. Nurs Stand 2014;28:32 | Relevance: the article does not compare different prescription lengths |
Lawrie E, Arkley J, Keane D, McNaughton S, Philip C, Kinnear M. Repeat prescribing systems and waste. Pharm World Sci 2009;31:89–90 | Not available |
Leslie RS, Laskowski TR, Adams RS, Patel BV. Implementation and evaluation of a 90-day retail benefit addition and Rx coupon program. J Manage Care Pharm 2009;15:588 | Relevance: the article does not compare different prescription lengths |
Mitchell AL, Hickey B, Hickey JL, Pearce SHS. Trends in thyroid hormone prescribing and consumption in the UK. BMC Public Health 2009;9:132 | Relevance: the article does not compare different prescription lengths |
Mitchell AL, Hickey B, Hickey JL, Pearce SHS. UK trends in prescribing thyroid hormone and patient satisfaction survey. Endocrine Abstracts 2009;19:P345 | Duplicate |
Rabbani A, Alexander GC. Cost savings associated with filling a three-month supply of prescription medicines. Value Health 2009;12:A83 | Duplicate |
Ray WA, Schaffner W, Oates JA. Therapeutic choice in the treatment of hypertension – initial treatment of newly diagnosed hypertension and secular trends in the prescribing of antihypertensive medications for Medicaid patients. Am J Med 1986;81:9–16 | Relevance: the article does not compare different prescription lengths |
Reenan J. Rx for physician prescribing practices. Virtual Mentor 2006;8:359–61 | Article type: editorial |
Reinhardt UE. Proper benchmark for drug prescribing needs to be found. BMJ 2002;324:1039 | Article type: letter |
Rey ME, Villalbi JR. Potential impact of primary health-care reform on prescription patterns in Spain – the Ciutat-Badia experience. Medicina Clinica 1987;89:141–3 | Not available |
Richards RN. Prescription restrictions. Can Med Assoc J 1972;106:854 | Article type: letter |
Rigler S, Webb M, Redford L, Brown E. Antidepressant prescribing trends in long-term care. J Am Geriatr Soc 1999;47:S38 | Not available |
Sun SX, Lee KY, McMurray J. Impact of a 90-day retail refill program on prescription drug utilisation and expenditures. Drug Benefit Trends 2007;19:314–19 | Not available |
Taitel M, Lou Y, Huang Z, Suwalski M. A comparison of patients with and without 90-day fills at a retail pharmacy: observing medication changes from single source brand to generic. Value Health 2013;16:A250 | Not relevant |
Tulloch AJ. Repeat prescribing for elderly patients. Br Med J (Clin Res Ed) 1981;282:1672–5 | Relevance: the article does not compare different prescription lengths |
Van Der Schoor P. Repeat prescription can be more efficient – the time has come: a round table discussion. Pharmaceutisch Weekblad 2005;140:832–6 | Not available |
White KG. UK interventions to control medicines wastage: a critical review. Int J Pharm Pract 2010;18:131–40 | Article type: review (does not follow systematic review methodology) |
Wilson PM, Kataria N, McNeilly A. Patient and carer experience of obtaining regular prescribed medication for chronic disease in the English National Health Service: a qualitative study. BMC Health Serv Res 2013;13:192 | Relevance: the article does not compare different prescription lengths |
Wong MC, Tam WW, Wang HH, Chan WM, Kwan MW, Cheung CS, et al. The association between initial antihypertensive prescription interval and medication adherence: a cohort study among 203,259 patients. Hypertension 2014;64:A435 | Relevance: the article compares 30-day prescriptions with shorter prescriptions |
Appendix 4 Risk-of-bias assessments: Risk Of Bias in Non-Randomized Studies – of Interventions
Bias domain | Signalling questions | Elaboration | Response options |
---|---|---|---|
Bias as a result of confounding | (1.1) Is there potential for confounding of the effect of intervention in this study? | In rare situations, such as when studying harms that are very unlikely to be related to factors that influence treatment decisions, no confounding is expected and the study can be considered to be at low risk of bias owing to confounding, equivalent to a fully randomised trial. There is no NI option for this signalling question | Y/PY/PN/N |
If Y/PY to 1.1 | |||
(1.2) Was the analysis based on splitting participants’ follow-up time according to intervention received? | If participants could switch between intervention groups then associations between intervention and outcome may be biased by time-varying confounding. This occurs when prognostic factors influence switches between intended interventions | NA/Y/PY/PN/N/NI | |
If Y/PY to 1.2 | |||
(1.3) Were intervention discontinuations or switches likely to be related to factors that are prognostic for the outcome? | If intervention switches are unrelated to the outcome, for example when the outcome is an unexpected harm, then time-varying confounding will not be present and only control for baseline confounding is required | NA/Y/PY/PN/N/NI | |
Baseline confounding | |||
(1.4) Did the authors use an appropriate analysis method that controlled for all the important confounding areas? | Appropriate methods to control for measured confounders include stratification, regression, matching, standardisation, and inverse probability weighting. They may control for individual variables or for the estimated propensity score. Inverse probability weighting is based on a function of the propensity score. Each method depends on the assumption that there is no unmeasured or residual confounding | NA/Y/PY/PN/N/NI | |
If Y/PY to 1.4 | |||
(1.5) Were confounding areas that were controlled for measured validly and reliably by the variables available in this study? | Appropriate control of confounding requires that the variables adjusted for are valid and reliable measures of the confounding domains. For some topics, a list of valid and reliable measures of confounding domains will be specified in the review protocol, but for others such a list may not be available. Study authors may cite references to support the use of a particular measure. If authors control for confounding variables with no indication of their validity or reliability pay attention to the subjectivity of the measure.30 Subjective measures (e.g. based on self-report) may have lower validity and reliability than objective measures such as laboratory findings | NA/Y/PY/PN/N/NI | |
(1.6) Did the authors control for any post-intervention variables? | Controlling for post-intervention variables that are affected by the intervention is not appropriate. Controlling for mediating variables estimates the direct effect of intervention and may introduce bias. Controlling for common effects of intervention and outcome introduces bias | NA/Y/PY/PN/N/NI | |
Baseline and time-varying confounding | |||
(1.7) Did the authors use an appropriate analysis method that adjusted for all the important confounding areas and for time varying confounding? | Adjustment for time-varying confounding is necessary to estimate the effect of starting and adhering to intervention, in both randomised trials and NRSI. Appropriate methods include those based on inverse probability weighting. Standard regression models that include time-updated confounders may be problematic if time-varying confounding is present | NA/Y/PY/PN/N/NI | |
If Y/PY to 1.7 | |||
(1.8) Were confounding areas that were adjusted for measured validly and reliably by the variables available in this study? | See 1.5 above | NA/Y/PY/PN/N/NI | |
Risk-of-bias judgement | Low/moderate/serious/critical/NI | ||
Bias in selection of participants into the study | (2.1) Was selection of participants into the study (or into the analysis) based on participant characteristics observed after the start of intervention? | This domain is concerned only with selection into the study based on participant characteristics observed after the start of intervention. Selection based on characteristics observed before the start of intervention can be addressed by controlling for imbalances between experimental intervention and comparator groups in baseline characteristics that are prognostic for the outcome (baseline confounding). Selection bias occurs when selection is related to an effect of either intervention or a cause of intervention and an effect of either the outcome or a cause of the outcome. Therefore, the result is at risk of selection bias if selection into the study is related to both the intervention and the outcome | Y/PY/PN/N/NI |
If N/PN to 2.1 go to 2.4 | |||
(2.2) Were the post-intervention variables that influenced selection likely to be associated with intervention? | NA/Y/PY/PN/N/NI | ||
(2.3) Were the post-intervention variables that influenced selection likely to be influenced by the outcome or a cause of the outcome? | NA/Y/PY/PN/N/NI | ||
(2.4) Do start of follow-up and start of intervention coincide for most participants? | If participants are not followed from the start of the intervention, then a period of follow-up has been excluded, and individuals who experienced the outcome soon after intervention will be missing from analyses. This problem may occur when prevalent, rather than new (incident), users of the intervention are included in analyses | Y/PY/PN/N/NI | |
If Y/PY to 2.2 and 2.3, or N/PN to 2.4 | |||
(2.5) Were adjustment techniques used that are likely to correct for the presence of selection biases? | It is, in principle, possible to correct for selection biases, for example by using inverse probability weights to create a pseudo-population in which the selection bias has been removed, or by modelling the distributions of the missing participants or follow-up times and outcome events, and including them using missing data methodology. However, such methods are rarely used, and the answer to this question will usually be ‘no’ | NA/Y/PY/PN/N/NI | |
Risk-of-bias judgement | Low/moderate/serious/critical/NI | ||
Bias in classification of interventions | (3.1) Were intervention groups clearly defined? | A prerequisite for an appropriate comparison of interventions is that the interventions are well defined. Ambiguity in the definition may lead to bias in the classification of participants. For individual-level interventions, criteria for considering individuals to have received each intervention should be clear and explicit, covering issues such as type, setting, dose, frequency, intensity and/or timing of intervention. For population-level interventions (e.g. measures to control air pollution), the question relates to whether or not the population is clearly defined, and the answer is likely to be ‘yes’ | Y/PY/PN/N/NI |
(3.2) Was the information used to define intervention groups recorded at the start of the intervention? | In general, if information about interventions received is available from sources that could not have been affected by subsequent outcomes, then differential misclassification of intervention status is unlikely. Collection of the information at the time of the intervention makes it easier to avoid such misclassification. For population-level interventions (e.g. measures to control air pollution), the answer to this question is likely to be ‘yes’ | Y/PY/PN/N/NI | |
(3.3) Could classification of intervention status have been affected by knowledge of the outcome or risk of the outcome? | Collection of the information at the time of the intervention may not be sufficient to avoid bias. The way in which the data are collected for the purposes of the NRSI should also avoid misclassification | Y/PY/PN/N/NI | |
Risk-of-bias judgement | Low/moderate/serious/critical/NI | ||
Bias as a result of departures from intended interventions | (4.1) Were there deviations from the intended intervention beyond what would be expected in usual practice? | Deviations that happen in usual practice following the intervention (e.g. cessation of a drug intervention because of acute toxicity) are part of the intended intervention and therefore do not lead to bias in the effect of assignment to intervention. Deviations may arise due to expectations of a difference between intervention and comparator (e.g. because participants feel unlucky to have been assigned to the comparator group and therefore seek the active intervention, or components of it, or other interventions). Such deviations are not part of usual practice, so may lead to biased effect estimates. However, these are not expected in observational studies of individuals in routine care | Y/PY/PN/N/NI |
If Y/PY to 4.1 | |||
(4.2) Were these deviations from intended intervention unbalanced between groups and likely to have affected the outcome? | Deviations from intended interventions that do not reflect usual practice will be important if they affect the outcome, but not otherwise. Furthermore, bias will arise only if there is imbalance in the deviations across the two groups | NA/Y/PY/PN/N/NI | |
If your aim for this study is to assess the effect of initiating and adhering to intervention (as in a per-protocol analysis), answer questions 4.3 to 4.6 | |||
(4.3) Were important cointerventions balanced across intervention groups? | Risk of bias will be higher if unplanned cointerventions were implemented in a way that would bias the estimated effect of intervention. Cointerventions will be important if they affect the outcome, but not otherwise. Bias will arise only if there is imbalance in such cointerventions between the intervention groups. Consider the cointerventions, including any prespecified cointerventions, that are likely to affect the outcome and to have been administered in this study. Consider whether these cointerventions are balanced between intervention groups | Y/PY/PN/N/NI | |
(4.4) Was the intervention implemented successfully for most participants? | Risk of bias will be higher if the intervention was not implemented as intended by, for example, the health care professionals delivering care during the trial. Consider whether or not implementation of the intervention was successful for most participants | Y/PY/PN/N/NI | |
(4.5) Did study participants adhere to the assigned intervention regimen? | Risk of bias will be higher if participants did not adhere to the intervention as intended. Lack of adherence includes imperfect compliance, cessation of intervention, crossovers to the comparator intervention and switches to another active intervention. Consider available information on the proportion of study participants who continued with their assigned intervention throughout follow up, and answer ‘No’ or ‘Probably No’ if this proportion is high enough to raise concerns. Answer ‘Yes’ for studies of interventions that are administered once, so that imperfect adherence is not possible. We distinguish between analyses where follow-up time after interventions switches (including cessation of intervention) is assigned to (1) the new intervention or (2) the original intervention. (1) is addressed under time-varying confounding, and should not be considered further here | Y/PY/PN/N/NI | |
If N/PN to 4.3, 4.4 or 4.5 | |||
(4.6) Was an appropriate analysis used to estimate the effect of starting and adhering to the intervention? | It is possible to conduct an analysis that corrects for some types of deviation from the intended intervention. Examples of appropriate analysis strategies include inverse probability weighting or instrumental variable estimation. It is possible that a paper reports such an analysis without reporting information on the deviations from intended intervention, but it would be hard to judge such an analysis to be appropriate in the absence of such information. Specialist advice may be needed to assess studies that used these approaches. If everyone in one group received a cointervention, adjustments cannot be made to overcome this | NA/Y/PY/PN/N/NI | |
Risk of bias judgement | Low/moderate/serious/critical/NI | ||
Bias as a result of missing data | (5.1) Were outcome data available for all, or nearly all, participants? | ‘Nearly all’ should be interpreted as ‘enough to be confident of the findings’, and a suitable proportion depends on the context. In some situations, availability of data from 95% (or possibly 90%) of the participants may be sufficient, providing that events of interest are reasonably common in both intervention groups. One aspect of this is that review authors would ideally try to locate an analysis plan for the study | Y/PY/PN/N/NI |
(5.2) Were participants excluded due to missing data on intervention status? | Missing intervention status may be a problem. This requires that the intended study sample is clear, which it may not be in practice | Y/PY/PN/N/NI | |
(5.3) Were participants excluded due to missing data on other variables needed for the analysis? | This question relates particularly to participants excluded from the analysis because of missing information on confounders that were controlled for in the analysis | Y/PY/PN/N/NI | |
If PN/N to 5.1 or Y/PY to 5.2 or 5.3 | |||
(5.4) Are the proportion of participants and reasons for missing data similar across interventions? | This aims to elicit whether either (1) differential proportion of missing observations or (2) differences in reasons for missing observations could substantially impact on our ability to answer the question being addressed. ‘Similar’ includes some minor degree of discrepancy across intervention groups as expected by chance | NA/Y/PY/PN/N/NI | |
If PN/N to 5.1 or Y/PY to 5.2 or 5.3 | |||
(5.5) Is there evidence that results were robust to the presence of missing data? | 5.5 Evidence for robustness may come from how missing data were handled in the analysis and whether sensitivity analyses were performed by the investigators, or occasionally from additional analyses performed by the systematic reviewers. It is important to assess whether or not assumptions employed in analyses are clear and plausible. Both content knowledge and statistical expertise will often be required for this. For instance, use of a statistical method such as multiple imputation does not guarantee an appropriate answer. Review authors should seek naive (complete-case) analyses for comparison, and clear differences between complete-case and multiple imputation-based findings should lead to careful assessment of the validity of the methods used | NA/Y/PY/PN/N/NI | |
Risk of bias judgement | Low/moderate/serious/critical/NI | ||
Bias in measurement of outcomes | (6.1) Could the outcome measure have been influenced by knowledge of the intervention received? | Some outcome measures involve negligible assessor judgement, for example all-cause mortality or non-repeatable automated laboratory assessments. Risk of bias owing to measurement of these outcomes would be expected to be low | Y/PY/PN/N/NI |
(6.2) Were outcome assessors aware of the intervention received by study participants? | If outcome assessors were blinded to intervention status, the answer to this question would be ‘No’. In other situations, outcome assessors may be unaware of the interventions being received by participants, despite there being no active blinding by the study investigators; the answer to this question would then also be ‘No’. In studies where participants report their outcomes themselves, for example in a questionnaire, the outcome assessor is the study participant. In an observational study, the answer to this question will usually be ‘Yes’ when the participants report their outcomes themselves | Y/PY/PN/N/NI | |
(6.3) Were the methods of outcome assessment comparable across intervention groups? | Comparable assessment methods (i.e. data collection) would involve the same outcome detection methods and thresholds, same time point, same definition, and same measurements | Y/PY/PN/N/NI | |
(6.4) Were any systematic errors in measurement of the outcome related to intervention received? | This question refers to differential misclassification of outcomes. Systematic errors in measuring the outcome, if present, could cause bias if they are related to the intervention or to a confounder of the intervention–outcome relationship. This will usually be because of either outcome assessors being aware of the intervention received or becase of non-comparability of outcome assessment methods, but there are examples of differential misclassification arising despite these controls being in place | Y/PY/PN/N/NI | |
Risk of bias judgement | Low/moderate/serious/critical/NI | ||
Bias in selection of the reported result | Is the reported effect estimate likely to be selected, on the basis of the results, from | ||
(7.1) multiple outcome measurements within the outcome domain? | For a specified outcome domain, it is possible to generate multiple effect estimates for different measurements. If multiple measurements were made, but only one or a subset is reported, there is a risk of selective reporting on the basis of results | Y/PY/PN/N/NI | |
(7.2) multiple analyses of the intervention–outcome relationship? | Because of the limitations of using data from non-randomised studies for analyses of effectiveness (need to control confounding, substantial missing data, etc.), analysts may implement different analytic methods to address these limitations. Examples include unadjusted and adjusted models, use of final value vs. change from baseline vs. analysis of covariance, different transformations of variables, a continuously scaled outcome converted to categorical data with different cut-points, different sets of covariates used for adjustment, and different analytic strategies for dealing with missing data. Application of such methods generates multiple estimates of the effect of the intervention vs. the comparator on the outcome. If the analyst does not prespecify the methods to be applied, and multiple estimates are generated but only one or a subset is reported, there is a risk of selective reporting on the basis of results | Y/PY/PN/N/NI | |
(7.3) different subgroups? | Particularly with large cohorts often available from routine data sources, it is possible to generate multiple effect estimates for different subgroups or simply to omit varying proportions of the original cohort. If multiple estimates are generated but only one or a subset is reported, there is a risk of selective reporting on the basis of results | Y/PY/PN/N/NI | |
Risk of bias judgement | Low/moderate/serious/critical/NI | ||
Overall bias | Low/moderate/serious/critical/NI |
Appendix 5 Clinical Practice Research Datalink product code lists
Appendix 5 is provided as a Microsoft Excel file containing five worksheets (one for each of the five case study conditions) (see Report Supplementary Material 1). Within each worksheet the lists of product codes obtained from the CPRD Research Applications Code Browser Version 3.0.0 that represent the possible medications that patients may be prescribed for the treatment of one of the five case study conditions is presented. Table 25 provides a high-level summary of the codes.
Study condition | Product code |
---|---|
Glucose control with oral drug therapy in T2DM | 2219 |
7912 | |
16602 | |
26218 | |
41558 | |
Treatment of hypertension in T2DM | 2 |
58 | |
1209 | |
1211 | |
1213 | |
Lipid management in T2DM | 65193 |
63140 | |
55034 | |
51200 | |
7374 | |
Secondary prevention of myocardial infarction | 59699 |
56850 | |
34544 | |
3310 | |
26995 | |
Treatment of depression | 2525 |
48065 | |
4690 | |
8831 | |
873 |
Appendix 6 Unit prescription drug cost calculations
Drug substance | DDD (mg) | NIC from PCA (pence) | Quantity from PCA | Strength from PCA (mg) | NIC/quantity (£) | Cost per daya (£) |
---|---|---|---|---|---|---|
Initial glucose control in T2DM | ||||||
Glibenclamide | 7 | 1,072,539 | 279,251 | 5 | 0.04 | 0.05 |
Gliclazide | 60 | 3,322,771 | 195,041 | 60 | 0.17 | 0.17 |
Glimepiride | 2 | 2,650,357 | 685,422 | 2 | 0.04 | 0.04 |
Glipizide | 10 | 8,679,376 | 762,384 | 5 | 0.11 | 0.23 |
Tolbutamide | 1500 | 17,494,096 | 266,373 | 500 | 0.66 | 1.97 |
Metformin | 2000 | 531,088,447 | 111,025,385 | 500 | 0.05 | 0.19 |
Acarbose | 300 | 108,607 | 1234 | 100 | 0.88 | 2.64 |
Alogliptin | 25 | 6,918,604 | 10,147 | 25 | 6.82 | 6.82 |
Canagliflozin | 200 | 58,466,442 | 447,445 | 100 | 1.31 | 2.61 |
Dapagliflozin | 10 | 191,937,124 | 1,468,764 | 10 | 1.31 | 1.31 |
Empagliflozin | 17.5 | 16,291,281 | 124,666 | 10 | 1.31 | 2.29 |
Exenatide | 1 | 10,031,280 | 1470 | 60 | 68.24 | 1.14 |
Linagliptin | 5 | 271,703,001 | 2,287,246 | 5 | 1.19 | 1.19 |
Liraglutide | 1.2 | 337,718,696 | 86,033 | 6 | 39.25 | 7.85 |
Lixisenatide | 0.02 | 45,851,757 | 15,830 | 0.28 | 28.97 | 2.07 |
Nateglinide | 360 | 631,585 | 17,827 | 180 | 0.35 | 0.71 |
Pioglitazone | 30 | 144,198,158 | 1,125,016 | 30 | 1.28 | 1.28 |
Repaglinide | 4 | 1,620,508 | 246,350 | 2 | 0.07 | 0.13 |
Saxagliptin | 5 | 80,113,342 | 709,874 | 5 | 1.13 | 1.13 |
Sitagliptin | 100 | 670,533,026 | 5,644,766 | 100 | 1.19 | 1.19 |
Vildagliptin | 100 | 18,582,165 | 312,012 | 50 | 0.60 | 1.19 |
Rosiglitazoneb | 6 | – | – | – | – | 2.34 |
Guar gumb,c | 68.5 | – | – | – | – | 2.34 |
Dulaglutide | 0.16 | 1,717,714 | 938 | 0.75 | 18.31 | 3.91 |
Hypertension in T2DM | ||||||
Bendroflumethiazide | 2.5 | 115,395,532 | 38,916,033 | 2.5 | 0.03 | 0.03 |
Chlortalidone | 25 | 410 | 70 | 50 | 0.06 | 0.03 |
Cyclopenthiazideb | 0.5 | – | – | – | – | 1.07 |
Indapamide | 2.5 | 40,603,410 | 7,849,048 | 2.5 | 0.05 | 0.05 |
Xipamide | 20 | 274,581 | 19,761 | 20 | 0.14 | 0.14 |
Chlorothiazide | 500 | 92,160 | 200 | 500 | 4.61 | 4.61 |
Hydrochlorothiazide | 25 | 743,206 | 4701 | 25 | 1.58 | 1.58 |
Hydroflumethiazideb | 25 | – | – | – | – | 1.07 |
Candesartan cilexetil | 8 | 27,228,854 | 6,187,293 | 8 | 0.04 | 0.04 |
Eprosartan | 600 | 7,848,330 | 156,629 | 600 | 0.50 | 0.50 |
Irbesartan | 150 | 15,321,402 | 2,527,161 | 150 | 0.06 | 0.06 |
Losartan potassium | 50 | 43,142,921 | 10,854,773 | 50 | 0.04 | 0.04 |
Olmesartan medoxomil | 20 | 23,454,180 | 507,120 | 20 | 0.46 | 0.46 |
Telmisartan | 40 | 2,105,968 | 429,294 | 40 | 0.05 | 0.05 |
Valsartan | 80 | 7,680,126 | 790,946 | 80 | 0.10 | 0.10 |
Captopril | 50 | 1,209,326 | 274,077 | 50 | 0.04 | 0.04 |
Enalapril maleate | 10 | 8,273,970 | 2,164,274 | 10 | 0.04 | 0.04 |
Fosinopril sodium | 15 | 1,717,978 | 32,658 | 10 | 0.53 | 0.79 |
Imidapril hydrochloride | 10 | 523,685 | 20,308 | 10 | 0.26 | 0.26 |
Lisinopril | 10 | 30,787,577 | 8,978,102 | 10 | 0.03 | 0.03 |
Moexipril hydrochloride | 15 | 22,272 | 896 | 15 | 0.25 | 0.25 |
Perindopril erbumine | 4 | 25,785,508 | 604,0253 | 4 | 0.04 | 0.04 |
Perindopril arginine | 4 | 1,125,376 | 53,760 | 5 | 0.21 | 0.17 |
Quinapril | 15 | 2,130,784 | 69,778 | 10 | 0.31 | 0.46 |
Ramipril | 2.5 | 75,859,752 | 18,621,897 | 2.5 | 0.04 | 0.04 |
Ramipril with felodipine | 2.5 | 456,727 | 5209 | 2.5 | 0.88 | 0.88 |
Trandolapril | 2 | 4,668,257 | 191,955 | 2 | 0.24 | 0.24 |
Cilazapril | 2.5 | 1,662,225 | 8985 | 5 | 1.85 | 0.93 |
Perindopril tosilate | 4 | 11,821 | 594 | 5 | 0.20 | 0.16 |
Amlodipine | 5 | 153,321,726 | 47,137,956 | 5 | 0.03 | 0.03 |
Diltiazem hydrochloride | 240 | 3,855,905 | 93,720 | 240 | 0.41 | 0.41 |
Felodipine | 5 | 63,115,662 | 4,197,918 | 5 | 0.15 | 0.15 |
Isradipine | 5 | 4,429,879 | 13,442 | 2.5 | 3.30 | 6.59 |
Lacidipine | 4 | 14,646,423 | 910,750 | 4 | 0.16 | 0.16 |
Lercanidipine hydrochloride | 10 | 77,973,540 | 3,825,663 | 10 | 0.20 | 0.20 |
Nicardipine hydrochloride | 90 | 397,902 | 35,119 | 30 | 0.11 | 0.34 |
Nifedipine | 30 | 3,656,530 | 149,471 | 30 | 0.24 | 0.24 |
Nisoldipineb | 20 | – | – | – | – | 0.93 |
Verapamil hydrochloride | 240 | 7,104,750 | 358,394 | 240 | 0.20 | 0.20 |
Doxazosin | 4 | 52,769,814 | 14,187,844 | 4 | 0.04 | 0.04 |
Indoraminc | 4.7 | 5,674,414 | 34,4947 | 20 | 0.16 | 0.04 |
Prazosin | 5 | 74,527 | 205 | 5 | 3.64 | 3.64 |
Terazosin | 5 | 1,372,116 | 136,216 | 5 | 0.10 | 0.10 |
Amiloride hydrochloride | 10 | 18,835,995 | 565,354 | 5 | 0.33 | 0.67 |
Amiloride hydrochloride with thiazide | 10 | 868,138 | 75,026 | 5 | 0.12 | 0.23 |
Triamterene | 100 | 211,025 | 1511 | 50 | 1.40 | 2.79 |
Triamterene with thiazide | 100 | 160,534 | 4540 | 50 | 0.35 | 0.71 |
Spironolactone | 75 | 24,174,720 | 4,339,112 | 25 | 0.06 | 0.17 |
Atenolol | 75 | 25,567,900 | 8,522,336 | 25 | 0.03 | 0.09 |
Hyperlipidaemia in T2DM | ||||||
Atorvastatin | 20 | 158,989,904 | 31,324,266 | 20 | 0.05 | 0.05 |
Fluvastatin | 60 | 1,475,946 | 163,264 | 20 | 0.09 | 0.27 |
Pravastatin | 30 | 6,780,883 | 1,648,896 | 10 | 0.04 | 0.12 |
Rosuvastatin | 10 | 134,267,696 | 2,085,108 | 10 | 0.64 | 0.64 |
Simvastatin | 30 | 15,635,680 | 5,206,048 | 10 | 0.03 | 0.09 |
Secondary prevention of myocardial infarction | ||||||
Captopril | 50 | 1,209,326 | 274,077 | 50 | 0.04 | 0.04 |
Enalapril maleate | 10 | 8,273,970 | 2,164,274 | 10 | 0.04 | 0.04 |
Fosinopril sodium | 15 | 1,717,978 | 32,658 | 10 | 0.53 | 0.79 |
Lisinopril | 10 | 30,787,577 | 8,978,102 | 10 | 0.03 | 0.03 |
Perindopril erbumine | 4 | 25,785,508 | 6,040,253 | 4 | 0.04 | 0.04 |
Perindopril arginine | 4 | 1,125,376 | 53,760 | 5 | 0.21 | 0.17 |
Quinapril | 15 | 728,515 | 23,878 | 5 | 0.31 | 0.92 |
Ramipril | 2.5 | 75,859,752 | 18,621,897 | 2.5 | 0.04 | 0.04 |
Ramipril with felodipine | 2.5 | 456,727 | 5209 | 2.5 | 0.88 | 0.88 |
Trandolapril | 2 | 4,668,257 | 191,955 | 2 | 0.24 | 0.24 |
Cilazapril | 2.5 | 1,662,225 | 8985 | 5 | 1.85 | 0.93 |
Perindopril tosilate | 4 | 11,821 | 594 | 5 | 0.20 | 0.16 |
Acebutolol | 400 | 1,202,284 | 18,079 | 400 | 0.67 | 0.67 |
Atenolol | 75 | 25,567,900 | 8,522,336 | 25 | 0.03 | 0.09 |
Bisoprolol | 10 | 20,420,456 | 5,828,018 | 10 | 0.04 | 0.04 |
Carvedilol | 37.5 | 2,684,299 | 577,569 | 6.25 | 0.05 | 0.28 |
Metoprolol tartrate | 150 | 15,398,869 | 2,318,257 | 50 | 0.07 | 0.20 |
Aspirinc | 127.5 | 154,533,218 | 52,719,924 | 75 | 0.03 | 0.05 |
Clopidogrel | 75 | 129,484,315 | 19,793,087 | 75 | 0.07 | 0.07 |
Ticagrelor | 180 | 190,143,810 | 1,950,196 | 90 | 0.97 | 1.95 |
Atorvastatin | 20 | 158,989,904 | 31,324,266 | 20 | 0.05 | 0.05 |
Fluvastatin | 60 | 1,475,946 | 163,264 | 20 | 0.09 | 0.27 |
Pravastatin | 30 | 6,780,883 | 1,648,896 | 10 | 0.04 | 0.12 |
Rosuvastatin | 10 | 134,267,696 | 2,085,108 | 10 | 0.64 | 0.64 |
Simvastatin | 30 | 15,635,680 | 5,206,048 | 10 | 0.03 | 0.09 |
Candesartan cilexetil | 8 | 27,228,854 | 6,187,293 | 8 | 0.04 | 0.04 |
Eprosartan | 600 | 7,848,330 | 156,629 | 600 | 0.50 | 0.50 |
Irbesartan | 150 | 15,321,402 | 2,527,161 | 150 | 0.06 | 0.06 |
Losartan potassium | 50 | 43,142,921 | 10,854,773 | 50 | 0.04 | 0.04 |
Olmesartan medoxomil | 20 | 23,454,180 | 507,120 | 20 | 0.46 | 0.46 |
Telmisartan | 40 | 2,105,968 | 429,294 | 40 | 0.05 | 0.05 |
Valsartan | 80 | 7,680,126 | 790,946 | 80 | 0.10 | 0.10 |
Depression | ||||||
Amitriptyline hydrochloride | 75 | 42,327,326 | 12,089,330 | 25 | 0.04 | 0.11 |
Amoxapine | 150 | 65,772 | 168 | 100 | 3.92 | 5.87 |
Clomipramine hydrochloride | 100 | 5,343,700 | 663,607 | 50 | 0.08 | 0.16 |
Dosulepin hydrochloride | 150 | 9,835,231 | 1,582,426 | 75 | 0.06 | 0.12 |
Doxepind | 100 | – | – | 50 | 0.20 | 0.41 |
Imipramine hydrochloride | 100 | 4,075,545 | 858,036 | 25 | 0.05 | 0.19 |
Lofepramine | 105 | 39,309,425 | 1,177,389 | 70 | 0.33 | 0.50 |
Nortriptyline | 75 | 115,762,972 | 1,077,134 | 25 | 1.07 | 3.22 |
Trazodone hydrochloride | 300 | 61,816,624 | 718,214 | 150 | 0.86 | 1.72 |
Trimipramine | 150 | 1672 | 56 | 50 | 0.30 | 0.90 |
Citalopram | 20 | 86,323,713 | 23,901,861 | 20 | 0.04 | 0.04 |
Escitalopram | 10 | 5,540,518 | 1,121,092 | 10 | 0.05 | 0.05 |
Fluoxetine | 20 | 87,922,802 | 23,735,904 | 20 | 0.04 | 0.04 |
Fluvoxamine maleate | 100 | 4,073,827 | 55,899 | 100 | 0.73 | 0.73 |
Paroxetine | 20 | 25,034,806 | 3,116,825 | 20 | 0.08 | 0.08 |
Sertraline | 50 | 100,699,567 | 15,289,272 | 50 | 0.07 | 0.07 |
Isocarboxazid | 15 | 3,453,630 | 12,118 | 10 | 2.85 | 4.28 |
Moclobemide | 300 | 1,057,512 | 22,678 | 300 | 0.47 | 0.47 |
Phenelzine | 60 | 2,647,884 | 117,682 | 15 | 0.23 | 0.90 |
Tranylcypromine | 10 | 45,505,295 | 51,406 | 10 | 8.85 | 8.85 |
Agomelatine | 25 | 8,035,533 | 74,999 | 25 | 1.07 | 1.07 |
Duloxetine | 60 | 276,427,017 | 2,904,742 | 60 | 0.95 | 0.95 |
Mirtazapine | 30 | 26,199,391 | 4,793,099 | 30 | 0.05 | 0.05 |
Reboxetine | 8 | 4,467,383 | 141,747 | 4 | 0.32 | 0.63 |
Tryptophanc | 44.6 | 18,495 | 566 | 50 | 0.33 | 0.29 |
Venlafaxine | 100 | 17,440,533 | 3,256,789 | 75 | 0.05 | 0.07 |
Appendix 7 Search strategy and study selection details for prescriber time data
Search strategy
Date of search: 8 July 2016.
Databases: Ovid MEDLINE (R) Epub Ahead of Print, In-Process & Other Non-Indexed Citations, Ovid MEDLINE (R) Daily and Ovid MEDLINE (R) 1946 to present; EMBASE 1974 to 7 July 2016.
-
general practitioner.ab,hw,kf,kw,ot,ti,xs.
-
GP.ab,hw,kf,kw,ot,ti,xs.
-
physician.ab,hw,kf,kw,ot,ti,xs.
-
clinician.ab,hw,kf,kw,ot,ti,xs.
-
doctor.ab,hw,kf,kw,ot,ti,xs.
-
medic.ab,hw,kf,kw,ot,ti,xs.
-
consultant.ab,hw,kf,kw,ot,ti,xs.
-
medical specialist.ab,hw,kf,kw,ot,ti,xs.
-
physician assistant.ab,hw,kf,kw,ot,ti,xs.
-
physician associate.ab,hw,kf,kw,ot,ti,xs.
-
nurse.ab,hw,kf,kw,ot,ti,xs.
-
pharmacist.ab,hw,kf,kw,ot,ti,xs.
-
healthcare professional.ab,hw,kf,kw,ot,ti,xs.
-
medical professional.ab,hw,kf,kw,ot,ti,xs.
-
medical staff.ab,hw,kf,kw,ot,ti,xs.
-
1 or 2 or 3 or 4 or 5 or 6 or 7 or 8 or 9 or 10 or 11 or 12 or 13 or 14 or 15
-
prescriber time.ab,hw,kf,kw,ot,ti,xs.
-
staff time.ab,hw,kf,kw,ot,ti,xs.
-
time utilization.ab,hw,kf,kw,ot,ti,xs.
-
time utilisation.ab,hw,kf,kw,ot,ti,xs.
-
workload.ab,hw,kf,kw,ot,ti,xs.
-
workflow.ab,hw,kf,kw,ot,ti,xs.
-
work processes.ab,hw,kf,kw,ot,ti,xs.
-
medication management.ab,hw,kf,kw,ot,ti,xs.
-
17 or 18 or 19 or 20 or 21 or 22 or 23 or 24
-
time study.ab,hw,kf,kw,ot,ti,xs.
-
time motion study.ab,hw,kf,kw,ot,ti,xs.
-
time-motion study.ab,hw,kf,kw,ot,ti,xs.
-
(time and motion method).ab,hw,kf,kw,ot,ti,xs.
-
time-and-motion method.ab,hw,kf,kw,ot,ti,xs.
-
(time and motion study).ab,hw,kf,kw,ot,ti,xs.
-
time-and-motion study.ab,hw,kf,kw,ot,ti,xs.
-
time motion analysis.ab,hw,kf,kw,ot,ti,xs.
-
time-motion analysis.ab,hw,kf,kw,ot,ti,xs.
-
(before and after study).ab,hw,kf,kw,ot,ti,xs.
-
before-and-after study.ab,hw,kf,kw,ot,ti,xs.
-
26 or 27 or 28 or 29 or 30 or 31 or 32 or 33 or 34 or 35 or 36
-
16 and 25 and 37 –
Total hits = 227.
Study selection details
The targeted literature search identified a total of 227 citations. After titles and abstracts were screened, 216 citations were excluded and 11 citations were reviewed in full-text. Four studies contained relevant information and the most appropriate evidence was selected from the four studies by prioritising evidence from larger sample sizes and studies that reported prescriber time for different types of prescriptions (e.g. new vs. renewals) and/or different types of prescribers (GP vs. nurse). 85,100–102 It should be noted that one of the four studies identified was a systematic review and led to the identification of two additional studies with relevant information based on their reporting of mean consultation times based on large sample sizes and in different types of prescribers. 103,104
Appendix 8 Differences in standardised (90-day) total costs for short and long prescription lengths
Scenarios | Values tested | Total cost standardised to 90 days | Difference (cost savings with ≥ 60 days) | ||
---|---|---|---|---|---|
< 60 days | ≥ 60 days | < 60 days | ≥ 60 days | ||
Glucose control with oral drug therapy in T2DM | |||||
Base case | – | – | 8.85 | 2.52 | (6.33) |
No prescriber time cost | 0 | 0 | 2.59 | 1.87 | (0.72) |
50% decrease quantity wasted, days | 0.43 | 2.48 | 8.41 | 1.68 | (6.73) |
50% increase quantity wasted, days | 1.29 | 7.44 | 9.30 | 3.36 | (5.94) |
50% decrease cost of drug per day | 0.18 | 0.14 | 8.41 | 1.68 | (6.73) |
50% increase cost of drug per day | 0.55 | 0.43 | 9.30 | 3.36 | (5.94) |
50% decrease dispensing fee | 0.46 | 0.52 | 8.01 | 2.42 | (5.59) |
50% increase dispensing fee | 1.38 | 1.57 | 9.70 | 2.62 | (7.08) |
50% decrease prescriber time cost | 1.70 | 1.72 | 5.72 | 2.20 | (3.52) |
50% increase prescriber time cost | 5.09 | 5.16 | 11.99 | 2.85 | (9.14) |
Treatment of hypertension in T2DM | |||||
Base case | – | – | 8.13 | 0.75 | (7.38) |
No prescriber time cost | 0 | 0 | 1.88 | 0.63 | (1.25) |
50% decrease quantity wasted, days | 0.62 | 3.49 | 7.99 | 0.46 | (7.53) |
50% increase quantity wasted, days | 1.85 | 10.47 | 8.28 | 1.05 | (7.23) |
50% decrease cost of drug per day | 0.042 | 0.041 | 7.99 | 0.46 | (7.53) |
50% increase cost of drug per day | 0.13 | 0.12 | 8.28 | 1.05 | (7.23) |
50% decrease dispensing fee | 0.45 | 0.46 | 7.34 | 0.74 | (6.60) |
50% increase dispensing fee | 1.35 | 1.39 | 8.93 | 0.77 | (8.16) |
50% decrease prescriber time cost | 1.77 | 1.77 | 5.01 | 0.69 | (4.32) |
50% increase prescriber time cost | 5.30 | 5.32 | 11.26 | 0.82 | (10.44) |
Lipid management in T2DM | |||||
Base case | – | – | 7.21 | 0.12 | (7.09) |
No prescriber time cost | 0 | 0 | 1.75 | 0.96 | (0.79) |
50% decrease quantity wasted, days | 0.31 | 8.11 | 7.12 | (0.49) | (6.63) |
50% increase quantity wasted, days | 0.94 | 24.32 | 7.30 | 0.73 | (6.57) |
50% decrease cost of drug per day | 0.052 | 0.052 | 7.12 | (0.49) | (6.63) |
50% increase cost of drug per day | 0.16 | 0.15 | 7.30 | 0.73 | (6.57) |
50% decrease dispensing fee | 0.45 | 0.50 | 6.42 | 0.25 | (6.17) |
50% increase dispensing fee | 1.35 | 1.50 | 7.99 | (0.013) | (8.00) |
50% decrease prescriber time cost | 1.56 | 1.57 | 4.48 | 0.54 | 3.94 |
50% increase prescriber time cost | 4.68 | 4.72 | 9.93 | (0.30) | (10.23) |
Secondary prevention of myocardial infarction | |||||
Base case | – | – | 8.89 | (0.18) | (9.07) |
No prescriber time cost | 0 | 0 | 1.88 | 0.28 | (1.6) |
50% decrease quantity wasted, days | 0.48 | 3.28 | 8.79 | (0.37) | (9.16) |
50% increase quantity wasted, days | 1.43 | 9.84 | 8.99 | 0.019 | (8.97) |
50% decrease cost of drug per day | 0.037 | 0.034 | 8.79 | (0.37) | (9.16) |
50% increase cost of drug per day | 0.11 | 0.10 | 8.99 | 0.019 | (8.97) |
50% decrease dispensing fee | 0.45 | 0.48 | 8.05 | (0.12) | (8.17) |
50% increase dispensing fee | 1.35 | 1.45 | 9.73 | (0.24) | (9.97) |
50% decrease prescriber time cost | 1.88 | 1.89 | 5.38 | 0.049 | (5.33) |
50% increase prescriber time cost | 5.63 | 5.66 | 12.39 | (0.40) | (11.59) |
Depression | |||||
Base case | – | – | 10.26 | 2.00 | (8.26) |
No prescriber time cost | 0 | 0 | 2.89 | 0.77 | (2.12) |
50% decrease quantity wasted, days | 0.93 | 1.35 | 9.84 | 1.79 | (8.05) |
50% increase quantity wasted, days | 2.80 | 4.04 | 10.67 | 2.20 | (8.47) |
50% decrease cost of drug per day | 0.068 | 0.054 | 9.84 | 1.79 | (8.05) |
50% increase cost of drug per day | 0.20 | 0.16 | 10.67 | 2.20 | (8.47) |
50% decrease dispensing fee | 0.45 | 0.48 | 9.23 | 1.81 | (7.42) |
50% increase dispensing fee | 1.36 | 1.44 | 11.29 | 2.18 | (9.11) |
50% decrease prescriber time cost | 1.61 | 1.59 | 6.58 | 1.39 | (5.19) |
50% increase prescriber time cost | 4.84 | 4.78 | 13.94 | 2.61 | (11.33) |
Appendix 9 Additional tables for decision modelling case study 2
Base case | Range (95% CI) | Source | |
---|---|---|---|
Clinical outcomes | |||
Acute treatment period | |||
Absolute risk of dropout (placebo) | 0.193 | NA | CG90 systematic review of clinical evidence for escitalopram vs. placebo (table 70, CG90)95 |
Relative risk of dropout (escitalopram) | 1.11 | 0.95 to 1.29 | |
Maintenance treatment period | |||
Absolute risk of non-remission (placebo) | 0.686 | NA | |
Relative risk of non-remission (escitalopram) | 0.88 | 0.82 to 0.94 | |
Probability of spontaneous remission for patients who drop out of initial treatment | 0.20 | 0.10 to 0.30 | Expert opinion cited in CG9095 |
Follow-up period | |||
Absolute risk of relapse (placebo) | 0.602 | NA | |
Relative risk of non-remission (escitalopram) | 0.81 | 0.75 to 0.88 | |
Probability of relapse for patients who discontinue initial treatment and in remission | 0.67 | NA |
CG90 systematic review of clinical evidence for escitalopram vs. placebo (table 70, CG90)95 |
Quality-of-life weights | |||
Moderate depression | 0.33 | 0.29 to 0.37 | Same as CG90 decision model93 |
Severe depression | 0.15 | 0.08 to 0.22 | |
Response with remission | 0.85 | 0.83 to 0.87 | |
Response without remission | 0.72 | 0.65 to 0.79 | |
No response | 0.58 | 0.60 to 0.66 |
Unit costs | Resource use | |||||
---|---|---|---|---|---|---|
Per unit | Source | Moderate depression | Severe depression | |||
90-day | 28-day | 90-day | 28-day | |||
Patients who continue treatment, remission and no relapse | ||||||
Escitalopram (20 mg/day), 9-month treatment phase | £0.90 per day | BNF: £25.20 per 28-tab pack106 | £246.38 | £246.38 | £246.38 | £246.38 |
Patient monitoring | ||||||
Moderate depression: seven GP consultations over 9-month treatment phase | £34.00 per GP consultation | Curtis, 2009107 | £238.00 | £238.00 | £577.50 | £577.50 |
Severe depression: seven consultations over 9-month treatment phase (on average 50% mental health outpatient and 50% GP consultation) | £130 per outpatient consultation | |||||
Total cost | £487.08 | £492.48 | £826.58 | £831.98 | ||
Patients who discontinue treatment | ||||||
Escitalopram (20mg/day), assumed take medication for 1 month only | £0.90 per day | BNF: £25.20 per 28-tab pack106 | £82.13 | £27.38 | £82.13 | £27.38 |
Dispensing fees | £0.90 per prescription | NHS Drug Tariff: Part IIIA – Professional Fees (Pharmacy Contractors) July 2016108 | £0.90 | £0.90 | £0.90 | £0.90 |
Patient monitoring | ||||||
Moderate depression: two GP consultations during first month | £34.00 per GP consultation | Curtis, 2009107 | £68.00 | £68.00 | £165.00 | £165.00 |
Severe depression: two consultations during first month (on average 50% mental health outpatient and 50% GP consultation) | £130 per outpatient consultation | |||||
Subsequent treatment: monthly mental health care for remainder of the 15 months, for 65% of patients who remain in contact with health services | Monthly cost of subsequent health care: £165 | NICE guidance95 | £1583.40 | £1583.40 | £1583.40 | £1583.40 |
Total cost | £1734.43 | £1679.68 | £1831.43 | £1776.68 | ||
Non-remission patients | ||||||
Escitalopram (20 mg/day), assumed take medication for 3 months | £0.90 per day | BNF: £25.20 per 28-tab pack106 | £82.13 | £82.13 | £82.13 | £82.13 |
Dispensing fees | £0.90 per prescription | NHS Drug Tariff: Part IIIA – Professional Fees (Pharmacy Contractors) July 2016108 | £0.90 | £2.70 | £0.90 | £2.70 |
Patient monitoring: | ||||||
Moderate depression: four GP consultations during acute treatment | £34.00 per GP consultation | Curtis, 2009107 | £136.00 | £136.00 | £330.00 | £330.00 |
Severe depression: four consultations during acute treatment (on average 50% mental health outpatient and 50% GP consultation) | £130 per outpatient consultation | |||||
Subsequent treatment: monthly mental health care, for 65% of patients who remain in contact with health services | Monthly cost of subsequent health care: £165 | NICE guidance95 | £2088.00 | £2088.00 | £2088.00 | £2088.00 |
Total cost | £2307.03 | £2308.83 | £2501.03 | £2502.83 | ||
Relapse patients | ||||||
Escitalopram (20 mg/day), assumed take medication for 7 months | £0.90 per day | BNF: £25.20 per 28-tab pack106 | £382.38 | £327.63 | £576.38 | £521.63 |
Dispensing fees | £0.90 per prescription | NHS Drug Tariff: Part IIIA – Professional Fees (Pharmacy Contractors) July 2016108 | £2.70 | £6.30 | £2.70 | £6.30 |
Patient monitoring | ||||||
Moderate depression: two GP consultations during first month | £34.00 per GP consultation | Curtis, 2009107 | £68.00 | £68.00 | £165.00 | £165.00 |
Severe depression: two consultations during first month (on average 50% mental health outpatient and 50% GP consultation) | £130 per outpatient consultation | |||||
Subsequent treatment: monthly mental health care for remainder of the 15 months, for 65% of patients who remain in contact with health services | Monthly cost of subsequent health care: £165 | NICE guidance95 | £1392.00 | £1392.00 | £1392.00 | £1392.00 |
Total cost | £1845.08 | £1793.93 | £2136.08 | £2084.93 |
FIGURE 12.
Case study 3: schematic representation of Markov model. Arrows represent the possible transitions between each of the health states. Copyright © NGC. Reproduced with permission. 91
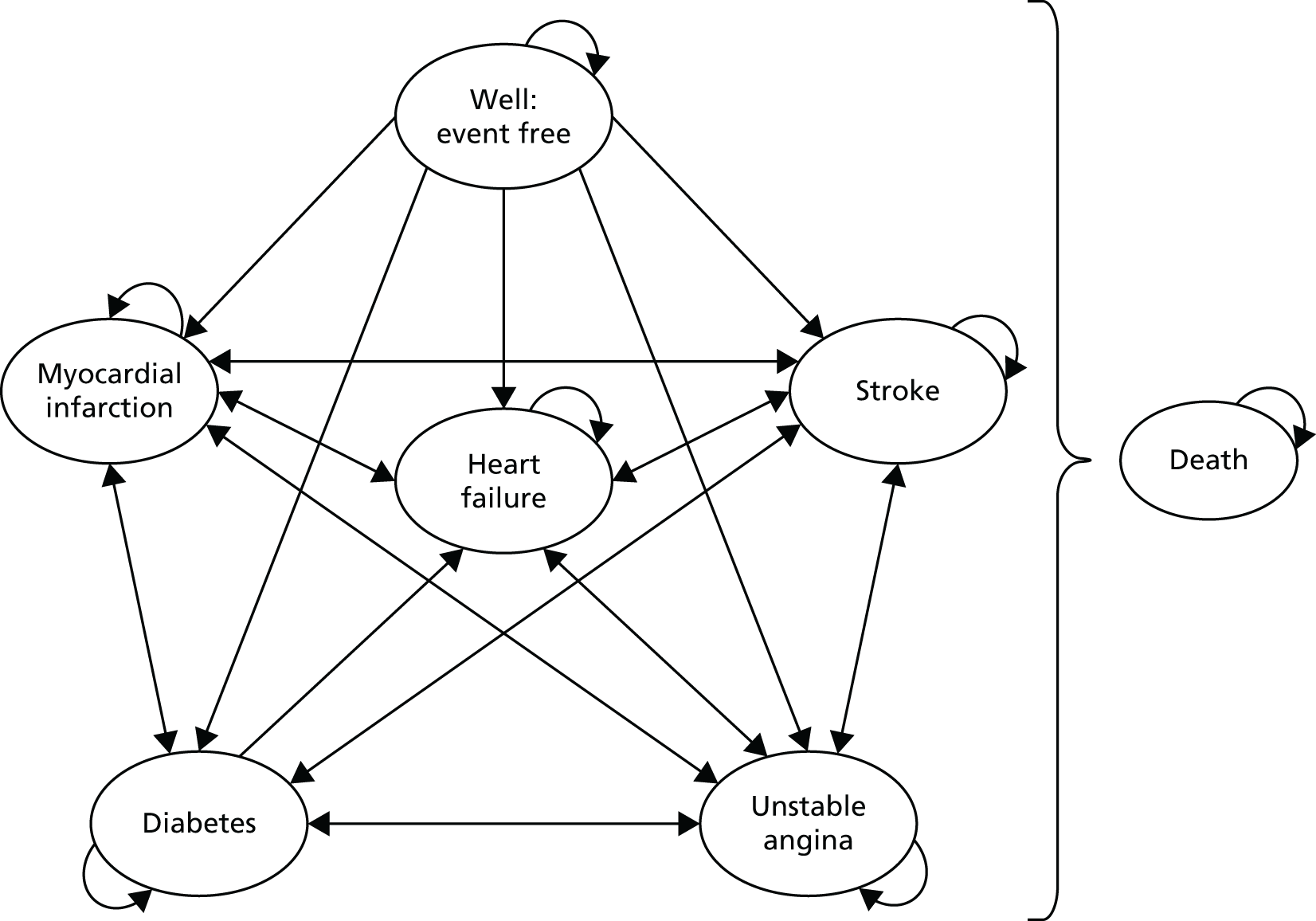
Appendix 10 Additional tables for decision modelling case study 3
Medication/condition | Source model | Adapted model | |
---|---|---|---|
Meta analysis – table 87, NICE91 | Adapted based on Taitel et al.45 study (RR = 0.85) | Adapted based on Hermes et al.35 study (RR = 0.92) | |
ACEIs/ARBs | |||
Unstable angina | 0.98 | 0.98 | 0.98 |
MI | 0.81 | 0.84 | 0.82 |
Diabetes | 0.70 | 0.75 | 0.73 |
Stroke | 0.74 | 0.78 | 0.76 |
Heart failure | 0.63 | 0.68 | 0.66 |
Death | 0.91 | 0.93 | 0.92 |
Beta-blockers | |||
Unstable angina | 0.98 | 0.99 | 0.98 |
MI | 0.85 | 0.88 | 0.87 |
Diabetes | 1.14 | 1.12 | 1.13 |
Stroke | 0.85 | 0.87 | 0.86 |
Heart failure | 0.76 | 0.80 | 0.78 |
Death | 0.94 | 0.95 | 0.94 |
Calcium channel blockers | |||
Unstable angina | 0.88 | 0.90 | 0.89 |
MI | 0.80 | 0.83 | 0.81 |
Diabetes | 0.81 | 0.84 | 0.82 |
Stroke | 0.65 | 0.71 | 0.68 |
Heart failure | 0.73 | 0.77 | 0.75 |
Death | 0.88 | 0.90 | 0.89 |
Thiazide-type diuretics | |||
Unstable angina | 0.89 | 0.91 | 0.90 |
MI | 0.78 | 0.81 | 0.80 |
Diabetes | 0.98 | 0.99 | 0.99 |
Stroke | 0.69 | 0.74 | 0.71 |
Heart failure | 0.53 | 0.60 | 0.57 |
Death | 0.91 | 0.92 | 0.92 |
Intervention | No treatment | ACEIs/ARBs | Beta-blockers | Calcium-channel blockers | Thiazide-type diuretics | Typical treatmenta |
---|---|---|---|---|---|---|
Cost (£) | 4689 | 4011 | 4548 | 4033 | 3911 | 4127 |
QALYs | 9.57 | 10.21 | 9.89 | 10.28 | 10.22 | 10.16 |
Incremental costs (£) | NA | –678 | –141 | –656 | –778 | –562 |
Incremental QALYs | NA | 0.64 | 0.32 | 0.71 | 0.65 | 0.59 |
Simple dominance | NA | Intervention dominant | ||||
ICER (£) | NA | –1059 | –441 | –924 | –1197 | –960 |
List of abbreviations
- ACEI
- angiotensin-converting enzyme inhibitor
- ARB
- angiotensin II receptor blocker
- BNF
- British National Formulary
- C
- cost
- CBT
- cognitive–behavioural therapy
- CCHSR
- Cambridge Centre for Health Services Research
- CI
- confidence interval
- CPRD
- Clinical Practice Research Datalink
- CVD
- cardiovascular disease
- DARE
- Database of Abstracts of Reviews and Effects
- DDD
- defined daily dose
- df
- degrees of freedom
- DH
- Department of Health
- EVPI
- expected value of perfect information
- GBP
- Great British pounds
- GP
- general practitioner
- GRADE
- Grading of Recommendations Assessment, Development and Evaluation
- HSDR
- Health Services and Delivery Research
- HTA
- Health Technology Assessment
- ICER
- incremental cost-effectiveness ratio
- INB
- incremental net benefit
- INsPIRE
- patIeNt and Public Involvement in REsearch
- MD
- mean difference
- MPR
- medication possession ratio
- ndd
- numerical daily dose
- NETSCC
- National Institute for Health Research Evaluation, Trials and Studies Coordinating Centre
- NIC
- net ingredient cost
- NICE
- National Institute for Health and Care Excellence
- NIHR
- National Institute for Health Research
- NMS
- New Medicine Service
- OR
- odds ratio
- PCA
- prescription cost analysis
- PDC
- proportion of days covered
- PRISMA
- Preferred Reporting Items for Systematic Reviews and Meta-Analyses
- PSNC
- Pharmaceutical Services Negotiating Committee
- PSSRU
- Personal Social Services Research Unit
- QALY
- quality-adjusted life-year
- RCT
- randomised controlled trial
- ROBINS-I
- Risk Of Bias in Non-Randomized Studies – of Interventions
- RR
- relative risk
- SD
- standard deviation
- SMD
- standardised mean difference
- SSRI
- selective serotonin reuptake inhibitor
- T2DM
- type 2 diabetes mellitus
- TUC
- total unnecessary cost
- UKPDS
- UK Prospective Diabetes Study