Notes
Article history
The research reported in this issue of the journal was commissioned and funded by the HTA programme on behalf of NICE as project number 16/30/03. The protocol was agreed in March 2017. The assessment report began editorial review in November 2017 and was accepted for publication in August 2018. The authors have been wholly responsible for all data collection, analysis and interpretation, and for writing up their work. The HTA editors and publisher have tried to ensure the accuracy of the authors’ report and would like to thank the reviewers for their constructive comments on the draft document. However, they do not accept liability for damages or losses arising from material published in this report.
Declared competing interests of authors
Robert C Stein is the chief investigator of the OPTIMA (Optimal Personalised Treatment of breast cancer using Multi-parameter Analysis) trial and has published results from the OPTIMA Prelim study.
Permissions
Copyright statement
© Queen’s Printer and Controller of HMSO 2019. This work was produced by Harnan et al. under the terms of a commissioning contract issued by the Secretary of State for Health and Social Care. This issue may be freely reproduced for the purposes of private research and study and extracts (or indeed, the full report) may be included in professional journals provided that suitable acknowledgement is made and the reproduction is not associated with any form of advertising. Applications for commercial reproduction should be addressed to: NIHR Journals Library, National Institute for Health Research, Evaluation, Trials and Studies Coordinating Centre, Alpha House, University of Southampton Science Park, Southampton SO16 7NS, UK.
2019 Queen’s Printer and Controller of HMSO
Chapter 1 Background and definition of the decision problem
This chapter is largely reproduced, with updates when necessary, from the study by Ward et al. 1 Contains information licensed under the Non-Commercial Government Licence v2.0.
Condition and aetiology
Breast cancer is the most commonly diagnosed cancer in women in England and Wales. In 2014, 55,222 new cases of breast cancer were diagnosed. 2 Treatment usually involves surgery to remove the primary tumour and any involved lymph nodes; this may be followed by radiation therapy, endocrine therapy and/or chemotherapy with or without trastuzumab depending on tumour and patient variables. A proportion of patients also receive neoadjuvant therapy prior to surgery.
Aetiology, pathology and prognosis
Aetiology
The causes of breast cancer are not completely understood. A range of risk factors have been identified, including genetic, hormonal and lifestyle factors. 3
It has been estimated that 12% of women with breast cancer have one affected family member and 1% have two or more affected family members. 4 Genetic predisposition is mediated by high-penetrance genes such as BReast CAncer 1 (BRCA1) and BReast CAncer 2 (BRCA2), which are responsible for around 80–90% of hereditary cancers, and low-penetrance genes, which confer both increased and decreased risk. 3
Environmental and lifestyle factors as well as genetic factors influence breast cancer risk. Asian migrants to the West have increased levels of risk compared with the indigenous population, whereas Asian Americans born in the West have incidence rates approximating the US average. 5 Lifestyle and environmental factors thought to increase risk include hormonal factors such as taking the oral contraceptive pill or hormone replacement therapy, higher age of menopause, early age of menarche, late age of first birth and not giving birth. Factors that decrease risk include higher folate intake, higher number of pregnancies, breastfeeding and younger age at first birth. 3 Obesity increases the risk of breast cancer in postmenopausal women. 6 The picture is less clear for premenopausal women, for whom the risk may be lower but prognosis is poorer. Physical activity in adolescence and young adulthood confers a decreased risk of breast cancer,7 which may be mediated hormonally.
Pathology
Breast cancer starts with genetic changes in a single cell or a small group of cells in the epithelia of the ducts or the lobules of the breast. The genetic change allows cells to reproduce uncontrollably, resulting in a tumour. Tumours that have not yet spread to surrounding tissue are known as ‘carcinoma in situ.’ Once it has spread to the surrounding tissue, a tumour is known as ‘invasive’. More rapid growth and spread occurs once a blood supply is secured. Cancer spreads via the lymphatic system or the bloodstream. Lymphatic spread is usually first to the axillary lymph nodes. Spread via the bloodstream can lead to distant metastases in the bone or viscera that are incurable.
The presence or absence of axillary lymph node metastases is a key indicator of disease and prognosis and adjuvant therapy is, in part, planned based on their presence and extent. 8 They are caused by a single cell or a small number of cells detaching from the main tumour, travelling via the lymphatic system and establishing themselves in the tissue of the axillary lymph nodes. Axillary metastases occur in approximately 41% of cases;9 prognosis is better when there is no axillary spread. When metastases are present, axillary clearance is indicated in order to prevent further spread and ensure local disease control.
Prognosis
Overall, the 5-year, age-standardised survival rate for women with breast cancer is 86.3%. 10 Survival varies with age (Figure 1) and stage of disease (Figure 2).
FIGURE 1.
Five-year net survival, by age, for women in England: 2009–13. Adapted with permission from Cancer Research UK. 11
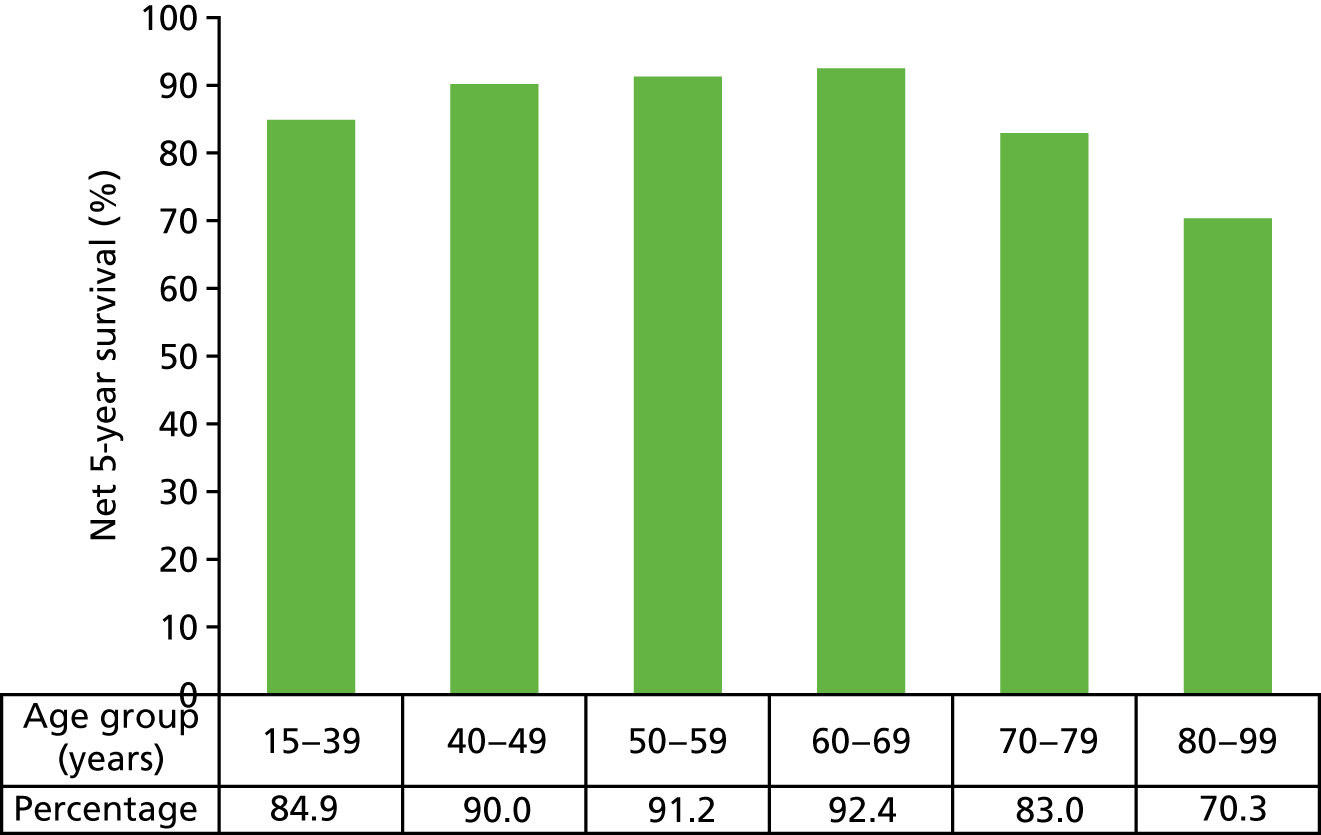
FIGURE 2.
Five-year relative survival, by stage, for women aged 15–99 years in the former Anglia Cancer Network: 2002–6. Adapted with permission from Cancer Research UK. 2
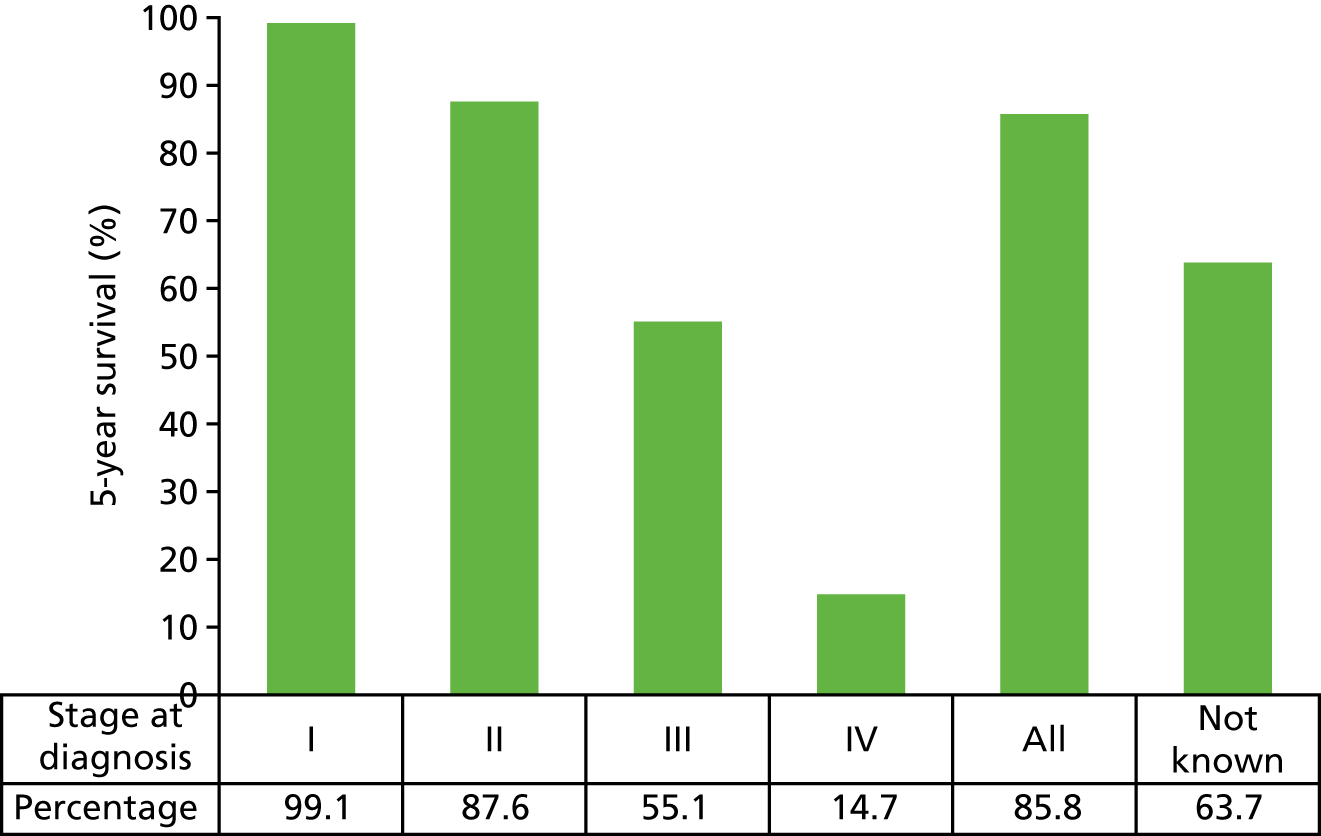
Other factors can also affect prognosis. Clinicians may use tools such as the Nottingham Prognostic Index (NPI),12 Predict (University of Cambridge, Cambridge, UK) or Adjuvant! Online (AOL) (University of Texas Health Sciences Center, San Antonio, TX, USA) to predict disease course and treatment options, although it should be noted that AOL is in the process of being updated and is not currently available. These tools take into account different patient and tumour factors and may give different risk predictions for the same patient.
In general, good prognosis is associated with small tumour size, lymph node-negative (LN0) status, younger age, oestrogen receptor positive (ER+) status and progesterone receptor positive (PR+) status. Overexpression of human epidermal growth factor receptor 2 (HER2) is associated with poorer prognosis.
Epidemiology and incidence
Incidence varies most in accordance with sex. Women are considerably more likely to develop breast cancer than men. For both sexes, incidence varies with age (see Appendix 1, Table 55). Over 81% of cases occur in women aged ≥ 50 years. Based on 2014 data, the highest incidence rates for women were reported in those in the 60- to 70-year age group. 13
Incidence varies with ethnicity. Asian, Chinese and black ethnic groups and those with mixed heritage have a lower incidence of breast cancer than the white ethnic group in England; the rate ratios are 0.65, 0.75, 0.49 and 0.58, respectively, when compared with the white group. 14
Based on data for the period 2006–10, the incidence of female breast cancer was highest in the least deprived 20% of the population; however, the more deprived group had statistically significantly higher mortality. 15 It is unclear why this is, but may be due to lower levels of screening compliance, worse overall general health status and lower levels of treatment attributable to access and compliance issues.
Significance in terms of ill health (burden of disease)
Breast cancer is the second largest cause of cancer deaths in women, after lung cancer, with an age-standardised mortality rate of 34.6 per 100,000 women. In 2014, breast cancer caused 11,360 deaths of women in the UK. 2
Measurement of disease
Breast cancer has few obvious symptoms and can easily go undetected for a few years. Among the more noticeable symptoms are a palpable lump in the breast, a change in breast shape and skin appearance or changes to the nipple, such as inversion, a rash or discharge.
A suspicious breast mass may be identified through screening, or via presentation to a general practitioner. Women between the ages of 50 and 70 years are routinely invited to attend regular screening; the NHS is currently in the process of extending the programme as a trial, offering screening to some women aged 47–73 years. A recent case–control study within the NHS England breast screening programme reported that attendance at breast screening resulted in a breast cancer mortality reduction of 39% [odds ratio (OR) 0.61, 95% confidence interval (CI) 0.44 to 0.85] after self-selection correction. 16 Screening increases the proportion of tumours detected in the early, more curable stages.
The breast mass and axillary areas are investigated clinically through palpation and by mammography or ultrasonography, and the status of the tumour is confirmed by histology of a percutaneous tissue biopsy. Staging of the disease depends on tumour size, the number of involved lymph nodes and the presence or absence of distant metastases. Tumour size and axillary metastases can be estimated by clinical examination and imaging techniques, but definitive status is achieved through surgery. Those with small tumours and no axillary metastases have the best prognosis, whereas those with distant metastases are considered incurable. Patients with high-risk early-stage breast cancer also undergo computerised tomography of the chest and abdomen and a bone scan to assess any distant metastases.
Current methods for staging of breast cancer
Three main factors are used to stage breast cancer: (1) tumour size, (2) metastases to the regional lymph nodes and (3) distant metastases. The tumour/node/metastases (TNM) staging system was developed and is maintained by the American Joint Committee on Cancer and the Union for International Cancer Control. 17 The T stage is classified in accordance with the size of the tumour and degree of local infiltration, the N stage is classified in accordance with the number and location of metastases to the lymph nodes in the axilla, between the ribs (internal mammary nodes) and above or below the collarbone (supraclavicular and infraclavicular nodes), and the M stage is classified by the presence of metastases beyond the breast and regional lymph nodes (see Table 56, Appendix 1). Early-stage breast cancer is generally defined as cancer that has not spread beyond the breast or the ipsilateral axillary lymph nodes and is confined to stages I, II or IIIA.
Current service provision
Management of early-stage breast cancer
Patients diagnosed with early-stage breast cancer currently follow the diagnosis/treatment pathway shown in Figure 3.
FIGURE 3.
Diagnosis and management pathway in breast cancer. For postmenopausal women whose tumours are greater than grade 1, many centres also use adjuvant bisphosphonates for up to 3 years. Patients may also be treated with adjuvant radiotherapy depending on whether they have had a wide local excision or mastectomy and depending on the characteristics of the primary tumour; this may include radiotherapy to not only the breast but also the chest wall, supraclavicular fossa and lymph node and axillar. Neoadjuvant treatment may include Pertuzumab (Perjeta®, Roche Products Ltd) and trastuzumab. Adjuvant chemotherapy may be given alongside biological therapy. Reproduced with permission from Ward et al. 1 Contains information licensed under the Non-Commercial Government Licence v2.0. FISH, fluorescence in situ hybridisation.
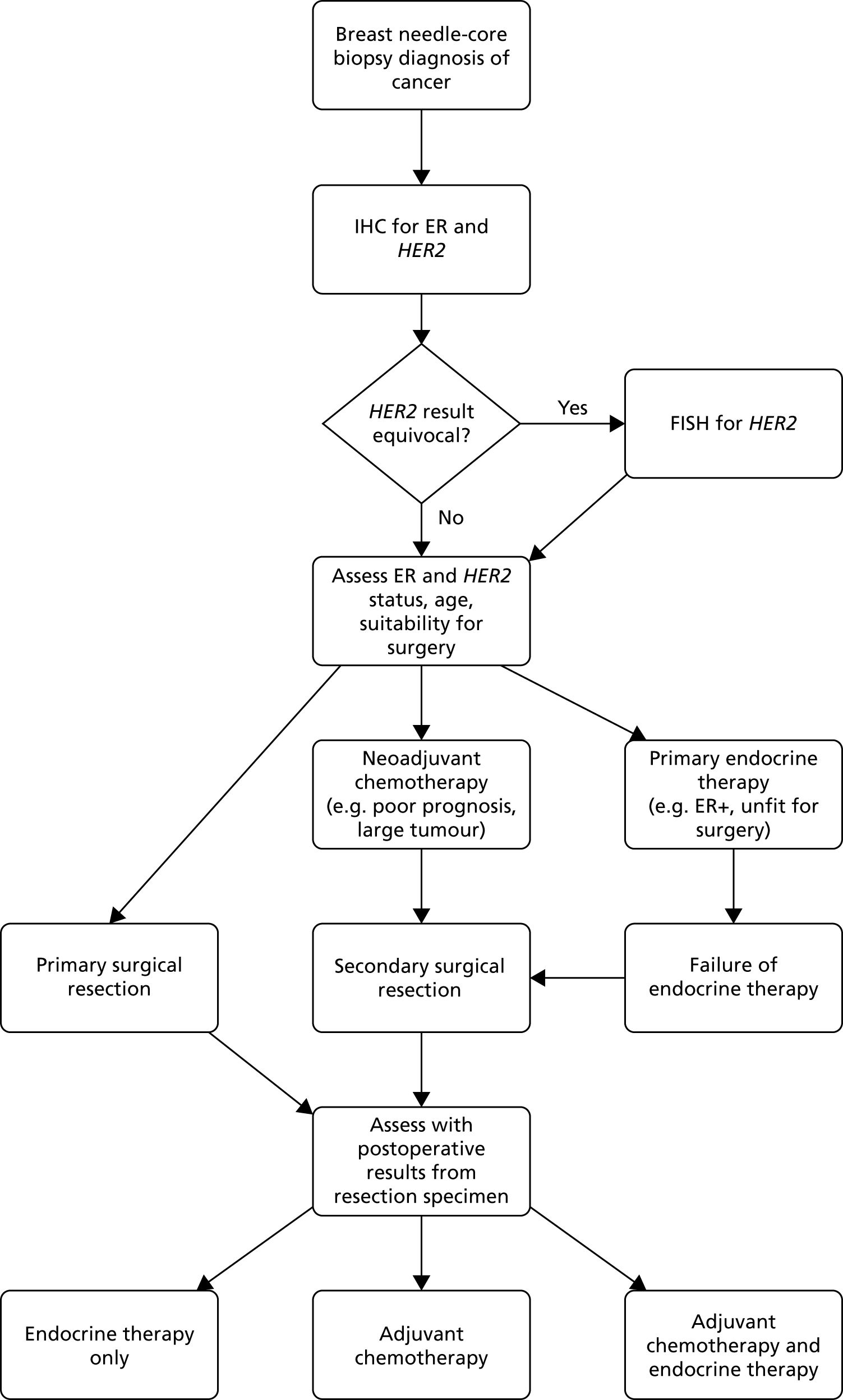
Use of adjuvant chemotherapy
Since 2002, the National Institute for Health and Care Excellence (NICE) has recommended that women at intermediate or high risk of recurrence (ROR) who have not had neoadjuvant chemotherapy should normally be offered a multi-agent chemotherapy that includes anthracyclines. 18 Chemotherapy is defined as the use of cytotoxic medications with the intention of preventing cancer recurrence in patients. It should be noted that, for the purposes of this assessment, chemotherapy does not include other forms of systemic therapy, such as endocrine treatments or targeted biological therapy (e.g. trastuzumab).
Meta-analyses of randomised clinical trials by the Early Breast Cancer Trialists’ Collaborative Group (EBCTCG) have indicated that the use of adjuvant chemotherapy (chemotherapy following surgery) is associated with a reduction in the risk of cancer recurrence and death in women with early-stage breast cancer. 19 However, chemotherapy is associated with considerable adverse events (AEs). Short- and long-term AEs will affect a proportion of patients receiving chemotherapy, imposing additional costs and reducing health-related quality of life (HRQoL). Short-term AEs that happen during chemotherapy are usually temporary and reversible. The most common AEs include nausea, vomiting, mouth soreness, diarrhoea, tiredness, hair loss and temporary lowering of the blood counts. Long-term AEs, such as damage to the heart, and a small increase in the risk of leukaemia are not reversible. Although chemotherapy may prevent relapse in some, not all women with early-stage breast cancer will benefit and many women remain recurrence-free at 10 years without chemotherapy. However, a subset of patients with a ‘good’ prognosis may still develop recurrence after curative surgery and adjuvant therapy. This presents a considerable challenge to clinicians in estimating prognosis and making the most appropriate therapeutic decisions relating to whether or not to use adjuvant chemotherapy in women with early-stage breast cancer.
Improved information on a patient’s ROR (i.e. prognostic risk) and/or likely response to chemotherapy (i.e. predictive benefit) may help target chemotherapy to those patients who will benefit the most. Avoiding chemotherapy in patients at low ROR, who would therefore obtain limited benefit, avoids the unpleasant side effects of chemotherapy and reduces expenditure on both the chemotherapy itself and the treatment of these adverse effects.
Current guidelines
The NICE Clinical Guideline 809 indicates that adjuvant therapy should be considered for all patients with early invasive breast cancer after surgery, based on assessment of the prognostic and predictive factors, the potential benefits and side effects of the treatment. Historically, clinicopathological factors, such as patient age, tumour size, nodal involvement, histological grade, oestrogen receptor (ER) expression, HER2 overexpression and comorbidities, have been assessed and considered alongside patient preference. The NICE guideline9 indicates that decisions regarding adjuvant therapy should be made following discussion of these factors with the patient and recommends consideration of the use of AOL to support estimations of individual prognosis and the absolute benefit of adjuvant treatment for patients with early invasive breast cancer. Although there is variation between centres, the NPI and Predict are also commonly used as the basis for many local guidelines on decisions regarding whether or not to use chemotherapy for patients with early-stage breast cancer. These risk prediction tools include different patient and tumour characteristics and may give different predictions for the same patient (see Table 56, Appendix 1).
The NICE CG809 does not make specific reference to the use of tumour profile tests to aid decision-making. However, the NICE Diagnostics Guidance (DG) 10 on tumour profiling20 recommends oncotype DX as an option for guiding adjuvant chemotherapy decisions for people with ER+, LN0, HER2- early-stage breast cancer at intermediate (clinical) risk if oncotype DX is likely to help in the decision of whether or not to use adjuvant chemotherapy.
Adjuvant! Online
The AOL computer program is designed to provide estimates of the benefits of adjuvant endocrine therapy and chemotherapy. The current version of AOL does not include HER2 status and the potential benefit of trastuzumab. Patient and tumour characteristics are entered into the program and provide an estimate of the baseline risk of mortality or relapse for patients without adjuvant therapy. Information about the efficacy of different therapy options are derived from the EBCTCG meta-analyses in order to provide estimates of reduction in risk at 10 years of breast-cancer-related death or relapse for selected treatments. These estimates are then provided on printed sheets in simple graphical and text formats to be used in consultations. At the time of writing this report (October 2017), AOL was in the process of being updated and was not accessible.
Nottingham Prognostic Index
The NPI is a composite prognostic parameter involving both time-dependent factors and aspects of biological aggressiveness. The NPI score is based on a combination of tumour grade, lymph node involvement and tumour size. To calculate the score, add numerical grade (1, 2 or 3), lymph node score (negative = 1, 1–3 nodes = 2, > 3 nodes = 3) and 0.2 × tumour size in cm. Patients can be divided into three prognostic groups on the basis of the NPI: a good prognosis group (NPI of ≤ 3.4), a moderate prognosis group (3.4 < NPI < 5.4) and a poor prognosis group (NPI of > 5.4).
Predict (version 2.0)
Predict (version 2.0) is an online computer program designed to help women with breast cancer and their doctors make informed decisions about treatment with chemotherapy or endocrine therapy following breast cancer surgery. Predict version 2.0 was developed using data from > 5000 women with breast cancer from England and has been tested on data from another 23,000 women with breast cancer from around the world. Patient and tumour characteristics are entered into the program, which provides an estimate of the overall survival (OS) for patients with or without adjuvant hormone therapy, adjuvant chemotherapy and trastuzumab.
Clinical opinion suggests that there is variation in clinical practice between NHS trusts in the UK, with some centres using single risk prediction tools and others using multiple tools in combination, in addition to other clinical parameters.
Description of technologies under assessment
Tumour profiling tests aim to improve the use of chemotherapy in breast cancer by improving the categorisation of patients in accordance with ROR or death, and by identifying those patients who will gain most benefit from chemotherapy. Tests predicting the ROR in a specific population are likely to be used after surgery, in conjunction with other information available about tumour size, grade, nodal status and other factors to guide the use of adjuvant chemotherapy. Tests that require samples to be sent away for central review, following surgery, may introduce a short delay (of up to 3 weeks) before the decision can be taken on whether or not to offer chemotherapy.
Five tests were identified in the final NICE scope21 and are included in this assessment: four are based on gene expression profiling [EndoPredict® (Myriad Genetics Ltd, London, UK), oncotype DX® (Genomic Health, Inc., Redwood City, CA, USA), MammaPrint® (Agendia, Inc., Amsterdam, the Netherlands) and Prosigna® (NanoString Technologies, Inc., Seattle, WA, USA)] and one is based on immunohistochemistry 4 (IHC4).
Gene expression profiling tests
Gene expression profiling tests investigate the expression of specific panels of genes (also known as gene profiles or gene signatures). They do this by assessing the identity and number of messenger ribonucleic acid (mRNA) transcripts in a specific tissue sample. As only a fraction of the genes encoded in the genome of a cell are expressed by being transcribed into mRNA, gene expression profiling provides information about the activity of genes that give rise to these mRNA transcripts. Given that mRNA molecules are translated into proteins, changes in mRNA levels are ultimately related to changes in the protein composition of the cells, and consequently to changes in the properties and functions of tissues and cells (both normal and malignant) in the body. Gene expression profiling tests work by making use of different techniques to measure mRNA levels in breast cancer specimens including real-time reverse transcription-polymerase chain reaction (RT-PCR) and deoxyribonucleic acid (DNA) microarrays.
There are various ways of preparing the ribonucleic acid (RNA), and different protocols may be used to prepare the specimens [e.g. formalin-fixed, paraffin-embedded (FFPE), snap-frozen and fresh samples]. The tests included in this assessment use FFPE tissue and do not require the use of fresh samples. Furthermore, there are varying algorithms that can be used to combine the raw data to obtain a summary measure. All of these factors can affect the reproducibility and reliability of gene expression profiling tests. These tests provide an estimate of the ROR.
Immunohistochemical-based tests
Immunohistochemistry (IHC) markers are being developed to provide similar information to that given by gene expression profiling tests. Some of these tests offer the advantage of using existing IHC technology (such as ER and HER2 markers) that is routinely available in all UK pathology departments, although methods for quantifying these markers in the format required for the test may not be routinely available.
Summary of tumour profiling tests included in the assessment
The key features of the five tests are summarised in the following sections and in Table 1.
Features | Test | ||||
---|---|---|---|---|---|
EndoPredict (Myriad Genetics) | MammaPrint (Agendia) | Oncotype DX (Genomic Health) | Prosigna (NanoString Technologies) | IHC4 | |
Purpose | Recurrence risk | Recurrence risk and chemotherapy benefit | Recurrence risk and chemotherapy benefit | Recurrence risk and intrinsic subtype | Recurrence risk |
Description | 12-gene assay (eight cancer genes; RT-qPCR) plus clinical factors | 70-gene array (microarray) | 21-gene assay (16 cancer genes; RT-qPCR) | 50-gene assay (50 cancer genes; direct mRNA counting) plus clinical factors | 4 IHC tests (ER, PR, HER2, Ki-67). IHC4+C includes IHC4 plus clinical factors |
Testing location | Local laboratory or test service (Germany) | Test service (the Netherlands) | Test service (USA) | Local laboratory or test service (UK) | Local laboratory |
Stage | Early stage | Early stage (stage I or II) | Early stage (stage I or II) | Early stage (stage I to IIIA) | Early stage |
Lymph node status | LN0 and LN+ (up to three positive) | LN0 or LN+ (up to three positive) | LN0 or LN+ (up to three positive) | LN0 and LN+ | LN0 and LN+ (1 to three positive nodes) |
Hormone receptor status | ER+ | ER+ or ER– | ER+ | ER+ | ER+ |
HER2 status | Negative | Negative or positive | Negative | Negative | Negative or positive |
Menopausal status | Premenopausal and postmenopausal | Premenopausal and postmenopausal | Premenopausal and postmenopausal | Postmenopausal | Postmenopausal |
Test result | Low risk or high risk | Low risk or high risk | Low risk, intermediate risk or high risk |
Low risk, intermediate risk or high risk Intrinsic subtype |
Low risk, intermediate risk or high risk |
Assumptions | Score assumes 5 years of hormonal treatment | Assumes no therapy | Score assumes 5 years of hormonal treatment | Score assumes 5 years of hormonal treatment | Score assumes 5 years of hormonal treatment |
Commercially available in England? | Yes | Yes | Yes | Yes | No |
Cost | £1500 | £2326 (based on conversion from Euros to pounds sterling) | £2580 (excludes PAS) | £1650 (kit cost only) | £202.52 (inflated to 2016 values) |
EndoPredict (Myriad Genetics)
EndoPredict is a Conformité Européenne (CE)-marked assay that is designed to assess the risk of distant recurrence within 10 years of initial diagnosis. The test is intended for use in premenopausal and postmenopausal women with early-stage breast cancer with all of the following clinical features:
-
oestrogen receptor positive
-
human epidermal growth factor receptor 2 negative (HER2–)
-
lymph node negative (no positive nodes) or lymph node positive (LN+) (up to three positive nodes).
EndoPredict measures the expression of 12 genes: three proliferation-associated genes, five hormone receptor-associated genes, three reference (normalisation) genes and one control gene.
EndoPredict requires RNA samples extracted from FFPE breast cancer tissue. The test can be conducted in a local laboratory using a VERSANT® kPCR Amplification Detection Module (Siemens Healthcare Diagnostics Inc, Erlangen, Germany). Alternatively, FFPE samples can be submitted to a Myriad Genetics pathology laboratory in Munich that is accredited by the Deutsche Akkreditierungsstelle, a national accreditation body for Germany.
The test process involves using a reverse transcription-quantitative polymerase chain reaction (RT-qPCR), in which target mRNAs are reverse transcribed, amplified and simultaneously detected. The raw data are then exported to online evaluation software (EndoPredict Report Generator; Myriad Genetics Ltd, London, UK), which performs a quality check and calculates the EndoPredict score and the EndoPredict Clinical (EPClin) score. The EndoPredict score is a number on a scale from 0 to 15, is the molecular score only and is not the final test result. An EndoPredict score of < 5 indicates a low risk of distant disease recurrence in the next 10 years. An EndoPredict score of ≥ 5 indicates a high risk of distant disease recurrence in the next 10 years. The EPClin score is calculated by adding clinical data about tumour size and nodal status to the EndoPredict score. From the EPClin score, the probability of metastasis formation within 10 years is estimated, assuming 5 years of hormonal treatment. The EPClin score (cut-off point of 3.3) provides a single low-/high-risk cut-off point; the threshold was set such that women with a low-risk result (EPClin score of < 3.3) have a < 10% risk of developing distant metastases over the next 10 years. It takes approximately 2 days to obtain the test results if the test is done in-house. If samples are sent away for testing, the turnaround time for the central service is 4 to 5 working days.
MammaPrint (Agendia)
MammaPrint is a CE-marked microarray that is designed to assess the risk of distant recurrence within 5 and 10 years and whether or not a woman would benefit from chemotherapy. The test is intended for use in premenopausal and postmenopausal women with stage I or II breast cancer with the following clinical features:
-
tumour size of ≤ 5 cm
-
lymph node negative or positive (up to three positive nodes).
The test can be used irrespective of ER and HER2 status; that is, it can be used for tumours that are ER negative (ER–) or ER+ and HER2– or human epidermal growth factor receptor 2 positive (HER2+). MammaPrint measures the expression of 70 genes, including genes associated with seven different parts of the metastatic pathway: (1) growth and proliferation, (2) angiogenesis, (3) local invasion, (4) entering the circulation, (5) survival in the circulation, (6) entering organs from the circulation and (7) adaption to the microenvironment at a secondary site. The MammaPrint test is offered as an off-site service. In Europe, samples are sent for analysis at the Agendia laboratory in Amsterdam, the Netherlands. The test requires a FFPE breast cancer tissue sample from a surgical specimen or core needle biopsy.
The test process involves isolation of RNA from a FFPE sample followed by reverse transcription of the mRNA to get complementary DNA (cDNA). The cDNA is amplified and labelled before being hybridised (bound) to the diagnostic microarray. The microarray is washed and then scanned using an Agilent Technologies, Inc. DNA microarray scanner (Santa Clara, CA, USA). The scan file is analysed using Agilent Technologies, Inc. Feature Extraction Software (Santa Clara, CA, USA) and an algorithm is used to calculate the correlation of the sample profile to a ‘low risk’ template profile on a scale of –1.000 to +1.000 with a cut-off point of 0. The threshold was set such that women with a low-risk result have a 10% risk of developing distant metastases over the next 10 years without any adjuvant hormone therapy or chemotherapy. Test results are available to health-care professionals within 10 days of submitting the sample.
Oncotype DX Breast Recurrence Score (Genomic Health)
Oncotype DX is designed to assess the risk of distant recurrence within 10 years and predict the likelihood of benefit from chemotherapy. The test also reports the underlying tumour biology: ER, progesterone receptor (PR) and HER2 status. The test is intended for use in premenopausal and postmenopausal women with stage I or II breast cancer that has the following clinical features:
-
lymph node negative or positive (up to three positive nodes)
-
oestrogen receptor positive
-
human epidermal growth factor receptor 2 negative.
Oncotype DX quantifies the expression of 21 genes. Of these, 16 are cancer-related genes correlated with distant recurrence/relapse-free survival (DRFS) and five are reference genes for normalising the expression of the cancer-related genes. This information is used to calculate the Breast Recurrence Score.
Oncotype DX is offered as a test service to the NHS. Samples are processed centrally at the Genomic Health laboratory in the USA, which is accredited by the American Association for Laboratory Accreditation and the College of American Pathologists. The test requires a FFPE breast cancer tissue sample from a biopsy or surgical resection, which can be sent as a paraffin-embedded block or as 15 unstained charged slides. The test process is based on RT-qPCR. The test gives a recurrence score of between 0 and 100, which is used to quantify the 10-year risk of distant recurrence, assuming 5 years of hormonal (endocrine) therapy. Based on current cut-off points for the oncotype DX test, a score of < 18 indicates low risk of distant recurrence, a score between 18 and 30 indicates intermediate risk and a score of ≥ 31 indicates high-risk. It should be noted that a number of studies, including the ongoing Trial Assigning Individualized Options for Treatment (Rx) (TAILORx) study,22 are testing the use of different cut-off points for oncotype DX. The recurrence score may also predict the benefit of chemotherapy. The oncotype DX results are typically reported within 7–10 days after the sample is received at the laboratory.
The oncotype DX Breast Recurrence Score can be combined with clinical and pathological factors (tumour size, tumour grade and patient age) using the recurrence score–pathology–clinical (RSPC) calculator; however, this calculator has not been validated.
Prosigna (NanoString Technologies)
Prosigna is a CE-marked assay designed to assess DRFS at 10 years. The test is intended for use in postmenopausal women with early-stage breast cancer that is:
-
lymph node negative or positive (up to three positive nodes)
-
oestrogen receptor positive
-
human epidermal growth factor receptor 2 negative.
The test requires RNA extracted from a FFPE breast tumour tissue sample and is done using the nCounter® analysis system (NanoString Technologies, Inc., Seattle, WA, USA). The test process involves fluorescent probe pairs that hybridise to the mRNA; the fluorescence is then detected by the nCounter Digital Analyzer (NanoString Technologies, Inc., Seattle, WA, USA).
Prosigna is based on the Prediction Analysis of Microarray 50 (PAM50) gene signature. 23 It measures the expression levels of 50 genes used to classify patients into one of four breast cancer subtypes. It also measures the expression of eight housekeeping genes used for signal normalisation, six positive controls and eight negative controls. Prosigna classifies samples into the following breast cancer subtypes based on their PAM50 gene expression signatures: luminal A, luminal B, HER2-enriched or basal-like. A ROR score, representing the risk of distant recurrence within 10 years (assuming 5 years of hormonal treatment), is then derived from an algorithm based on the results of the PAM50 gene signature plus clinicopathological factors. Four versions of the ROR score exist in the research setting: (1) ROR based on PAM50 subtype information (ROR-S), (2) ROR-S based on PAM50 information plus proliferation score (ROR-P), (3) ROR-S plus tumour size (ROR-T or ROR-C) and (4) ROR-S plus proliferation score and tumour size [PAM50 subtype call, proliferation score and ROR score (ROR-PT)]. The proliferation score is based on a subset of the PAM50 genes associated with the proliferation pathway.
The Prosigna test uses ROR-PT and therefore includes the PAM50 breast cancer subtype, tumour size and proliferation score. Nodal status is also used in converting the score into a risk category. The ROR score is a numerical score on a scale of 0 to 100. Based on this score and the nodal status, samples are classified into risk categories:
-
node negative: low risk (score of 0–40), intermediate risk (score of 41–60) or high risk (score of 61–100)
-
node positive (up to three positive nodes): low risk (score of 0–15), intermediate risk (score of 16–40) or high risk (score of 41–100).
This assessment includes all studies assessing ROR-PT, whether they use the commercial Prosigna test (using the nCounter system) or other methods (such as RT-qPCR). However, studies assessing ROR-S (subtype), ROR-T/ROR-C (subtype and tumour size) or ROR-P (subtype and proliferation score) are excluded. Studies are also excluded if they only assess PAM50 breast cancer subtypes (luminal A, etc.) rather than ROR-PT score.
IHC4 test
Immunohistochemistry 4 is a laboratory-developed test that combines the results of four IHC-measured parameters. The test can be combined with clinical and pathological features; this is known as IHC4 plus clinical factors (IHC4+C). The test is designed to quantify the risk of distant recurrence in breast cancer patients, assuming 5 years of endocrine therapy. The test is intended for use in postmenopausal women with early-stage breast cancer with the following clinical features:
-
oestrogen receptor positive
-
lymph node negative or positive (up to three positive nodes).
The components of the test are four immunohistochemical assays: ER, PR, HER2 and the proliferation marker Ki-67. The IHC4 test is currently used within the Royal Marsden Breast Cancer Unit service, but it has been suggested that the test could be run in local NHS laboratories if quality assurance programmes for the individual assays were in place. IHC4 uses FFPE breast tumour tissue samples and IHC techniques that are universally available in NHS pathology departments. ER and HER2 markers are commonly measured in NHS laboratories, although methods for quantifying these markers in the format required for the test may not be routinely available. Although PR and Ki-67 markers are not routinely measured in breast tumour tissue samples, the assays are commonly available for use if needed. The quantitative assessment of Ki-67 required for the IHC4 test is not currently conducted in most NHS laboratories and, therefore, further training for pathologists and biomedical scientists is likely to be needed.
The IHC4+C test involves an algorithm that calculates a risk score for distant recurrence based on the results of the four IHC assays and clinical factors including age, nodal status, tumour size and tumour grade. The algorithm has been published and validated24,25 and is freely available, and a calculator is available for use on request. A distant recurrence score of < 10% is categorised as low risk for distant recurrence at 10 years, a score of ≥ 10% but < 20% is categorised as intermediate risk and a score of ≥ 20% is categorised as high risk for distant recurrence at 10 years. At the Royal Marsden NHS Foundation Trust, the test is processed with an average estimated turnaround time of 1 week; however, results may be made available in 2 working days if they are urgently required.
Current usage of tumour profiling tests in the NHS
A previous systematic review and economic evaluation (Ward et al. 1) published in 2013 considered the clinical effectiveness and cost-effectiveness of tumour profiling tests compared with current prognostic tools in guiding the use of adjuvant chemotherapy in people with early-stage breast cancer in England and Wales. This report informed the NICE decision to approve the use of oncotype DX as an option for guiding adjuvant chemotherapy decisions for people with ER+, LN0, HER2– early-stage breast cancer assessed to be at intermediate ROR of breast cancer after surgery. The use of the other tumour profiling tests in the NHS remains limited (mainly to clinical trial use).
Description of the decision problem
This assessment aims to assess whether or not tumour profiling tests used for guiding adjuvant chemotherapy decisions for people with early-stage breast cancer represent a clinically effective and cost-effective use of NHS resources.
Interventions
The following tumour profiling tests are included, in combination with current decision-making:
-
EndoPredict and EPClin
-
MammaPrint
-
oncotype DX Breast Recurrence Score and oncotype DX breast RSPC
-
Prosigna (or ROR-PT, which is equivalent)
-
IHC4 and IHC4+C.
Comparators
The comparator for the assessment is standard UK practice for chemotherapy decision-making, which may include any tool, or clinical and pathological features, used to assess risk. Clinicopathological tools used in current practice include Predict, NPI and AOL. The use of these tools varies between centres.
Population and important subgroups
The intended population for the assessment relates to people with ER+ (and/or PR-positive), HER2–, early-stage breast cancer (stages I or II) with zero to three positive lymph nodes (LN0–3).
In practice, it was anticipated that many potentially relevant studies would include a broader population. Therefore, all relevant studies of early-stage breast cancer were eligible for inclusion, and the findings are interpreted with reference to how closely the study population matched the intended population.
The following subgroups are considered within this assessment:
-
people with LN0 cancer, people with micrometastases in the lymph nodes and people with one to three positive lymph nodes (LN1–3)
-
premenopausal and postmenopausal women
-
people predicted to be at low, intermediate or high risk using a risk assessment tool or using clinical and pathological features
-
males and females
-
people of different ethnicities.
These tests will only have an impact on the health of patients if they lead to changes in patient management. This is most likely to happen in populations in which the decision of whether or not to offer chemotherapy is currently uncertain. One such group is patients with ER+, LN0, HER2– early-stage breast cancer for whom prognostic variables suggest that they are at intermediate risk. The definition of this ‘intermediate group’ is not clear cut. Clinical advice suggests that patients with a NPI of ≤ 3.4 are typically considered at low risk either using current prognostic tools (except for a few very young women with aggressive early-stage breast cancer) or based on the new tests and are unlikely to receive chemotherapy; therefore, their management is unlikely to change. Few patients with ER+, LN0, HER2– early-stage breast cancer will have a NPI of > 5.4 and, therefore, those with a NPI of > 3.4 can be considered as being at intermediate risk. Some LN+ patients may also be considered to be at intermediate risk.
Current treatment protocols indicate that women with HER2+, ER– early-stage breast cancer or with more than three positive nodes are likely to receive chemotherapy in most centres in England. Although the use of tumour profiling tests might be able to spare a proportion of these patients from chemotherapy, the evidence base for the use of these tests in this population is more limited and clinical opinion, therefore, considered the assessment of these tests in this population to be a lower priority; this population was therefore excluded from the NICE scope. Currently, patients with micrometastases who are clinically managed as LN0 patients are excluded from NHS-funded testing using oncotype DX, even if they fall within the intermediate-risk group. Patients with micrometastases are included in the NICE scope.
Patients with ER+, HER2– early-stage breast cancer, who either are LN0 or have one to three positive nodes, are therefore considered to be an important population in which to assess these tests, given the current evidence base. The scope therefore focuses on the ER+, HER2– population with zero to three lymph nodes. Within this population, an important subgroup consists of patients at clinically intermediate risk for whom the decision about whether or not to offer chemotherapy is not clear cut.
Outcomes
The clinical effectiveness review considers the clinical effectiveness of the tests in relation to the following broad categories (these are described further in Chapter 2, Methods, which also lists the relevant study designs for each outcome):
-
Analytic validity (i.e. the ability of the test to accurately and reliably measure the expression of mRNA or proteins by breast cancer tumour cells). Owing to time constraints, it was not possible to conduct a full review of analytic validity for all tests. A rapid review of IHC4 was conducted.
-
Prognostic ability (i.e. the degree to which the test could accurately predict the risk of an outcome such as disease recurrence and discriminate patients with different outcomes).
-
Prediction of chemotherapy benefit (i.e. whether or not the effect of chemotherapy vs. no chemotherapy on patient outcomes differs between test risk groups).
-
Clinical utility (this is defined differently throughout the prognostic literature); here, we define clinical utility studies as those that assess the ability of the test to affect patient outcomes (e.g. recurrence and survival) through the prospective use of the test to guide treatment decisions.
-
Decision impact (i.e. how the tests influence decision-making in terms of which patients will be offered or administered chemotherapy; this design does not include follow-up of clinical outcomes such as recurrence or survival). The review included only UK and European studies because chemotherapy rates differ widely between European and non-European countries.
-
Health-related quality of life and anxiety.
-
Time-to-test results.
Assessment of the above outcomes involves making comparisons (between study groups or between risk groups for the test) in terms of clinical patient outcomes such as recurrence and survival. Key clinical outcomes included for this purpose are listed in Chapter 2, Methods.
The outcomes of interest for the economic evaluation are the morbidity and mortality associated with invasive breast cancer and its treatment. Outcomes from the model are expressed in terms of the incremental cost per quality-adjusted life-year (QALY) gained.
Aims and objectives of the assessment
The overall aim of the assessment is to address the question ‘Do tumour profiling tests used for guiding adjuvant chemotherapy decision in patients with early-stage breast cancer represent a clinically effective and cost-effective use of NHS resources?’. This includes an update of the systematic review and cost-effectiveness analysis1 undertaken to inform NICE DG10. 20
The objectives of the assessment are to:
-
conduct a systematic review of the published evidence on the effectiveness and cost-effectiveness of the five tumour profiling tests
-
develop a health economic model to assess the cost-effectiveness associated with the use of tumour profiling tests compared with current prognostic tools to guide the use of adjuvant chemotherapy in early-stage breast cancer from the perspective of the NHS and Personal Social Services (PSS).
Chapter 2 Clinical evidence
A systematic review was undertaken to assess the effectiveness of tumour profiling tests for guiding adjuvant chemotherapy decisions in early-stage breast cancer. The methods of the systematic review are described in Methods. The results of the review are reported in Results.
Methods
This report contains reference to confidential information provided as part of the NICE appraisal process. This information has been removed from the report and the results, discussions and conclusions of the report do not include the confidential information. These sections are clearly marked in the report.
A registered protocol of this systematic review (CRD42017059561) is available on the PROSPERO website at www.crd.york.ac.uk/prospero/display_record.asp?ID=CRD42017059561 (accessed 10 May 2018). The review was conducted following the general principles recommended in the Centre for Reviews and Dissemination (CRD)’s guidance,26 in the Preferred Reporting Items for Systematic Reviews and Meta-Analyses (PRISMA) statement,27 in the NICE Diagnostic Assessment Programme manual28 and by the Cochrane Prognosis Methods Group. 29
The protocol included a mapping stage, following which minor amendments were made to the inclusion criteria and review methods in consultation with NICE and clinical advisors in order to focus the evidence review to studies of most relevance to the decision problem.
Identification of studies
This systematic review search provided an update to the previous systematic review (by Ward et al. 1) conducted for NICE DG10. 20 The search strategy was adapted to retrieve clinical studies and systematic reviews of five tumour profiling tests (with or without clinicopathological factors) in early-stage breast cancer management: EndoPredict, oncotype DX, MammaPrint, IHC4 and Prosigna.
The search approach involved:
-
searching of electronic databases
-
contact with experts in the field
-
scrutiny of bibliographies of retrieved papers
-
identification of relevant studies from the previous review by Ward et al. 1 conducted for NICE DG1020 (see below)
-
references included within the evidence dossiers provided by the manufacturers to NICE.
Electronic database searches
The search strategy comprised medical subject headings (MeSHs) or Emtree thesaurus terms and free-text synonyms for ‘breast cancer’ combined with the individually named tumour profiling tests. Searches were translated across databases and were not limited by language. Searches for oncotype DX, MammaPrint, IHC and Prosigna were limited by publication date from 2011 (the search date in the review by Ward et al. ,1 because these tests were included in this review), whereas no date limits were applied to EndoPredict (as it was not included in the review by Ward et al. 1).
The search strategies are presented in Appendix 2. Literature searching was undertaken in February 2017 in the following electronic databases and trials registries:
-
MEDLINE Epub Ahead of Print, In-Process & Other Non-Indexed Citations (via Ovid): 1946 to present.
-
EMBASE (via Ovid): 1974 to 24 February 2017.
-
Cochrane Database of Systematic Reviews (via Wiley Interscience): 1996 to present.
-
Database of Abstracts of Reviews of Effects (via Wiley Interscience): 1995 to 2015 (until close of database).
-
Cochrane Central Register of Controlled Trials (via Wiley Interscience): 1995 to present.
-
Health Technology Assessment (HTA) Database (via Wiley Interscience): 1995 to 2016 (until close of database).
-
NHS Economic Evaluation Database (via Wiley Interscience): 1995 to 2015 (until close of database).
-
Science Citation Index Expanded (via Web of Science): 1900 to present.
-
Conference Proceedings Citation Index – Science (via Web of Science): 1990 to present.
-
World Health Organization International Clinical Trials Registry Platform (http://apps.who.int/trialsearch/; accessed 19 January 2017) (no date limit applied).
-
American Society of Clinical Oncology (ASCO) (via Web of Science) (date range searched: 2011–17).
-
European Society for Medical Oncology (via Web of Science) (date range searched: 2011–17).
Supplementary searches
References of relevant systematic reviews, primary studies and company submissions were checked to identify additional studies.
Inclusion and exclusion criteria
The inclusion and exclusion criteria for selecting studies are described in the following sections.
Population and setting
The intended population included people with ER+ (and/or PR-positive), HER2–, early-stage breast cancer (stages I or II) with LN0–3.
In practice, it was anticipated that many potentially relevant studies would include a broader population. Therefore, all relevant studies of early-stage breast cancer were eligible for inclusion. When subgroups were reported for the intended population (described above), these were used in the assessment. When no subgroups were reported, the study was included and the findings were interpreted with reference to how closely the study population matched the intended population.
The following subgroups were considered within this assessment:
-
people with LN0 cancer, people with micrometastases in the lymph nodes and people with LN1–3
-
premenopausal and postmenopausal women
-
people predicted to be at low, intermediate or high risk using a risk assessment tool or using clinical and pathological features
-
males and females
-
people of different ethnicities.
This assessment focuses on the use of tumour profiling tests to guide decisions about adjuvant chemotherapy. The use of these tests to guide endocrine therapy decisions, or decisions about neoadjuvant chemotherapy (to shrink the tumour before surgery), was not evaluated.
Interventions
The following tumour profiling tests were included:
-
EndoPredict and EPClin
-
MammaPrint
-
oncotype DX Breast Recurrence Score and oncotype DX Breast RSPC
-
Prosigna (or ROR-PT, which is equivalent)
-
IHC4 and IHC4+C.
Commercial versus in silico tests
Studies were included if they assessed the commercially available versions of the tests. For IHC4, as there is no commercially available version of the test, any methodology was included. In addition, some studies used in silico (electronic database) versions of tests using publicly available genetic databases, usually based on whole-genome-expression microarray data. Owing to uncertainty about their similarity to the commercially available tests, these studies were analysed separately. It was beyond the scope of the review to ascertain the quality of the methods used or the degree of overlap between cohorts for these in silico studies.
Comparators
The comparator for the assessment is standard UK practice for chemotherapy decision-making. This was taken to include combinations of clinicopathological factors (e.g. within multivariable models), plus clinicopathological risk tools used in the UK, including Predict, the NPI and AOL. The clinical treatment score (CTS), a combination of commonly used clinicopathological variables, was also included as a comparator even though it is not commonly used in practice as a tool, because it is used in a number of key studies and includes a set of variables that are used in practice.
Other non-UK local or national guidelines, such as St Gallen and the National Comprehensive Cancer Network (NCCN) guidelines, were excluded when a study also reported comparisons with Predict, NPI or AOL, but were otherwise included.
Relevant comparators within individual studies varied in accordance with the study type:
-
Studies assessing prognostic performance. No comparator is needed as the aim is to compare outcomes between risks groups for the test being studied.
-
Studies assessing prediction of chemotherapy benefit. No comparator is needed as the aim is to compare the effect of chemotherapy between risks groups for the test being studied.
-
Clinical utility studies. The relevant comparator is standard clinical practice as defined in the first paragraph of this section.
-
Decision impact studies. The relevant comparator is standard clinical practice as above (for pre-test chemotherapy decisions).
Outcomes and study designs
The clinical effectiveness review considers the clinical effectiveness of the tests in relation to the following broad categories:
-
Analytic validity (i.e. the ability of the test to accurately and reliably measure the expression of mRNA or proteins by breast cancer tumour cells). Owing to time constraints, it was not possible to conduct a full review of analytic validity for all tests. A rapid review of IHC4 was conducted.
-
Prognostic performance (i.e. the degree to which the test can accurately predict the risk of an outcome such as disease recurrence and discriminate patients with different outcomes). This is usually assessed by conducting the test on stored tumour samples for which longer-term patient outcome data are available, but when the test did not influence treatment. Study designs include –
-
Reanalysis of randomised controlled trial (RCT) data.
-
Analysis of prospective or retrospective cohorts in which the test did not influence treatment.
-
-
Prediction of chemotherapy benefit (i.e. whether or not the effect of chemotherapy vs. no chemotherapy on patient outcomes differs between test risk groups). This is usually assessed by conducting the test on stored tumour samples for which longer-term outcome data are available. Study designs include –
-
Reanalysis of RCTs in which some patients received chemotherapy and some did not.
-
Analysis of prospective or retrospective cohorts in which some patients received chemotherapy and some did not. These could include cohorts in which the test did or did not influence practice.
-
-
The difference between absolute and relative benefit should be noted: for a test that is prognostic, a difference in absolute benefit of chemotherapy between groups would be expected, whereas for a test to be considered predictive of chemotherapy benefit, a difference in relative benefit should be seen. As an example, if distant recurrence rates in the test high-risk group were 30% without chemotherapy and 20% with chemotherapy, the absolute benefit of chemotherapy would be 10%. Likewise, if distant recurrence rates in the test low-risk group were 3% without chemotherapy and 2% with chemotherapy, the absolute benefit of chemotherapy would be 1% (i.e. much smaller). However, the relative benefit would be the same in both groups [relative risk (RR) of 0.67, i.e. chemotherapy reduces recurrence by one-third]. If the test is predictive of chemotherapy benefit, the RR would be expected to be different in different risk groups.
-
Clinical utility: this is defined differently throughout the prognostic literature. Here, we define clinical utility studies as those that assess the ability of the test to affect patient outcomes (such as recurrence and survival) through the prospective use of the test to guide treatment decisions (the study may be prospective or retrospective, but use of the test should have been prospective, i.e. used in clinical practice rather than conducted on stored tumour samples). Study designs include –
-
RCTs randomising patients to chemotherapy guided by the test or guided by a comparator (e.g. clinical practice).
-
Observational studies reporting clinical outcomes for patients whose treatment was guided by the test. As these studies do not have a comparator, we are primarily interested in outcomes for patients with low-risk disease, who, as a group, have mostly avoided chemotherapy. The observation of good outcomes in this group could, alongside other evidence, support the avoidance of chemotherapy in this group.
-
-
Decision impact (i.e. how the tests influence decision-making in terms of which patients will be offered chemotherapy). Clinical advice to the External Assessment Group (EAG) suggests that chemotherapy rates differ between countries, with lower rates in the UK and Europe than in the USA. The review therefore included only UK and European studies. Study designs include –
-
Studies assessing change in chemotherapy recommendations and/or decisions before and after use of the test (this design does not include follow-up of clinical outcomes such as recurrence or survival).
-
-
Health-related quality of life and anxiety. Study designs include –
-
Studies assessing impact of the test versus usual practice on HRQoL and anxiety.
-
Studies assessing HRQoL and anxiety before and after test use.
-
-
Time-to-test results: studies assessing the time taken to obtain test results.
-
Concordance: concordance is defined in this review as the degree to which tests assign the same patients to the same risk groups. Such studies do not report long-term outcomes. A full systematic review of studies that only assess concordance between tests (with no patient outcome data) was beyond the scope of this assessment. However, the Optimal Personalised Treatment of early breast cancer usIng Multi-parameter Analysis preliminary (OPTIMA Prelim) study30 was included as a key example of concordance between tests.
Clinical patient outcomes
Assessment of clinical utility, prognostic ability and prediction of chemotherapy benefit involves making comparisons (between study groups or between risk groups for the test) in terms of clinical patient outcomes. Key clinical outcomes included for this purpose are listed below. Standard definitions for breast cancer outcomes, defined by Hudis et al. ,31 are given below, although these are not always consistently or clearly defined in study reports. Within this review, DRFS and distant recurrence/relapse-free interval (DRFI) have been combined in some sections where insufficient detail was provided in study reports to distinguish between them. The standard definitions for breast cancer outcomes are:
-
distant recurrence/relapse-free survival, also referred to as distant metastasis-free survival (DMFS) or distant disease-free survival (DFS) – events include distant recurrence and death from any cause
-
distant recurrence/relapse-free interval, also referred to as distant metastasis-free interval (DMFI) – events include distant recurrence and death from breast cancer
-
recurrence/relapse-free survival (RFS) – events include ipsilateral, locoregional or distant invasive recurrence and death from any cause [not contralateral disease, non-breast cancers or ductal carcinoma in situ (DCIS)]
-
recurrence/relapse-free interval (RFI) – events include ipsilateral, locoregional or distant recurrence and death from breast cancer (not contralateral disease, non-breast cancers or DCIS)
-
invasive disease-free survival (IDFS) – events include ipsilateral, locoregional or distant invasive recurrence, contralateral and non-breast cancers, and death from any cause (not DCIS)
-
disease-free survival – events include ipsilateral, locoregional or distant recurrence, DCIS, contralateral or non-breast cancers, and death from any cause
-
breast-cancer-specific survival (BCSS) – events include breast cancer death only
-
overall survival – events include death from any cause only
-
disease-related morbidity and mortality
-
chemotherapy-related morbidity and mortality.
For the above clinical outcomes, studies were only included if follow-up was ≥ 5 years for survival outcomes (OS and BCSS) or ≥ 3 years for other outcomes.
The following outcomes were excluded:
-
locoregional recurrence (i.e. within the region of the original tumour), because chemotherapy decisions will mainly impact distant recurrence and survival
-
clinician confidence and patient decisional conflict relating to decision impact of the test (this is beyond the scope of this assessment)
-
prediction of benefit from one type of chemotherapy versus another (the assessment is restricted to benefit of chemotherapy vs. no chemotherapy).
Studies not published in the English language were included if sufficient PICOS (population, intervention, comparator, outcome, study design) data could be extracted from non-English-language full-texts or from an existing English language abstract. Non-peer-reviewed reports or abstracts were only included if the data were presented in a succinct and accessible manner (e.g. a manuscript prepared for submission to a journal), if sufficient methodological details were reported to allow critical appraisal of the study quality and if results were reported in sufficient detail.
Study selection process
All records retrieved from the search were exported into a reference management database [EndNote version X7; Clarivate Analytics (formerly Thomson Reuters), Philadelphia, PA, USA]. After deduplication, titles/abstracts were assessed for relevance, followed by examination of full texts of potentially includable studies. Study selection was conducted by one reviewer, with discussion between two reviewers for any studies giving rise to uncertainty. A 10% sample was checked by a second reviewer early in the process to ensure mutual understanding of study inclusion and to correct if necessary.
Data extraction
A data extraction form was constructed in Microsoft Excel® (Microsoft Corporation, Redmond, WA, USA) and piloted using two examples of each study design. Data were extracted by one reviewer and checked by a second reviewer. Disagreements were resolved by discussion. Study authors were contacted for any missing or ambiguous data when time allowed. When multiple publications related to the same patient cohort, or when pooled analyses were identified, the references selected for inclusion were those that provided the most complete follow-up and the most useful clinical outcomes (DRFS or DRFI were preferred based on clinical advice and use in the health economic model; see Chapter 3), avoiding double-counting of patients/cohorts when possible.
Quality assessment
The methodological quality of included studies was assessed using quality assessment tools relevant to the study design. Quality assessment was undertaken by one reviewer and checked by a second reviewer. The quality and design of studies was considered within the narrative synthesis of results.
For clinical utility studies (for which the highest level of evidence is a RCT of the test vs. usual practice), quality was assessed using the Cochrane risk-of-bias tool for RCTs. 32
For studies assessing prognostic ability and prediction of chemotherapy benefit, quality was assessed using relevant criteria selected from the draft Prediction model study Risk Of Bias ASsessment Tool (PROBAST) (Dr Robert Wolff, Kleijnen Systematic Reviews Ltd, January 2017, personal communication). The PROBAST tool has been developed specifically for use in systematic reviews of prediction models by the Cochrane Prognosis Methods Group,29 but is not yet validated or published. Criteria were selected on the basis of relevance to this review. Table 2 shows the quality criteria used in this assessment and how they were scored.
Number | Criterion | Scoring |
---|---|---|
Risk-of-bias questions | ||
1 | Is the study design appropriate? | Yes (prognosis): reanalysis of RCT or cohort or nested case control AND patients did not receive chemotherapy |
Yes (predicting chemotherapy benefit): RCT or reanalysis of RCT | ||
No (prognostic): non-nested case control or case series AND/OR some/all patients had chemotherapy | ||
No (predicting chemotherapy benefit): patients not randomised to chemotherapy vs. no chemotherapy | ||
2 | Are all eligible patients included? | Yes: all eligible patients from trial or consecutive eligible patients from prospective registry |
No: some eligible patients excluded (e.g. not sent for testing, insufficient tissue, test failures, missing data, AND/OR non-prospective registry) | ||
Unclear: if unclear | ||
4 | Were test assessors blinded to clinical outcomes? | Yes: blinded |
No: not blinded | ||
Unclear: if unclear | ||
6 | Was the outcome definition standardised or defined a priori? | Yes: reported outcomes that were standardised (e.g. DRFS, OS) or defined a priori |
No: outcomes non-standardised and not defined a priori | ||
Unclear: if either item unclear | ||
Applicability questions | ||
3 | Does the patient spectrum match the review question? | Yes: all patients in scope (HR+, HER2–, LN0–3) |
Mostly: < 20% out of scope | ||
No: > 20% out of scope | ||
Unclear: if unclear | ||
5 | Is the test as per the decision problem? | Yes: same as commercially available tests or IHC4 conducted as per Cuzick et al.25 |
No: different from commercially available tests (e.g. FFPE vs. fresh samples, test methods) |
Studies assessing decision impact, analytic validity and HRQoL/anxiety were not quality assessed owing to time constraints.
Data presentation and synthesis
Data were summarised and presented as tabular and narrative syntheses. Meta-analysis was not considered appropriate owing to significant heterogeneity between studies. Interpretation of the evidence base was conducted with reference to published hierarchies for predictive studies33–35 and with regard to the ability of the study design to adequately address the decision problem. Interpretation of results also considered how closely the study population matched the intended population, the methodological quality of the studies and the treatment received by patients (in terms of endocrine therapy and chemotherapy).
Results
Quantity of evidence
Figure 4 is the PRISMA flow diagram for study selection. The database searches and searches of other sources identified a total of 2330 unique references to screen. Of these, 1797 were excluded at the title/abstract stage and 533 full-text articles were screened, of which 380 were excluded (reasons are listed in Appendix 3). In total, 153 references were included in the assessment.
FIGURE 4.
The PRISMA flow diagram.
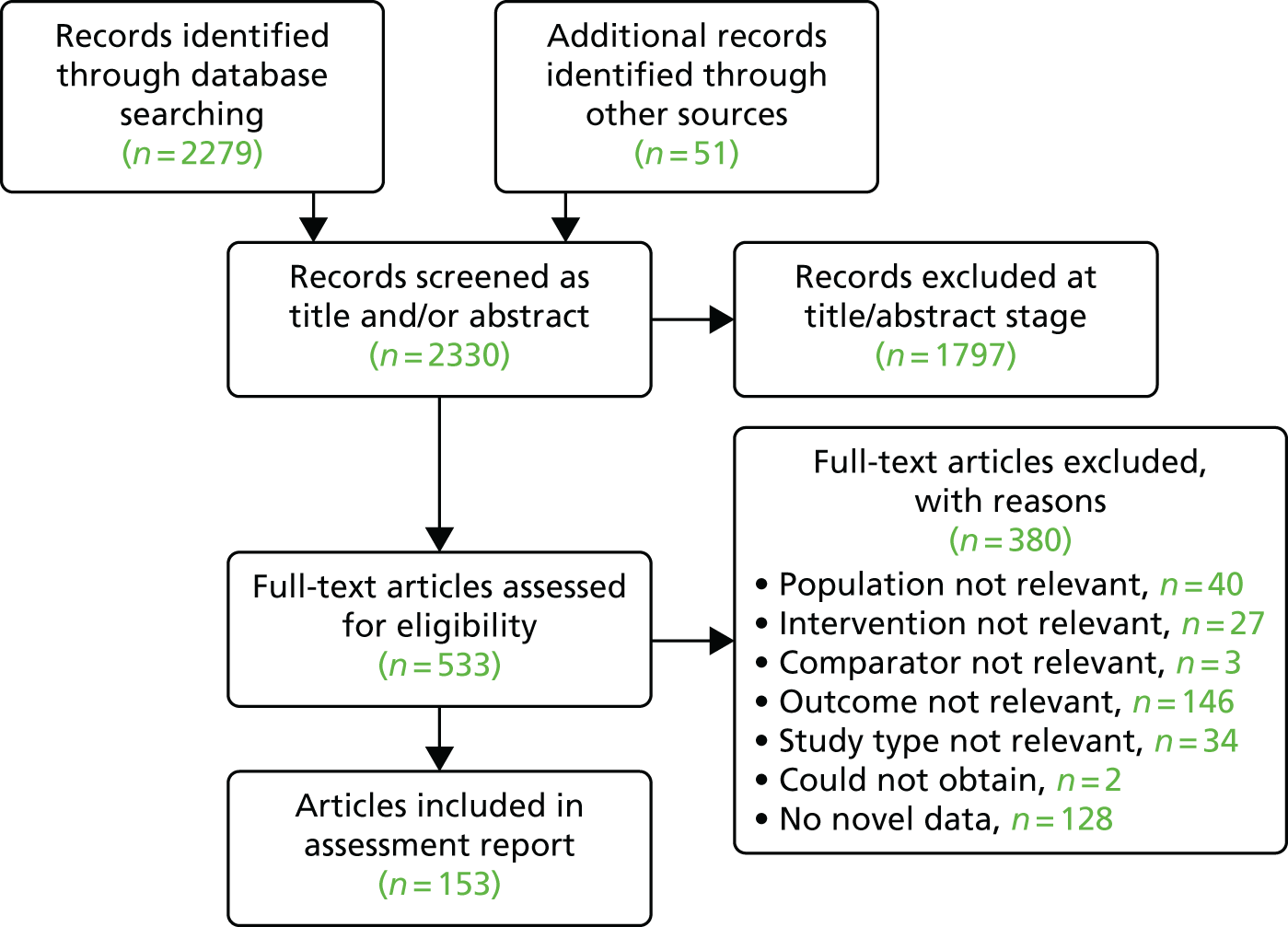
There are numerous Translational substudies of the Arimidex, Tamoxifen, Alone or in Combination (TransATAC) publications that met the criteria for the review. 25,36–44 ATAC45 was an international trial, which evaluated anastrozole alone, tamoxifen alone, and the combination of both treatments in breast cancer patients. Recruitment ended in 2006. TransATAC was a series of translational studies utilising samples and data from the ATAC trial. Throughout the report we present data provided by the TransATAC team as personal communications (Ivana Sestak, Queen Mary University of London, July 2017, personal communication) to the EAG, provided on request from the EAG, which restricts to UK patients in the tamoxifen arm, with hormone receptor-positive (HR+), HER2– and LN0–3 patients. 46 Subsequently, a very similar analysis was published by the TransATAC team. 47 Some concerns were expressed during the NICE consultation about the suitability of the TransATAC bespoke analysis and its comparability with other sources of data, and these are addressed in Report Supplementary Material 1.
Overview of results
To orientate the reader to the broad sweep of the evidence and to facilitate interpretation of the detailed evidence base, a summary of the results is provided in Appendix 4. A more complete summary of DRFS/DRFI/DFS data for each test is then provided in section Results: oncotypeDX through to Time-to-test results, with more detailed narrative syntheses (including outcomes OS and BCSS) in Report Supplementary Material 2–10. We have separated the evidence into the following broad categories:
-
Development – a description of the development of the test. A full review of analytic validity was not possible owing to time constraints. A rapid review of IHC4 was conducted.
-
Prognostic performance – studies reporting on the ability of the test to predict ROR and/or survival. The most commonly reported data are Kaplan–Meier estimates of risk of outcome per test risk group and hazard ratios (HRs) between groups, although a small number of studies report C-index data [which, in this case, are identical to the area under the curve (AUC)] and likelihood ratios. In keeping with the majority of studies, we first present unadjusted data, and separately report analyses (usually multivariable Cox proportional hazards models) that adjust for clinicopathological factors, which show whether or not the test potentially has prognostic value over clinicopathological variables. The C-index is a measure of the goodness of fit of a model with binary outcomes (in this case, it is identical to the AUC). A value of < 0.5 indicates a poor model, a value of 0.5 indicates that the model is no better than chance, a value of > 0.7 indicates a good model, a value of > 0.8 indicates a strong model and a value of 1 indicates a perfect model. 48
-
Chemotherapy benefit – studies in this category compare treatment benefit across risk categories, and most commonly reanalyse RCT data in which patients were randomised to chemotherapy or no chemotherapy, and conduct a test for the interaction between treatment and tumour profiling test risk group. The interaction test tells us whether or not the tumour profiling test is able to predict a differential treatment effect by risk group. We have also included any observational studies that report treatment benefit across risk categories, with or without interaction tests, with appropriate caveats about the possibility of confounding in such studies. Note that the difference between absolute benefit and relative benefit, as described in Data extraction, is of critical importance.
-
Clinical utility – studies reporting the impact on patient outcomes (such as recurrence and survival) of the prospective use of the test to guide adjuvant chemotherapy treatment decisions. Ideally, such studies would randomise patients to treatment guided by the test or to treatment guided by usual clinical practice; however, given the paucity of RCT evidence, the inherent ethical issues with randomising all patients to chemotherapy and issues with powering such studies, observational studies have also been included in this section.
-
Decision impact – studies that report the impact of test results on actual chemotherapy decisions or recommendations. Such studies do not report long-term follow-up of patients.
There were no data available for clinical utility for Prosigna, EndoPredict or IHC4. Chemotherapy benefit only applies to MammaPrint and oncotype DX, as only these tests claim to be able to identify patients who will benefit from chemotherapy, rather than just those patients who are at high risk of relapse. For this reason, the clinical review comprises the following main sections in Chapter 2, each with a number of relevant subheadings:
-
Overview of results.
-
Results: oncotype DX (additional data in Report Supplementary Material 2–4).
-
Development: oncotype DX.
-
Prognostic performance: oncotype DX.
-
Chemotherapy benefit: oncotype DX.
-
Clinical utility: oncotype DX.
-
-
Results: MammaPrint (additional data in Report Supplementary Material 5).
-
Development: MammaPrint.
-
Prognostic performance: MammaPrint.
-
Chemotherapy benefit: MammaPrint.
-
Clinical utility: MammaPrint.
-
-
Results: Prosigna (additional data in Report Supplementary Material 6).
-
Prognostic performance: Prosigna.
-
-
Results: EndoPredict and EndoPredict Clinical (additional data in Report Supplementary Material 7).
-
Development: EndoPredict and EndoPredict Clinical.
-
Prognostic performance: EndoPredict and EndoPredict Clinical.
-
-
Results: IHC4 (additional data in Report Supplementary Material 8).
-
Development and analytic validity: IHC4.
-
Prognostic performance: IHC4 and IHC4+C.
-
-
Results: decision impact studies (additional data in Report Supplementary Material 9).
-
Anxiety and health-related quality of life.
-
Time-to-test results.
Additional sections that appear in Report Supplementary Material are:
-
Report Supplementary Material 1: Comparison of TransATAC data to other study data (risk classification and prognosis).
-
Report Supplementary Material 8: Narrative synthesis and additional tables for IHC4 analytical validity.
-
Appendix 5: results: all tests compared with each other:
-
studies reporting more than one test
-
microarray studies
-
concordance.
-
Results: oncotype DX
Development: oncotype DX
See Report Supplementary Material 2 for a description of the development of oncotype DX.
Prognostic performance: oncotype DX
Study characteristics: oncotype DX
Oncotype DX was validated in 11 distinct data sets. Seven were reanalyses of RCTs [the National Surgical Adjuvant Breast and Bowel Project (NSABP) B14,49 B2050 and B2851,52 trials; the Southwest Oncology Group (SWOG) trial 8814;53 the Eastern Cooperative Oncology Group E219754,55 trial; UK patients from the TransATAC37,46 trial; and a French trial, PACS0156] and four were retrospective cohort studies (one from the USA,57 two from China58,59 and one from Japan60). All studies recruited patients with either ER+ or HR+ tumours, but only TransATAC37,46 and one Chinese study58 recruited or reported a subgroup of exclusively HER2– patients. Two studies (TransATAC37,46 and the SWOG 8814 trial)53 recruited only postmenopausal patients.
The test was designed to predict outcomes in patients who received 5 years of endocrine therapy without chemotherapy. Only three studies treated patients with endocrine monotherapy, although it was not always clear if this was for 5 years; of these, one study recruited mixed lymph node status patients and reported separate analyses for LN0 and LN+ patients,37,46 one recruited LN0 patients49 and one recruited LN+ patients. 53 Of the remaining studies, one treated some patients with endocrine monotherapy and some with endocrine therapy and chemotherapy (LN0 patients50), two treated all patients with endocrine therapy and chemotherapy (one study recruited mixed lymph node status patients54,55 and one recruited LN+ patients51,52) and one treated all patients with chemotherapy and some with endocrine therapy (LN+ patients). 56 The retrospective studies treated patients in accordance with usual practice (without oncotype DX) with varying levels of endocrine therapy and chemotherapy. 57–60 The total number of patients included was 4929. A detailed narrative synthesis of study characteristics is provided in Report Supplementary Material 2. Study characteristics data are presented in Report Supplementary Material 2, Table 1.
Two studies did not report how oncotype DX was conducted (PACS01 study56 and Russell et al. 57). In all but three other cases, the test was conducted on fixed, paraffin-embedded tissue by Genomic Health using the commercial oncotype DX assay. The three exceptions were the two studies from China in which the test was not carried out by Genomic Health58,59 and the study by Paik et al. ,49 as Paik et al. (2006)50 described the assay used in Paik et al. (2004)49 as being ‘a preliminary version of the RT-PCR assay (lacking standardized reagents, calibrators, and controls)’. In these three studies, the equivalence of the tests to the commercially offered oncotype DX assay is unknown.
Quality assessment: oncotype DX
Quality assessment is summarised in Report Supplementary Material 2. All studies were validation studies. Only three studies37,46,49,53 used an appropriate study design, as eight50–52,54–59 included patients who had been treated with chemotherapy or did not report the proportion of patients treated with chemotherapy. Undertreatment with endocrine therapy and overtreatment with chemotherapy both have the potential to affect recurrence and may alter the observed HRs for outcomes between risk groups. No studies included all eligible patients and only three37,46,53,54 stated that they blinded test assessors to patient outcomes. A lack of blinding is likely to have a low impact as the test is objective. There are concerns about patient spectrum bias in all studies, mainly owing to the retrospective nature of the studies and the exclusion of tumour samples with insufficient tissue probably leading to the loss of patients with smaller tumours. The potential loss of patients with small tumours is of unknown concern, as it is unknown whether or not the test would have a different prognostic performance in these patients.
Results: oncotype DX
The following is a summary of key results from the review. A detailed narrative synthesis of all study results is provided in Report Supplementary Material 2.
Distribution of patients by risk group: oncotype DX
Data are presented in Table 3. The proportion of patients classified as being at low risk ranged from 48%60 to 64%46 in LN0 cohorts and was generally lower, ranging from 36%51,52 to 57%,46 in LN+ cohorts. The proportion of patients who were classified as being at intermediate risk ranged from 20%60 to 27%46 in LN0 cohorts, and was generally higher in LN+ cohorts, ranging from 30%56 to 34%. 51,52 The proportion of patients who were classified as being at high risk ranged from 9% to 33% in LN0 patients and was similar among LN+ patients, ranging from 11% to 32%. The number of patients who are likely to be prescribed chemotherapy on the basis of their test results will, to a large extent, depend on how intermediate-risk patients are handled and whether or not they would be handled in the same way in the LN0 and LN+ groups.
Reference (first author and year) | Cohorts | Population | Nodal status | ET/chemotherapy | Cut-off points | Percentage of patients per group | DRFS/DRFI/DFS: % risk of outcome (95% CI) | DRFS/DRFI/DFS: HR (95% CI) | ||||||||
---|---|---|---|---|---|---|---|---|---|---|---|---|---|---|---|---|
0–5 years | 0–10 years | |||||||||||||||
Low | Intermediate | High | Low | Intermediate | High | Low | Intermediate | High | 0–5 years | 0–10 years | ||||||
DRFSa | ||||||||||||||||
LN0, variable ET and chemotherapy | ||||||||||||||||
Gong 201658 (n = 153) | SYSMH, CCSYU and 3rd HNC | 100% HR+, 100% HER2– | LN0 | 100% ET; 79% chemotherapy | NR | 49 | 26 | 25 | 0–5 years:
|
|||||||
DRFI | ||||||||||||||||
LN0/+ | ||||||||||||||||
Variable ET and chemotherapy | ||||||||||||||||
Sun 201159 (n = 93)a | HAAMMS | 100% HR+, 86% HER2– | LN+/LN0 | LN+/LN0, 75.3% ET, 80.6% chemotherapy | 18–30 | 37 | 31 | 32 | 5.5 years median follow-up
|
5.5 years median follow-up, RS 50-point Difference: 2.35 (1.58 to 3.49); p < 0.001 | ||||||
LN0 | ||||||||||||||||
100% ET monotherapy | ||||||||||||||||
Paik 2004,49 Tang 201161 and Wolmark 201652 (n = 668) | NSABP B-14 | 100% ER+, HER2+/HER2–, % NR | LN0 | 100% ET | 18–30 | 51 | 22 | 27 | 97.9 (95.6 to 99.0)b | 90.8 (84.7 to 94.5)b | 77.9 (71.1 to 83.4)b | 93.2 (90.4 to 96.0) | 85.7 (79.7 to 91.7) | 69.5 (62.6 to 76.4) | RS 50-point difference: 6.04 (3.88 to 9.41); p < 0.001b |
Intermediate vs. low: 2.21 (1.28 to 3.81) High vs. low: 3.8 (2.36 to 6.1); p < 0.001 |
Log rank p < 0.001b | p < 0.001 high vs. low | |||||||||||||||
5–10 years: 95.2 (92.1 to 97.2)b | 5–10 years: 94.4 (88.0 to 97.5)b | 5–10 years: 89.2 (82.4 to 93.4)b | 5–10 years: RS 50-point difference – 1.55 (0.81 to 2.97); p = 0.20b | |||||||||||||
Log rank p = 0.06b high vs. low | ||||||||||||||||
5–15 years: 93.3 (89.6 to 95.7)b | 5–15 years: 88.1 (79.9 to 93.1)b | 5–15 years: 86.4 (79.0 to 91.3)b | ||||||||||||||
Toi 201060 (n = 200) | 8 Japanese hospitals | 100% ER+, HER2 NR | LN0 | 100% ET | 18–30 | 48 | 20 | 33 | 96.7 (90.0 to 98.9) | 100 (NR) | 75.2 (62.2 to 84.3) | 50-point increase: 6.20 (2.27 to 17.0); p < 0.001 | ||||
p < 0.001 log rank (low vs. high) | ||||||||||||||||
Sestak 201746 (data request) and Dowsett 201037 (n = 829) | TransATAC | 100% HR+, 100% HER2–, postmenopausal | LN0 | 100% ET | 18–30 | 64 | 27 | 9 | 99.1 (NR) | 94 (NR) | 88.9 (NR) | 94.9 (NR) | 87.7 (NR) | 77.2 (NR) |
Intermediate vs. low: 6.37 (2.27 to 17.87) High vs. low: 12.39 (4.05 to 37.89) |
Intermediate vs. low: 2.67 (1.53 to 4.68) High vs. low: 5.43 (2.84 to 10.35) |
100% ET+ chemotherapy | ||||||||||||||||
Paik 200650 (n = 424) | NSABP B-20 | 100% ER+, HER2+/HER2– | LN0 | 100% ET and chemotherapy | 18–30 | 51 | 21 | 28 | 95.6 (92.7 to 98.6) | 89.1 (82.4 to 95.9) | 88.1 (82.0 to 94.2) | |||||
Variable ET and chemotherapy | ||||||||||||||||
Sun 201159 (n = 57)a | HAAMMS | 100% HR+, 86% HER2– | LN0 | 75.3% ET, 80.6% chemotherapy | 18–30 | – | – | – | 84.4 (77.2 to 91.6) | 72.6 (62.1 to 83.1) | 41.7 (27.5 to 55.9) | 57.9 (41.4 to 74.4) | 43.0 (23.7 to 62.3) | 20.8 (4.4 to 37.2) | ||
p = 0.02 | ||||||||||||||||
LN+ | ||||||||||||||||
100% ET monotherapy | ||||||||||||||||
Sestak 201746 (data request) and Dowsett 201037 (n = 219) | TransATAC | 100% HR+, 100% HER2–, postmenopausal | LN1–3 | 100% ET | 18–30 | 57 | 32 | 11 | 95.9 (NR) | 84.8 (NR) | 83.6 (NR) | 81.8 (NR) | 75.4 (NR) | 68.6 (NR) |
Intermediate vs. low: 3.84 (1.31 to 11.23) High vs. low: 4.45 (1.19 to 16.58) |
Intermediate vs. low: 1.66 (0.86 to 3.23) High vs. low: 2.35 (0.99 to 5.60) |
Variable ET and chemotherapy | ||||||||||||||||
Wolmark 201652 and Mamounas 201251 (n = 1065) | NSABP-28 | 100% ER+, HER2 NR | LN+ | 100% chemotherapy and ET | 18–30 | 36 | 34 | 30 | 91.6 (88.3 to 94.0) | 81.2 (76.8 to 84.9) | 70.3 (64.9 to 75.1) | 80.9 (76.4 to 84.6)c | 64.9 (59.6 to 69.7)c | 55.8 (50.0 to 61.2)c | RS 50-point difference: 4.22 (2.93 to 6.07); p < 0.001 | |
Log rank p < 0.001 | p < 0.001 | |||||||||||||||
5–10 years: 88.3 (84.3 to 91.4) | 5–10 years: 79.9 (74.7 to 84.2) | 5–10 years: 79.3 (73.1 to 84.3) | 5–10 years, RS 50-point Difference: 1.66 (1.05 to 2.61); p = 0.04 | |||||||||||||
Log rank p = 0.02 | ||||||||||||||||
Penault-Llorca 201456 (n = 530) | PACS01 | 100% HR+ | LN+ | 100% chemotherapy, 74.2% ET | NR | 39 | 30 | 31 | 93.7 (89.4 to 96.3) | 87.3 (81.0 to 91.6) | 69.3 (61.5 to 75.8) | p < 0.001 | 7.7 years median follow-up, RS 50-point difference: 4.1 (CI NR); p < 0.001 | |||
Sun 201159 (n = 35)a | HAAMMS | 100% HR+, 86% HER2– | LN+ | LN+/LN0, 75.3% ET, 80.6% chemotherapy | 18–30 | 62.5 (45.4 to 79.6) | 66.7 (51.0 to 82.4) | 16.7 (7.9 to 25.5) | 62.5 (45.4 to 79.6) | 33.3 (8.5 to 58.1) | 16.7 (7.9 to 25.5) | |||||
p = 0.038 | ||||||||||||||||
DFSd | ||||||||||||||||
LN+ | ||||||||||||||||
100% ET monotherapy | ||||||||||||||||
Albain 201053 (n = 148) | SWOG-8814 | 100% HR+, 91% HER2–, postmenopausal | LN+, 100% LN > 3, 37% | 100% ET | 18–30 | 37 | 31 | 32 | – | – | – | 60 | 49 | 43 | RS 50-point difference: 2.64 (1.33 to 5.27); p = 0.006) |
Assumption of proportional hazards not met (p = 0.0016) 0–5 years: HR 5.55 (2.32 to 3.28); p = 0.0002 5–10 years: HR 0.86 (0.27 to 2.74); p = 0.80 |
Variable ET and chemotherapy | ||||||||||||||||
Penault-Llorca 201456 (n = 530) | PACS01 | 100% HR+ | LN+ | 100% chemotherapy, 74.2% ET | NR | 39 | 30 | 31 | 90.8 (86.0 to 94.1) | 84.9 (78.3 to 89.6) | 64.6 (56.7 to 71.4) | 7.7 years median follow-up, RS 50-point difference: 3.3 (CI NR); p < 0.001 | ||||
p < 0.001 | ||||||||||||||||
Wolmark 201652 and Mamounas 201251 (n = 1065) | NSABP-28 | 100% ER+, HER2 NR, menopausal status NR, female NR | LN+ | 100% chemotherapy and ET | 18–30 | 36 | 34 | 30 | 75.8 (71.1 to 79.8) | 57.0 (51.6 to 61.9) | 48.0 (42.3 to 53.4) | |||||
p < 0.001 | ||||||||||||||||
LN status NR | ||||||||||||||||
ET and chemotherapy NR | ||||||||||||||||
Russell 201657 (n = 135) | University of South Florida and Morton Plan Hospital | 100% ER+, HER2– NR, menopausal status NR, female NR | NR | NR – usual practice guided by MammaPrint | NR | 53 | 26 | 21 | 4.5 years median follow-up: Mantel–Cox log-rank test
|
Prognostic performance, unadjusted analyses: oncotype DX
Data are presented in Table 3. The 10-year DRFI rates in low-risk LN0 patients treated with endocrine monotherapy ranged from 93% to 97% (three studies46,49,60), and was similar when 100% patients received endocrine therapy and chemotherapy (96%, one study50). LN+ patients had much lower 10-year DRFI rates: 82% (one study46) with endocrine monotherapy and 81% (one study51,52) when 100% of patients received endocrine therapy and chemotherapy. The 10-year DRFI rates in LN0 intermediate-risk patients treated with endocrine monotherapy ranged from 86% to 100% (three studies46,49,60), and was similar when 100% of patients received endocrine therapy and chemotherapy (89%, one study50). LN+ intermediate-risk patients had much lower 10-year DRFI rates: 75% (one study46) with endocrine monotherapy and 65% (one study51,52) when 100% of patients received endocrine therapy and chemotherapy. The 10-year DRFI rates in LN0 high-risk patients treated with endocrine monotherapy ranged from 70% to 77% (three studies46,49,60), and was higher when 100% of patients received endocrine therapy and chemotherapy (88%, one study50). LN+ high-risk patients treated with endocrine monotherapy had similar 10-year DRFI rates to LN0 patients, at 69% (one study46); in studies in which all patients received endocrine therapy and some or all received chemotherapy, the DRFI was lower, at 56% (one study51,52). All the DRFI rates in this paragraph exclude one study from China, which appeared an outlier with very low DRFI rates (Sun et al. 59). The study from Japan60 also reported some unusual results in that intermediate-risk patients had better DRFI than low-risk patients (e.g. 10-year DRFI rate was 97% and 100%, respectively). It is unclear if, for both of these studies,59,60 the unusual results are due to small sample sizes (n = 9859 and n = 20060), differences in treatment practices in Japan and China compared with Western countries or differences in ethnicity.
Despite confounding from treatment, the studies reporting prognostic performance data reported largely statistically significant differences between high-risk and low-risk groups, whether through HRs or through analyses of event rates per group, and this was the case regardless of lymph node status. However, differences between the intermediate-risk group and the high- or low-risk groups were not always statistically significant, particularly in the LN+ population (see Table 3).
Two studies reported a C-index (AUC). One study54,55 was in LN+/LN0 patients and the other was in LN0 patients. 58 In both cases, the C-index was 0.69, which indicated that the model was better than chance at placing patients into appropriate risk categories and nearly reaches the cut-off point for a ‘good’ test of 0.7. 48
Data for OS, BCSS, RFS and RFI can be found in Report Supplementary Material 2. These data support observations for DRFS, DRFI and DFS.
Additional prognostic value, adjusted analyses: oncotype DX
Data are presented in Table 4. The analyses that reported multivariable Cox proportional hazards models that were adjusted for clinicopathological variables generally indicated that the prognostic performance of oncotype DX had additional benefit over these factors, as HRs remained significant in most analyses (the exception being RFS and OS analyses by Toi et al. 60). This was consistent regardless of lymph node status and variables adjusted for, which included age, tumour size and LN status (when applicable) in all cases and grade in most cases (Toi et al. 60 and Sun et al. 59 being the exceptions). However, covariates included in multivariate analyses varied, and it is not clear if all important covariates were included in all analyses. The use of the 50-point difference in the adjusted analyses of prognostic performance indicated that the recurrence score may be prognostic over clinicopathological factors, but does not provide information about the clinical significance of the 18–30 recurrence score cut-off points.
Reference (first author and year) | Cohorts | Population | Nodal status | ET/chemotherapy | Cut-off point | Outcome | Test or comparatora | Multivariable Cox proportional hazards model (adjusted for clinicopathological factorsa): HR (95% CI) unless otherwise stated | |
---|---|---|---|---|---|---|---|---|---|
LN+/LN0, 100% ET and chemotherapy | |||||||||
Goldstein 200854 and Sparano 201255 (n = 465) | E2197 (ECOG trial) | 100% HR+, 44% HER2–, premenopausal/postmenopausal |
LN0, 56.5% LN1–3, 43.5% |
All ET and chemotherapy, 40% aromatase inhibitor | 18–30 | RFI | Oncotype DX vs. clinicopathological factorsa |
5 year:54 HR (RS 50-point difference): 2.12 (0.97 to 4.65, p = 0.06)b 3.13 (1.60 to 6.14, p = 0.0009)b |
10 year55 HR (RS 50-point difference): 2.27 (1.04 to 4.97, p NR) |
Oncotype DX vs. integrator based on AOLb | 5 year:54 for 50-point difference using central grade:
|
||||||||
100% HR+, 100% HER2– | NR | 5 year:54 C-index (AUC):
|
|||||||
Sestak 2017 (data request)46 and Dowsett 201037 (n = 774) | TransATAC | 100% HR+, 100% HER2–, postmenopausal | LN+/LN0 | 100% ET | 18–30 | DRFI | Increase in likelihood ratio χ2 over comparator | ||
Oncotype DX vs. CTS | 10 year: 15.22 (p = 0.0001) | ||||||||
Oncotype DX vs. NPI | 10 year: 11.89 (p = 0.0006) | ||||||||
LN0, 100% ET monotherapy | |||||||||
Paik 200449 and Tang 201161 (n = 668) | NSABP B-14 | 100% ER+, HER2+/HER2–, % NR | LN0 | 100% ET | 18–30 | DRFI | Increase in likelihood ratio χ2 over clinical factorsa or comparator | Multivariable Cox proportional hazards model (adjusted for clinicopathological factorsa) | |
Oncotype DX vs. clinicopathological factorsa |
|
RS 50-point difference: Cox proportional hazards model 1: 3.21 (2.23 to 4.61) p < 0.001 Cox proportional hazards model 2: 2.81 (1.70 to 4.64) p < 0.001 Cox proportional hazards model 3:c 2.34 (1.56 to 3.5); p < 0.001 |
|||||||
DRFI | Multivariable Cox proportional hazards model (adjusted for clinicopathological factorsa) | ||||||||
Oncotype DX vs. AOLd | Cox proportional hazards model 4 (only AOL and RS):
|
||||||||
Oncotype DX vs. AOL and clinicopathological factorsb | Cox proportional hazards model 5 (AOL, RS, age, tumour size, grade):
|
||||||||
Toi 201060 (n = 200) | 8 Japanese hospitals (unnamed) | 100% ER+, HER2 NR, menopausal status NR, % female NR | LN0 | 100% ET | 18–30 | DRFI | Oncotype DX vs. clinicopathological factorsa | RS 50-point difference: 6.03 (2.17 to 16.7); p < 0.001 | |
RFI | RS 50-point difference: 3.38 (1.32 to 8.69) | ||||||||
RFS | RS 50-point difference: 2.09 (0.84 to 5.20) | ||||||||
OS | RS 50-point difference: 2.67 (0.93 to 7.62) | ||||||||
Sestak 2017 (data request)46 and Dowsett 201037 (n = 591) | TransATAC | 100% HR+, 100% HER2–, postmenopausal | LN0 | 100% ET | 18–30 | DRFI | Increase in likelihood ratio χ2 over comparator | ||
Oncotype DX vs. CTS | 5 year: 10.03 (p = 0.002) | 10 year: 10.64 (p = 0.001) | |||||||
Oncotype DX vs. NPI | 5 year: 9.62 (p = 0.002) | 10 year: 8.82 (p = 0.003) | |||||||
LN0, variable ET and chemotherapy | |||||||||
Sun 201159 (n = 57) | HAAMMS | 100% HR+, 86% HER2– (7.5% unclear) | LN0 | 75.3% ET, 80.6% chemotherapy | 18–30 | DRFS | RS (not Genomic Health) vs. clinicopathological factorsa | RS 1-point difference: 1.03 (1.01 to 1.06); p = 0.017 | |
LN+, variable ET and chemotherapy | |||||||||
Penault-Llorca 201456 (n = 530) | PACS01 | 100% HR+ | LN+ | 100% chemotherapy, 74.2% ET | NR | NR, assume DRFI | Oncotype DX vs. clinicopathological factorsa | p < 0.001 | |
Sun 201159 (n = 35) | HAAMMS | 100% HR+, 86% HER2– (7.5% unclear) | LN+ | 75.3% ET, 80.6% chemotherapy | 18–30 | DRFS | RS (not Genomic Health) | RS 1-point difference: 1.03 (1.00 to 1.07); p = 0.039 | |
Sestak 2017 (data request)46 and Dowsett 201037 (n = 183) | TransATAC | 100% HR+, 100% HER2–, postmenopausal | LN+ | 100% ET | 18–30 | DRFI | Increase in likelihood ratio χ2 over comparator | ||
Oncotype DX vs. CTS | 5 year: 3.29 (p = 0.07) | 10 year: 3.56 (p = 0.06) | |||||||
Oncotype DX vs. NPI | 5 year: 2.47 (p = 0.1) | 10 year: 2.14 (p = 0.1) | |||||||
Wolmark 201652 and Mamounas 201251 (n = 1065) | NSABP-28 | 100% ER+, HER2 NR, menopausal status NR, female NR | LN+ | 100% chemotherapy and ET | 18–30 | DFS, DRFI, OS | Oncotype DX vs. clinicopathological factorsa | p < 0.001 |
The likelihood ratio χ2 in the TransATAC data set was statistically significantly higher for oncotype DX than for CTS or NPI only for LN0 patients [10-year DRFI likelihood ratio χ2 10.64 (p = 0.001) and 8.82 (p = 0.003), respectively], while the difference was not statistically significant for LN+ patients [3.56 (p = 0.06) and 2.14 (p = 0.1), respectively]. 46 Compared with AOL, and with a model based on AOL but with 5-year outcomes, oncotype DX also appeared to provide additional prognostic information (see Table 4). 49,61
Oncotype DX RSPC
Data are presented in Table 5. One study (Tang et al. 44) derived the RSPC score in a meta-analysis of NSABP B-14 and TransATAC (LN+/LN0; n = 1735), and performed a limited validation in NSABP B-20 (LN0; n = 625), which included 233 patients used to derive the oncotype DX recurrence score. For this reason, both NSABP B-14 and B-20 data were used to derive part of the algorithm. Based on the NSABP B-14 analysis set, the oncotype DX RSPC algorithm (oncotype DX plus age, tumour size and grade) appeared to provide additional prognostic information over oncotype DX and over clinicopathological variables, and was able to classify more patients into a low-risk category than oncotype DX while maintaining a roughly equivalent rate of distant recurrence in the low-risk group. In the NSABP B-20 cohort, RSPC had prognostic value in a univariate analysis.
Reference (first author and year) | Cohorts | Population | Nodal status | ET/chemotherapy | Cut-off point | Outcome | Test | % of patients per group | Discrimination | Reclassification | Additional prognostic value, difference in likelihood ratio χ2 | |||||
---|---|---|---|---|---|---|---|---|---|---|---|---|---|---|---|---|
% 10-year DRFI (95% CI) | HR, p-value | |||||||||||||||
Low risk | Intermediate risk | High risk | Low risk | Intermediate risk | High risk | |||||||||||
Node negative and node positive | ||||||||||||||||
Tang 201144
|
NSABP, B-14 and TransATAC meta-analysed | ER+, HER2+/ HER2–, % NR | LN0 (B-14); LN+/LN0 (TransATAC) | 100% ET | 12–20% risk | DRFI 10 years | RSPC | 64 | 18 | 18 | 93.5 (91.5 to 95.5) | 82.4 (77.1 to 87.7) | 73.8 (68.4 to 79.2) | HR/CI NR, p < 0.001 with increasing risk group |
RSPC vs. RS: DRFI risks not significantly different between RS and RSPC within each risk group (p = 0.68, p = 0.27 and p = 0.42 for low-, intermediate- and high-risk groups) RS intermediate-risk patients (n = 272):RS low-risk patients (n = 783):RS high-risk patients (n NR): |
RSPC vs. oncotype DX RS: 76.9, p < 0.001 RSPC vs. grade, tumour size, age: 45.4, p < 0.001 |
RS | 54 | 27 | 19 | 94.1 (92.2 to 96.0) | 86.2 (81.9 to 90.5) | 70.5 (63.4 to 76.5) | HR/CI NR, p < 0.001 with increasing risk group | |||||||||
NSABP and B-20 | ER+, HER2+/ HER2–, % NR | LN0 | 100% ET; 64% chemotherapy | RSPC |
RSPC: 2.43, p < 0.001 RS: 2.22, p < 0.001 |
Conclusion: oncotype DX and RSPC prognostic performance
Seven reanalyses of RCTs and four retrospective cohort studies were included, with a total of 4929 patients. The generalisability of the evidence base to the decision problem is uncertain owing to the loss of patients with insufficient tumour samples. Generally, when comparing LN0 patients with LN+ patients, similar numbers were at high risk, but more were at low risk in LN0 cohorts, and more at intermediate risk in LN+ cohorts. How many patients would be prescribed chemotherapy would depend in large part on how intermediate patients are handled. The 10-year DRFI rates suggest that patients in the LN0 low-risk group46,49,60 are at very low ROR (10-year DRFI range 93–97%) in the absence of chemotherapy, and patients in the intermediate risk group may be at somewhat higher risk (10-year DRFI range 86–100%). In the LN+ study using endocrine monotherapy, patients were generally at higher risk of recurrence than LN0 patients in both the low and the intermediate categories (10-year DRFI < 85% and ≤ 75%, respectively). Unadjusted analyses indicated that oncotype DX had prognostic power (statistically significant differences between low-risk and high-risk groups) across various recurrence outcomes, regardless of lymph node status. HRs between the intermediate-risk group and the high- or low-risk groups were not always statistically significant. Oncotype DX provided additional prognostic information over the most commonly used clinicopathological variables (age, grade, size and nodal status) regardless of lymph node status (although it was not always clear if all relevant variables had been included in the analyses), and over CTS and NPI in LN0 (but not LN+) patients. On the basis of proportions classified as low risk and DRFI rates, RSPC may outperform oncotype DX in LN0 patients, but these data are from the derivation cohort, with only limited validation data from a cohort (NSABP B-20) that included patients who were used to derive one of its constituent parts (oncotype DX Breast Recurrence Score), and it has not been tested in premenopausal or LN+ patients.
Chemotherapy benefit: oncotype DX
Chemotherapy benefit relates to the ability of the test to predict which patients will respond to chemotherapy, and can be assessed by considering whether or not the effect of chemotherapy versus no chemotherapy on patient outcomes differs in accordance with the test score (e.g. by comparing HRs or p-values between risk groups). Formal assessments of chemotherapy benefit include interaction tests that assess whether or not the difference is statistically significant. The results of the review are summarised here, and a full narrative synthesis is provided in Report Supplementary Material 3.
Study design and patient characteristics
Five data sets, reported across 10 published references44,50,53,61,64–69 and one academic-in-confidence (AiC) manuscript (see Report Supplementary Material 3, Table 3), have conducted analyses that assess the ability of oncotype DX to predict the benefit of chemotherapy. Two were reanalyses of RCTs: the American (NSABP) B-20 study (n = 651) in LN0 was reported by Paik et al. ,50 Tang et al. 61 and Tang et al. ;44 and the American SWOG 8814 study (n = 367) in LN+ patients (38% had four or more lymph nodes) was reported by Albain et al. 53 In both trials, patients were randomised to tamoxifen only, or tamoxifen plus cyclophosphamide. NSABP-B20 did not select participants by HER2 status, but an analysis of HER2– patients was provided as a personal communication (Professor Tang via NICE, University of Pittsburgh, personal communication, February 2018) as part of the NICE assessment process. SWOG-881453 recruited patients with any HER2 status (12% were HER2+) and all patients were postmenopausal. Both reanalyses had high attrition rates (72% and 60%, respectively), owing to missing clinical variables, missing samples, insufficient tissue and test failures.
The three remaining studies were observational64–67,70 (total approximately 44,000 with some double-counting). Two LN0 studies were from the USA [MD Anderson Center, n = 1424;64,65 Surveillance, Epidemiology, and End Results (SEER) registry, n = 40,13467] and one study recruiting a mix of LN+ and LN0 patients was from Israel [Clalit Health Services one lymph node micrometastasis (LN1micro)–LN3, n = 620; LN0–LN1micro, n = 159466]. Additional analyses were provided to the EAG as AiC data but cannot be reported here. Patients were treated in accordance with local routine practice in conjunction with their oncotype DX score.
Quality assessment
Report Supplementary Material 3, Table 4, presents the quality assessment of the included studies. Among the RCTs, neither trial scored well on every item. The key concerns included the use of the derivation cohort in Paik et al. 50/Tang et al. 61 and high attrition rates in both trials. The comparison of baseline characteristics between patients included in the analysis and those excluded from the analysis showed differences in patient age at recruitment (Paik et al. 49) and tumour grade. (Paik et al. 50).
The three observational studies64–67,69 are limited by their non-randomised design, whereby patients who received chemotherapy are likely to be systematically different in terms of known (and potentially unknown) prognostic variables (e.g. age) and treatment effect modifiers to those who did not, leading to a high risk of confounding. They also only recruited patients for whom an oncotype DX test had been ordered and it is unclear how this may have affected the patient spectrum and generalisability to the decision problem. However, owing to their prospective use of the test in clinical practice, three studies blinded the test assessors to the long-term outcomes. 64–69
Results
Both RCT reanalysis studies showed that unadjusted HRs for the effect of chemotherapy versus no chemotherapy on survival and recurrence outcomes were most favourable in the higher-risk groups. HRs were generally statistically significant in high-risk groups but not in low- or intermediate-risk groups. In the B20 study (LN0 patients),44,50,61 unadjusted HRs for 10-year DRFI in the low-, intermediate- and high-risk groups were 1.31, 0.61 and 0.26, respectively. HRs restricted to HER2– patients (adjusted and unadjusted) showed the same pattern (Table 6; Professor Tang, personal communication). However, it is interesting to note that absolute differences (for chemotherapy vs. no chemotherapy) were very small in the low- and intermediate-risk groups (1.1% and 1.8%, respectively, both favouring no chemotherapy), although they were greater in the high-risk group (27.6% favouring chemotherapy).
Study | Outcome | % recurrence free, absolute benefit | HR for chemotherapy vs. no chemotherapy (95% CI) | Interaction tests | |||||
---|---|---|---|---|---|---|---|---|---|
Low | Intermediate | High | Low | Intermediate | High | Unadjusted | Adjusted | ||
NSABP-B20: LN0, ER+ (n = 651) Paik 2006,50 Tang 201161 and Professor Tang, personal communication |
10-year DRFI unadjusted, HER2– unadjusted, HER2– adjusted a |
Chemotherapy: 95.6% No chemotherapy: 96.8% Absolute difference: 1.1% |
Chemotherapy: 89.1% No chemotherapy: 90.9% Absolute difference: 1.8% |
Chemotherapy: 88.1% No chemotherapy: 60.5% Absolute difference: 27.6% |
1.31 (0.46 to 3.78); p = 0.61 1.21 (0.41 to 3.55); p = 0.73 1.18 (0.40 to 3.53); p = 0.76a |
0.61 (0.24 to 1.59); p = 0.39 0.78 (0.29 to 2.11); p = 0.62 0.67 (0.24 to 1.87); p = 0.44a |
0.26 (0.13 to 0.53); p < 0.001 0.21 (0.08 to 0.53); p < 0.001 0.20 (0.07 to 0.52); p = 0.001 a |
Interaction (continuous RS) p = 0.031 or p = 0.038 (Tang 201161 and Paik 200650) | Interactiona (continuous RS) adjusted for age, tumour size, grade, ER and PR: |
10-year DFS | 0.91 (0.57 to 1.45) | 0.79 (0.43 to 1.47) | 0.41 (0.23 to 0.71) | p = 0.082 | |||||
10-year OS | 1.37 (0.63 to 3.01) | 0.94 (0.4 to 2.25) | 0.31 (0.16 to 0.60) | p = 0.011 | |||||
SWOG-8814: LN+, HR+, HER2+/HER2– (n = 367) Albain 201053 |
10-year DFS |
Chemotherapy: 64% No chemotherapy: 60% Absolute difference: 4% |
Chemotherapy: 55% No chemotherapy: 43% Absolute difference: 12% |
1.02 (0.54 to 1.93); p = 0.97c | 0.72 (0.39 to 1.31); p = 0.48c | 0.59 (0.35 to 1.01); p = 0.033c |
Interaction (continuous RS) adjusted for positive nodes:Interaction (continuous RS) adjusted for each of age, ethnicity, size, grade, PR, P53, HER2: significant (p = NR) Interaction adjusted for Allred-scored ER: p = 0.15 |
||
0- to 5-year DFS | 1.34 (0.47, 3.82)c | 0.95 (0.43, 2.14)c | 0.59 (0.32, 1.11)c | ||||||
5- to 10-year DFS | 0.88 (0.38, 1.92)c | 0.52 (0.21, 1.27)c | 0.60 (0.22, 1.62)c | ||||||
10-year BCSS |
Chemotherapy: 73% No chemotherapy: 54% Absolute difference: 19% |
p = 0.56 | p = 0.89 | p = 0.033 c | |||||
10-year OS |
Chemotherapy: 68% No chemotherapy: 51% Absolute difference: 17% |
1.18 (0.55 to 2.54); p = 0.68c p = 0.63 log-rank |
0.84 (0.40 to 1.78); p = 0.65c p = 0.85 log-rank |
0.56 (0.31 to 1.02); p = 0.057c p = 0.027 log-rank |
Interaction (continuous RS):c
|
In SWOG-8814 (LN+),53 DRFI was not reported. HRs for 10-year DFS for low-, intermediate- and high-risk groups, adjusted for number of positive nodes, were 1.02, 0.72 and 0.59, respectively.
Unadjusted interaction tests were statistically significant for 10-year DRFI and OS in NSABP B-20 (LN0) (p = 0.031 and p = 0.011, respectively). 50,61 Albain et al. 53 (LN+) found that the effect of recurrence score on treatment varied over time and that recurrence score is a treatment effect modifier in the first 5 years (interaction p-value 0.029) but not after 5 years (interaction p-value 0.580).
Interaction tests adjusted for clinicopathological factors in NSABP-B20 were borderline significant for the full cohort for DRFI (p = 0.035, p = 0.039 and p = 0.068 for different methods of assessing tumour grade),50 (Professor Tang, personal communication), whereas for the HER2– subgroup, the equivalent analyses were statistically significant (p = 0.007, p = 0.018 and p = 0.022) (Professor Tang, personal communication). The EAG report71 stated that it was unclear whether or not all factors were adjusted for simultaneously in B20; however, a personal communication with the biostatistician (Professor Tang, personal communication) confirmed that this was the case. Interaction tests in SWOG-8814 adjusted individually for each of age, ethnicity, tumour size, grade, PR, P53 and HER2 were also statistically significant (p = not reported). Initially, the EAG interpreted this as a model including all clinicopathological variables; however, clarification from the authors in a personal communication (Professor Barlow, University of Washington School of Public Health, personal communication, March 2018) stated that each variable was included in a separate model. An interaction test adjusted for Allred-scored ER status was not significant (p = 0.15). No interaction test that included all clinicopathological variables together was available.
The oncotype DX cut-off point below which chemotherapy could be avoided was reported to be approximately 20 in SWOG-8814,53 but NSABP B-20 authors could not determine a cut-off point as there was no point below which chemotherapy did not confer an advantage. 50,61
The analyses and available data were subject to some criticisms in the context of this decision problem. First, it was not clear whether or not all stratification factors used in randomising patients to treatment were included in the interaction test model. Second, categorising the continuous oncotype Breast Recurrence Score into risk groups may lead to loss of information and has the potential to create spurious interactions between recurrence score and chemotherapy benefit due to imbalances in clinicopathological variables between risk groups. Third, none of the analyses were conducted in the clinically-intermediate-risk patient group of interest to the decision problem; in particular, it is plausible that even if there is no chemotherapy benefit for clinically-low, oncotype DX-low patients, there could be benefit for clinically-intermediate (NPI of > 3.4) oncotype DX-low patients. Fourth, patients from the no-chemotherapy arm of the B20 study were used to derive the oncotype DX score. Therefore, oncotype DX may be overfitted in this study arm (i.e. recurrence rates may be artificially low in oncotype low-risk patients and artificially high in oncotype DX high-risk patients). This could lead to an overestimate of chemotherapy benefit because the chemotherapy arm was not used in derivation; therefore, recurrence rates in this arm may show less separation between the low- and high-risk groups. A more thorough discussion of this and other quality issues is provided in Report Supplementary Material 3.
Other potential biases in the reanalyses of RCTs included attrition of samples, exclusion of patients owing to missing data for covariates and inclusion of HER2+ patients (applies only to SWOG-8814 LN+ patients), who are out of the scope of this assessment.
Observational studies: results
Data are presented in Table 7. From the three observational cohort studies,64–67,69,70 evidence was mixed and at high risk of confounding, because patients who received chemotherapy were likely to be at higher risk of recurrence than patients who did not. Only one study reported an interaction test between recurrence score categories and chemotherapy treatment, and this was statistically significant (p = 0.03), but only adjusted for grade, tumour size, age and race (omitting ER and PR),67,70 and for recurrence score as a continuous variable (p < 0.001). The other two studies only reported HRs for chemotherapy versus no chemotherapy in intermediate- and high-risk patients,64–66,69 and in one study these were statistically non-significant, even after adjustment for confounders. 64,65 In the other study,66,69 patients with LN0/LN1micro did not appear to receive benefit from chemotherapy in the intermediate group (DRFI for chemotherapy vs. no chemotherapy: 94.4% vs. 94.7%, respectively), whereas those in the high-risk group did (DRFI for chemotherapy vs. no chemotherapy: 86.7% vs. 78.9%, respectively). In the LN1micro–LN3 subgroup, patients did appear to receive benefit from chemotherapy in the intermediate-risk group (DRFI for chemotherapy vs. no chemotherapy: 97.8% vs. 90.4%, respectively); data for high risk patients were not reported. A more detailed narrative is provided in Report Supplementary Material 3.
Reference (first author and year) | Study | Outcome | Cut-off point | Prediction of chemotherapy benefit: chemotherapy vs. no chemotherapy | Additional predictive value, adjusted HR, chemotherapy vs. no chemotherapy (95% CI) | ||||
---|---|---|---|---|---|---|---|---|---|
Low RS | Intermediate RS | High RS | Low RS | Intermediate RS | High RS | ||||
Barcenas 201764 Le Du 201565 Median follow-up: 58 months HR+, HER2–, LN0, Stage I–II, had oncotype DX test All risk groups, all years: n = 1424 Diagnosed 2005 to 2011 and included in K–M analysis: |
MD Anderson Center, USA | 5-year DRFS | 11–25 | NR | Actuarial 5-year rate:
|
HR NR, p = 0.74 | NR | 1.25 (0.32 to 4.92); p = 0.746a | 0.67 (0.16 to 2.78); p = 0.584a |
18–30 | NR | NR | NR | NR, too few events | 0.80 (0.23 to 2.71); p = 0.716 | 0.32 (0.07 to 1.47); p = 0.143 | |||
5-year DRFSb | 18–30 | NR | Stage 1 disease, intermediate risk (RS 18–30) onlyb (HRs NR):
|
NR | NR | NR | NR | ||
5-year IDFS | 11–25 | NR | Actuarial 5-year rate:
|
HR NR, p = 0.56 | NR | 1.64 (0.73 to 3.71); p = 0.233a | 0.67 (0.21 to 2.07); p = 0.483a | ||
18–30 | NR | NR | NR | 1.09 (0.14 to 8.62, p = 0.938) | 0.78 (0.34 to 1.80); p = 0.571 | 0.50 (0.13 to 2.02); p = 0.334 | |||
5-year RFS | 11–25 | NR | Actuarial 5-year rate:
|
HR NR, p = 0.94 | 1.46 (0.41 to 5.23); p = 0.564a | 0.78 (0.17 to 3.52); p = 0.748a | |||
18–30 | NR | NR | NR | NR, too few events | 0.98 (0.32 to 3.06); p = 0.975 | NR | |||
5-year OSc | 11–25 | NR | Actuarial 5-year rate:
|
HR NR, p = 0.18 | NR | 2.19 (0.44 to 11.0); p = 0.340a | 0.28 (0.04 to 2.05); p = 0.209a | ||
18–30 | NR | NR | NR | NR, too few events | 0.86 (0.15 to 4.91); p = 0.861 | 0.13 (0.01 to 1.30); p = 0.082 | |||
Stemmer 201666 Stemmer 201669 Median follow-up: 6 years ER+, HER2–, had oncotype DX test N0/1micro: n = 1594 LN1micro–LN3: n = 627e |
Clalit Health Services,66 Israel | 5-year DRFId | 18–30 | NR | LN0/1micro:
|
LN0/1micro:
|
NR | ||
18–30 | NR | LN1micro–LN3 (% DRF):
|
NR | ||||||
11–25 | NR | LN1micro–LN3 (% DRF):
|
NR | NR | |||||
5-year BCSSd | 18–30 | NR | LN1micro–LN3:
|
NR | NR | ||||
11–25 | NR | LN1micro–LN3:
|
NR | NR | |||||
Petkov 201670 Roberts 201667 Roberts 201768 Follow-up: 38 months HR+, HER2–, LN0f n = 40,134 |
SEER registry, USA | Actuarial 5-year BCSSc | 18–30 | NR | Multivariable modelg including chemotherapy treatment: RS remained significantly prognostic for 5-year BCSS for both chemotherapy-treated and untreated (or unknown) patients, but strength of association between RS categories and BCSS attenuated for those with chemotherapy reported as ‘yes’ (p = 0.03 for covariate-adjusted interaction) vs. those reported as ‘no/unknown’. Similar analysis with RS as continuous variable also significant both with and without adjustment for covariates (p < 0.001 for both) |
RSPC results
Recurrence score–pathology–clinical results were derived in TransATAC and NSABP B-14,44 and validated in NSABP B-20. 44 Only LN0 data were available. An interaction test was non-significant (p = 0.10), with a standardised HR of 0.65 (95% CI 0.39 to 1.09) (data not tabulated). 44
In practice, it is unlikely that chemotherapy decisions would be made on oncotype DX scores independent of clinicopathological variables. Evidence relating to the ability of the test to predict chemotherapy benefit over and above routinely collected clinicopathological variables was provided in both RCT data sets in the adjusted interaction tests. 50,53,61 Interestingly, Tang et al. 61 tested the ability of AOL to predict benefit from chemotherapy in a large cohort of 1952 patients, and found it to have predictive ability for OS. However, the inclusion of clinicopathological variables alongside recurrence score in the RSPC algorithm resulted in a loss of predictive ability (p = 0.10), indicating that the incorporation of clinicopathological factors may reduce prediction of chemotherapy benefit; therefore, if chemotherapy decisions are based on an informal consideration of clinicopathological factors alongside the oncotype DX score, this may reduce any predictive ability of oncotype DX in clinical practice.
Conclusion: oncotype DX and RSPC chemotherapy benefit
In conclusion, there is some evidence from two reanalyses of RCTs to suggest that oncotype DX may predict benefit from chemotherapy, and that benefit from chemotherapy is highest in oncotype DX high-risk patients. Unadjusted interaction tests between oncotype DX risk group and chemotherapy benefit were mainly statistically significant. Adjusted interaction tests were borderline significant in the LN0 NSABP B20 study (significant in HER2– patients), whereas in the LN+ SWOG-8814 study they were significant when adjusted for some clinicopathological variables individually, but not when adjusting for ER determined by Allred status. Only data from the derivation cohort (NSABP B-20) were available for LN0 patients and this may have biased the results. The RSPC algorithm (oncotype DX plus age, tumour size and grade) showed a non-significant interaction test between chemotherapy benefit and RSPC risk group, and also used the NSABP B-20 data set. Three observational cohort studies were at high risk of confounding; one reported a statistically significant interaction test but this was only adjusted for limited factors. If predictive ability was assumed, it is unclear below which exact cut-off point patients could avoid chemotherapy (although one study suggests that this is a recurrence score of 20), as chemotherapy benefit is uncertain in the intermediate-risk group. Although TAILORx22 (an ongoing RCT comparing long-term outcomes in patients treated with usual care vs. patients treated with usual care in conjunction with oncotype DX) will address the issue of whether or not low- and intermediate-risk patients can avoid chemotherapy, it is unclear to what extent it will address the question of whether or not the test can predict chemotherapy benefit. Considering the limitations of the available data, the EAG concludes that there remains uncertainty surrounding whether or not oncotype DX is associated with a predictive benefit of chemotherapy (i.e. a difference in relative effect by genomic risk group) and, if so, that there is uncertainty in the likely magnitude of this predictive effect within the clinical subgroups considered in this appraisal.
The TAILORx study22 reported during the course of the assessment, and included some data relating to chemotherapy benefit prediction. The EAG prepared a preliminary appraisal of this evidence, which can be accessed via the NICE website. 72
Clinical utility: oncotype DX
In this review, clinical utility relates to the impact of the prospective use of the test on patient outcomes such as survival and recurrence. The ideal study design would be a RCT in which patients are randomised to treatment guided by the test or treatment in accordance with usual practice. Observational studies (either prospective or retrospective) in which patients received the test prospectively in clinical practice are at a higher risk of bias from confounding. Such studies cannot address the question of whether or not the test can improve patient outcomes relative to usual practice. They can, however, reveal something about the ability of the test to identify a group of patients at very low ROR who could avoid chemotherapy. Data relating to risk in intermediate- and high-risk categories are, without a no-test comparator arm, difficult to interpret in the context of clinical utility. Therefore, we have focused on outcome data in low-risk patients and the use of chemotherapy in all groups. A full narrative synthesis of results can be found in Report Supplementary Material 4.
Study and patient characteristics
Five data sets reported across nine publications22,65,66,68,69,73–75 and one AiC manuscript were included. Study characteristics are presented in Report Supplementary Material 4. Two studies had a prospective trial design (within RCTs),22,73,74 and the remainder were retrospective analyses of observational data. One further study, the SEER registry,67,68 did not meet the inclusion criteria for the review in that only survival outcomes with < 5 years follow-up were reported, but it reported useful data on certain subgroups of interest to the review (micrometastases and race) and is presented here due to the paucity of other data relating to there characteristics.
Only one study (TAILORx)22 randomised patients (HR+, HER2– and LN0) to treatment guided by the test or treatment in accordance with usual practice. Women with recurrence scores of < 11 were assigned to endocrine therapy only, whereas women with recurrence scores of 11–25 were randomised to either endocrine therapy plus chemotherapy or endocrine therapy only. As of July 2017, this study had only reported results for the low-risk (recurrence score of < 11) group (n = 1626). Data for this group are effectively prospective observational data. The study reported in full in June 201876 and the EAG prepared a preliminary appraisal of this evidence, which can be accessed via the NICE website. 72
The West German Study Group (WSG) PlanB73,74,77 trial (n = 3198) is also a prospective RCT, but does not aim to assess the clinical utility of oncotype DX, as it randomises clinically high-risk patients {pathological tumour stage (pT)1–T4c; LN+, or LN0 with a risk factor [≥ pT2, grade 2/3, high urokinase plasminogen activator (uPA)/plasminogen activator inhibitor-1 (PAI-1), aged < 35 years or HR-negative]} with 0–3 positive lymph nodes with a recurrence score of ≥ 12 to two different sorts of chemotherapy. However, a translational research aim was to assess the ROR in patients with recurrence scores of < 12 who were not treated with adjuvant chemotherapy. This group is, again, effectively a prospective observational cohort.
There were three retrospective cohorts in which patients were treated using oncotype DX,65,66,69,75 and one further retrospective cohort that did not meet the inclusion criteria for the review. 67,70 The total number of patients included in these retrospective analyses is ≈54,000 (some double-counting from the Clalit Health Services cohort). 66,69 Patients were ER+, HER2– and must have had an oncotype DX test (it was unclear how patients were selected for testing, and this may have introduced spectrum bias). Two studies recruited only LN0 to lymph node micrometastases (LNmicro) patients,65,75 and one reported LN0-LNmicro69 and patients with micro metastases or between one and three lymph node metastases (LNmicro–LN3). 66,69 The study that did not meet the inclusion criteria recruited patients with LN0–3, and is included as it subgrouped patients in accordance with age (40–85 years), lymph node status (LN0, LNmicro–LN3, LNmicro only) and race (black, white, other). 67,70
Quality assessment
The highest level of evidence for clinical utility is a RCT of treatment guided by the test versus treatment guided in accordance with usual practice. Assessment with the Cochrane risk-of-bias tool for RCTs indicates that all studies are of too poor quality to meet this aim.
Results: oncotype DX clinical utility
Data relating to the clinical utility of oncotype DX are presented in Table 8. A more detailed narrative synthesis is provided in Report Supplementary Material 4. All studies report data relating to recurrence or survival, but differences in cut-off points (recurrence score of < 11, < 12 and < 18), patient populations (clinically high risk, LN0 or LN+), treatment regimens (some patients had chemotherapy in some studies) and outcome measures (DRFS, DFS, DRFI, BCSS and OS) precluded a meaningful meta-analysis.
Study, first author and year | Study design | Patients | Subgroup | Treatment | Outcome (5 years) | Cut-off pointa | Risk group, % risk of outcome (95% CI) | Comparison | Adjusted HR (95% CI)b | ||
---|---|---|---|---|---|---|---|---|---|---|---|
Low | Intermediate | High | |||||||||
DRFS/DRFI/IDFS | |||||||||||
LN0, cut-off point RS 18–30 | |||||||||||
Clalit Health Services, Stemmer 201669 | R | ER+, HER2–, had oncotype DX test | LN0–1micro, n = 159469 |
|
DRFI | 18–30 | 99.5 (98.4 to 99.8) | 98.8 (97.2 to 99.4) | 93.1 (87.1 to 96.3) | ||
MD Anderson, Le Du 201565 | R | ER+, HER2–, stage 1, had oncotype DX test | LN0/LNmicro, n = 1030 | Chemotherapy per group:
|
DRFS | 18–30 | 95.9 (93.0 to 97.6)c | NR | 76.4 (59.2 to 87.1)c | p < 0.0001 |
|
Memorial Sloan Kettering, Wen 201775 | R | ER+, HER2–, stage 1 and 2, had oncotype DX test, low RS | LN0 or LNmicro, n = 1406 | Chemotherapy:
|
DRFI | < 18 | 99.6%d | ||||
LN0, cut-off point RS 11 (or 12)–25 | |||||||||||
Memorial Sloan Kettering, Wen 201775 | R | ER+, HER2–, stage 1 and 2, had oncotype DX test, low RS | LN0 or LNmicro, n = 1406 | Chemotherapy:
|
DRFI | < 11 | 99.9%d | ||||
TAILORx, Sparano 201522 | P | HR+, HER2–, tumour sizee | LN0, n = 1626 | 100% endocrine therapy | DRFS | < 11 | 99.3 (98.7 to 99.6) | ||||
IDFS | < 11 | 93.8 (92.4 to 94.9) | |||||||||
LN+, cut-off point RS 18–30 | |||||||||||
Clalit Health Services, Stemmer 201666 | R | ER+, HER2–, had oncotype DX test | LN1micro–LN3, n = 62766 | Chemotherapy per group:
|
DRFI | 18–30 | 96.8 (NR) | 93.4 (NR) | 83.6 (NR) | ||
LN1micro, n = 27066 | DRFI | 99.3 (NR) | 89.2 (NR) | 80.6 (NR) | |||||||
LN+, cut-off point RS 11–25 | |||||||||||
Clalit Health Services, Stemmer 201666 | R | ER+, HER2–, had oncotype DX test | LN1micro–LN3, n = 62766 |
|
DRFI | 11–25 | 95.1 (NR) | 96.1 (NR) | 86.8 (NR) | ||
LN1micro, n = 27066 | 97.8 (NR) | 95.9 (NR) | 83.9 (NR) | ||||||||
LN+/LN0, cut-off point RS 12–25 | |||||||||||
WSG PlanB,f Nitz 201773,74,77 | P | Clinically high-risk,g HR+, HER2– patients | LN0–3,73 n = 2642 |
|
IDFS | 12–25 | 94.2 (91.2 to 97.3)h,i | 94.3 (92.8 to 95.8)h | 84.2 (80.6 to 87.8)h | HR = 2.33 (1.73 to 3.14); p < 0.001j | For continuous score (100–75th vs. 0–25th percentiles): 1.73 (1.21 to 2.47); p = 0.001 |
BCSS and OS | |||||||||||
LN0, cut-off point RS 18–30 | |||||||||||
Clalit Health Services, Stemmer 201669 | R | ER+, HER2–, had oncotype DX test | LN0–1micro, n = 159469 |
|
BCSS | 18–30 | 99.9 (99.0 to 100.0) | 98.5 (97.1 to 99.2) | 90.6 (84.5 to 94.4) | ||
SEER registry, Petkov 2016,70 Roberts 201667 | R | HR+, HER2–k | LN0, 40–84 years of age, n = 38,568 | LN0, 40–85 years:
|
BCSS < 5 years | 18–30 | 99.6 (99.4 to 99.7) | 98.6 (98.3 to 98.9) | 95.6 (94.4 to 96.6) |
|
|
LN0, black, n = 2890 | BCSS < 5 years | 18–30 | 99.2 (0.28) | 98.2 (0.58) | 94.3 (2.17) | p < 0.0001 | |||||
LN0, white, n = 33,684 | BCSS < 5 years | 18–30 | 99.6 (0.07) | 98.6 (0.15) | 95.6 (0.61) | p < 0.0001 | |||||
LN0, other race, n = 3321 | BCSS < 5 years | 18–30 | 99.8 (0.15) | 99.2 (0.36) | 95.3 (1.89) | p < 0.0001 | |||||
LN0, cut-off point RS 11–25 | |||||||||||
SEER registry, Petkov 2016,70 Roberts 201667 | R | HR+, HER2–k | LN0, all ages, n = 40,134 | Chemotherapy per group: NR | BCSS < 5 years | 11–25 | 99.6 (99.4 to 99.8) | 99.3 (99.2 to 99.4) | 96.4 (95.6 to 97.0) | p < 0.001 | |
TAILORx, Sparano 201522 | P (RCT) | HR+, HER2–, tumour size,e n = 1629 | LN0 | 100% endocrine therapy | OS | < 11 | 98.0 (97.1 to 98.6) | N/A | N/A | N/A | |
LN+, cut-off point RS 18–30 | |||||||||||
Clalit Health Services, Stemmer 201666 | R | ER+, HER2–, had oncotype DX test | LN1micro–LN3, n = 62766 | Chemotherapy per group:
|
BCSS | 18–30 | 99.1 (NR) | 97.4 (NR) | 86.9 (NR) | ||
LN1micro, n = 27066 | BCSS | 18–30 | 99.3 (NR) | 96.8 (NR) | 83.9 (NR) | ||||||
SEER registry, Petkov 2016,70 Roberts 201667 | R | HR+, HER2–k | LNmicro–LN3, all ages, n = 4691 | LN1–3:
|
BCSS < 5 yearsm,n | 99.0 (98.0 to 99.5)118 | 97.7 (95.9 to 98.7) | 85.7 (76.2 to 91.6) | p < 0.001 | ||
LN1–3, black, n = 328 | BCSS < 5 yearsm,n | 18–30 | 99.4 (0.56) | 98.9 (1.12) | 91.3 (8.31) | p = 0.4117 | |||||
LN1–3, white, n = 4,021 | BCSS < 5 yearsm,n | 18–30 | 99.0 (0.39) | 97.6 (0.75) | 84.1 (4.21) | p < 0.0001 | |||||
LN1–3, other race, n = 320 | BCSS < 5 yearsm,n | 18–30 | 98.5 (1.53) | 99.1 (0.92) | 100 (0) | p = 0.8427 | |||||
LNmicro, n = 282068 | NR | BCSS < 5 yearsm,n | 98.9 (97.4 to 99.6) | 99.1 (97.9 to 99.6) | 84 (74.1 to 90.4) | ||||||
LN+, cut-off point RS 11–25 | |||||||||||
Clalit Health Services, Stemmer 201666 | R | ER+, HER2–, had oncotype DX test | LN1micro–LN3, n = 62766 |
|
BCSS | 11–25 | 98.0 (NR) | 99.0 (NR) | 90.4 (NR) | ||
Ln1micro, n = 27066 | BCSS | 97.8 (NR) | 98.8 (NR) | 89.3 (NR) | |||||||
SEER registry, Petkov 2016,70 Roberts 201667 | R | HR+, HER2–k | LNmicro–LN3, all ages, n = 4691 | LN1–3:
|
BCSS < 5 yearsm,n | 11–25 | l99.0 (98.0 to 99.5)118 | 97.7 (95.9 to 98.7) | 85.7 (76.2 to 91.6) | p < 0.001 |
Across the studies in which chemotherapy was delivered in accordance with usual practice using oncotype DX, chemotherapy rates in patients at low risk (recurrence score of < 18) ranged from 1%69 to 12%75 (four studies65,67,69,70,75) in LN0 patients and from 7% to 23% (two studies66,67,70) in LN+ patients. In intermediate-risk (recurrence score of 18–30) patients, chemotherapy rates ranged from 26%69 to 43%65 (three studies65,69,75) in LN0 patients and from 40%66 to 47%67,70 (two studies66,67,69) in LN+ patients. These data perhaps indicate that lymph node status was considered in treatment decisions, although no formal comparison has been made. In high-risk patients, chemotherapy rates were similar in LN0 (90%65 and 89%69) and LN+ patients (90%). 66
Studies generally reported different outcomes [5-year DRFS (n = 2),22,65 DRFI (n = 2),66,69,75 IDFS (n = 2),22,73,74,77 BCSS (n = 3)66,67,69,70 and OS (n = 1)22], making comparisons across studies difficult. For outcomes including recurrence (DRFS, DRFI and IDFS), low-risk patients with recurrence scores of < 1865,66,69,75 had outcomes ranging from 95.9%65 (5-year DRFI) to 99.6%75 (5-year DRFI) in LN0 patients (n = 3) and 97%66 (5-year DRFI) in LN+ patients, whereas low-risk patients with recurrence scores of < 11 had outcomes of 94%22 (5-year IDFS) and 99.9%75 (5-year DRFI) in LN0 (n = 2)22,75 and 95%66 (5-year DRFI) in LN+ patients (n = 1). 66 Clinical advice to the EAG suggests that these levels of recurrence are acceptable in a low-risk population.
It was beyond the scope of the assessment to determine whether the newer cut-off points (of 11–25) should be used, or whether the original cut-off points of recurrence score 18–30 would be preferable. Data relating to this are summarised in the narrative synthesis (see Report Supplementary Material 4) and Table 8, and the general observation can be made that although use of lower cut-off points may result in better outcomes in the low-risk group (although data are mixed on this point), it would also result in fewer patients being classified as low risk.
Data relating to intermediate- and high-risk patients are included in the narrative synthesis (see Report Supplementary Material 4) and appear in Table 8. It was not possible to draw any conclusions regarding whether or not patients in intermediate- and high-risk categories had better outcomes as a result of using oncotype DX to guide treatment, as there were no comparator (no-oncotype DX) groups.
The data on micrometastases are difficult to interpret as there is no analysis that reports all nodal statuses in the same patient group (i.e. LN0, LNmicro, LN1–3). The analyses that have been done show that the trend for worse outcomes with increasing risk group holds true in patients with micrometastases. 66,67
The data relating to the performance of the test in patients of different races showed that BCSS survival differed similarly in accordance with risk categories in all race categories. 67
Conclusions
Without the highest level of evidence, it is not possible to conclude whether or not patient outcomes would be affected by use of the test in a clinical setting. In LN0 patients, use of the test in clinical practice appears to result in low rates of chemotherapy use in low-risk patients (1–12%), with acceptable outcomes (DRFS/DRFI/IDFS 96–99.6%). Rates of chemotherapy use increased with increasing risk category, and were generally higher in LN+ patients; only one study reported DRFS/DRFI/IDFS for LN+ patients, which was 97% (7% received chemotherapy). It was not possible to draw any conclusions regarding whether or not patients in intermediate- and high-risk categories had better outcomes as a result of using oncotype DX owing to the observational nature of the studies.
Results: MammaPrint
Development: MammaPrint
A description of the development of MammaPrint is provided in Report Supplementary Material 5.
Prognostic performance: MammaPrint
Here we summarise some key findings relating to DRFS/DRFI. See Report Supplementary Material 5 for a full narrative synthesis and data relating to survival outcomes.
Study characteristics
Several publications describe validation of the prognostic value of MammaPrint. Many include overlapping cohorts of patients, sometimes pooled with other cohorts, sometimes focusing on patient subgroups (e.g. ER+ or LN0/LN+), sometimes updating the data with longer follow-up and reporting a range of different outcomes. Therefore, it should be noted that there is some overlap between patient cohorts within the references included here. Table 9 shows both the study reference(s) (column 1) and the cohort(s) (column 2) used for each analysis, and Report Supplementary Material 5 describes the cohorts in more detail.
Reference (first author and year) | Cohorts | Population | Nodal status | ET/chemotherapy | Test or comparator | Percentage of patients per group | Percentage DRFS/DRFI risk: 0–5 years | Percentage DRFS/DRFI risk: 0–10 years | DRFS/DRFI: HR (95% CI) | |||
---|---|---|---|---|---|---|---|---|---|---|---|---|
Low | High | Low | High | Low | High | |||||||
Pooled analyses of patient cohorts: LN status mixed | ||||||||||||
Variable ET and chemotherapy | ||||||||||||
Beumer 2016,78 n = 217 Lobular cancer |
Lobular cancers, five pooled series79–82 | 94% ER+, 92% HER2– |
LN0, 66% LN+, 34% |
59% ET, 22% chemotherapy | MammaPrint | 76 | 24 | NR | NR | NR | NR | 0–10 years: 3.31 (1.79 to 6.12); p < 0.001 |
93% ER+, 93% HER2– | LN0 | 51% ET, 12% chemotherapy | MammaPrint | 82 | 18 | NR | NR | NR | NR | 0–10 years: 7.81 (2.89 to 21.07); p < 0.001 | ||
Knauer 2010,83 n = 541 | Pooled six series79–81,84–86 | 90% ER+, 89% HER2– |
LN0, 49% LN1–3, 51% |
All ET, 42% chemotherapy | MammaPrint | 47 | 53 | 95 | 82 | NR | NR | 0–5 years: 3.88 (1.99 to 7.58); p < 0.01 |
Mook 2010,87 n = 964 |
Pooled seven series79–81,84–86,88 T1 only |
84% ER+, 68% HER2– |
LN0, 72% LN+, 27% |
32% ET, 22% chemotherapy | MammaPrint | 54 | 46 | 95 | 80 | 87 | 72 | 0–10 years: 2.70 (1.88 to 3.88); p < 0.001 |
n = 552 | No ET, no chemotherapy | MammaPrint | 96 | 78 | 86 | 70 | 0–10 years: 2.90 (1.83 to 4.79); p < 0.001 | |||||
Retrospective studies: LN status mixed | ||||||||||||
Variable ET and chemotherapy | ||||||||||||
Drukker 2014,89 n = 295 | van de Vijver 200279 |
77% ER+ HER2 NR |
LN0, 51%, LN1–3, 36%, LN > 3, 13% | 14% ET, 37% chemotherapy | MammaPrint | 39 | 61 | 94.7 | 58.5 | 82.0 | 50.0 |
0–5 years: 9.6 (4.2 to 22.1); p = NR 5–10 years: 1.1 (0.5 to 2.5); p = NR 10–15 years: 1.2 (0.2 to 6.0); p = NR 15–20 years: 1.1 (0.1 to 17.9); p = NR 20–25 years: 0.3 (0 to 2.9); p = NR 0–25 years: 3.1 (2.02 to 4.86); p < 0.0001 |
Yao 2015,90 n = 238 | NorthShore & Fox Chase, USA |
All HR+ All HER2– |
LN0, 72%, LN+, 28% | 87% ET, 43% chemotherapy | MammaPrint | 60 | 40 | NR | NR | 96 | 87 | 0–10 years: 2.91 (0.97 to 8.68); p = 0.045 |
HR+/HR– | LN+/LN0 | No chemotherapy | MammaPrint | 61 | 39 | NR | NR | 98 | 85 | NR | ||
Reanalyses of RCTs: LN0 | ||||||||||||
100% ET monotherapy | ||||||||||||
van ‘t Veer 2017,91 Esserman92 ET: n = 281 |
STO-3 trial: ER+ analysis |
All ER+ HER2 NR |
LN0 | All ET, no chemotherapy | MammaPrint | 71 | 29 | NR | NR | 93 | 85 | NR |
No ET and chemotherapy | ||||||||||||
van ‘t Veer 2017,91 Esserman92 No ET: n = 257 |
STO-3 trial: ER+ analysis |
All ER+ HER2 NR |
LN0 | No ET, no chemotherapy | MammaPrint | 67 | 33 | NR | NR | 83 | 70 | NR |
Pooled analyses of patient cohorts: LN0 | ||||||||||||
No ET and chemotherapy | ||||||||||||
Bueno-de-Mesquita 201193 |
n = 186 |
76% ER+ 76% HER2– |
LN0 | No ET/chemotherapy | MammaPrint | 45 | 55 | NR | NR | 88 | 55 | NR |
Retrospective studies: LN0 | ||||||||||||
Variable ET and chemotherapy | ||||||||||||
Bueno-de-Mesquita 2009,84 n = 123 | Bueno-de-Mesquita 200984 |
76% ER+ 93% HER2– |
LN0 | 22% ET, 25% chemotherapy | MammaPrint | 52 | 48 | 98 | 78 | NR | NR | 0–5 years: 5.7 (1.6 to 20); p = 0.007 |
AOL | NR | NR | NR | NR | NR | NR | 0–5 years: 4.6 (0.61 to 35.1); p = 0.14 | |||||
NPI | NR | NR | NR | NR | NR | NR | 0–5 years: 2.2 (0.78 to 6.5); p = 0.14 | |||||
Buyse 200688 Company submission94 |
TRANSBIG88 n = 302 |
70% ER+ HER2 NR |
LN0 | No ET/chemotherapy | MammaPrint | 37 | 63 | NR | NR | 90 | 71 |
DRFI all follow-up (median 13.6 years) 2.32 (1.35 to 4.00); p = 0.002 |
AOL | 1.68 (0.92 to 3.07); p = 0.092 | |||||||||||
NPI | 1.65 (1.02 to 2.66); p = 0.043 | |||||||||||
Drukker 2014,89 Bueno-de-Mesquita 2009,84 n = 151 | van de Vijver 200279 |
72% ER+ HER2 NR |
LN0 | 4% ET, 4% chemotherapy | MammaPrint | 40 | 60 | 94.989 | 52.489 | 8684 | 5084 |
0–10 years: 5.5 (2.5 to 12); p < 0.00184 0–25 years: 4.57 (2.31 to 9.04); p < 0.000189 |
AOL | NR | NR | NR | NR | NR | NR | 0–10 years: 1.7 (0.84 to 3.6); p = 0.14 | |||||
NPI | NR | NR | NR | NR | NR | NR | 0–10 years: 3.1 (1.6 to 5.9); p < 0.001 | |||||
Ishitobi 2010,95 n = 102 | Osaka Medical Centre |
51% ER+ HER2 NR |
LN0 | 73% ET, 28% chemotherapy | MammaPrint | 20 | 80 | 100 | 94 | NR | NR | NR |
Mook 2010,86 n = 148 | NKI 1984–9686 (55–71 years) |
78% ER+ HER2 NR |
LN0 | 18% ET, no chemotherapy | MammaPrint | 61 | 39 | 93 | 72 | 80 | 67 |
0–5 years: 4.6 (1.8 to 12.0); p = 0.001 0–10 years: data per group, but p-values NR |
Wittner 2008,96 n = 100 | Massachusetts, USA |
80% ER+ HER2 NR |
LN0 | 24% ET, 21% chemotherapy | MammaPrint | 27 | 73 | NR | NR | NR | NR | DRFI:
|
Retrospective studies: LN+ | ||||||||||||
Variable ET and chemotherapy | ||||||||||||
Drukker 2014,89 n = 144 | van de Vijver 200279 | ER+/ER–, HER2 NR | LN1–3, 74%, LN > 3, 26% | Some ET, some chemotherapy | MammaPrint | 38 | 62 | 94.5 | 64.7 | 78.6 | 54.3 | 0–25 years: 2.24 (1.25 to 4.00); p = 0.01 |
Mook 2009,85 n = 241 | NKI and Italy85 | 79% ER+, 84% HER2– | LN1–3 | 73% ET, 56% chemotherapy | MammaPrint | 41 | 59 | 98 | 80 | 91 | 76 | 0–10 years: 4.13 (1.72 to 9.96); p = 0.002 |
There were nine main cohorts, which were small, retrospective analyses of consecutive patient series: four were from the Netherlands,79,80,84,87,89 two were multi-European,85,88 two were from the USA90,96 and one was from Japan95 (total n = 1805). They covered a mix of LN0 and LN+ patients, with variable proportions receiving endocrine therapy and chemotherapy. In most studies, around 70–80% were ER+, and HER2 status was not well reported. Four analyses pooling some of the above cohorts78,83,87,93 are also included due to their focus on specific subgroups. In addition, there was one reanalysis of a RCT [the Swedish Stockholm Tamoxifen-3 (STO-3) trial; n = 538], of which a subgroup had endocrine monotherapy. 78,91,92,94,97
Quality assessment
One study (van de Vijver et al. 79) included a small proportion of patients from the derivation cohort, and may therefore overestimate prognostic performance, although a ‘leave-one-out’ analysis was used to mitigate this problem to some extent. Most analyses excluded some patients from the original cohort, some because of insufficient tumour sample, which may introduce bias due to attrition of patients with smaller tumours. Blinding of test assessors to outcomes was reported in around half of the studies. Outcomes did not always match standardised definitions and it was not always possible to tell from the publication whether or not all deaths and breast cancer deaths were counted as events or were censored. Different levels of treatment use in the high- and low-risk groups may confound results by reducing separation between the groups in recurrence rates, and selection of patients in accordance with treatment may introduce spectrum bias because these patients may be systematically different from the whole population. Many studies included a proportion of patients who were not in the scope of this research.
Distribution of patients by risk group
The percentage of patients categorised as low risk ranged from 20% to 71% and the percentage of those categorised as high risk ranged from 29% to 80% across seven analyses of LN0 patients. 84,86,88,89,91,95,96 In two analyses of LN+ patients,85,89 the percentages categorised as low risk were 38% and 41%, whereas the percentages categorised as high risk were 59% and 62%.
Prognostic performance: unadjusted analyses
Prognostic data for MammaPrint is provided in Tables 10 and 11. Among LN0/LN+ studies, Mook et al. 87 pooled 964 patients from seven series79–81,84–86,88 (one-third had endocrine therapy and one-quarter had chemotherapy) and showed that MammaPrint was statistically significantly prognostic for 10-year DRFS (HR 2.70, 95% CI 1.88 to 3.88; p < 0.0001), with 10-year DRFS of 87% in low-risk patients. 87 Knauer et al. 83 pooled 541 patients (restricted to LN0–3 patients, 100% endocrine therapy, 42% chemotherapy) from six of these series, and also reported statistically significant results. In terms of longer follow-up of the original van de Vijver et al. 79 cohort (51% LN0, 37% had chemotherapy and 14% had endocrine therapy), MammaPrint was statistically significantly prognostic in an unadjusted analysis of DRFS over 0–25 years89 in a LN0/LN+ cohort;79 most of this difference was in the first 5 years (HR 9.6, 95% CI 4.2 to 22.1). A separate US series (Yao et al. ;90 72% LN0, 43% had chemotherapy and 87% had endocrine therapy) also showed statistically significant prognostic ability for DRFS at 10 years (HR 2.91, 95% CI 0.97 to 8.68; p = 0.045) (see Table 9) with DRFS rates in the low-risk group of 96% at 10 years; results were similar (low-risk 10-year DRFS 98%) in a subset with no chemotherapy.
Reference (first author and year) | Cohorts | Population | Nodal status | ET/chemotherapy | Outcome | Test or comparator | Percentage of patients per group | Percentage risk of outcomes: 0–5 years | Percentage risk of outcomes: 0–10 years | HR (95% CI) | |||
---|---|---|---|---|---|---|---|---|---|---|---|---|---|
Low | High | Low | High | Low | High | ||||||||
Low risk via AOL + NPI + St Gallen criteria (LN0) | |||||||||||||
Bueno-de-Mesquita 201193 | Pooled,79,84 n = 139 | ER+, low clinical risk | LN0 | No ET/chemotherapy | DRFI | MammaPrint | 77 | 23 | NR | NR | 87 | 70 | 0–10 years: HR NR; p = 0.19 |
OS | MammaPrint | 77 | 23 | NR | NR | 100 | 86 | 0–10 years: HR NR; p = 0.016 | |||||
Discordant risk via AOL + NPI + St Gallen criteria (LN0) | |||||||||||||
Bueno-de-Mesquita 201193 | Pooled,79,84 n = 139 | ER+, discordant clinical risk | LN0 | No ET/chemotherapy | DRFI | MammaPrint | 66 | 34 | NR | NR | 91 | 63 | 0–10 years: HR NR; p = 0.004 |
OS | MammaPrint | 66 | 34 | NR | NR | 88 | 58 | 0–10 years: HR NR; p = 0.06 | |||||
High risk via AOL + NPI + St Gallen criteria (LN0) | |||||||||||||
Bueno-de-Mesquita 201193 | Pooled,79,84 n = 139 | ER+, high clinical risk | LN0 | No ET/chemotherapy | DRFI | MammaPrint | 27 | 73 | NR | NR | 77 | 45 | 0–10 years: HR NR; p = 0.19 |
OS | MammaPrint | 27 | 73 | NR | NR | 77 | 53 | 0–10 years: HR NR; p = 0.17 | |||||
High risk via AOL (LN+) | |||||||||||||
Mook 200985 | NKI plus Italy85 n = 209 | High-risk AOL | LN1–3 | Some ET, some chemotherapy | BCSS | MammaPrint | NR | NR | NR | NR | 94 | 76 | 0–10 years: 4.12 (1.45 to 11.76); p = 0.008 |
Reference (first author and year) | Cohorts | Population | Nodal status | ET/chemotherapy | Test or comparatora | C-index (AUC) (95% CI) | Increase in LR χ2 over clinicopathological factorsa | Multivariable model adjuvant for clinicopathological factors,a AOLb or NPI:c HR (95% CI) |
---|---|---|---|---|---|---|---|---|
Pooled analyses of patient cohorts: LN status mixed | ||||||||
Variable ET and chemotherapy | ||||||||
Beumer 2016,78 n = 217 Lobular cancer |
Lobular cancers, five pooled series79–82 | 94% ER+, 92% HER2– | LN0, 66%; LN+, 34% | 59% ET, 22% chemotherapy | MammaPrint | 10 years: 2.08 (1.05 to 4.14); p = 0.037a | ||
93% ER+, 93% HER2– | LN0 | 51% ET, 12% chemotherapy | MammaPrint | 10 years: 6.40 (2.14 to 19.17); p = 0.001a | ||||
Mook 2010,87 n = 941 | Pooled seven series79–81,84–86,88 | 84% ER+, 68% HER2– | LN0, 72%; LN+, 27% | 32% ET, 22% chemotherapy | MammaPrint | 10 years: 2.43 (1.56 to 3.77); p < 0.001a | ||
All ER+ (n = 788) | LN+/LN0 | Some ET/chemotherapy | MammaPrint | 10 years: 2.51 (1.60 to 3.95); p < 0.001a | ||||
n = 552 | LN+/LN0 | No ET/chemotherapy | MammaPrint | 10 years: 2.54 (1.49 to 4.34); p = 0.001a | ||||
Retrospective studies: LN status mixed | ||||||||
Variable ET and chemotherapy | ||||||||
van de Vijver,79 n = 295 | van de Vijver79 | 77% ER+, HER2 NR |
LN0, 51%; LN+, 49% |
14% ET, 37% chemotherapy | MammaPrint | 10 years: 4.6 (2.3 to 9.2); p < 0.001a | ||
Yao 2015,90 n = 373 | NorthShore & Fox Chase | 74% ER+, 83% HER2– |
LN0, 72%; LN+, 28% |
65% ET, 58% chemotherapy | MammaPrint | 10 years: 3.01 (0.88 to 10.33); p = 0.08a | ||
Pooled analyses of patient cohorts: LN0 | ||||||||
No ET and chemotherapy | ||||||||
Bueno-de-Mesquita 2011,93 n = 186 | Pooled79,84 | 76% ER+, 76% HER2– | LN0 | No ET/chemotherapy | MammaPrint | Change log-likelihood, p < 0.001 | ||
Retrospective studies: LN0 | ||||||||
Variable ET and chemotherapy | ||||||||
Bueno-de-Mesquita 2009,84 n = 123 | Bueno-de-Mesquita84 | 76% ER+, 93% HER2– | LN0 | 22% ET, 25% chemotherapy | MammaPrint |
|
Change log-likelihood 5.5, p = 0.023 |
5 years: 4.8 (1.3 to 17); p = 0.018b 5.4 (1.4 to 21); p = 0.015c |
van de Vijver 2002;79 Bueno-de-Mesquita 2009;84 Buyse 2006,88 n = 151 | van de Vijver79 | 72% ER+, HER2 NR | LN0 | 4% ET, 4% chemotherapy | MammaPrint |
|
Change log-likelihood 15.8, p < 0.01 | |
No ET and chemotherapy | ||||||||
Buyse 2006,88 n = 302 | TRANSBIG88 | 70% ER+, HER2 NR | LN0 | No ET/chemotherapy | MammaPrint | |||
Retrospective studies: LN+ | ||||||||
Variable ET and chemotherapy | ||||||||
Mook 2009,85 n = 241 | NKI plus Italy85 | 79% ER+, 84% HER2– | LN1–3 | 73% ET, 56% chemotherapy | MammaPrint | 10 years: 2.99 (0.996 to 8.99); p = 0.051a |
Among LN0 patients, in the only reanalysis of a RCT the STO-3 trial (van ‘t Veer et al. 91) reported 10-year DRFS rates (93% in low-risk patients, 85% in high-risk patients) (see Table 9) but no statistically significant levels were reported. 91 Four out of five retrospective LN0 cohorts reported statistically significant prognostic performance of MammaPrint for DRFS/DRFI at varying time points, based on unadjusted HRs between risk groups. 84,86,88,89 The 10-year DRFS/DRFI rates in low-risk patients ranged from 80% to 90% across three analyses (with varying rates of endocrine therapy and chemotherapy use). 84,86,88
Three of the LN0 cohorts84,88,89 included comparisons with AOL and NPI, which appeared to have less prognostic value than MammaPrint, although no statistical comparisons were reported. There were no comparisons with other risk tools such as Predict or Modified Adjuvant! Online (mAOL). Among LN+ patients, two cohorts reported statistically significant prognostic performance of MammaPrint based on unadjusted HRs between risk groups, with 10-year DRFS rates in low-risk patients of 79% and 91% (with varying rates of endocrine therapy and chemotherapy use). 85,89
See Report Supplementary Material 5 for subgroup analyses in patients with low or high clinical risk and in patients with lobular breast cancer, and for OS and BCSS outcomes.
Additional prognostic value
Several studies reported adjusted analyses relating to the additional prognostic value of MammaPrint over existing clinicopathological risk scores and clinicopathological variables. A pooled analysis of LN0/LN+ patients from seven series79–81,84,85,87,88 showed that MammaPrint was statistically significantly prognostic for 10-year DRFS in a multivariable analysis adjusting for clinicopathological variables. However, in the US series (Yao et al. 90), MammaPrint was borderline non-statistically significant in a multivariable analysis (p = 0.08). Among LN0 patients, MammaPrint was statistically significantly prognostic for DRFI when adjusted for AOL or NPI in three cohorts reported across two publications. 84,88 C-indices from two79,84 of these cohorts showed higher values when MammaPrint was included alongside clinicopathological factors than for either alone, although differences were not statistically compared. 84 In one analysis of LN+ patients, MammaPrint was borderline statistically significant for 10-year DRFS and statistically significantly prognostic for 10-year BCSS,85 although in another analysis79 BCSS at 10 years was borderline non-statistically significant.
Conclusions: MammaPrint prognostic performance
The prognostic value of MammaPrint is based on nine retrospective analyses, four pooled analyses (including six of the nine retrospective series and one prospective series) and one reanalysis of a RCT. Studies were variable in terms of nodal status, ER status and receipt of endocrine therapy and chemotherapy. The percentage of LN0 patients categorised as low risk ranged from 20% to 71% and the percentage of those categorised as high risk ranged from 29% to 80%. In LN+ patients, the percentage categorised as low risk was 38% to 41% and the percentage categorised as high risk ranged from 59% to 62%. MammaPrint was statistically significantly prognostic for 10-year DRFS in almost all unadjusted analyses of LN0 and LN+ patients (as well as in pooled analyses). For LN0 patients, 10-year DRFS/DRFI rates for low-risk patients ranged from 80% to 90% (with varying rates of endocrine therapy and chemotherapy use), whereas the reanalysis of a RCT reported 10-year DRFS of 93% with endocrine monotherapy and 83% without endocrine therapy or chemotherapy. Interestingly, although on the whole MammaPrint low-risk 10-year DRFS rates are lower than those for the other in-scope tests, the 93% figure for patients having endocrine monotherapy is more in line with other tests and may better reflect the population used in studies of other tests (ER+, endocrine monotherapy). For LN+ patients, 10-year DRFS rates in low-risk patients ranged from 79% to 91% (with varying rates of endocrine therapy and chemotherapy use). In terms of additional prognostic value, MammaPrint was statistically significantly prognostic for 10-year DRFS/DRFI in multivariable analyses adjusted for clinicopathological risk tools (AOL and NPI) and various combinations of clinicopathological variables in LN0/LN+ and LN0 cohorts, whereas adjusted analyses in LN+ cohorts were statistically significant or borderline significant.
Chemotherapy benefit: MammaPrint
Study designs and patients
Two publications have reported the ability of MammaPrint to predict the benefit of chemotherapy. Knauer et al. 83 reported a pooled analysis of 541 patients (100% received endocrine therapy, 42% received chemotherapy) from six consecutive patient series (see Report Supplementary Material 5, Table 7). Overall, 90% were ER+ and 89% were HER2–, and half were LN0 and half had one to three positive nodes (LN1–3). In addition, the article by Mook et al. 85 reported a pooled analysis of two out of the six patient series from Knauer et al. 83 (see Report Supplementary Material 5, Table 7), with an extended follow-up (10 years), but restricted to LN1–3 patients (including micrometastases).
Quality assessment
Both studies were pooled retrospective cohorts in which patients were treated in accordance with usual practice [in addition, one of the six cohorts in Knauer et al. 83 was the prospective MicroarRAy-prognoSTics-in-breast-cancER (RASTER) study,81 in which patients were treated in accordance with usual practice plus MammaPrint]. For this reason, those who received chemotherapy are likely to be systematically different in terms of known (and potentially unknown) prognostic factors to those who did not, leading to a high risk of confounding. Both studies blinded the test assessors to clinical outcomes, and both used standard outcome definitions. Both studies included a proportion of patients outside the scope (ER– and/or HER2+). See Report Supplementary Material 5, Table 7.
Results
The pooled analysis of six consecutive series by Knauer et al. 83 reported that at 5 years, there was a statistically significant effect of chemotherapy in the MammaPrint high-risk group but no statistically significant effect in the low-risk group, although HRs favoured chemotherapy in both groups (Table 12). Unadjusted HRs for DRFS (for no chemotherapy vs. chemotherapy) were 0.26 (95% CI 0.03 to 2.02; p = 0.20) in the low-risk group and 0.35 (95% CI 0.17 to 0.71; p < 0.01) in the high-risk group, whereas unadjusted HRs for BCSS were 0.58 (95% CI 0.07 to 4.98; p = 0.62) in the low-risk group and 0.21 (95% CI 0.07 to 0.59; p < 0.01) in the high-risk group. Multivariable analyses of the effect of chemotherapy on 5-year BCSS were again statistically significant in the high-risk group (HR 0.21, 95% CI 0.06 to 0.80; p = 0.02) but not in the low-risk group (HR not estimable; p = 0.98) (see Table 12). However, the interaction test for chemotherapy treatment and risk group was not statistically significant (p = 0.45; the interaction test appears to relate to 5-year BCSS as opposed to DRFS but this is unclear in the publication83). This indicates that the effect of chemotherapy versus no chemotherapy on 5-year BCSS was not statistically significantly different between risk groups. It is unclear whether this interaction test relates to the adjusted or unadjusted analysis.
Reference (first author and year) | Cohorts | Population | Percentage of patients in each risk group | Outcome | Low risk | High risk | Adjusted HRsa | Interaction tests | |||||
---|---|---|---|---|---|---|---|---|---|---|---|---|---|
Low | High | No chemotherapy: % risk | Chemotherapy: % risk | HR (95% CI) | No chemotherapy: % risk | Chemotherapy: % risk | HR (95% CI) | ||||||
Knauer 201083 Pooled cohorts n = 541 |
Pooled six series: |
|
47 | 53 |
DRFS 5 years |
93 | 99 | 0.26 (0.03 to 2.02); p = 0.20 | 76 | 88 | 0.35 (0.17 to 0.71); p < 0.01 | NR | NR |
BCSS 5 years |
97 | 99 | 0.58 (0.07 to 4.98); p = 0.62 | 81 | 94 | 0.21 (0.07 to 0.59); p < 0.01 | No chemotherapy vs. CTa (95% CI):
|
Interactionb (risk group plus chemotherapy): p = 0.45 | |||||
Mook 200985 (LNmicro–3) Retrospective n = 347 |
|
41 | 59 | 10-year BCSS | NR | NR | NR | NR | NR | NR | Interaction (risk group plus chemotherapy, series 1 + 2 pooled, multivariablea): p = 0.95 |
For the two pooled LNmicro–3 cohorts reported by Mook et al. 85 (these were subsets of two of the six cohorts pooled in Knauer et al. 83), the only evidence relating to prediction of chemotherapy benefit was a test of the interaction between chemotherapy treatment and risk group (within a multivariable analysis of 10-year BCSS), which was not statistically significant (p = 0.95) (see Table 12).
In the analysis of six series,83 it was unclear whether the interaction test was unadjusted or adjusted, and, if so, for which factors. In the analysis of LN1–3 patients from two series,85 the interaction test was conducted within a multivariable analysis adjusted for clinicopathological variables.
Conclusions: MammaPrint chemotherapy benefit
In a pooled analysis of 541 patients (half LN0, half LN1–3) in which patients were treated in accordance with usual practice, the effect of chemotherapy versus no chemotherapy on 5-year DRFS and BCSS was statistically significant in the MammaPrint high-risk group but not in the low-risk group in unadjusted analyses for 5-year DRFS and BCSS and in adjusted analyses for 5-year BCSS. However, the interaction test for chemotherapy treatment and risk group (for 5-year BCSS) was non-significant (p = 0.45). A further pooled analysis of two of the above series, restricted to LN1–3 patients, also reported a statistically non-significant interaction between chemotherapy treatment and risk group for 10-year BCSS (p = 0.95). The evidence for the ability of MammaPrint to predict chemotherapy benefit is extremely limited; although unadjusted analyses suggest a greater effect of chemotherapy in high-risk groups, adjusted analyses were only reported for one outcome, and the non-significant interaction tests suggest that there was no statistically significant difference in effect of chemotherapy between risk groups.
Clinical utility: MammaPrint
Overview
Two studies reported evidence relating to clinical utility of MammaPrint (the impact of prospective use of the test on clinical outcomes). Microarray In Node-negative Disease may Avoid ChemoTherapy (MINDACT) is a RCT of MammaPrint versus clinical practice. 98 RASTER81,99,100 is a prospective observational study in which patients were treated in accordance with usual practice plus MammaPrint. As these two studies are very different in design, they are reported separately in the following sections.
This section of the report summarises the main points; a full description can be found in Report Supplementary Material 5.
Clinical utility randomised controlled trial: MINDACT
Study design
MINDACT (Cardoso et al. 98) is a partially randomised prospective study of MammaPrint versus clinical practice. Patients with discordant risk scores (high risk/low risk or low risk/high risk) as assessed by MammaPrint and mAOL (mAOL was calculated HER2 status as well as the usual grade, nodal status and tumour size, and is not a tool used in clinical practice in the UK, which limits the generalisability of findings of this trial) were randomised to chemotherapy or no chemotherapy; this also means that discordant-risk patients were randomised to treatment determined by MammaPrint or treatment determined by mAOL.
Patients with concordant risk were not randomised, but were followed as prospective cohorts. High/high-risk patients (via both MammaPrint and mAOL) were recommended to receive chemotherapy, whereas no chemotherapy was recommended for low/low-risk patients.
The primary aim was to determine whether or not patients who were at high-clinical and low-MammaPrint risk could avoid chemotherapy by comparing outcomes for patients randomised to chemotherapy or no chemotherapy. The results were also presented for low-clinical-risk, high-MammaPrint-risk patients. Secondary analyses included an analysis of discordant patients in accordance with treatment group (chemotherapy vs. no chemotherapy) and for all patients when chemotherapy was recommended in accordance with clinical risk or with MammaPrint risk. The percentage of patients assigned to chemotherapy with each strategy was also reported.
Patients and tests
MINDACT enrolled 6693 patients from nine European countries (see Report Supplementary Material 5, Table 9, for details of group assignments). Of these patients, 88% were hormone-receptor-positive and 90% were HER2–; 79% were LN0 and 21% were LN1–3. However, this varied by group (see Report Supplementary Material 5, Table 9).
Quality assessment
Randomisation sequence and allocation concealment were judged as having a low risk of bias. No details of blinding were reported (see Report Supplementary Material 5, Table 10). Intention-to-treat (ITT) and per-protocol analyses were reported. Some patients did not adhere to their recommended chemotherapy or no chemotherapy allocation. Other patients had a change in clinical risk group owing to initial incorrect reporting of clinical characteristics, or a change in MammaPrint risk group owing to a change in the RNA extraction solution that affected the calculation of risk group. For the ITT analysis, patients were analysed in their originally allocated clinical/MammaPrint risk groups and in their randomised treatment groups. Per-protocol analysis excluded patients who were ineligible, were non-adherent to chemotherapy recommendations or who had a change in their clinical or MammaPrint risk group. This report uses ITT results (when available).
Results
High-clinical-risk, low-MammaPrint-risk group
In this group (n = 1497; 52% LN0), 5-year DMFS was 95.9% (95% CI 94.0% to 97.2%) with chemotherapy and 94.4% (95% CI 92.3% to 95.9%) without chemotherapy; this was an absolute difference of 1.5% favouring chemotherapy, although the HR was not statistically significant (adjusted HR 0.78, 95% CI 0.50 to 1.21; p = 0.267). Similar differences between chemotherapy and no chemotherapy were reported for 5-year DMFI, DFS and OS, as well as among both LN0 and LN1–3 patients and a LN0, HR+, HER2– subgroup (Table 13). Statistically, this met the primary objective in that the lower bound of the 95% CI for 5-year DMFS in the no-chemotherapy group was ≥ 92%. This finding was interpreted by the authors as showing little difference in outcomes for chemotherapy versus no chemotherapy, implying that patients who were at high-clinical but low-MammaPrint risk could potentially avoid chemotherapy. Clinical advice to the EAG suggests that chemotherapy would usually only be indicated when it is likely to provide an absolute improvement in 5-year DRFS of 2–3%, which suggests that it may be reasonable to withhold chemotherapy in patients with high-clinical, low-MammaPrint risk given the above absolute difference in 5-year DRFS of 1.5% for chemotherapy versus no chemotherapy.
Study (first author and year) | Subgroup | n | Population | Nodal status | Outcome | Percentage risk of outcome (95% CI) | HR adjusteda (95% CI) | Absolute difference (95% CI) | |
---|---|---|---|---|---|---|---|---|---|
No chemotherapy | Chemotherapy | ||||||||
High clinical, low MammaPrint group (randomised to chemotherapy or no chemotherapy; ITTb) | |||||||||
Node negative and node positive | |||||||||
Cardoso 201698 | High mAOL, low MammaPrint | 1497 | 98% HR+, 92% HER2– | LN0, 52%; LN1–3, 48% | 5-year DMFS | 94.4 (92.3 to 95.9) | 95.9 (94.0 to 97.2) | 0.78 (0.50 to 1.21); p = 0.267 | 1.5% |
5-year DRFI | 95.3 (93.4 to 96.6) | 96.6 (94.8 to 97.8) | 0.76 (0.47 to 1.22); p = 0.253 | 1.3% | |||||
5-year DFS | 90.1 (87.5 to 92.1) | 92.9 (90.5 to 94.7) | 0.71 (0.50 to 1.01); p = 0.055 | 2.8% | |||||
5-year OS | 97.0 (95.4 to 98.1) | 98.4 (97.0 to 99.1) | 0.69 (0.35 to 1.35); p = 0.278 | 1.4% | |||||
Node negative | |||||||||
Cardoso 201698 | High mAOL, low MammaPrint | 787 | NR | LN0 | 5-year DMFS | 93.2 (90.1 to 95.4) | 95.7 (93.0 to 97.4) | 0.69 (0.39 to 1.21); p = 0.193 | 2.5% |
699 | All HR+, all HER2– | LN0 | 5-year DMFS | 93.9 (90.6 to 96.1) | 95.5 (92.5 to 97.3) | 0.80 (0.44 to 1.45); p = 0.456 | 1.6% | ||
Node positive | |||||||||
Cardoso 201698 | High mAOL, low MammaPrint | 709 | NR | LN1–3 | 5-year DMFS | 95.6 (92.7 to 97.4) | 96.3 (93.1 to 98.1) | 0.88 (0.42 to 1.82); p = 0.724 | 0.7% |
Low clinical, high MammaPrint group (randomised to chemotherapy or no chemotherapy; ITTb) | |||||||||
Node negative and node positive | |||||||||
Cardoso 201698 | Low mAOL, high MammaPrint | 690 | 90% HR+, 88% HER2– | LN0, 98%; LN1–3, 2% | 5-year DMFS | 95.0 (91.8 to 97.0) | 95.8 (92.9 to 97.6) | 1.17 (0.59 to 2.28); p = 0.657 | 0.8% |
5-year DRFI | 95.6 (92.5 to 97.5) | 98.1 (95.7 to 99.1) | 0.63 (0.27 to 1.47); p = 0.282 | 2.5% | |||||
5-year DFS | 90.1 (86.1 to 93.0) | 92.1 (88.3 to 94.6) | 0.87 (0.53 to 1.45); p = 0.603 | 2.0% | |||||
5-year OS | 97.8 (95.5 to 99.0) | 97.1 (94.5 to 98.5) | 1.28 (0.54 to 3.02); p = 0.578 | –0.7% | |||||
Node negative | |||||||||
Cardoso 201698 | Low mAOL, high MammaPrint | 635 | NR | LN0 | 5-year DMFS | 95.1 (91.9 to 97.1) | 96.0 (93.1 to 97.7) | 1.09 (0.54 to 2.19); p = 0.815 | 0.9% |
534 | All HR+, all HER2– | LN0 | 5-year DMFS | 95.5 (91.6 to 97.6) | 95.1 (91.5 to 97.2) | 1.45 (0.68 to 3.08); p = 0.333 | –0.4% | ||
Node positive | |||||||||
Cardoso 201698 | Low mAOL, high MammaPrint | NR (number too small) | NR | LN1–3 | 5-year DMFS | NR | NR | NR | NR |
Outcomes for non-randomised groupsb | |||||||||
Low clinical, low MammaPrint (node negative and node positive) | |||||||||
Cardoso 201698 | Low mAOL, low MammaPrint | 2745 | 100% HR+, 96% HER2– | LN0, 94%; LN1–3, 6% | 5-year DMFS | 97.6 (96.9 to 98.1) | N/A | ||
5-year DFS | 92.8 (91.7 to 93.7) | N/A | |||||||
5-year OS | 98.4 (97.8 to 98.9) | N/A | |||||||
High clinical, high MammaPrint (node negative and node positive) | |||||||||
Cardoso 201698 | High mAOL, high MammaPrint | 1806 | 62% HR+, 81% HER2– | LN0, 74%; LN1–3, 26% | 5-year DMFS | N/A | 90.6 (89.0 to 92.0) | ||
5-year DFS | N/A | 85.3 (83.4 to 87.0) | |||||||
5-year OS | N/A | 94.7 (93.4 to 95.7) |
Low-clinical-risk, high-MammaPrint-risk group
Among these patients (n = 690; 98% LN0) (see Table 13), 5-year DMFS was 95.8% (95% CI 92.9% to 97.6%) with chemotherapy and 95.0% (95% CI 91.8% to 97.0%) without chemotherapy, an absolute difference of 0.8% (adjusted HR 1.17, 95% CI 0.59 to 2.28; p = 0.657). These findings could be interpreted as showing that use of MammaPrint in low-clinical-risk patients could lead to more patients being prescribed chemotherapy but not receiving a survival benefit from treatment.
Non-randomised concordant-risk groups
Patients with low/low-risk (recommended no chemotherapy) had a 5-year DMFS of 97.6% (95% CI 96.9% to 98.1%). Conversely, patients with high/high-risk (recommended chemotherapy) had a 5-year DMFS of 90.6% (95% CI 89.0% to 92.0%). The results for DFS and OS followed a similar pattern (see Table 13).
Estimated outcomes in accordance with clinical and MammaPrint treatment strategies
Additional analyses assessed strategies in which chemotherapy recommendations for all patients were determined by either clinical risk or MammaPrint risk. These included concordant (non-randomised) and discordant (randomised) patients who had treatment that matched either their clinical risk (treatment determined by clinical risk group) or MammaPrint risk (treatment determined by MammaPrint risk group). Of all 6693 patients, 3356 (50%) were at high clinical risk using mAOL and 2398 (36%) were at high MammaPrint risk (data not tabulated). Therefore 14% fewer (958/6693) patients were categorised as being at high risk using MammaPrint than using mAOL, and of those at high clinical risk, 46% (1550/3356) could be reclassified to low risk by MammaPrint. The 5-year DMFS rate was very similar between the clinical strategy (5-year DMFS rate 95.0%) and the MammaPrint strategy (5-year DMFS rate 94.7%). This was interpreted as the MammaPrint strategy leading to little difference in outcomes while sparing many patients from chemotherapy. Given the results in the low-clinical-risk group (in which treatment in accordance with MammaPrint risk groups would result in more patients receiving chemotherapy but with no DMFS advantage), the most advantageous strategy may be to only test clinical high-risk patients with MammaPrint. However, the comparator in this study was mAOL, and it is unclear whether or not the same would be true for other clinical risk scores.
Multivariable analysis
In a multivariable analysis adjusted for chemotherapy use, clinical risk and patient and tumour characteristics, MammaPrint low/high-risk grouping was statistically significantly associated with 5-year DMFS (HR for high vs. low-risk 2.41, 95% CI 1.79 to 3.26; p < 0.001).
Conclusions: randomised controlled trial of clinical utility for MammaPrint (MINDACT)
MINDACT randomised patients with discordant MammaPrint and mAOL risks to chemotherapy or no chemotherapy. For patients who were high-clinical, low-MammaPrint risk, 5-year DMFS was 95.9% with chemotherapy and 94.4% without chemotherapy, an absolute difference of 1.5%. This raises the possibility of avoiding chemotherapy in these patients. In patients who were low-clinical, high-MammaPrint risk, 5-year DMFS was 95.8% with chemotherapy and 95.0% without chemotherapy, an absolute difference of 0.8%. This could be interpreted as showing that MammaPrint may not be useful in this group as it would increase chemotherapy rates without improving outcomes. However, the comparator was mAOL, and it is unclear whether or not the same would be true for other clinical risk measures.
Clinical utility observational study: RASTER
Study design, patients and tests
RASTER (Drukker et al. ,99 Drukker et al. ,100 Bueno-de-Mesquita et al. 81 and Vliek et al. 101) is a prospective observational study in which LN0 (n = 427, 80% ER+, 84% HER2–) patients in the Netherlands were treated in accordance with MammaPrint plus usual clinical practice [2004 Dutch Institute of Healthcare Improvement (Central Accompagnement Organization) guidelines102 and clinician and patient preference]. The aims were to assess the impact of MammaPrint on treatment decisions and to prospectively record outcomes for patients categorised as being at high or low risk via MammaPrint, via clinical risk tools and for various combinations of MammaPrint risk and clinical risk. An additional analysis conducted retrospectively in LN+ patients (n = 164) was reported separately (Vliek et al. 103). Frozen tumour samples were used99 in the LN0 study and FFPE samples were used in the retrospective analysis of LN+ patients. 103 MammaPrint 70-gene microarray was used, stating that cut-off points were the same as in previous studies99 (see Report Supplementary Material 5, Table 13).
Quality assessment
Because RASTER was not a RCT, it was judged to be at a high risk of bias using standard RCT criteria (see Report Supplementary Material 5, Table 14). Prognostic results from this study are confounded by the differing rates of chemotherapy in different risk groups (usually more chemotherapy in the high-risk group than in the low-risk group).
Results for lymph node-negative patients
Results for MammaPrint (in conjunction with Central Accompagnement Organization guidelines and patient/clinician preference)
In LN0 patients (n = 427), 51% were at low risk (of whom 15% received chemotherapy) and 49% were at high risk (of whom 81% received chemotherapy). At 5 years, DRFI was 97.0% for low-risk patients and 91.7% for high-risk patients (p = 0.03 between groups, HR not reported) (Table 14). 99,100 The 10-year DRFI was 93.7% for low-risk and 86.8% for high-risk patients (HR 1.4, 95% CI 1.0 to 1.9). Results at 10 years were similar for the 342 ER+ patients, although not statistically significant (Vliek et al. 101) (see Table 14). The 5-year OS was not statistically significantly different between MammaPrint groups (p = 0.35, HR not reported) (see Report Supplementary Material 5, Table 15). 99,100
Study | Subgroup | Population: ET/chemotherapy | Nodal status | Outcome | Test or comparator | Percentage of patients per group | Percentage chemotherapy per group | Percentage DRFI risk: 0–5 years | Percentage DRFI risk: 0–10 years | HR (95% CI); p-value | |||||
---|---|---|---|---|---|---|---|---|---|---|---|---|---|---|---|
Low | High | Low | High | Low | High | Low | High | 0–5 years | 0–10 years | ||||||
Node negative | |||||||||||||||
LN0 |
All patients, n = 427 |
|
LN0 | DRFI | MammaPrint | 51 | 49 | 15 | 81 | 97.0 | 91.7 | 93.7 | 86.8 | p = 0.03 | 1.4 (1.0 to 1.9) |
AOL | 31 | 69 | 18 | 60 | 96.7 | 93.4 | NR | NR | p = 0.24 | NR | |||||
NPI | 58 | 42 | 21 | 84 | 96.7 | 91.3 | NR | NR | p = 0.03 | NR | |||||
Predict Plus (University of Cambridge, Cambridge, UK) | 53 | 47 | 20 | 78 | 96.8 | 91.7 | NR | NR | p = 0.004 | NR | |||||
mAOL | 57 | 43 | NR | NR | NR | NR | 91.7 | 88.2 | NR | 1.4 (0.8 to 2.6) | |||||
ER+ patients | |||||||||||||||
LN0 |
ER+ patients, n = 342 |
|
LN0 | DRFI | MammaPrint | 63 | 37 | NR | NR | NR | NR | 93.6 | 88.8 | NR | 1.6 (0.8 to 3.3) |
mAOL | NR | NR | NR | NR | NR | NR | 91.6 | 91.9 | NR | NR | |||||
High clinical risk | |||||||||||||||
LN0 |
AOL high, n = 295 |
|
LN0 | DRFI | MammaPrint | 42 | 58 | 24 | 87 | 98.4 | 89.8 | NR | NR | NR | NR |
NPI high, n = 179 | 25 | 75 | 57 | 93 | 95.5 | 89.9 | NR | NR | NR | NR | |||||
Predict Plus high, n = 199 | 25 | 75 | 41 | 91 | 93.9 | 91.0 | NR | NR | NR | NR | |||||
mAOL high, n = 183 | 25 | 75 | NR | NR | NR | NR | 90.9 | 87.3 | NR | NR | |||||
High clinical risk, untreated | |||||||||||||||
LN0 |
AOL high; no chemotherapy (n = 117) |
|
LN0 | DRFI | MammaPrint | 80 | 20 | 0 | 0 | 98.9 | NR | NR | NR | NR | NR |
AOL high; no ET/chemotherapy (n = 75) | 93 | 7 | 0 | 0 | 100.0 | NR | NR | NR | NR | NR | |||||
Low clinical risk | |||||||||||||||
LN0 |
AOL low, n = 132 |
|
LN0 | DRFI | MammaPrint | 72 | 28 | 3 | 57 | 95.3 | 100.0 | NR | NR | NR | NR |
NPI low, n = 248 | 71 | 29 | 5 | 59 | 97.4 | 95.3 | NR | NR | NR | NR | |||||
Predict Plus low, n = 228 | 75 | 25 | 8 | 57 | 98.0 | 93.9 | NR | NR | NR | NR | |||||
mAOL low, n = NR | NR | NR | NR | NR | NR | NR | 94.4 | 88.5 | NR | NR | |||||
Low clinical risk, untreated | |||||||||||||||
LN0 |
AOL low; no chemotherapy (n = 108) |
|
LN0 | DRFI | MammaPrint | 85 | 15 | 0 | 0 | 95.1 | NR | NR | NR | NR | NR |
AOL low; no ET/chemotherapy (n = 93) | 95 | 5 | 0 | 0 | 95.0 | NR | NR | NR | NR | NR |
Results for clinical risk tools
MammaPrint results were compared with the results of the NPI and Predict Plus clinical risk tools applied retrospectively to the data (see Tables 14 and 15). Results were similar to MammaPrint for proportions in low- and high-risk categories and for 5-year DRFI rates. Both NPI and Predict Plus showed a significant difference between groups (p = 0.03 and p = 0.004, respectively). 99,100
Study | Subgroup | Population | Nodal status | ET/chemotherapy | Outcome | Test or comparator | Percentage of patients per group | Percentage chemotherapy per group | C-index (AUC) | Increase in C-index (AUC) over clinicopathological factors | ||
---|---|---|---|---|---|---|---|---|---|---|---|---|
Low | High | Low | High | |||||||||
Node negative | ||||||||||||
RASTER99–101 | All patients, n = 427 |
|
LN0 |
|
5-year DRFI | MammaPrint | 51 | 49 | 15 | 81 | NR | |
AOL | 31 | 69 | 18 | 60 | 0.532 | |||||||
AOL plus MammaPrint | NR | NR | NR | NR | 0.619 | p = 0.03 | ||||||
NPI | 58 | 42 | 21 | 84 | 0.591 | |||||||
NPI plus MammaPrint | NR | NR | NR | NR | 0.638 | p = 0.05 | ||||||
Predict Plus | 53 | 47 | 20 | 78 | 0.627 | |||||||
Predict Plus plus MammaPrint | NR | NR | NR | NR | 0.662 | p = 0.27 |
Conversely, AOL categorised more patients as high risk compared with MammaPrint, NPI or Predict Plus, (69% compared with 49%, 42% and 47%, respectively). High-risk AOL patients had a lower chemotherapy rate (60% compared with 81%, 84% and 78%, respectively), and 5-year DRFI was similar for the low-risk group (96.7% compared with 97.0%, 96.7 and 96.8%, respectively) but was higher in the high-risk group (93.4%, compared with 91.7%, 91.3% and 91.7%, respectively). The difference between AOL low- and high-risk groups was p = 0.24 (see Table 14). 99,100 mAOL categorised similar numbers of patients as being at high risk and low risk as MammaPrint, NPI and Predict Plus. The 10-year DRFI for mAOL was more favourable for the low-risk group than the high-risk group, but this was not statistically significant (HR 1.4, 95% CI 0.8 to 2.6) and any difference was lost when restricting to ER+ patients (see Table 14 and Vliek et al. 101).
MammaPrint results for patients at high/low clinical risk
Results by MammaPrint risk group for patients at a high or low clinical risk in accordance with NPI, Predict Plus and AOL are also presented. The results are presented in detail in Report Supplementary Material 5. In summary, observed 5-year DRFI rates were lower in patients with high NPI risk/low MammaPrint risk and in patients with high Predict Plus risk/low MammaPrint risk (5-year DRFI rates 95.5% and 93.9%, respectively) than in the whole MammaPrint low-risk group (5-year DRFI rate 97.0%), and were similar in the high-clinical-risk/high-MammaPrint-risk groups (5-year DRFI rates 89.9% and 91.0%, respectively) compared with a 5-year DRFI rate of 91.7% in the MammaPrint high-risk group. AOL classified more patients as being at high risk than NPI and Predict Plus and had a 5-year DRFI of 90.6%; a higher proportion of these patients were reclassified by MammaPrint as being at low risk.
Additional prognostic value of MammaPrint
Table 15 shows C-indices (AUC) for clinical risk tools only and in addition to MammaPrint. The addition of MammaPrint to AOL or NPI statistically significantly increased the C-index (AUC) (p = 0.03 and p = 0.05, respectively), whereas the addition of MammaPrint to Predict Plus did not statistically significantly increase the C-index (AUC) (p = 0.27) (see Table 15). 100
Results for lymph node-positive patients
MammaPrint was retrospectively conducted in 164 LN+ patients (Table 16). Over 95% of patients received chemotherapy. MammaPrint categorised 48% of LN1–3 patients as low risk. The 5-year DRFI was 98.4% for low-risk and 86.9% for high-risk patients, whereas 10-year DRFI was 94.9% for low-risk and 80.7% for high-risk patients, showing a statistically significant difference between groups (HR 4.7, 95% CI 1.3 to 16.2). A comparison was made with the mAOL, although this analysis included 30 additional patients with LN > 3 who were automatically classed as high risk. The mAOL categorised only 14% of patients as being at low risk; 10-year DRFI was 94.4% for low-risk and 85.8% for high-risk patients, which was not statistically significantly different (HR 3.7, 95% CI 0.5 to 28.5). Within the mAOL high-risk group, 10-year DRFI was statistically significantly better in MammaPrint low-risk (95.2%) than high-risk (79.6%) patients (HR 4.8, 95% CI 1.1 to 21.4). 101
Study (first author and year) | Subgroup | Population: ET/chemotherapy | Nodal status | Outcome | Test or comparator | Percentage of patients per group | Percentage chemotherapy per group | Percentage DRFI risk | HR (95% CI), p-value | ||||||
---|---|---|---|---|---|---|---|---|---|---|---|---|---|---|---|
0–5 years | 0–10 years | ||||||||||||||
Low | High | Low | High | Low | High | Low | High | 30–5 years | 0–10 years | ||||||
Node positive | |||||||||||||||
RASTER LN+, Vliek 2017101 | LN1–3, n = 134 |
|
LN1–3 | DRFI | MammaPrint | 48 | 52 | NR | NR | 98.4 | 86.9 | 94.9 | 80.7 | NR | 4.7 (1.3 to 16.2); p = 0.008 |
All (three were missing data), n = 161 |
|
|
mAOL | 14 | 86 | NR | NR | 100 | 90.8 | 94.4 | 85.8 | NR | 3.7 (0.5 to 28.5); p = 0.173 | ||
High clinical risk | |||||||||||||||
RASTER LN+, Vliek 2017101 | mAOL high risk, n = 109 |
|
LN+ | DRFI | MammaPrint | 40 | 60 | NR | NR | 97.7 | 86.1 | 95.2 | 79.6 | NR | 4.8 (1.1 to 21.4) |
Conclusions: observational study of clinical utility for MammaPrint (RASTER)
RASTER is a prospective observational study in which patients were treated in accordance with MammaPrint plus usual clinical practice (LN0) or in accordance with usual clinical practice (LN+). The 5-year DRFI for LN0 patients was 97.0% for low-risk patients (15% had chemotherapy) and 91.7% for high-risk patients (81% received chemotherapy). The 10-year DRFI for LN0 patients was 93.7% for low-risk and 86.8% for high-risk patients. The DRFI rates in the MammaPrint low-risk group may be considered sufficiently low for these patients to avoid chemotherapy. MammaPrint provided additional prognostic information over AOL and NPI, but not over Predict Plus when considering C-indices. Estimates of prognostic performance between risk groups are likely to be affected by the differing rates of chemotherapy in each group and the fact that chemotherapy use was influenced by MammaPrint.
Results: Prosigna
A description of Prosigna and its development is given in Report Supplementary Material 6. A summary of the key results from the review is provided in this section. A detailed narrative synthesis of all study results is also provided in Report Supplementary Material 6.
Prosigna is based on a ROR score called ROR-PT, which incorporates the PAM50 gene signature, a weighting for a proliferation score (P, a subset of the 50 genes) and information on tumour size (T). Nodal status is then used when converting the score into a risk category. The results for the commercial test using nCounter and for research-based versions using other methods (e.g. RT-qPCR) are reported here. Studies assessing ROR-PT via whole-transcriptome microarray are summarised in Report Supplementary Material 10. ROR-S (subtype), ROR-T/ROR-C (subtype and tumour size) and ROR-P (subtype and proliferation score) were outside the scope of this review. Within this section, the test is referred to as ROR-PT.
Prognostic performance: Prosigna
Study designs: Prosigna prognostic performance
Eight data sets were used to assess the prognostic performance of ROR-PT (see Report Supplementary Material 6, Table 1). These included six reanalyses of RCTs [TransATAC,38,46 Austrian Breast and Colorectal Cancer Study Group (ABCSG) 8,104,105 Cancer and Leukemia Group B (CALGB 9741),106 National Cancer Institute of Canada (NCIC) MA.21,107 Grupo Español de Investigación en Cáncer de Mama (GEICAM) 9906108,109 and NCIC MA.12110] and two retrospective analyses of prospective cohorts [the Danish Breast Cancer Cooperative Group (DBCG) cohort111–114 and two analyses of the British Columbia cohort115,116].
Patients: Prosigna prognostic performance
Two of the RCTs (TransATAC38,46 and ABCSG 8;104,105 total n = 2252) and the two retrospective analyses (total n = 3508) included patients who were all/mostly ER+ and HER2– and received endocrine monotherapy. The other four RCTs106–110 (total n = 3358) included higher-risk patients (not restricted to ER+ and HER2–, higher proportion LN+) and all received chemotherapy (see Report Supplementary Material 6, Table 1). Two studies recruited only LN+ patients (CALGB 9741106 and GEICAM 9906108,109), one recruited LN0 patients (DBCG111–114) and the remainder recruited both LN0 and LN+ patients.
Tests and comparators: Prosigna prognostic performance
Four analyses of RCTs38,46,104–107 and two analyses of prospective cohorts111–115 measured ROR-PT using the nCounter device, and two analyses of RCTs108–110 and one of a prospective cohort116 used RT-qPCR (see Report Supplementary Material 6, Table 1). The cut-off points used to define risk groups varied across studies, and some analyses assessed ROR-PT as a continuous score (see Report Supplementary Material 6, Table 1).
Quality assessment: Prosigna prognostic performance
See Report Supplementary Material 6, Table 1. All analyses excluded some patients recruited to the original trial or cohort. Blinding of test assessors to outcomes was reported in five analyses. All used standardised outcomes.
Results: Prosigna prognostic performance
Distribution of patients by risk group
For LN0 patients, the percentages categorised as low risk were reported in two analyses: 55% in TransATAC46 and 48% in ABCSG 8. 104,105 Among LN+ patients, far fewer patients were categorised as low risk: 8% in TransATAC,46 4% in ABCSG 8,104,105 19% in GEICAM 9906108,109 and 25% in DBCG. 112 The percentages of patients categorised as intermediate risk were 30%46 and 32%104,105 in LN0 patients and ranged from 27% to 56% in LN+ patients. 46,104,105,108,109,112 The number of patients who are likely to be prescribed chemotherapy on the basis of their test result will depend on how intermediate-risk patients are handled and whether or not they would be handled in the same way in LN0 and LN+ groups.
Prognostic performance: unadjusted analyses
For LN0 patients, ROR-PT was statistically significantly prognostic for DRFS/DFMS/DRFI in all three data sets (TransATAC,46 ABCSG 8104,105 and DBCG112). HRs and p-values between groups are reported in many differing formats and time points so are summarised in Table 17 rather than in the text. ROR-PT was also statistically significantly prognostic for late (5- to 15-year) recurrence in the one study reporting this. 104,105
Reference (first author and year) | Cohort, design, country | Population | Nodal status | ET/chemotherapy | Test | Percentage of patients per group | Percentage DRFS/DRFI risk | DMFS/DRFS:a HR (95% CI) | |||||||
---|---|---|---|---|---|---|---|---|---|---|---|---|---|---|---|
0–5 years | 0–10 years | ||||||||||||||
Low | Intermediate | High | Low | Intermediate | High | Low | Intermediate | High | |||||||
LN status mixed | |||||||||||||||
100% ET monotherapy | |||||||||||||||
Sestak 2017 (data request)46 (reduced data set)b | TransATAC, R-RCT, UK |
ER+, HER2– n = 774 |
LN0, 76% LN1–3, 24% |
All ET, no chemotherapy | ROR-PT, nCounter | 43 | 30.5 | 26.5 | NR | NR | NR | NR | NR | NR | 0–10 years:
|
Gnant 2014,104 Filipits 2014105 | ABCSG 8, R-RCT, Austria |
ER+, HER2– n = 1397 |
LN0, 71%c LN1–3, 26%c LN> 3, 3%c |
All ET, no chemotherapy | ROR-PT, nCounter | 35 | 32 | 33 | NR | NR | NR | 96.6 | 91.1 | 79.9 | 5–15 years: |
Laenkholm 2015,112 2015113 | DBCG, cohort, Denmark |
HR+, HER2 NR n = 2722 |
LN0, 46% LN1–3, 54% |
All ET, no chemotherapy | ROR-PT | 27 | 29 | 44 | NR | NR | NR | 95.7 | – | 79.2 | 5–10 years:
|
LN0 | |||||||||||||||
100% ET monotherapy | |||||||||||||||
Sestak 2017 (data request)46 (full data set)b | TransATAC, R-RCT, UK |
ER+, HER2– n = 663 |
LN0 | All ET, no chemotherapy | ROR-PT, nCounter | 55 | 30 | 15 | 98.6 | 95.9 | 87.9 | 97.4 | 86.6 | 69.0 | 0–5 years:
|
Gnant 2014,104 Filipits 2014105 | ABCSG 8, R-RCT, Austria |
ER+, HER2– n = 984 |
LN0 | All ET, no chemotherapy | ROR-PT, nCounter | 48 | 32 | 20 | NR | NR | NR | 96.5 | 90.0 | 84.7 | 5–15 years: |
Laenkholm 2015112 | DBCG, cohort, Denmark |
HR+, HER2 NR n = 1256 |
LN0 | All ET, no chemotherapy | ROR-PT | NR | NR | NR | NR | NR | NR | 95.1 | 92.7 | 81.5 | 0–10 years:
|
LN+ | |||||||||||||||
100% ET monotherapy | |||||||||||||||
Sestak 2017 (data request)46 (full data set)b | TransATAC, R-RCT, UK |
ER+, HER2– n = 192 |
LN1–3 | All ET, no chemotherapy | ROR-PT, nCounter | 8 | 32 | 60 | 100.0 | 91.7 | 87.4 | 100.0 | 80.7 | 70.7 | 0–5 years:
|
Gnant 2014,104 Filipits 2014105 | ABCSG 8, R-RCT, Austria |
ER+, HER2– n = 413 |
All ET, no chemotherapy | ROR-PT, nCounter | 4 | 34 | 62 | NR | NR | NR | 100 | 93.6 | 76.2 | 5–15 years:
|
|
Laenkholm 2015112 | DBCG, cohort, Denmark |
HR+, HER2 NR n = 1466 |
LN1–3 | All ET, no chemotherapy | ROR-PT | 25 | 27 | 48 | NR | NR | NR | 95.2 | 78.1 | 0–10 years:
|
|
100% chemotherapy and ET | |||||||||||||||
Martin 2016,108 2014109 | GEICAM 9906, R-RCT, Spain |
ER+, HER2– n = 536 |
|
All ET, no chemotherapy | ROR-PT (research) | 19 | 56 | 26 | NR | NR | NR | 92 | 74 | 66 | 0–10 years:
|
For LN+ patients, ROR-PT was statistically significantly prognostic for 10-year DRFS/DFMS/DRFI in all four data sets (TransATAC,46 ABCSG 8,104,105 DBCG112 and GEICAM 9906108,109). ROR-PT was also statistically significantly prognostic for late (5- to 10-year) recurrence in the two studies reporting this. 104,105,112,113
Other outcomes and subgroups (pre/post menopausal, ductal/lobular cancer) are reported in Report Supplementary Material 6.
Additional prognostic value
In terms of additional prognostic value (Table 18), multivariable analyses of two data sets (ABCSG 8 and DBCG)104,111,112 showed that ROR-PT was an independent prognostic parameter for 10-year DMFS/DRFS after adjustment for clinicopathological variables across LN0/LN+ and LN+ patients. Two data sets (TransATAC46 and ABCSG 8104) reported an increase in likelihood ratio χ2 for ROR-PT plus CTS/clinical linear predictor (CLP)/NPI over CTS/CLP/NPI only; this increase was statistically significant in LN0 and LN+ patients in ABCSG 8,104 and in TransATAC the increase was statistically significant in LN0 patients and borderline significant in LN+ patients. 46 One study reported an increase in likelihood ratio χ2 (p < 0.0001) for ROR-PT over clinicopathological variables in DBCG, a mixed cohort of LN+/LN0 patients. 112
Reference (first author and year) | Cohort | Population | Nodal status | ET/chemotherapy | Outcome | Test or comparatora | Likelihood ratio χ2 | Increase in likelihood ratio χ2 over clinicopathological factorsa | C-index (AUC) | Increase in C-index (AUC) over clinicopathological factorsa | Multivariable model (adjuvant for clinicopathological factorsa): HR (95% CI) |
---|---|---|---|---|---|---|---|---|---|---|---|
LN status mixed | |||||||||||
100% ET monotherapy | |||||||||||
Sestak 2017 (data request)46 (reduced data set)b | TransATAC, R-RCT |
ER+, HER2– n = 774 |
|
All ET, no chemotherapy | 10-year DRFI | ROR-PT, nCounter | 61.47 (p < 0.0001) |
Over CTS: 26.30 (p < 0.001) Over NPI: 23.91 (p < 0.0001) |
|||
Gnant 2014,104 Filipits 2014105 | ABCSG 8, R-RCT |
ER+, HER2– n = 1397 |
All ET, no chemotherapy | 10-year DRFS | ROR-PT, nCounter | 29.94 (p < 0.0001) | 0.720 | NR |
Low vs. intermediate: 2.15 (1.21 to 3.81); p = 0.009 Low vs. high: 4.26 (2.44 to 7.43); p < 0.0001 |
||
CLP | 0.688 | ||||||||||
Laenkholm 2015112 | DBCG, cohort |
HR+, HER2 NR n = 2722 |
|
All ET, no chemotherapy | 10-year DRFS | ROR-PT, nCounter | p < 0.0001 | HR (20-point change in ROR): 1.7 (1.5 to 1.9) | |||
LN0 | |||||||||||
100% ET monotherapy | |||||||||||
Sestak 2017 (data request)46 (reduced data set)b | TransATAC, R-RCT |
ER+, HER2– n = 591 |
LN0 | All ET, no chemotherapy | 10-year DRFI | ROR-PT, nCounter | 50.77 (p < 0.0001) |
Over CTS: 23.71 (p < 0.0001) Over NPI: 25.54 (p < 0.0001) |
|||
Gnant 2014,104 Filipits 2014105 | ABCSG 8, R-RCT |
ER+, HER2– n = 984 |
LN0 | All ET, no chemotherapy | 10-year DRFS | ROR-PT, nCounter | Over CLP: 20.32 (p < 0.0001) | 0.692 | NR | ||
CLP | 0.639 | ||||||||||
Wallden 2015115 | British Columbia, cohort |
ER+, 91%; HER2– n = 232 |
LN0 | All ET, no chemotherapy | DRFS (time NR) | ROR-PT, nCounter | 0.675d | NR | |||
AOL | 0.587d | NR | |||||||||
IHC-T | 0.590d | NR | |||||||||
LN+ | |||||||||||
100% ET monotherapy | |||||||||||
Sestak 2017 (data request)46 (reduced data set)b | TransATAC, R-RCT, UK |
ER+, HER2– n = 183 |
LN1–3 | All ET, no chemotherapy | 10-year DRFI | ROR-PT, nCounter | 8.51 (p = 0.004) |
Over CTS: 4.39 (p = 0.04) Over NPI: 2.71 (p = 0.09) |
|||
Gnant 2014,104 Filipits 2014105 | ABCSG 8, R-RCT |
ER+, HER2– n = 413 |
All ET, no chemotherapy | 10-year DRFS | ROR-PT, nCounter | Over CLP: 17.45 (p = 0.0002) | 0.743 | NR | |||
CLP | 0.667 | ||||||||||
Ejlertsen 2015111 | DBCG, cohort |
HR+, HER2 NR n = 1466 |
LN1–3 | All ET, no chemotherapy | 10-year DMFS | ROR-PT, nCounter |
|
||||
100% chemotherapy and ET | |||||||||||
Martin 2016,108 2014109 | GEICAM 9906, R-RCT |
ER+, HER2– n = 536 |
|
All ET, no chemotherapy | 10-year DMFS | ROR-PT, RT-qPCR (research) | 0.644 | Adding ROR-PT to EPClin plus clinicopathological: p = 0.567 |
Conclusions: Prosigna prognostic performance
Based on six reanalyses of RCTs and two retrospective analyses of prospective cohorts, Prosigna/ROR-PT was statistically significantly prognostic for unadjusted analyses of 10-year DRFS/DRFI in LN0 and LN+ patients. Among LN0 patients, approximately 50% were categorised as low risk, 30% as intermediate risk and 15% to 20% as high risk. Among LN+ patients, 4% to 25% were at low risk, 27% to 56% were at intermediate risk and 26% to 62% were at high risk. The 10-year DRFS/DRFI rates for low-risk patients were 95% to 97% in three studies of LN0 patients (all endocrine therapy only), and in LN+ patients these were 100% in two studies (endocrine therapy only) and 92% in one study (all endocrine therapy and chemotherapy). ROR-PT added prognostic information over clinicopathological variables or CTS/CLP/NPI in three studies; this was statistically significant in LN0 patients and either significant or borderline significant in LN+ patients.
Results: EndoPredict and EndoPredict Clinical
Development: EndoPredict and EndoPredict Clinical
EndoPredict and EPClin risk scores were trained on 964 ER+, HER2– endocrine-treated samples (65% node negative) from a range of sources (Filipits et al. 117). EndoPredict generates an EndoPredict score based on the gene signature alone. The EPClin score is calculated from the EndoPredict score plus information on tumour size and nodal status.
Prognostic performance: EndoPredict and EndoPredict Clinical
Study characteristics
The prognostic value of EPClin was assessed in three reanalyses of RCTs,36,46,108,109,118–120 which included four RCTs (total n = 3135): UK patients from TransATAC (n = 878), the ABCSG 6 and ABCSG 8 (total n = 1702) and the Spanish GEICAM 9906 trial (n = 555). All recruited only, or reported a subgroup of, patients who were ER+ and HER2–. One reported on LN0 patients (total n = 680)36,39,46 and two36,46,108,109 on LN+ patients (total n = 753; one108,109 included 36% patients with at least three positive nodes). One reported on patients unselected by lymph node status,120 and one on both LN0 and LN1–3 together;46 additional analyses118 were provided to the EAG as commercial-in-confidence data and cannot be reported here. Patients received endocrine monotherapy in two trials36,46,120 and all patients received endocrine therapy and chemotherapy in the GEICAM trial. 108,109 All excluded some original trial participants (or this was unclear). A detailed narrative synthesis of study characteristics is provided in Report Supplementary Material 7. This supplement also contains relevant data in tables.
Quality assessment
The EAG’s assessment of study quality is provided in Report Supplementary Material 7, Table 2. All analyses excluded some original trial patients (or this was unclear), sometimes owing to insufficient tumour samples, which may introduce bias owing to attrition of patients with smaller tumours. Blinding of test assessors to outcomes was reported in two analyses. 36,108,109 All used standardised outcomes. A discussion of how these factors might affect results is given in Results: oncotype DX.
Results
A summary of key results from the review is provided in this section. A detailed narrative synthesis of all study results is provided in Report Supplementary Material 7.
Distribution of patients by risk group
Data are presented in Table 19. The percentage of LN0 patients categorised as low risk in accordance with EPClin was 73%46 and the percentage categorised as high risk was 27%. 46 For LN+ patients,36,46,108,109 the percentages categorised as low risk were 13%108,109 and 24%46 and the percentages categorised as high risk were 87%108,109 and 76%. 46
Reference (first author and year) | Cohort, design, country | Population | Nodal status | ET/chemotherapy | Test or comparator | Percentage of patients per group | Percentage DRFS/DRFI risk | DMFS/DRFS/DRFI:a HR (95% CI) | ||||
---|---|---|---|---|---|---|---|---|---|---|---|---|
0–5 years | 0–10 years | |||||||||||
Low | High | Low | High | Low | High | |||||||
Reanalyses of RCTs: LN status mixed | ||||||||||||
100% ET monotherapy | ||||||||||||
Sestak 2017 (data request)46 (reduced data set)b | TransATAC, R-RCT, UK |
ER+, HER2– n = 774 |
|
All ET, no chemotherapy | EPClin | 61 | 39 | NR | NR | NR | NR | 0–10 years: 4.65 (2.98 to 7.24) |
Dubsky 2013,120 2013119 | ABCSG-6 plus ABCSG 8, R-RCT, Austria |
ER+, HER2– n = 1702 |
|
All ET, no chemotherapy | EndoPredict | 49 | 51 | NR | NR | NR | NR |
|
EPClin | 63 | 37 | NR | NR | 95.3 | NR |
|
|||||
Reanalyses of RCTs: LN0 | ||||||||||||
100% ET monotherapy | ||||||||||||
Sestak 2017 (data request)46 (full data set)b | TransATAC, R-RCT, UK |
ER+, HER2– n = 680 |
LN0 | All ET, no chemotherapy | EPClin | 73 | 27 | 97.9 | 92.2 | 94.1 | 80.0 |
|
Reanalyses of RCTs: LN+ | ||||||||||||
100% ET monotherapy | ||||||||||||
Sestak 2017 (data request)46 (full data set)b | TransATAC, R-RCT, UK |
ER+, HER2– n = 198 |
LN1–3 | All ET, no chemotherapy | EPClin | 24 | 76 | 97.9 | 87.6 | 95.0 | 71.6 |
|
100% chemotherapy and ET | ||||||||||||
Martin 2016,108 2014109 | GEICAM 9906, R-RCT, Spain |
ER+, HER2– n = 555 |
|
All ET, no chemotherapy | EndoPredict | 25 | 75 | NR | NR | 93 | 70 | 0–10 years: 4.8, (2.5 to 9.6); p < 0.0001 |
EPClin | 13 | 87 | NR | NR | 100 | 72 | 0–10 years: not estimable, p < 0.0001 | |||||
Premenopausal, n = 300 | NR |
|
EndoPredict | 24 | 76 | 93 | 67 | 0–10 years: 6.7 (2.4 to 18.3); p < 0.0001 | ||||
Postmenopausal, n = 255 | NR | EndoPredict | 27 | 73 | 92 | 74 | 0–10 years: 3.3 (1.3 to 8.5); p = 0.0069 | |||||
Premenopausal, n = 300 | NR |
|
EPClin | 12 | 88 | 100 | 70 | 0–10 years: HR NR, p = 0.0006 | ||||
Postmenopausal, n = 255 | NR | EPClin | 13 | 87 | 100 | 76 | 0–10 years: HR NR, p = 0.0023 | |||||
High/intermediate risk via clinical guidelines (LN0/LN+) | ||||||||||||
Dubsky 2013120 | ABCSG-6 plus ABCSG 8, R-RCT, Austria | NCCN, n = 1603 | LN+/LN0 (% NR) | All ET, no chemotherapy | EPClin | 61 | 39 | NR | NR | 95 | 77 | 0–10 years: 5.09 (3.42 to 7.58); p < 0.001 |
St Gallen, n = 1358 | EPClin | 58 | 42 | NR | NR | 95 | 75 | 0–10 years: 5.18 (3.38 to 7.93); p < 0.001 | ||||
S3c, n = 1454 | EPClin | 58 | 42 | NR | NR | 95 | 76 | 0–10 years: 5.60 (3.64 to 8.61); p < 0.001 |
Prognostic performance: unadjusted analyses
Data are presented in Table 19. EPClin was a statistically significantly prognostic for DRFS/DRFI for all unadjusted analyses at 10 years (and most analyses at 5 years) in LN0 and LN+ patients46,108,109,120 and in one analysis of patients at high clinical risk. 120 The rate of 10-year DRFS/DRFI was 94% for EPClin low-risk LN0 patients46 and 95% for LN+ patients46,118–120 in one study in which patients had endocrine monotherapy46 and 100% in one study using endocrine therapy and chemotherapy. 108,109 Use of chemotherapy in the GEICAM study108,109 could influence patient outcomes in either direction: negatively owing to the potential selection of higher-risk patients or positively owing to the effect of chemotherapy. The HR in LN0 patients for the low- versus high-risk groups was 3.90 (95% CI 2.33 to 6.53; p-value not reported) in TransATAC. 46 HRs in LN+ patients for the low- versus high-risk groups were 6.77 (95% CI 1.63 to 28.07; p-value not reported) in TransATAC,46 and were not estimable for GEICAM because there were no events in the low-risk group (p < 0.0001). 108,109 Report Supplementary Material 7 provides data comparing EPClin with clinical guidelines and a subgroup analysis of patients at high clinical risk.
Additional prognostic value: adjusted analyses
Data are presented in Table 20. In terms of additional prognostic value, TransATAC reported statistically significant increases in likelihood ratio χ2 for 10-year DRFI for EPClin plus CTS/NPI over CTS/NPI only, in both LN0 and LN+ patients [LN0 patients 15.22 (p < 0.0001) over CTS and 17.00 (p < 0.0001) over NPI; LN+ patients 7.36 (p = 0.007) over CTS and 5.57 (p = 0.02) over NPI]. 46 Two further studies reported that the EndoPredict score added statistically significant information over clinicopathological variables in LN+ and mixed LN0/LN+ patients [based on multivariable analyses and differences in C-index (AUC) for 10-year DMFS/DRFI]; however, neither study reported the additional prognostic value of EPClin. 108,109,118–120
Reference (first author and year) | Cohort, design, country | Population | Nodal status | ET/chemotherapy | Outcome | Test or comparatora | Likelihood ratio χ2 | Increase in likelihood ratio χ2 over clinicopathological factors | C-index (AUC) | Increase in C-index (AUC) over clinicopathological factorsa | Multivariable model (adjuvant for clinicopathological factorsa): HR (95% CI) |
---|---|---|---|---|---|---|---|---|---|---|---|
Reanalyses of RCTs: LN status mixed | |||||||||||
100% ET monotherapy | |||||||||||
Sestak 2017 (data request)46 (reduced data set)b | TransATAC, R-RCT, UK |
ER+, HER2– n = 774 |
|
All ET, no chemotherapy | 10-year DRFI | EPClin | 69.31 (p < 0.0001) |
|
|||
Dubsky 2013,120 2013119 | ABCSG 6 plus ABCSG 8, R-RCT, Austria |
ER+, HER2– n = 1702 |
|
All ET, no chemotherapy | 0- to 5-year DMFS | EndoPredict | 1.20 (1.10 to 1.31); p < 0.001 | ||||
EPClin | |||||||||||
5- to 10-year DMFS | EndoPredict | 1.28 (1.10 to 1.48); p = 0.001 | |||||||||
EPClin | 0.786 | ||||||||||
EndoPredict vs. AOL | 0.765 | p < 0.001 | |||||||||
EndoPredict vs. clinicopathologicala | 0.716 | p < 0.001 | |||||||||
AOL | 0.674 | ||||||||||
Clinicopathological factorsa | 0.644 | ||||||||||
Reanalyses of RCTs: LN0 | |||||||||||
100% ET monotherapy | |||||||||||
Sestak 2017 (data request)46 (reduced data set)b | TransATAC, R-RCT, UK |
ER+, HER2– n = 591 |
LN0 | All ET, no chemotherapy | 5-year DRFI | EPClin | 16.62 (p < 0.0001) |
|
|||
10-year DRFI | 40.60 (p < 0.0001) |
|
|||||||||
Reanalyses of RCTs: LN+ | |||||||||||
100% ET monotherapy | |||||||||||
Sestak 2017 (data request)46 (reduced data set)b | TransATAC, R-RCT, UK |
ER+, HER2– n = 183 |
LN1–3 | All ET, no chemotherapy | 5-year DRFI | EPClin | 4.24 (p < 0.039 |
|
|||
10-year DRFI | 12.91 (p < 0.001) |
|
|||||||||
100% chemotherapy and ET | |||||||||||
Martin 2016,108 2014109 | GEICAM 9906, R-RCT, Spain |
ER+, HER2– n = 536 |
|
All ET, all chemotherapy | 10-year DMFS | EPClin | 0.693 | NR | |||
EndoPredict vs. clinicopathologicala | 0.672 | p = 0.0018 | |||||||||
EndoPredict | 0.657 | 1.1 (1.0 to 1.2); p = 0.003 | |||||||||
Clinicopathological factorsa | 0.654 |
Conclusions: EndoPredict and EndoPredict Clinical prognostic performance
Based on three reanalyses of RCTs (total n = 3135) in ER+, HER2–, endocrine-treated patients, EPClin was statistically significantly prognostic for unadjusted analyses of 10-year DRFS/DRFI in LN0 and LN+ patients. The percentage of patients categorised as EPClin low risk was 73% for LN0 patients and was 13% and 24% for LN+ patients. The 10-year DRFS/DRFI rates for low-risk patients were approximately 95% in LN0 and LN+ patients receiving endocrine therapy only. EPClin added statistically significant information over CTS/NPI in LN0 and LN+ patients in TransATAC, and in two further studies the EndoPredict score added statistically significant information over clinicopathological variables in mixed LN0/LN+ and LN+ patients (no data for EPClin). There was no evidence relating to chemotherapy benefit or clinical utility for EndoPredict or EPClin.
Results: IHC4
Development and analytic validity: IHC4
A narrative synthesis and data tables relating to the development of IHC4 and IHC4+C are provided in Report Supplementary Material 8. Although derivation data sets were excluded from the review for the other tests, for IHC4 the derivation was conducted in the TransATAC data set and is included in our tables to facilitate comparisons between tests. It should be noted, however, that estimates from TransATAC for IHC4 are likely to be an overestimate of true prognostic performance owing to overfitting.
Analytic validity
A rapid review of the analytic validity of IHC4 can be found in Report Supplementary Material 8.
Prognostic performance: IHC4 and IHC4+C
Eleven separate validation cohorts24,25,58,73,74,77,122–129 have reported prognostic performance data for IHC4, with a total of 13,434 patients.
Study designs, patients and treatments: IHC4 prognostic performance
Five cohorts [Tamoxifen vs Exemestane Adjuvant Multinational (TEAM), the WSG PlanB, Intergroup Exemestane Study (IES), GEICAM 9906 and West German Study Group epirubicine and cyclophosphamide-Doc (WSG-AGO-Doc)]24,73,74,77,122,123,126,127,129 were reanalyses of RCT data (three LN+/LN0 studies, n = 8496; no LN0 studies; two LN+ studies, n = 1705) and six25,58,124–126,128 were reanalyses of routinely collected data when patients were treated in accordance with usual practice without the use of IHC4 or IHC4+C (five LN+/LN0 studies, n = 3128; one LN0 study, n = 105; no LN+ studies). All studies recruited HR+ or ER+ patients except the IES RCT127 and the study from Taiwan. 124 TransATAC,46 WSG PlanB,73,74,77 GEICAM 9906,129 WSG-AGO-Doc,123 the Kaiser Permanente cohort,125 the Institut Curie128 cohort and the Chinese58 cohort all recruited or reported a subgroup of HER2– patients. Only one validation cohort (Stephen et al. 126) treated 100% of patients with endocrine monotherapy, and the remainder treated varying proportions of patients with endocrine therapy and chemotherapy. Two observational studies were from East Asia;58,124 clinical advice received by the EAG suggests that these two East Asian studies may be less generalisable to the English context because (1) patients were treated in accordance with usual clinical practice and this may differ between these countries and England enough to affect prognostic outcomes and (2) it is possible that people of different ethnicities have different underlying risk profiles and disease natural histories.
A detailed narrative synthesis of patient characteristics and treatments is provided in Report Supplementary Material 8. Overall, only the derivation cohort (TransATAC)46 reported an analysis of 100% ER+, HER2–, LN0–3 patients who had not undergone chemotherapy but had received 5 years of endocrine therapy. For this reason, most of the evidence base has low generalisability to the decision problem.
IHC4 methodology and cut-off points: IHC4 and IHC4+C prognostic performance
The methodology for conducting IHC4 is not standardised outside the Royal Marsden Hospital. Report Supplementary Material 8 details the methods reported in the included studies, and a judgement provided by personal communication with the IHC4 methodologists [Andrew Dodson, National External Quality Assessment Service (UK), personal communication, September 2017] regarding whether or not the methods used are compatible with their own method is provided in Report Supplementary Material 8 and Table 21. Seven data sets were analysed using IHC4 methodologies that were the same as or very similar to the IHC4 team’s own methodology (referred to from here on in as the ‘standard IHC4 methodology’) [TransATAC,46 TEAM,24,122,126 the Nottingham cohort,25 the Edinburgh Breast Conservation Series (BCS) cohort,126 the Institut Curie128 cohort, GEICAM 9906129 and WSG-AGO-Doc123], whereas the remaining five data sets were analysed using methodologies that were unclear or dissimilar to the IHC4 team’s methods (WSG PlanB,73,74,77 the Kaiser Permanente cohort,125 IES,127 the Chinese cohort58 and the Taiwanese cohort124). Results have not been excluded by IHC4 methodology, as methodologies are not currently standardised and, for this reason, all data are of some relevance.
Reference (first author and year) | Cohorts (country) | Study design | Details of testa | Compatibilityb and algorithm | Population | Nodal status | ET/chemotherapy |
---|---|---|---|---|---|---|---|
Subgroup, relevant to the decision problem, of derivation cohort: LN0/LN+ | |||||||
TransATAC, Sestak46 n = 1005c |
TransATAC (UK) | R-RCT |
FFPE. Biomarker expression was measured by IHC. HER2 was confirmed by FISH if ≥ IHC2+. ER used 6F11 antibody (Vector Laboratories, Burlingame, CA, USA), PgR used diluted 1 : 40, clone 16 (Vector Laboratories) and Ki-67 used the diluted 1 : 100, or SP6 antibody (Abcam, Cambridge, MA, USA) diluted 1 : 100. ER positive if H > 1; PR scored as % positive cells; HER2 by manufacturer’s instructions; Ki-67 using Ariol image system (Genetix, San Jose, CA, USA) Similar methods and scoring algorithms were used for the Nottingham cohort, except that the MiB1 antibody was used on whole sections for Ki-67, and TMAs were used for ER, PgR and HER2 |
|
|
|
100% ET monotherapy |
Validation cohorts: LN0/LN+ | |||||||
Bartlett 201624 (n = 2919) Christiansen 2012122 (n = 4598) |
TEAM (UK/Ireland, the Netherlands, Belgium, Germany, Greece) | R-RCT |
FFPE samples Ariol SL50 image platform Staining as per Bartlett et al. 2011130 Scoring as per Faratian et al. 2007131 Scores normalised |
|
|
LN0/+, % NR | 100% ET, some chemotherapy,% NR130 |
Cuzick 201125 n = 786 | Nottingham (UK) | R-RD | As TransATAC46 |
|
|
|
52% ET, % chemotherapy NR |
Nitz 2017,77 Gluz 2016,73 Gluz 201674 n = 2642 55-month follow-up |
WSG PlanB (Germany) | R-RCT | Tissue microarrays (1.4-mm diameter): ER [Rabbit (SP1)], PR (mouse monoclonal PgR636) and Ki-67 (clone 30–9 rabbit monoclonal). ER and PR positive if ≥ 1% stained. Ki-67 scored by one expert, > 100 cells, semi-quantitatively and quantitatively. FISH for HER2 (unclear if confirmatory). Instead of H-score a modified score was used as described in Prat et al. 2013129 |
|
|
RS < 12 ET only; RS ≥ 12, chemotherapy plus ETe | |
Rohan, 2014125 n = 295 (147 cases; 148 controls)f | Kaiser Permanente Northwest (USA) | CC, R-RD |
FFPE samples ER, PR and HER2 in accordance with ASCO-CAP. 132,133 HER2 defined as ≥ 3 |
|
|
Any LN, % NR (for ER+/HER2– subgroup) | Some ET and chemotherapy, % NR (for ER+/HER2– subgroup) |
Stephen, 2014126 a) BCS n = 831 b) TEAM n = 2513 |
a) BCS b) TEAM (UK/Ireland, the Netherlands, Belgium, Germany, Greece) |
a) Cohort b) R-RCT |
FFPE a) 0.6-mm2 TMA cores. Dual scoring by experts134 b) As Bartlett 201624 Scores normalised. FISH for HER2– (unclear if confirmatory) |
|
|
|
100% ET monotherapy |
Viale 2013127 n = 1256 |
IES135 (37 countries) | R-RCT | FFPE samples. Biomarker expression was measured by IHC. HER2 was confirmed by FISH if ≥ IHC2+. Tumours were deemed positive for ER/PR if IHC ≥ 1% or Allred ≥ 3 and for HER2 if IHC3+ or if FISH amplified. Ki-67 was high if > 11% LI (median) |
|
|
|
100% ET, 19% chemotherapy |
Validation cohorts: LN0 | |||||||
Vincent-Salomon, 2013128 n = 105 | Institut Curie (France) | R-RD | FFPE. For each antibody, internal and external controls were included |
|
|
LN0 100% | 9.5% ET, 0% chemotherapy |
Validation cohorts: LN+ | |||||||
Prat, 2013129 n = 1246 |
GEICAM 9906136 (Spain) | R-RCT | Sections air-dried overnight. General intensity score instead of H-score for ER expression129 |
|
|
|
ET if HER2–, 100% chemotherapy |
Gluz 2016123 n = 459 |
WSG-AGO-Doc137 (Germany) | R-RCT | Paraffin-embedded tumour blocks, no further details |
|
LN1–3 | ET in accordance with clinical guidelines,138 100% chemotherapy | |
Retrospective studies: uncertain generalisability to UK context | |||||||
Gong 201658 n = 611 |
SYSMH; CCSYU; 3rd HNC (China) | R-RD |
FFPE Scores normalised. Other details as per Cuzick et al. 2011. 130 FISH to confirm HER2 if ≥ IHC2+ |
|
100% HR+ 100% HER2– 61% postmenopausal % female NR non-metastatic |
|
100% ET, 76.8% chemotherapy |
Lin 2015124 n = 605 | National Taiwan University Hospital (Taiwan) | R-RD |
FFPE samples. Different IHC methodologies used, used percentiles to account for differences to Cuzick et al. 2011. 25 FISH to confirm HER2 if ≥ IHC2+ |
|
HR+ NR 76.2% HER2– Menopausal status NR Female NR |
Any LN, % NR | ET NR, 74.6% chemotherapy |
Data definitely stated to relate to IHC4+C were only available for the Nottingham cohort25 and TransATAC. 46 For three studies, it was unclear whether the IHC4 score or the IHC4+C score was used. Other studies used IHC4 (TEAM analyses by Barlett et al. 24 and Stephen et al. ,126 the Edinburgh cohort,126 WSG PlanB,73,74,77 GEICAM 9906,129 the Kaiser Permanente cohort,125 the China cohort58 and the Taiwan cohort). 124 See Report Supplementary Material 8 and Table 21 for a description of which cut-off points were used that included using tertiles and/or quartiles, using < 10%, 10–20% and > 20% ROR or using the score as a continuous variable.
Quality assessment: IHC4 and IHC4+C prognostic performance
No study scored well on all items (see Report Supplementary Material 8, Table 4). A high number of studies included patients who had received chemotherapy treatment and a high number were not able to include all relevant patients owing to missing samples or insufficient tissue. Very few studies reported that they blinded test assessors.
Results: IHC4 prognostic performance – unadjusted analyses
See Table 22 and Report Supplementary Material 8, Tables 5–7. Across the studies reporting prognostic performance data from unadjusted analyses, none reported survival or recurrence outcomes per risk group. In the validation cohorts, HR analyses showed statistically significant performance when high-risk groups (defined by quartiles or tertiles) were compared with low-risk groups (when reported), whether in LN0/LN+ (n = 4),24,73,74,77,124,127 LN0-only (n = 3)58,124,125 or LN+-only (n = 1)123 patients, and regardless of patient spectrums and treatments received. However, analyses using continuous scores were not always statistically significant or did not report this. The use of continuous scores, quartiles and tertiles does not allow conclusions to be drawn about which cut-off points should be used in clinical practice and how these would perform.
Reference (first author and year) | Cohorts | Population | Nodal status | ET/chemotherapy | Percentage of patients per risk group | DRFS/DRFI: HR (95% CI) unless stated otherwise (0–5 years) | ||
---|---|---|---|---|---|---|---|---|
Low | Intermediate | High | ||||||
DRFS | ||||||||
LN0, some ET and chemotherapy | ||||||||
Rohan 2014125 n = 295 (147 cases; 148 controls) | Kaiser Permanente Northwest | 100% ER+, 100% HER2– | Any LN, % NR (for ER+/HER2– subgroup) | Some ET and chemotherapy,% NR (for ER+/HER2– subgroup) | 40.7a | 51.9a | 7.5a | OR: |
Continuous | OR per 10 units:
|
|||||||
Retrospective studies: uncertain generalisability to UK context LN0/LN+, some/all ET and chemotherapy | ||||||||
Gong 201658 n = 611 |
SYSMH; CCSYU; 3rd HNC | 100% HR+, 100% HER2– |
|
100% ET, 76.8% chemotherapy | 25.7 | 48.4 | 25.9 | |
Lin 2015 n = 605124 |
National Taiwan University Hospital | HR+ NR, 76.2% HER2– | Any LN, % NR | ET NR, 74.6% chemotherapy | Used quartiles | |||
DRFI | ||||||||
LN0/LN+, some ET and chemotherapy | ||||||||
Cuzick 201125 n = 786 | Nottingham | 100% HR+, 95% HER2–, premenopausal/postmenopausal |
|
52% ET, % chemotherapy NR | 0–25th, 26th–75th, 76th–100th | Below 25th vs. above 75th quartile: 4.1 (2.5 to 6.8) | ||
LN0, some/all ET and chemotherapy | ||||||||
Viale 2013127 | IES | % ER+ NR, % HER2– NR | LN0 | 100% ET, 19% chemotherapy | Used tertiles (not further defined) |
|
Additional prognostic value: IHC4
The additional prognostic value of IHC4 was analysed in six data sets24,73,74,77,123–126,129 in total. Data are presented in Table 23. Data from the study by Stephen et al. 126 in the separate cohorts (BCS and TEAM126) indicated that IHC4 provided more prognostic information than clinicopathological variables in the LN0/LN+ mixed group, based on D-statistics but not when considering HRs, and was more informative for years 0–5 than for years 5–10. The same study reported HRs for only LN0 and LN+ subgroups adjusted for clinicopathological factors, and these were not statistically significant. WSG PlanB73,74,77 reported additional value over clinicopathological factors in LN0/LN+ patients; in the Kaiser Permanente cohort,125 IHC4 did not add additional prognostic value. Three further studies123,124,129 reported on LN+ subgroups, two of which124,129 reported statistically significant additional prognostic value of IHC4 over clinicopathological factors.
Reference (author and year) | Cohorts | Population | Nodal status | ET/chemotherapy | Outcome | Test or comparatora | Likelihood ratio χ2 | Increase in likelihood ratio χ2 over clinicopathological factorsa | Other analyses |
---|---|---|---|---|---|---|---|---|---|
LN0/LN+, 100% ET, 0% chemotherapy | |||||||||
Stephen 2014126 a) BCS n = 831 b) TEAM n = 2513 |
a) BCS | 100% ER+, % HER2– NR | LN0/+, % NR | 100% ET monotherapy | DRFI | IHC4 vs. clinicopathological factors |
MV model (adjuvant for clinicopathological factorsb): % R2 (95% CI)D-statistic (95% CI):c5 years:bD-statistic (95% CI):cClinicopathological plus IHC4 vs. clinicopathological: |
||
b) TEAM | LN0/+, % NR | MV model (adjuvant for clinicopathological factorsa), HR (95% CI):b
|
|||||||
LN0/LN+, 100% ET, some chemotherapy (or chemotherapy NR) | |||||||||
Bartlett 2016,24 Christiansen 2012122 n = 4598122 |
TEAM | 100% HR+, % HER2– NR | LN0/LN+, % NR | 100% ET, some chemotherapy, % NR130 | IDFS | IHC4 vs. clinicopathological factors | 170.024 | 38.5 (29%)24 | 8 years. MV model (adjuvant for clinicopathological factorsa). HR (95% CI):d 1.007 (1.005 to 1.009)122 |
n = 2642 |
WSG PlanB |
|
|
100% ET, RS < 12 no chemotherapy; RS ≥ 12, chemotherapy | IDFS | IHC4 vs. clinicopathological factors | MV model (adjuvant for clinicopathological factorsa). HR (95% CI): 1.59 (95 CI 1.15 to 2.2); p = 0.005e | ||
LN0/LN+, some ET and chemotherapy | |||||||||
Rohan 2014125 n = 295 (147 cases; 148 controls) |
Kaiser Permanente Northwest | 100% ER+, 100% HER2– | Any LN, % NR (for ER+/HER2– subgroup) | Some ET and chemotherapy, % NR (for ER+/HER2– subgroup) | DRFSf | IHC4 vs. clinicopathological factors |
Follow-up year NR OR (95% CI): |
||
LN0, 100% ET, 0% chemotherapy | |||||||||
Stephen 2014126 a) BCS n = 657 b) TEAM n = 1208 |
a) BCS | 100% ER+, % HER2– NR | LN0 | 100% ET, 0% chemotherapy | DRFI | IHC4 vs. clinicopathological factors | MV model (adjuvant for clinicopathological factorsa). HR (95% CI):
|
||
b) TEAM | LN0 | MV model (adjuvant for clinicopathological factorsa). HR (95% CI):
|
|||||||
LN+, 100% ET, 0% chemotherapy | |||||||||
Stephen 2014126 a) BCS n = 174 b) TEAM n = 1296 |
a) BCS | 100% ER+, % HER2– NR | LN+ | 100% ET monotherapy | DRFI | IHC4 vs. clinicopathological factors | MV model (adjuvant for clinicopathological factorsa). HR (95% CI):
|
||
b) TEAM | LN+ | MV model (adjuvant for clinicopathological factorsa). HR (95% CI):
|
|||||||
LN+,% ET NR, 100% chemotherapy | |||||||||
Gluz 2016123 n = NRe |
WSG-AGO-Doc137 | 100% HR+, 100% HER2– | LN1–3 | % ET NR, 100% chemotherapy | IDFS | IHC4 vs. clinicopathological factors | 5 year MV model (adjuvant for clinicopathological factorsa). HR (95% CI):
|
||
LN+, 100% ET, 100% chemotherapy | |||||||||
Prat 2013129 n = 580 |
GEICAM 9906136 | 100% ER+, 100% HER2– 45% postmenopausal | 100% LN+, % LN > 3 NR | 100% ET, 100% chemotherapy | IDFS | IHC4 score vs. clinicopathological |
Follow-up year NR 13.5; p < 0.05 (estimated from graph) |
||
Retrospective studies: uncertain generalisability to UK context | |||||||||
LN0/LN+, variable ET and chemotherapy | |||||||||
Lin 2015124 n = 605 | National Taiwan University Hospital | HR+ NR, 76.2% HER2– | Any LN, % NR | ET NR, 74.6% chemotherapy | RFS | MV model (adjuvant for clinicopathological factorsa). HR (95% CI):
|
Broadly speaking, results did not appear to be influenced by the compatibility of the IHC4 methodology with the standard methodology, with both statistically significant and non-significant results being reported in both compatible and non-compatible studies.
Results: IHC4+C prognostic performance – unadjusted analyses
Data relating to the prognostic performance of IHC4+C are presented in Table 24. Most information relating to IHC4+C comes from the TransATAC trial, which was the derivation cohort, in which unadjusted HRs between risk groups (using predefined cut-off points) for DRFI were statistically significant in LN0–3, LN0 and LN1–3 analyses, and similar results were seen for OS. Additional data from the Nottingham cohort25 and IES127 (although the description is ambiguous) are limited in nature, but support the observations in the TransATAC derivation trial. The TransATAC results suggest that IHC4+C is prognostic for DRFI, with HRs for high-risk versus low-risk groups (for different subgroups and time points) ranging from 4.73 (95% CI 2.79 to 8.03) to 11.39 (95% CI 4.05 to 32.01).
Reference (first author and year) | Cohorts | Population | Nodal status | ET/chemotherapy | Percentage of patients per group | Percentage DRFI risk | DRFI: HR (95% CI) | ||||||||
---|---|---|---|---|---|---|---|---|---|---|---|---|---|---|---|
0–5 years | 0–10 years | ||||||||||||||
Low | Intermediate | High | Low | Intermediate | High | Low | Intermediate | High | 0–5 years | 0–10 years | |||||
LN0/LN+, 100% ET, 0% chemotherapy | |||||||||||||||
TransATAC, Sestak46 n = 1005 |
TransATAC | 100% ER+, HER2– | LN0–3 (n = 774a) | 100% ET, 0% chemotherapy | 60a | 24a | 16a | ||||||||
LN0/LN+, 52% ET, NR chemotherapy | |||||||||||||||
Cuzick 201125 n = 786 | Nottingham |
|
|
52% ET, % chemotherapy NR | Tertiles | Visual inspection of predicted vs. observed DRFI plot (Kaplan–Meier curves plus 95% CIs; see figure 5 in Cuzick et al.25) showed good agreement between predicted and observed scores, although agreement appeared to decrease over time, with lines diverging after 6 years in the high-risk group (67–100%) and 8 years in the low- (0–33%) and intermediate-risk (33–66%) groups | |||||||||
LN0, 100% ET, 0% chemotherapy | |||||||||||||||
TransATAC46 n = 1005 |
TransATAC | 100% ER+, HER2– | LN0 | 100% ET, 0% chemotherapy | 70a | 21a | 9a | 98.9 | 93.1 | 88.1 | 95.6 | 81.8 | 77.3 | ||
LN0, 100% ET, some chemotherapy | |||||||||||||||
Viale 2013127 | IES135 | % ER+ NR, % HER2– NR | LN0 | 100% ET, 19% chemotherapy | States ‘addition of clinical variable to IHC made the effect more profound’ | ||||||||||
LN+, 100% ET, 0% chemotherapy | |||||||||||||||
TransATAC, Sestak46 n = 219 |
TransATAC | 100% ER+, HER2– | LN1–3 | 100% ET, 0% chemotherapy | 28a | 34a | 38a | 98.3 | 90.2 | 85.8 | 96.1 | 75.8 | 67.2 |
Additional prognostic value: IHC4+C
Data relating to the additional prognostic value of IHC4+C are presented in Table 25. The IHC4+C appeared to have additional prognostic value over NPI and CTS, but this was based on the derivation cohort (TransATAC), with increases in likelihood ratio χ2 for DRFI, ranging from 17 to 26 across LN0/LN+, LN0 and LN1–3 groups, compared with CTS and NPI (increases were statistically significant for LN0 patients but not for LN+ patients). In the validation cohort (Nottingham25), the HR adjusted for clinicopathological variables remained statistically significant (HR 3.9, 95% CI 2.3 to 6.5).
Reference (first author and year) | Cohorts | Population | Nodal status | ET/chemotherapy | Outcome | Test or comparator | Likelihood ratio χ2 | Increase in likelihood ratio χ2 over clinicopathological factors | Other analyses | |
---|---|---|---|---|---|---|---|---|---|---|
LN0/LN+, 100% ET, 0% chemotherapy | ||||||||||
TransATAC46 n = 774 (reduced data set) |
TransATAC | 100% ER+, HER2– | LN0–3 | 100% ET, 0% chemotherapy | DRFI | IHC4+C (continuous) vs. CTS | 10 years: 75.30, p < 0.0001 | 10 years: 20.07 (p < 0.0001) | ||
IHC4+C (continuous) vs. NPI | 10 years: 22.84 (p < 0.0001) | |||||||||
Cuzick 201125 n = 786 | Nottingham |
|
|
52% ET, % chemotherapy NR | DRFI | IHC4+C vs. clinical score | 25.89, p < 0.0001 | MV model (adjuvant for clinicopathological factors). HR (95% CI):
|
||
LN0, 100% ET, 0% chemotherapy | ||||||||||
TransATAC46 n = 591 (reduced data set) |
TransATAC | 100% ER+, HER2– | LN0 | 100% ET, 0% chemotherapy | DRFI | IHC4+C (continuous) vs. CTS |
|
5 years: 18.03, p < 0.0001 | 10 years: 17.14, p < 0.0001 | |
IHC4+C (continuous) vs. NPI | 5 years: 17.89, p < 0.0001 | 10 years: 21.92, p < 0.0001 | ||||||||
LN+, 100% ET, 0% chemotherapy | ||||||||||
TransATAC46 n = 183 (reduced data set) |
TransATAC | 100% ER+, HER2– | LN1–3 | 100% ET, 0% chemotherapy | DRFI | IHC4+C (continuous) vs. CTS |
|
5 years: 2.64, p = 0.10 | 10 years: 3.08, p = 0.08 | |
IHC4+C (continuous) vs. NPI | 5 years: 2.65, p = 0.10 | 10 years: 2.45, p = 0.10 |
Conclusions: IHC4 and IHC4+C
The IHC4 score has been validated in five reanalyses of RCTs and six retrospective cohort studies, and provides statistically significant prognostic information consistently in unadjusted analyses in LN+/LN0, LN0 and LN+ groups. However, most studies used quartiles or tertiles to define risk groups, which does not allow conclusions to be drawn about which cut-off points should be used in clinical practice and how these would perform. Many studies used laboratory methods that differed from the derivation study methodology. Only one validation study, by Stephen et al. ,126 reports using the cut-off points from the original analysis,25 which provides a second and third validation cohort (BCS and TEAM), but only for the IHC4 component of the test, not including the clinical factors component (i.e. IHC4+C). IHC4 was shown to have additional prognostic value over clinicopathological factors in some studies. Test methodologies did not appear to have an impact on the statistical significance of test results, but this does not mean that their performance is necessarily the same, and concerns remain about the conduct of the test in laboratories other than that used to derive the score. IHC4+C had prognostic value in unadjusted analyses in the derivation cohort and one validation cohort. Additional prognostic value has been reported in the derivation cohort in which IHC4+C provided statistically significantly more information than NPI in LN0 patients but not in LN+ patients, and in one validation cohort (Nottingham25) in which statistical significance was maintained after adjustments for clinicopathological factors.
Results: all tests compared with each other
The results of all tests compared with each other can be found in Appendix 5, which provides an overview of three types of studies that allow some form of comparison between tests:
-
Studies reporting more than one test – these are studies in which two or more of the tests were conducted and patient outcomes were reported, such that the prognostic performance of two or more tests in the same cohort can be compared. Very few studies conduct formal comparisons between tests.
-
Microarray studies – these are studies in which the commercial versions of the tests were not used, but rather test algorithms were applied to genetic profiles obtained using microarray techniques. Mostly, these are publicly available in in silico (electronic database) genetic profiles, complete with patient outcome data. As with the studies that report more than one test, the comparisons provided are not always formal. There were also a number of microarray studies that reported data for one test only, which are detailed in Report Supplementary Material 10.
-
Concordance in risk categorisation between tests – focusing on the OPTIMA Prelim study.
Results: decision impact studies
Decision impact: study and patient characteristics
Decision impact studies assess how decisions to use or not to use chemotherapy changed pre and post availability of the test. Report Supplementary Material 9, Tables 1–5, show the study characteristics of the included decision impact studies, including whether studies were prospective or retrospective and whether the data were for chemotherapy recommendations or actual treatment decisions. The ER, HER2 and nodal statuses are also shown.
Five UK studies139–145 and 12 other European studies146–158 assessed the decision impact of oncotype DX (see Report Supplementary Material 9, Table 1); the results of one further study were provided as commercial in confidence and could not be presented here. 62 One UK study159 and three other European studies160–162 assessed the decision impact of EndoPredict (EPClin) (see Report Supplementary Material 9, Table 2). One UK study163 and no other European studies assessed the decision impact of IHC4+C (see Report Supplementary Material 9, Table 3). No UK studies and three other European studies164–166 assessed the decision impact of Prosigna (see Report Supplementary Material 9, Table 4). No UK studies and eight European studies81,167–173 assessed the decision impact of MammaPrint (see Report Supplementary Material 9, Table 5). 62
Decision impact: results
In most studies, patients were allocated pre test to either chemotherapy or no chemotherapy. This could be a recommendation (by a physician or multidisciplinary team) or an actual treatment decision (what the patient actually received). They were then split into four post-test groups: those whose decision/recommendation (1) remained chemotherapy, (2) remained no chemotherapy, (3) changed from no chemotherapy to chemotherapy or (4) changed from chemotherapy to no chemotherapy. Tables 26–30 provide these data. These data are also summarised in terms of the proportion of patients undergoing any treatment change (either to or from chemotherapy), the total proportion allocated to chemotherapy both pre and post test and the net change in chemotherapy use. Within each results table subheading, studies are broadly ordered as LN0, then mixed nodal status and then LN+.
Study (first author and year) | Country | Population | Nodal status | Pre test | Post test | Number of patients | No chemotherapy, unchanged | No chemotherapy, changed to chemotherapy | Chemotherapy, unchanged | Chemotherapy, changed to no chemotherapy | Treatment change, n (%) | Pre-test chemotherapy, n (%) | Post-test chemotherapy, n (%) | Net change in chemotherapy, n (%) |
---|---|---|---|---|---|---|---|---|---|---|---|---|---|---|
UK studies: recommendation | ||||||||||||||
aKiernan 2016143 | UK | ER+, HER2– (assumed) | LN0 (assumed) | Recommendation | Recommendation | 50 | NR | NR | NR | NR | NR | 21 (42)a | 14 (28)a | –7 (–14) |
Kuchel 2016144 | UK | ER+, HER2– | LN0–3 | Recommendation | Recommendation | 135 | 54 | 12 | 26 | 43 | 55 (41) | 69 (51) | 38 (28) | –31 (–23) |
ER+, HER2–, NPI intermediate | LN0–3 | Recommendation | Recommendation | 67 | 17 | 10 | 17 | 23 | 33 (49) | 40 (60) | 27 (40) | –13 (–19) | ||
UK studies: decision | ||||||||||||||
Holt 2013,141 Albanell 2016142 | UK | ER+, HER2– (subgroup) | LN0 | Decision | Decision | 94 | 45 | 9 | 18 | 22 | 31 (33) | 40 (43) | 27 (29) | –13 (–14) |
Kuchel 2016144 | UK | ER+, HER2– | LN0–3 | Decision | Decision | 131 | 66 | 13 | 24 | 28 | 41 (31) | 52 (40) | 37 (28) | –15 (–11) |
ER+, HER2–, NPI intermediate | LN0–3 | Decision | Decision | 65 | 31 | 7 | 15 | 12 | 19 (29) | 27 (42) | 22 (34) | –5 (–8) | ||
UK studies: recommendation to decision | ||||||||||||||
Hassan 2015,139 Hassan 2015140 | UK | ER+, HER2– (assumed) | LN0 (assumed) | Recommendation | Decision | 26 | 9 | 2 | 7 | 8 | 10 (38) | 15 (58) | 9 (35) | –6 (–23) |
Loncaster 2017145 | UK | ER+, HER2– | LN0 | Recommendation | Decision (largely on test) | 136 | NR | NR | NR | NR | NR | 136 (100) | 54 (40) | N/A |
LN+ | 65 | NR | NR | NR | NR | NR | 65 (100) | 20 (31) | N/A | |||||
European studies: recommendation | ||||||||||||||
Albanell 2012146 (trans-GEICAM) | Spain | ER+, HER2– | LN0 | Recommendation | Recommendation | 107 | 56 | 12 | 17 | 22 | 34 (32) | 39 (36) | 29 (27) | –10 (–9) |
Dieci 2016149 | Italy | ER+, HER2– | LN0 | Recommendation | Recommendation | 123 | 71 | 5 | 37 | 10 | 15 (12) | 47 (38) | 42 (34) | –5 (–4) |
Eiermann 2013151 | Germany | ER+, HER2– | LN0 | Recommendation | Recommendation | 244 | 99 | 28 | 72 | 45 | 73 (30) | 117 (48) | 100 (41) | –17 (–7) |
Hejduk 2016,153 Petrakova 2016154 | Czech Republic | ER+, HER2– | LN0 | Recommendation | Recommendation | 196 | 43 | 3 | 27 | 123 | 126 (64) | 150 (77) | 30 (15) | –120 (–61) |
Gligorov 2015152 (SWITCH) | France | ER+, HER2– | LN0micro | Recommendation | Recommendation | 95 | 41 | 5 | 19 | 30 | 35 (37) | 49 (52) | 24 (25) | –25 (–26) |
Novas 2016156 | Spain | NR | LN1micro | Recommendation | Recommendation | 35 | 21 | 1 | 5 | 8 | 9 (26) | 13 (37) | 6 (17) | –7 (–20) |
Bodmer 2015147 | Switzerland | ER+, HER2– | LN0 or LN+ | Recommendation | Recommendation | 60 | 19 | 3 | 13 | 25 | 28 (47) | 38 (63) | 16 (27) | –22 (–37) |
Dreyfus 2015150 | France |
HR+, HER2– Indicated for chemotherapy |
|
Recommendation | Recommendation | 39 | 0 | 0 | 13 | 26 | 26 (67) | 39 (100) | 13 (33) | N/A |
Mouysset 2016155 | France | ER+, HER2– |
|
Recommendation | Recommendation | 603 | NR | NR | NR | NR | 425 (70) | 529 (88) | 145 (24) | –384 (–64) |
Pestalozzi 2015157 | Switzerland | ER+, HER2– | pN0 or pN1a | Recommendation | Recommendation | 221 | 124 | 8 | 52 | 37 | 45 (20) | 89 (40) | 60 (27) | –29 (–13) |
Wassermann 2015158 | France | HR+, HER2– |
|
Recommendation | Recommendation | 72 | NR | NR | NR | NR | NR | 41 (57) | 14 (19) | –27 (–38) |
Eiermann 2013151 | Germany | ER+, HER2– | LN1–3 | Recommendation | Recommendation | 122 | 18 | 12 | 58 | 34 | 46 (38) | 92 (75) | 70 (57) | –22 (–18) |
European studies: recommendation to decision | ||||||||||||||
Dieci 2016149 | Italy | ER+, HER2– | LN0 | Recommendation | Decision | 73 | 3 | 31 | 16 | 19 (15) | 47 (38) | 34 (28) | –13 (–11) | |
Eiermann 2013151 | Germany | ER+, HER2– | LN0 | Recommendation | Decision | 244 | NR | NR | NR | NR | NR | 117 (48) | 83 (34) | –34 (–14) |
De San Vicente 2015148 | Spain |
HR+, HER2– Intermediate oncotype DX |
|
Recommendation | Decision | 37 | 27 | 1 | 8 | 1 | 2 (5) | 9 (24) | 9 (24) | 0 (0) |
Eiermann 2013151 | Germany | ER+, HER2– | LN1–3 | Recommendation | Decision | 122 | NR | NR | NR | NR | NR | 92 (75) | 57 (47) | –35 (–29) |
Study (first author and year) | Country | Population | Nodal status | Pre test | Post test | Number of patients | No chemotherapy, unchanged | No chemotherapy, changed to chemotherapy | Chemotherapy, unchanged | Chemotherapy, changed to no chemotherapy | Treatment change, n (%) | Pre-test chemotherapy, n (%) | Post-test chemotherapy, n (%) | Net change in chemotherapy, n (%) |
---|---|---|---|---|---|---|---|---|---|---|---|---|---|---|
UK studies: decision | ||||||||||||||
Bloomfield 2017159 | UK | ER+, HER2– | NR | Decision | Decision | 149 | 60 | 28 | 34 | 27 | 55 (37) | 61 (41) | 62 (42) | +1 (+1) |
European studies: recommendation | ||||||||||||||
Penault-Llorca 2016162 (ADENDOM) | France | ER+, HER2– | LN0micro | Recommendation | Recommendation | 200 | 85 | 20 | 40 | 55 | 75 (38) | 95 (48) | 60 (30) | –35 (–18) |
Ettl 2015160 | Germany | ER+, HER2– |
|
Recommendation | Recommendation | 217 | NR | 16 | NR | 73 | 89 (41) | NR | NR | –57 (–26) |
Muller 2013161 | Germany | ER+, HER2– |
|
Recommendation | Recommendation | 130 | 31 | 16 | 50 | 33 | 49 (38) | 83 (64) | 66 (51) | –17 (–13) |
European studies: recommendation to decision | ||||||||||||||
Penault-Llorca 2016162 (ADENDOM) | France | ER+, HER2– | LN0micro | Recommendation | Decision | 200 | 90 | 15 | 38 | 57 | 72 (36) | 95 (48) | 53 (27) | –42 (–21) |
Study (first author and year) | Country | Population | Nodal status | Pre test | Post test | Number of patients, n | No chemotherapy, unchanged | No chemotherapy, changed to chemotherapy | Chemotherapy, unchanged | Chemotherapy, changed to no chemotherapy | Treatment change, n (%) | Pre-test chemotherapy, n (%) | Post-test chemotherapy, n (%) | Net change in chemotherapy, n (%) |
---|---|---|---|---|---|---|---|---|---|---|---|---|---|---|
UK studies: recommendation | ||||||||||||||
aYeo 2015163 | UK | ER+ HER2– | LN0, 74%; LN1–3, 26% | Recommendation | Recommendation | 124 | 49 | 1 | 41 | 33 | 34 (27) | 45 (36) to 74 (60)a | 42 (34) | –3 (–2) to –32 (–26) |
European studies | ||||||||||||||
None |
Study (first author and year) | Country | Population | Nodal status | Pre test | Post test | Number of patients | No chemotherapy, unchanged | No chemotherapy, changed to chemotherapy | Chemotherapy, unchanged | Chemotherapy, changed to no chemotherapy | Treatment change, n (%) | Pre-test chemotherapy, n (%) | Post-test chemotherapy, n (%) | Net change in chemotherapy, n (%) |
---|---|---|---|---|---|---|---|---|---|---|---|---|---|---|
UK studies | ||||||||||||||
None | ||||||||||||||
European studies: recommendation | ||||||||||||||
Martin 2015164 (GEICAM) | Spain | ER+, HER2– | LN0 | Recommendation | Recommendation | 200 | 122 | 18 | 38 | 22 | 40 (20) | 60 (30) | 56 (28) | –4 (–2) |
Wuerstlein 2016166 | Germany | ER+, HER2– | LN0 | Recommendation | Recommendation | 198 | 131 | 22 | 40 | 5 | 27 (14) | 45 (23) | 62 (31) | +17 (+9) |
Van Asten 2016165 | Belgium | ER+, HER2– | NR | Recommendation | Recommendation | 51 | 15 | 11 | 15 | 10 | 21 (41) | 25 (49) | 26 (51) | +1 (+2) |
Study (first author and year) | Country | Population | Nodal status | Pre test | Post test | Number of patients | No chemotherapy, unchanged | No chemotherapy, changed to chemotherapy | Chemotherapy, unchanged | Chemotherapy, changed to no chemotherapy | Treatment change, n (%) | Pre-test chemotherapy, n (%) | Post-test chemotherapy, n (%) | Net change in chemotherapy, n (%) |
---|---|---|---|---|---|---|---|---|---|---|---|---|---|---|
UK studies | ||||||||||||||
None | ||||||||||||||
European studies: recommendation | ||||||||||||||
aDrukker 2014168 (RASTER) | The Netherlands, Germany, France, Italy, Portugal | ER+/ ER–, HER2+/HER2– | LN0 | Recommendation | Recommendation | 37 (414)a | 202 | 9 | 144 | 59 | 68 (16) | 203 (49) | 153 (37) | –50 (–12) |
Exner 2014169 | Austria | ER+, HER2– | LN0 | Recommendation | Recommendation | 75 | 40 | 4 | 21 | 10 | 14 (19) | 31 (41) | 25 (33) | –6 (–8) |
Bueno-de-Mesquita 200781 (RASTER) | The Netherlands |
|
LN0micro | Recommendation | Recommendation | 427 | NR | NR | NR | NR | NR | 186 (44) | 219 (51) | +33 (+8) |
bCusumano 2014167 | The Netherlands, Belgium, Italy, Spain | ER+, HER2– |
|
Recommendation | Recommendation | 151 (453)a | 149 | 68 | 161 | 75 | 143 (32) | 236 (52) | 229 (51) | –7 (–2) |
cKuijer 2016171 | The Netherlands | ER+ (HER2 NR) | NR | Recommendation | Recommendation | 377c | 69 | 38 | 114 | 156 | 194 (51) | 270 (72) | 152 (40) | –118 (–31) |
Wuerstlein 2016173 (WSG PRIMe) | Germany | HR+, HER2– |
|
Recommendation | Recommendation (unclear) | 430 | 201 | 65 | 107 | 57 | 122 (28) | 164 (38) | 172 (40) | +8 (+2) |
European studies: recommendation to decision | ||||||||||||||
Bueno-de-Mesquita 200781 (RASTER) | The Netherlands |
|
LN0micro | Recommendation | Decision | 427 | 206 | 35 | 167 | 19 | 54 (13) | 186 (44) | 202 (47) | +16 (+4) |
Rullan 2016172 | Spain | HR+, HER2– | 94% LN0micro | Recommendation | Decision | 129 | NR | NR | NR | NR | NR | 119 (92) | 45 (35) | –74 (–57) |
European studies: decision to recommendation | ||||||||||||||
Hartmann 2012170 | Germany | HR+, HER2– |
|
Decision | Recommendation | 60 | 47 | 6 | 2 | 5 | 11 (18) | 7 (12) | 8 (13) | +1 (+2) |
Oncotype DX
Among four UK studies,139–144 the percentage of patients with any change in treatment recommendation or decision (either to or from chemotherapy) ranged from 29% to 49% (Table 26). Across 11 European (non-UK) studies,146–157 the percentage of patients with any change in treatment recommendation or decision ranged from 5% to 70%. There was little clear difference in results in accordance with LN status.
Among UK studies, the net reduction in chemotherapy recommendations (pre test to post test) was 14% to 23% across two studies,143,144 and the net reduction in chemotherapy decisions was 8% to 14% across two studies. 141,142,144 Two further UK studies139,140,145 reported changes from pre-test chemotherapy recommendation to the post-test decision, which may overestimate the net change: one reported a reduction of 23% in chemotherapy use139,140 and the other assessed only patients with an initial recommendation for chemotherapy so it is misleading to calculate the absolute change. 145 Across 11 European (non-UK) studies,146–149,151–158 the net reduction in chemotherapy recommendations or decisions ranged from 0% to 64%. Again, there was little clear difference in results in accordance with LN status.
EndoPredict
In the one UK study of EndoPredict,159 37% of patients had a change in treatment decision (either to or from chemotherapy) (see Table 27). Across three European (non-UK) studies,160–162 the percentage of patients with any change in treatment recommendation ranged from 38% to 41%. In the UK study, the net increase in chemotherapy use (pre test to post test) was 1% (because treatment changes took place in both directions). 159 However, across three European (non-UK) studies,160–162 there was a net reduction in chemotherapy recommendations, ranging from 13% to 26%. There was insufficient data to assess results by lymph node status.
IHC4+C
In the one UK study of IHC4+C (mix of LN+/LN0),163 27% of patients had a change in treatment recommendation (either to or from chemotherapy) (see Table 28). Pre-test decisions included either ‘recommend chemotherapy’ or ‘discuss chemotherapy’. The net reduction in patients who were definitively recommended chemotherapy was 2%. However, if pre-test chemotherapy recommendations were assumed to include both ‘recommend chemotherapy’ and ‘discuss chemotherapy’, the net reduction could be up to 26%. There were no other European studies of IHC4.
Prosigna
There were no UK studies of Prosigna. Across three European (non-UK) studies (either LN0 or lymph node status not reported),164–166 the percentage with any change in treatment recommendation ranged from 14% to 41% (see Table 29). The net change in chemotherapy recommendations (pre test to post test) was a reduction of 2% in one study164 and an increase of 2% to 9% in two studies. 165,166
MammaPrint
There were no UK studies of MammaPrint. Across seven European (non-UK) studies,81,167–171,173 the percentage with any change in treatment recommendation or decision ranged from 13% to 51% (see Table 30). The net change in chemotherapy recommendations (pre test to post test) ranged from a reduction of 31% to an increase of 8% across six studies. 81,167–169,171,173 Again there were insufficient data to assess results by lymph node status.
Summary and discussion of decision impact studies
The percentage of patients with any change in treatment recommendation or decision (either to or from chemotherapy) among UK studies was 29% to 49% across four oncotype DX studies,62,139–142,144 37% in one EndoPredict study159 and 27% in one IHC4+C study. 163 Ranges across European (non-UK) studies were 5% to 70% for oncotype DX,146–157 38% to 41% for EndoPredict,160–162 14% to 41% for Prosigna164–166 and 13% to 51% for MammaPrint. 81,167–171,173
The net changes in the percentage of patients with a chemotherapy recommendation or decision (pre test to post test) among UK studies were a reduction of 8% to 23% across four oncotype DX studies,141–144 an increase of 1% in one EndoPredict study159 and a reduction of between 2% and 26% in one IHC4+C study (unclear owing to category definitions). 163 Net changes across European (non-UK) studies were a reduction of 0% to 64% for oncotype DX,146–149,151–158 a reduction of 13% to 26% for EndoPredict,160–162 a reduction of 2% to an increase of 9% for Prosigna164–166 and a reduction of 31% to an increase of 8% for MammaPrint. 81,167–169,171,173
Anxiety and health-related quality of life
Six studies (reported in seven publications)159,164,166,174–177 reported outcomes relating to anxiety (including worry and distress) and HRQoL (Table 31). Studies reporting outcomes such as decision conflict and patient satisfaction did not meet the inclusion criteria for the review and were excluded.
Reference (first author and year) | Test | Cohort | Country | Study design | Details of test | Cut-off points | Number of patients | Population | Nodal status | Outcomes |
---|---|---|---|---|---|---|---|---|---|---|
Oncotype DX | ||||||||||
Evans 2016174 | Oncotype DX | Four centres (Washington, Maryland and Florida) | USA | Pre–post test | NR | NR | 193 |
|
LN+/LN0 (LN > 3 NR) | IES178 |
Lo 2010176 | Oncotype DX | NR | USA | Pre–post test | Genomic Health | NR | 93 |
|
LN+/LN0 (LN > 3 NR) | STAI; FACT-B, FACT-G |
MammaPrint | ||||||||||
Retèl 2013177 | MammaPrint | MINDACT-enrolled and MINDACT-ineligible patients | The Netherlands | Non-randomised clinical trial | NR | NR | 347 | ESBC | LN+/LN0 | Lynch’s distress scale (adapted); Lerman’s Cancer Worry Scale (adapted); FACT-B breast cancer subscale |
EPClin | ||||||||||
Bloomfield 2017159,175 | EPClin (EndoPredict plus NS plus TS) | Eight hospitals | South-east England | Pre–post test | NR | NR | 149 |
|
NR | STAI |
Prosigna | ||||||||||
Martin 2015164 | Prosigna | 15 centres | Spain | Pre–post test | Manufacturer’s specifications | NR | 200 |
|
LN0 | STAI; FACT-G |
Wuerstlein 2016166 | Prosigna | 11 centres | Germany | Pre–post test | Manufacturer’s specifications | 40–60 | 198 |
|
LN0 | STAI; FACT-G |
Oncotype DX
Two studies174,176 reported data for oncotype DX. Both adopted a pre–post test design, and included LN+ or LN0 patients. Evans et al. 174 used the Impact of Events Scale178 and showed no difference between pre- and post-test values (p = 0.09), and there were no differences by recurrence score risk group (interaction tests reported as ‘not statistically significant’). Lo et al. ,176 on the other hand, reported a statistically significant improvement in overall State–Trait Anxiety Inventory (STAI) score between pre- and post-test values (p = 0.007), but no difference in trait anxiety (p = 0.27). Results for state anxiety were not reported. Only Lo et al. 176 reported HRQoL using Functional Assessment of Cancer Therapy – Breast cancer (FACT-B) and Functional Assessment of Cancer Therapy – General (FACT-G) and reported no statistically significant change (p = 0.55 and p = 0.49, respectively) (Table 32).
Reference (first author and year) | Test | Country | Study design | Population | Nodal status | Anxiety | HRQoL |
---|---|---|---|---|---|---|---|
Oncotype DX | |||||||
Evans 2016174 n = 193 |
Oncotype DX | USA | PPT | ER+ | LN+/LN0 (LN > 3 NR) |
|
NR |
Lo 2010176 n = 93 |
Oncotype DX | USA | PPT |
|
LN+/LN0 (LN > 3 NR) | STAI mean score (SD)
|
FACT-B mean score (SD)
|
MammaPrint | |||||||
Retèl 2013177 n = 347 |
MammaPrint | The Netherlands | Non-randomised clinical trial | ESBC | LN+/LN0 |
Lynch’s distress scale (adapted) Adjusted regression analysis:aLerman’s Cancer Worry Scale (adapted) Adjusted regression analysis:a |
FACT-B, breast cancer subscale Adjusted regression analysis:a |
EPClin | |||||||
n = 149 |
EPClin | UK | PPT | ER+, HER2–, equivocal by AOL | NR |
|
NR |
Prosigna | |||||||
Martin 2015164 n = 200 |
Prosigna | Spain | PPT | ER+, HER2– | LN0 | Trait anxiety, mean (SD) (n = 180)
|
FACT-G
|
Wuerstlein 2016166 n = 198 |
Prosigna | Germany | PPT |
|
LN0 | State anxiety, mean difference (SD)
|
FACT-G No statistically significant differences in any group, for any subscale, except emotional and functional well-being |
MammaPrint
One study reported data for MammaPrint177 (see Table 31). The study recruited exclusively from patients who had been screened for eligibility in the MINDACT trial, but included both those eligible and those ineligible for MINDACT (due to having more than three positive lymph nodes or having a test failure). A modified version of Lynch’s distress scale179 and one of Lerman’s Cancer Worry Scale180 were used. Patients were separated into seven subgroups in accordance with their clinical risk, MammaPrint risk, whether or not they were assigned to chemotherapy and whether or not the MammaPrint test result was missing (see Table 32). Regression analyses adjusted for sociodemographics, understanding of genomic results, timing of test results, perceived risk and satisfaction with the process showed statistically significantly (the analysis plan set the significance p-value to < 0.01 to avoid type I errors) higher distress when the genomic test failed, when the patient was categorised as high risk by both clinical scoring and MammaPrint and in patients with discordant results when the treatment matched the MammaPrint score (i.e. clinical low/genomic high, prescribed chemotherapy; clinical high/genomic low, not prescribed chemotherapy). Only patients with high clinical risk and no genomic test result or high clinical risk and high genomic risk had a statistically significant decrease in FACT-B HRQoL.
EndoPredict
One study159 reported data for EndoPredict (see Table 31). The study was a pre–post test design and reported a statistically significant decrease in STAI for those whose treatment decision changed from chemotherapy to no chemotherapy on the basis of EndoPredict (p < 0.01), and an increase in STAI for those whose treatment decision changed from no chemotherapy to chemotherapy (p < 0.001) (see Table 32).
Prosigna
Two studies164,166 reported data for Prosigna (see Table 31). Both adopted a pre–post test design and included only LN0 patients. In both studies, there was no difference in trait anxiety scores (p = 0.858164 and p = 0.431166), and in both studies state anxiety changed significantly in low-risk (by Prosigna) patients (p < 0.001164 and p = 0.008166) but not in the intermediate- or high-risk groups (see Table 32). Both studies reported FACT-G; Martin et al. 164 reported no change in overall scores, whereas Wuerstlein et al. 166 reported a statistically significant analysis of variance p-value for emotional and physical well-being (p = 0.030, p = 0.005, respectively).
Discussion
There were no data relating to the impact of IHC4 on anxiety or HRQoL. Other available data are limited in terms of study designs (pre–post test) and patient spectrum. The lack of a comparator makes it difficult to tell whether or not similar changes would have occurred were patients to have received a definitive decision based on their clinical risk factors alone. Across tests, and when reported, state anxiety decreased post test and total FACT-G generally stayed the same. The results for one study suggest that patients had higher distress when the genomic test failed, when the patient was deemed to be at high risk by both clinical scoring and genomic test and in patients with discordant results when the treatment matched the genomic score, although it was unclear if this was due to distress associated with change (in treatment decision) or a lack of trust in the genomic score.
Conclusions
Genomic testing may reduce state anxiety in some patients in some contexts, but, generally, there was little impact on HRQoL.
Time-to-test results
Two articles reported time to test results: Losk et al. 181 and Müller et al. 161 Losk et al. 181 reported factors associated with delays in chemotherapy initiation (defined as ≥ 42 days from surgery to chemotherapy) in breast cancer patients at a US cancer centre in 2011–13. Of 263 HR+, HER2– women receiving adjuvant chemotherapy, 82 had an oncotype DX test ordered. Of those for whom an oncotype DX test was ordered, 31% had a delay of ≥ 42 days to chemotherapy initiation, compared with 20% of patients for whom oncotype DX was not ordered. Müller et al. 161 reports the time to test result for EndoPredict. In this study, the median handling time was 3 working days (range 0–11 days), and 59% of tests were conducted in ≤ 3 days.
Chapter 3 Cost-effectiveness
This chapter presents the methods and results of a de novo model-based health economic evaluation of each of the tumour profiling tests compared with current practice. A systematic review of economic analyses of tumour profiling tests for early-stage breast cancer, undertaken to inform the model structure published since NICE DG10,20 is presented in Appendix 6. A critique of economic analyses made available to the EAG by the manufacturers of oncotype DX62 and MammaPrint94 and by the chief investigator of the EndoPredict decision impact study182 is presented in Appendix 7.
Independent economic evaluation
Scope of the External Assessment Group economic analysis
The EAG developed a de novo model to assess the cost-effectiveness of oncotype DX, Prosigna, IHC4+C, EPClin and MammaPrint versus current practice only. The scope of the EAG model is summarised in Table 33. The model assesses the health outcomes and costs associated with each strategy over a lifetime horizon from the perspective of the UK NHS and PSS. All costs and health outcomes are discounted at a rate of 3.5% per annum. Unit costs are valued at 2015/16 prices. The principal sources of evidence used to inform the analyses of oncotype DX, Prosigna, IHC4+C and EPClin are the TransATAC study46 and the NHS England Access Scheme Database. 183 As the TransATAC study does not include the MammaPrint test, the MINDACT study98 was instead used as the basis for estimating classification probabilities and conditional DMFS probabilities for MammaPrint. Additional studies identified within the clinical evidence review (see Chapter 2), which provide alternative relevant data on test risk classification probabilities, 10-year DMFS probabilities conditional on risk classification and post-test chemotherapy probabilities (decision impact) are explored within the sensitivity analyses.
Element of economic analysis | Description |
---|---|
Population |
Women with ER+, HER2– and early-stage breast cancer (LN0–3) For oncotype DX, Prosigna, IHC4+C and EPClin, analyses are presented for three discrete patient subgroups:For the evaluation of MammaPrint, the modelled population reflects the ITT population of the MINDACT trial. 98 Additional analyses are also presented for the mAOL clinical high-risk subgroup and the mAOL clinical low-risk subgroups separately |
Interventions |
|
Comparator |
The comparator for all analyses is current practice (including a mix of risk prediction tools and diagnostic guidelines) For MammaPrint, current practice is based on mAOL, as per the design of the MINDACT trial98 Because of evidence limitations,b the competing tests were not compared incrementally against one another |
Primary health economic outcome | Incremental cost per QALY gained |
Perspective | NHS and PSS |
Time horizon | Lifetime |
Discount rate | 3.5% per annum |
Price year | 2015/16 |
Population
The population reflected in the model relates to women with ER+, HER2– early-stage breast cancer with LN0–3. For oncotype DX, IHC4+C, Prosigna and EPClin, the economic analysis is presented for three discrete subgroups: (1) women with node-negative disease and a NPI of ≤ 3.4 (clinical low risk), (2) women with node-negative disease and a NPI of > 3.4 (clinical intermediate risk) and (3) node positive (one to three nodes). The modelled population for these four tests reflects that of the TransATAC study,46 as this is used as the source of data on risk classification for each test and the 10-year DMFS probability conditional on each risk classification. Within the LN0 population, a NPI cut-off point of 3.4 was chosen as a means of distinguishing between clinical low risk and clinical intermediate risk for oncotype DX, IHC4+C, Prosigna and EPClin, as data by NPI score were available from the TransATAC trial46 and the National Cancer Registration and Analysis Service (NCRAS) cancer registration data set. 184 Predict scores were not available in either data set, so this tool could not be used to define clinical risk.
MammaPrint was not included in the TransATAC study, hence an alternative source was required. The economic analysis of MammaPrint was instead largely based on data reported within the original paper and supplementary material of the MINDACT trial publication. 98 As the randomisation schedule within the MINDACT trial was conducted using a modified version of AOL (mAOL) and sufficient data were not presented separately for patients with 1–3 lymph nodes, the population of the primary analysis largely reflects the MINDACT ITT population. 98 Additional analyses are also presented for the mAOL high-risk subgroup and the mAOL low-risk subgroups.
Interventions
The EAG’s economic analysis includes all five tests included in the final NICE scope21 (see Table 2). The tests are modelled in line with how their manufacturers state that they will be used in clinical practice: IHC4 and EndoPredict are assumed to be applied together with clinicopathological factors (IHC4+C and EPClin, respectively). RSPC (oncotype DX in conjunction with clinicopathological factors) is considered separately within the sensitivity analyses but is not included in the EAG’s base case. The EAG’s economic analysis also assumes that all pathology analysis is undertaken centrally; local pathology analysis is not considered within the EAG’s base case.
Comparator
The most commonly used tools for predicting the ROR after surgery to guide the use of adjuvant chemotherapy for breast cancer in England are Predict and NPI. At the time of writing, AOL is being updated and has been temporarily disabled. As noted previously, a modified version of AOL was used to inform the randomisation schedule for the discordant clinical and genomic risk groups within the MINDACT trial. 98 For this reason, the comparator for the analysis of MammaPrint is current practice using mAOL.
Owing to the use of a different evidence source for MammaPrint98 compared with the other four tumour profiling tests, and the use of the unrestricted TransATAC trial data set,46 each test is compared only against current practice; tests were not assessed incrementally against each other.
Model structure
The general structure of the EAG model is based on the model previously developed by Ward et al. 1 to inform NICE DG10. 20 This is also broadly consistent with the majority of studies identified within the review of published economic evaluations (see Appendix 6). The EAG model takes the form of a hybrid decision-tree/Markov model (Figures 5 and 6). The decision tree component of the model classifies patients in the current practice (no test) group and the tumour profiling test group into high-, intermediate- and low-risk categories based on the results of the test. For EPClin and MammaPrint, the intermediate-risk category is not relevant as these tests provide results in terms of high and low risk only. The treatment group (test or no test) and the risk level predicted by the test determines the probability that the patient will subsequently receive adjuvant chemotherapy. Within both the test group and the current practice group, the decision tree determines the probability that a patient will be assigned to one of six groups: (1) low risk, chemotherapy; (2) low risk, no chemotherapy; (3) intermediate risk, chemotherapy; (4) intermediate risk, no chemotherapy; (5) high risk, chemotherapy; and (6) high risk, no chemotherapy (for the analyses of EPClin and MammaPrint, four branches are used owing to the absence of an intermediate-risk category). Each of the branches is then linked to a Markov model that predicts lifetime QALYs and costs in accordance with the patient’s risk of distant recurrence and whether or not they receive chemotherapy.
FIGURE 5.
The EAG model: decision tree component. For EPClin and MammaPrint, four branches are used owing to the absence of an intermediate-risk category for these tests.
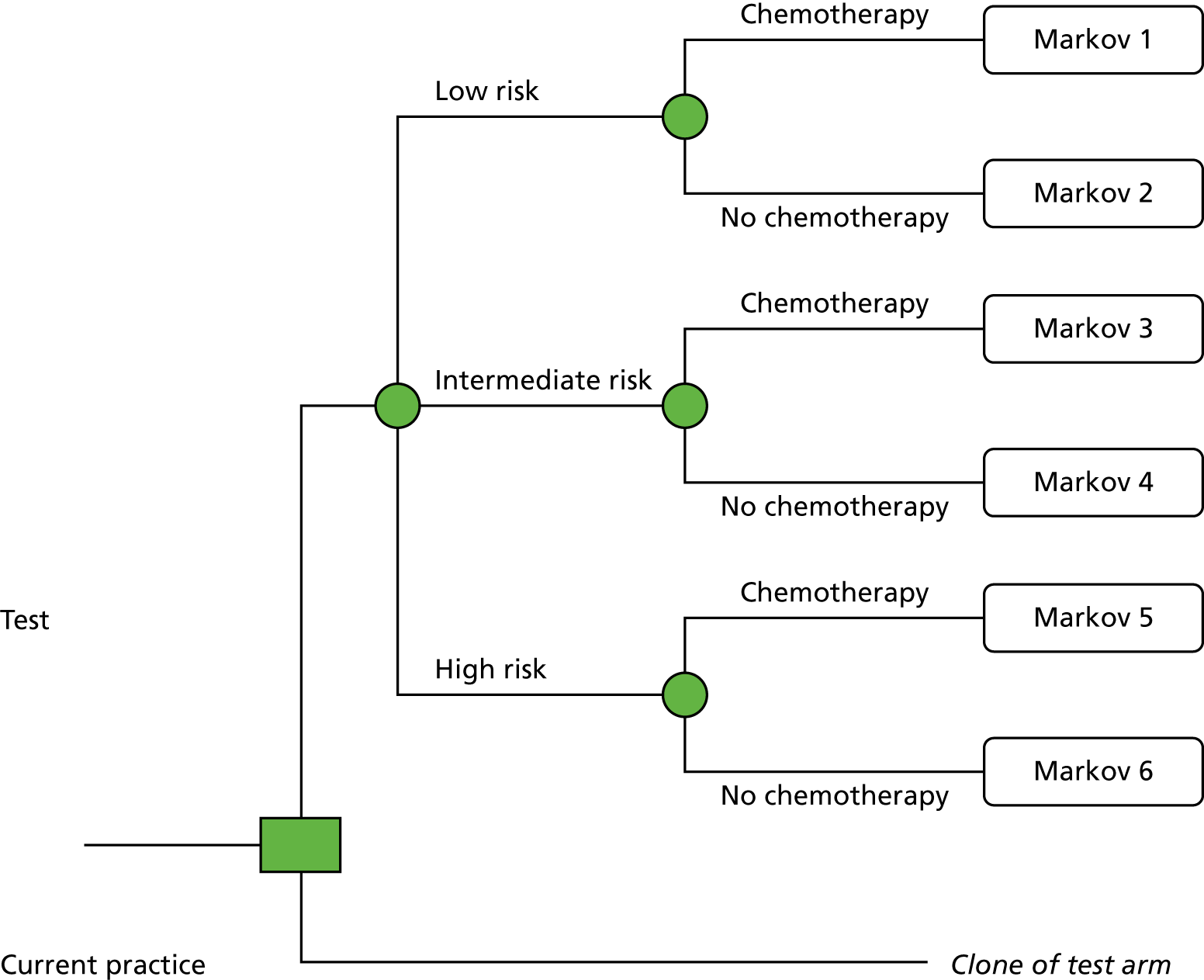
FIGURE 6.
The EAG model: state-transition model component. AML, acute myeloid leukaemia.
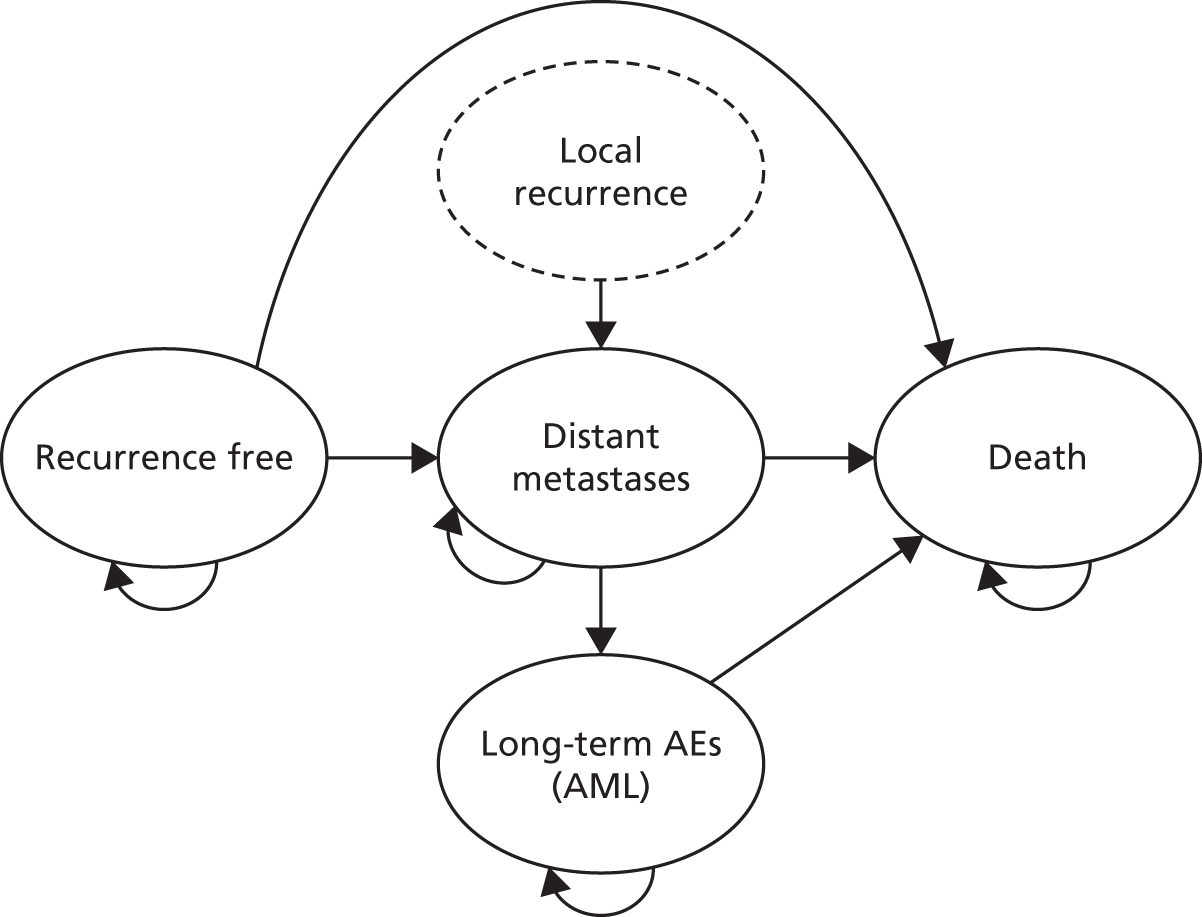
Figure 6 illustrates the Markov nodes of the model. Each Markov node is evaluated over 84 6-month cycles (42 years): patients are assumed to enter the model aged 58 years and the evaluation is continued until the cohort has reached the age of 100 years. Each Markov node includes four health states: (1) recurrence-free, (2) distant recurrence, (3) long-term AEs [acute myeloid leukaemia (AML)] and (4) death. Each Markov node differs with respect to the patient’s risk of distant metastases (determined by their risk classification and whether or not they receive adjuvant chemotherapy). For all Markov nodes, patients enter the model in the recurrence-free health state. During any 6-month cycle, patients who are recurrence-free can remain in their current health state, transit to the long-term AEs state, develop distant metastases or die. Patients in the distant metastases state can remain in their current health state, transit to the long-term AEs (AML) state or die. Patients in the long-term AEs (AML) state are assumed to remain in this state until death (if free from breast cancer recurrence, they cannot subsequently develop distant metastases owing to their breast cancer). Patients may die because of breast cancer, AML or other causes. A HRQoL decrement is applied during the first model cycle for patients receiving adjuvant chemotherapy to account for health losses associated with short-term chemotherapy-related AEs. The benefit of adjuvant chemotherapy is modelled using a RR of distant recurrence within each risk classification group. The impact of each test is therefore captured in the model only by changing the probability that patients with each test risk classification receive adjuvant chemotherapy. In the evaluation of oncotype DX, a sensitivity analysis is included in which the test is assumed to provide a predictive benefit of chemotherapy, hence different RRs of developing distant metastases (for chemotherapy vs. no chemotherapy) are applied across the low-, intermediate- and high-risk groups.
Different health utilities are applied to each of the modelled health states. The model assumes that a proportion of patients who experience distant recurrence will also have previously developed local recurrence: this is assumed to be associated with additional costs and a once-only QALY loss. The model includes costs associated with the tumour profiling test (in the intervention group only), adjuvant chemotherapy acquisition and administration and associated toxicity, endocrine therapy (all patients), routine follow-up visits and tests, additional therapies [zoledronic acid and granulocyte colony-stimulating factor (G-CSF)], treatments for local recurrence and treatments for distant metastases. The costs and health outcomes for each Markov node differ owing to the different risks of recurrence associated with each tumour profiling test and whether or not chemotherapy is given (together with its associated benefits, AEs and costs).
Key External Assessment Group model assumptions
The EAG model makes the following structural assumptions:
-
Within the base-case analysis, the proportion of patients who receive chemotherapy under current practice (no test) is assumed to be the same for each test risk classification (low, intermediate and high risk). However, this proportion is assumed to differ between subgroups defined in accordance with clinical risk (LN0 NPI ≤ 3.4, LN0 NPI > 3.4, LN1–3, MINDACT ITT, MINDACT mAOL low risk and MINDACT mAOL high risk).
-
The model assumes that clinicians interpret each of the three-level tests in the same way (e.g. an oncotype DX high-risk score would lead to the same chemotherapy decision as a Prosigna high-risk score). The model also assumes that clinicians interpret each of the two-level tests in the same way (a MammaPrint high-risk score would lead to the same chemotherapy decision as an EPClin high-risk score).
-
Within the base-case analysis, the relative benefit of adjuvant chemotherapy is assumed to be the same across all risk score categories for all tests (the same RR is applied to all patients, irrespective of test risk score). The impact of assuming a predictive benefit for oncotype DX, which is applied by assuming different RRs between test risk score categories, is explored within the sensitivity analyses.
-
The impact of the tests is modelled by changing which patients receive adjuvant chemotherapy.
-
A proportion of patients who develop distant metastases are assumed to have previously developed local recurrence. Local recurrence is not modelled as a separate event or health state. QALY losses and costs associated with local recurrence are applied once only (on entry into the distant metastases state).
-
A disutility associated with short-term AEs related to adjuvant chemotherapy is applied once during the first model cycle only (while the patient is receiving treatment).
-
Patients can enter the long-term AEs (AML) health state from either the recurrence-free state or the distant metastases state. The prognosis of patients with AML and the costs and QALYs accrued within the AML state are assumed to be independent of whether or not the patient has previously developed distant metastases due to their breast cancer. Once a patient develops AML, the model assumes that this alone determines their survival prognosis. Although congestive heart failure (CHF) is also a potentially relevant long-term AE associated with chemotherapy, this was excluded from the model owing to a lack of evidence on the joint survival impact of CHF and metastatic breast cancer. The importance of AEs not included in the model on the cost-effectiveness of the tests is explored within sensitivity analyses.
-
Costs associated with endocrine therapy, bisphosphonates (zoledronic acid), follow-up appointments and mammograms are assumed to differ in accordance with time since model entry.
-
Across all three analysis subgroups, patients are assumed to enter the model aged 58 years, based on the mean age of patients in the NHS England Access Scheme Database183 (rounded down to an integer value).
-
The model includes both premenopausal and postmenopausal women. However, the TransATAC study relates only to postmenopausal women.
Evidence sources used to inform the model parameters
Table 34 summarises the evidence sources used to inform the parameters of the EAG model. The individual parameter values are discussed in further detail in the subsequent sections; data tables relating to these model inputs can be found in Appendix 8.
Parameter group | Source |
---|---|
Patient age | Based on the NHS England Access Scheme Database183 |
Risk classification probabilities for oncotype DX, EPClin, Prosigna and IHC4+C | TransATAC bespoke data request.46 Analysed by subgroup [LN0 NPI ≤ 3.4, LN0 NPI > 3.4 and LN1–3] |
Risk classification probabilities for MammaPrint | MINDACT.98 Analysed in accordance with ITT trial population and mAOL low-risk and high-risk subgroups |
Distant recurrence rates (10 years) conditional on test risk classification (oncotype DX, EPClin, Prosigna and IHC4+C) | TransATAC bespoke data request.46 Analysed by subgroup [LN0 NPI ≤ 3.4, LN0 NPI > 3.4 and LN1–3] |
Distant recurrence rates (10 years) conditional on test risk classification (MammaPrint) | MINDACT.98 Analysed in accordance with ITT trial population and mAOL low-risk and high-risk subgroups. All analyses involve extrapolation from the 5-year data |
Baseline probability of receiving adjuvant chemotherapy (current practice) |
LN0 NPI ≤ 3.4 subgroup: NCRAS bespoke data request184 LN0 NPI > 3.4 subgroup: NHS England Access Scheme Database183 LN1–3 subgroup: NCRAS bespoke data request184 MINDACT population (MammaPrint only): clinical judgement (Professor Robert Stein, UCL, 2017, estimates weighted by proportion with LN0 and LN+ disease and mAOL low risk/high risk) |
Probability of receiving chemotherapy conditional on results of test (three-level tests – oncotype DX, IHC4+C and Prosigna) |
LN0 NPI ≤ 3.4 subgroup: UKBCG survey (see Appendix 9) LN0 NPI > 3.4 subgroup: NHS England Access Scheme Database183 LN1–3 subgroup: Loncaster et al. 145 |
Probability of receiving chemotherapy conditional on results of test (two-level tests – EPClin and MammaPrint) | Bloomfield et al.159 Applied to all analysis subgroups |
10-year relative ROR for chemotherapy vs. no chemotherapy | EBCTCG 2012 meta-analysis185 |
Predictive chemotherapy benefit: oncotype DX (applied in sensitivity analysis only) |
LN0 subgroups: Paik et al. 50 LN1–3 subgroup: Albain et al. 53 |
Probability of death following distant recurrence | Thomas et al.186 |
Probability of local recurrence | de Bock et al.187 |
Probability of AML | Wolff et al.188 |
Probability of death following onset of AML | Edlin et al.189 |
Other-cause mortality (life tables) | ONS190 |
HRQoL |
Utility – recurrence-free and distant recurrence: Lidgren et al. 191 Utility AML: Younis et al. 192 HRQoL decrement – local recurrence and AEs related to adjuvant chemotherapy: Campbell et al. 193 |
Tumour profiling test costs | Test manufacturers |
Costs | |
Adjuvant chemotherapy | Hall et al.30 |
Endocrine therapy | BNF194 |
G-CSF | BNF194 and PSSRU195 |
Routine follow-up | NHS Reference Costs 2015/16196 and Campbell et al.193 |
Bisphosphonates (zoledronic acid) | BNF194 and NHS Reference Costs 2015/16196 |
Local recurrence | Karnon et al.197 |
Distant metastases | Thomas et al.186 |
Patient age
The mean age was assumed to be 58 years, based on the NHS England Access Scheme Database183 (rounded down to an integer value).
Risk classification probabilities using each test: oncotype DX, Prosigna, IHC4+C and EPClin
Data relating to risk classification probabilities for each test were obtained from a bespoke analysis of the TransATAC trial provided by the trial investigators46 (see Appendix 8, Table 76). As discussed in Chapter 2, the ATAC trial evaluated the efficacy and safety of anastrozole versus tamoxifen. The TransATAC trial tested tumour blocks from postmenopausal patients who had been included in the monotherapy arms of the Arimidex, Tamoxifen, Alone or in Combination (ATAC) trial198 in order to determine whether or not the tests could provide independent information on the risk of distant recurrence. Separate data analyses were provided by the trial investigators for ER+, HER2– patients for the three modelled subgroups [LN0 NPI ≤ 3.4, LN0 NPI > 3.4 and LN1–3]. In order to maximise the information available for each test, data were not restricted to only those with information on all four tests. The EAG considers that the use of this study has particular value as (1) it includes the use of four of the five tests included in the final NICE scope (oncotype DX, Prosigna, IHC4+C and EPClin) within the same patient population, (2) it provides a source of data on 10-year DMFI probabilities conditional on test risk classification, thereby avoiding confounding due to the use of different evidence sources for these parameters, and (3) TransATAC is a large UK study. However, two caveats should be noted with respect to the choice of this data source. First, the non-restricted TransATAC data set was used for the analysis – this maximises the sample size for each individual test; however, as each additional test was analysed, more tissue was required and for some samples insufficient tissue was left. This reduces the number of patients with available data and may introduce bias comparing across tests. In addition, whereas the ATAC trial included only postmenopausal women, the economic analysis assumes that the risk classification and DMFI probabilities obtained from the TransATAC analysis can be translated to a premenopausal population; this assumption introduces an additional degree of uncertainty with respect to the generalisability of the analysis.
Risk classification probabilities: MammaPrint
The evaluation of MammaPrint was based on the MINDACT trial. 98 This study was selected for inclusion in the analysis for three reasons: (1) the trial publication and supplementary material provide sufficient information to estimate risk classification probabilities and DMFS probabilities conditional on risk classification within the same patient populations, (2) it includes a large sample size and (3) the study allows for the estimation of the benefit of chemotherapy between discordant groups.
Risk classification probabilities for MammaPrint were obtained from the trial publication of the MINDACT trial98 and the accompanying supplementary material (see Appendix 8, Table 77).
Probability of developing distant metastases (without chemotherapy): oncotype DX, Prosigna, IHC4+C and EPClin
The probability of developing distant metastases was based on 10-year DMFI/DMFS outcomes for each test risk classification. For oncotype DX, Prosigna, IHC4+C and EPClin, these were obtained from a bespoke data analysis of the TransATAC study46 (see Appendix 8, Table 78).
The 10-year DMFI probability was converted to a cumulative probability of recurrence for each test within each risk classification category (1 – DMFI) and converted to a 6-month probability of distant recurrence assuming a constant rate.
Probability of developing distant metastases (without chemotherapy): MammaPrint
Cardoso et al. 98 report 5-year DMFS probabilities for patients who did, or did not, receive adjuvant chemotherapy in the discordant risk groups in the MINDACT trial. 98 Additional information is also provided on chemotherapy use and 5-year DMFS in the concordant risk groups. For the economic analysis based on the MINDACT ITT population, it was necessary to estimate DMFS probabilities for all concordant and discordant groups in accordance with clinical and genomic risk classification and whether or not patients received chemotherapy. This was done as follows (refer to data presented in Appendix 8, Table 79):
-
The 10-year DMFS outcomes were estimated for all concordant and discordant clinical and genomic risk groups in accordance with whether or not patients received adjuvant chemotherapy (EAG group labels A–H) based on 5-year DMFS outcomes, assuming a constant event rate. The proportions of patients who received chemotherapy were obtained from the supplementary material of the Cardoso et al. 98 trial publication. An adjustment was made to the mAOL high-risk, MammaPrint high-risk group to estimate counterfactual 10-year DMFS for patients not receiving chemotherapy (EAG group label H); this was done by estimating the 10-year DMFS probability for this group (with chemotherapy) and multiplying this value by the reciprocal of the estimated 10-year RR of distant metastases for chemotherapy versus no chemotherapy for the overall discordant population (RR 0.77, adjusted 10-year DMFS for group 0.766).
-
The 10-year DMFS outcomes for the MammaPrint low-risk group (without adjuvant chemotherapy) were estimated by weighting the estimated 10-year DMFS probabilities for the MammaPrint low-risk no-chemotherapy groups (EAG group labels B and D) in accordance with the number of mAOL low-risk and high-risk patients in these groups.
-
The 10-year DMFS outcomes for the MammaPrint high-risk group (without adjuvant chemotherapy) were estimated by weighting the estimated 10-year DMFS probabilities for the genomic high-risk no-chemotherapy groups (EAG group labels F and H, including the adjustment described above) in accordance with the number of mAOL low-risk and high-risk patients in these groups.
Tapering of risk of recurrence over time
The EAG notes that there is uncertainty with respect to the long-term risk of distant recurrence. The EAG model makes the same assumptions regarding long-term distant metastasis risk as the previous model reported by Ward et al. 1 The model assumes that the risk of distant metastases between 10 and 15 years is equal to half of the risk during the preceding period (0–10 years); beyond 15-years, the risk of distant recurrence is assumed to be zero. The EAG notes that this is a simplification. This general decrease in the hazard of recurrence can be seen in the 10- to 15-year control arm annualised recurrence data reported in the 2005 EBCTCG meta-analysis. 199 Although there is some evidence that suggests that for some patients with particular disease subtypes, recurrence rates remain approximately constant between 5 and 20 years,200 there is also uncertainty surrounding the duration over which the benefit of chemotherapy is sustained; hence, constraining recurrence at 15 years reduces the likelihood of overestimating this benefit of chemotherapy. The impact of removing this assumption of recurrence risk tapering is explored within the sensitivity analyses.
Probability of receiving chemotherapy in the current practice group
The EAG identified two empirical sources that could be used to inform the probability that a patient receives chemotherapy without tumour profile testing: (1) the NCRAS data set184 and (2) the NHS England Access Scheme Database (intermediate clinical risk only). 183 These alternative sources are discussed briefly in the following sections.
National Cancer Registration and Analysis Service data set
A bespoke data request was placed with the NCRAS to obtain aggregate data relating to the use of adjuvant chemotherapy in women with early-stage breast cancer in England (see Appendix 8, Table 80). The NCRAS cancer registration data sets were used to estimate current levels of chemotherapy use in each of the three model subgroups [LN0 NPI ≤ 3.4, LN0 NPI > 3.4 and LN1–3]. An age restriction of 55–75 years was applied with the intention of only selecting those patients who were sufficiently fit to undergo chemotherapy and therefore may benefit from tumour profile testing, and also of removing younger patients who are more likely to receive chemotherapy and are less reflective of the populations used to estimate risk classification probabilities and distant recurrence risk. 46 An additional data analysis on chemotherapy use for the whole population aged < 75 years was also obtained. As shown in Appendix 8, Table 80, within the 55- to 75-year age group, the proportions of women receiving chemotherapy are 7.19%, 40.01% and 62.72% in the LN0 NPI ≤ 3.4, LN0 NPI > 3.4 and LN1–3 subgroups, respectively. As expected, the proportion of women receiving chemotherapy is higher in the broader aged ≤ 75 years population.
It should be noted that the NCRAS data set reflects an unselected population who are not necessarily eligible for tumour profile testing; this may increase the size of the denominator and, hence, in reality, the proportion of women who are eligible for testing who go on to receive adjuvant chemotherapy may be greater than the estimates generated using this data set.
NHS England oncotype DX Access Scheme Database
The NHS England Access Scheme Database183 contains data on the pre-test chemotherapy decision for women who received the oncotype DX test in England. It should be noted that this data set relates only to women who were deemed to be at intermediate clinical risk, hence the data may not provide a good reflection of pre- and post-test chemotherapy decision-making for women with LN0 disease and a NPI score of ≤ 3.4 or for women with LN+ disease. The pre-test probability of receiving adjuvant chemotherapy is 0.431. This estimate is only slightly higher than the estimate generated using the NCRAS data set184 (probability of 0.40).
Within the EAG base-case analysis, the following selections were made:
-
For the LN0 NPI ≤ 3.4 subgroup, the NCRAS data set184 was used as these are the only data on baseline chemotherapy use available for this patient subgroup.
-
For the LN1–3 subgroup, the NCRAS data set184 was used as these are the only data on baseline chemotherapy use available for this patient subgroup.
-
For the LN0 NPI > 3.4 subgroup, the NHS England Access Scheme Database183 was used. This source was selected on the basis of consistency: the same data set is used to inform the post-test probabilities of receiving chemotherapy conditional on risk score. It should also be noted that the collection of these data were requested by the NICE Diagnostics Appraisal Committee in NICE DG10. 20
-
For the MammaPrint analyses, the EAG is not aware of any empirical evidence source that provides estimates of baseline chemotherapy use (without testing) for patients who are mAOL high risk or mAOL low risk. For this reason, these parameters were informed by expert opinion (Professor Rob Stein, University College London, 2017, personal communication). The following estimates were applied in the model, based on the modified version of AOL applied in the MINDACT trial –
-
LN0, mAOL high risk, baseline chemotherapy probability = 70%
-
LN+, mAOL high risk, baseline chemotherapy probability = 90%
-
LN0, mAOL low risk, baseline chemotherapy probability = 15%
-
LN+, mAOL low risk, baseline chemotherapy probability = 30%.
-
These estimates were then weighted in accordance with the proportion of women with LN0 and LN+ disease within the overall MINDACT population and within the mAOL high-risk and low-risk subgroups. This leads to baseline probabilities of 0.46, 0.77 and 0.16 for the MINDACT overall trial population, the mAOL high-risk subgroup and the mAOL low-risk subgroup, respectively.
When appropriate, the source not selected for inclusion in the EAG base case was tested in the sensitivity analyses.
Probability of receiving chemotherapy conditional on test risk classification
Based on the review of decision impact studies presented in Chapter 2, Results: decision impact studies, five UK-based sources relating largely to the three analysis subgroups [LN0 NPI ≤ 3.4, LN0 NPI > 3.4 and LN1–3] were identified as providing potentially usable data relating to the probability that a patient receives adjuvant chemotherapy conditional on the risk score given by the tumour profiling test. Evidence selection for these parameters was focused on UK-based studies as these are more likely to reflect how clinicians will use the tests in England, although European studies were considered when the UK-based evidence was particularly limited (specifically for the two-level tests). The five UK-based studies identified are (1) the NHS England Access Scheme Database,183 (2) Holt et al. ,201 (3) Loncaster et al. ,145 (4) Bloomfield et al. 159 and (5) the UK Breast Cancer Group (UKBCG) survey (see Appendix 9). The advantages and disadvantages of using each of these studies are summarised in Table 35.
Study | Disease type | EAG comments |
---|---|---|
NHS England Access Scheme Database183 | LN0, intermediate clinical risk |
This data collection exercise was requested by the NICE Diagnostics Appraisal Committee within the guidance for NICE DG10. 20 The data set includes only patients with intermediate clinical risk and is likely to be relevant only to patients with LN0 disease and a NPI of > 3.4. The data relate to the actual chemotherapy decision rather than a recommendation The NHS England Access Scheme data were provided as AiC and cannot be reported here |
Holt et al.201 | LN0 or pN1mic (micrometastasis) | Prospective UK clinical study of the impact of oncotype DX on adjuvant treatment decisions and risk classification by NPI and oncotype DX recurrence score. Results were available for 74 patients. The data relate to the chemotherapy recommendation rather than the final decision. The EAG notes that this study has been published only in abstract form and few details are available regarding the methods |
Bloomfield et al.159 | Unclear |
UK study of decision impact of EndoPredict. Fourteen oncologists in eight UK hospitals saw 149 patients judged by clinical teams to have equivocal indications for chemotherapy. Patients and oncologists discussed provisional treatment decisions based on conventional prognostic factors. Initial decisions were reconsidered when EndoPredict results were available. The data appear to relate to the final decision rather than recommendations The EAG notes that this is the only available UK study that relates to decision impact with a two-level tumour profiling test. The population relates to patients for whom there was no clear decision on whether or not chemotherapy should be given. This study is unlikely to accurately represent the use of chemotherapy in women with LN+ disease |
Loncaster et al.145 | LN0 and LN+ |
A prospective UK pilot study designed to evaluate the clinical value of oncotype DX testing. Testing was carried out in 201 women with newly diagnosed, ER+, HER2–, invasive breast cancer who underwent breast surgery with curative intent. Separate estimates are provided for the LN0 and LN+ subgroups. The data appear to relate to recommendations rather than the final decision The EAG notes that patients enrolled in this study had already been recommended chemotherapy, therefore the use of this study may exaggerate the proportion of women for whom the final decision was to receive chemotherapy |
UKBCG survey (see Appendix 9) | LN0 NPI ≤ 3.4, LN0 NPI > 3.4 and LN1–3 |
The UKBCG network disseminated a bespoke unfunded survey designed by the EAG to its members (see Appendix 9). Respondents were asked ‘Based on your own subjective opinion, please estimate the probability that a woman in each of these subgroups and with each genomic/immunohistochemical test result would go on to receive adjuvant chemotherapy’. Responses were requested for two-level and three-level tests for the LN0 NPI ≤ 3.4, LN0 NPI > 3.4 and LN1–3 subgroups. Eleven usable responses were received from participating oncologists The results indicate considerable variation in practice. Several respondents noted uncertainty with respect to the two-level tests as they do not currently have access to these technologies |
Estimates of the use of adjuvant chemotherapy conditional on test risk classification based on these alternative sources are summarised in Appendix 8, Table 81.
With respect to the EAG base case, the following study selections were made:
-
For the use of the three-level tests (oncotype DX, Prosigna and IHC4+C) in the LN0 NPI ≤ 3.4 subgroup, the UKBCG survey data were used. This selection was made owing to the absence of any published UK evidence on the decision impact of tumour profiling tests in this patient subgroup.
-
For the use of the three-level tests (oncotype DX, Prosigna and IHC4+C) in the LN0 NPI > 3.4 subgroup, the NHS England Access Scheme Database183 was used. This selection was made for two reasons: (1) this source is consistent with the source used to inform the baseline chemotherapy probabilities without testing and (2) the EAG considers that this source provides the best reflection of the way in which three-level tumour profiling tests are used in clinical practice in England.
-
For the use of the three-level tests (oncotype DX, Prosigna and IHC4+C) in the LN1–3 subgroup, the Loncaster et al. 145 LN+ estimates were selected as this is the only published UK evidence on decision impact that specifically relates to this patient subgroup.
-
For the two-level tests (EPClin and MammaPrint), the Bloomfield et al. 159 study was selected for use in all three analysis subgroups as this is the only available published UK study that relates to a two-level tumour profiling test. Given the limited UK-based evidence relating to the impact of two-level tests, two additional European studies are explored in the sensitivity analyses. 162,167
The other sources not selected for inclusion in the EAG base case were included in the sensitivity analyses.
Adjuvant chemotherapy treatment effect on distant recurrence: oncotype DX, Prosigna, IHC4+C and EPClin
As noted in Chapter 2, Chemotherapy benefit: oncotype DX, the evidence relating to the predictive benefit of oncotype DX is limited to two reanalyses of RCTs50,53 that do not provide consistent conclusions regarding this aspect of the value of the test across the range of analyses reported. Within the base-case analysis, all tests are assumed to be associated with prognostic benefit only (the relative benefit of chemotherapy is assumed to be the same across all test risk classification groups). For the analyses of oncotype DX, Prosigna, IHC4+C and EPClin, the RR of recurrence for chemotherapy versus no chemotherapy was derived from a meta-analysis reported by the EBCTCG (2012). 185 Based on data presented in the supplementary material (see EBCTCG publication185 extra web material, page 12, any anthracycline-based regimen vs. no chemotherapy, distant recurrence), the 10-year risk of distant recurrence for chemotherapy and no chemotherapy was estimated by projecting forward the annualised risk of distant metastases (3.3% per year for chemotherapy, 4.6% per year for no chemotherapy). The RR for chemotherapy versus no chemotherapy was then calculated based on the difference between the projected 10-year DMFS probabilities for the two groups: this gives a 10-year RR of 0.76. The same RR was assumed to apply to the LN0 and LN+ subgroups. The impact of assuming higher and lower RRs for distant recurrence are explored in the sensitivity analyses. Further sensitivity analyses were also undertaken to explore the impact of assuming a predictive benefit of chemotherapy associated with oncotype DX, based on the studies reported by Paik et al. 50 (LN0) and Albain et al. 53 (LN+). Within the model, this is implemented by applying different RRs to each of the risk classification groups, based on these two studies.
Adjuvant chemotherapy treatment effect on distant recurrence: MammaPrint
Within the analysis of MammaPrint, the benefit of adjuvant chemotherapy was estimated using data reported within the MINDACT trial publication,98 rather than from an external source. The 10-year RR of relapse for adjuvant chemotherapy versus no adjuvant chemotherapy was estimated based only on the discordant clinical and genomic risk group data (see Appendix 8, Table 79, EAG group labels C, D, E and F), extrapolated beyond the study end point. RRs of chemotherapy versus no chemotherapy were calculated for each of the two discordant groups (clinical low, genomic high, and clinical high, genomic low) based on estimated 10-year DMFS; these were then weighted in accordance with the number of patients in each discordant group. The weighted RR for the discordant populations was estimated to be 0.77. Within the mAOL low-risk and mAOL high-risk subgroups, the RRs of recurrence for each subgroup were based only on the discordant population relevant to that subgroup (mAOL low-risk RR 0.84, mAOL high-risk RR 0.74). The impact of assuming higher and lower RRs for distant recurrence are explored in the sensitivity analyses.
The RRs of recurrence applied in the EAG base-case analysis and sensitivity analyses are detailed in Appendix 8, Table 78.
It should be noted that the model translates 10-year DMFS probabilities (without chemotherapy) into 6-month event probabilities assuming a constant rate. As a RR relates only to the specified time point of the analysis, it is inappropriate to apply this directly to the 6-month probability of recurrence. Instead, the EAG model applies a conversion by (1) estimating the 10-year DMFS probability with chemotherapy based on the 10-year DMFS probability without chemotherapy and the 10-year RR of recurrence for chemotherapy versus no chemotherapy; (2) estimating the HR for the DMFS outcomes at 10 years for chemotherapy versus no chemotherapy, assuming a constant event rate; (3) applying the estimated HR to the 6-month DMFS probability for the no-chemotherapy group; and (4) converting this HR-adjusted 6-month DMFS probability with chemotherapy to a 6-month distant recurrence probability. This approach ensures that the relative distance between the predicted chemotherapy group and the observed no-chemotherapy group is maintained at 10 years.
Survival following onset of distant metastases
The survival prognosis of patients with distant metastases was based on analysis of complete hospital and community records of 77 women randomly selected from 232 women who had relapsed breast cancer between 2000 and 2005 (Thomas et al. 186). The population included in this study had an average age of 62.3 years and included patients who had originally been diagnosed with LN+ disease (44%) and who were LN0 (56%). Forty-five per cent of women were ER+ and 21% of women were HER2+. Median survival was reported to be 40.1 months following distant recurrence. The 6-month probability of death was estimated by fitting an exponential distribution with a median of 40.1 months; based this approach, the 6-month probability of death following distant recurrence was estimated to be 0.098, assuming a constant rate. The model assumes that the rate of death due to distant metastases is constant across the different model subgroups and across each test risk classification group owing to a lack of population-specific or risk-group-specific data.
Probability of local recurrence
The model assumes that 10.5% of patients entering the distant recurrence health state have previously experienced a local recurrence. This estimate was based on a study by de Bock et al. ,187 which analysed 3601 women enrolled in three EORTC (European Organisation for Research and Treatment of Cancer) trials. The study included both LN0 and LN+ women who had been treated for early-stage breast cancer. Of the 1224 women who developed distant metastases, 129 women (10.54%) experienced a previous locoregional recurrence. The model does not take into account the time spent alive with local recurrence; instead, the impact of local recurrence is applied crudely in the model as a once-only cost and QALY loss.
Probability of developing acute myeloid leukaemia
The probability of developing AML following chemotherapy was taken from an analysis of 20,063 patients with stage I–III breast cancer treated at US academic centres between 1998 and 2007 (Wolff et al. 188). Within the cohort of 3227 patients, the estimated 10-year risk of developing AML was reported to be 0.49%. The 6-month probability of developing AML was estimated to be 0.00025, assuming a constant event rate.
Survival following onset of acute myeloid leukaemia
Survival following the onset of AML was estimated from the NICE single technology appraisal of azacitidine (Vidaza®, Celgene) for myelodysplastic syndromes (MDSs). 202 Within this appraisal, the manufacturer estimated mean survival following the onset of AML to be approximately 8 months; assuming a constant event rate, this gives a 6-month probability of death following AML of 0.53.
Health utilities associated with relapse-free and distant metastases
Systematic searches were undertaken to identify studies reporting on HRQoL associated with different health states for women with breast cancer. Searches were undertaken in July 2017 in the following electronic databases:
-
MEDLINE Epub Ahead of Print, In-Process & Other Non-Indexed Citations (Ovid, 1946 to present)
-
EMBASE (Ovid, 1974 to 7 July 2017)
-
Science Citation Index Expanded (Web of Science, 1900 to present)
-
Conference Proceedings Citation Index – Science (Web of Science, 1990 to present).
The searches specifically focused on studies that reported HRQoL estimates for health states that were measured and valued using the Euroqol 5-Dimensions (EQ-5D). The search strategy comprised sensitive MeSH or Emtree Thesauri terms and free-text synonyms for ‘breast cancer’ combined with free-text synonyms for ‘EQ-5D’. The search strategies are presented in Appendix 2. Studies were considered potentially relevant if they reported EQ-5D valuations in patients both with non-metastatic disease and with relapsed disease, thereby reflecting key health states in the model. Studies that reported disutilities associated with AEs resulting from the use of chemotherapy were retained for separate consideration. Studies were sifted in accordance with their titles and abstracts; full texts were retrieved for studies that potentially met the inclusion criteria on the basis of the information provided in their title and abstract. HRQoL estimates for other modelled health states and events were not based on new searches; instead, these were derived through consideration of estimates that have been identified from a previous systematic review of health utilities (Peasgood et al. 203).
The EAG’s searches identified a total of 227 studies. Of these, only four studies reported EQ-5D valuations for both non-metastatic and metastatic breast cancer states (see Appendix 8, Table 83). Three of the identified studies were reported as full papers, and the fourth study was reported only in abstract form. None of the identified studies were undertaken with UK patients: the studies were undertaken in Finland (Farkkila et al. 204), Sweden (Lidgren et al. 191), Iran (Yousefi et al. 205) and Canada (Naik et al. 206).
The study reported by Lidgren et al. 191 was selected for use in the EAG base-case analysis on the basis that this population was most likely to best reflect the population of ER+ women with breast cancer who are treated in England. This study reported values for the recurrence-free (receiving endocrine therapy) health state and for the distant recurrence health state of 0.824 and 0.685, respectively. This same study was used to inform the health state utility estimates within the earlier model reported by Ward et al. 1 and the Myriad model. 182
Health utilities associated with other model health states and events
The disutility associated with local recurrence was taken from a published model of first-, second- and third-generation adjuvant chemotherapy regimens for breast cancer reported by Campbell et al. 193 Within this study, the 6-month disutility associated with local recurrence was estimated to be 0.108 [standard error (SE) 0.04]. The HRQoL impact of chemotherapy-related AEs was also taken from Campbell et al. ;193 the model assumes a disutility of 0.04 (assumed SE 0.004) during the first 6-month model cycle. The health utility associated with AML was assumed to be 0.26 based on a previous economic evaluation. 192
Health utility estimates applied in the External Assessment Group model
Appendix 8, Table 84, summarises the health utilities assumed in the EAG’s base-case analysis.
Resource use and costs
The model includes the following cost components:
-
costs associated with the tumour profiling test
-
costs of adjuvant chemotherapy acquisition and administration (including chemotherapy-related toxicity)
-
costs associated with endocrine therapy
-
costs of routine follow-up visits and tests
-
costs of other therapies (zoledronic acid and G-CSF)
-
costs of treating local recurrence (once-only cost)
-
costs associated with treating distant metastases.
Test costs
The costs of the tumour profiling tests were sourced from information provided to NICE by the manufacturers as part of the appraisal process. The cost of oncotype DX includes the price discount offered through the Patient Access Scheme (PAS) for this product. The manufacturers of oncotype DX, MammaPrint and EndoPredict submitted a cost for sample testing in each of their centralised laboratories. IHC4 and Prosigna have no established centralised laboratory system. The manufacturers provided prices for conducting these two tests in NHS laboratories, as outlined in Appendix 8, Table 85. NanoString Technologies submitted a cost of £1970; this is in line with the £1596 (2013 prices) cost of the Prosigna test estimated as part of the OPTIMA Prelim trial. 207 EndoPredict can also be conducted within a NHS laboratory; the impact of assuming a lower cost is considered within the sensitivity analyses. During the course of the appraisal, access proposals were offered by several of the test manufacturers; the impact of these proposals is not included in this report.
Costs of adjuvant chemotherapy acquisition and administration (including toxicity)
The costs associated with adjuvant chemotherapy were obtained from a previous costing analysis undertaken to inform the economic analysis of the OPTIMA Prelim trial (Hall et al. ,30 see Appendix 8, Table 86). The fully executable spreadsheet developed to inform the OPTIMA Prelim analysis was made available to the EAG by the study authors (Professor Robert Stein, University College London, personal communication). Within this analysis, standard supportive medication, procurement, laboratory, pharmacy and administration costs were taken from the drugs and pharmaceutical electronic market information tool,208 the British National Formulary (BNF)194 and NHS Reference Costs 2013 to 2014. 209 Unit costs associated with the management of chemotherapy-related grade 3/4 toxicity were based on NHS Reference Costs 2013 to 2014. 209 Within the original costing analysis, all costs were valued at 2013/14 prices; within the EAG analysis, these costs were uplifted to current values using the Hospital and Community Health Service (HCHS) indices. 195 The EAG model assumes that women with ER+, HER2–, early-stage breast cancer with zero to three nodes typically receive one of four adjuvant chemotherapy regimens:
-
fluorouracil, epirubicin, cyclophosphamide and docetaxel (FEC100-T) (three plus three cycles, assumed to be given to 25% of patients)
-
docetaxel and cyclophosphamide (TC) (four cycles, assumed to be given to 20% of patients)
-
fluorouracil, epirubicin and cyclophosphamide (FEC75) (six cycles, assumed to be given to 45% of patients)
-
fluorouracil, epirubicin, cyclophosphamide and weekly paclitaxel (FEC100-Pw) (three plus three cycles, assumed to be given to 10% of patients).
The weighted mean cost of adjuvant chemotherapy acquisition, delivery and toxicity was estimated to be £3145.19 per course. All adjuvant chemotherapy costs are applied during the first model cycle. The EAG notes that the choice and proportionate use of alternative chemotherapy regimens may differ between centres; for this reason, the use of alternative chemotherapy cost assumptions are explored in the sensitivity analyses.
Costs of endocrine therapy
The model assumes that all surviving patients receive endocrine therapy for a period of between 5 and 8 years. The costs associated with endocrine therapy were based on the assumptions employed within the previous economic analysis reported by Ward et al. 1 The model assumes that patients may receive one of four endocrine therapy regimens: (1) tamoxifen for 5 years, (2) anastrozole for 5 years, (3) letrozole for 5 years or (4) tamoxifen for 2 years then exemestane for 3 years. The proportion of patients receiving each regimen was taken from Ward et al. 1 (tamoxifen 40% of patients, anastrozole 20% of patients, letrozole 20% of patients, tamoxifen then exemestane 20% of patients; see Appendix 8, Table 87). In line with the previous model reported by Ward et al. ,1 10% of patients are assumed to receive extended letrozole for 3 further years (years 6–8).
Costs of additional treatments (zoledronic acid)
The model assumes that 30% of women with early-stage breast cancer will receive 4 mg of bisphosphonates (zoledronic acid) every 6 months by intravenous infusion for up to 3 years (cost per 36-month course = £58.50). Treatment is assumed to be given in a day-case setting, based on the cost of delivering simple parenteral chemotherapy (unit cost of £199.94, based on NHS Reference Costs 2015 to 2016,196 outpatient, currency code SB12Z).
Follow-up costs
The model assumes that all patients receive two routine follow-up visits during the first year following surgery, with annual visits thereafter for a period of 5 years. Patients are also assumed to undergo a routine annual mammogram for up to 5 years. The cost of a routine follow-up visit was taken from NHS Reference Costs 2015 to 2016196 (mean cost £162.84, SE £6.48, consultant-led, non-admitted, face-to-face attendance, follow-up, medical oncology, service code 370). The cost of a mammogram was not available within the NHS Reference Costs 2015 to 2016 tariff: this unit cost was instead taken from Campbell et al. 193 (mean cost £46.37, SE £9.27) and uplifted to current values using the HCHS index. 195
Costs of treatments for local and distant recurrence
The costs associated with treating local recurrence were taken from a UK-based patient-level costing analysis of breast cancer recurrence reported by Karnon et al. 197 This cost estimate was uplifted to current prices using the HCHS index195 (uplifted mean cost £13,912.92, assumed SE £2010.20). This is applied as a once-only cost on the incidence of distant recurrence.
The costs associated with treating distant metastases were derived from the study reported by Thomas et al. 186 These costs included costs associated with visits, drugs, pharmacy, hospital admission and intervention, imaging, radiotherapy, pathology and transport. Cost components specifically associated with terminal care were excluded. The 6-monthly cost of treating metastatic breast cancer was estimated to be £4082. This estimate was uplifted to current prices using the HCHS index195 (uplifted mean cost £4541, assumed SE £908.13).
Methods for model evaluation
The incremental health outcomes and costs of each test versus standard care were evaluated in a pairwise fashion; the cost-effectiveness of each test was not compared against the other tests. Central estimates of cost-effectiveness were based on the expectation of the mean. Uncertainty was evaluated using probabilistic sensitivity analysis (PSA) and deterministic sensitivity analyses (DSAs). PSA was undertaken using simple Monte Carlo sampling methods (10,000 samples). The choice of distribution assumed for each group of parameters in the model is summarised in Table 36. The results of the PSA are presented as cost-effectiveness acceptability curves (CEACs) and in tabular form. DSAs were undertaken to explore the impact of alternative assumptions and evidence sources regarding the probability of receiving chemotherapy with and without the tests, risk classification probabilities, recurrence rates, the potential predictive benefit of oncotype DX, the magnitude of chemotherapy benefit, HRQoL estimates and costs.
Model parameter group | Distribution | EAG comments |
---|---|---|
Classification probabilities with/without test | Dirichlet | – |
Chemotherapy use (conditional on test result) | Beta | – |
Recurrence rates (conditional on test result) | Beta | Distribution parameters fitted to 95% CI around 10-year RFS data from TransATAC46 or based on number of patients in treatment/risk group in MINDACT98 |
RR chemotherapy vs. no chemotherapy | Log-normal | SE assumed |
Distant recurrence risk taper parameters | Fixed | – |
OS rate following distant recurrence | Beta | SE estimated using 95% CI of Kaplan–Meier curve in Thomas et al.186 |
Probability of local recurrence | Beta | – |
Probability of AML | Beta | – |
OS rate following incidence of AML | Beta | – |
HRQoL | Beta | – |
Chemotherapy costs | Normal | SE assumed to reflect uncertainty in delivery costs only |
Endocrine therapy costs | Fixed | – |
Zoledronic acid costs | Normal | SE for delivery costs estimated from NHS Reference Costs 2015 to 2016196 using interquartile ranges and number of submissions |
Mammogram costs | Normal | SE taken from Campbell et al.193 |
Follow-up/visit costs | Normal | SE estimated from NHS Reference Costs 2015 to 2016196 using interquartile range and number of submissions |
Local recurrence cost | Normal | SE assumed to be equal to 20% of mean |
Distant recurrence cost | Normal | SE assumed to be equal to 20% of mean |
AML cost (one off) | Normal | SE assumed to be equal to 20% of mean |
Test costs | Fixed | – |
The model was subjected to a number of DSAs, which are listed in Table 37.
DSA description | DSA undertaken for test? | ||||
---|---|---|---|---|---|
Oncotype DX | IHC4+C | Prosigna | EPClin | MammaPrint | |
Deterministic base-case analysis | Yes | Yes | Yes | Yes | Yes |
Post-test chemotherapy probabilities based on NHS England Access Scheme Database183 (clinical intermediate risk) (see Appendix 8, Table 82) | Yes | Yes | Yes | No | No |
Post-test chemotherapy probabilities based on Holt et al.201 (see Appendix 8, Table 82) | Yes | Yes | Yes | No | No |
Post-test chemotherapy probabilities based on Loncaster et al.145 (see Appendix 8, Table 82) | Yes | Yes | Yes | No | No |
Post-test chemotherapy probabilities based on UKBCG survey (see Appendix 8, Table 82) | Yes | Yes | Yes | Yes | Yes |
Post-test chemotherapy probabilities based on NHS England Access Scheme Database183 (see Appendix 8, Table 83); baseline chemotherapy probabilities from NCRAS184 (see Appendix 8, Table 81) | Yes | Yes | Yes | No | No |
Post-test chemotherapy probabilities based on Holt et al.201 (see Appendix 8, Table 83); baseline chemotherapy probabilities from NCRAS184 (see Appendix 8, Table 81) | Yes | Yes | Yes | No | No |
Post-test chemotherapy probabilities based on Loncaster et al.145 (see Appendix 8, Table 83); baseline chemotherapy probabilities from NCRAS184 (see Appendix 8, Table 81) | Yes | Yes | Yes | No | No |
Post-test chemotherapy probabilities based on UKBCG survey (see Appendix 8, Table 83); baseline chemotherapy probabilities from NCRAS184 (see Appendix 8, Table 81) | Yes | Yes | Yes | No | No |
Post-test chemotherapy probabilities based on Cusumano et al.167 (see Appendix 8, Table 89) | No | No | No | Yes | Yes |
Post-test chemotherapy probabilities based on Penault-Llorca et al.162 (LN0 only, NPI > 3.4 assumed) (see Appendix 8, Table 90) | No | No | No | Yes | Yes |
Baseline chemotherapy probabilities adjusted by oncotype DX risk score (see Appendix 8, Table 91) | Yes | No | No | No | No |
Chemotherapy assumptions (with and without test) based on Ward et al.1 (LN0, NPI > 3.4 only) | Yes | Yes | Yes | No | No |
Oncotype DX benefit assumed to be associated with predictive benefit. (LN0 RRs based on Paik et al.50 – low risk 1.31, intermediate risk 0.61, high risk 0.26; LN+ RRs based on Albain et al.53 – low risk 1.02, intermediate risk 0.72, high risk 0.59) | Yes | No | No | No | No |
Risk classification and distant metastases probabilities based on oncotype DX RPSC46 (LN0 only) (see Appendix 8, Table 92) | Yes | No | No | No | No |
Prosigna risk classification and distant metastases probabilities derived from Gnant et al.104 (LN+ only) (see Appendix 8, Table 93) | No | No | Yes | No | No |
EPClin risk classification and distant metastases probabilities derived from Dubsky et al.120 (LN+ only) (see Appendix 8, Table 94) | No | No | No | Yes | No |
MammaPrint risk classification and distant metastases probabilities derived from van ’t Veer et al.91 (LN0 only) (see Appendix 8, Table 95) | No | No | No | No | Yes |
Subgroup analysis in ER+, HER2–, LN+ subgroup | No | No | No | No | Yes |
Assume MammaPrint low-risk patients receive no chemotherapy, MammaPrint high-risk patients receive 100% chemotherapy | No | No | No | No | Yes |
10% lower cost per test owing to increased efficiency (local NHS testing) | No | No | Yes | Yes | No |
10% higher cost per test owing to decreased efficiency (local NHS testing) | No | No | Yes | No | No |
Baseline chemotherapy use halved | No | No | No | No | Yes |
Start age based on TransATAC37 (64 years) | Yes | Yes | Yes | Yes | Yes |
RR of distant metastases for chemotherapy vs. no chemotherapy = 0.70 | Yes | Yes | Yes | Yes | Yes |
RR of distant metastases for chemotherapy vs. no chemotherapy = 0.80 | Yes | Yes | Yes | Yes | Yes |
Removal of distant metastases risk tapering | Yes | Yes | Yes | Yes | Yes |
Utilities derived from Farkkila et al.204 (RFS = 0.818, DM = 0.746) | Yes | Yes | Yes | Yes | Yes |
Distant metastases death rate doubled | Yes | Yes | Yes | Yes | Yes |
Distant metastases death rate halved | Yes | Yes | Yes | Yes | Yes |
AML removed from model | Yes | Yes | Yes | Yes | Yes |
Chemotherapy cost doubled | Yes | Yes | Yes | Yes | Yes |
Chemotherapy cost halved | Yes | Yes | Yes | Yes | Yes |
Endocrine therapy costs doubled | Yes | Yes | Yes | Yes | Yes |
Endocrine therapy costs halved | Yes | Yes | Yes | Yes | Yes |
Local and distant metastases costs doubled | Yes | Yes | Yes | Yes | Yes |
Local and distant metastases costs halved | Yes | Yes | Yes | Yes | Yes |
Model verification and validation
The EAG undertook a number of measures to ensure the credibility of the model:
-
peer review of the economic analysis by a modeller not involved in the assessment
-
verification and scrutiny of the executable model by two model developers
-
double-programming of the deterministic version of the model for all pairwise comparisons presented in the EAG base case
-
double-checking of the accuracy of all model inputs against sources
-
comparison of model results using point estimates of parameters and the expectation of the mean
-
comparison of mean of all probabilistic parameter samples against point estimates of parameters
-
examination of all identified sources of discrepancy
-
model testing using sensitivity analysis and use of extreme parameter values.
Cost-effectiveness results
Oncotype DX versus current practice
Central estimates of cost-effectiveness: probabilistic
Central estimates of cost-effectiveness for oncotype DX versus current practice are presented in Table 38. All estimates are based on the probabilistic version of the EAG model. Within the LN0 NPI ≤ 3.4 subgroup, oncotype DX is expected to produce 0.01 additional QALYs at an additional cost of £1313 per woman tested compared with current practice; this corresponds to an incremental cost-effectiveness ratio (ICER) of £122,725 per QALY gained. Within the LN0 NPI > 3.4 subgroup, oncotype DX is expected to produce 0.01 fewer QALYs at an additional cost of £881 per woman tested compared with current practice; within this subgroup, oncotype DX is expected to be dominated. Within the LN1–3 subgroup, oncotype DX is expected to produce 0.07 fewer QALYs at an additional cost of £687 per woman tested compared with current practice; within this subgroup, oncotype DX is, again, expected to be dominated. As shown in Table 39, the PSA indicates that the probability that oncotype DX produces more net benefit than current practice at willingness-to-pay (WTP) thresholds of £20,000 and £30,000 per QALY gained is ≤ 0.04 across all three subgroups. The results for the LN0 NPI > 3.4 subgroup and the LN1–3 subgroup are primarily driven by the lower use of chemotherapy (and the benefits forgone) with oncotype DX than with current practice (see Appendix 10 for the impact of all tests).
Option | QALYs | Costs | Incremental QALYs | Incremental costs | ICER (per QALY gained) |
---|---|---|---|---|---|
LN0 NPI ≤ 3.4 | |||||
Oncotype DX | 13.89 | £5474 | 0.01 | £1313 | £122,725 |
No test | 13.88 | £4161 | – | – | – |
LN0 NPI > 3.4 | |||||
Oncotype DX | 12.73 | £11,806 | –0.01 | £881 | Dominated |
No test | 12.74 | £10,925 | – | – | – |
LN1–3 | |||||
Oncotype DX | 12.48 | £13,212 | –0.07 | £687 | Dominated |
No test | 12.55 | £12,525 | – | – | – |
Subgroup | Probability (λ = £20,000 per QALY gained) | Probability (λ = £30,000 per QALY gained) | ||
---|---|---|---|---|
Oncotype DX | Current practice | Oncotype DX | Current practice | |
LN0 NPI ≤ 3.4 | 0.00 | 1.00 | 0.00 | 1.00 |
LN0 NPI > 3.4 | 0.01 | 0.99 | 0.04 | 0.96 |
LN1–3 | 0.00 | 1.00 | 0.01 | 0.99 |
Deterministic sensitivity analysis
The results of the DSAs for oncotype DX are presented in Table 40 (shaded cells reflect analyses that are unchanged from the EAG’s base case). The DSAs indicate the following results across the three subgroups:
-
LN0 NPI ≤ 3.4 – the ICER for oncotype DX versus current practice remains in excess of £34,000 per QALY gained across all scenarios. The only analysis in which the ICER is < £70,000 per QALY gained relates to the scenario in which oncotype DX is assumed to be predictive of chemotherapy benefit, based on RRs reported by Paik et al. 50
-
LN0 NPI > 3.4 – oncotype DX is either dominated or has an ICER in excess of £35,000 per QALY gained across almost all scenarios. The only exception is the scenario in which oncotype DX is assumed to be predictive of chemotherapy benefit, based on RRs reported by Paik et al. 50 Within this analysis, oncotype DX dominates current practice.
-
LN1–3 – oncotype DX remains dominated across the majority of scenarios tested. The exceptions are (1) the scenario in which oncotype DX is assumed to be predictive of chemotherapy benefit, based on treatment effect estimates reported by Albain et al. ,53 and (2) the scenario in which the cost of adjuvant chemotherapy is doubled.
Scenario | Subgroup | ||||||||
---|---|---|---|---|---|---|---|---|---|
LN0 NPI ≤ 3.4 | LN0 NPI > 3.4 | LN1–3 | |||||||
Incremental QALYs | Incremental costs | ICER | Incremental QALYs | Incremental costs | ICER | Incremental QALYs | Incremental costs | ICER | |
Base case (deterministic) | 0.01 | £1317 | £120,144 | –0.02 | £869 | Dominated | –0.07 | £647 | Dominated |
LN0 NPI ≤ 3.4 post-test P(chemotherapy) (NHSE183) | 0.01 | £1458 | £117,326 | –0.02 | £869 | Dominated | –0.07 | £647 | Dominated |
LN0 NPI ≤ 3.4 post-test P(chemotherapy) (Holt et al.141) | 0.01 | £1849 | £173,680 | –0.02 | £869 | Dominated | –0.07 | £647 | Dominated |
LN0 NPI ≤ 3.4 post-test P(chemotherapy) (Loncaster et al.145) | 0.01 | £1640 | £129,527 | –0.02 | £869 | Dominated | –0.07 | £647 | Dominated |
LN0 NPI > 3.4 post-test P(chemotherapy) (Holt et al.141) | 0.01 | £1317 | £120,144 | 0.02 | £1138 | £60,831 | –0.07 | £647 | Dominated |
LN0 NPI > 3.4 post-test P(chemotherapy) (Loncaster et al.145) | 0.01 | £1317 | £120,144 | 0.00 | £999 | £651,857 | –0.07 | £647 | Dominated |
LN0 NPI > 3.4 post-test P(chemotherapy) (UKBCG survey) | 0.01 | £1317 | £120,144 | 0.00 | £978 | Dominated | –0.07 | £647 | Dominated |
LN0 NPI > 3.4 baseline P(chemotherapy) (NCRAS184), post-test P(chemotherapy) (Holt et al.141) | 0.01 | £1317 | £120,144 | 0.03 | £1207 | £44,817 | –0.07 | £647 | Dominated |
LN0 NPI > 3.4 baseline P(chemotherapy) (NCRAS184), post-test P(chemotherapy) (Loncaster et al.145 LN0 subgroup) | 0.01 | £1317 | £120,144 | 0.01 | £1069 | £109,429 | –0.07 | £647 | Dominated |
LN0 NPI > 3.4 baseline P(chemotherapy) (NCRAS184), post-test P(chemotherapy) (UKBCG survey) | 0.01 | £1317 | £120,144 | 0.01 | £1048 | £161,535 | –0.07 | £647 | Dominated |
LN+ post-test P(chemotherapy) (UKBCG survey) | 0.01 | £1317 | £120,144 | –0.02 | £869 | Dominated | 0.00 | £1155 | Dominated |
Baseline P(chemotherapy) adjusted by oncotype DX RS | 0.01 | £1317 | £120,144 | –0.02 | £888 | Dominated | –0.07 | £647 | Dominated |
Ward et al.1 scenario: baseline P(chemotherapy) WMCIU1, post-test P(chemotherapy) (Holt et al.141) | 0.01 | £1317 | £120,144 | 0.04 | £1268 | £35,782 | –0.07 | £647 | Dominated |
Oncotype DX predictive benefit | 0.04 | £1211 | £34,245 | 0.27 | –£364 | Dominating | 0.09 | –£68 | Dominating |
Oncotype DX RSPC LN0 | 0.02 | £1146 | £70,435 | –0.02 | £847 | Dominated | –0.07 | £647 | Dominated |
Chemotherapy disutility doubled | 0.01 | £1317 | £121,879 | –0.01 | £869 | Dominated | –0.06 | £647 | Dominated |
Chemotherapy disutility halved | 0.01 | £1317 | £119,294 | –0.02 | £869 | Dominated | –0.08 | £647 | Dominated |
Start age based on TransATAC38,46 (64 years) | 0.01 | £1319 | £156,971 | –0.01 | £867 | Dominated | –0.05 | £638 | Dominated |
Farkkila et al.204 utilities (RFS = 0.818, DM = 0.746) | 0.01 | £1317 | £125,021 | –0.01 | £869 | Dominated | –0.07 | £647 | Dominated |
Chemotherapy RR = 0.70 | 0.01 | £1305 | £94,920 | –0.02 | £905 | Dominated | –0.10 | £759 | Dominated |
Chemotherapy RR = 0.80 | 0.01 | £1325 | £145,102 | –0.01 | £845 | Dominated | –0.05 | £573 | Dominated |
No risk tapering | 0.01 | £1292 | £92,613 | –0.03 | £974 | Dominated | –0.10 | £870 | Dominated |
Distant metastases death rate doubled | 0.01 | £1339 | £106,090 | –0.02 | £803 | Dominated | –0.09 | £443 | Dominated |
Distant metastases death rate halved | 0.01 | £1282 | £154,090 | –0.01 | £974 | Dominated | –0.05 | £972 | Dominated |
AML removed | 0.01 | £1318 | £119,771 | –0.03 | £879 | Dominated | –0.09 | £663 | Dominated |
Chemotherapy cost doubled | 0.01 | £1330 | £121,322 | –0.02 | £374 | Dominated | –0.07 | –£266 | £3700 |
Chemotherapy cost halved | 0.01 | £1311 | £119,554 | –0.02 | £1116 | Dominated | –0.07 | £1103 | Dominated |
Endocrine therapy costs doubled | 0.01 | £1317 | £120,149 | –0.02 | £869 | Dominated | –0.07 | £646 | Dominated |
Endocrine therapy costs halved | 0.01 | £1317 | £120,141 | –0.02 | £869 | Dominated | –0.07 | £647 | Dominated |
Local and distant recurrence costs doubled | 0.01 | £1268 | £115,630 | –0.02 | £1017 | Dominated | –0.07 | £1106 | Dominated |
Local and distant recurrence costs halved | 0.01 | £1342 | £122,400 | –0.02 | £795 | Dominated | –0.07 | £417 | Dominated |
IHC4+C versus current practice
Central estimates of cost-effectiveness: probabilistic
Central estimates of cost-effectiveness for IHC4+C versus current practice are presented in Table 41. All estimates are based on the probabilistic version of the EAG model. Within the LN0 NPI ≤ 3.4 subgroup, IHC4+C is expected to produce 0.01 additional QALYs at an additional cost of £22 per woman tested compared with current practice; this corresponds to an ICER of £2654 per QALY gained. Within the LN0 NPI > 3.4 subgroup, IHC4+C is expected to produce 0.01 additional QALYs and cost savings of £90 per woman tested compared with current practice; within this subgroup, IHC4+C is expected to dominate current practice. Within the LN1–3 subgroup, IHC4+C is expected to produce 0.05 additional QALYs and cost savings of £287 per woman tested compared with current practice; within this subgroup, IHC4+C is expected to dominate current practice. As shown in Table 42, the PSA indicates that within the LN0 NPI ≤ 3.4 subgroup, the probability that IHC4+C produces more net benefit than current practice at WTP thresholds of £20,000 and £30,000 per QALY gained is 0.95 and 0.97, respectively. Within the LN0 NPI > 3.4 subgroup, the probability that IHC4+C produces more net benefit than current practice at WTP thresholds of £20,000 and £30,000 per QALY gained is 0.69 and 0.67, respectively. Within the LN1–3 subgroup, the probability that IHC4+C produces more net benefit than current practice at these WTP thresholds is ≥ 0.94.
Option | QALYs | Costs | Incremental QALYs | Incremental costs | ICER (per QALY gained) |
---|---|---|---|---|---|
LN0 NPI ≤ 3.4 | |||||
IHC4+C | 13.86 | £4291 | 0.01 | £22 | £2654 |
No test | 13.86 | £4269 | – | – | – |
LN0 NPI > 3.4 | |||||
IHC4+C | 12.73 | £10,941 | 0.01 | –£90 | Dominating |
No test | 12.72 | £11,031 | – | – | – |
LN1–3 | |||||
IHC4+C | 12.59 | £12,268 | 0.05 | –£287 | Dominating |
No test | 12.54 | £12,554 | – | – | – |
Subgroup | Probability (λ = £20,000 per QALY gained) | Probability (λ = £30,000 per QALY gained) | ||
---|---|---|---|---|
IHC4+C | Current practice | IHC4+C | Current practice | |
LN0 NPI ≤ 3.4 | 0.95 | 0.05 | 0.97 | 0.03 |
LN0 NPI > 3.4 | 0.69 | 0.31 | 0.67 | 0.33 |
LN1–3 | 0.95 | 0.05 | 0.94 | 0.06 |
Deterministic sensitivity analysis
The results of the DSAs for IHC4+C are presented in Table 43 (shaded cells reflect analyses that are unchanged from the EAG’s base case). The DSAs indicate the following:
-
LN0 NPI ≤ 3.4 – the ICER for IHC4+C versus current practice remains below £16,000 per QALY gained across all scenarios, except in the analysis in which post-test chemotherapy probabilities are derived from Holt et al. 201 IHC4+C dominates current practice in the scenario in which the cost of adjuvant chemotherapy is doubled.
-
LN0 NPI > 3.4 – IHC4+C dominates current practice or has an ICER of < £6000 per QALY gained across all scenarios.
-
LN1–3 – IHC4+C dominates current practice across all scenarios except the analysis in which the probability of receiving chemotherapy conditional on IHC4+C risk level is based on the UKBCG survey; within this analysis, the ICER is estimated to be £1929 per QALY gained.
Scenario | Subgroup | ||||||||
---|---|---|---|---|---|---|---|---|---|
LN0 NPI ≤ 3.4 | LN0 NPI > 3.4 | LN1–3 | |||||||
Incremental QALYs | Incremental costs | ICER | Incremental QALYs | Incremental costs | ICER | Incremental QALYs | Incremental costs | ICER | |
Base case (deterministic) | 0.01 | £22.43 | £2752 | 0.01 | –£89.12 | Dominating | 0.05 | –£269.39 | Dominating |
LN0 NPI ≤ 3.4 post-test P(chemotherapy) (NHSE183) | 0.01 | £94.18 | £9265 | 0.01 | –£89.12 | Dominating | 0.05 | –£269.39 | Dominating |
LN0 NPI ≤ 3.4 post-test P(chemotherapy) (Holt et al.141) | 0.01 | £390.39 | £36,259 | 0.01 | –£89.12 | Dominating | 0.05 | –£269.39 | Dominating |
LN0 NPI ≤ 3.4 post-test P(chemotherapy) (Loncaster et al.145) | 0.01 | £195.20 | £15,875 | 0.01 | –£89.12 | Dominating | 0.05 | –£269.39 | Dominating |
LN0 NPI > 3.4 post-test P(chemotherapy) (Holt et al.141) | 0.01 | £22.43 | £2752 | 0.05 | £194.16 | £4147 | 0.05 | –£269.39 | Dominating |
LN0 NPI > 3.4 post-test P(chemotherapy) (Loncaster et al.145) | 0.01 | £22.43 | £2752 | 0.03 | £52.99 | £1864 | 0.05 | –£269.39 | Dominating |
LN0 NPI > 3.4 post-test P(chemotherapy) (UKBCG survey) | 0.01 | £22.43 | £2752 | 0.02 | £23.00 | £1040 | 0.05 | –£269.39 | Dominating |
LN0 NPI > 3.4 baseline P(chemotherapy) (NCRAS184), post-test P(chemotherapy) (Holt et al.141) | 0.01 | £22.43 | £2752 | 0.06 | £262.95 | £4760 | 0.05 | –£269.39 | Dominating |
LN0 NPI > 3.4 baseline P(chemotherapy) (NCRAS184), post-test P(chemotherapy) (Loncaster et al.145 LN0 subgroup) | 0.01 | £22.43 | £2752 | 0.04 | £121.78 | £3305 | 0.05 | –£269.39 | Dominating |
LN0 NPI > 3.4 baseline P(chemotherapy) (NCRAS184), post-test P(chemotherapy) (UKBCG survey) | 0.01 | £22.43 | £2752 | 0.03 | £91.80 | £3005 | 0.05 | –£269.39 | Dominating |
LN+ post-test P(chemotherapy) (UKBCG survey) | 0.01 | £22.43 | £2752 | 0.01 | –£89.12 | Dominating | 0.09 | £167.12 | £1929 |
Ward et al.1 scenario: baseline P(chemotherapy) (WMCIU), post-test P(chemotherapy) (Holt et al.141) | 0.01 | £22.43 | £2752 | 0.06 | £325.33 | £5160 | 0.05 | –£269.39 | Dominating |
Chemotherapy disutility doubled | 0.01 | £22.43 | £2304 | 0.01 | –£89.12 | Dominating | 0.05 | –£269.39 | Dominating |
Chemotherapy disutility halved | 0.01 | £22.43 | £3049 | 0.01 | –£89.12 | Dominating | 0.05 | –£269.39 | Dominating |
Start age based on TransATAC38,46 (64 years) | 0.01 | £23.21 | £3542 | 0.01 | –£88.85 | Dominating | 0.04 | –£264.75 | Dominating |
Farkkila et al.204 utilities (RFS = 0.818, DM = 0.746) | 0.01 | £22.43 | £2802 | 0.01 | –£89.12 | Dominating | 0.05 | –£269.39 | Dominating |
Chemotherapy RR = 0.70 | 0.01 | £19.09 | £2138 | 0.01 | –£86.90 | Dominating | 0.06 | –£314.00 | Dominating |
Chemotherapy RR = 0.80 | 0.01 | £24.62 | £3223 | 0.01 | –£90.60 | Dominating | 0.05 | –£240.14 | Dominating |
No risk tapering | 0.01 | £19.17 | £2221 | 0.00 | –£51.24 | Dominating | 0.06 | –£282.61 | Dominating |
Distant metastases death rate doubled | 0.01 | £28.48 | £3309 | 0.01 | –£93.23 | Dominating | 0.06 | –£188.59 | Dominating |
Distant metastases death rate halved | 0.01 | £12.78 | £1722 | 0.01 | –£82.69 | Dominating | 0.04 | –£398.33 | Dominating |
AML removed | 0.00 | £26.13 | £5560 | 0.00 | –£83.05 | Dominating | 0.04 | –£260.08 | Dominating |
Chemotherapy cost doubled | 0.01 | –£108.78 | Dominating | 0.01 | –£326.21 | Dominating | 0.05 | –£499.21 | Dominating |
Chemotherapy cost halved | 0.01 | £88.03 | £10,803 | 0.01 | £29.42 | £4056 | 0.05 | –£154.48 | Dominating |
Endocrine therapy costs doubled | 0.01 | £22.45 | £2755 | 0.01 | –£89.10 | Dominating | 0.05 | –£269.11 | Dominating |
Endocrine therapy costs halved | 0.01 | £22.41 | £2751 | 0.01 | –£89.14 | Dominating | 0.05 | –£269.53 | Dominating |
Local and distant recurrence costs doubled | 0.01 | £8.80 | £1079 | 0.01 | –£79.87 | Dominating | 0.05 | –£451.35 | Dominating |
Local and distant recurrence costs halved | 0.01 | £29.24 | £3588 | 0.01 | –£93.75 | Dominating | 0.05 | –£178.41 | Dominating |
Prosigna versus current practice
Central estimates of cost-effectiveness: probabilistic
Central estimates of cost-effectiveness for Prosigna versus current practice are presented in Table 44. All estimates are based on the probabilistic version of the EAG model. Within the LN0 NPI ≤ 3.4 subgroup, Prosigna is expected to produce 0.02 additional QALYs at an additional cost of £1884 per woman tested compared with current practice; this corresponds to an ICER of £91,028 per QALY gained. Within the LN0 NPI > 3.4 subgroup, Prosigna is expected to produce 0.06 additional QALYs at an additional cost of £1686 per woman tested compared with current practice; the corresponding ICER is £26,058 per QALY gained. Within the LN1–3 subgroup, Prosigna is expected to produce 0.07 additional QALYs at an additional cost of £1936 per woman tested compared with current practice; the corresponding ICER is £28,731 per QALY gained. As shown in Table 45, the PSA indicates that within the LN0 NPI ≤ 3.4 subgroup, the probability that Prosigna produces more net benefit than current practice at WTP thresholds of £20,000 and £30,000 per QALY gained is approximately zero. Within the LN0 NPI > 3.4 subgroup, the probabilities that Prosigna produces more net benefit than current practice at these WTP thresholds are 0.24 and 0.60, respectively. Within the LN1–3 subgroup, the probabilities that Prosigna produces more net benefit than current practice at these WTP thresholds are 0.24 and 0.55, respectively.
Option | QALYs | Costs | Incremental QALYs | Incremental costs | ICER (per QALY gained) |
---|---|---|---|---|---|
LN0 NPI ≤ 3.4 | |||||
Prosigna | 13.87 | £6201 | 0.02 | £1884 | £91,028 |
No test | 13.84 | £4318 | – | – | – |
LN0 NPI > 3.4 | |||||
Prosigna | 12.65 | £13,330 | 0.06 | £1686 | £26,058 |
No test | 12.59 | £11,644 | – | – | – |
LN1–3 | |||||
Prosigna | 12.47 | £15,172 | 0.07 | £1936 | £28,731 |
No test | 12.40 | £13,236 | – | – | – |
Subgroup | Probability (λ = £20,000 per QALY gained) | Probability (λ = £30,000 per QALY gained) | ||
---|---|---|---|---|
Prosigna | Current practice | Prosigna | Current practice | |
LN0 NPI ≤ 3.4 | 0.00 | 1.00 | 0.00 | 1.00 |
LN0 NPI > 3.4 | 0.24 | 0.76 | 0.60 | 0.40 |
LN1–3 | 0.24 | 0.76 | 0.55 | 0.45 |
Deterministic sensitivity analysis
The results of the DSAs for Prosigna are presented in Table 46 (shaded cells reflect analyses that are unchanged from the EAG’s base case). The DSAs indicate the following:
-
LN0 NPI ≤ 3.4 – the ICER for Prosigna versus current practice is estimated to be > £71,000 per QALY gained across all scenarios.
-
LN0 NPI > 3.4 – the ICER for Prosigna versus current practice is estimated to be < £30,000 per QALY gained across most scenarios. The DSAs indicate that the ICER for Prosigna versus current practice is > £30,000 per QALY gained for scenarios in which (1) an older start age is assumed and (2) the RR of distant metastases for chemotherapy versus no chemotherapy is set equal to 0.80.
-
LN1–3 – the ICER for Prosigna versus current practice is estimated to be consistently below £38,000 per QALY gained across all analyses. Less favourable ICERs were estimated for scenarios in which (1) the disutility associated with chemotherapy-related AEs is doubled, (2) an older cohort age is assumed, (3) the RR of distant metastases for chemotherapy versus no chemotherapy is set equal to 0.80, (4) the cost of chemotherapy is doubled, (5) the costs of treating local and distant recurrence are halved, (6) the mortality rate for distant metastases is halved and (7) the cost per test is assumed to be increased owing to lower efficiency. The analysis in which risk classification probabilities and associated DMFS probabilities were taken from Gnant et al. 104 was not evaluable as no events occurred at 10 years within the low-risk Prosigna category.
Scenario | Subgroup | ||||||||
---|---|---|---|---|---|---|---|---|---|
LN0 NPI ≤ 3.4 | LN0 NPI > 3.4 | LN1–3 | |||||||
Incremental QALYs | Incremental costs | ICER | Incremental QALYs | Incremental costs | ICER | Incremental QALYs | Incremental costs | ICER | |
Base case (deterministic) | 0.02 | £1891.35 | £89,693 | 0.07 | £1712.67 | £25,857 | 0.07 | £1966.54 | £28,666 |
LN0 NPI ≤ 3.4 post-test P(chemotherapy) (NHSE183) | 0.02 | £2025.87 | £84,090 | 0.07 | £1712.67 | £25,857 | 0.07 | £1966.54 | £28,666 |
LN0 NPI ≤ 3.4 post-test P(chemotherapy) (Holt et al.141) | 0.02 | £2421.22 | £109,620 | 0.07 | £1712.67 | £25,857 | 0.07 | £1966.54 | £28,666 |
LN0 NPI ≤ 3.4 post-test P(chemotherapy) (Loncaster et al.145) | 0.02 | £2213.71 | £93,938 | 0.07 | £1712.67 | £25,857 | 0.07 | £1966.54 | £28,666 |
LN0 NPI > 3.4 post-test P(chemotherapy) (Holt et al.141) | 0.02 | £1891.35 | £89,693 | 0.10 | £1991.89 | £19,356 | 0.07 | £1966.54 | £28,666 |
LN0 NPI > 3.4 post-test P(chemotherapy) (Loncaster et al.145) | 0.02 | £1891.35 | £89,693 | 0.09 | £1993.10 | £21,216 | 0.07 | £1966.54 | £28,666 |
LN0 NPI > 3.4 post-test P(chemotherapy) (UKBCG survey) | 0.02 | £1891.35 | £89,693 | 0.08 | £1820.85 | £22,420 | 0.07 | £1966.54 | £28,666 |
LN0 NPI > 3.4 baseline P(chemotherapy) (NCRAS184), post-test P(chemotherapy) (Holt et al.141) | 0.02 | £1891.35 | £89,693 | 0.11 | £2056.25 | £18,288 | 0.07 | £1966.54 | £28,666 |
LN0 NPI > 3.4 baseline P(chemotherapy) (NCRAS184), post-test P(chemotherapy) (Loncaster et al.145 LN0 subgroup) | 0.02 | £1891.35 | £89,693 | 0.09 | £1922.48 | £20,971 | 0.07 | £1966.54 | £28,666 |
LN0 NPI > 3.4 baseline P(chemotherapy) NCRAS184, post-test P(chemotherapy) (UKBCG survey) | 0.02 | £1891.35 | £89,693 | 0.09 | £1885.20 | £20,774 | 0.07 | £1966.54 | £28,666 |
LN+ post-test P(chemotherapy) (UKBCG survey) | 0.02 | £1891.35 | £89,693 | 0.07 | £1712.67 | £25,857 | 0.11 | £2227.53 | £20,427 |
Ward et al.1 scenario: baseline P(chemotherapy) (WMCIU), post-test P(chemotherapy) (Holt et al.141) | 0.02 | £1891.35 | £89,693 | 0.13 | £2109.68 | £16,568 | 0.07 | £1966.54 | £28,666 |
Risk classification and DMFS probabilities from Gnant et al.104 LN+ subgroup | 0.02 | £1891.35 | £89,693 | 0.07 | £1712.67 | £25,857 | Not evaluable | ||
10% lower cost per test owing to increased efficiency (local NHS testing) | 0.02 | £1694.00 | £80,348 | 0.07 | £1516 | £22,882 | 0.07 | £1769 | £25,794 |
10% higher cost per test owing to decreased efficiency (local NHS testing) | 0.02 | £2088 | £99,038 | 0.07 | £1910 | £28,832 | 0.07 | £2164 | £31,539 |
Chemotherapy disutility doubled | 0.02 | £1891.35 | £90,123 | 0.07 | £1712.67 | £25,935 | 0.07 | £1966.54 | £30,026 |
Chemotherapy disutility halved | 0.02 | £1891.35 | £89,480 | 0.07 | £1712.67 | £25,818 | 0.07 | £1966.54 | £28,032 |
Start age based on TransATAC38,46 (64 years) | 0.02 | £1893.35 | £115,741 | 0.05 | £1718.65 | £33,348 | 0.05 | £1973.37 | £37,480 |
Farkkila et al.204 utilities (RFS = 0.818, DM = 0.746) | 0.02 | £1891.35 | £93,183 | 0.06 | £1712.67 | £26,854 | 0.07 | £1966.54 | £29,913 |
Chemotherapy RR = 0.70 | 0.03 | £1869.14 | £71,107 | 0.08 | £1643.59 | £19,926 | 0.09 | £1884.89 | £21,508 |
Chemotherapy RR = 0.80 | 0.02 | £1905.92 | £107,875 | 0.06 | £1757.96 | £31,645 | 0.06 | £2020.11 | £36,018 |
No risk tapering | 0.02 | £1870.73 | £78,043 | 0.07 | £1681.49 | £23,298 | 0.08 | £1874.66 | £23,138 |
Distant metastases death rate doubled | 0.02 | £1931.61 | £80,059 | 0.08 | £1837.80 | £24,281 | 0.08 | £2114.58 | £26,505 |
Distant metastases death rate halved | 0.02 | £1827.15 | £112,523 | 0.05 | £1513.02 | £29,575 | 0.05 | £1730.53 | £34,081 |
AML removed | 0.02 | £1892.76 | £91,182 | 0.06 | £1717.28 | £26,432 | 0.07 | £1965.75 | £26,851 |
Chemotherapy cost doubled | 0.02 | £1899.68 | £90,088 | 0.07 | £1729.27 | £26,107 | 0.07 | £2223.61 | £32,414 |
Chemotherapy cost halved | 0.02 | £1887.19 | £89,496 | 0.07 | £1704.37 | £25,731 | 0.07 | £1838.01 | £26,793 |
Endocrine therapy costs doubled | 0.02 | £1891.48 | £89,699 | 0.07 | £1713.06 | £25,863 | 0.07 | £1966.96 | £28,673 |
Endocrine therapy costs halved | 0.02 | £1891.29 | £89,690 | 0.07 | £1712.47 | £25,854 | 0.07 | £1966.33 | £28,663 |
Local and distant recurrence costs doubled | 0.02 | £1800.69 | £85,393 | 0.07 | £1430.87 | £21,602 | 0.07 | £1633.14 | £23,806 |
Local and distant recurrence costs halved | 0.02 | £1936.69 | £91,843 | 0.07 | £1853.57 | £27,984 | 0.07 | £2133.24 | £31,096 |
EndoPredict Clinical versus current practice
Central estimates of cost-effectiveness: probabilistic
Central estimates of cost-effectiveness for EPClin versus current practice are presented in Table 47. All estimates are based on the probabilistic version of the EAG model. Within the LN0 NPI ≤ 3.4 subgroup, EPClin is expected to produce 0.01 additional QALYs at an additional cost of £1679 per woman tested compared with current practice; this corresponds to an ICER of £147,419 per QALY gained. Within the LN0 NPI > 3.4 subgroup, EPClin is expected to produce 0.03 additional QALYs at an additional cost of £1388 per woman tested compared with current practice; the corresponding ICER is £46,788 per QALY gained. Within the LN1–3 subgroup, EPClin is expected to produce 0.05 additional QALYs at an additional cost of £1164 per woman tested compared with current practice; the corresponding ICER is £21,458 per QALY gained. As shown in Table 48, the PSA indicates that within the LN0 NPI ≤ 3.4 subgroup, the probability that EPClin produces more net benefit than current practice at WTP thresholds of £20,000 and £30,000 per QALY gained is zero. Within the LN0 NPI > 3.4 subgroup, the probabilities that EPClin produces more net benefit than current practice at these WTP thresholds are 0.09 and 0.26, respectively. Within the LN1–3 subgroup, the probabilities that EPClin produces more net benefit than current practice at these WTP thresholds are 0.44 and 0.73, respectively.
Option | QALYs | Costs | Incremental QALYs | Incremental costs | ICER (per QALY gained) |
---|---|---|---|---|---|
LN0 NPI ≤ 3.4 | |||||
EPClin | 13.85 | £6034 | 0.01 | £1679 | £147,419 |
No test | 13.84 | £4355 | – | – | – |
LN0 NPI > 3.4 | |||||
EPClin | 12.71 | £12,612 | 0.03 | £1388 | £46,788 |
No test | 12.68 | £11,224 | – | – | – |
LN1–3 | |||||
EPClin | 12.52 | £14,080 | 0.05 | £1164 | £21,458 |
No test | 12.46 | £12,916 | – | – | – |
Subgroup | Probability (λ = £20,000 per QALY gained) | Probability (λ = £30,000 per QALY gained) | ||
---|---|---|---|---|
EPClin | Current practice | EPClin | Current practice | |
LN0 NPI ≤ 3.4 | 0.00 | 1.00 | 0.00 | 1.00 |
LN0 NPI > 3.4 | 0.09 | 0.91 | 0.26 | 0.74 |
LN0 (one to three nodes) | 0.44 | 0.56 | 0.73 | 0.27 |
Deterministic sensitivity analysis
The results of the DSAs for EPClin are presented in Table 49 (shaded cells reflect analyses that are unchanged from the EAG’s base case). The DSAs indicate the following:
-
LN0 NPI ≤ 3.4 – the ICER for EPClin versus current practice remains in excess of £91,000 per QALY gained across all scenarios.
-
LN0 NPI > 3.4 – the ICER for EPClin versus current practice remains in excess of £30,000 per QALY gained across almost all of the analyses. The exceptions are the scenarios in which (1) the UKBCG survey is used to inform the probability of receiving chemotherapy conditional on the EPClin test result and (2) Cusumano et al. 167 is used to inform the probability of receiving chemotherapy conditional on the EPClin test result.
-
LN1–3 – the ICER for EPClin versus current practice remains < £30,000 per QALY gained across all scenarios.
Scenario | Subgroup | ||||||||
---|---|---|---|---|---|---|---|---|---|
LN0 NPI ≤ 3.4 | LN0 NPI > 3.4 | LN1–3 | |||||||
Incremental QALYs | Incremental costs | ICER | Incremental QALYs | Incremental costs | ICER | Incremental QALYs | Incremental costs | ICER | |
Base case (deterministic) | 0.01 | £1685.68 | £141,848 | 0.03 | £1400.62 | £46,482 | 0.06 | £1184.94 | £21,489 |
Post-test P(chemotherapy) (UKBCG survey) | 0.01 | £1470.85 | £101,514 | 0.06 | £1630.80 | £25,250 | 0.12 | £1632.35 | £13,132 |
Chemotherapy disutility doubled | 0.01 | £1685.68 | £181,242 | 0.03 | £1400.62 | £46,938 | 0.06 | £1184.94 | £21,140 |
Chemotherapy disutility halved | 0.01 | £1685.68 | £127,943 | 0.03 | £1400.62 | £46,257 | 0.05 | £1184.94 | £21,667 |
Risk classification and DMFS (Dubsky et al.119 LN+ subgroup) | 0.01 | £1685.68 | £141,848 | 0.03 | £1400.62 | £46,482 | 0.05 | £1179.22 | £21,450 |
Post-test P(chemotherapy) (Penault-Llorca et al.56 LN0 subgroup) | 0.02 | £1515.12 | £91,800 | 0.04 | £1425.80 | £33,212 | 0.06 | £1184.94 | £21,489 |
Post-test P(chemotherapy) (Cusumano et al.167) | 0.02 | £1673.61 | £109,964 | 0.06 | £1532.67 | £26,689 | 0.14 | £1668.00 | £12,205 |
10% lower cost per test owing to increased efficiency (local NHS testing) | 0.01 | £1536 | £129,225 | 0.03 | £1251 | £41,504 | 0.06 | £1035 | £18,768 |
Start age based on TransATAC38,46 (64 years) | 0.01 | £1687.17 | £194,520 | 0.02 | £1403.45 | £60,061 | 0.04 | £1190.15 | £27,705 |
Farkkila et al.204 utilities (RFS = 0.818, DM = 0.746) | 0.01 | £1685.68 | £150,858 | 0.03 | £1400.62 | £48,314 | 0.05 | £1184.94 | £22,275 |
Chemotherapy RR = 0.70 | 0.02 | £1664.55 | £99,445 | 0.04 | £1368.43 | £36,317 | 0.07 | £1130.67 | £16,663 |
Chemotherapy RR = 0.80 | 0.01 | £1699.55 | £195,508 | 0.03 | £1421.74 | £56,485 | 0.05 | £1220.54 | £26,089 |
No risk tapering | 0.02 | £1638.80 | £94,376 | 0.03 | £1380.99 | £41,242 | 0.06 | £1146.32 | £18,707 |
Distant metastases death rate doubled | 0.01 | £1724.04 | £116,644 | 0.03 | £1458.96 | £42,242 | 0.06 | £1283.29 | £20,510 |
Distant metastases death rate halved | 0.01 | £1624.61 | £223,409 | 0.02 | £1307.57 | £56,592 | 0.04 | £1028.09 | £23,745 |
AML removed | 0.02 | £1681.60 | £99,734 | 0.03 | £1402.34 | £46,797 | 0.05 | £1190.90 | £22,954 |
Chemotherapy cost doubled | 0.01 | £1899.47 | £159,838 | 0.03 | £1424.85 | £47,286 | 0.06 | £1109.61 | £20,122 |
Chemotherapy cost halved | 0.01 | £1578.79 | £132,853 | 0.03 | £1388.51 | £46,080 | 0.06 | £1222.61 | £22,172 |
Endocrine therapy costs doubled | 0.01 | £1685.77 | £141,855 | 0.03 | £1400.80 | £46,488 | 0.06 | £1185.25 | £21,494 |
Endocrine therapy costs halved | 0.01 | £1685.64 | £141,844 | 0.03 | £1400.53 | £46,479 | 0.06 | £1184.79 | £21,486 |
Local and distant recurrence costs doubled | 0.01 | £1599.30 | £134,579 | 0.03 | £1269.24 | £42,122 | 0.06 | £963.46 | £17,472 |
Local and distant recurrence costs halved | 0.01 | £1728.87 | £145,482 | 0.03 | £1466.31 | £48,662 | 0.06 | £1295.69 | £23,497 |
MammaPrint versus current practice (Modified Adjuvant! Online)
Central estimates of cost-effectiveness: probabilistic
Central estimates of cost-effectiveness for MammaPrint versus current practice (mAOL) are presented in Table 50. Estimates are based on the probabilistic version of the EAG model. Within the overall MINDACT population, MammaPrint is expected to produce 0.01 additional QALYs at an additional cost of £1760 per woman tested compared with current practice; this corresponds to an ICER of £131,482 per QALY gained. Within the mAOL high-risk subgroup, MammaPrint is expected to produce 0.04 fewer QALYs at an additional cost of £1413; within this subgroup, MammaPrint is expected to be dominated by current practice. Within the mAOL low-risk subgroup, MammaPrint is expected to generate an additional 0.01 QALYs at an additional cost of £2410; this corresponds to an expected ICER of £414,202 per QALY gained. The PSA indicates that within the overall MINDACT population and both subgroups, the probability that MammaPrint produces more net benefit than current practice at WTP thresholds of £20,000 and £30,000 per QALY gained is approximately zero (Table 51).
Option | QALYs | Costs | Incremental QALYs | Incremental costs | ICER (per QALY gained) |
---|---|---|---|---|---|
MINDACT ITT population | |||||
MammaPrint | 13.51 | £9151 | 0.01 | £1760 | £131,482 |
No test | 13.49 | £7391 | – | – | – |
MINDACT mAOL high-risk subgroup | |||||
MammaPrint | 12.86 | £12,727 | –0.04 | £1413 | Dominated |
No test | 12.90 | £11,313 | – | – | – |
MINDACT mAOL low-risk subgroup | |||||
MammaPrint | 13.70 | £7777 | 0.01 | £2410 | £414,202 |
No test | 13.69 | £5366 | – | – | – |
Subgroup | Probability (λ = £20,000 per QALY gained) | Probability (λ = £30,000 per QALY gained) | ||
---|---|---|---|---|
MammaPrint | Current practice | MammaPrint | Current practice | |
MINDACT overall population | 0.00 | 1.00 | 0.00 | 1.00 |
mAOL high-risk subgroup | 0.00 | 1.00 | 0.00 | 1.00 |
mAOL low-risk subgroup | 0.00 | 1.00 | 0.00 | 1.00 |
Deterministic sensitivity analysis
The results of the DSAs for MammaPrint are presented in Table 52 (shaded cells reflect analyses that are unchanged from the EAG’s base case). The DSAs indicate the following:
-
Within the overall MINDACT population, the ICER for MammaPrint versus current practice is estimated to be > £76,000 per QALY gained across all scenarios.
-
Within the mAOL high-risk subgroup, MammaPrint is dominated by current practice across almost all scenarios. The most favourable ICER relates to the scenario in which the probability of receiving chemotherapy under current practice is halved.
-
Within the mAOL low-risk subgroup, the ICER for MammaPrint versus current practice is > £161,000 per QALY gained across all analyses.
Scenario | Subgroup | ||||||||
---|---|---|---|---|---|---|---|---|---|
MINDACT ITT population | MINDACT mAOL high-risk subgroup | MINDACT mAOL low-risk subgroup | |||||||
Incremental QALYs | Incremental costs | ICER | Incremental QALYs | Incremental costs | ICER | Incremental QALYs | Incremental costs | ICER | |
Base case (deterministic) | 0.01 | £1756.58 | £134,059 | –0.04 | £1380.11 | Dominated | 0.01 | £2415.05 | £399,182 |
Risk classification and DMFS probabilities (van ‘t Veer et al.91) | 0.01 | £1609.52 | £169,183 | –0.04 | £1380.11 | Dominated | 0.01 | £2415.05 | £399,182 |
ER+, HER2–, LN0 subgroup | 0.01 | £1756.58 | £134,059 | –0.04 | £1400.94 | Dominated | 0.01 | £2415.05 | £399,182 |
Post-test P(chemotherapy) (Penault-Llorca et al.56 LN0 subgroup) | 0.02 | £1724.59 | £97,939 | –0.03 | £1386.99 | Dominated | 0.01 | £2291.88 | £257,484 |
Post-test P(chemotherapy) (Cusumano et al.167) | 0.02 | £1874.42 | £91,453 | –0.01 | £1492.18 | Dominated | 0.01 | £2454.55 | £336,904 |
Post-test P(chemotherapy) (UKBCG) | 0.01 | £1610 | £130,970 | –0.01 | £1601 | Dominated | –0.01 | £3421 | Dominated |
MammaPrint low-risk patients receive no chemotherapy; MammaPrint high-risk patients all receive chemotherapy | 0.02 | £1846.54 | £76,201 | 0.00 | £1497.09 | £375,444 | 0.01 | £2350.50 | £242,895 |
Baseline chemotherapy probabilities halved | 0.03 | £2512.88 | £96,782 | 0.07 | £2243.31 | £32,800 | 0.00 | £2704.26 | £903,528 |
Chemotherapy disutility doubled | 0.02 | £1756.58 | £93,877 | –0.03 | £1380.11 | Dominated | 0.00 | £2415.05 | £503,351 |
Chemotherapy disutility halved | 0.01 | £1756.58 | £170,560 | –0.05 | £1380.11 | Dominated | 0.01 | £2415.05 | £361,750 |
Start age based on TransATAC38,46 (64 years) | 0.01 | £1757.57 | £158,110 | –0.03 | £1374.22 | Dominated | 0.00 | £2415.84 | £547,979 |
Farkkila et al.204 utilities (RFS = 0.818, DM = 0.746) | 0.01 | £1756.58 | £133,215 | –0.04 | £1380.11 | Dominated | 0.01 | £2415.05 | £423,893 |
Chemotherapy RR = 0.70 | 0.01 | £1762.13 | £148,424 | –0.06 | £1431.22 | Dominated | 0.01 | £2377.25 | £161,338 |
Chemotherapy RR = 0.80 | 0.01 | £1753.86 | £127,971 | –0.03 | £1296.55 | Dominated | 0.01 | £2403.58 | £276,670 |
No risk tapering | 0.01 | £1784.24 | £173,280 | –0.07 | £1591.64 | Dominated | 0.01 | £2391.23 | £270,639 |
Distant metastases death rate doubled | 0.01 | £1747.73 | £140,551 | –0.06 | £1218.99 | Dominated | 0.01 | £2434.08 | £325,055 |
Distant metastases death rate halved | 0.01 | £1770.62 | £125,010 | –0.03 | £1636.40 | Dominated | 0.00 | £2384.61 | £636,029 |
AML removed | 0.00 | £1768.44 | £1,353,592 | –0.07 | £1401.41 | Dominated | 0.01 | £2413.62 | £291,353 |
Chemotherapy cost doubled | 0.01 | £1292.39 | £98,632 | –0.04 | £351.31 | Dominated | 0.01 | £2518.68 | £416,311 |
Chemotherapy cost halved | 0.01 | £1988.67 | £151,772 | –0.04 | £1894.51 | Dominated | 0.01 | £2363.24 | £390,617 |
Endocrine therapy costs doubled | 0.01 | £1756.59 | £134,060 | –0.04 | £1379.77 | Dominated | 0.01 | £2415.09 | £399,189 |
Endocrine therapy costs halved | 0.01 | £1756.57 | £134,058 | –0.04 | £1380.28 | Dominated | 0.01 | £2415.03 | £399,178 |
Local and distant recurrence costs doubled | 0.01 | £1776.51 | £135,580 | –0.04 | £1743.07 | Dominated | 0.01 | £2372.26 | £392,109 |
Local and distant recurrence costs halved | 0.01 | £1746.61 | £133,298 | –0.04 | £1198.63 | Dominated | 0.01 | £2436.45 | £402,719 |
Comparison between the Genomic Health model, the current External Assessment Group model and the previous External Assessment Group model (lymph node negative, clinical intermediate-risk subgroup)
There are notable differences between the cost-effectiveness estimates for oncotype DX versus current practice generated using the current EAG model and those produced using the Genomic Health model62 (see Appendix 7) and the earlier EAG model reported by Ward et al. :1
-
The current EAG model indicates that within the ER+ LN0 NPI > 3.4 subgroup, oncotype DX is expected to be dominated by current practice. This finding sharply contrasts with the findings of the Genomic Health model and the previous EAG model.
-
The Genomic Health model62 produces a base-case ICER of (confidential information has been removed) per QALY gained, assuming that the test is used for women with ER+, LN0 early-stage breast cancer who are deemed to be at clinical intermediate risk.
-
The previous EAG model (Ward et al. 1) produced a base-case ICER for oncotype DX versus current practice of £22,572 per QALY gained, assuming that the test is given to women with ER+, LN0 early-stage breast cancer with NPI > 3.4 (deemed to be at clinical intermediate risk).
In order to understand the differences between these results, it is important to consider the differences between the key parameters and structural assumptions between the three models (Table 53):
-
The general modelling approach is very similar between the three models, although the Ward et al. 1 model defined test risk classification in accordance with both oncotype DX Breast Recurrence Score and IHC4, rather than oncotype DX Breast Recurrence Score only.
-
Within the original and current EAG models, data on risk reclassification (the proportion of patients with a low, intermediate and high recurrence score) were taken from analyses of the TransATAC trial46 (albeit using different data sets). Conversely, the Genomic Health model derives these proportions from the NHS England Access Scheme Database. 183
-
Data on the risk of distant recurrence in the absence of chemotherapy were taken from the ATAC trial in all three models. 198 The updated EAG model uses newer data from the ATAC trial. 46
-
The proportions of women who are assumed to receive chemotherapy conditional on the oncotype DX risk score were taken from the NHS England Access Scheme Database183 in both the updated EAG model and the Genomic Health model. Ward et al. 1 used unpublished data (Holt et al. 141) to estimate the probability of receiving chemotherapy conditional on oncotype DX Breast Recurrence Score.
-
The proportion of patients receiving chemotherapy in the standard care arm was taken from the NHS England Access Scheme Database183 in both the updated EAG model and the Genomic Health model. Conversely, Ward et al. 1 derived estimates of these proportions from English cancer registry data sets.
-
Within both the current and earlier EAG models, the benefit of chemotherapy was assumed to be constant across all oncotype DX Breast Recurrence Score classifications (non-predictive); the RR of distant recurrence was taken from EBCTCG meta-analyses. The current EAG model uses a different mathematical approach to apply this RR, which ensures that modelled treatment effect at 10 years is maintained within the Markov trace.
-
The Genomic Health model assumes a predictive benefit and uses different treatment effects across the low, intermediate and high recurrence score classifications, based on Paik et al. 50 These differential effects are applied only to the oncotype DX testing group; a constant treatment effect is applied in the current practice group.
-
The current and earlier EAG models both apply a HRQoL decrement associated with short-term chemotherapy-related AEs in the first model cycle. In contrast, the Genomic Health model applies a decrement during every model cycle; the implicit assumption is that patients who receive adjuvant chemotherapy remain less well, relative to those do not receive adjuvant chemotherapy, for the remainder of their lives.
Assumption/evidence source | Current EAG model | Genomic Health model62 | Original EAG model (Ward et al.1) |
---|---|---|---|
Approach | Risk classification based on oncotype DX RS | Risk classification based on oncotype DX RS | Risk classification based on oncotype DX RS and IHC4 |
Data on risk classification | TransATAC46 | NHS England Access Scheme Database183 | TransATAC46 |
Data on ROR | TransATAC46 (updated) | TransATAC46 | TransATAC46 |
Proportion of people receiving chemotherapy in the oncotype DX arm | NHS England Access Scheme Database183 | NHS England Access Scheme Database183 | Holt et al.141 |
Proportion of people receiving chemotherapy in the standard care arm | NHS England Access Scheme Database183 | NHS England Access Scheme Database183 | Registry data |
Benefit of adjuvant chemotherapy | No predictive effect (based on EBCTCG meta-analysis) | Predictive effect only in the oncotype DX group. No predictive effect assumed in people with same risk score in current practice group | No predictive effect (based on EBCTCG meta-analysis) |
HRQoL decrement associated with chemotherapy | Applied to the first cycle only | Applied to all model cycles over patients’ remaining lifetimes | Applied to the first cycle only |
As described in Appendix 7, the EAG identified several errors within the Genomic Health model. Three key errors are corrected here:
-
The application of risk reclassification in the model. Although Genomic Health use data from the NHS England Access Scheme Database for the risk reclassification, this is applied incorrectly in the model. This can be seen by examining the proportion of women receiving chemotherapy predicted by the model. The NHS England Access Scheme data were provided as AiC and cannot be reported here. 62
-
The application of the HRQoL decrement associated with chemotherapy-related AEs. The Genomic Health dossier62 stated that the utilities in their model were the same as those used by Ward et al. (2013)1 (page 159), and Table 6-4 (page 159) of the dossier states that the disutility associated with chemotherapy is –0.038. However, the Genomic Health model applies this decrement for women receiving chemotherapy during every model cycle, including for decades after the adjuvant treatment has been discontinued. This overestimates the health losses associated with chemotherapy and is therefore favourable to oncotype DX, as the test is estimated to reduce the proportion of women receiving chemotherapy.
-
Predictive chemotherapy benefit. The Genomic Health model assumes that women with a low, intermediate and high oncotype DX Breast Recurrence Score experience different benefits of chemotherapy in the modelled oncotype DX group compared with the same patients across these recurrence score classifications in the modelled current practice group. Irrespective of whether oncotype DX is predictive of chemotherapy benefit, the modelling approach adopted by the company is illogical, as the benefits of chemotherapy for women within these recurrence score classifications will be identical irrespective of whether the test is used to classify that level of risk or not (they are exactly the same patients).
In order to understand the differences between the results of the three models, the errors identified above were corrected by the EAG. In addition, the Genomic Health model was modified to assume a prognostic benefit only, thereby making it consistent with the current EAG base-case model. The earlier EAG model (Ward et al. 1) was also modified to include the chemotherapy probabilities (with and without the test) from the NHS England Access Scheme Database. Although there are other differences between the models, these are more difficult to align across the models and/or are expected to have only a negligible impact on results. The results of the current EAG model, the amended Ward et al. 1 model and the corrected Genomic Health model are presented in Table 54.
Model | QALYs | Costs | Incremental QALYs | Incremental costs | ICER | ||
---|---|---|---|---|---|---|---|
Oncotype DX | No test | Oncotype DX | No test | ||||
Assuming no predictive effect | |||||||
Current EAG model (no predictive effect) | 12.68 | 12.70 | £11,249 | £10,380 | –0.02 | £869 | Dominated |
Uncorrected Genomic Health model62 (with predictive effect) | 10.50 | 10.43 | Confidential information has been removed | Confidential information has been removed | 0.07 | Confidential information has been removed | Confidential information has been removed |
Corrected Genomic Health model (no predictive effect) | 10.59 | 10.62 | Confidential information has been removed | Confidential information has been removed | –0.03 | Confidential information has been removed | Confidential information has been removed |
Ward et al.1 model (no predictive effect) | 12.85 | 12.80 | £10,172 | £8897 | 0.06 | £1275 | £22,572 |
Ward et al.1 model, including NHS England Access Scheme Database for proportion of people who receive chemotherapy (no predictive effect) | 12.83 | 12.83 | £9861 | £9253 | –0.00 | £608 | Dominated |
Assuming predictive effect | |||||||
Current EAG model (predictive effect) | 12.87 | 12.60 | £10,457 | £10,822 | 0.27 | –£364 | Dominating |
Uncorrected Genomic Health model62 (predictive effect) | 10.50 | 10.43 | Confidential information has been removed | Confidential information has been removed | 0.07 | Confidential information has been removed | Confidential information has been removed |
Corrected Genomic Health model (predictive effect) | 10.74 | 10.69 | Confidential information has been removed | Confidential information has been removed | 0.05 | Confidential information has been removed | Confidential information has been removed |
Ward et al.1 model (predictive effect) | 13.06 | 12.83 | £9681 | £8816 | 0.23 | £865 | £3720 |
Ward et al.1 model, including NHS England Access Scheme Database for proportion of people who receive chemotherapy (including predictive effect) | 13.02 | 12.91 | £9412 | £9078 | 0.11 | £334 | £2917 |
In the scenario in which all three models use pre- and post-test chemotherapy probabilities from the NHS England Access Scheme Database and no predictive benefit is assumed, all three models produce the same economic conclusion: oncotype DX is dominated by current practice. When a predictive effect is incorporated into these versions of the models, these three models consistently suggest that oncotype DX has an ICER that is < £7000 per QALY gained.
Discussion
The EAG undertook a systematic review of existing economic evaluations of tumour profiling tests to guide treatment decisions in people with early-stage breast cancer (see Appendix 6). Only those studies that were published since the previous appraisal of tumour profiling tests (NICE DG1020) were included in the review. The review suggests a high level of consistency in terms of the general modelling approach and structure: the majority of published models adopted a decision tree–Markov approach based on test risk classification and DMFS outcomes conditional on test risk classification probabilities. None of the published analyses included all relevant tumour profiling tests listed in the final NICE scope.
Two manufacturers provided economic evidence to inform the appraisal (Agendia94 and Genomic Health62) (see Appendix 7). The models developed to inform these two analyses were made available to the EAG for scrutiny. In addition, the chief investigator of the EndoPredict UK decision impact study provided a draft cost-effectiveness paper that compares EPClin with AOL. 182 The model supporting this analysis was not made available to the EAG.
Agenda submitted a model, which was critiqued by the EAG as part of the assessment process, but it cannot be reported here as Agendia withdrew permission to reproduce the model.
Genomic Health provided a model that compares oncotype DX with current practice in patients with LN0 early-stage breast cancer. The EAG notes that the model includes a number of errors. Based on the uncorrected model, the Genomic Health submission presents a base-case ICER for oncotype DX versus current practice of (confidential information has been removed) per QALY gained. Three errors were corrected by the EAG [see Comparison between the Genomic Health model, the current External Assessment Group model and the previous External Assessment Group model (lymph node-negative, clinical intermediate-risk subgroup)]; these relate to (1) the incorrect application of risk classifications, (2) the application of health losses associated with short-term chemotherapy-related AEs during every model cycle and (3) the inconsistent handling of predictive benefits of chemotherapy between the test and no-test groups. The EAG’s corrected version of the model suggests that under the assumption of no predictive benefit of chemotherapy, oncotype DX is dominated by current practice. When the test is assumed to be predictive of chemotherapy benefit, the ICER for oncotype DX versus current practice is estimated to be (confidential information has been removed) per QALY gained. The EAG notes that other errors may remain within the company’s model.
The draft cost-effectiveness paper assessing EPClin versus AOL suggests that the expected ICER for EPClin versus AOL is £26,836 per QALY gained. The EAG has some concerns regarding this analysis, in particular the use of separate evidence sources to estimate test risk classification probabilities and DMFS probabilities conditional on test risk classification.
The EAG developed a de novo health economic model to assess the cost-effectiveness of oncotype DX, MammaPrint, Prosigna, EPClin and IHC4+C, each versus current practice. The health economic analysis was undertaken from the perspective of the NHS and PSS and was largely based on the model developed to inform NICE DG10. 1 The EAG model adopts a hybrid decision tree–Markov structure. The model parameters were informed by a number of sources including a bespoke analysis of the TransATAC trial,46 the MINDACT trial,98 a bespoke analysis of the NCRAS data set,184 a bespoke survey disseminated by the UKBCG, the NHS England Access Scheme Database,183 standard costing sources and other literature. The EAG’s base-case model suggests the following results.
-
Oncotype DX – within the LN0 NPI ≤ 3.4 subgroup, the ICER for oncotype DX versus current practice is expected to be £122,725 per QALY gained (£34,245 per QALY gained assuming a predictive benefit). Within the LN0 NPI > 3.4 and LN1–3 subgroups, oncotype DX is expected to be dominated by current practice (conversely, oncotype DX dominates current practice if a predictive benefit is assumed). The results generated using the EAG model are primarily driven by the modelled reduction in the use of adjuvant chemotherapy using the oncotype DX test.
-
IHC4+C – within the LN0 NPI ≤ 3.4 subgroup, the ICER for IHC4+C versus current practice is expected to be £2654 per QALY gained. Within the LN0 NPI > 3.4 and LN1–3 subgroups, IHC4+C is expected to dominate current practice.
-
Prosigna – within the LN0 NPI ≤ 3.4 subgroup, the ICER for Prosigna versus current practice is expected to be £91,028 per QALY gained. Within the LN0 NPI > 3.4 and LN1–3 subgroups, the ICERs for Prosigna versus current practice are £26,058 and £28,731 per QALY gained, respectively.
-
EPClin – within the LN0 NPI ≤ 3.4 subgroup, the ICER for EPClin versus current practice is expected to be £147,419 per QALY gained. Within the LN0 NPI > 3.4 subgroup, the ICER for EPClin versus current practice is expected to be £46,788 per QALY gained. Within the LN1–3 subgroup, the ICER for EPClin versus current practice is expected to be £21,458 per QALY gained.
-
MammaPrint – within the overall MINDACT population, the ICER for MammaPrint versus current practice is expected to be £131,482 per QALY gained. Within the mAOL high-risk subgroup, MammaPrint is expected to be dominated by current practice. Within the mAOL low-risk subgroup, the ICER for MammaPrint versus current practice is expected to be £414,202 per QALY gained.
The EAG model is subject to the following strengths:
-
The model structure is consistent with the general approach used in a number of previous economic analyses of tumour profiling tests for early-stage breast cancer (see Appendix 6, Tables 69 and 70).
-
For all tests, test risk classification probabilities and DMFS probabilities are derived from the same source – this maintains correlation between these parameters and avoids the potential for spectrum bias to produce spurious results.
-
Within the LN0 intermediate-risk subgroup (NPI > 3.4, analysis of three-level tests), the probability of receiving chemotherapy with and without the test is based on the same source – the NHS England Access Scheme Database. 183 The EAG takes the view that this source is likely to best reflect how the three-level tumour profiling tests would be used in clinical practice in England. However, this evidence source relates only to the clinical intermediate-risk group; the UK-specific evidence surrounding decision impact within the LN0 NPI ≤ 3.4 and LN+ subgroups is considerably weaker.
-
When based on the same test risk classification probabilities, recurrence rates and the same estimates of pre- and post-test chemotherapy use, the EAG model produces similar results to the previous model reported by Ward et al. 1 and the Genomic Health model. 62
-
A large number of scenarios have been considered to explore the impact of alternative evidence choices and assumptions on the cost-effectiveness of the alternative tests.
The EAG model is also subject to a number of limitations and uncertainties:
-
Test risk classification probabilities and DMFS probabilities for oncotype DX, Prosigna, IHC4+C and EPClin are based on a postmenopausal population only (TransATAC). It is expected that the tumour profiling tests may also be used in premenopausal women.
-
The subgroups employed within the analysis are defined in accordance with NPI. In practice, other tools may be used to define risk (e.g. Predict). The EAG explored the possibility of framing the analyses around Predict; however, this was not possible as Predict scores were not available within either the TransATAC data set or the NCRAS data set, nor was an analysis presented by Predict within the publication of the MINDACT trial. 98 It may be possible to calculate Predict scores within these data sets in the future; however this would require access to the individual patient-level data.
-
The analysis of MammaPrint using the MINDACT trial compares the test only against mAOL and may therefore not reflect current practice in England. This issue is particularly relevant to determining the baseline level of chemotherapy use for the current practice group within this population.
-
Within the current practice group of the EAG model, the probability of receiving chemotherapy is assumed to be the same irrespective of test risk score. This is unlikely to be realistic, as those with higher test risk scores may already be more likely to receive adjuvant chemotherapy, and those with lower test risk scores may already be less likely to receive adjuvant chemotherapy. It was possible to explore this assumption for the evaluation of oncotype DX within the sensitivity analyses (and the conclusions were unchanged); however, there were insufficient data available to undertake similar analyses for the other four tests.
-
The TransATAC trial was the derivation study for IHC4+C. This means that there is potential for the overestimation of prognostic performance; this leads to additional uncertainty around the likely cost-effectiveness profile of this test.
-
The MINDACT trial used to inform the analyses of MammaPrint is limited as this study does not provide information regarding predictive benefit. In addition, the follow-up period for this study was limited to a duration of 5 years.
-
Across all analyses, it is clear that the model results are dependent on assumptions about pre- and post-test chemotherapy use. This aspect of the evidence base is subject to considerable uncertainty. In particular, there is only one UK-based decision impact study relating to a two-level tumour profiling test (Bloomfield et al. 159); the characteristics of patients enrolled into this study, and their relevance to the modelled subgroups, are unclear. As shown in the DSAs, the use of alternative European studies162,167 and the UKBCG survey appear to lead to generally more favourable cost-effectiveness estimates for EPClin and MammaPrint. In addition, the use of the Loncaster et al. 145 study to estimate chemotherapy use in the LN+ population may be biased as this study included a preselected population for whom chemotherapy had already been recommended.
-
As NanoString Technologies does not offer a centralised testing service for Prosigna, the cost per test will depend on the efficiency of local testing centres and the number of tests undertaken within each centre. This may affect the cost-effectiveness estimates presented here.
-
The model does not include CHF as a long-term AE associated with adjuvant chemotherapy; this was excluded from the model owing to a lack of evidence on the joint survival impact of CHF and metastatic breast cancer. Although CHF is a more common event than AML, the development of cancer is likely to have more serious consequences and is expected to be associated with a greater impact on health-care resources.
-
There is uncertainty surrounding whether or not oncotype DX is predictive of chemotherapy benefit; based on the current EAG model, the inclusion of this potential test characteristic has a marked impact on the conclusions drawn from the analysis. Although the ongoing TAILORx study may generate additional evidence to inform this, the cut-off points used within this trial differ from those employed within the TransATAC analysis.
-
Overall, there remains uncertainty regarding the cost-effectiveness of all tests. It is noteworthy that the inclusion of additional data collected through the NHS England Access Scheme Database has a significant impact on the conclusions drawn from the oncotype DX analysis within NICE DG1020 (moving from an ICER of £22,572 per QALY gained to a situation in which oncotype DX is dominated in the LN0 NPI > 3.4 subgroup). The EAG considers that additional UK-based data collection relating to pre- and post-test chemotherapy use for EPClin, IHC4+C, Prosigna and MammaPrint may be important in reducing existing uncertainty surrounding the cost-effectiveness of these tests.
Chapter 4 Discussion and conclusions
Statement of principal findings
Clinical effectiveness: principal findings
The review included 153 studies across all five tests and across all outcomes listed in the NICE scope.
Among studies of LN0 patients receiving endocrine monotherapy, percentages categorised as high risk ranged from 9% to 33% across all five tests. In LN+ patients, three tests (Prosigna/ROR-PT, EPClin and IHC4+C) categorised far more (38% to 76%) LN+ patients than LN0 patients as being at high risk among studies of endocrine monotherapy, whereas oncotype DX categorised a similar number as high risk in LN0 and LN+ groups. However, oncotype DX categorised more patients in the LN+ group as being at low risk than other tests (57% for oncotype DX vs. 4–28% for other tests), but with worse 10-year DRFS/DRFI outcomes (82% for oncotype DX vs. 95–100% for other tests).
In terms of prognostic performance, all tests had statistically significant prognostic power in unadjusted analyses in LN0 and LN+ populations. However, RSPC was only validated in LN0 patients, and unadjusted analyses using clinical cut-off points were not reported in the validation sets for IHC4 or IHC4+C. All tests provided additional prognostic information over most commonly used clinicopathological factors and over CTS and NPI in LN0 patients. Results were more varied in LN+ patients.
There was some evidence of differential chemotherapy benefit between risk groups for oncotype DX, as shown by significant interaction tests between risk group and chemotherapy treatment in unadjusted analyses, but interaction tests sometimes became non-significant when clinicopathological factors were adjusted for and key LN0 data came from the derivation cohort (NSABP B-20) for oncotype DX, putting it at high risk of bias. Oncotype DX RSPC (oncotype DX plus age, tumour size and grade) was prognostic but not statistically significantly predictive for chemotherapy benefit, indicating that the incorporation of clinicopathological factors (formally or informally in a clinical setting) in oncotype DX may reduce prediction of chemotherapy benefit; this study also used NSABP B-20 and is at risk of bias as a consequence. Considering the limitations of the available data, the EAG concludes that there remains uncertainty surrounding whether or not oncotype DX is associated with a predictive benefit of chemotherapy (i.e. a difference in relative effect by genomic risk group) and, if so, that there is uncertainty in the likely magnitude of this predictive effect in clinical practice.
Evidence relating to the ability of MammaPrint to predict benefit from chemotherapy was extremely limited. Although the effect of chemotherapy was significant in high-risk groups and not in low-risk groups, interaction tests between risk groups and chemotherapy treatment were not significant, suggesting that there was no statistically significant difference in the effect of chemotherapy between risk groups.
For oncotype DX and MammaPrint, evidence from observational, non-comparative studies assessing the impact of the test used prospectively in clinical practice suggested that recurrence/survival outcomes in low-risk groups were acceptable even with low rates of chemotherapy. There was no similar evidence relating to the other tests.
The MINDACT RCT for MammaPrint98 was the only RCT to have reported in full. It reported that for patients who were high mAOL, low MammaPrint risk, chemotherapy gave a non-significant absolute benefit of 1.5% in 5-year DMFS (p = 0.267). This met the primary objective in that the lower bound of the 95% CI for 5-year DMFS in the no-chemotherapy group was ≥ 92%. This finding was interpreted by the authors as implying that patients who were high clinical risk but low MammaPrint risk could potentially avoid chemotherapy. In patients who were low mAOL risk, high MammaPrint risk, chemotherapy gave an absolute benefit of 0.8%. This could be interpreted to mean that MammaPrint would not be a useful test in mAOL-low-risk patients, as it would not alter treatment decisions.
Decision impact studies from the UK and Europe reported that the percentage of patients with any change in chemotherapy recommendation or decision pre/post test ranged from 27% to 49% across UK studies (included oncotype DX, EndoPredict and IHC4+C) and from 5% to 70% across European studies (included all tests except IHC4). The net change in the percentage of patients with a chemotherapy recommendation or decision pre/post test ranged from an increase of 1% to a decrease of 23% among UK studies and from a decrease of 0% to a decrease of 64% across European studies.
Concordance between tests was not fully reviewed, but one UK study (OPTIMA Prelim30), which compared oncotype DX, MammaPrint, Prosigna and IHC4, concluded that although tests assigned similar proportions of patients to low/intermediate- and high-risk categories, test results for an individual patient could differ markedly depending on which test was used.
Data relating to anxiety and HRQoL were limited as most studies did not include a comparator, instead adopting a pre–post test design. Anxiety generally reduced post test, but it is unclear to what extent this would happen after a definitive treatment decision made in accordance with clinical factors. HRQoL improved in some analyses.
Microarray studies support conclusions from studies using the commercial versions of the assays in suggesting that oncotype DX, MammaPrint and EndoPredict can discriminate between high- and low-risk patients regardless of LN status (there were no relevant microarray studies for Prosigna or IHC4).
Cost-effectiveness: principal findings
The EAG developed a de novo health economic model to assess the cost-effectiveness of oncotype DX, MammaPrint, Prosigna, EPClin and IHC4+C, each versus current practice. The health economic analysis was undertaken from the perspective of the NHS and PSS and was largely based on the model developed to inform NICE DG10. 20 The EAG model adopts a hybrid decision tree–Markov structure. The model parameters were informed by a number of sources including a bespoke analysis of the TransATAC trial, the MINDACT trial, a bespoke analysis of the NCRAS data set, a bespoke survey disseminated by the UKBCG, the NHS England Access Scheme Database, standard costing sources and other literature. The EAG’s base-case model suggests the following results:
-
Oncotype DX – within the LN0 NPI ≤ 3.4 subgroup, the ICER for oncotype DX versus current practice is expected to be £122,725 per QALY gained (£34,245 per QALY gained assuming a predictive chemotherapy benefit). Within the LN0 NPI > 3.4 and LN1–3 subgroups, oncotype DX is expected to be dominated by current practice (conversely, oncotype DX dominates current practice if a predictive chemotherapy benefit is assumed). The results generated using the EAG model are primarily driven by the modelled reduction in the use of adjuvant chemotherapy using the oncotype DX test.
-
IHC4+C – within the LN0 NPI ≤ 3.4 subgroup, the ICER for IHC4+C versus current practice is expected to be £2654 per QALY gained. Within the LN0 NPI > 3.4 and LN1–3 subgroups, IHC4+C is expected to dominate current practice.
-
Prosigna – within the LN0 NPI ≤ 3.4 subgroup, the ICER for Prosigna versus current practice is expected to be £91,028 per QALY gained. Within the LN0 NPI > 3.4 and LN1–3 subgroups, the ICERs for Prosigna versus current practice are £26,058 and £28,731 per QALY gained, respectively.
-
EPClin – within the LN0 NPI ≤ 3.4 subgroup, the ICER for EPClin versus current practice is expected to be £147,419 per QALY gained. Within the LN0 NPI > 3.4 subgroup, the ICER for EPClin versus current practice is expected to be £46,788 per QALY gained. Within the LN1–3 subgroup, the ICER for EPClin versus current practice is expected to be £21,458 per QALY gained.
-
MammaPrint – within the overall MINDACT population, the ICER for MammaPrint versus current practice is expected to be £131,482 per QALY gained. Within the mAOL high-risk subgroup, MammaPrint is expected to be dominated by current practice. Within the mAOL low-risk subgroup, the ICER for MammaPrint versus current practice is expected to be £414,202 per QALY gained.
Strengths and limitations of the assessment
Strengths and limitations in the clinical evidence base
The clinical review benefited from a comprehensive search strategy and a high-quality, prospectively designed systematic review methodology.
The evidence base was large, but included only one RCT of a test being used in clinical practice versus usual clinical practice that had reported in full (MINDACT, for MammaPrint). 98 A number of reanalyses of RCTs, which are generally considered to be a high-quality source of data, were also included in the reviews of prognosis and prediction of chemotherapy benefit. However, nearly all studies excluded patients who did not have enough tissue sample (although this is unavoidable in retrospective analyses), which leaves the evidence base at potential risk of spectrum bias, as patients with smaller tumours (who may be systematically different from those with large tumours) will likely be under-represented.
Although, due to time constraints, we did not record every instance of industry funding for included studies, a large number of key studies were funded by industry, and the risk of bias from this should be borne in mind when interpreting the evidence base.
Many prognostic studies were observational in nature, and these are subject to confounding, whereby exclusion of patients who received chemotherapy is likely to introduce bias as these patients are likely to be systematically different in terms of known (and potentially unknown) prognostic variables. Equally, studies that included patients who received chemotherapy may underestimate prognostic effect and/or have more higher-risk patients included.
There were some key gaps in the literature for IHC4+C and RSPC. Notably, the IHC4+C algorithm has been validated only in one cohort (in an adjusted analysis), and RSPC has also been validated in only one cohort (in an unadjusted analysis, and for chemotherapy benefit). In both cases, the validation study was conducted as part of the derivation study. The IHC4/IHC4+C evidence base was also limited in that most of the data related to the IHC4 score alone, without the clinical score, and most studies used tertiles and quartiles to define low-, intermediate- and high-risk patients, which may not be useful in a clinical setting. In addition, there are known problems with conducting the analyses required for IHC4, and although a number of studies report methodologies that are largely compliant with the original methodology, it is unclear whether or not the absolute IHC4 values obtained would be similar across centres.
Much of the evidence base relates to unadjusted analyses, which do not assess the crucial question of whether or not a test has additional value over clinicopathological factors. When adjusted analyses were conducted, the clinicopathological variables included were not always consistent, and it is unclear if all important factors (including stratification factors from the original RCT studies, when applicable) were included in all analyses.
There were limited data relating to the ability of oncotype DX and MammaPrint to predict benefit from chemotherapy, and some of the analyses conducted were also subject to criticisms relating to adjustment for all relevant variables. It may be difficult to conduct further such studies as there are few studies in which patients were randomised to chemotherapy versus no chemotherapy, and tissue samples may not be available.
Data relating to the ability of the test to affect patient outcomes (such as recurrence and survival) through the prospective use of the test to guide treatment decisions were also limited. Most studies were observational in nature, and the selection of patients on the basis of them having received a test may have introduced spectrum bias, and, for this reason, these studies may not match the decision problem. They also do not, by their nature, include a comparator arm, and it is difficult to draw any firm conclusions about the effect of the test in real clinical practice. Similarly, such studies that reported data relating to chemotherapy effects in different risk groups are subject to the same limitations in terms of spectrum bias, but are also at risk of bias from confounding whereby patients who received chemotherapy are likely to be systematically different in terms of known (and potentially unknown) prognostic variables (e.g. age) and treatment effect modifiers from those who did not, which may have an impact on observed recurrence rates and estimates of chemotherapy benefit.
Retrospective observational studies (in which patients were treated in accordance with usual practice without the tests) reporting data relating to prognostic performance are also at risk of confounding in that chemotherapy rates per risk group may differ (and thus affect estimates of prognostic performance). Observational studies that excluded patients who received chemotherapy, in order to obtain a group of patients unaffected by treatment, are likely to be subject to spectrum bias, as patients who receive chemotherapy are likely to be systematically different from those who do not, and this may also affect estimates of prognostic performance. These problems were particularly relevant to the MammaPrint evidence base, in which most studies were observational in nature rather than reanalyses of RCTs. MammaPrint was also unusual, in that many of the included studies pooled multiple cohorts, and, for this reason, it was not possible to gauge the degree of double-counting of patients. The overall sample size was also low (total n = 1805) compared with the evidence base for most other tests.
The evidence base relating to the impact of tests on treatment decisions (decision impact studies) was limited in that use of chemotherapy differs across countries and there were no UK studies for two tests (MammaPrint and Prosigna) and only one UK study for another two tests (EndoPredict and IHC4+C).
Strengths and limitations relating to the health economic analysis
The EAG model has a number of strengths; in particular:
-
For all tests, risk classification and DMFS probabilities are derived from the same source (TransATAC or MINDACT).
-
Within the LN0 intermediate-risk subgroup (NPI > 3.4, analysis of three-level tests), the probability of receiving chemotherapy with and without the test is based on the NHS England Access Scheme Database – this is likely to best reflect how the three-level tumour profiling tests would be used in clinical practice in England.
-
The model structure is consistent with that of other published models of tumour profiling tests – when similar data inputs are used, the EAG model produces similar results to the previous EAG model and the Genomic Health model.
-
Extensive DSAs have been conducted to explore the impact of uncertainty on the model results.
However, the model is also subject to several limitations, most of which stem from uncertainties in the evidence base. The main limitations and uncertainties relating to the cost-effectiveness analysis are:
-
With the exception of oncotype DX in the LN0 NPI > 3.4 group (clinical intermediate risk), the evidence surrounding the pre- and post-test chemotherapy probabilities is subject to considerable uncertainty – this has the propensity to influence the conclusions regarding the cost-effectiveness of all tests.
-
There is uncertainty regarding whether or not oncotype DX and MammaPrint are predictive of chemotherapy benefit – the inclusion of such effects are likely to strongly influence economic conclusions drawn from the analysis.
-
The analysis of MammaPrint is based on a different data source than the other four tests.
-
The TransATAC study used to estimate test risk classification and DMFS probabilities was the derivation study for IHC4 – for this reason, there is potential for the overestimation of prognostic performance for this test.
Uncertainties
Because of time and data constraints, it was not possible to conduct a thorough analysis of how the baseline clinicopathological characteristics of patients (e.g. tumour grade, stage and age) affect prognostic performance.
The evidence relating to the impact on patient outcomes when the test is used in clinical practice remains largely unanswered, and is impeded by the long-term follow-up required, the large sample sizes required and ethical problems with withholding chemotherapy from clinically high-risk patients.
Evidence relating to key subgroups defined in the scope were largely lacking. Data relating specifically to micrometastases were rarely reported, there were no data at all in male-only subgroups or cohorts and data relating to people of different ethnicities were difficult to interpret due to differences in treatment practices in different countries. A more detailed consideration of the available evidence base may have allowed some observations to be drawn regarding premenopausal and postmenopausal patients, but time constraints prevented this.
The IHC4 test is known to have implementation issues in terms of conducting the test in other laboratories, especially local laboratories. The precise details are beyond the expertise of the EAG. It is uncertain if these could be overcome. Furthermore, it is somewhat unclear what cut-off values should be used for IHC4 and IHC4+C.
Generalisability
The EAG notes that there may be issues relating to the generalisability of the evidence contained within this report. In particular, issues with spectrum bias (loss of patients with small tumours with insufficient tissue to test) were evident throughout the clinical evidence base. Furthermore, the classification of risk by NPI will not reflect current practice across all centres. In addition, the TransATAC study that was used to inform test risk classification and DMFS probabilities for oncotype DX, Prosigna, IHC4+C and EPClin relates only to a postmenopausal population only; it is expected that the tumour profile testing may also be used in premenopausal women.
Implications for service provision
The per-test costs for Prosigna provided by NanoString Technologies (used in the EAG economic analyses) are based on an efficient level of throughput. This may not hold if centres do not undertake the anticipated number of tests (e.g. in smaller centres, or if multiple tumour profiling tests are available). Furthermore, as NanoString Technologies does not offer a centralised testing service, local testing services will need to be established.
The IHC4 test is not currently commercially available. Standardisation of IHC4 and quality-assurance programmes are required before this test may be used routinely within the NHS.
Suggested research priorities
-
There is uncertainty regarding whether or not oncotype DX and MammaPrint are predictive of chemotherapy benefit. Further studies that adjust for all relevant clinicopathological factors in validation cohorts are required.
-
There is limited evidence demonstrating long-term impacts resulting from the use of the five tumour profiling tests. Future studies assessing the comparative long-term impact of the tests compared with risk prediction tools commonly used in clinical practice would be valuable.
-
There is uncertainty regarding the cost-effectiveness of all five tests included in the NICE scope. It is noteworthy that under the assumption of no predictive chemotherapy benefit, the inclusion of additional data collected through the NHS England Access Scheme Database has a significant impact on the conclusions previously drawn from the oncotype DX analysis within NICE DG1020 (moving from an ICER of £22,572 per QALY gained to a situation in which oncotype DX is dominated in the LN0 NPI > 3.4 subgroup). Additional UK-based data collection relating to pre- and post-test chemotherapy use for EPClin, IHC4+C, Prosigna and MammaPrint may be important in reducing existing uncertainty surrounding the cost-effectiveness of these tests.
Acknowledgements
We would like to thank Professor Matt Stevenson, Dr John Graham and Professor John Bartlett for providing comments on the draft report, and Andrea Shippam and Gill Rooney [School of Health and Related Research (ScHARR)] for providing administrative support and in preparing and formatting the report. We also thank the TransATAC team, the NCRAS and the UKBCG for providing bespoke analyses and data to inform this assessment.
Contributions of authors
Sue Harnan and Katy Cooper undertook the clinical evidence review. Paul Tappenden, Alice Bessey, Rachid Rafia and Sue Ward undertook the health economic analysis. John Stevens provided statistical advice. Ruth Wong undertook the literature search. Robert C Stein and Janet Brown provided clinical advice. All authors were involved in drafting and commenting on the final report.
About the School of Health and Related Research
The School of Health and Related Research is one of the four schools that constitute the Faculty of Medicine at the University of Sheffield. ScHARR brings together a wide range of medical- and health-related disciplines including public health, general practice, mental health, epidemiology, health economics, management sciences, medical statistics, operational research and information science. It includes the Sheffield unit of the Trent Institute for Health Services Research, which is funded by NHS R&D (research and development) to facilitate high-quality health services research and capacity development.
The ScHARR Technology Assessment Group (ScHARR-TAG) synthesises research on the clinical effectiveness and cost-effectiveness of health-care interventions for the National Institute for Health Research Health Technology Assessment programme on behalf of a range of policy-makers, including NICE. ScHARR-TAG is part of a wider collaboration of a number of units from other regions including the Southampton Health Technology Assessment Centre, University of Southampton; Aberdeen Health Technology Assessment Group, University of Aberdeen; Liverpool Reviews & Implementation Group, University of Liverpool; Peninsular Technology Assessment Group, University of Exeter; the NHS CRD, University of York; Warwick Evidence, The University of Warwick; the British Medical Journal Group; and Kleijnen Systematic Reviews.
Data-sharing statement
All data requests should be submitted to the corresponding author for consideration. Please note that exclusive use will be retained until the publication of major outputs. Access to anonymised data may be granted following review.
Disclaimers
This report presents independent research funded by the National Institute for Health Research (NIHR). The views and opinions expressed by authors in this publication are those of the authors and do not necessarily reflect those of the NHS, the NIHR, NETSCC, the HTA programme or the Department of Health and Social Care. If there are verbatim quotations included in this publication the views and opinions expressed by the interviewees are those of the interviewees and do not necessarily reflect those of the authors, those of the NHS, the NIHR, NETSCC, the HTA programme or the Department of Health and Social Care.
References
- Ward S, Scope A, Rafia R, Pandor A, Harnan S, Evans P, et al. Gene expression profiling and expanded immunohistochemistry tests to guide the use of adjuvant chemotherapy in breast cancer management: a systematic review and cost-effectiveness analysis. Health Technol Assess 2013;17. https://doi.org/10.3310/hta17440.
- Statistics by Cancer Type. London: Cancer Research UK; 2017.
- Dumitrescu RG, Cotarla I. Understanding breast cancer risk – where do we stand in 2005?. J Cell Mol Med 2005;9:208-21. https://doi.org/10.1111/j.1582-4934.2005.tb00350.x.
- Collaborative Group on Hormonal Factors in Breast Cancer . Familial breast cancer: collaborative reanalysis of individual data from 52 epidemiological studies including 58,209 women with breast cancer and 101,986 women without the disease. Lancet 2001;358:1389-99. https://doi.org/10.1016/S0140-6736(01)06524-2.
- Ziegler RG, Hoover RN, Pike MC, Hildesheim A, Nomura AM, West DW, et al. Migration patterns and breast cancer risk in Asian–American women. J Natl Cancer Inst 1993;85:1819-27. https://doi.org/10.1093/jnci/85.22.1819.
- Brush J, Boyd K, Chappell F, Crawford F, Dozier M, Fenwick E, et al. The value of FDG positron emission tomography/computerised tomography (PET/CT) in pre-operative staging of colorectal cancer: a systematic review and economic evaluation. Health Technol Assess 2011;15. https://doi.org/10.3310/hta15350.
- Lagerros YT, Hsieh SF, Hsieh CC. Physical activity in adolescence and young adulthood and breast cancer risk: a quantitative review. Eur J Cancer Prev 2004;13:5-12. https://doi.org/10.1097/00008469-200402000-00002.
- Fisher B, Bauer M, Wickerham DL, Redmond CK, Fisher ER, Cruz AB, et al. Relation of number of positive axillary nodes to the prognosis of patients with primary breast cancer. An NSABP update. Cancer 1983;52:1551-7. https://doi.org/10.1002/1097-0142(19831101)52:9<1551::AID-CNCR2820520902>3.0.CO;2-3.
- Early and Locally Advanced Breast Cancer: Diagnosis and Treatment. Clinical Guideline [CG80]. London: National Institute for Health and Care Excellence; 2017.
- Survival Rates in England, Patients Diagnosed 2001–2006 followed up to 2007. Newport: Office for National Statistics; 2007.
- Cancer Research UK . Breast Cancer (C50), Five-Year Net Survival by Age, Women, England, 2009–2013 2017. www.cancerresearchuk.org/health-professional/cancer-statistics/statistics-by-cancer-type/breast-cancer/survival#collapseZero (accessed 11 April 2019).
- Galea MH, Blamey RW, Elston CE, Ellis IO. The Nottingham Prognostic Index in primary breast cancer. Breast Cancer Res Treat 1992;22:207-19. https://doi.org/10.1007/BF01840834.
- Cancer Registration Statistics, England. Newport: Office for National Statistics; 2017.
- National Cancer Intelligence Network. Cancer Incidence and Survival by Major Ethnic Group, England, 2002–2006. London: Cancer Research UK; 2009.
- Cancer by Deprivation in England 1996–2011. London: National Cancer Registration and Analysis Service; 2017.
- Massat NJ, Dibden A, Parmar D, Cuzick J, Sasieni PD, Duffy SW. Impact of screening on breast cancer mortality: the UK program 20 years on. Cancer Epidemiol Biomarkers Prev 2016;25:455-62. https://doi.org/10.1158/1055-9965.EPI-15-0803.
- AJCC Cancer Staging Manual. Springer International Publishing; 2017.
- Improving Outcomes in Breast Cancer. London: National Institute for Health and Care Excellence; 2011.
- Early Breast Cancer Trialists’ Collaborative Group . Effects of chemotherapy and hormonal therapy for early stage breast cancer on recurrence and 15-year survival: an overview of the randomised trials. Lancet 2011;365:1687-717.
- Gene Expression Profiling and Expanded Immunohistochemistry Tests for Guiding Adjuvant Chemotherapy Decisions in Early Stage Breast Cancer Management: MammaPrint, Oncotype DX, IHC4 and Mammostrat. Diagnostics Guidance 10. London: National Institute for Health and Care Excellence; 2013.
- Tumour Profiling Tests to Guide Adjuvant Chemotherapy Decisions in People With Breast Cancer (Update of DG10) – Final Scope. London: National Institute for Health and Care Excellence; 2017.
- Sparano JA, Gray RJ, Makower DF, Pritchard KI, Albain KS, Hayes DF, et al. Prospective validation of a 21-gene expression assay in breast cancer. N Engl J Med 2015;373:2005-14. https://doi.org/10.1056/NEJMoa1510764.
- Parker JS, Mullins M, Cheang MC, Leung S, Voduc D, Vickery T, et al. Supervised risk predictor of breast cancer based on intrinsic subtypes. J Clin Oncol 2009;27:1160-7. https://doi.org/10.1200/JCO.2008.18.1370.
- Bartlett JM, Christiansen J, Gustavson M, Rimm DL, Piper T, van de Velde CJ, et al. Validation of the IHC4 breast cancer prognostic algorithm using multiple approaches on the multinational TEAM clinical trial. Arch Pathol Lab Med 2016;140:66-74. https://doi.org/10.5858/arpa.2014-0599-OA.
- Cuzick J, Dowsett M, Pineda S, Wale C, Salter J, Quinn E, et al. Prognostic value of a combined estrogen receptor, progesterone receptor, Ki-67, and human epidermal growth factor receptor 2 immunohistochemical score and comparison with the Genomic Health recurrence score in early breast cancer. J Clin Oncol 2011;29:4273-8. https://doi.org/10.1200/JCO.2010.31.2835.
- Systematic Review: CRD’s Guidance for Undertaking Reviews in Health Care. York: CRD, University of York; 2009.
- Liberati A, Altman DG, Tetzlaff J, Mulrow C, Gøtzsche PC, Ioannidis JP, et al. The PRISMA statement for reporting systematic reviews and meta-analyses of studies that evaluate healthcare interventions: explanation and elaboration. BMJ 2009;339. https://doi.org/10.1136/bmj.b2700.
- Diagnostics Assessment Programme Manual. London: National Institute for Health and Care Excellence; 2011.
- Waintraub SE, McNamara DM, Graham DA, Pecora A, Min J, Wu T, et al. Early economic benefits of gene expression profiling using a 21-gene panel among patients with early stage, lymph node negative, hormone receptor positive, HER2-neu oncogene negative breast cancer. J Clin Oncol Conf 2016;34.
- Hall P, Smith A, Hulme C, Vargas-Palacios A, Makris A, Hughes-Davies L, et al. Value of information analysis of multiparameter tests for chemotherapy in early breast cancer: the OPTIMA-prelim trial. Value Health 2017;20:1311-18. https://doi.org/10.1016/j.jval.2017.04.021.
- Hudis CA, Barlow WE, Costantino JP, Gray RJ, Pritchard KI, Chapman JA, et al. Proposal for standardized definitions for efficacy end points in adjuvant breast cancer trials: the STEEP system. J Clin Oncol 2007;25:2127-32. https://doi.org/10.1200/JCO.2006.10.3523.
- Higgins JPT, Altman DG, Sterne JAC on behalf of the Cochrane Statistical Methods Group and the Cochrane Bias Methods Group. Assessing Risk of Bias in Included Studies. Cochrane Handbook for Systematic Reviews of Interventions Version 5.1.0 2011. www.handbook-5-1.cochrane.org/ (accessed 11 April 2019).
- Michiels S, Ternès N, Rotolo F. Statistical controversies in clinical research: prognostic gene signatures are not (yet) useful in clinical practice. Ann Oncol 2016;27:2160-7. https://doi.org/10.1093/annonc/mdw307.
- Teutsch SM, Bradley LA, Palomaki GE, Haddow JE, Piper M, Calonge N, et al. The Evaluation of Genomic Applications in Practice and Prevention (EGAPP) initiative: methods of the EGAPP working group. Genet Med 2009;11:3-14. https://doi.org/10.1097/GIM.0b013e318184137c.
- Moons KG, de Groot JA, Bouwmeester W, Vergouwe Y, Mallett S, Altman DG, et al. Critical appraisal and data extraction for systematic reviews of prediction modelling studies: the CHARMS checklist. PLOS Med 2014;11. https://doi.org/10.1371/journal.pmed.1001744.
- Buus R, Sestak I, Kronenwett R, Denkert C, Dubsky P, Krappmann K, et al. Comparison of EndoPredict and EPclin with oncotype DX recurrence score for prediction of risk of distant recurrence after endocrine therapy. J Natl Cancer Inst 2016;108. https://doi.org/10.1093/jnci/djw149.
- Dowsett M, Cuzick J, Wale C, Forbes J, Mallon EA, Salter J, et al. Prediction of risk of distant recurrence using the 21-gene recurrence score in node-negative and node-positive postmenopausal patients with breast cancer treated with anastrozole or tamoxifen: a TransATAC study. J Clin Oncol 2010;28:1829-34. https://doi.org/10.1200/JCO.2009.24.4798.
- Dowsett M, Sestak I, Lopez-Knowles E, Sidhu K, Dunbier AK, Cowens JW, et al. Comparison of PAM50 risk of recurrence score with oncotype DX and IHC4 for predicting risk of distant recurrence after endocrine therapy. J Clin Oncol 2013;31:2783-90. https://doi.org/10.1200/JCO.2012.46.1558.
- Sestak I, Buus R, Cuzick J, Dubsky P, Kronenwett R, Ferree S, et al. Comprehensive Comparison of Prognostic Signatures for Breast Cancer Recurrence in TransATAC n.d.
- Sestak I, Cuzick J, Dowsett M, Lopez-Knowles E, Filipits M, Dubsky P, et al. Prediction of late distant recurrence after 5 years of endocrine treatment: a combined analysis of patients from the Austrian Breast and Colorectal Cancer Study Group 8 and arimidex, tamoxifen alone or in combination randomized trials using the PAM50 risk of recurrence score. J Clin Oncol 2015;33:916-22. https://doi.org/10.1200/JCO.2014.55.6894.
- Sestak I, Dowsett M, Zabaglo L, Lopez-Knowles E, Ferree S, Cowens JW, et al. Factors predicting late recurrence for estrogen receptor-positive breast cancer. J Natl Cancer Inst 2013;105:1504-11. https://doi.org/10.1093/jnci/djt244.
- Sestak I, Zhang Y, Schroeder BE, Schnabel CA, Dowsett M, Cuzick J, et al. Cross-stratification and differential risk by breast cancer index and recurrence score in women with hormone receptor-positive lymph node-negative early-stage breast cancer. Clin Cancer Res 2016;22:5043-8. https://doi.org/10.1158/1078-0432.CCR-16-0155.
- Sgroi DC, Sestak I, Cuzick J, Zhang Y, Schnabel CA, Schroeder B, et al. Prediction of late distant recurrence in patients with oestrogen-receptor-positive breast cancer: a prospective comparison of the breast-cancer index (BCI) assay, 21-gene recurrence score, and IHC4 in the TransATAC study population. Lancet Oncol 2013;14:1067-76. https://doi.org/10.1016/S1470-2045(13)70387-5.
- Tang G, Cuzick J, Costantino JP, Dowsett M, Forbes JF, Crager M, et al. Risk of recurrence and chemotherapy benefit for patients with node-negative, estrogen receptor-positive breast cancer: recurrence score alone and integrated with pathologic and clinical factors. J Clin Oncol 2011;29:4365-72. https://doi.org/10.1200/JCO.2011.35.3714.
- Howell A, Cuzick J, Baum M, Buzdar A, Dowsett M, Forbes JF, et al. Results of the ATAC (Arimidex, Tamoxifen, Alone or in Combination) trial after completion of 5 years’ adjuvant treatment for breast cancer. Lancet 2009;365:60-2. https://doi.org/ 10.1016/S0140-6736(04)17666-6.
- Sestak I, Dowsett M, Cuzick J. NICE Request – TransATAC Data Analysis 2017.
- Sestak I, Buus R, Cuzick J, Dubsky P, Kronenwett R, Denkert C, et al. Comparison of the performance of 6 prognostic signatures for estrogen receptor-positive breast cancer: a secondary analysis of a randomized clinical trial. JAMA Oncol 2018;4:545-53. https://doi.org/10.1001/jamaoncol.2017.5524.
- Statistics How To . Statistics How To 2017. www.statisticshowto.com/c-statistic/ (accessed 17 October 2017).
- Paik S, Shak S, Tang G, Kim C, Baker J, Cronin M, et al. A multigene assay to predict recurrence of tamoxifen-treated, node-negative breast cancer. N Engl J Med 2004;351:2817-26. https://doi.org/10.1056/NEJMoa041588.
- Paik S, Tang G, Shak S, Kim C, Baker J, Kim W, et al. Gene expression and benefit of chemotherapy in women with node-negative, estrogen receptor-positive breast cancer. J Clin Oncol 2006;24:3726-34. https://doi.org/10.1200/JCO.2005.04.7985.
- Mamounas EP, Tang G, Paik S, Baehner FL, Liu Q, Jeong JH, et al. Prognostic impact of the 21-gene recurrence score (RS) on disease-free and overall survival of node-positive, ER-positive breast cancer patients (pts) treated with adjuvant chemotherapy: Results from NSABP B-28. J Clin Oncol Conf 2012;30.
- Wolmark N, Mamounas EP, Baehner FL, Butler SM, Tang G, Jamshidian F, et al. Prognostic impact of the combination of recurrence score and quantitative Estrogen Receptor Expression (ESR1) on predicting late distant recurrence risk in estrogen receptor-positive breast cancer after 5 years of tamoxifen: results from NRG oncology/national surgical adjuvant breast and bowel project B-28 and B-14. J Clin Oncol 2016;34:2350-8. https://doi.org/10.1200/JCO.2015.62.6630.
- Albain KS, Barlow WE, Shak S, Hortobagyi GN, Livingston RB, Yeh IT, et al. Prognostic and predictive value of the 21-gene recurrence score assay in postmenopausal women with node-positive, oestrogen-receptor-positive breast cancer on chemotherapy: a retrospective analysis of a randomised trial. Lancet Oncol 2010;11:55-6. https://doi.org/10.1016/S1470-2045(09)70314-6.
- Goldstein LJ, Gray R, Badve S, Childs BH, Yoshizawa C, Rowley S, et al. Prognostic utility of the 21-gene assay in hormone receptor-positive operable breast cancer compared with classical clinicopathologic features. J Clin Oncol 2008;26:4063-71. https://doi.org/10.1200/JCO.2007.14.4501.
- Sparano JA, O’Neill A, Gray RJ, Perez EA, Shulman LN, Martino S, et al. 10-year update of E2197: phase III doxorubicin/docetaxel (AT) versus doxorubicin/cyclophosphamide (AC) adjuvant treatment of LN+ and high-risk LN-breast cancer and the comparison of the prognostic utility of the 21-gene recurrence score (RS) with clinicopathologic features. J Clin Oncol Conf 2012;30.
- Penault-Llorca FM, Filleron T, Asselain B, Baehner FL, Fumoleau P, Lacroix-Triki M, et al. Prediction of recurrence with the oncotype DX recurrence score in node-positive, HR-positive, breast cancer patients treated with adjuvant chemotherapy: results from PACS01 trial. J Clin Oncol Conf 2014;32.
- Russell S, Shivers SC, Blumencranz L. Long-term follow-up of early stage breast cancer patients with results of MammaPrint, oncotype DX and MammoStrat risk classification assays. Cancer Res 2017;77.
- Gong C, Tan W, Chen K, You N, Zhu S, Liang G, et al. Prognostic value of a BCSC-associated microRNA signature in hormone receptor-positive HER2-negative breast cancer. EBioMedicine 2016;11:199-20. https://doi.org/10.1016/j.ebiom.2016.08.016.
- Sun B, Zhang F, Wu SK, Guo X, Zhang LL, Jiang ZF, et al. Gene expression profiling for breast cancer prognosis in Chinese populations. Breast J 2011;17:172-9. https://doi.org/10.1111/j.1524-4741.2010.01049.x.
- Toi M, Iwata H, Yamanaka T, Masuda N, Ohno S, Nakamura S, et al. Clinical significance of the 21-gene signature (oncotype DX) in hormone receptor-positive early stage primary breast cancer in the Japanese population. Cancer 2010;116:3112-18. https://doi.org/10.1002/cncr.25206.
- Tang G, Shak S, Paik S, Anderson SJ, Costantino JP, Geyer CE, et al. Comparison of the prognostic and predictive utilities of the 21-gene Recurrence Score assay and Adjuvant! for women with node-negative, ER-positive breast cancer: results from NSABP B-14 and NSABP B-20. Breast Cancer Res Treat 2011;127:133-42. https://doi.org/10.1007/s10549-010-1331-z.
- Genomic Health, Inc . Oncotype DX – Information Provided to NICE 2017.
- Goldhirsch A, Wood WC, Gelber RD, Coates AS, Thürlimann B, Senn HJ. Progress and promise: highlights of the international expert consensus on the primary therapy of early breast cancer 2007. Ann Oncol 2007;18:1133-44. https://doi.org/10.1093/annonc/mdm271.
- Barcenas CH, Raghavendra A, Sinha AK, Syed MP, Hsu L, Patangan MG, et al. Outcomes in patients with early-stage breast cancer who underwent a 21-gene expression assay. Cancer 2017;123:2422-31. https://doi.org/10.1002/cncr.30618.
- Le Du F, Gonzalez-Angulo AM, Park M, Liu DD, Hortobagyi GN, Ueno NT. Effect of 21-gene RT-PCR assay on adjuvant therapy and outcomes in patients with stage I breast cancer. Clin Breast Cancer 2015;15:458-66. https://doi.org/10.1016/j.clbc.2015.06.006.
- Stemmer SM, Steiner M, Rizel S, Geffen D, Nisenbaum B, Peretz T, et al. First prospectively-designed outcome study in estrogen receptor (ER)+ breast cancer (BC) patients (pts) with N1mi or 1-3 positive nodes in whom treatment decisions in clinical practice incorporated the 21-gene recurrence score (RS) result. Ann Oncol Conference, 41st European Society for Medical Oncology Congress, ESMO 2016;27.
- Roberts M, Petkov VI, Miller DP, Shak S, Howlader N, Cronin K, et al. Breast cancer specific survival in patients with node-positive hormone receptor positive invasive breast cancer and oncotype DX recurrence score results in the SEER database. J Clin Oncol Conf 2016;34.
- Roberts MC, Miller DP, Shak S, Petkov VI. Breast cancer-specific survival in patients with lymph node-positive hormone receptor-positive invasive breast cancer and oncotype DX recurrence score results in the SEER database. Breast Cancer Res Treat 2017;163:303-10. https://doi.org/10.1007/s10549-017-4162-3.
- Stemmer SM, Steiner M, Rizel S, Soussan-Gutman L, Geffen DB, Nisenbaum B, et al. Real-life analysis evaluating 1594 N0/Nmic breast cancer patients for whom treatment decisions incorporated the 21-gene recurrence score result: 5-year KM estimate for breast cancer specific survival with recurrence score results < 30 is > 98%. Cancer Res 2016;76:P5-08. https://doi.org/10.1158/1538-7445.SABCS15-P5-08-02.
- Petkov VI, Miller DP, Howlader N, Gliner N, Howe W, Schussler N, et al. Breast-cancer-specific mortality in patients treated based on the 21-gene assay: a SEER population-based study. NPJ Breast Cancer 2016;2. https://doi.org/10.1038/npjbcancer.2016.17.
- Harnan S, Tappenden P, Cooper K, Stevens J, Bessey A, Rafia R, et al. Tumour Profiling Tests to Guide Adjuvant Chemotherapy Decisions in People with Breast Cancer (update of DG10). London: National Institute for Health and Care Excellence; 2017.
- TAILORx (Sparano 2018): EAG Summary of Key Points. London: National Institute for Health and Care Excellence; 2018.
- Gluz O, Nitz U, Chrlstgen M, Kates RE, Clemens M, Kraemer S, et al. Prognostic impact of 21 gene recurrence score, IHC4, and central grade in high-risk HR+/HER2– early breast cancer (EBC): 5-year results of the prospective Phase III WSG PlanB trial. J Clin Oncol Conf 2016;34.
- Gluz O, Nitz UA, Christgen M, Kates RE, Shak S, Clemens M, et al. West German Study Group phase III planB trial: first prospective outcome data for the 21-gene recurrence score assay and concordance of prognostic markers by central and local pathology assessment. J Clin Oncol 2016;34:2341-9. https://doi.org/10.1200/JCO.2015.63.5383.
- Wen HY, Krystel-Whittemore M, Patil S, Pareja F, Bowser ZL, Dickler MN, et al. Breast carcinoma with an oncotype Dx recurrence score < 18: rate of distant metastases in a large series with clinical follow-up. Cancer 2017;123:131-7. https://doi.org/10.1002/cncr.30271.
- Sparano JA, Gray RJ, Makower DF, Pritchard KI, Albain KS, Hayes DF, et al. Adjuvant chemotherapy guided by a 21-gene expression assay in breast cancer. N Engl J Med 2018;379:111-21. https://doi.org/10.1056/NEJMoa1804710.
- Nitz U, Gluz O, Christgen M, Kates RE, Clemens M, Malter W, et al. Reducing chemotherapy use in clinically high-risk, genomically low-risk pN0 and pN1 early breast cancer patients: five-year data from the prospective, randomised phase 3 West German Study Group (WSG) PlanB trial. Breast Cancer Res Treat 2017;165:573-83. https://doi.org/10.1007/s10549-017-4358-6.
- Beumer IJ, Persoon M, Witteveen A, Dreezen C, Chin SF, Sammut SJ, et al. Prognostic value of MammaPrint® in invasive lobular breast cancer. Biomark Insights 2016;11:139-46. https://doi.org/10.4137/BMI.S38435.
- van de Vijver MJ, He YD, van ’t Veer LJ, Dai H, Hart AA, Voskuil DW, et al. A gene-expression signature as a predictor of survival in breast cancer. N Engl J Med 2002;347:1999-200. https://doi.org/10.1056/NEJMoa021967.
- Kok M, Koornstra RH, Mook S, Hauptmann M, Fles R, Jansen MP, et al. Additional value of the 70-gene signature and levels of ER and PR for the prediction of outcome in tamoxifen-treated ER-positive breast cancer. Breast 2012;21:769-78. https://doi.org/10.1016/j.breast.2012.04.010.
- Bueno-de-Mesquita JM, van Harten WH, Retèl VP, van ‘t Veer LJ, van Dam FS, Karsenberg K, et al. Use of 70-gene signature to predict prognosis of patients with node-negative breast cancer: a prospective community-based feasibility study (RASTER). Lancet Oncol 2007;8:1079-87. https://doi.org/10.1016/S1470-2045(07)70346-7.
- Michaut M, Chin SF, Majewski I, Severson TM, Bismeijer T, de Koning L, et al. Integration of genomic, transcriptomic and proteomic data identifies two biologically distinct subtypes of invasive lobular breast cancer. Sci Rep 2016;6. https://doi.org/10.1038/srep18517.
- Knauer M, Mook S, Rutgers EJ, Bender RA, Hauptmann M, van de Vijver MJ, et al. The predictive value of the 70-gene signature for adjuvant chemotherapy in early breast cancer. Breast Cancer Res Treat 2010;120:655-61. https://doi.org/10.1007/s10549-010-0814-2.
- Bueno-de-Mesquita JM, Linn SC, Keijzer R, Wesseling J, Nuyten DS, van Krimpen C, et al. Validation of 70-gene prognosis signature in node-negative breast cancer. Breast Cancer Res Treat 2009;117:483-95. https://doi.org/10.1007/s10549-008-0191-2.
- Mook S, Schmidt MK, Viale G, Pruneri G, Eekhout I, Floore A, et al. The 70-gene prognosis-signature predicts disease outcome in breast cancer patients with 1-3 positive lymph nodes in an independent validation study. Breast Cancer Res Treat 2009;116:295-302. https://doi.org/10.1007/s10549-008-0130-2.
- Mook S, Knauer M, Bueno-de-Mesquita JM, Retèl VP, Wesseling J, Linn SC, et al. Metastatic potential of T1 breast cancer can be predicted by the 70-gene MammaPrint signature. Ann Surg Oncol 2010;17:1406-13. https://doi.org/10.1245/s10434-009-0902-x.
- Mook S, Schmidt MK, Weigelt B, Kreike B, Eekhout I, van de Vijver MJ, et al. The 70-gene prognosis signature predicts early metastasis in breast cancer patients between 55 and 70 years of age. Ann Oncol 2010;21:717-22. https://doi.org/10.1093/annonc/mdp388.
- Buyse M, Loi S, van ’t Veer L, Viale G, Delorenzi M, Glas AM, et al. Validation and clinical utility of a 70-gene prognostic signature for women with node-negative breast cancer. J Natl Cancer Inst 2006;98:1183-92. https://doi.org/10.1093/jnci/djj329.
- Drukker CA, van Tinteren H, Schmidt MK, Rutgers EJ, Bernards R, van de Vijver MJ, et al. Long-term impact of the 70-gene signature on breast cancer outcome. Breast Cancer Res Treat 2014;143:587-92. https://doi.org/10.1007/s10549-013-2831-4.
- Yao K, Goldschmidt R, Turk M, Wesseling J, Stork-Sloots L, de Snoo F, et al. Molecular subtyping improves diagnostic stratification of patients with primary breast cancer into prognostically defined risk groups. Breast Cancer Res Treat 2015;154:81-8. https://doi.org/10.1007/s10549-015-3587-9.
- van ‘t Veer LJ, Yau C, Yu NY, Benz CC, Nordenskjold B, Fornander T, et al. Tamoxifen therapy benefit for patients with 70-gene signature high and low risk. Breast Cancer Res Treat 2017;166:593-601. https://doi.org/10.1007/s10549-017-4428-9.
- Esserman LJ, Thompson CK, Yau C, van ’t Veer LJ, Borowsky AD, Tobin NP, et al. Identification of tumors with an indolent disease course: MammaPrint ultralow signature validation in a retrospective analysis of a Swedish randomized tamoxifen trial. Cancer Res 2016;76. https://doi.org/10.1158/1538-7445.SABCS15-P6-09-01.
- Bueno-de-Mesquita JM, Sonke GS, van de Vijver MJ, Linn SC. Additional value and potential use of the 70-gene prognosis signature in node-negative breast cancer in daily clinical practice. Ann Oncol 2011;22:2021-30. https://doi.org/10.1093/annonc/mdp547.
- MammaPrint – Evidence Provided to NICE. Amsterdam: Agendia, Inc.; 2017.
- Ishitobi M, Goranova TE, Komoike Y, Motomura K, Koyama H, Glas AM, et al. Clinical utility of the 70-gene MammaPrint profile in a Japanese population. Jpn J Clin Oncol 2010;40:508-12. https://doi.org/10.1093/jjco/hyp195.
- Wittner BS, Sgroi DC, Ryan PD, Bruinsma TJ, Glas AM, Male A, et al. Analysis of the MammaPrint breast cancer assay in a predominantly postmenopausal cohort. Clin Cancer Res 2008;14:2988-93. https://doi.org/10.1158/1078-0432.CCR-07-4723.
- Lindstrom LS, Benz CC, Yau C, van ’t Veer LJ, Thompson CK, Esserman LJ. MammaPrint accurately predicts long-term survival (25 years) and adjuvant tamoxifen therapy benefit in lymph node negative patients. Cancer Res 2015;75. https://doi.org/10.1158/1538-7445.SABCS14-P4-11-12.
- Cardoso F, van ’t Veer LJ, Bogaerts J, Slaets L, Viale G, Delaloge S, et al. 70-gene signature as an aid to treatment decisions in early-stage breast cancer. N Engl J Med 2016;375:717-29. https://doi.org/10.1056/NEJMoa1602253.
- Drukker CA, Bueno-de-Mesquita JM, Retèl VP, van Harten WH, van Tinteren H, Wesseling J, et al. A prospective evaluation of a breast cancer prognosis signature in the observational RASTER study. Int J Cancer 2013;133:929-36. https://doi.org/10.1002/ijc.28082.
- Drukker CA, Nijenhuis MV, Bueno-de-Mesquita JM, Retèl VP, van Harten WH, van Tinteren H, et al. Optimized outcome prediction in breast cancer by combining the 70-gene signature with clinical risk prediction algorithms. Breast Cancer Res Treat 2014;145:697-705. https://doi.org/10.1007/s10549-014-2954-2.
- Vliek SB, Retèl V, Drukker C, Rutgers E, van Tinteren H, Van de Vijver MJ, et al. 10 years follow up of the RASTER study; implementing a genomic signature in daily practice (poster). Ann Oncol 2017;28:43-67. https://doi.org/10.1093/annonc/mdx362.008.
- Kwaliteitsinstituut voor de Gezondheidszorg CBO VvlK . Adjuvante Systemische Therapie Voor Het Operabel Mammacarcinoom. Behandeling Van Het Mammacarcinoom 2004.
- Vliek SB, Retèl V, Drukker C, Bueno-De-Mesquita JM, Rutgers E, van Tinteren H, et al. 195P - The 70-gene signature in node positive breast cancer: 10-year follow-up of the observational RASTER study. Ann Oncol 2017;28:43-67. https://doi.org/10.1093/annonc/mdx362.
- Gnant M, Filipits M, Greil R, Stoeger H, Rudas M, Bago-Horvath Z, et al. Predicting distant recurrence in receptor-positive breast cancer patients with limited clinicopathological risk: using the PAM50 Risk of Recurrence score in 1478 postmenopausal patients of the ABCSG-8 trial treated with adjuvant endocrine therapy alone. Ann Oncol 2014;25:339-45. https://doi.org/10.1093/annonc/mdt494.
- Filipits M, Nielsen TO, Rudas M, Greil R, Stöger H, Jakesz R, et al. The PAM50 risk-of-recurrence score predicts risk for late distant recurrence after endocrine therapy in postmenopausal women with endocrine-responsive early breast cancer. Clin Cancer Res 2014;20:1298-305. https://doi.org/10.1158/1078-0432.CCR-13-1845.
- Liu MC, Pitcher BN, Mardis ER, Davies SR, Friedman PN, Snider JE, et al. PAM50 gene signatures and breast cancer prognosis with adjuvant anthracycline- and taxane-based chemotherapy: correlative analysis of C9741 (Alliance). NPJ Breast Cancer 2016;2. https://doi.org/10.1038/npjbcancer.2015.23.
- Liu S, Chapman JA, Burnell MJ, Levine MN, Pritchard KI, Whelan TJ, et al. Prognostic and predictive investigation of PAM50 intrinsic subtypes in the NCIC CTG MA.21 phase III chemotherapy trial. Breast Cancer Res Treat 2015;149:439-48. https://doi.org/10.1007/s10549-014-3259-1.
- Martin M, Brase JC, Ruiz A, Prat A, Kronenwett R, Calvo L, et al. Prognostic ability of EndoPredict compared to research-based versions of the PAM50 risk of recurrence (ROR) scores in node-positive, estrogen receptor-positive, and HER2-negative breast cancer. A GEICAM/9906 sub-study. Breast Cancer Res Treat 2016;156:81-9. https://doi.org/10.1007/s10549-016-3725-z.
- Martin M, Brase JC, Calvo L, Krappmann K, Ruiz-Borrego M, Fisch K, et al. Clinical validation of the EndoPredict test in node-positive, chemotherapy-treated ER+/HER2– breast cancer patients: results from the GEICAM 9906 trial. Breast Cancer Res 2014;16. https://doi.org/10.1186/bcr3642.
- Chia SK, Bramwell VH, Tu D, Shepherd LE, Jiang S, Vickery T, et al. A 50-gene intrinsic subtype classifier for prognosis and prediction of benefit from adjuvant tamoxifen. Clin Cancer Res 2012;18:4465-72. https://doi.org/10.1158/1078-0432.CCR-12-0286.
- Ejlertsen B, Jensen MB, Eriksen JO, Kiboll T, Rasmussen BB, Knoop AS, et al. Validation of prediction of distant recurrence (DR) by Prosigna (PAM50) in subgroups of a Danish Breast Cancer Cooperative Group (DBCG) cohort of node-positive (N1), hormone receptor positive (HR+), post-menopausal early breast cancer (EBC) patients allocated 5yr of endocrine therapy (ET). J Clin Oncol Conf 2015;33.
- Laenkholm AV, Jensen MB, Eriksen JO, Kiboll T, Rasmussen BB, Knoop AS, et al. Prediction of 10yr distant recurrence (DR) using the Prosigna (PAM50) assay in a Danish Breast Cancer Cooperative Group (DBCG) cohort of post-menopausal Danish women with hormone receptor-positive (HR+) early breast cancer (EBC) allocated to 5yr of endocrine therapy (ET) alone. J Clin Oncol Conf 2015;33.
- Laenkholm A, Jensen M, Eriksen J. Prediction of late distant recurrence (DR) using the Prosigna (PAM50) test in a Danish Breast Cancer Cooperative Group (DBCG) cohort of postmenopausal women diagnosed with hormone receptor-positive (HR+) early breast cancer (EBC) allocated to 5yr of endocrine therapy (ET). J Clin Oncol 2015;33. https://doi.org/10.1200/jco.2015.33.15_suppl.544.
- Laenkholm AV, Jensen MB, Buckingham W, Schaper C, Knoop A, Eriksen JO, et al. Prediction of 10yr Distant Recurrence (DR) Using the Prosigna® (PAM50) Assay in Histological Subgroups of a Danish Breast Cancer Group (DBCG) Cohort of Postmenopausal Danish Women With Hormone Receptor-Positive (HR+) Early Breast Cancer (EBC) Allocated to 5yr of Endocrine Therapy (ET) Alone n.d.
- Wallden B, Storhoff J, Nielsen T, Dowidar N, Schaper C, Ferree S, et al. Development and verification of the PAM50-based Prosigna breast cancer gene signature assay. BMC Med Genomics 2015;8. https://doi.org/10.1186/s12920-015-0129-6.
- Nielsen TO, Parker JS, Leung S, Voduc D, Ebbert M, Vickery T, et al. A comparison of PAM50 intrinsic subtyping with immunohistochemistry and clinical prognostic factors in tamoxifen-treated estrogen receptor-positive breast cancer. Clin Cancer Res 2010;16:5222-32. https://doi.org/10.1158/1078-0432.CCR-10-1282.
- Filipits M, Rudas M, Jakesz R, Dubsky P, Fitzal F, Singer CF, et al. A new molecular predictor of distant recurrence in ER-positive, HER2-negative breast cancer adds independent information to conventional clinical risk factors. Clin Cancer Res 2011;17:6012-20. https://doi.org/10.1158/1078-0432.CCR-11-0926.
- EPclin in ABCSG-6+8: Subgroup Analyses. London: Myriad Genetics Ltd; 2015.
- Dubsky P, Brase JC, Jakesz R, Rudas M, Singer CF, Greil R, et al. The EndoPredict score provides prognostic information on late distant metastases in ER+/HER2- breast cancer patients. Br J Cancer 2013;109:2959-64. https://doi.org/10.1038/bjc.2013.671.
- Dubsky P, Filipits M, Jakesz R, Rudas M, Singer CF, Greil R, et al. EndoPredict improves the prognostic classification derived from common clinical guidelines in ER-positive, HER2-negative early breast cancer. Ann Oncol 2013;24:640-7. https://doi.org/10.1093/annonc/mds334.
- Rahilly-Tierney C, Walton SM. Cost-effectiveness of the 70-gene signature versus adjuvant! Online and systematic chemotherapy for risk stratification of patients with node-negative breast cancer: does accuracy matter?. J Clin Oncol 2015;33:1628-9. https://doi.org/10.1200/JCO.2014.60.5006.
- Christiansen J, Bartlett JMS, Gustavson M, Rimm D, Robson T, Van De Velde CJH, et al. Validation of IHC4 algorithms for prediction of risk of recurrence in early breast cancer using both conventional and quantitative IHC approaches. J Clin Oncol Conf 2012;30.
- Gluz O, Liedtke C, Huober J, Peyro-Saint-Paul H, Kates RE, Kreipe HH, et al. Comparison of prognostic and predictive impact of genomic or central grade and immunohistochemical subtypes or IHC4 in HR+/HER2– early breast cancer: WSG-AGO EC-Doc Trial. Ann Oncol 2016;27:1035-40. https://doi.org/10.1093/annonc/mdw070.
- Lin CH, Chen IC, Huang CS, Hu FC, Kuo WH, Kuo KT, et al. TP53 mutational analysis enhances the prognostic accuracy of IHC4 and PAM50 assays. Sci Rep 2015;5. https://doi.org/10.1038/srep17879.
- Rohan TE, Xue X, Lin HM, D’Alfonso TM, Ginter PS, Oktay MH, et al. Tumor microenvironment of metastasis and risk of distant metastasis of breast cancer. J Natl Cancer Inst 2014;106. https://doi.org/10.1093/jnci/dju136.
- Stephen J, Murray G, Cameron DA, Thomas J, Kunkler IH, Jack W, et al. Time dependence of biomarkers: non-proportional effects of immunohistochemical panels predicting relapse risk in early breast cancer. Br J Cancer 2014;111:2242-7. https://doi.org/10.1038/bjc.2014.530.
- Viale G, Speirs V, Bartlett JM, Mousa K, Kalaitzaki E, Palmieri C, et al. Pr prognostic and predictive value of IHC4 and erb1 in the intergroup exemestane study (IES)-on behalf of the pathies investigators. Ann Oncol 2013;24:iii29-30. https://doi.org/10.1093/annonc/mdt084.3.
- Vincent-Salomon A, Benhamo V, Gravier E, Rigaill G, Gruel N, Robin S, et al. Genomic instability: a stronger prognostic marker than proliferation for early stage luminal breast carcinomas. PLOS ONE 2013;8. https://doi.org/10.1371/journal.pone.0076496.
- Prat A, Cheang MC, Martín M, Parker JS, Carrasco E, Caballero R, et al. Prognostic significance of progesterone receptor-positive tumor cells within immunohistochemically defined luminal A breast cancer. J Clin Oncol 2013;31:203-9. https://doi.org/10.1200/JCO.2012.43.4134.
- Bartlett JM, Brookes CL, Robson T, van de Velde CJ, Billingham LJ, Campbell FM, et al. Estrogen receptor and progesterone receptor as predictive biomarkers of response to endocrine therapy: a prospectively powered pathology study in the Tamoxifen and Exemestane adjuvant multinational trial. J Clin Oncol 2011;29:1531-8. https://doi.org/10.1200/JCO.2010.30.3677.
- Faratian D, Kay C, Campbell F, Robson T, Grant M, Rea D, et al. Automated image analysis for high-throughput quantitative detection of ER and PgR expression levels in large-scale clinical studies: the TEAM trial experience. Breast Cancer Res Treat 2007;106.
- Hammond ME, Hayes DF, Dowsett M, Allred DC, Hagerty KL, Badve S, et al. American Society of Clinical Oncology/College of American Pathologists guideline recommendations for immunohistochemical testing of estrogen and progesterone receptors in breast cancer (unabridged version). Arch Pathol Lab Med 2010;134:e48-72. https://doi.org/10.1043/1543-2165-134.7.e48.
- Wolff AC, Hammond ME, Schwartz JN, Hagerty KL, Allred DC, Cote RJ, et al. American Society of Clinical Oncology/College of American Pathologists guideline recommendations for human epidermal growth factor receptor 2 testing in breast cancer. J Clin Oncol 2007;25:118-45. https://doi.org/10.1200/JCO.2006.09.2775.
- Kirkegaard T, Edwards J, Tovey S, McGlynn LM, Krishna SN, Mukherjee R, et al. Observer variation in immunohistochemical analysis of protein expression, time for a change?. Histopathology 2006;48:787-94. https://doi.org/10.1111/j.1365-2559.2006.02412.x.
- Coombes RC, Hall E, Gibson LJ, Paridaens R, Jassem J, Delozier T, et al. A randomized trial of exemestane after two to three years of tamoxifen therapy in postmenopausal women with primary breast cancer. N Engl J Med 2004;350:1081-92. https://doi.org/10.1056/NEJMoa040331.
- Martín M, Rodríguez-Lescure A, Ruiz A, Alba E, Calvo L, Ruiz-Borrego M, et al. Randomized phase 3 trial of fluorouracil, epirubicin, and cyclophosphamide alone or followed by Paclitaxel for early breast cancer. J Natl Cancer Inst 2008;100:805-14. https://doi.org/10.1093/jnci/djn151.
- Nitz U, Gluz O, Huober J, Kreipe HH, Kates RE, Hartmann A, et al. Final analysis of the prospective WSG-AGO EC-Doc versus FEC phase III trial in intermediate-risk (pN1) early breast cancer: efficacy and predictive value of Ki67 expression. Ann Oncol 2014;25:1551-7. https://doi.org/10.1093/annonc/mdu186.
- Arbeitsgemeinschaft Gynäkologische Onkologie . Aktuelle Empfehlungen Zur Diagnostik Und Therapie primärer Und Metastasierter Mammakarzinome Der Kommission MAMMA in Der AGO 2006. www.ago-online.de (accessed November 2017).
- Hassan S, Ooi J, Garnsey C, Walker P. Does oncotype DX testing prove to be both cost effective and clinically beneficial in patients with early breast cancer? A single unit observational study. Br J Surg 2015;102.
- Hassan S, Ooi J, Welsh R, Pearson M. Oncotype DX testing: our experience at the Royal Bolton Hospital. Eur J Surg Oncol 2015;41. https://doi.org/10.1016/j.ejso.2015.03.039.
- Holt S, Bertelli G, Humphreys I, Valentine W, Durrani S, Pudney D, et al. A decision impact, decision conflict and economic assessment of routine oncotype DX testing of 146 women with node-negative or pNImi, ER-positive breast cancer in the U.K. Br J Cancer 2013;108:2250-8. https://doi.org/10.1038/bjc.2013.207.
- Albanell J, Svedman C, Gligorov J, Holt SD, Bertelli G, Blohmer JU, et al. Pooled analysis of prospective European studies assessing the impact of using the 21-gene Recurrence Score assay on clinical decision making in women with oestrogen receptor-positive, human epidermal growth factor receptor 2-negative early-stage breast cancer. Eur J Cancer 2016;66:104-13. https://doi.org/10.1016/j.ejca.2016.06.027.
- Kiernan T, Olsson-Brown AC, Innes H, Holcombe C, Thorp N, O’Hagan J, et al. Knowledge of oncotype Dx recurrence score increases confidence and concordance in adjuvant decisions of U.K. Oncologists. Cancer Res 2016;76.
- Kuchel A, Robinson T, Comins C, Shere M, Varughese M, Sparrow G, et al. The impact of the 21-gene assay on adjuvant treatment decisions in oestrogen receptor-positive early breast cancer: a prospective study. Br J Cancer 2016;114:731-6. https://doi.org/10.1038/bjc.2016.48.
- Loncaster J, Armstrong A, Howell S, Wilson G, Welch R, Chittalia A, et al. Impact of oncotype DX breast recurrence score testing on adjuvant chemotherapy use in early breast cancer: real world experience in Greater Manchester, UK. Eur J Surg Oncol 2017;43:931-7. https://doi.org/10.1016/j.ejso.2016.12.010.
- Albanell J, González A, Ruiz-Borrego M, Alba E, García-Saenz JA, Corominas JM, et al. Prospective transGEICAM study of the impact of the 21-gene recurrence score assay and traditional clinicopathological factors on adjuvant clinical decision making in women with estrogen receptor-positive (ER+) node-negative breast cancer. Ann Oncol 2012;23:625-31. https://doi.org/10.1093/annonc/mdr278.
- Bodmer A, Hugli A, Diebold Berger S, Favet L, Guetty Alberto M, Exquis B. Usefulness of the 21-gene assay to guide adjuvant chemotherapy decision-making: Geneva experience. Breast 2015;24. https://doi.org/10.1016/S0960-9776(15)70273-0.
- De San Vicente BL, Novas P, Calvo EG, Arrazubi V, Sala MA, Fernandez S, et al. Impact of the intermediate oncotype DX Recurrence Score results on adjuvant treatment recommendations in hormone receptor-positive early breast cancer in a single center. J Clin Oncol Conf 2015;33.
- Dieci MV, Guarneri V, Mion M, Tortora G, Morandi P, Gori S, et al. First prospective multicenter Italian study on the impact of the 21-gene recurrence score (RS) in adjuvant clinical decisions for ER + HER2–early breast cancer patients. Ann Oncol Conference: 41st European Society for Medical Oncology Congress, ESMO 2016;27.
- Dreyfus C, Ballester M, Gligorov J, Agranat P, Antoine M, Tengher I, et al. Impact of the 21-gene assay in decision-making during multidisciplinary breast meeting: a French experience. Gynecol Obstet Fertil 2015;43:780-5. https://doi.org/10.1016/j.gyobfe.2015.10.002.
- Eiermann W, Rezai M, Kümmel S, Kühn T, Warm M, Friedrichs K, et al. The 21-gene recurrence score assay impacts adjuvant therapy recommendations for ER-positive, node-negative and node-positive early breast cancer resulting in a risk-adapted change in chemotherapy use. Ann Oncol 2013;24:618-24. https://doi.org/10.1093/annonc/mds512.
- Gligorov J, Pivot XB, Jacot W, Naman HL, Spaeth D, Misset JL, et al. Prospective clinical utility study of the use of the 21-gene assay in adjuvant clinical decision making in women with estrogen receptor-positive early invasive breast cancer: results from the SWITCH study. Oncologist 2015;20:873-9. https://doi.org/10.1634/theoncologist.2014-0467.
- Hejduk K, Petrakova K, Petruzelka L, Bielcikova Z, Kolarova I, Finek J, et al. Economic assessment of routine oncotype DX testing of estrogen receptor positive (ER+) early breast cancer (EBC) patients with grade 2 tumours in Czech Republic. Value Health 2016;19. https://doi.org/10.1016/j.jval.2016.09.2140.
- Petrakova K, Petruzelka LB, Holanek M, Svoboda T, Palacova M, Kolarova I, et al. The impact of the 21-gene assay in the Czech Republic on adjuvant chemotherapy (chemotherapy) recommendations and costs in estrogen receptor positive (ER+) early stage breast cancer (ESBC) patients with grade 2 tumors and risk factors. J Clin Oncol Conf 2016;34.
- Mouysset J, Laplaige P, Fignon A, Jallais L, Lafuma A, Michaud P, et al. The 21-gene assay in the decision impact assessment of ER+, HER2– breast cancer: a French real life prospective study. Value Health 2016;19. https://doi.org/10.1016/j.jval.2016.09.1945.
- Novas P, Calvo EG, Gonzalez MAS, Arteaga JFA, San Vicente BLD, Arrazubi V, et al. Impact of onco-type DX recurrence score in the management of pN1mic early breast cancer. J Clin Oncol Conf 2016;34.
- Pestalozzi BC, Tausch C, Dedes KJ, Rochlitz C, Zimmermann S, Von Moos R, et al. Adjuvant treatment recommendations for ER+ early breast cancer patients by Swiss tumor boards (SAKK 26/10). Eur J Cancer 2015;51. https://doi.org/10.1016/S0959-8049(16)30891-7.
- Wassermann J, Toledano A, Gligorov J, Conforti R, Grapin JP, Benothman S, et al. Routine practice use of oncotype Dx assay in French breast cancer patients. Eur J Cancer 2015;51. https://doi.org/10.1016/S0959-8049(16)30916-9.
- Bloomfield DJ, Arbon A, Cox J, Hack B, Hall J, Harper-Wynne C. Patient/oncologist decisions about adjuvant chemotherapy in ER+ve, HER2–ve early breast cancer following EndoPredict testing. J Clin Oncol 2017;35.
- Ettl J, Lackmann KG, Hapfelmeier A, Klein E, Paepke S, Petry C, et al. Prospective comparison of conventional clinicopathological factors, uPA/PAI-1 and EndoPredict-clin score (EPclin) for adjuvant clinical decision making in ER-positive, HER2– breast cancer: Progesterone receptor expression is strongly associat. Cancer Res 2015;75. https://doi.org/10.1158/1538-7445.SABCS14-P4-11-27.
- Müller BM, Keil E, Lehmann A, Winzer KJ, Richter-Ehrenstein C, Prinzler J, et al. The EndoPredict gene-expression assay in clinical practice - performance and impact on clinical decisions. PLOS ONE 2013;8. https://doi.org/10.1371/journal.pone.0068252.
- Penault-Llorca F, Kwaitkovski F, Grenier J, Levy C, Leheurteur M, Uwer L, et al. UCBG: 2-14: A Prospective Multicenter Non-Randomized Trial Evaluating the Effect of Endo Predict® (Epclin®) Clinico-Genomic Test on Treatment Decision Making Among Patients With Intermediate Clinical Risk n.d.
- Yeo B, Zabaglo L, Hills M, Dodson A, Smith I, Dowsett M. Clinical utility of the IHC4+C score in oestrogen receptor-positive early breast cancer: a prospective decision impact study. Br J Cancer 2015;113:390-5. https://doi.org/10.1038/bjc.2015.222.
- Martin M, Gonzalez-Rivera M, Morales S, De La Haba J, Gonzalez-Cortijo L, Manso L, et al. Prospective study of the impact of the ProsignaTM assay on adjuvant clinical decision-making in women with estrogen receptor-positive, HER2–, node-negative breast cancer: a GEICAM study. Cancer Res 2015;75.
- Van Asten K, Neven P, Puthod V, Ghali N, Lintermans A, Jongen L, et al. Concordance between PAM50 and clinico-pathological prognostic markers when deciding on adjuvant chemotherapy in early breast cancer. Eur J Cancer 2016;57.
- Wuerstlein R, Sotlar K, Gluz O, Otremba B, von Schumann R, Witzel I, et al. The West German Study Group breast cancer intrinsic subtype study: a prospective multicenter decision impact study utilizing the Prosigna assay for adjuvant treatment decision-making in estrogen-receptor-positive, HER2-negative early-stage breast cancer. Curr Med Res Opin 2016;32:1217-24. https://doi.org/10.1185/03007995.2016.1166102.
- Cusumano PG, Generali D, Ciruelos E, Manso L, Ghanem I, Lifrange E, et al. European inter-institutional impact study of MammaPrint. Breast 2014;23:423-8. https://doi.org/10.1016/j.breast.2014.02.011.
- Drukker CA, van den Hout HC, Sonke GS, Brain E, Bonnefoi H, Cardoso F, et al. Risk estimations and treatment decisions in early stage breast cancer: agreement among oncologists and the impact of the 70-gene signature. Eur J Cancer 2014;50:1045-54. https://doi.org/10.1016/j.ejca.2014.01.016.
- Exner R, Bago-Horvath Z, Bartsch R, Mittlboeck M, Retèl VP, Fitzal F, et al. The multigene signature MammaPrint impacts on multidisciplinary team decisions in ER+, HER2– early breast cancer. Br J Cancer 2014;111:837-42. https://doi.org/10.1038/bjc.2014.339.
- Hartmann S, Gerber B, Elling D, Heintze K, Reimer T. The 70-gene signature as prognostic factor for elderly women with hormone receptor-positive, HER2-negative breast cancer. Breast Care 2012;7:19-24. https://doi.org/10.1159/000336552.
- Kuijer A, Straver M, Elias S, Smorenburg C, Wesseling J, Linn S, et al. Impact of 70-Gene Signature Use on Adjuvant Chemotherapy Decisions in Early Breast Cancer Patents: Results of the Prospective Symphony Triple A Study n.d.
- Rullan AJ, Pernas S, Margeli M, Joan D, Quiroga V, Stradella A, et al. Use of Mammaprint (c) (MMP) genetic signature in early breast cancer patients. Economic analisys of a 129 patient cohort treated in three Spanish hospitals. Cancer Res 2016;76. https://doi.org/10.1158/1538-7445.SABCS15-P6-11-04.
- Wuerstlein R, Gluz O, Kates R, Persoon M, Wasmayr M, Knauer M, et al. Results of Multigene Assay (MammaPrint®) and Molecular Subtyping (BluePrint®) Substantially Impact Treatment Decision Making in Early Breast Cancer: Final Analysis of the WSG PRIME Decision Impact Study n.d.
- Evans CN, Brewer NT, Vadaparampil ST, Boisvert M, Ottaviano Y, Lee MC, et al. Impact of genomic testing and patient-reported outcomes on receipt of adjuvant chemotherapy. Breast Cancer Res Treat 2016;156:549-55. https://doi.org/10.1007/s10549-016-3780-5.
- Hinde S, Theriou C, May S, Matthews L, Arbon A, Fallowfield L, et al. The cost-effectiveness of EndoPredict to inform adjuvant chemotherapy decisions in early breast cancer. Ann Oncol 2017;28. https://doi.org/10.1093/annonc/mdx375.012.
- Lo SS, Mumby PB, Norton J, Rychlik K, Smerage J, Kash J, et al. Prospective multicenter study of the impact of the 21-gene recurrence score assay on medical oncologist and patient adjuvant breast cancer treatment selection. J Clin Oncol 2010;28:1671-6. https://doi.org/10.1200/JCO.2008.20.2119.
- Retèl VP, Groothuis-Oudshoorn CG, Aaronson NK, Brewer NT, Rutgers EJ, van Harten WH. Association between genomic recurrence risk and well-being among breast cancer patients. BMC Cancer 2013;13. https://doi.org/10.1186/1471-2407-13-295.
- Horowitz M, Wilner N, Alvarez W. Impact of event scale: a measure of subjective stress. Psychosom Med 1979;41:209-18. https://doi.org/10.1097/00006842-197905000-00004.
- Lynch HT, Lemon SJ, Durham C, Tinley ST, Connolly C, Lynch JF, et al. A descriptive study of BRCA1 testing and reactions to disclosure of test results. Cancer 1997;79:2219-28. https://doi.org/10.1002/(SICI)1097-0142(19970601)79:11<2219::AID-CNCR21>3.0.CO;2-Y.
- Lerman C, Daly M, Masny A, Balshem A. Attitudes about genetic testing for breast-ovarian cancer susceptibility. J Clin Oncol 1994;12:843-50. https://doi.org/10.1200/JCO.1994.12.4.843.
- Losk K, Vaz-Luis I, Camuso K, Batista R, Lloyd M, Tukenmez M, et al. Factors associated with delays in chemotherapy initiation among patients with breast cancer at a comprehensive cancer center. J Natl Compr Canc Netw 2016;14:1519-26. https://doi.org/10.6004/jnccn.2016.0163.
- Evidence provided to NICE. London: Myriad Genetics Ltd; 2017.
- NHS England Oncotype DX Access Scheme Dataset (unpublished, data held on file by Genomic Health, Inc.). London: NHS England; 2016.
- Bespoke Data Request – Chemotherapy Use Amongst Women with Early breast cancer (data held on file). London: National Cancer Registration and Analysis Service; 2017.
- Early Breast Cancer Trialists’ Collaborative Group (EBCTCG) . Comparisons between different polychemotherapy regimens for early breast cancer: meta-analyses of long-term outcome among 100 000 women in 123 randomised trials. Lancet 2012;379:432-44. https://doi.org/10.1016/S0140-6736(11)61625-5.
- Thomas RJ, Williams M, Marshall C, Glen J, Callam M. The total hospital and community UK costs of managing patients with relapsed breast cancer. Br J Cancer 2009;100:598-600. https://doi.org/10.1038/sj.bjc.6604911.
- de Bock GH, Putter H, Bonnema J, van der Hage JA, Bartelink H, van de Velde CJ. The impact of loco-regional recurrences on metastatic progression in early-stage breast cancer: a multistate model. Breast Cancer Res Treat 2009;117:401-8. https://doi.org/10.1007/s10549-008-0300-2.
- Wolff AC, Blackford AL, Visvanathan K, Rugo HS, Moy B, Goldstein LJ, et al. Risk of marrow neoplasms after adjuvant breast cancer therapy: the national comprehensive cancer network experience. J Clin Oncol 2015;33:340-8. https://doi.org/10.1200/JCO.2013.54.6119.
- Edlin R, Connock M, Tubeuf S, Round J, Fry-Smith A, Hyde C, et al. Azacitidine for the treatment of myelodysplastic syndrome, chronic myelomonocytic leukaemia and acute myeloid leukaemia. Health Technol Assess 2010;14. https://doi.org/10.3310/hta14Suppl1/10.
- National Life Tables, UK: 2013–15. Newport: Office for National Statistics; 2016.
- Lidgren M, Wilking N, Jönsson B, Rehnberg C. Health related quality of life in different states of breast cancer. Qual Life Res 2007;16:1073-81. https://doi.org/10.1007/s11136-007-9202-8.
- Younis T, Rayson D, Skedgel C. Adjuvant chemotherapy in breast cancer: is TC a cost-effective regimen compared to AC?. Cancer Res 2008;69.
- Campbell HE, Epstein D, Bloomfield D, Griffin S, Manca A, Yarnold J, et al. The cost-effectiveness of adjuvant chemotherapy for early breast cancer: a comparison of no chemotherapy and first, second, and third generation regimens for patients with differing prognoses. Eur J Cancer 2011;47:2517-30. https://doi.org/10.1016/j.ejca.2011.06.019.
- British National Formulary. London: BMJ Group and Pharmaceutical Press; 2017.
- Curtis L, Burns A. Unit Costs of Health and Social Care 2016. Canterbury: Personal Social Services Research Unit, University of Kent; 2016.
- NHS Reference Costs 2015/16. London: Department of Health and Social Care; 2017.
- Karnon J, Kerr GR, Jack W, Papo NL, Cameron DA. Health care costs for the treatment of breast cancer recurrent events: estimates from a UK-based patient-level analysis. Br J Cancer 2007;97:479-85. https://doi.org/10.1038/sj.bjc.6603887.
- Cuzick J, Sestak I, Baum M, Buzdar A, Howell A, Dowsett M, et al. ATAC/LATTE investigators. Effect of anastrozole and tamoxifen as adjuvant treatment for early-stage breast cancer: 10-year analysis of the ATAC trial. Lancet Oncol 2010;11:1135-41. https://doi.org/10.1016/S1470-2045(10)70257-6.
- Early Breast Cancer Trialists’ Collaborative Group . Effects of chemotherapy and hormonal therapy for early breast cancer on recurrence and 15-year survival: an overview of the randomised trials. Lancet 2005;365:1687-717. https://doi.org/10.1016/S0140-6736(05)66544-0.
- Pan H, Gray R, Davies C, Peto R, Bergh J, Pritchard KI, et al. Long-Term Recurrence Risks After Use of Endocrine Therapy for Only 5 Years: Relevance of Breast Tumour Characteristics 2016.
- Holt S, Bertelli G, Brinkworth E, Durrani S, Jones S, Khawaja S, et al. Results from a prospective clinical study on the impact of oncotype DX on adjuvant treatment decision making in a cohort of 142 UK patients. Cancer Res 2011;71. https://doi.org/10.1158/0008-5472.SABCS11-P5-14-26.
- Azacitidine for the Treatment of Myelodysplastic Syndromes, Chronic Myelomonocytic Leukaemia and Acute Myeloid Leukaemia. Technology Appraisal Guidance 218. London: NICE; 2011.
- Peasgood T, Ward SE, Brazier J. Health state utility values in breast cancer: a review and meta-analysis. Value Health 2011;14. https://doi.org/10.1016/j.jval.2011.08.1250.
- Farkkila N, Roine R, Jahkola T, Sintonen H, Hanninen J, Taari K, et al. Health state utilities in breast cancer. Value Health 2011;14. https://doi.org/10.1016/j.jval.2011.08.1238.
- Yousefi M, Najafi S, Ghaffari S, Mahboub-Ahari A, Ghaderi H. Comparison of SF-6D and EQ-5D scores in patients with breast cancer. Iran Red Crescent Med J 2016;18. https://doi.org/10.5812/ircmj.23556.
- Naik H, Howell D, Su S, Qiu X, Brown MC, Vennettilli A, et al. EQ-5D Health utility scores: data from a comprehensive canadian cancer centre. Patient 2017;10:105-15. https://doi.org/10.1007/s40271-016-0190-z.
- Stein RC, Dunn JA, Bartlett JM, Campbell AF, Marshall A, Hall P, et al. OPTIMA prelim: a randomised feasibility study of personalised care in the treatment of women with early breast cancer. Health Technol Assess 2016;20. https://doi.org/10.3310/hta20100.
- Drugs and Pharmaceutical Electronic Market Information (eMit). Leeds: Department of Health and Social Care; 2017.
- NHS Reference Costs 2013 to 2014. London: Department of Health and Social Care; 2015.
- Ademuyiwa FO, Miller A, O’Connor T, Edge SB, Thorat MA, Sledge GW, et al. The effects of oncotype DX recurrence scores on chemotherapy utilization in a multi-institutional breast cancer cohort. Breast Cancer Res Treat 2011;126:797-802. https://doi.org/10.1007/s10549-010-1329-6.
- Bargallo JE, Lara F, Shaw-Dulin R, Perez-Sánchez V, Villarreal-Garza C, Maldonado-Martinez H, et al. A study of the impact of the 21-gene breast cancer assay on the use of adjuvant chemotherapy in women with breast cancer in a Mexican public hospital. J Surg Oncol 2015;111:203-7. https://doi.org/10.1002/jso.23794.
- Basaran G, Saglam S, Tansan S, Demirel M, Gokmen E, Aktan S, et al. The impact of 21-gene recurrence score (RS) scores on treatment decisions: retrospective evaluation in Turkish early-stage breast cancer (BC) patients. J Clin Oncol Conf: ASCO Annual Meeting 2011;29.
- Bayewitz AY, Schneider JG, Ogden L, Turi G. Discrepancies in three commercial prognostic breast cancer assays used in adjuvant chemotherapy decisions. J Clin Oncol Conf 2014;32.
- Biroschak JR, Schwartz GF, Palazzo JP, Toll AD, Brill KL, Jaslow RJ, et al. Impact of oncotype DX on treatment decisions in ER+, node-negative breast cancer with histologic correlation. Breast J 2013;19:269-75. https://doi.org/10.1111/tbj.12099.
- Cheung PS, Tong AC, Leung RC, Kwan WH, Yau TC. Initial experience with the oncotype DX assay in decision-making for adjuvant therapy of early oestrogen receptor-positive breast cancer in Hong Kong. Hong Kong Med J 2014;20:401-6. https://doi.org/10.12809/hkmj134140.
- Chin-Lenn L, Segelov E, De Boer R, Marx G, Hughes TM, McCarthy N, et al. Indications for, and impact of oncotype DX on adjuvant treatment recommendations when third party funding is unavailable. Cancer Res 2016;76. https://doi.org/10.1158/1538-7445.SABCS15-P5-15-02.
- Crumbaker M, Rutovitz J, Chin R, Currer T, Hasovits C, Hughes M, et al. Effect of oncoytpe DX testing on adjuvant treatment recommendations in hormone sensitive early breast cancer. Asia-Pacific J Clin Oncol 2015;11.
- Davidson JA, Cromwell I, Ellard SL, Lohrisch C, Gelmon KA, Shenkier T, et al. A prospective clinical utility and pharmacoeconomic study of the impact of the 21-gene Recurrence Score® assay in oestrogen receptor positive node negative breast cancer. Eur J Cancer 2013;49:2469-75. https://doi.org/10.1016/j.ejca.2013.03.009.
- de Boer RH, Baker C, Speakman D, Chao CY, Yoshizawa C, Mann GB. The impact of a genomic assay (oncotype DX) on adjuvant treatment recommendations in early breast cancer. Med J Aust 2013;199:205-8. https://doi.org/10.5694/mja12.11334.
- Denkert C, Weber K, Krappmann K, Huober JB, Marine F, Schem C, et al. Risk assessment after neoadjuvant chemotherapy in luminal breast cancer: a prospectively planned validation of gene expression based and clinical prognostic scores in 428 residual tumor samples from two neoadjuvant clinical trials. J Clin Oncol Conf 2016;34.
- Fried G, Moskovitz M. Treatment decisions in estrogen receptor-positive early breast cancer patients with intermediate oncotype DX recurrence score results. SpringerPlus 2014;3. https://doi.org/10.1186/2193-1801-3-71.
- Geffen DB, Abu-Ghanem S, Sion-Vardy N, Braunstein R, Tokar M, Ariad S, et al. The impact of the 21-gene recurrence score assay on decision making about adjuvant chemotherapy in early-stage estrogen-receptor-positive breast cancer in an oncology practice with a unified treatment policy. Ann Oncol 2011;22:2381-6. https://doi.org/10.1093/annonc/mdq769.
- Jaafar H, Bashir MA, Taher A, Qawasmeh K, Jaloudi M. Impact of oncotype DX testing on adjuvant treatment decisions in patients with early breast cancer: a single-center study in the United Arab Emirates. Asia Pac J Clin Oncol 2014;10:354-60. https://doi.org/10.1111/ajco.12259.
- Jinyin Y, Xiaoyun Z, Ning L, Yuexing N, Haifeng C, Jianrong L, et al. The use of 21-gene breast cancer assay on adjuvant chemotherapy choice in women with breast cancer. Basic Clin Pharmacol Toxicol 2015;117.
- Joh JE, Esposito NN, Kiluk JV, Laronga C, Lee MC, Loftus L, et al. The effect of oncotype DX recurrence score on treatment recommendations for patients with estrogen receptor-positive early stage breast cancer and correlation with estimation of recurrence risk by breast cancer specialists. Oncologist 2011;16:1520-6. https://doi.org/10.1634/theoncologist.2011-0045.
- Jørgensen CL, Nielsen TO, Bjerre KD, Liu S, Wallden B, Balslev E, et al. PAM50 breast cancer intrinsic subtypes and effect of gemcitabine in advanced breast cancer patients. Acta Oncol 2014;53:776-87. https://doi.org/10.3109/0284186X.2013.865076.
- Lee MH, Han W, Lee JE, Kim KS, Park H, Kim J, et al. The clinical impact of 21-gene recurrence score on treatment decisions for patients with hormone receptor-positive early breast cancer in Korea. Cancer Res Treat 2015;47:208-14. https://doi.org/10.4143/crt.2013.223.
- Leitzin L, Sikorsky N, Leviov M, Grikstass E, Steiner M. Oncotype Dx; St. Gallen risk group and Adjuvant!online nomogram as prognostic predictor for lymph nodes positive breast cancer patients. Breast 2011;20. https://doi.org/10.1016/S0960-9776(11)70138-2.
- Leung RC, Yau TC, Chan MC, Chan SW, Chan TW, Tsang YY, et al. The impact of the oncotype DX breast cancer assay on treatment decisions for women with estrogen receptor-positive, node-negative breast carcinoma in Hong Kong. Clin Breast Cancer 2016;16:372-8. https://doi.org/10.1016/j.clbc.2016.03.002.
- Levine MN, Julian JA, Bedard PL, Eisen A, Trudeau ME, Higgins B, et al. Prospective evaluation of the 21-gene recurrence score assay for breast cancer decision-making in Ontario. J Clin Oncol 2016;34:1065-71. https://doi.org/10.1200/JCO.2015.62.8503.
- Li Y, Kurian AW, Bondarenko I, Taylor JMG, Jagsi R, Ward KC, et al. The influence of 21-gene recurrence score assay on chemotherapy use in a population-based sample of breast cancer patients. Breast Cancer Res Treat 2017;161:587-95. https://doi.org/10.1007/s10549-016-4086-3.
- Marshall DA, Deal K, Bombard Y, Leighl N, MacDonald KV, Trudeau M. How do women trade-off benefits and risks in chemotherapy treatment decisions based on gene expression profiling for early-stage breast cancer? A discrete choice experiment. BMJ Open 2016;6. https://doi.org/10.1136/bmjopen-2015-010981.
- Millien J, Edwards C, Kaltman R, McSwain AP, McGrail L, Teal CB. The 21-gene recurrence score influences treatment recommendations for patients with node-positive breast cancer. Ann Surg Oncol 2014;21:83-4.
- Oratz R, Kim B, Chao C, Skrzypczak S, Ory C, Bugarini R, et al. Physician survey of the effect of the 21-gene recurrence score assay results on treatment recommendations for patients with lymph node-positive, estrogen receptor-positive breast cancer. J Oncol Pract 2011;7:94-9. https://doi.org/10.1200/JOP.2010.000046.
- Ozmen V, Atasoy A, Gokmen E, Ozdogan M, Guler N, Uras C, et al. Impact of oncotype DX recurrence score on treatment decisions: results of a prospective multicenter study in Turkey. Cureus 2016;8. https://doi.org/10.7759/cureus.522.
- Partin JF, Mamounas EP. Impact of the 21-gene recurrence score assay compared with standard clinicopathologic guidelines in adjuvant therapy selection for node-negative, estrogen receptor-positive breast cancer. Ann Surg Oncol 2011;18:3399-406. https://doi.org/10.1245/s10434-011-1698-z.
- Pohl H, Kotze MJ, Grant KA, van der Merwe L, Pienaar FM, Apffelstaedt JP, et al. Impact of MammaPrint on clinical decision-making in South African patients with early-stage breast cancer. Breast J 2016;22:442-6. https://doi.org/10.1111/tbj.12605.
- Rayhanabad JA, Difronzo LA, Haigh PI, Romero L. Changing paradigms in breast cancer management: introducing molecular genetics into the treatment algorithm. Am Surg 2008;74:887-90.
- Saghatchian M, Mook S, Pruneri G, Viale G, Glas AM, Guerin S, et al. Additional prognostic value of the 70-gene signature (MammaPrint(®)) among breast cancer patients with 4-9 positive lymph nodes. Breast 2013;22:682-90. https://doi.org/10.1016/j.breast.2012.12.002.
- Schneider JG, Khalil DN. Why does oncotype DX recurrence score reduce adjuvant chemotherapy use?. Breast Cancer Res Treat 2012;134:1125-32. https://doi.org/10.1007/s10549-012-2134-1.
- Smith JS, Kass R, Kauffman G. Changes in treatment recommendations based on the 21-gene recurrence score even in node-positive patients. Ann Surg Oncol 2012;1:105-6.
- Torres S, Trudeau M, Gandhi S, Warner E, Verma S, Pritchard K, et al. Prospective evaluation of the impact of the 21-gene recurrence score assay on adjuvant treatment decisions for women with node-positive breast cancer in Ontario, Canada. Ann Oncol Conf 2016;27. https://doi.org/10.1093/annonc/mdw364.39.
- Tsai ML, Untch S, Treece T, Lo SS. The 70-gene signature to provide risk stratification and treatment guidance for patients classified as intermediate by the 21-gene assay. J Clin Oncol Conf 2016;34.
- Turnbull A, Lee Y, Pearce D, Martinez-Perez C, Uddin S, Webb H, et al. A test utilising diagnostic and on-treatment biomarkers to improve prediction of response to endocrine therapy in breast cancer. J Clin Oncol Conf 2016;34.
- Williams SA, Tatarian T, Teal CB, McSwain AP, Siegel RS, Martin ON, et al. Clinical utility and therapeutic implications of oncotype analysis in patients with breast cancer. J Clin Oncol Conf 2012;30.
- Wolf DM, Daemen A, Yau C, Davis S, Boudreau A, Swigart L, et al. MammaPrint ultra-high risk score is associated with response to neoadjuvant chemotherapy in the I-SPY 1 TRIAL (CALGB 150007/15 0012; ACRIN 6657). Cancer Res 2013;73. https://doi.org/10.1158/0008-5472.SABCS13-P1-08-01.
- Yamauchi H, Nakagawa C, Takei H, Chao C, Yoshizawa C, Yagata H, et al. Prospective study of the effect of the 21-gene assay on adjuvant clinical decision making in Japanese women with estrogen receptor-positive, node-negative, and node positive breast cancer. Clin Breast Cancer 2014;14:191-7. https://doi.org/10.1016/j.clbc.2013.10.017.
- Zhang YN, Zhou YD, Mao F, Sun Q. Impact of the 21-gene recurrence score assay in adjuvant chemotherapy selection for node-negative, hormone receptor-positive breast cancer in the Chinese population. Neoplasma 2015;62:658-65. https://doi.org/10.4149/neo_2015_079.
- Afentakis M, Dowsett M, Sestak I, Salter J, Howell T, Buzdar A, et al. Immunohistochemical BAG1 expression improves the estimation of residual risk by IHC4 in postmenopausal patients treated with anastrazole or tamoxifen: a TransATAC study. Breast Cancer Res Treat 2013;140:253-62. https://doi.org/10.1007/s10549-013-2628-5.
- Budczies J, Bockmayr M, Denkert C, Klauschen F, Lennerz JK, Györffy B, et al. Classical pathology and mutational load of breast cancer - integration of two worlds. J Pathol Clin Res 2015;1:225-38. https://doi.org/10.1002/cjp2.25.
- Chang JM, McCullough AE, Dueck AC, Kosiorek HE, Ocal IT, Lidner TK, et al. Back to basics: traditional nottingham grade mitotic counts alone are significant in predicting survival in invasive breast carcinoma. Ann Surg Oncol 2015;22:509-15. https://doi.org/10.1245/s10434-015-4616-y.
- Cheang MC, Voduc KD, Tu D, Jiang S, Leung S, Chia SK, et al. Responsiveness of intrinsic subtypes to adjuvant anthracycline substitution in the NCIC.CTG MA.5 randomized trial. Clin Cancer Res 2012;18:2402-12. https://doi.org/10.1158/1078-0432.CCR-11-2956.
- D’Alfonso TM, van Laar RK, Vahdat LT, Hussain W, Flinchum R, Brown N, et al. BreastPRS is a gene expression assay that stratifies intermediate-risk oncotype DX patients into high- or low-risk for disease recurrence. Breast Cancer Res Treat 2013;139:705-15. https://doi.org/10.1007/s10549-013-2604-0.
- Dowsett M, Sestak I, Buus R, Lopez-Knowles E, Cuzick J. Oestrogen module of 21-gene recurrence score predicts increased late recurrence for ER+HER2–breast cancer. Eur J Cancer 2014;50:S105-6.
- Dowsett M, Sestak I, Buus R, Lopez-Knowles E, Mallon E, Howell A, et al. Estrogen receptor expression in 21-gene recurrence score predicts increased late recurrence for estrogen-positive/HER2-negative breast cancer. Clin Cancer Res 2015;21:2763-70. https://doi.org/10.1158/1078-0432.CCR-14-2842.
- Fumagalli D, Jose V, Salgado R, Majjaj S, Singhal S, Vincent D, et al. Effect of sample preservation method and transportation duration on tumor gene expression profiling in breast cancer. Cancer Res 2012;72. https://doi.org/10.1158/0008-5472.SABCS12-P1-07-08.
- Jones LW, Kwan ML, Weltzien E, Chandarlapaty S, Sternfeld B, Sweeney C, et al. Exercise and prognosis on the basis of clinicopathologic and molecular features in early-stage breast cancer: the LACE and pathways studies. Cancer Res 2016;76:5415-22. https://doi.org/10.1158/0008-5472.CAN-15-3307.
- Kuniyoshi RK, Gehrke Fde S, Alves BC, Vilas-Bôas V, Coló AE, Sousa N, et al. Gene profiling and circulating tumor cells as biomarker to prognostic of patients with locoregional breast cancer. Tumour Biol 2015;36:8075-83. https://doi.org/10.1007/s13277-015-3529-5.
- Kurshumliu F, Gashi-Luci L, Kadare S, Alimehmeti M, Gozalan U. Classification of patients with breast cancer according to Nottingham prognostic index highlights significant differences in immunohistochemical marker expression. World J Surg Oncol 2014;12. https://doi.org/10.1186/1477-7819-12-243.
- Lisette SS, Yao K, Turk M, Kaul K, Weaver JE, De Snoo F, et al. Molecular subtyping using MammaPrint and BluePrint as an outcome predictor in 180 U.S. breast cancer (BC) patients. J Clin Oncol Conf 2012;30.
- Marin M, Migliosi G, Pagliari C, Fontana P, Baiocco R, Indelicato A, et al. Translating gene expression profiling of breast cancer into clinical practice: a multidisciplinary study in an Italian hospital. Am J Pathol 2014;1.
- Marin MG, Baiocco R, Fontana P, Bertalot G, Pagliari C, Giovanni M, et al. Translating gene expression profiling of breast cancer into clinical practice: experience in an Italian breast cancer hospital unit. Biochim Clin 2013;37.
- Martín M, Prat A, Rodríguez-Lescure A, Caballero R, Ebbert MT, Munárriz B, et al. PAM50 proliferation score as a predictor of weekly paclitaxel benefit in breast cancer. Breast Cancer Res Treat 2013;138:457-66. https://doi.org/10.1007/s10549-013-2416-2.
- Martin M, Rodriguez-Lescure A, Stijleman IJ, Munarriz B, Ruiz-Borrego M, Davis C, et al. PAM50 proliferation index predicts response to weekly adjuvant paclitaxel in node-positive operable breast cancer. Cancer Res 2011;71. https://doi.org/10.1158/0008-5472.SABCS11-P1-06-04.
- Milburn M, Rosman M, Mylander C, Tafra L. Is oncotype DX recurrence score (RS) of prognostic value once HER2-positive and. low-ER expression patients are removed?. Breast J 2013;19:357-64. https://doi.org/10.1111/tbj.12126.
- Milioli HH, Vimieiro R, Riveros C, Tishchenko I, Berretta R, Moscato P. The discovery of novel biomarkers improves breast cancer intrinsic subtype prediction and reconciles the labels in the METABRIC data set. PLOS ONE 2015;10. https://doi.org/10.1371/journal.pone.0129711.
- Milioli HH, Vimieiro R, Tishchenko I, Riveros C, Berretta R, Moscato P. Iteratively refining breast cancer intrinsic subtypes in the METABRIC dataset. BioData Min 2016;9. https://doi.org/10.1186/s13040-015-0078-9.
- Muniz J, Kidwell KM, Henry NL. Associations between metabolic syndrome, breast cancer recurrence, and the 21-gene recurrence score assay. Breast Cancer Res Treat 2016;157:597-603. https://doi.org/10.1007/s10549-016-3846-4.
- Muranen TA, Blomqvist C, Dörk T, Jakubowska A, Heikkilä P, Fagerholm R, et al. Patient survival and tumor characteristics associated with CHEK2:p.I157T - findings from the Breast Cancer Association Consortium. Breast Cancer Res 2016;18. https://doi.org/10.1186/s13058-016-0758-5.
- Neapolitan RE, Jiang X. Study of integrated heterogeneous data reveals prognostic power of gene expression for breast cancer survival. PLOS ONE 2015;10. https://doi.org/10.1371/journal.pone.0117658.
- Netanely D, Avraham A, Ben-Baruch A, Evron E, Shamir R. Erratum to: Expression and methylation patterns partition luminal-A breast tumors into distinct prognostic subgroups. Breast Cancer Res 2016;18. https://doi.org/10.1186/s13058-016-0775-4.
- Nishio M, Naoi Y, Tsunashima R, Nakauchi C, Kagara N, Shimoda M, et al. 72-gene classifier for predicting prognosis of estrogen receptor-positive and node-negative breast cancer patients using formalin-fixed, paraffin-embedded tumor tissues. Clin Breast Cancer 2014;14:e73-80. https://doi.org/10.1016/j.clbc.2013.11.006.
- Rakha EA, Agarwal D, Green AR, Ashankyty I, Ellis IO, Ball G, et al. Prognostic stratification of oestrogen receptor-positive HER2-negative lymph node-negative class of breast cancer. Histopathology 2017;70:622-31. https://doi.org/10.1111/his.13108.
- Stavridi F, Kalogeras KT, Pliarchopoulou K, Wirtz RM, Alexopoulou Z, Zagouri F, et al. Comparison of the ability of different clinical treatment scores to estimate prognosis in high-risk early breast cancer patients: a hellenic cooperative oncology group study. PLOS ONE 2016;11. https://doi.org/10.1371/journal.pone.0164013.
- Yamamoto-Ibusuki M, Yamamoto Y, Yamamoto S, Fujiwara S, Fu P, Honda Y, et al. Comparison of prognostic values between combined immunohistochemical score of estrogen receptor, progesterone receptor, human epidermal growth factor receptor 2, Ki-67 and the corresponding gene expression score in breast cancer. Mod Pathol 2013;26:79-86. https://doi.org/10.1038/modpathol.2012.151.
- Barton MK. Researchers find discordance between standard human epidermal growth factor receptor 2 (HER2) testing and HER2 status reported on oncotype DX. CA Cancer J Clin 2012;62:71-2. https://doi.org/10.3322/caac.21133.
- Baxter E, Gondara L, Lohrisch C, Chia S, Gelmon K, Hayes M, et al. Using proliferative markers and oncotype DX in therapeutic decision-making for breast cancer: the B.C. experience. Curr Oncol 2015;22:192-8. https://doi.org/10.3747/co.22.2284.
- Clark BZ, Dabbs DJ, Cooper KL, Bhargava R. Impact of progesterone receptor semiquantitative immunohistochemical result on oncotype DX recurrence score: a quality assurance study of 1074 cases. Appl Immunohistochem Mol Morphol 2013;21:287-91. https://doi.org/10.1097/PAI.0b013e31826f80c9.
- Acs G, Khkapour N, Kiluk J, Lee M, Laronga C. Prediction of the oncotype DX recurrence score: prospective validation of two equations with clinicopathologic analysis and outcome. Lab Invest 2015;95.
- Acs G, Khkapour N, Kiluk J, Lee M, Laronga C. Can we predict the oncotype DX recurrence score? A clinicopathologic analysis of discrepant cases as determined by two equations with clinical outcome. Lab Invest 2015;95.
- Ahmad FK, Deris S, Abdullah MS. Synergy network based inference for breast cancer metastasis. WCIT 2011;3.
- Alvarado MD, Prasad C, Rothney M, Cherbavaz DB, Sing AP, Baehner FL, et al. A prospective comparison of the 21-gene recurrence score and the PAM50-based prosigna in estrogen receptor-positive early-stage breast cancer. Adv Ther 2015;32:1237-47. https://doi.org/10.1007/s12325-015-0269-2.
- Atehortua NA, Issa AM. A method to measure clinical practice patterns of breast cancer genomic diagnostics in health systems. Per Med 2012;9:585-92. https://doi.org/10.2217/pme.12.72.
- Azim HA, Michels S, Bedard P, Fumagalli D, Singhal SK, Haibe-Kains B, et al. Elucidating the biological basis of prognosis in young women with early breast cancer (BC) using gene expression profiling. J Clin Oncol Conf: ASCO Annual Meeting 2011;29.
- Azim HA, Michiels S, Bedard P, Singhal SK, Haibe-Kains B, Piccart M, et al. The prognostic performance of first generation gene expression signatures (FGS) in young women with operable breast cancer (BC). Ann Oncol 2011;22.
- Baehner FL, Butler SM, Anderson JM, Ballard J, Tan V, Shak S. Consistency and control in clinical assay technology over time: the oncotype DX recurrence score and assessment of single gene expression levels. Cancer Res 2011;71. https://doi.org/10.1158/0008-5472.SABCS11-P1-07-11.
- Balassanian R, Engelberg JA, Bishop JW, Borowsky AD, Cardiff RD, Carpenter PM, et al. Harmonization of immunohistochemical stains for breast cancer biomarkers-an athena pathology collaboration. Lab Invest 2013;93.
- Barnes M, Srinivas C, Xu C, Dean S, Singh S, Henricksen LA, et al. Early stage breast cancer prognostication using whole tumor or Ki67 heterogeneity-based digital imaging. Cancer Res 2016;76. https://doi.org/10.1158/1538-7445.SABCS15-P1-01-04.
- Barry WT, Marcom PK, Geradts J, Datto MB. Retrospective evaluation of precision of gene-expression-based signatures of prognosis and tumor biology in replicate surgical biospecimens from patients with breast cancer. Cancer Res 2012;72. https://doi.org/10.1158/0008-5472.SABCS12-P4-09-01.
- Bartlett JMS, Sabine VS, Haider S, Drake C, Crozier C, Yao CQ, et al. Theranostic multiparametric tests improve residual risk assessment in early luminal breast cancer. Cancer Res 2015;75. https://doi.org/10.1158/1538-7445.SABCS14-S2-01.
- Barton S, Zabaglo L, A’Hern R, Turner N, Ferguson T, O’Neill S, et al. Assessment of the contribution of the IHC4+C score to decision making in clinical practice in early breast cancer. Br J Cancer 2012;106:1760-5. https://doi.org/10.1038/bjc.2012.166.
- Bastien RR, Rodríguez-Lescure Á, Ebbert MT, Prat A, Munárriz B, Rowe L, . PAM50 breast cancer subtyping by RT-qPCR and concordance with standard clinical molecular markers. BMC Med Genomics 2012;5. https://doi.org/10.1186/1755-8794-5-44.
- Bastien RRL, Vaughn C, Elsberry D, Stijleman IJ, Bernard PS. Clinical validation of the Prosigna breast cancer prognostic gene signature assay on formalin-fixed paraffin embedded breast cancer tumors with comparison to standard molecular markers. J Clin Oncol Conf 2014;32.
- Beamish R, McCaffrey J, Smith M, Crown J, O’Connor M, McGee SF, et al. A comparative analysis of distant recurrence risk assessments by oncotype DX recurrence score alone and integrated with clinicopathologic factors in early-stage breast cancer. J Clin Oncol Conf 2013;31.
- Beck AH, West RB, Van De Vijver M, Koller D. Computational image analysis identifies new morphologic features that predict breast cancer outcome. Lab Invest 2011;91.
- Beitsch P, Jia A, De Snoo F, Whitworth P, Patel R, Rutgers E, et al. Mammaprint 70-gene assay predicts risk of local-regional recurrence. Ann Surg Oncol 2012;1:25-6.
- Bernard PS, Davis C, Munarriz B, Stijleman IJ, Ruiz-Borrego M, Ebbert MTW, et al. Determining agreement between immunohistochemistry and RT-qPCR for standard biomarkers in breast cancer: validation on GEICAM 9906 clinical trial. J Clin Oncol Conf: ASCO Annual Meeting 2011;29.
- Bhargava R, Brufsky AM, Lembersky BC, Jankovic R, Puhalla SL, Oesterreich S, et al. Treatment decisions and five year outcomes of the oncotype DX test: an independent assessment. Cancer Res 2013;73. https://doi.org/10.1158/0008-5472.SABCS13-P6-06-46.
- Biermann J, Neusser S, Philipp L. Retrospective cost analysis of the EndoPredict test in patients with primary breast cancer in a German breast center. Abstract Senology Meeting 2016.
- Bishop JW, Engelberg J, Apple S, Balassanian R, Borowsky AD, Cardiff RD, et al. Raising the bar: breast cancer biomarkers IHC4 harmonization from University of California-Athena pathology collaboration. J Clin Oncol Conf: ASCO’s Quality Care Symposium 2012;30.
- Blohmer JU, Rezai M, Kümmel S, Kühn T, Warm M, Friedrichs K, et al. Using the 21-gene assay to guide adjuvant chemotherapy decision-making in early-stage breast cancer: a cost-effectiveness evaluation in the German setting. J Med Econ 2013;16:30-4. https://doi.org/10.3111/13696998.2012.722572.
- Boer K, Rozsa P, Horvath Z, Juhos E, Rubovszky G, Kahan Z, et al. Economic impact of oncotype DX results guided adjuvant treatments in Hungary. Breast 2013;22.
- Bonneterre J, Prat A, Galván P, Morel P, Giard S. Value of a gene signature assay in patients with early breast cancer and intermediate risk: a single institution retrospective study. Curr Med Res Opin 2016;32:835-9. https://doi.org/10.1185/03007995.2016.1146664.
- Borowsky A, Balassanian R, Yau C, Engelberg JA, Thompson CK, Retallack HEG, et al. Interobserver agreement of breast cancer IHC4 after ‘score the core’ training. Mod Pathol 2016;29.
- Borowsky A, Balassanian R, Yau C, Engelberg JA, Thompson CK, Retallack HEG, et al. Interobserver agreement of breast cancer IHC4 after ‘score the core’ training. Lab Invest 2016;96.
- Borowsky A, Thompson CK, Balassanian R, Yau C, Chen YY, Krings G, et al. Phenotype and immunophenotype analysis of gene expression defined ‘indolent risk’ breast cancers. Lab Invest 2016;96.
- Bosl A, Spitzmueller A, Haid A, Jasarevic Z, Baruschke K, Jaeger S, et al. Comparison of EndoPredict and MammaPrint in hormone receptor positive, HER2 negative breast cancer. Breast 2015;24:S46-7. https://doi.org/10.1016/S0960-9776(15)70108-6.
- Brase JC, Muller BM, Haufe F, Weber KE, Budzies J, Petry C, et al. Comparison of an RNA-based multigene test between core biopsies and corresponding surgical breast cancer sections. Ann Oncol 2012;23.
- Brownlie D, Chatterjee S, Saad Z, Wilson G, Bashir M. Impact of oncotype DX on the decision for adjuvant chemotherapy: retrospective analysis of the Salford Royal Foundation Trust cohort. Eur J Surg Oncol 2015;41. https://doi.org/10.1016/j.ejso.2015.03.210.
- Buechler S, Badve SS, Gokmen-Polar Y. INDUCT: a risk score to predict relapse in estrogen-receptor-positive breast cancer. J Clin Oncol Conf 2014;32.
- Buscariollo D, Cronin A, Bleicher RJ, Cohen A, Hassett MJ, Javid S, et al. Association between the 21-gene recurrence score and isolated local-regional recurrence in hormone receptor-positive breast cancer. Int J Radiat Oncol Biol Phys 2016;96. https://doi.org/10.1016/j.ijrobp.2016.06.627.
- Cai L, Riojas J, McDowell G, Wang Y, Sebastian J. Evaluation and clinical specimen testing experiences on prosigna breast cancer prognostic gene signature assay. J Molec Diagn 2015;17.
- Chadha M, Stewart R, Wallach J. The association of clinical-pathologic factors and oncotype DX recurrence score (RS) in the outcome of early stage breast cancer. J Clin Oncol Conf 2015;33.
- Chen YY, Tseng LM, Yang CF, Lien PJ, Hsu CY. Adjust cut point values of immunohistochemistry models to predict risk of distant recurrence in invasive breast carcinoma patients. Modern Pathology 2016;2. https://doi.org/10.1016/j.jcma.2016.06.004.
- Clough KB, Poulet B, Jamshidian F, Butler S, Svedman C, Levy E. Risk classification of early stage breast cancer as assessed by MammaPrint and oncotype DX genomic assays. Cancer Res 2012;72.
- Conlon N, Ross DS, Howard J, Catalano JP, Dickler MN, Tan LK. Is There a role for oncotype DX testing in invasive lobular carcinoma?. Breast J 2015;21:514-19. https://doi.org/10.1111/tbj.12445.
- Connell LC, Teo M, O’Reilly S, Beamish R, Moylan EJ. Does oncotype DX recurrence score correlate with survival benefit in ‘good prognosis’ patients as estimated by AdjuvantOnline!?. J Clin Oncol Conf 2011;29.
- Cotzia P, Corney D, Mollaee M, Peiper S, Palazzo J, Xuan Wang Z. Impact of tumor microenvironment on prosigna breast cancer prognostic score. Am J Clin Pathol 2015;144. https://doi.org/10.1093/ajcp/144.suppl2.279.
- Crager M, Tang G, Shak S. Using the 21-gene recurrence score and the recently developed Recurrence Score-Clinical-Pathologic to assess recurrence risk in patients with node-negative, ER-positive early-stage breast cancer receiving aromatase inhibitor treatment alone. J Clin Oncol Conf: ASCO Annual Meeting 2011;29.
- Cuallar MT, Telatar M, Louie C, Mehta V, Fong C, Chu P, et al. Validation of the prosigna breast cancer prognostic gene signature in the clinical lab. J Mol Diagn 2015;17.
- Dabbs DJ, Brufsky A, Jankowitz RC, Puhalla S, Lee A, Oesterreich S, et al. Comparison of test results and clinical outcomes of patients assessed with both MammaPrint and oncotype DX with pathologic variables: An independent study. J Clin Oncol 2014;32. https://doi.org/10.1200/jco.2014.32.15_suppl.550.
- Dakin A, Cotter M, Walshe J, Quinn C. Oncotype DX testing reduces the number of patients receiving chemotherapy for invasive breast carcinoma. Ir J Med Sci 2013;1.
- Deck KB, Sinha R, Kerlin D, Barone J, Rivera E, Garcia AA, et al. Comparison of MammaPrint and TargetPrint with clinical parameters in patients with breast cancer: findings from a prospective U.S. cohort. J Clin Oncol Conf: ASCO Annual Meeting 2011;29.
- Denduluri N, Rugo HS, Davis SE, Favret A, Hong R, Au A, et al. Concordance between the 21-gene recurrence score (RS) and the 70-gene profile (MP) in breast cancer (BC) patients (pts). J Clin Oncol Conf 2011;29.
- Dodson A, Zabaglo L, Martins V, Yeo B, Hayes D, McShane L, et al. Between-lab variability in Ki67 scoring by a standardised method in core-cuts has little impact on risk estimates by the IHC4+Clinical (IHC4+C) Score. A study presented on behalf of the International Ki67 in Breast Cancer Working Group of the Breast International Group. Eur J Cancer 2016;57:S142-3.
- Dowsett M. Pathology challenges for biology-driven trials: the Ki67 experience. Eur J Cancer 2013;49:S1-2. https://doi.org/10.1016/S0959-8049(13)70081-9.
- Dowsett M, Leung SCY, Zabaglo L, Arun I, Badve SS, Bane AL, et al. Analytical validation of a standardized scoring protocol for Ki67: phase-3 of an international multicenter collaboration. Cancer Res 2016;76. https://doi.org/10.1158/1538-7445.SABCS15-P1-01-01.
- Drak Alsibai K, Azoulay S, Languille-Mimoune O, Poulet B, Barres A, Adem C. KI67 evaluation in breast cancer: molecular pathology using digital imaging. Lab Invest 2013;93.
- Drukker CA, Elias SG, Nijenhuis MV, Wesseling J, Bartelink H, Elkhuizen P, et al. Gene expression profiling to predict the risk of locoregional recurrence in breast cancer: a pooled analysis. Breast Cancer Res Treat 2014;148:599-613. https://doi.org/10.1007/s10549-014-3188-z.
- Drukker CA, Nijenhuis MV, Elias SG, Wesseling J, Russell NS, De Snoo F, et al. Gene expression profiling to predict the risk of locoregional recurrence in breast cancer. Cancer Res 2012;72. https://doi.org/10.1158/0008-5472.SABCS12-P2-10-42.
- Ebbert MTW, Bastien RRL, Rowe LR, Miller PA, Anderson D, Boucher KM, et al. PAM50 breast cancer intrinsic classifier: clinical validation of a multianalyte laboratory developed test. J Clin Oncol Conf: ASCO Annual Meeting 2011;29.
- Ellis MJ, Chen B, Chapman JAW, Luo J, Ma D, Shepherd L, et al. CADER prognostic gene signature for disease free survival in hormone receptor positive breast cancer: NCIC CTG MA.12 phase III placebo-controlled tamoxifen trial. Cancer Res 2015;75. https://doi.org/10.1158/1538-7445.SABCS14-P4-11-11.
- Espinel CF, Keating S, Hibshoosh H, Taback B, Joseph KA, El-Tamer M, et al. MammaPrint feasibility in a large tertiary urban medical center: an initial experience. Scientifica 2012;2012. https://doi.org/10.6064/2012/942507.
- Ettl J, Grose Lackmann K, Klein E, Paepke S, Petry C, Specht K, et al. Feasibility and utility of the endopredict test in clinical routine. Breast 2013;22.
- Fitzal F, Filipits M, Fesl C, Rudas M, Dubsky PC, Bartsch R, et al. Predicting local recurrence using PAM50 in postmenopausal endocrine responsive breast cancer patients. J Clin Oncol Conf 2014;32.
- Fitzal F, Filipits M, Rudas M, Greil R, Dietze O, Samonigg H, et al. The genomic expression test EndoPredict is a prognostic tool for identifying risk of local recurrence in postmenopausal endocrine receptor-positive, HER2neu-negative breast cancer patients randomised within the prospective ABCSG 8 trial. Br J Cancer 2015;112:1405-10. https://doi.org/10.1038/bjc.2015.98.
- Fitzal F, Filipits MF, Rudas M, Greil R, Dietze O, Samonigg H, et al. Tailoring local therapy in post-menopausal endocrine responsive HER2neu negative breast cancer patients based on their genetic risk profile using Endopredict. Eur J Cancer 2014;50.
- Freitas MR, Simon SD. Comparison between oncotype DX test and standard prognostic criteria in estrogen receptor positive early-stage breast cancer. Einstein 2011;9:354-8. https://doi.org/10.1590/S1679-45082011AO2039.
- Fresno C, González GA, Merino GA, Flesia AG, Podhajcer OL, Llera AS, et al. A novel non-parametric method for uncertainty evaluation of correlation-based molecular signatures: its application on PAM50 algorithm. Bioinformatics 2017;33:693-700. https://doi.org/10.1093/bioinformatics/btw704.
- Galatenko VV, Lebedev AE, Nechaev IN, Shkurnikov MY, Tonevitskii EA, Podol’skii VE. On the construction of medical test systems using greedy algorithm and support vector machine. Bull Exp Biol Med 2014;156:706-9. https://doi.org/10.1007/s10517-014-2430-3.
- Garcia-Estevez L, Hernandez E, Acosta D, Lopez-Rios F, Prieto M, Calvo I. Effect of oncotype DX Recurrence Score (RS) on chemotherapy (CT) decision-making by providing information beyond intrinsic subtypes in both luminal A and B breast cancer (BC) patients (pts): a retrospective study in the Spanish population. J Clin Oncol Conf 2016;34.
- Garcia-Estevez L, Hernandez E, Acosta D, Lopez-Rios F, Prieto Pozuelo M, Calvo I. Use of oncotype DX Recurrence Score (RS) reduces chemotherapy (CT) beyond treatment decisions using Ki67-based determinations of luminal A and B breast cancer subtypes: a retrospective study in the Spanish population. Ann Oncol Conference: 41st European Society for Medical Oncology Congress, ESMO 2016;27.
- Gilman D, Del Rosario G, Moore TD, Renker PR, Sickle-Santanello B. Oncotype DX recurrence score correlation with histologic grade and older decision-making tools for predicting breast cancer recurrence risk. Ann Surg Oncol 2011;18.
- Gnant M, Sestak I, Filipits M, Dowsett M, Balic M, Lopez-Knowles E, et al. Identifying clinically relevant prognostic subgroups of postmenopausal women with node-positive hormone receptor-positive early-stage breast cancer treated with endocrine therapy: a combined analysis of ABCSG-8 and ATAC using the PAM50 risk of recurrence score and intrinsic subtype. Ann Oncol 2015;26:1685-91. https://doi.org/10.1093/annonc/mdv215.
- Goossens-Beumer I, Witteveen A, Delahaye L, Snel M, Zheng J, van ‘t Veer L, et al. MammaPrint in large clinical studies (MINDACT) and in diagnostics; how similar are they?. Eur J Cancer 2016;57.
- Gurard-Levin ZA, Pancaldi V, Wilson LOW, Marangoni E, Roman-Roman S, Valencia A, et al. Epigenetic profiling of chemotherapy sensitivity. Cancer Research Conference: 106th Annual Meeting of the American Association for Cancer Research, AACR 2015;75.
- Hanna MG, Bleiweiss IJ, Nayak A, Jaffer S. Correlation of oncotype DX recurrence score with histomorphology and immunohistochemistry in over 500 patients. Int J Breast Cancer 2017;2017. https://doi.org/10.1155/2017/1257078.
- Hedjazi L, Le Lann MV, Kempowsky T, Dalenc F, Aguilar-Martin J, Favre G. Symbolic data analysis to defy low signal-to-noise ratio in microarray data for breast cancer prognosis. J Comput Biol 2013;20:610-20. https://doi.org/10.1089/cmb.2012.0249.
- Hideo S, Horimoto Y, Sonoue H, Arakawa A, Stork-Sloots L, De Snoo F, et al. Application of a 70-gene expression profiling tool to Japanese breast cancer patients. Breast 2013;22.
- Hu Z, Li Y, Fan C, Weck K, Perou CM, Gulley ML. Validation of PAM50 breast cancer intrinsic subtypes using nanostring ncounter gene expression assay. J Molec Diagn 2012;14.
- Hu Z, Li Y, Treece A, Weck K, Gulley ML. Verification of the nanostring prosigna breast cancer prognostic gene signature assay in a clinical laboratory. J Molec Diagn 2014;16.
- Jegadeesh NK, Kim S, Prabhu RS, Oprea GM, Yu DS, Godette KG, et al. The 21-gene recurrence score and locoregional recurrence in breast cancer patients. Ann Surg Oncol 2015;22:1088-94. https://doi.org/10.1245/s10434-014-4252-y.
- Jiang H, Denduluri N, Majure M, Favret A, Rugo HS. Comparison of risk prediction with the 21-gene recurrence score (oncotype DX) and the 70-gene signature (MammaPrint) in patients with estrogen receptor-positive early stage breast cancer. Cancer Res 2016;76. https://doi.org/10.1158/1538-7445.SABCS15-P5-08-06.
- Kao KJ, Chang KM, Hsu HC, Huang AT. Correlation of microarray-based breast cancer molecular subtypes and clinical outcomes: implications for treatment optimization. BMC Cancer 2011;11. https://doi.org/10.1186/1471-2407-11-143.
- Karagiannis GS, Goswami S, Jones JG, Oktay MH, Condeelis JS. Selective gene-expression profiling of metastasizing breast tumor cell subpopulations complements the predictive power of Mammaprint Dx and oncotype Dx. Cancer Research Conference: 107th Annual Meeting of the American Association for Cancer Research, AACR 2016;76.
- Kelly CM, Beamish R, McCaffrey J, Smith M, Crown J, O’Connor M, et al. A comparative analysis of distant recurrence risk assessments by oncotype DX recurrence score alone and integrated with clinicopathologie factors in early-stage breast cancer. J Clin Oncol 2013;31.
- Khawaja S, Udayasankar S, Munir A, Thomas D, Huws A, Shariaha Y, et al. The long term follow up of patients undergoing oncotype Dx testing in a multicenter study in Southwest Wales, UK. Eur J Cancer 2016;57.
- Krishnamurti U, Wetherilt CS, Yang J, Peng L, Li X. Tumor-infiltrating lymphocytes are significantly associated with better overall survival and disease-free survival in triple negative but not estrogen receptor positive breast cancers. Hum Path 2017;30. https://doi.org/10.1016/j.humpath.2017.01.004.
- Kuderer NM, Barry WT, Geradts J, Ginsburg GS, Lyman GH, Datto M, et al. A cross-platform comparison of genomic signatures and oncotypeDx score to discover potential prognostic/predictive genes and pathways. Cancer Res 2012;72. https://doi.org/10.1158/0008-5472.SABCS12-P2-10-03.
- Kunz G. Use of a genomic test (MammaPrint™) in daily clinical practice to assist in risk stratification of young breast cancer patients. Arch Gynecol Obstet 2011;283:597-602. https://doi.org/10.1007/s00404-010-1454-9.
- Lakhanpal R, Sestak I, Shadbolt B, Bennett GM, Brown M, Phillips T, et al. IHC4 score plus clinical treatment score predicts locoregional recurrence in early breast cancer. Breast 2016;29:147-52. https://doi.org/10.1016/j.breast.2016.06.019.
- Lamond NW, Skedgel C, Rayson D, Lethbridge L, Younis T. Cost-utility of the 21-gene recurrence score assay in node-negative and node-positive breast cancer. Breast Cancer Res Treat 2012;133:1115-23. https://doi.org/10.1007/s10549-012-1989-5.
- Lee AV, Lin Y, Lin HM, Cooper KL, Normolle DP, Brufsy AM, et al. Intra-tumor heterogeneity affects multi-gene test prognostic risk stratification. Cancer Res 2013;73. https://doi.org/10.1158/0008-5472.SABCS13-P2-11-10.
- Lewin R, Sulkes A, Shochat T, Tsoref D, Rizel S, Liebermann N, et al. oncotype-DX recurrence score distribution in breast cancer patients with BRCA1/2 mutations. Breast Cancer Res Treat 2016;157:511-16. https://doi.org/10.1007/s10549-016-3836-6.
- Li H, Zhu Y, Burnside ES, Drukker K, Hoadley KA, Fan C, et al. MR imaging radiomics signatures for predicting the risk of breast cancer recurrence as given by research versions of MammaPrint, oncotype DX, and PAM50 gene assays. Radiology 2016;281:382-91. https://doi.org/10.1148/radiol.2016152110.
- Liang Y, Chen XS, Wu JY, Huang O, Zong Y, Mao Y, et al. Analysis of multidisciplinary team decision-making modification related factors in early breast cancer adjuvant chemotherapy. Chinese. Chinese Journal of Cancer Prevention and Treatment 2015;22:1769-73.
- Lima Pereira AA, Santini FC, Shimada AK, Nascimento EC, Katz A, Mano MS. Discordance between oncotype Dx and Saint Gallen criteria, Adjuvant!, NCCN 2011 guidelines, and initial physician treatment recommendation. J Clin Oncol Conf 2012;30.
- Lima Pereira AA, Shimada AK, Santini FC, Nascimento EC, Ribeiro KB, Marques RJ, et al. oncotype DX-the sirio-libanes hospital cancer center experience. Ann Oncol 2012;23.
- Lo SS, Mai H, McCroskey Z, Guo R, Ersahin C, Gaynor ER, et al. The 70-gene signature (70-GS) in a lymph node-negative patient series with intermediate risk 21-gene recurrence scores (RS) and known adjuvant treatment recommendations. Cancer Res 2013;73. https://doi.org/10.1158/0008-5472.SABCS13-P2-11-13.
- Lund MJ, Mosunjac M, Davis KM, Gabram-Mendola S, Rizzo M, Bumpers HL, et al. 21-gene recurrence scores: racial differences in testing, scores, treatment, and outcome. Cancer 2012;118:788-96. https://doi.org/10.1002/cncr.26180.
- Luo J, Chang LW, Tao Y, Hoog J, Leung S, Nielsen TO, et al. A copy number aberration driven endocrine response gene signature stratifies risk in estrogen receptor positive breast cancer. Cancer Res 2015;75. https://doi.org/10.1158/1538-7445.SABCS14-P5-10-02.
- Luo J, Chang LW, Van Tine BA, Tao Y, Hoog J, Giuntoli T, et al. An amplicon-driven aromatase inhibitor response (ADAIR) signature provides an orthogonal risk classifier for ER+ breast cancer. Cancer Res 2011;71. https://doi.org/10.1158/0008-5472.SABCS11-P1-06-13.
- Mai HP, Kliethermes S, McCroskey Z, Ersahin C, Gaynor ER, Robinson PA, et al. Comparison of the intermediate risk 21-gene recurrence score (RS) with the 70-gene signature (GS) as a continuous variable, Magee equations, and Adjuvant! Online (AOL). J Clin Oncol Conf 2014;32.
- Mamounas EP, Tang G, Fisher B, Paik S, Shak S, Costantino JP, et al. Association between the 21-gene recurrence score assay and risk of locoregional recurrence in node-negative, estrogen receptor-positive breast cancer: results from NSABP B-14 and NSABP B-20. J Clin Oncol 2010;28:1677-83. https://doi.org/10.1200/JCO.2009.23.7610.
- Mamounas EP, Liu Q, Paik S, Baehner FL, Tang G, Jeong JH, et al. 21-gene recurrence score and locoregional recurrence in node-positive/ER-positive breast cancer treated with chemo-endocrine therapy. J Natl Cancer Inst 2017;109. https://doi.org/10.1093/jnci/djw259.
- Mamounas EP, Tang G, Paik S, Baehner FL, Liu Q, Jeong J, et al. The 21-gene recurrence score (RS) predicts risk of loco-regional recurrence (LRR) in node (+), ER (+) breast cancer (BC) after adjuvant chemotherapy and tamoxifen: results from NSABP B- 28. Ann Surg Oncol 2013;1.
- Marcinkowski EF, Kauffman R, Ottesen R, Niland J, Yuan Y, Kruper L, et al. Acceptance of adjuvant chemotherapy in patients with early stage breast cancer. Ann Surg Oncol 2015;1.
- Markopoulos C, Xepapadakis G, Venizelos V, Tsiftsoglou A, Misitzis J, Panoussis D, et al. Clinical experience of using oncotype DX as an additional treatment decision tool in early breast cancer – a retrospective analysis from 5 Greek institutions. Eur J Surg Oncol 2012;38:413-19. https://doi.org/10.1016/j.ejso.2012.02.183.
- Maroun R, Saleh R, Asselah J, Omeroglu A, Gulbeyaz A, Meterissian SH, et al. A head-to-head comparison of mammaprint and oncotype Dx: a McGill University health center experience. J Clin Oncol Conf 2015;33.
- McVeigh TP, Hughes LM, Miller N, Sheehan M, Keane M, Sweeney KJ, et al. The impact of oncotype DX testing on breast cancer management and chemotherapy prescribing patterns in a tertiary referral centre. Eur J Cancer 2014;50:2763-70. https://doi.org/10.1016/j.ejca.2014.08.002.
- Michaud P, Mouysset J, Dohollou N, Laplaige P, Lafuma A, Fignon A. French prospective multi-center cohort on the decision impact assessment. Value Health 2016;19. https://doi.org/10.1016/j.jval.2016.03.730.
- Miller DV, Jensen TA, Covington AD, Rosenthal R, Avent JM, Hansen J, et al. Comparing recurrence risk scores derived from 4 immunohistochemistry stains (IHC4) versus oncoType DX. Cancer Res 2013;73. https://doi.org/10.1158/0008-5472.SABCS13-P6-06-34.
- Moinfar F, Luschin-Ebengreuth G, Tsybrovskyy O, Regitnig P, Mannweiler S, Ratschek M, et al. Prediction of results of MammaPrint’s 70 gene signatures by conventional histopathological and biological approaches in patients with breast cancer. Cancer Res 2011;71. https://doi.org/10.1158/0008-5472.SABCS11-P5-11-02.
- Morales-Estevez C, Moreno A, Porras I, Cano MT, Sanchez P, Fuentes H, et al. The genomic profile (GP) of early breast cancer (EBC): daily practice analysis. J Clin Oncol Conf 2016;34.
- Mullinax J, Carr D, Vera N, Sun W, Lee MC, Hoover S, et al. Distant recurrence risk with prospective use of the 21-gene assay at a single institution. J Clin Oncol Conf 2015;33.
- Muniz J, Kidwell KM, Henry NL. Metabolic syndrome and breast cancer recurrence within the 21-gene recurrence score assay. J Clin Oncol Conf 2015;33.
- Na KY, Kim KS, Lee JE, Kim HJ, Yang JH, Ahn SH, et al. The 70-gene prognostic signature for korean breast cancer patients. J Breast Cancer 2011;14:33-8. https://doi.org/10.4048/jbc.2011.14.1.33.
- Nijenhuis MV, Drukker CD, Elias SG, Wesseling J, Bartelink H, Whitworth P, et al. The 70-gene signature predicts the risk of locoregional recurrence after adequate breast surgery. Eur J Cancer 2013;49.
- Ole Eriksen J, Jensen MB, Laenkholm AV, Kiboll T, Bruun Rasmussen B, Knoop AS, et al. Validation of prediction of local recurrence (LR) by Prosigna (PAM50) in a Danish breast cancer cooperative group (DBCG) cohort of hormone receptor positive (HR+), postmenopausal early breast cancer (EBC) patients allocated to 5yr of endocrine therapy (ET). Cancer Res 2016;76. https://doi.org/10.1158/1538-7445.SABCS15-P2-08-10.
- Onoda T, Yamauchi H, Yagata H, Tsugawa K, Suzuki K, Nakamura S. Evaluating conventional pathological factors against the standardized 21-gene signature for hormone receptor positive breast cancer patients. Breast 2011;20:S46-7. https://doi.org/10.1016/S0960-9776(11)70150-3.
- Osaki A, Takeuchi H, Nakamiya N, Shigekawa T, Matsuura K, Misumi M, et al. Feasibility of Mammaprint risk assessment using vacuum-assisted breast biopsy (Mammotome) in early breast cancer. Breast 2011;20. https://doi.org/10.1016/S0960-9776(11)70148-5.
- Özmen V, Atasoy A, Gökmen E, Özdoğan M, Güler N, Uras C, et al. Correlations between oncotype DX recurrence score and classic risk factors in early breast cancer: results of a prospective multicenter study in Turkey. J Breast Health 2016;12:107-11. https://doi.org/10.5152/tjbh.2016.2874.
- Pelaez Garcia A, Yebenes L, Angulo A, Berjon A, Zamora P, Sanchez Mendez JI, et al. Comparison of risk classification between EndoPredict and Mammaprint scores in ER+/HER2–invasive breast cancer. Virchows Archiv 2016;469.
- Prendergast A, Martin K, Doolan P, Clarke C, Clynes M, Evoy D, et al. Can the Ki67 proliferation index predict the oncotype DX recurrence score in lymph node negative, ER positive breast cancer?. Cancer Res 2011;71. https://doi.org/10.1158/0008-5472.SABCS11-P5-13-12.
- Quintyne KI, Woulfe B, Gupta RK. Correlation between 21-gene recurrence score assay with Nottingham prognostic index (NPI) and Adjuvant! Online (AO) prognostic tools in newly diagnosed patients with early-stage breast cancer in midwestern Ireland. J Clin Oncol Conf 2012;30.
- Retèl VP, Joore MA, Drukker CA, Bueno-de-Mesquita JM, Knauer M, van Tinteren H, et al. Prospective cost-effectiveness analysis of genomic profiling in breast cancer. Eur J Cancer 2013;49:3773-9. https://doi.org/10.1016/j.ejca.2013.08.001.
- Rouzier R, Gentien D, Guinebretiere JM, Mouret-Reynier MA, Dubot C, Cottu PH, et al. Prospective multicenter study of the impact of the Prosigna assay on adjuvant clinical decision-making in women with early stage breast cancer: which patients are the best candidates?. J Clin Oncol Conf 2016;34.
- Schlake G, Kronenwett R, Tiecke F, Kastrup K, Bleuel N, Petry C, et al. EndoPredict-based treatment decision can reduce chemotherapy usage in ER+, HER2–breast cancer. Breast 2015;24. https://doi.org/10.1016/S0960-9776(15)70270-5.
- Sestak I, Dowsett M, Ferree S, Baehner FL, Cuzick J. Retrospective analysis of molecular scores for the prediction of distant recurrence according to baseline risk factors. Breast Cancer Res Treat 2016;159:71-8. https://doi.org/10.1007/s10549-016-3868-y.
- Shimizu H, Horimoto Y, Arakawa A, Sonoue H, Kurata M, Kosaka T, et al. Application of a 70-gene expression profile to Japanese breast cancer patients. Breast Care 2015;10:118-22. https://doi.org/10.1159/000376562.
- Shivers SC, Clark L, Esposito N, Howard N, King J, Acs G, et al. Direct comparison of risk classification between MammaPrint, oncotype DX and MammoStrat assays in patients with early stage breast cancer. Cancer Res 2013;73. https://doi.org/10.1158/0008-5472.SABCS13-P6-06-02.
- Smyth L, Watson G, Walsh EM, Kelly CM, Keane M, Kennedy MJ, et al. Economic impact of 21-gene recurrence score testing on early breast cancer in Ireland. The Breast 2015;24. https://doi.org/10.1007/s10549-015-3555-4.
- Solin LJ, Gray R, Goldstein LJ, Recht A, Baehner FL, Shak S, et al. Prognostic value of biologic subtype and the 21-gene recurrence score relative to local recurrence after breast conservation treatment with radiation for early stage breast carcinoma: results from the Eastern Cooperative Oncology Group E2197 study. Breast Cancer Res Treat 2012;134:683-92. https://doi.org/10.1007/s10549-012-2072-y.
- Sparano JA, Goldstein LJ, Davidson NE, Sledge GW, Gray R. TOP2A RNA expression and recurrence in estrogen receptor-positive breast cancer. Breast Cancer Res Treat 2012;134:751-7. https://doi.org/10.1007/s10549-012-2112-7.
- Stull TS, Goodwin MC, Anderson JM, Baehner FL, Sing AP, Yoshizawa CN, et al. Comparison of oncotype DX recurrence scores between surgical and core biopsy specimens in breast cancer patients. Cancer Res 2011;71. https://doi.org/10.1158/0008-5472.SABCS11-P3-06-05.
- Svedman C, Clough K, Poulet B, Jamshidian F, Butler SM, Cherbavaz D, et al. A comparison of risk classification as assessed by the MammaPrint and oncotype DX assays. Breast 2013;22.
- Torrisi R, Garcia-Etienne CA, Losurdo A, Morenghi E, Di Tommaso L, Gatzemeier W, et al. Potential impact of the 70-gene signature in the choice of adjuvant systemic treatment for ER positive, HER2 negative tumors: a single institution experience. Breast 2013;22:419-24. https://doi.org/10.1016/j.breast.2013.03.013.
- van den Broek AJ, Broeks A, Horlings HM, Canisius SV, Braaf LM, Langerød A, et al. Association of the germline TP53 R72P and MDM2 SNP309 variants with breast cancer survival in specific breast tumor subgroups. Breast Cancer Res Treat 2011;130:599-608. https://doi.org/10.1007/s10549-011-1615-y.
- Van Der Velden BHM, Loo CE, Gilhuijs KGA. Parenchymal enhancement of the contralateral breast in DCE-MRI and outcome of patients with early breast cancer: Complementary value of the 70-gene signature. Eur J Cancer 2016;57.
- Varga Z, Sinn P, Fritzsche F, von Hochstetter A, Noske A, Schraml P, et al. Comparison of EndoPredict and oncotype DX test results in hormone receptor positive invasive breast cancer. PLOS ONE 2013;8. https://doi.org/10.1371/journal.pone.0058483.
- Viale G, Snoo F, van ’t Veer L, Rutgers E, Piccart M, Bogaerts J, et al. Are all small tumors low risk? Characterization of small invasive node negative breast cancers (BC) enrolled in the EORTC 10041/BIG 3-04 (MINDACT) trial. Cancer Res 2015;75. https://doi.org/10.1158/1538-7445.SABCS14-P2-03-01.
- Vidal M, Peg V, Galván P, Tres A, Cortés J, Ramón y Cajal S, et al. Gene expression-based classifications of fibroadenomas and phyllodes tumours of the breast. Mol Oncol 2015;9:1081-90. https://doi.org/10.1016/j.molonc.2015.01.003.
- Wagner LI, Gray RJ, Sledge GW, Whelan TJ, Hayes DF, Geyer CE, et al. Patient-reported cognitive impairments among women with breast cancer randomly assigned to hormonal therapy (HT) alone versus chemotherapy followed by hormonal therapy (C+HT): results from the Trial Assigning Individualized Options for Treatment (TAILORx). J Clin Oncol Conf 2012;30.
- Wang X, Ring B, Hicks D, Yeh S, Woolf K, Beck R. The expression of tocopherol associated protein (TAP) is associated with recurrence and survival rates in node positive breast cancer patients. Lab Invest 2011;91:68A-9A.
- Wang X, Ring BZ, Seitz RS, Ross DT, Woolf K, Beck RA, et al. Expression of a-tocopherol-associated protein (TAP) is associated with clinical outcome in breast cancer patients. BMC Clin Pathol 2015;15. https://doi.org/10.1186/s12907-015-0021-5.
- Wexelman BA, Gallagher A, Smith BL, Isakoff SJ. The impact of the oncotype DX recurrence score pathology-clinical (RSPC) on the predicted recurrence risk for node negative breast cancer patients: a cancer center experience. J Clin Oncol Conf 2014;32.
- Woltmann A, Chen B, Lascorz J, Johansson R, Eyfjörd JE, Hamann U, et al. Systematic pathway enrichment analysis of a genome-wide association study on breast cancer survival reveals an influence of genes involved in cell adhesion and calcium signaling on the patients’ clinical outcome. PLOS ONE 2014;9. https://doi.org/10.1371/journal.pone.0098229.
- Woodward WA, Barlow WE, Jagsi R, Buchholz TA, Shak S, Baehner F, et al. The 21-gene recurrence score and locoregional recurrence rates in patients with node-positive breast cancer treated on SWOG S8814. Int J Radiat Oncol Biol Phys 2016;96:S146-S.
- Yan L, Tian L, Liu S. Combining large number of weak biomarkers based on AUC. Stat Med 2015;34:3811-30. https://doi.org/10.1002/sim.6600.
- Yao K, Turk M, Goldschmidt R, Kaul K, Stork-Sloots L, De Snoo F, et al. MammaPrint as a predictor of local-regional recurrence: findings from a United States early-stage breast cancer patient cohort. Ann Surg Oncol 2014;21.
- Yerushalmi R, Raiter A, Nalbandyan K, Hardy B. Cell surface GRP78: A potential marker of good prognosis and response to chemotherapy in breast cancer. Oncol Lett 2015;10:2149-55. https://doi.org/10.3892/ol.2015.3579.
- Zanconati F, Cusumano P, Tinterri C, Di Napoli A, Lutke Holzik M, Poulet B, et al. The 70-gene expression profile, Mammaprint, for breast cancer patients in mainly European hospitals. Breast 2011;20. https://doi.org/10.1016/S0960-9776(11)70146-1.
- Zardavas D, Fumagalli D, Borwn DN, Desmedt C, Metzger-Filho O, Piccart M, et al. Understanding the biology and prognosis of PIK3CA gene mutations in primary breast cancer using gene expression profiling: a pooled analysis. Cancer Res 2013;73. https://doi.org/10.1158/0008-5472.SABCS13-P2-11-02.
- Zhao X, Rødland EA, Tibshirani R, Plevritis S. Molecular subtyping for clinically defined breast cancer subgroups. Breast Cancer Res 2015;17. https://doi.org/10.1186/s13058-015-0520-4.
- Andre F, Delaloge S, Guinebretiere JM, Petit T, Pierga JY, Zarca D, et al. Proliferation of breast cancer and decisional biomarkers in RPC practice (RPC 2013). Oncologie 2013;15:594-606. https://doi.org/10.1007/s10269-013-2341-3.
- Anonymous . MammaPrint reduces breast cancer overtreatment. Cancer Discovery 2016;6. https://doi.org/10.1158/2159-8290.CD-NB2016-047.
- Augustovski F, Soto N, Caporale J, Gonzalez L, Gibbons L, Ciapponi A. Response to real-life decision-making impact of oncotype DX. Breast Cancer Res Treat 2015;154. https://doi.org/10.1007/s10549-015-3602-1.
- Azim HA. Has the time come for genomic tests to guide the use of adjuvant chemotherapy in node-positive breast cancer?. Ann Oncol 2015;26:1521-3. https://doi.org/10.1093/annonc/mdv248.
- Baker H. 21-gene assay identifies patients who can avoid chemotherapy. Lancet Oncol 2015;16. https://doi.org/10.1016/S1470-2045(15)00376-9.
- Bargalló-Rocha JE, Lara-Medina F, Pérez-Sánchez V, Vázquez-Romo R, Villarreal-Garza C, Martínez-Said H, et al. Cost-effectiveness of the 21-gene breast cancer assay in Mexico. Adv Ther 2015;32:239-53. https://doi.org/10.1007/s12325-015-0190-8.
- Bernhardt SM, Dasari P, Walsh D, Townsend AR, Price TJ, Ingman WV. Hormonal modulation of breast cancer gene expression: implications for intrinsic subtyping in premenopausal women. Front Oncol 2016;6. https://doi.org/10.3389/fonc.2016.00241.
- Bertelli G, Holt S. Real-life decision-making impact of oncotype DX. Breast Cancer Res Treat 2015;154:209-10. https://doi.org/10.1007/s10549-015-3600-3.
- Blank PR, Filipits M, Dubsky P, Gutzwiller F, Lux MP, Brase JC, et al. Cost-effectiveness analysis of prognostic gene expression signature-based stratification of early breast cancer patients. PharmacoEconomics 2015;33:179-90. https://doi.org/10.1007/s40273-014-0227-x.
- Bonastre J, Marguet S, Lueza B, Michiels S, Delaloge S, Saghatchian M. Cost effectiveness of molecular profiling for adjuvant decision making in patients with node-negative breast cancer. J Clin Oncol 2014;32:3513-19. https://doi.org/10.1200/JCO.2013.54.9931.
- Christgen M, Harbeck N, Gluz O, Nitz U, Kreipe HH. Recognition and handling of discordant negative human epidermal growth factor receptor 2 classification by oncotype DX in patients with breast cancer. J Clin Oncol 2012;30:3313-14. https://doi.org/10.1200/JCO.2012.42.1990.
- Dowsett M, Salter J, Zabaglo L, Mallon E, Howell A, Buzdar AU, et al. Predictive algorithms for adjuvant therapy: TransATAC. Steroids 2011;76:777-80. https://doi.org/10.1016/j.steroids.2011.02.032.
- Gerson Cwilich R, Alban de la Torre LF, Villalobos Prieto A, Serrano Olvera JA. Clinicopathological features, prognosis and influence in the adjuvant treatment of the risk recurrence groups determined by the 21 gene expression profile, oncotype Dx®, in early breast cancer. Gac Med Mex 2012;148:117-24.
- Hall PS, McCabe C, Stein RC, Cameron D. Economic evaluation of genomic test-directed chemotherapy for early-stage lymph node-positive breast cancer. J Natl Cancer Inst 2012;104:56-6. https://doi.org/10.1093/jnci/djr484.
- Katz G, Romano O, Foa C, Vataire AL, Chantelard JV, Hervé R, et al. Economic impact of gene expression profiling in patients with early-stage breast cancer in France. PLOS ONE 2015;10. https://doi.org/10.1371/journal.pone.0128880.
- Kondo M, Hoshi SL, Yamanaka T, Ishiguro H, Toi M. Economic evaluation of the 21-gene signature (oncotype DX) in lymph node-negative/positive, hormone receptor-positive early-stage breast cancer based on Japanese validation study (JBCRG-TR03). Breast Cancer Res Treat 2011;127:739-49. https://doi.org/10.1007/s10549-010-1243-y.
- Margenthaler JA, Cyr AE. Molecular profiling assays in breast cancer: beyond prime time and into syndication. Oncology 2012;26:362-4.
- Nerich V, Curtit E, Bazan F, Montcuquet P, Villanueva C, Chaigneau L, et al. Economic assessment of the routine use of oncotype DX® assay for early breast cancer in Franche-Comte region. Bull Cancer 2014;101:681-9. https://doi.org/10.1684/bdc.2014.1923.
- Nicolini A, Ferrari P, Fallahi P, Antonelli A. An iron regulatory gene signature in breast cancer: more than a prognostic genetic profile?. Future Oncol 2012;8:131-4. https://doi.org/10.2217/fon.11.148.
- Printz C. New genetic test reduces chemotherapy use in patients with e early-stage breast cancer. Cancer 2016;122:1964-5. https://doi.org/10.1002/cncr.30125.
- Raison C. NanoString® launches its first commercial diagnostic product. Expert Rev Mol Diagn 2013;13. https://doi.org/10.1586/erm.13.21.
- Ross JS. A 50-gene intrinsic subtype classifier for prognosis and prediction of benefit from adjuvant tamoxifen. Breast Diseases 2013;24:264-5. https://doi.org/10.1016/j.breastdis.2013.07.029.
- Sakata S, Cronk M. The financial burden of using oncotype Dx for patients with lymph node-negative and estrogen receptor-positive breast cancer in Australia. Asia Pac J Clin Oncol 2014;10:94-5. https://doi.org/10.1111/ajco.12077.
- Schmidt C. Mammaprint reveals who can skip chemotherapy for breast cancer. J Natl Cancer Inst 2016;108. https://doi.org/10.1093/jnci/djw197.
- Seguí MA, Crespo C, Cortés J, Lluch A, Brosa M, Becerra V, et al. In response: Genomic profile of breast cancer. Expert Rev Pharmacoecon Outcomes Res 2015;15:395-7. https://doi.org/10.1586/14737167.2015.1025760.
- Sparano JA. A 21-gene expression assay in breast cancer. N Engl J Med 2016;374. https://doi.org/10.1056/NEJMc1515988.
- Storz-Pfennig P, Wolf K. Biomarkers in early breast cancer and beyond: who needs all those tests?. Lancet Oncol 2014;15:919-20. https://doi.org/10.1016/S1470-2045(14)70270-0.
- Teig B. Genomic signature in breast cancer: oncotype DX(®). Ann Pathol 2013;33:225-8. https://doi.org/10.1016/j.annpat.2013.04.001.
- Thewes B, Prins J, Friedlander M. 70-gene signature in early-stage breast cancer. N Engl J Med 2016;375:2199-200. https://doi.org/10.1056/NEJMc1612048.
- van ’t Veer LJ, Rutgers EJT, Piccart M. 70-gene signature in early-stage breast cancer. N Engl J Med 2016;375:2200-1. https://doi.org/10.1056/NEJMc1612048.
- Vignatelli L, Negro A, Giovannini T, Pirini G, Trimaglio F, Ballini L. In vitro test for the evaluation of individual risk of metastasis in surgically treated women for breast cancer (structured abstract). Health Technol Assess Database 2011.
- Webb E. Biomarker index that may accurately identify women at long-term risk of breast cancer relapse. Women’s Health 2013;9.
- Yamauchi H, Nakagawa C, Yamashige S, Takei H, Yagata H, Yoshida A, et al. Societal cost-effectiveness analysis of the 21-gene assay in estrogen-receptor-positive, lymph-node-negative early-stage breast cancer in Japan. BMC Health Serv Res 2014;14. https://doi.org/10.1186/1472-6963-14-372.
- Pathmanathan N, Balleine RL. Ki67 and proliferation in breast cancer. J Clin Pathol 2013;66:512-16. https://doi.org/10.1136/jclinpath-2012-201085.
- Bidard FC, Peeters DJ, Fehm T, Nolé F, Gisbert-Criado R, Mavroudis D, et al. Clinical validity of circulating tumour cells in patients with metastatic breast cancer: a pooled analysis of individual patient data. Lancet Oncol 2014;15:406-14. https://doi.org/10.1016/S1470-2045(14)70069-5.
- Albanell J, Colomer R, Ruiz-Borrego M, Alba E, Garcia Saenz JA, Martin M, et al. Prospective trans-GEICAM study of the impact of the 21-gene recurrence score assay and traditional clinico-pathological factors on clinical decision making in women with estrogen receptor-positive, HER2-negative, node-negative breast cancer. Breast 2011;20. https://doi.org/10.1016/S0960-9776(11)70139-4.
- Albanell J, Gligorov J, Holt SD, Blohmer J, Eiermann W, Svedman C. Pooled analysis of 4 European studies assessing the impact of oncotype DX on treatment decisions. Breast 2013;22.
- Alvarado M, Prasad C, Rothney M, Cherbavaz D, Sing AP, Baehner F, et al. A laboratory comparison of the 21-gene assay and PAM50-ROR. Breast 2015;24:S110-11. https://doi.org/10.1016/S0960-9776(15)70279-1.
- Alvarado M, Prasad C, Rothney M, Cherbavaz DB, Sing AP, Baehner FL, et al. A laboratory comparison of the 21-gene assay and PAM50-ROR. Ann Surg Oncol 2015;1. https://doi.org/10.1016/S0960-9776(15)70279-1.
- Armstrong A, Howell S, Wardley A, Wilson G, Loncaster J, Welch R, et al. Experience with the oncotype DX assay in a UK centre. Eur J Cancer 2014;50.
- Bailey H, Alvarado M, Baehner F, Prasad C, Rothney M, Chervabaz D, et al. A laboratory comparison of the 21-gene assay and PAM50-ror. Lab Invest 2015;95.
- Barcenas CH, Sinha AK, Raghavendra AS, Syed MP, Hsu L, Patangan M, et al. Outcomes after chemotherapy in early-stage breast cancer (EBC) patients who underwent a 21-gene expression assay. J Clin Oncol Conf 2016;34.
- Bargalló-Rocha JE, Lara F, Shaw Dulin RJ, Perez-Sanchez V, Villarreal-Garza C, Maldonado-Martinez H, et al. A study of the impact of the 21-gene breast cancer assay on the use of adjuvant chemotherapy in women with breast cancer in a Mexican public hospital. Ann Oncol 2012;23:ix107-8.
- Bender RA, Knauer M, Rutgers EJ, Glas AM, de Snoo FA, Linn SC, et al. The 70-gene profile and chemotherapy benefit in 1,600 breast cancer patients. J Clin Oncol 2009;27.
- Blohmer JU, Kuhn T, Rezai M, Kummel S, Warm M, Eggemann H, et al. German multicentre decision impact study of oncotype DX recurrence score (RS) on adjuvant treatment in estrogen receptor positive (ER+) node negative (N0) and node positive (N+) early breast cancer. Breast 2011;20. https://doi.org/10.1016/S0960-9776(11)70147-3.
- Blohmer JU, Rezai M, Kummel S, Kuhn T, Warm M, Friedrichs K, et al. Impact of the oncotype DX recurrence score assay ontherapy recommendations for ER-positive (ER+), node negative (N0) and node positive (N+) early breast cancer – results of an interim analysis of the German decision impact study. Onkologie 2011;34:119-20.
- Bonneterre J, Prat A, Galvan P, Giard S. Is PROSIGNA useful to determine adjuvant treatment in intermediate prognosis early breast cancer (EBC)?. Eur J Cancer 2015;51. https://doi.org/10.1016/S0959-8049(16)30889-9.
- Braybrooke J, Kuchel A, Robinson T, Comins C, Shere M, Varughese M, et al. A prospective multi-centre study of the impact of oncotype DX on adjuvant treatment decisions in patients in the UK with estrogen receptor positive early breast cancer. Eur J Cancer 2013;49:S466-7.
- Bundred N, Armstrong A, Howell S, Wardley A, Wilson G, Loncaster J, et al. Experience with the oncotype DX assay in a UK centre. Eur J Surg Oncol 2014;40:606-7. https://doi.org/10.1016/j.ejso.2014.02.211.
- Cheng WY, Ou Yang TH, Maurer M, Anastassiou D. BCAM (breast cancer attractor metagenes): a new tool for assessing breast cancer prognosis. Cancer Research Conference: 105th Annual Meeting of the American Association for Cancer Research, AACR 2014;74.
- Cusumano G, Generali D, Lifrange E, Jerusalem G, Andreis D, Hilderink-Koertshuis R, et al. Inter-institutional comparison of impact of Mammaprint on the routine treatment decision-making process in early breast cancer patient populations from three European hospitals. Breast 2011;20. https://doi.org/10.1016/S0960-9776(11)70144-8.
- Cuzick J, Sestak I, Ferree S, Cowens JW, Dowsett M. Prediction of late breast cancer recurrence by the ROR (PAM50) score in postmenopausal women in the TransATAC cohort. Ann Oncol 2012;23:ix75-6.
- Dalton L. Invasive breast cancer: stratification of histological grade by gene-based assays: a still relevant example from an older data set. Histopathology 2014;65:429-33. https://doi.org/10.1111/his.12423.
- Davidson JA, Cromwell I, Ellard SL, Lohrisch C, Gelmon KA, Shenkier T, et al. A prospective clinical utility and pharmacoeconomic study of the impact of the 21-gene recurrence score® assay in estrogen receptor positive node negative breast cancer. Eur J Cancer 2013;49:2469-75.
- De Boer RH, Baker C, Speakman D, Mann B. Australian decision impact study: the impact of oncotype DX recurrence score (RS) on adjuvant treatment decisions in hormone receptor positive (HR+), node negative (N0) and node positive (N+) early stage breast cancer (ESBC) in the multidisciplinary clinic (MDC). Cancer Res 2011;71.
- Deck K, Kerlin D, Barone JL, Rivera E, Garcia AA, Sinha R. Comparison of the mammaprint 70-gene expression profile with clinical parameters in patients with breast cancer: Findings from a United States cohort. Ann Surg Oncol 2011;18.
- Dodson A, Zabaglo L, Yeo B, Miller K, Smith I, Dowsett M. Risk of recurrence estimates with IHC4 are tolerant of variations in staining and scoring. Cancer Res 2015;75. https://doi.org/10.1158/1538-7445.SABCS14-P5-10-06.
- Dowsett M, Lopez-Knowles E, Sidhu K, Pineda S, Cowens JW, Ferree S, et al. Comparison of PAM50 Risk of Recurrence (ROR) score with oncotypedx and IHC4 for predicting residual risk of RFS and distant-(D)RFS after endocrine therapy: a TransATAC study. Cancer Res 2011;71. https://doi.org/10.1158/0008-5472.SABCS11-S4-5.
- Dowsett M, Sestak I, Buus R, Kronenwett R, Denkert C, Krappmann K, et al. EndoPredict (EPclin) score for estimating residual distant recurrence (DR) risk in ER+/HER2– breast cancer (br ca) patients treated with 5 years adjuvant endocrine therapy alone: validation and comparison with the oncotype DX recurrence score (RS). Cancer Res 2016;76. https://doi.org/10.1158/1538-7445.SABCS15-S3-01.
- Drukker C, Van Den Hout HC, Sonke GS, Linn SC, Schmidt MK. Risk estimations and treatment decisions in early stage breast cancer; agreement among oncologists and the impact of the 70-gene signature. Eur J Cancer 2013;49.
- Drukker CA, Bueno-De-Mesquita JM, Retèl VP, Van Harten WH, Van Tinteren H, Wesseling J, et al. Comparing the 70-gene signature to the Dutch Breast Cancer guidelines in the prospective RASTER study. Eur J Cancer 2013;49:S402-3.
- Drukker CA, Nijenhuis MV, Bueno-De-Mesquita JM, Retèl VP, Van Tinteren H, Schmidt MK, et al. Optimized prediction of clinical outcome by the PREDICT plus tool and the 70-gene signature in early stage node-negative breast cancer. Cancer Res 2013;73. https://doi.org/10.1158/0008-5472.SABCS13-P6-06-13.
- Dubsky P, Brase JC, Fisch K, Jakesz R, Singer CF, Greil R, et al. The EndoPredict score identifies late distant metastases in ER+/HER2–breast cancer patients. Cancer Res 2012;72. https://doi.org/10.1158/0008-5472.SABCS12-S4-3.
- Eiermann W, Rezai M, Kummel S, Kuhn T, Warm M, Friedrichs K, et al. Using the 21-gene breast cancer assay in adjuvant decision-making in ER-positive (ER+) early breast cancer (EBC) is cost-effective: results of a large prospective German multicenter study. Eur J Cancer 2012;48. https://doi.org/10.1016/S0959-8049(12)70368-4.
- Ettl J, Lackmann KG, Hapfelmeier A, Klein E, Paepke S, Petry C, et al. Prospective comparison of uPA/PAI-1 and EndoPredict-clin score in ER-positive, HER2-negative breast cancer: impact on risk stratification and treatment decisions. J Clin Oncol Conf 2013;31.
- Filipits M, Dubsky PC, Rudas M, Brase JC, Kronenwett R, Weber KE, et al. Impact of the EndoPredict-clin score on risk stratification in ER-positive, HER2-negative breast cancer after considering clinical guidelines. J Clin Oncol Conf 2012;30.
- Fried G, Moskovitz M. Retrospective analysis of treatment decisions in patients with intermediate recurrence score results. Breast 2013;22:S84-5.
- Gligorov J, Pivot XB, Naman HL, Jacot W, Spaeth D, Misset JL, et al. Prospective study of the impact of using the 21-gene recurrence score assay on clinical decision making in women with estrogen receptor-positive, HER2-negative, early-stage breast cancer in France. J Clin Oncol Conf 2012;30.
- Gluz O, Nitz U, Kreipe HH, Christgen M, Kates RE, Hofmann D, et al. Clinical impact of risk classification by central/local grade or luminal-like subtype vs. oncotype DX: first prospective survival results from the WSG phase III planB trial. Eur J Cancer 2015;51. https://doi.org/10.1016/S0959-8049(16)30886-3.
- Gnant M, Dowsett M, Filipits M, Lopez-Knowles E, Greil R, Balic M, et al. Identifying clinically relevant prognostic subgroups in node-positive postmenopausal HR+ early breast cancer patients treated with endocrine therapy: a combined analysis of 2,485 patients from ABCSG-8 and ATAC using the PAM50 risk of recurrence (ROR) score and intrinsic subtype. J Clin Oncol Conf 2013;31.
- Gnant M, Filipits M, Dubsky P, Rudas M, Balic M, Greil R, et al. Pr predicting risk for late metastasis: the PAM50 risk of recurrence (ROR) score after 5 years of endocrine therapy in postmenopausal women with HR+ early breast cancer: a study on 1,478 patients from the ABCSG-8 trial. Ann Oncol 2013;24. https://doi.org/10.1093/annonc/mdt084.1.
- Gnant M, Filipits M, Mlineritsch B, Dubsky P, Jakesz R, Kwasny W, et al. Clinical validation of the PAM50 risk of recurrence (ROR) score for predicting residual risk of distant-recurrence (DR) after endocrine therapy in postmenopausal women with ER+ early breast cancer (EBC): an ABCSG study. Cancer Res 2012;72. https://doi.org/10.1158/0008-5472.SABCS12-P2-10-02.
- Gong C, Tan W, Chen K, You N, Zhu S, Liang G, et al. Prognostic value of a BCSC-associated MicroRNA signature in hormone receptor-positive HER2-negative breast cancer. EBioMedicine 2016;11:199-20. https://doi.org/10.1016/j.ebiom.2016.08.016.
- Halpern N, Sonnenblick A, Uziely B, Divinsky L, Goldberg Y, Hamburger T, et al. Oncotype Dx recurrence score among BRCA1/2 germline mutation carriers with hormone receptors positive breast cancer. Int J Cancer 2017;140:2145-9. https://doi.org/10.1002/ijc.30616.
- Hannouf MB, Xie B, Brackstone M, Zaric GS. Cost-effectiveness of a 21-gene recurrence score assay versus Canadian clinical practice in women with early-stage estrogen- or progesterone-receptor-positive, axillary lymph-node negative breast cancer. BMC Cancer 2012;12. https://doi.org/10.1186/1471-2407-12-447.
- Hannouf MB, Xie B, Brackstone M, Zaric GS. Cost effectiveness of a 21-gene recurrence score assay versus Canadian clinical practice in post-menopausal women with early-stage estrogen or progesterone-receptor-positive, axillary lymph-node positive breast cancer. PharmacoEconomics 2014;32:135-47. https://doi.org/10.1007/s40273-013-0115-9.
- Hartmann S, Gerber B, Elling D, Doell D, Reimer T. The 70-gene Mammaprint signature is an independent prognostic factor for elderly women with hormone receptor-positive, HER2-negative breast cancer. Breast 2011;20:S43-4. https://doi.org/10.1016/S0960-9776(11)70140-0.
- Holt SDH, Bertelli G, Pudney D, Rolles M, Moe M, Brinkworth E, et al. Results from a prospective clinical study on the impact of oncotype DX on adjuvant treatment decision and risk classification by Nottingham prognostic index (NPI) and recurrence score (RS). Breast 2011;20. https://doi.org/10.1016/S0960-9776(11)70137-0.
- Jaafar H, Taher A, Quasmeh K, Jaloudi M. Impact of the oncotype DX assay on decision-making in estrogen receptor positive early breast cancer. Breast 2013;22.
- Jegadeesh N, Prabhu RS, Oprea G, Godette K, Mister D, Torres M. The 21-gene recurrence score predicts local recurrence in breast cancer patients treated with mastectomy alone but not in patients treated with radiation. Int J Radiat Oncol Biol Phys 2013;1. https://doi.org/10.1016/j.ijrobp.2013.06.261.
- Jones J, Xue X, Lin H, Oktay M, Robinson B, Gertler F, et al. Tumor microenvironment of metastasis: an imaging based marker of risk for distant metastasis of breast cancer. Eur J Cancer 2013;49. https://doi.org/10.1016/S0959-8049(13)70134-5.
- Jones J, Xue X, Lin HM, D’Alfonso T, Ginter P, Oktay M, et al. Tumor MicroEnvironment of Metastasis (TMEM) is a novel approach to the assessment of metastatic risk in breast cancer. Lab Invest 2014;94.
- Knauer M. Genomic profiling in breast cancer. Eur J Surg Oncol 2012;38. https://doi.org/10.1016/j.ejso.2012.06.078.
- Krystel-Whittemore M, Brogi E, Bowser ZL, Dickler M, Hudis C, Wen HY. Distant metastases in breast cancer patients with oncotype Dx recurrence score lower than 18. Lab Invest 2016;96.
- Krystel-Whittemore M, Brogi E, Bowser ZL, Dickler M, Hudis C, Wen HY. Distant metastases in breast cancer patients with oncotype dx recurrence score lower than 18. Mod Pathol 2016;29:52A-A.
- Kuijer A, Straver M, Elias SG, Smorenburg C, Van Bommel ACM, Linn S, et al. Impact of the 70-gene signature on adjuvant systemic therapy decisions in Dutch early breast cancer patients: preliminary results of a prospective multicentre observational study. Eur J Cancer 2016;57:S90-1.
- Kuijer A, Straver M, Elias SG, Smorenburg C, Wesseling J, Linn SC, et al. Impact of the 70-gene signature on adjuvant systemic therapy decisions in early breast cancer patients: preliminary results of a prospective multicenter observational study. Ann Surg Oncol 2016;1:S50-1.
- Kummel S, Eiermann W, Rezai M, Kuhn T, Warm M, Friedrichs K, et al. The oncotype DX Recurrence Score Assay impacts adjuvant therapy recommendations for ER-positive (ER+), node negative (N0) and node positive (N+) early breast cancer-final results of the German decision impact study. J Cancer Res Clin Oncol 2012;138:24-5.
- Le Du F, Theriault RL, Willey JS, Valero V, Hortobagyi GN, Gonzalez-Angulo AM, et al. Impact of 21-gene RT-PCR assay on adjuvant therapy for stage I breast cancer. J Clin Oncol Conf 2014;32.
- Levine MN, Cochrane BL, Julian JA, Trudeau ME, Eisen A, Bedard PL, et al. Population-based evaluation of 21-gene assay in treatment decision making for early breast cancer in Ontario. J Clin Oncol Conf 2014;32.
- Linn SC, Drukker CA, Retèl VP, Bueno-de-Mesquita JM, Van Harten WH, Van Tinteren H, et al. 70-gene signature prospectively predicts prognosis of patients with node-negative breast cancer: 5 year follow-up of the raster study. Eur J Cancer 2012;48. https://doi.org/10.1016/S0959-8049(12)70275-7.
- Liu MC, Pitcher BN, Mardis ER, Davies SR, Snider JE, Vickery T, et al. PAM50 gene signature is prognostic for breast cancer patients treated with adjuvant anthracycline and taxane based chemotherapy. Cancer Res 2012;72. https://doi.org/10.1158/0008-5472.SABCS12-P2-10-01.
- Losk K, Vaz Duarte Luis I, Camuso K, Lloyd M, Kadish S, Hirshfield-Bartek J, et al. Factors associated with delays in chemotherapy initiation among patients with breast cancer. Cancer Res 2016;76.
- Mamounas E, Wolmark N, Baehner FL, Butler SM, Tang G, Jamshidian F, et al. Predicting late distant recurrence risk in ER+ breast cancer after five years of tamoxifen. Breast 2015;24. https://doi.org/10.1016/S0960-9776(15)70296-1.
- Mamounas EP, Tang G, Paik S, Baehner FL, Liu Q, Jeong JH, et al. Association between the 21-gene recurrence score (RS) and benefit from adjuvant paclitaxel (Pac) in node-positive (N+), ER+ breast cancer patients (pts): results from NSABP B-28. Cancer Res 2012;72. https://doi.org/10.1158/0008-5472.SABCS12-S1-10.
- Marchionni L, Afsari B, Geman D, Leek JT. A simple and reproducible breast cancer prognostic test. BMC Genomics 2013;14. https://doi.org/10.1186/1471-2164-14-336.
- Martin M, Brase JC, Perou CM, Ruiz A, Prat A, Weber KE, et al. Comparison of PAM50 risk of recurrence (ROR) scores with EndoPredict for predicting risk of distant metastasis in ER+/HER2–, early node-positive breast cancer patients treated with adjuvant chemotherapy-A GEICAM/ 9906 sub-study. Cancer Res 2013;73. https://doi.org/10.1158/0008-5472.SABCS13-P2-11-06.
- Martin M, Brase JC, Ruiz-Borrego M, Krappmann K, Munarriz B, Fisch K, et al. Prognostic performance of the EndoPredict score in node-positive chemotherapy-treated ER+/HER2–breast cancer patients: results from the GEICAM/9906 trial. Cancer Res 2012;72. https://doi.org/10.1158/0008-5472.SABCS12-P2-10-11.
- Miller DP, Roberts M, Petkov VI, Shak S, Howlader N, Cronin K, et al. Breast cancer-specific survival in > 4,600 patients with lymph node-positive (LN+) hormone receptor-positive (HR+) invasive breast cancer (BC) and 21-gene recurrence score (RS) results in the SEER registries. Ann Oncol Conference: 41st European Society for Medical Oncology Congress, ESMO 2016;27.
- Mittempergher L, De Ronde JJ, Nieuwland M, Kerkhoven RM, Simon I, Wessels LFA, et al. Microarray gene expression analysis on formalin-fixed, paraffin embedded material is feasible and the resulting profiles are comparable to profiles from fresh frozen matched material. Cancer Research Conference: 102nd Annual Meeting of the American Association for Cancer Research, AACR 2011;71.
- Nagarajan R, Upreti M. An approach for deciphering patient-specific variations with application to breast cancer molecular expression profiles. J Biomed Inform 2016;63:120-30. https://doi.org/10.1016/j.jbi.2016.07.022.
- Nielsen T, McDonald S, Kulkarni S, Storhoff J, Schaper C, Wallden B, et al. Analytical reproducibility of the breast cancer intrinsic subtyping test and ncounter analysis system using formalin-fixed paraffin-embedded (FFPE) breast tumor specimens. Lab Invest 2013;93.
- Nitz U, Gluz O, Kates RE, Hofmann D, Kreipe HH, Christgen M, et al. Prognostic impact of discordance between different risk assessment tools in early breast cancer (recurrence score, central grade, Ki67): early outcome analysis from the prospective phase III WSG-PlanB trial. Cancer Res 2015;75. https://doi.org/10.1158/1538-7445.SABCS14-P4-11-01.
- Nitz U, Gluz O, Kreipe H, Christgen M, Kates RE, Clemens M, et al. Risk assessment by St.Gallen 2013 recommendation and oncotype DX: results from the WSG PlanB trial. Breast 2015;24. https://doi.org/10.1016/S0960-9776(15)70278-X.
- Ozmen V, Atasoy A, Gokmen E, Ozdogan M, Guler EN, Uras C, et al. Results of the Turkish prospective multi-center study utilizing the 21-gene oncotype DX assay: decision impact analysis. J Clin Oncol Conf 2015;33.
- Park MM, Ebel JJ, Zynger DL. ER and PR immunohistochemistry and HER2 FISH vs. oncotype DX: implications for breast cancer treatment. Lab Invest 2013;93.
- Perez E, Butler S, Dueck A, Baehner F, Jamshidian F, Cherbavaz D, et al. The relationship between quantitative HER2 gene expression by the 21-gene RT-PCR assay and adjuvant trastuzumab (H) benefit in NCCTG (alliance) N9831. Lab Invest 2015;95.
- Perez EA, Butler SM, Dueck AC, Baehner FL, Jamshidian F, Cherbavaz DB, et al. The relationship between quantitative HER2 gene expression by the 21-gene RT-PCR assay and adjuvant trastuzumab (H) benefit in NCCTG (Alliance) N9831. J Clin Oncol Conf 2013;31.
- Piccart M, Rutgers E, van ’t Veer L, Slaets L, Delaloge S, Viale G, et al. Primary analysis of the EORTC 10041/ BIG 3-04 MINDACT study: a prospective, randomized study evaluating the clinical utility of the 70-gene signature (MammaPrint) combined with common clinical-pathological criteria for selection of patients for adjuvant chemotherapy in breast cancer with 0 to 3 positive nodes. Cancer Research Conference: 107th Annual Meeting of the American Association for Cancer Research, AACR 2016;76.
- Prasad C, Rothney M, Cherbavaz DB, Sing AP, Baehner FL, Svedman C, et al. A pilot laboratory study comparing the 21-gene assay and PAM50-ROR. J Clin Oncol Conf 2014;32.
- Prat A, Cheang MC, Martin M, Carrasco E, Caballero R, Tyldesley S, et al. Prognostic significance of progesterone receptor-positive tumor cells within immunohistochemically-defined luminal a breast cancer. Ann Oncol 2012;23:ii17-18.
- Prat A, Galvan P, Buckingham W, Vidal M, Diaz S, Nuciforo P, et al. Feasibility of the PROSIGNA multigene test in core biopsies and comparison to corresponding surgical breast cancer sections. Cancer Res 2015;75. https://doi.org/10.1158/1538-7445.SABCS14-P6-01-06.
- Prat A, Parker JS, Fan C, Cheang MCU, Miller LD, Bergh J, et al. Concordance among gene-expression-based predictors for ER-positive breast cancer treated with adjuvant tamoxifen. J Clin Oncol Conf: ASCO Annual Meeting 2011;29.
- Rezai M, Eiermann W, Kummel S, Kuhn T, Warm M, Friedrichs K, et al. Impact of the recurrence score on adjuvant decision-making in ER-positive early breast cancer – results of a large prospective multicentre decision impact study in node negative and node positive disease. Cancer Res 2011;71. https://doi.org/10.1158/0008-5472.SABCS11-P2-12-26.
- Saghatchian M, Mittempergher L, Michiels S, Casinius S, Glas A, Lazar V, et al. Characterization of breast cancer distant metastasis based on outcome over time using a gene expression profiling approach and identification of pathway activities of late relapse. Cancer Res 2011;71. https://doi.org/10.1158/0008-5472.SABCS11-S1-6.
- Saghatchian M, Mittempergher L, Michiels S, Wolf D, Canisius SV, Dessen P, et al. Microarray anlyses of breast cancers identify CH25H, a cholesterol gene, as a potential marker and target for late metastatic reccurences. Cancer Res 2012;72. https://doi.org/10.1158/0008-5472.SABCS12-P4-09-05.
- Saghatchian M, Mook S, Pruneri G, Viale G, Glas A, Eekhout I, et al. Combining genomic profiling (70 Gene-Mammaprint) with nodal status allows to classify patients with primary breast cancer and positive lymph nodes (1-9) into very distinct prognostic subgroups that could help tailor treatment strategies. Cancer Res 2009;69:506S-S.
- Saito M, Shimizu H, Miura H, Nakai K, Kosaka T, Senuma K, et al. Discordance of prognostic risk between histopathology and gene signature in Japanese early breast cancer. Breast 2011;20. https://doi.org/10.1016/S0960-9776(11)70151-5.
- Sestak I, Cuzick J, Dowsett M, Filipits M, Dubsky P, Cowens W, et al. Prediction of late distant recurrence after 5 years of endocrine treatment: a combined analysis of 2485 patients from the ABCSG-8 and transATAC studies using the PAM50 risk of recurrence (ROR) score. Cancer Res 2013;73. https://doi.org/10.1158/0008-5472.SABCS13-S6-04.
- Sestak I, Cuzick J, Dowsett M, Salter J, Quinn E, Zabaglo L, et al. Prediction of residual risk of recurrence after 5 years of follow-up by clinicopathologic variables and 4 IHC markers: a TransATAC study. Cancer Res 2011;71. https://doi.org/10.1158/0008-5472.SABCS11-P2-12-09.
- Sestak I, Dowsett M, Ferree S, Baehner FL, Cowens JW, Butler S, et al. Analysis of molecular scores for the prediction of distant recurrence according to body mass index and age at baseline. Ann Oncol 2014;25. https://doi.org/10.1093/annonc/mdu066.1.
- Sestak I, Dowsett M, Sgroi D, Erlander M, Ferree S, Cowens JW, et al. Pr comparison of five different scores for the prediction of late recurrence for oestrogen receptor-positive breast cancer. Ann Oncol 2013;24. https://doi.org/10.1093/annonc/mdt084.2.
- Sestak I, Zhang Y, Schnabel CA, Schroeder B, Erlander M, Goss PE, et al. Clinical impact of differential risk stratification by breast cancer index (BCI) versus recurrence score (RS) in HR+ early-stage breast cancer: a TransATAC study. J Clin Oncol Conf 2014;32.
- Sgroi DC, Sestak I, Cuzick J, Zhang Y, Schnabel CA, Erlander MG, et al. Comparative performance of breast cancer index (BCI) vs. oncotype Dx and IHC4 in the prediction of late recurrence in hormonal receptor-positive lymph node-negative breast cancer patients: a TransATAC Study. Cancer Res 2012;72. https://doi.org/10.1158/0008-5472.SABCS12-S1-9.
- Shak S, Miller DP, Howlader N, Gliner N, Howe W, Schussler N, et al. Outcome disparities by age and 21-gene recurrence score (RS) result in hormone receptor positive (HR+) breast cancer (BC). Ann Oncol Conference: 41st European Society for Medical Oncology Congress, ESMO 2016;27.
- Shak S, Petkov V, Miller DP, Howlader N, Gliner N, Howe W, et al. Breast cancer specific mortality in patients with early-stage hormone receptor-positive invasive breast cancer and oncotype DX recurrence score results in the SEER database. J Clin Oncol Conf: ASCO’s Quality Care Symposium 2016;34.
- Shak S, Petkov VI, Miller DP, Howlader N, Gliner N, Howe W, et al. Breast cancer specific survival in 38,568 patients with node negative hormone receptor positive invasive breast cancer and oncotype DX recurrence score results in the SEER database. Cancer Res 2016;76. https://doi.org/10.1158/1538-7445.SABCS15-P5-15-01.
- Sinn BV, Muller BM, Keil E, Lehmann A, Richter-Ehrenstein C, Prinzler J, et al. A novel multigene assay in clinical practice-performance and impact on clinical decisions. Ann Oncol 2013;24:iii33-4. https://doi.org/10.1093/annonc/mdt084.17.
- Sparano J, Gray R, Zujewski JA, Makower D, Pritchard K, Albain K, et al. Prospective trial of endocrine therapy alone in patients with estrogen-receptor positive, HER2-negative, node-negative breast cancer: results of the TAILORx low risk registry. Eur J Cancer 2015;51. https://doi.org/10.1016/S0959-8049(16)31935-9.
- Sparano JA, Goldstein LJ, Davidson NE, Sledge GW, Gray R. Topoisomerase 2 alpha (TOP2A) RNA expression provides prognostic information in hormone receptor positive breast cancer that is complementary to a simulated algorithm for recurrence score. Cancer Res 2011;71. https://doi.org/10.1158/0008-5472.SABCS11-P2-12-13.
- Sparano JA, Gray RJ, Makower DF, Pritchard KI, Albain KS, Hayes DF, et al. Prospective trial of endocrine therapy alone in patients with estrogen receptor positive, HER2-negative, node-negative breast cancer: results of the TAILORx low risk registry. Cancer Res 2016;76. https://doi.org/10.1158/1538-7445.SABCS15-P2-08-01.
- Sparano JA, Rohan TE, Xue X, Lin HM, D’Alfonso TM, Ginter PS, et al. A tumor microenvironment of metastasis (TMEM) biomarker in early breast cancer provides prognostic information that is complementary to IHC4: a prospective validation study. Cancer Res 2013;73. https://doi.org/10.1158/0008-5472.SABCS13-P2-11-03.
- Stalhammar G, Rosin G, Kis L, Lippert M, Moelholm I, Grunkin M, et al. Digital image analysis outperforms manual scoring for breast cancer subclassification and prognostication. Cancer Res 2016;76. https://doi.org/10.1158/1538-7445.SABCS15-P1-01-06.
- Stein R, Makris A, Hughes-Davies L, Macpherson I, Marshall A, Campbell A, et al. OPTIMA: a prospective randomised trial to validate the predictive utility and cost-effectiveness of gene expression test directed chemotherapy decisions. Eur J Surg Oncol 2016;42. https://doi.org/10.1016/j.ejso.2016.07.057.
- Stein RC, Makris A, Hughes-Davies L, Bartlett JMS, Marshall A, Hall PS, et al. Results of the OPTIMA (Optimal Personalized Treatment of early breast cancer usIng Multi-parameter Analysis) prelim study. Eur J Cancer 2015;51. https://doi.org/10.1016/S0959-8049(16)30762-6.
- Stemmer S, Steiner M, Rizel S, Ben-Baruch N, Soussan-Gutman L, Rosengarten O, et al. First prospective outcome data in 930 patients with more than 5 year median follow up in whom treatment decisions in clinical practice have been made incorporating the 21-gene recurrence score. Eur J Cancer 2015;51. https://doi.org/10.1016/S0959-8049(16)30911-X.
- Stephen J, Murray G, Cameron D, Thomas J, Kunkler I, Jack W, et al. Time dependence of biomarkers: non-proportional effects of immunohistochemical panels predicting relapse risk in early breast cancer. Cancer Res 2015;75. https://doi.org/10.1158/1538-7445.SABCS14-P4-11-10.
- Stephen J, Murray G, Cameron D, Thomas J, Kunkler I, Jack W, et al. Comparison of immunohistochemical residual risk panels to predict risk in early breast cancers treated with endocrine therapy. Cancer Res 2015;75. https://doi.org/10.1158/1538-7445.SABCS14-P4-11-09.
- Stork-Sloots L, Generali D, Ciruelos E, Manso L, Ghanem I, Lifrange E, et al. European inter-institutional impact study of MammaPrint. Breast 2013;22:S62-3.
- Tobin NP, Lindstrom LS, Carlson JW, Bergh J, Wennmalm K. Strong prognostic concordance between Ki67 and binary, but not multi-level gene expression signatures. Cancer Res 2012;72. https://doi.org/10.1158/0008-5472.SABCS12-PD06-08.
- Torres S, Trudeau ME, Gandhi S, Warner E, Verma S, Pritchard KI, et al. Impact of the 21-gene recurrence score assay on the adjuvant treatment of breast cancer patients with 1–3 positive lymph nodes in an academic centre in Ontario. J Clin Oncol Conf 2016;34.
- Torrisi R, Garcia-Etienne CA, Losurdo A, Morenghi E, Di Tommaso L, Gatzemeier W, et al. Can we predict the benefit of the 70-gene signature in the choice of adjuvant systemic treatment for ER positive, HER2 negative tumors in daily practice? A single institution experience. Cancer Res 2011;71. https://doi.org/10.1158/0008-5472.SABCS11-P4-09-27.
- Vollan HKM, Rueda OM, Borresen-Dale AL, Aparicio S, Caldas C. A tumor DNA complexity index is an independent predictor of survival in a dataset of 1950 breast cancers; a METABRIC group study. Cancer Res 2012;72. https://doi.org/10.1158/0008-5472.SABCS12-P2-10-20.
- Wolmark N, Mamounas EP, Baehner FL, Butler SM, Tang G, Jamshidian F, et al. Recurrence score and quantitative ER expression to predict in late distant recurrence risk in ER+ BC after 5 years of tamoxifen. J Clin Oncol Conf 2014;32.
- Wuerstlein R, Sotlar K, Gluz O, Hofmann D, Otremba B, Von Schumann R, et al. Significance of prospective multicenter decision impact WSG-BCIST Study in post-menopausal ER+ HER2-N0 early breast cancer (EBC) for molecular testing for intrinsic subtype definition. J Clin Oncol Conf 2015;33.
- Wuerstlein R, Sotlar K, Gluz O, Hofmann D, Otremba B, Von Schumann R, et al. Prosigna results impact on adjuvant decision making in early breast cancer (EBC): final analysis of the prospective WSG study. Cancer Res 2016;76. https://doi.org/10.1158/1538-7445.SABCS15-P5-07-03.
- Wurstlein R, Sotlar K, Gluz O, Hofmann D, Otremba B, Von Schumann R, et al. WSG BCIST study: Prosigna results impact on adjuvant decision making in early breast cancer (EBC). Oncol Res Treat 2016;39.
- Yamauchi H, Nakagawa C, Yamashige S, Takei H, Yagata H, Yoshida A, et al. Decision impact and economic evaluation of the 21-gene recurrence score (RS) assay for physicians and patients in Japan. Eur J Cancer 2011;47. https://doi.org/10.1016/S0959-8049(11)71603-3.
- Yamauchi H, Nakagawa C, Yamashige S, Takei H, Yagata H, Yoshida A, et al. Societal economics of the 21-gene recurrence score in estrogen-receptor-positive early-stage breast cancer in Japan. Cancer Res 2012;72. https://doi.org/10.1158/0008-5472.SABCS12-P5-15-06.
- Yao K, Turk M, Kaul K, Weaver J, De Snoo F, Stork-Sloots L, et al. Molecular subtyping using MammaPrint and BluePrint as an outcome predictor in U.S. breast cancer (BC) patients. J Clin Oncol Conf 2012;30.
- Yao K, Turk M, Kaul K, Wesseling J, Stork-Sloots L, De Snoo F, et al. MammaPrint and BluePrint in early breast cancer: clinical implications of prognostic stratification and molecular subtyping. Cancer Res 2013;73. https://doi.org/10.1158/0008-5472.SABCS13-P2-11-23.
- Yeo BJ, Dowsett M, Smith IE, Zabaglo L. The clinical impact of using the IHC4 score: our MDT experience in a prospective series of postmenopausal women with er positive early breast cancer. Ann Oncol 2014;25. https://doi.org/10.1093/annonc/mdu062.1.
- D’Alfonso TM, Van Laar RK, Flinchum R, Brown N, Saint John L, Shin SJ. BreastPRS effectively separates OncotypeDX intermediate risk group to low and high risk groups. Lab Invest 2013;93.
- Petkov VI, Miller DP, Howlader N, Gliner N, Howe W, Schussler NC, et al. Outcome disparities by age and 21-gene recurrence score (RS) in hormone receptor positive (HR+) breast cancer (BC). J Clin Oncol Conf 2016;34.
- Gluz O, Nitz U, Christgen M, Kates R, Clemens M, Kraemer S, et al. Prospective WSG phase III PlanB trial: clinical outcome at 5 year follow up and impact of 21 gene recurrence score result, central/local-pathological review of grade, ER, PR and Ki67 in HR+/HER2– high risk node-negative and-positive breast cancer. Eur J Cancer 2016;57.
- Drukker CA, Van Tinteren H, Schmidt MK, . Impact of the 70-gene signature on long term breast cancer outcome. Eur J Cancer 2014;50. https://doi.org/10.1007/s10549-013-2831-4.
- Martin M, Gonzalez-Rivera M, Morales S, de la Haba J, González-Cortijo L, Manso L, et al. Prospective Study of the Impact of the ProsignaTM Assay on Adjuvant Clinical Decision-Making in Women With Estrogen Receptor-Positive, HER2-Negative, Node-Negative Breast Cancer: A GEICAM Study n.d. https://doi.org/10.1158/1538-7445.SABCS14-P6-08-10.
- Holt SDH, Bertelli G, Pudney D, Rolles M, Moe M, Brinkworth E, et al. Results from a prospective clinical study on the impact of Oncotype DX on adjuvant treatment decision and risk classification by Nottingham prognostic index (NPI) and recurrence score (RS). Breast 2011;20. https://doi.org/10.1016/S0960-9776(11)70137-0.
- Smyth L, Watson G, Kelly CM, Keane M, Kennedy MJ, Grogan L, et al. Economic impact of 21-gene recurrence score testing on early stage breast cancer in Ireland. Breast 2015;24. https://doi.org/10.1016/S0960-9776(15)70285-7.
- Bartlett JMS, Stein RC, Bayani J, Marshall A, Dunn JA, Campbell AF, et al. Comparison of Multiparameter Tests in the UK OPTIMA-Prelim Trial. n.d. https://doi.org/10.1158/1538-7445.SABCS14-P4-11-07.
- D’Alfonso TM, Van Laar RK, Flinchum R, Brown N, Saint John L, Shin SJ. Breastprs effectively separates oncotypedx intermediate risk group to low and high risk groups. 2013. Laboratory Investigation n.d.
- Eiermann W, Rezai M, Kummel S, Kuhn T, Warm M, Friedrichs K, et al. Using the 21-gene breast cancer assay in adjuvant decision-making in ER-positive (ER+) early breast cancer (EBC) is cost-effective: results of a large prospective German multicenter study. Eur J Cancer 2012;48. https://doi.org/10.1016/S0959-8049(12)70368-4.
- van ‘t Veer LJ, Dai H, van de Vijver MJ, He YD, Hart AA, Mao M, et al. Gene expression profiling predicts clinical outcome of breast cancer. Nature 2002;415:530-6. https://doi.org/10.1038/415530a.
- Bartlett JM, Bayani J, Marshall A, Dunn JA, Campbell A, Cunningham C, et al. Comparing breast cancer multiparameter tests in the OPTIMA prelim trial: no test Is more equal than the others. J Natl Cancer Inst 2016;108. https://doi.org/10.1093/jnci/djw050.
- Howell A, Cuzick J, Baum M, Buzdar A, Dowsett M, Forbes JF, et al. Results of the ATAC (Arimidex, Tamoxifen, Alone or in Combination) trial after completion of 5 years’ adjuvant treatment for breast cancer. Lancet 2005;365:60-2. https://doi.org/10.1016/S0140-6736(04)17666-6.
- Montemurro F, Prat A, Rossi V, Valabrega G, Sperinde J, Peraldo-Neia C, et al. Potential biomarkers of long-term benefit from single-agent trastuzumab or lapatinib in HER2-positive metastatic breast cancer. Mol Oncol 2014;8:20-6. https://doi.org/10.1016/j.molonc.2013.08.013.
- Ahn SG, Lee HM, Lee HW, Lee SA, Lee SR, Leem SH, et al. Prognostic discrimination using a 70-gene signature among patients with estrogen receptor-positive breast cancer and an intermediate 21-gene recurrence score. Int J Mol Sci 2013;14:23685-99. https://doi.org/10.3390/ijms141223685.
- Bianchini G, Pusztai L, Karn T, Iwamoto T, Rody A, Kelly C, et al. Proliferation and estrogen signaling can distinguish patients at risk for early versus late relapse among estrogen receptor positive breast cancers. Breast Cancer Res 2013;15. https://doi.org/10.1186/bcr3481.
- Cockburn JG, Hallett RM, Gillgrass AE, Dias KN, Whelan T, Levine MN, et al. The effects of lymph node status on predicting outcome in ER+ /HER2– tamoxifen treated breast cancer patients using gene signatures. BMC Cancer 2016;16. https://doi.org/10.1186/s12885-016-2501-0.
- Fan C, Oh DS, Wessels L, Weigelt B, Nuyten DS, Nobel AB, et al. Concordance among gene-expression-based predictors for breast cancer. N Engl J Med 2006;355:560-9. https://doi.org/10.1056/NEJMoa052933.
- Finetti P, Guille A, Adelaide J, Birnbaum D, Chaffanet M, Bertucci F. ESPL1 is a candidate oncogene of luminal B breast cancers. Breast Cancer Res Treat 2014;147:51-9. https://doi.org/10.1007/s10549-014-3070-z.
- Győrffy B, Karn T, Sztupinszki Z, Weltz B, Müller V, Pusztai L. Dynamic classification using case-specific training cohorts outperforms static gene expression signatures in breast cancer. Int J Cancer 2015;136:2091-8. https://doi.org/10.1002/ijc.29247.
- Jonsdottir K, Assmus J, Slewa A, Gudlaugsson E, Skaland I, Baak JP, et al. Prognostic value of gene signatures and proliferation in lymph-node-negative breast cancer. PLOS ONE 2014;9. https://doi.org/10.1371/journal.pone.0090642.
- Li LF, Xu XJ, Zhao Y, Liu ZB, Shen ZZ, Jin WR, et al. Integrated gene expression profile predicts prognosis of breast cancer patients. Breast Cancer Res Treat 2009;113:231-7. https://doi.org/10.1007/s10549-008-9925-4.
- Loi S, Haibe-Kains B, Desmedt C, Lallemand F, Tutt AM, Gillet C, et al. Definition of clinically distinct molecular subtypes in estrogen receptor-positive breast carcinomas through genomic grade. J Clin Oncol 2007;25:1239-46. https://doi.org/10.1200/JCO.2006.07.1522.
- Naoi Y, Kishi K, Tsunashima R, Shimazu K, Shimomura A, Maruyama N, et al. Comparison of efficacy of 95-gene and 21-gene classifier (oncotype DX) for prediction of recurrence in ER-positive and node-negative breast cancer patients. Breast Cancer Res Treat 2013;140:299-306. https://doi.org/10.1007/s10549-013-2640-9.
- Prat A, Parker JS, Fan C, Cheang MC, Miller LD, Bergh J, et al. Concordance among gene expression-based predictors for ER-positive breast cancer treated with adjuvant tamoxifen. Ann Oncol 2012;23:2866-73. https://doi.org/10.1093/annonc/mds080.
- Tobin NP, Lindström LS, Carlson JW, Bjöhle J, Bergh J, Wennmalm K. Multi-level gene expression signatures, but not binary, outperform Ki67 for the long term prognostication of breast cancer patients. Mol Oncol 2014;8:741-52. https://doi.org/10.1016/j.molonc.2014.02.007.
- Vollan HK, Rueda OM, Chin SF, Curtis C, Turashvili G, Shah S, et al. A tumor DNA complex aberration index is an independent predictor of survival in breast and ovarian cancer. Mol Oncol 2015;9:115-27. https://doi.org/10.1016/j.molonc.2014.07.019.
- Xu W, Jia G, Cai N, Huang S, Davie JR, Pitz M, et al. A 16 Yin Yang gene expression ratio signature for ER+/node- breast cancer. Int J Cancer 2017;140:1413-24. https://doi.org/10.1002/ijc.30556.
- Ou Yang TH, Cheng WY, Zheng T, Maurer MA, Anastassiou D. Breast cancer prognostic biomarker using attractor metagenes and the FGD3-SUSD3 metagene. Cancer Epidemiol Biomarkers Prev 2014;23:2850-6. https://doi.org/10.1158/1055-9965.EPI-14-0399.
- Yin ZQ, Liu JJ, Xu YC, Yu J, Ding GH, Yang F, et al. A 41-gene signature derived from breast cancer stem cells as a predictor of survival. J Exp Clin Cancer Res 2014;33. https://doi.org/10.1186/1756-9966-33-49.
- Zemmour C, Bertucci F, Finetti P, Chetrit B, Birnbaum D, Filleron T, et al. Prediction of early breast cancer metastasis from DNA microarray data using high-dimensional Cox regression models. Cancer Informatics 2015;14:129-38. https://doi.org/10.4137/CIN.S17284.
- Zhao X, Rødland EA, Sørlie T, Vollan HK, Russnes HG, Kristensen VN, et al. Systematic assessment of prognostic gene signatures for breast cancer shows distinct influence of time and ER status. BMC Cancer 2014;14. https://doi.org/10.1186/1471-2407-14-211.
- Cockburn JG, Hallett RM, Gillgrass AE, Dias KN, Whelan T, Levine MN, et al. The effects of lymph node status on predicting the outcome in ER+ HER2- tamoxifen treated breast cancer patients using gene signatures. BMC Cancer 2016;16. https://doi.org/10.1186/s12885-016-2501-0.
- Tumour Profiling Tests to Guide Adjuvant Chemotherapy Decisions in People with Breast Cancer (Update of DG10) – Protocol. Sheffield: School of Health and Related Research; 2017.
- Bartlett J, Canney P, Campbell A, Cameron D, Donovan J, Dunn J, et al. Selecting breast cancer patients for chemotherapy: the opening of the UK OPTIMA trial. Clin Oncol 2013;25:109-16. https://doi.org/10.1016/j.clon.2012.10.005.
- Peasgood T, Ward SE, Brazier J. Health-state utility values in breast cancer. Expert Rev Pharmacoecon Outcomes Res 2010;10:553-66. https://doi.org/10.1586/erp.10.65.
- Simes RJ, Coates AS. Patient preferences for adjuvant chemotherapy of early breast cancer: how much benefit is needed?. J Natl Cancer Inst Monogr 2001:146-52. https://doi.org/10.1093/oxfordjournals.jncimonographs.a003453.
- Milne RJ, Heaton-Brown KH, Hansen P, Thomas D, Harvey V, Cubitt A. Quality-of-life valuations of advanced breast cancer by New Zealand women. PharmacoEconomics 2006;24:281-92. https://doi.org/10.2165/00019053-200624030-00007.
- Remák E, Brazil L. Cost of managing women presenting with stage IV breast cancer in the United Kingdom. Br J Cancer 2004;91:77-83. https://doi.org/10.1038/sj.bjc.6601890.
- Loncaster J, Armstrong A, Howell S, Wilson G, Welch R, Chittalia A, et al. Impact of Oncotype DX breast Recurrence Score testing on adjuvant chemotherapy use in early breast cancer: Real world experience in Greater Manchester, UK. Eur J Surg Oncol 2017;43:931-37. https://doi.org/10.1016/j.ejso.2016.12.010.
- Paik S, Tang G, Shak S, Kim C, Baker J, Kim W, et al. Gene expression and benefit of chemotherapy in women with node-negative, estrogen receptor-positive breast cancer. J Clin Oncol 2006;24:3726-34. https://doi.org/10.1200/JCO.2005.04.7985.
- Jahn B, Rochau U, Kurzthaler C, Hubalek M, Miksad R, Sroczynski G, et al. Cost effectiveness of personalized treatment in women with early breast cancer: the application of oncotypeDX and Adjuvant! Online to guide adjuvant chemotherapy in Austria. SpringerPlus 2015;4. https://doi.org/10.1186/s40064-015-1440-6.
- Paulden M, Franek J, Pham B, Bedard PL, Trudeau M, Krahn M. Cost-effectiveness of the 21-gene assay for guiding adjuvant chemotherapy decisions in early breast cancer. Value Health 2013;16:729-39. https://doi.org/10.1016/j.jval.2013.03.1625.
- Reed SD, Dinan MA, Schulman KA, Lyman GH. Cost-effectiveness of the 21-gene recurrence score assay in the context of multifactorial decision making to guide chemotherapy for early-stage breast cancer. Genet Med 2013;15:203-11. https://doi.org/10.1038/gim.2012.119.
- Retèl VP, Joore MA, Linn SC, Rutgers EJ, van Harten WH. Scenario drafting to anticipate future developments in technology assessment. BMC Res Notes 2012;5. https://doi.org/10.1186/1756-0500-5-442.
- Retèl VP, Joore MA, van Harten WH. Head-to-head comparison of the 70-gene signature versus the 21-gene assay: cost-effectiveness and the effect of compliance. Breast Cancer Res Treat 2012;131:627-36.
- Retèl VP, Grutters JP, van Harten WH, Joore MA. Value of research and value of development in early assessments of new medical technologies. Value Health 2013;16:720-8. https://doi.org/10.1016/j.jval.2013.04.013.
- Retèl VP, Joore MA, Drukker CA, Bueno-de-Mesquita JM, Knauer M, van Tinteren H, et al. Prospective cost-effectiveness analysis of genomic profiling in breast cancer. Eur J Cancer 2013;49:3773-9.
- Kondo M, Hoshi SL, Ishiguro H, Toi M. Economic evaluation of the 70-gene prognosis-signature (MammaPrint®) in hormone receptor-positive, lymph node-negative, human epidermal growth factor receptor type 2-negative early stage breast cancer in Japan. Breast Cancer Res Treat 2012;133:759-68. https://doi.org/10.1007/s10549-012-1979-7.
- Mislick K, Schonfeld W, Bodnar C, Tong KB. Cost-effectiveness analysis of Mammostrat® compared with oncotype DX® to inform the treatment of breast cancer. Clinicoecon Outcomes Res 2014;6:37-4. https://doi.org/10.2147/CEOR.S53142.
- Tiwana SK, Smith A, Leggett L, MacKean G, Lorenzetti D, Clement F. Use of oncotype DX for guiding adjuvant chemotherapy decisions in early stage invasive breast cancer patients in Alberta. Calgary, Canada: Health Technology Assessment; 2013.
- Vanderlaan BF, Broder MS, Chang EY, Oratz R, Bentley TG. Cost-effectiveness of 21-gene assay in node-positive, early-stage breast cancer. Am J Manag Care 2011;17:455-64.
- Wong WB, Ramsey SD, Barlow WE, Garrison LP, Veenstra DL. The value of comparative effectiveness research: projected return on investment of the RxPONDER trial (SWOG S1007). Contemp Clin Trials 2012;33:1117-23. https://doi.org/10.1016/j.cct.2012.08.006.
- Ramsey SD, Barlow WE, Gonzalez-Angulo AM, Tunis S, Baker L, Crowley J, et al. Integrating comparative effectiveness design elements and endpoints into a phase III, randomized clinical trial (SWOG S1007) evaluating oncotypeDX-guided management for women with breast cancer involving lymph nodes. Contemp Clin Trials 2013;34:1-9. https://doi.org/10.1016/j.cct.2012.09.003.
- Yang M, Rajan S, Issa AM. Cost effectiveness of gene expression profiling for early stage breast cancer: a decision-analytic model. Cancer 2012;118:5163-70. https://doi.org/10.1002/cncr.27443.
- Asad J, Jacobson AF, Estabrook A, Smith SR, Boolbol SK, Feldman SM, et al. Does oncotype DX recurrence score affect the management of patients with early-stage breast cancer?. Am J Surg 2008;196:527-9. https://doi.org/10.1016/j.amjsurg.2008.06.021.
Appendix 1 Additional tables for Chapter 1
Age (years) | Sex (n) | |
---|---|---|
Male | Female | |
All | 319 | 45,764 |
0–24 | 0 | 21 |
25–29 | 0 | 191 |
30–34 | 4 | 593 |
35–39 | 3 | 1071 |
40–44 | 7 | 2299 |
45–49 | 11 | 4369 |
50–54 | 17 | 5386 |
55–59 | 23 | 4589 |
60–64 | 30 | 5072 |
65–69 | 57 | 6502 |
70–74 | 45 | 4436 |
75–79 | 52 | 3889 |
80–84 | 42 | 3419 |
≥ 85 | 28 | 3927 |
Characteristic | Tool | ||
---|---|---|---|
NPI | AOL | Predict | |
Factors included in prediction algorithm |
|
|
|
Outcome(s) predicted | Mortality | Mortality or relapse | Mortality |
Appendix 2 Literature search strategies
Clinical effectiveness
Epub Ahead of Print, In-Process & Other Non-Indexed Citations, Ovid MEDLINE(R) Daily and Ovid MEDLINE(R)
Date range searched: 1946 to search date.
Search date: 27 February 2017.
Search strategy
# | Searches |
1 | exp Breast Neoplasms/ |
2 | exp mammary neoplasms/ |
3 | exp “Neoplasms, Ductal, Lobular, and Medullary”/ |
4 | exp breast/ |
5 | exp neoplasms/ |
6 | 4 and 5 |
7 | (breast* adj5 (neoplasm* or cancer* or tumo?r* or carcinoma* or adenocarcinoma* or sarcoma* or dcis or ductal or infiltrat* or intraductal* or lobular or medullary)).mp. |
8 | (mammar* adj5 (neoplasm* or cancer* or tumo?r* or carcinoma* or adenocarcinoma* or sarcoma* or dcis or ductal or infiltrat* or intraductal* or lobular or medullar)).mp. |
9 | 1 or 2 or 3 or 6 or 7 or 8 |
10 | EndoPredict.mp. |
11 | myriad genetics.mp. |
12 | sividon diagnostics.mp. |
13 | ep score.mp. |
14 | epclin score.mp. |
15 | MammaPrint.mp. |
16 | 70-gene.mp. |
17 | gene70.mp. |
18 | gene?seventy.mp. |
19 | seventy?gene.mp. |
20 | amsterdam profile.mp. |
21 | oncotype.mp. |
22 | oncotype dx.mp. |
23 | 21-gene.mp. |
24 | gene21.mp. |
25 | gene?twentyone.mp. |
26 | twentyone?gene.mp. |
27 | ghi recurrence score.mp. |
28 | ghi-rs.mp. |
29 | 92-gene.mp. |
30 | gene92.mp. |
31 | gene?ninetytwo.mp. |
32 | ninetytwo?gene.mp. |
33 | (rct-pcr adj5 “21”).mp. |
34 | prosigna.mp. |
35 | pam50.mp. |
36 | 50-gene.mp. |
37 | gene50.mp. |
38 | gene?fifty.mp. |
39 | fifty?gene.mp. |
40 | breast bioclassifier.mp. |
41 | ihc4.mp. |
42 | or/10–14 |
43 | or/15–41 |
44 | 9 and 42 |
45 | 9 and 43 |
46 | limit 45 to yr=“2011 -Current” |
47 | 44 or 46 |
EMBASE
Date range searched: 1974 to 24 February 2017.
Search date: 27 February 2017.
Search strategy
# | Searches |
1 | exp breast tumor/ |
2 | exp breast/ |
3 | exp neoplasm/ |
4 | 2 and 3 |
5 | (breast* adj5 (neoplasm* or cancer* or tumo?r* or carcinoma* or adenocarcinoma* or sarcoma* or dcis or ductal or infiltrat* or intraductal* or lobular or medullary)).mp. |
6 | (mammar* adj5 (neoplasm* or cancer* or tumo?r* or carcinoma* or adenocarcinoma* or sarcoma* or dcis or ductal or infiltrat* or intraductal* or lobular or medullar)).mp. |
7 | 1 or 4 or 5 or 6 |
8 | EndoPredict.mp. |
9 | myriad genetics.mp. |
10 | sividon diagnostics.mp. |
11 | ep score.mp. |
12 | epclin score.mp. |
13 | MammaPrint.mp. |
14 | 70-gene.mp. |
15 | gene70.mp. |
16 | gene?seventy.mp. |
17 | seventy?gene.mp. |
18 | amsterdam profile.mp. |
19 | oncotype.mp. |
20 | oncotype dx.mp. |
21 | 21-gene.mp. |
22 | gene21.mp. |
23 | gene?twentyone.mp. |
24 | twentyone?gene.mp. |
25 | ghi recurrence score.mp. |
26 | ghi-rs.mp. |
27 | 92-gene.mp. |
28 | gene92.mp. |
29 | gene?ninetytwo.mp. |
30 | ninetytwo?gene.mp. |
31 | (rct-pcr adj5 “21”).mp. |
32 | prosigna.mp. |
33 | pam50.mp. |
34 | 50-gene.mp. |
35 | gene50.mp. |
36 | gene?fifty.mp. |
37 | fifty?gene.mp. |
38 | breast bioclassifier.mp. |
39 | ihc4.mp. |
40 | or/8-12 |
41 | or/13-39 |
42 | 7 and 40 |
43 | 7 and 41 |
44 | limit 43 to yr=“2011 -Current” |
45 | 42 or 44 |
Web of Science Core Collection databases
Date range searched: 1900–search date.
Search date: 27 February 2017.
Science Citation Index Expanded
Date range searched: 1900–search date.
Search date: 27 February 2017.
Conference Proceedings Citation Index – Science
Date range searched: 1900–search date.
Search date: 27 February 2017.
Search strategy
# | Searches |
# 1 | TOPIC: ((breast* NEAR/5 (neoplasm* or cancer* or tumo?r* or carcinoma* or adenocarcinoma* or sarcoma* or dcis or ductal or infiltrat* or intraductal* or lobular or medullary))) |
# 2 | TOPIC: ((mammar* NEAR/5 (neoplasm* or cancer* or tumo?r* or carcinoma* or adenocarcinoma* or sarcoma* or dcis or ductal or infiltrat* or intraductal* or lobular or medullar))) |
# 3 | #2 OR #1 |
# 4 | TOPIC: (EndoPredict) OR TOPIC: (myriad genetics) OR TOPIC: (sividon diagnostics) OR TOPIC: (ep score) OR TOPIC: (epclin score) |
# 5 | TS=(MammaPrint) OR TS=(70-gene) OR TS=(gene70) OR TS=(gene?seventy) OR TS=(seventy?gene) OR TS=(amsterdam profile) |
# 6 | TS=(oncotype) OR TS=(oncotype dx) OR TS=(21-gene) OR TS=(gene21) OR TS=(gene?twentyone) OR TS=(twentyone?gene) OR TS=(ghi recurrence score) OR TS=(ghi-rs) OR TS=(92-gene) OR TS=(gene92) OR TS=(gene?ninetytwo) OR TS=(ninetytwo?gene) OR TS=((rct-pcr NEAR/5 ‘21’)) |
# 7 | TOPIC: (prosigna) OR TOPIC: (pam50) OR TOPIC: (50-gene) OR TOPIC: (gene50) OR TOPIC: (gene?fifty) OR TOPIC: (fifty?gene) OR TOPIC: (breast bioclassifier) |
# 8 | TOPIC: (ihc4) |
# 9 | #8 OR #7 OR #6 OR #5 |
# 10 | #9 AND #3 Indexes=SCI-EXPANDED, CPCI-S Timespan=2011–2017 |
# 11 | #4 AND #3 |
# 12 | #11 OR #10 |
Wiley Interscience databases
Cochrane Database of Systematic Reviews: Wiley Interscience
Date range searched: 1996–search date.
Search date: 28 February 2017.
Database of Abstracts of Reviews of Effects: Wiley Interscience
Date range searched: 1995–2015.
Search date: 28 February 2017.
Cochrane Central Register of Controlled Trials: Wiley Interscience
Date range searched: 1995–search date.
Search date: 28 February 2017.
Health Technology Assessment Database: Wiley Interscience
Date range searched: 1995–2016.
Search date: 28 February 2017.
NHS Economic Evaluation Database: Wiley Interscience
Date range searched: 1995–2015.
Search date: 28 February 2017.
Search strategy
# | Searches |
#1 | MeSH descriptor: [Breast Neoplasms] explode all trees |
#2 | MeSH descriptor: [Neoplasms, Ductal, Lobular, and Medullary] explode all trees |
#3 | MeSH descriptor: [Breast] explode all trees |
#4 | MeSH descriptor: [Neoplasms] explode all trees |
#5 | #3 and #4 |
#6 | (breast* near/5 (neoplasm* or cancer* or tumor* or tumour* or carcinoma* or adenocarcinoma* or sarcoma* or dcis or ductal or infiltrat* or intraductal* or lobular or medullary)) |
#7 | (mammar* near/5 (neoplasm* or cancer* or tumor* or tumour* or carcinoma* or adenocarcinoma* or sarcoma* or dcis or ductal or infiltrat* or intraductal* or lobular or medullar)) |
#8 | #1 or #2 or #5 or #6 or #7 |
#9 | EndoPredict |
#10 | myriad genetics |
#11 | sividon diagnostics |
#12 | ep score |
#13 | epclin score |
#14 | MammaPrint |
#15 | 70-gene |
#16 | gene70 |
#17 | gene*seventy |
#18 | seventy*gene |
#19 | amsterdam profile |
#20 | oncotype |
#21 | oncotype dx |
#22 | 21-gene |
#23 | gene21 |
#24 | gene*twentyone |
#25 | twentyone*gene |
#26 | ghi recurrence score |
#27 | ghi-rs |
#28 | 92-gene |
#29 | gene92 |
#30 | gene*ninetytwo |
#31 | ninetytwo*gene |
#32 | (rct-pcr near/5 ‘21’) |
#33 | prosigna |
#34 | pam50 |
#35 | 50-gene |
#36 | gene50 |
#37 | gene*fifty |
#38 | fifty*gene |
#39 | breast bioclassifier |
#40 | ihc4 |
#41 | (or #9-#13) |
#42 | 28–#40–#40 |
#43 | #8 and #41 |
#44 | #8 and #42 Publication Year from 2011 |
#45 | #43 or #44 |
World Health Organization International Clinical Trials Registry Platform
Search date: 19 January 2017.
Search strategy
# | Searches |
1 | EndoPredict or MammaPrint or oncotype or IHC4 or Prosigna |
Cost-effectiveness studies of tumour profiling tests
Epub Ahead of Print, In-Process & Other Non-Indexed Citations, Ovid MEDLINE(R) Daily and Ovid MEDLINE(R)
Date range searched: 1946 to present.
Search date: 6 March 2017.
Search strategy
# | Searches |
1 | exp Breast Neoplasms/ |
2 | exp mammary neoplasms/ |
3 | exp “Neoplasms, Ductal, Lobular, and Medullary”/ |
4 | exp breast/ |
5 | exp neoplasms/ |
6 | 4 and 5 |
7 | (breast* adj5 (neoplasm* or cancer* or tumo?r* or carcinoma* or adenocarcinoma* or sarcoma* or dcis or ductal or infiltrat* or intraductal* or lobular or medullary)).mp. |
8 | (mammar* adj5 (neoplasm* or cancer* or tumo?r* or carcinoma* or adenocarcinoma* or sarcoma* or dcis or ductal or infiltrat* or intraductal* or lobular or medullar)).mp. |
9 | 1 or 2 or 3 or 6 or 7 or 8 |
10 | EndoPredict.mp. |
11 | myriad genetics.mp. |
12 | sividon diagnostics.mp. |
13 | ep score.mp. |
14 | epclin score.mp. |
15 | MammaPrint.mp. |
16 | 70-gene.mp. |
17 | gene70.mp. |
18 | gene?seventy.mp. |
19 | seventy?gene.mp. |
20 | amsterdam profile.mp. |
21 | oncotype.mp. |
22 | oncotype dx.mp. |
23 | 21-gene.mp. |
24 | gene21.mp. |
25 | gene?twentyone.mp. |
26 | twentyone?gene.mp. |
27 | ghi recurrence score.mp. |
28 | ghi-rs.mp. |
29 | 92-gene.mp. |
30 | gene92.mp. |
31 | gene?ninetytwo.mp. |
32 | ninetytwo?gene.mp. |
33 | (rct-pcr adj5 ‘21’).mp. |
34 | prosigna.mp. |
35 | pam50.mp. |
36 | 50-gene.mp. |
37 | gene50.mp. |
38 | gene?fifty.mp. |
39 | fifty?gene.mp. |
40 | breast bioclassifier.mp. |
41 | ihc4.mp. |
42 | or/10-14 |
43 | or/15-41 |
44 | 9 and 42 |
45 | 9 and 43 |
46 | limit 45 to yr=“2011 -Current” |
47 | 44 or 46 |
48 | exp “Costs and Cost Analysis”/ |
49 | Economics/ |
50 | exp Economics, Hospital/ |
51 | exp Economics, Medical/ |
52 | Economics, Nursing/ |
53 | exp models, economic/ |
54 | Economics, Pharmaceutical/ |
55 | exp “Fees and Charges”/ |
56 | exp Budgets/ |
57 | budget$.tw. |
58 | ec.fs. |
59 | cost$.ti. |
60 | (cost$adj2 (effective$or utilit$or benefit$or minimi$)).ab. |
61 | (economic$or pharmacoeconomic$or pharmaco-economic$).ti. |
62 | (price$or pricing$).tw. |
63 | (financial or finance or finances or financed).tw. |
64 | (fee or fees).tw. |
65 | (value adj2 (money or monetary)).tw. |
66 | quality-adjusted life years/ |
67 | (qaly or qalys).af. |
68 | (quality adjusted life year or quality adjusted life years).af. |
69 | or/48-68 |
70 | 47 and 69 |
EMBASE
Date range searched: 1974 to 3 March 2017.
Search date: 6 March 2017.
Search strategy
# | Searches |
1 | exp breast tumor/ |
2 | exp breast/ |
3 | exp neoplasm/ |
4 | 2 and 3 |
5 | (breast* adj5 (neoplasm* or cancer* or tumo?r* or carcinoma* or adenocarcinoma* or sarcoma* or dcis or ductal or infiltrat* or intraductal* or lobular or medullary)).mp. |
6 | (mammar* adj5 (neoplasm* or cancer* or tumo?r* or carcinoma* or adenocarcinoma* or sarcoma* or dcis or ductal or infiltrat* or intraductal* or lobular or medullar)).mp. |
7 | 1 or 4 or 5 or 6 |
8 | EndoPredict.mp. |
9 | myriad genetics.mp. |
10 | sividon diagnostics.mp. |
11 | ep score.mp. |
12 | epclin score.mp. |
13 | MammaPrint.mp. |
14 | 70-gene.mp. |
15 | gene70.mp. |
16 | gene?seventy.mp. |
17 | seventy?gene.mp. |
18 | amsterdam profile.mp. |
19 | oncotype.mp. |
20 | oncotype dx.mp. |
21 | 21-gene.mp. |
22 | gene21.mp. |
23 | gene?twentyone.mp. |
24 | twentyone?gene.mp. |
25 | ghi recurrence score.mp. |
26 | ghi-rs.mp. |
27 | 92-gene.mp. |
28 | gene92.mp. |
29 | gene?ninetytwo.mp. |
30 | ninetytwo?gene.mp. |
31 | (rct-pcr adj5 ‘21’).mp. |
32 | prosigna.mp. |
33 | pam50.mp. |
34 | 50-gene.mp. |
35 | gene50.mp. |
36 | gene?fifty.mp. |
37 | fifty?gene.mp. |
38 | breast bioclassifier.mp. |
39 | ihc4.mp. |
40 | or/8-12 |
41 | or/13-39 |
42 | 7 and 40 |
43 | 7 and 41 |
44 | limit 43 to yr=“2011 -Current” |
45 | 42 or 44 |
46 | Socioeconomics/ |
47 | Cost benefit analysis/ |
48 | Cost effectiveness analysis/ |
49 | Cost of illness/ |
50 | Cost control/ |
51 | Economic aspect/ |
52 | Financial management/ |
53 | Health care cost/ |
54 | Health care financing/ |
55 | Health economics/ |
56 | Hospital cost/ |
57 | (fiscal or financial or finance or funding).tw. |
58 | Cost minimization analysis/ |
59 | (cost adj estimate$).mp. |
60 | (cost adj variable$).mp. |
61 | (unit adj cost$).mp. |
62 | or/46-61 |
63 | 45 and 62 |
Web of Science Core Collection databases
Date range searched: 1900–search date.
Search date: 6 March 2017.
Science Citation Index Expanded
Date range searched: 1900–search date.
Search date: 6 March 2017.
Conference Proceedings Citation Index – Science
Date range searched: 1900–search date.
Search date: 6 March 2017.
Search strategy
# | Searches |
# 1 | TOPIC: ((breast* NEAR/5 (neoplasm* or cancer* or tumo?r* or carcinoma* or adenocarcinoma* or sarcoma* or dcis or ductal or infiltrat* or intraductal* or lobular or medullary))) |
# 2 | TOPIC: ((mammar* NEAR/5 (neoplasm* or cancer* or tumo?r* or carcinoma* or adenocarcinoma* or sarcoma* or dcis or ductal or infiltrat* or intraductal* or lobular or medullar))) |
# 3 | #2 OR #1 |
# 4 | TOPIC: (EndoPredict) OR TOPIC: (myriad genetics) OR TOPIC: (sividon diagnostics) OR TOPIC: (ep score) OR TOPIC: (epclin score) |
# 5 | TS=(MammaPrint) OR TS=(70-gene) OR TS=(gene70) OR TS=(gene?seventy) OR TS=(seventy?gene) OR TS=(amsterdam profile) |
# 6 | TS=(oncotype) OR TS=(oncotype dx) OR TS=(21-gene) OR TS=(gene21) OR TS=(gene?twentyone) OR TS=(twentyone?gene) OR TS=(ghi recurrence score) OR TS=(ghi-rs) OR TS=(92-gene) OR TS=(gene92) OR TS=(gene?ninetytwo) OR TS=(ninetytwo?gene) OR TS=((rct-pcr NEAR/5 ‘21’)) |
# 7 | TOPIC: (prosigna) OR TOPIC: (pam50) OR TOPIC: (50-gene) OR TOPIC: (gene50) OR TOPIC: (gene?fifty) OR TOPIC: (fifty?gene) OR TOPIC: (breast bioclassifier) |
# 8 | TOPIC: (ihc4) |
# 9 | #8 OR #7 OR #6 OR #5 |
# 10 | #9 AND #3 Indexes=SCI-EXPANDED, CPCI-S Timespan=2011-2017 |
# 11 | #4 AND #3 |
# 12 | #11 OR #10 |
# 13 | TS=(cost* and (effective* or utilit* or benefit* or minimi*)) OR TS=(cost*) OR TI=(economic* or pharmacoeconomic* or pharmaco-economic*) OR TS=(price* or pricing*) OR TS=(financial or finance or finances or financed) OR TS=(fee or fees) OR TS=(value and (money or monetary)) OR TS=(economic*) OR TS=(economic* and (hospital or medical or nursing or pharmaceutical)) OR TS=(“quality adjusted life year” or “quality adjusted life years”) OR TS=(qaly or qalys) OR TS=(budget*) |
# 14 | #13 AND #12 |
Health Technology Assessment Database: Wiley Interscience
Date range searched: 1995–2016.
Search date: 7 March 2017.
NHS Economic Evaluation Database: Wiley Interscience
Date range searched: 1995–2015.
Search date: 7 March 2017.
Search strategy
# | Searches |
1 | MeSH DESCRIPTOR Breast Neoplasms EXPLODE ALL TREES |
2 | MeSH DESCRIPTOR Neoplasms, Ductal, Lobular, and Medullary EXPLODE ALL TREES |
3 | MeSH DESCRIPTOR Breast EXPLODE ALL TREES |
4 | MeSH DESCRIPTOR Neoplasms EXPLODE ALL TREES |
5 | #3 AND #4 |
6 | ((breast* ADJ5 (neoplasm* or cancer* or tumor* or tumour* or carcinoma* or adenocarcinoma* or sarcoma* or dcis or ductal or infiltrat* or intraductal* or lobular or medullary))) |
7 | ((mammar* ADJ5 (neoplasm* or cancer* or tumor* or tumour* or carcinoma* or adenocarcinoma* or sarcoma* or dcis or ductal or infiltrat* or intraductal* or lobular or medullar))) |
8 | #1 OR #2 OR #5 OR #6 OR #7 |
9 | (EndoPredict or myriad genetics or sividon diagnostics or ep score or epclin score) |
10 | (MammaPrint or 70-gene or gene70 or gene*seventy or seventy*gene or amsterdam profile) |
11 | (oncotype or oncotype dx or 21-gene or gene21 or gene*twentyone or twentyone*gene or ghi recurrence score or ghi-rs or 92-gene or gene92 or gene*ninetytwo or ninetytwo*gene or (rct-pcr ADJ5 ‘21’)) |
12 | (prosigna or pam50 or 50-gene or gene50 or gene*fifty or fifty*gene or breast bioclassifier) |
13 | (ihc4) |
14 | #8 AND #9 |
15 | #10 OR #11 OR #12 OR #13 |
16 | (#8 AND #15) FROM 2011 TO 2017 |
17 | (#8 AND #15) IN HTA FROM 2011 TO 2017 |
18 | (#8 AND #15) IN NHSEED FROM 2011 TO 2017 |
Cost-effectiveness reviews for breast cancer
Epub Ahead of Print, In-Process & Other Non-Indexed Citations, Ovid MEDLINE(R) Daily and Ovid MEDLINE(R)
Date range searched: 1946 to present.
Search date: 7 March 2017.
Search strategy
# | Searches |
1 | exp Breast Neoplasms/ |
2 | exp mammary neoplasms/ |
3 | exp “Neoplasms, Ductal, Lobular, and Medullary”/ |
4 | exp breast/ |
5 | exp neoplasms/ |
6 | 4 and 5 |
7 | (breast* adj5 (neoplasm* or cancer* or tumo?r* or carcinoma* or adenocarcinoma* or sarcoma* or dcis or ductal or infiltrat* or intraductal* or lobular or medullary)).ti. |
8 | (mammar* adj5 (neoplasm* or cancer* or tumo?r* or carcinoma* or adenocarcinoma* or sarcoma* or dcis or ductal or infiltrat* or intraductal* or lobular or medullar)).ti. |
9 | 1 or 2 or 3 or 6 or 7 or 8 |
10 | exp “Costs and Cost Analysis”/ |
11 | Economics/ |
12 | exp Economics, Hospital/ |
13 | exp Economics, Medical/ |
14 | Economics, Nursing/ |
15 | exp models, economic/ |
16 | Economics, Pharmaceutical/ |
17 | exp “Fees and Charges”/ |
18 | exp Budgets/ |
19 | budget$.tw. |
20 | ec.fs. |
21 | cost$.ti. |
22 | (cost$adj2 (effective$or utilit$or benefit$or minimi$)).ab. |
23 | (economic$or pharmacoeconomic$or pharmaco-economic$).ti. |
24 | (price$or pricing$).tw. |
25 | (financial or finance or finances or financed).tw. |
26 | (fee or fees).tw. |
27 | (value adj2 (money or monetary)).tw. |
28 | quality-adjusted life years/ |
29 | (qaly or qalys).af. |
30 | (quality adjusted life year or quality adjusted life years).af. |
31 | or/10-30 |
32 | 9 and 31 |
33 | meta-analysis/ |
34 | meta-analysis as topic/ |
35 | (meta analy* or metanaly* or metaanaly*).ti,ab. |
36 | ((systematic* or evidence*) adj3 (review* or overview*)).ti,ab. |
37 | (reference list* or bibliograph* or hand search* or manual search* or relevant journals).ab. |
38 | (search strategy or search criteria or systematic search or study selection or data extraction).ab. |
39 | (search* adj4 literature).ab. |
40 | (medline or pubmed or cochrane or embase or psychlit or psyclit or psychinfo or psycinfo or cinahl or scie nce citation index or bids or cancerlit).ab. |
41 | cochrane.jw. |
42 | ((multiple treatment* or indirect or mixed) adj2 comparison*).ti,ab. |
43 | or/33-42 |
44 | 32 and 43 |
45 | limit 44 to yr=“2011 -Current” |
EMBASE
Date range searched: 1974 to 6 March 2017.
Search date: 7 March 2017.
Search strategy
# | Searches |
1 | exp breast tumor/ |
2 | exp breast/ |
3 | exp neoplasm/ |
4 | 2 and 3 |
5 | (breast* adj5 (neoplasm* or cancer* or tumo?r* or carcinoma* or adenocarcinoma* or sarcoma* or dcis or ductal or infiltrat* or intraductal* or lobular or medullary)).ti. |
6 | (mammar* adj5 (neoplasm* or cancer* or tumo?r* or carcinoma* or adenocarcinoma* or sarcoma* or dcis or ductal or infiltrat* or intraductal* or lobular or medullar)).ti. |
7 | 1 or 4 or 5 or 6 |
8 | Socioeconomics/ |
9 | Cost benefit analysis/ |
10 | Cost effectiveness analysis/ |
11 | Cost of illness/ |
12 | Cost control/ |
13 | Economic aspect/ |
14 | Financial management/ |
15 | Health care cost/ |
16 | Health care financing/ |
17 | Health economics/ |
18 | Hospital cost/ |
19 | (fiscal or financial or finance or funding).tw. |
20 | Cost minimization analysis/ |
21 | (cost adj estimate$).mp. |
22 | (cost adj variable$).mp. |
23 | (unit adj cost$).mp. |
24 | or/8-23 |
25 | 7 and 24 |
26 | systematic review/ |
27 | meta-analysis/ |
28 | (meta analy* or metanaly* or metaanaly*).ti,ab. |
29 | ((systematic or evidence) adj3 (review* or overview*)).ti,ab. |
30 | (reference list* or bibliograph* or hand search* or manual search* or relevant journals).ab. |
31 | (search strategy or search criteria or systematic search or study selection or data extraction).ab. |
32 | (search* adj4 literature).ab. |
33 | (medline or pubmed or cochrane or embase or psychlit or psyclit or psychinfo or psycinfo or cinahl or science citation index or bids or cancerlit).ab. |
34 | cochrane.jw. |
35 | ((multiple treatment* or indirect or mixed) adj2 comparison*).ti,ab. |
36 | or/26-35 |
37 | 25 and 36 |
38 | limit 37 to yr=“2011 -Current” |
Web of Science Core Collection databases
Date range searched: 1900–search date.
Search date: 7 March 2017.
Science Citation Index Expanded
Date range searched: 1900–search date.
Search date: 7 March 2017.
Conference Proceedings Citation Index – Science
Date range searched: 1900–search date.
Search date: 7 March 2017.
Search strategy
# | Searches |
# 1 | TI=((breast* NEAR/5 (neoplasm* or cancer* or tumo?r* or carcinoma* or adenocarcinoma* or sarcoma* or dcis or ductal or infiltrat* or intraductal* or lobular or medullary))) |
# 2 | TI=((mammar* NEAR/5 (neoplasm* or cancer* or tumo?r* or carcinoma* or adenocarcinoma* or sarcoma* or dcis or ductal or infiltrat* or intraductal* or lobular or medullar))) |
# 3 | #2 OR #1 |
# 4 | TS=(cost* and (effective* or utilit* or benefit* or minimi*)) OR TS=(cost*) OR TI=(economic* or pharmacoeconomic* or pharmaco-economic*) OR TS=(price* or pricing*) OR TS=(financial or finance or finances or financed) OR TS=(fee or fees) OR TS=(value and (money or monetary)) OR TS=(economic*) OR TS=(economic* and (hospital or medical or nursing or pharmaceutical)) OR TS=(“quality adjusted life year” or “quality adjusted life years”) OR TS=(qaly or qalys) OR TS=(budget*) |
# 5 | #4 AND #3 |
# 6 | TS=(meta-analysis or meta analy* or metaanaly*) OR TS=(“review literature” or “literature review”) OR TS=(“systematic review*” or “systematic overview*”) OR TS=(cochrane or embase or psychlit or psyclit or psychinfo or psycinfo or cinahl or cinhal or science citation index or bids or cancerlit) OR TS=(“reference list*” or bibliograph* or hand-search* or “relevant journals” or “manual search*”) OR TS=((“selection criteria” or “data extraction”) and review) |
# 7 | #6 AND #5 Indexes=SCI-EXPANDED, CPCI-S Timespan=2011-2017 |
Health Technology Assessment Database: Centre for Reviews and Dissemination
Date range searched: 1995–2016.
Search date: 7 March 2017.
NHS Economic Evaluation Database: Centre for Reviews and Dissemination
Date range searched: 1995–2015.
Search date: 7 March 2017.
Search strategy
# | Searches |
1 | MeSH DESCRIPTOR Breast Neoplasms EXPLODE ALL TREES |
2 | MeSH DESCRIPTOR Neoplasms, Ductal, Lobular, and Medullary EXPLODE ALL TREES |
3 | MeSH DESCRIPTOR Breast EXPLODE ALL TREES |
4 | MeSH DESCRIPTOR Neoplasms EXPLODE ALL TREES |
5 | #3 AND #4 |
6 | ((breast* ADJ5 (neoplasm* or cancer* or tumor* or tumour* or carcinoma* or adenocarcinoma* or sarcoma* or dcis or ductal or infiltrat* or intraductal* or lobular or medullary))) |
7 | ((mammar* ADJ5 (neoplasm* or cancer* or tumor* or tumour* or carcinoma* or adenocarcinoma* or sarcoma* or dcis or ductal or infiltrat* or intraductal* or lobular or medullar))) |
8 | #1 OR #2 OR #5 OR #6 OR #7 |
9 | (EndoPredict or myriad genetics or sividon diagnostics or ep score or epclin score) |
10 | (MammaPrint or 70-gene or gene70 or gene*seventy or seventy*gene or amsterdam profile) |
11 | (oncotype or oncotype dx or 21-gene or gene21 or gene*twentyone or twentyone*gene or ghi recurrence score or ghi-rs or 92-gene or gene92 or gene*ninetytwo or ninetytwo*gene or (rct-pcr ADJ5 ‘21’)) |
12 | (prosigna or pam50 or 50-gene or gene50 or gene*fifty or fifty*gene or breast bioclassifier) |
13 | (ihc4) |
14 | #8 AND #9 |
15 | #10 OR #11 OR #12 OR #13 |
16 | (#8 AND #15) FROM 2011 TO 2017 |
17 | (#8 AND #15) IN HTA FROM 2011 TO 2017 |
18 | (#8 AND #15) IN NHSEED FROM 2011 TO 2017 |
Quality-of-life reviews for breast cancer
Epub Ahead of Print, In-Process & Other Non-Indexed Citations, Ovid MEDLINE(R) Daily and Ovid MEDLINE(R)
Date range searched: 1946 to present.
Search date: 7 March 2017.
Search strategy
# | Searches |
1 | exp Breast Neoplasms/ |
2 | exp mammary neoplasms/ |
3 | exp “Neoplasms, Ductal, Lobular, and Medullary”/ |
4 | exp breast/ |
5 | exp neoplasms/ |
6 | 4 and 5 |
7 | (breast* adj5 (neoplasm* or cancer* or tumo?r* or carcinoma* or adenocarcinoma* or sarcoma* or dcis or ductal or infiltrat* or intraductal* or lobular or medullary)).ti. |
8 | (mammar* adj5 (neoplasm* or cancer* or tumo?r* or carcinoma* or adenocarcinoma* or sarcoma* or dcis or ductal or infiltrat* or intraductal* or lobular or medullar)).ti. |
9 | 1 or 2 or 3 or 6 or 7 or 8 |
10 | “Quality of Life”/ |
11 | (qol or (quality adj2 life)).ab,ti. |
12 | (value adj2 (money or monetary)).tw. |
13 | value of life/ |
14 | quality adjusted life year/ |
15 | quality adjusted life.tw. |
16 | (qaly$or qald$or qale$or qtime$).tw. |
17 | disability adjusted life.tw. |
18 | daly$.tw. |
19 | health status indicators/ |
20 | (sf36 or sf 36 or short form 36 or shortform 36 or sf thirtysix or sf thirty six or shorform thirtysix or shortform thirty six or short form thirtysix or short form thirty six).tw. |
21 | (sf 6 or sf6 or short form 6 or shortform 6 or sf six or sfsix or shortform six or short form six).tw. |
22 | (sf12 or sf 12 or short form 12 or shortform 12 or sf twelve or sftwelve or shortform twelve or short form twelve).tw. |
23 | (sf6D or sf 6D or short form 6D or shortform 6D or sf six D or sfsixD or shortform six D or short form six D).tw. |
24 | (sf20 or sf 20 or short form 20 or shortform 20 or sf twenty or sftwenty or shortform twenty or short form twenty).tw. |
25 | (euroqol or euro qol or eq5d or eq 5d).tw. |
26 | (hql or hqol or h qol or hrqol or hr qol).tw. |
27 | (hye or hyes).tw. |
28 | health$year$equivalent$.tw. |
29 | health utilit$.tw. |
30 | (hui or hui1 or hui2 or hui3).tw. |
31 | disutilit$.tw. |
32 | rosser.tw. |
33 | (quality adj2 wellbeing).tw. |
34 | qwb.tw. |
35 | (willingness adj2 pay).tw. |
36 | standard gamble$.tw. |
37 | time trade off.tw. |
38 | time tradeoff.tw. |
39 | tto.tw. |
40 | letter.pt. |
41 | editorial.pt. |
42 | comment.pt. |
43 | 40 or 41 or 42 |
44 | or/10-39 |
45 | 44 not 43 |
46 | 9 and 45 |
47 | meta-analysis/ |
48 | meta-analysis as topic/ |
49 | (meta analy* or metanaly* or metaanaly*).ti,ab. |
50 | ((systematic* or evidence*) adj3 (review* or overview*)).ti,ab. |
51 | (reference list* or bibliograph* or hand search* or manual search* or relevant journals).ab. |
52 | (search strategy or search criteria or systematic search or study selection or data extraction).ab. |
53 | (search* adj4 literature).ab. |
54 | (medline or pubmed or cochrane or embase or psychlit or psyclit or psychinfo or psycinfo or cinahl or scie nce citation index or bids or cancerlit).ab. |
55 | cochrane.jw. |
56 | ((multiple treatment* or indirect or mixed) adj2 comparison*).ti,ab. |
57 | or/47-56 |
58 | 46 and 57 |
59 | limit 58 to yr=“2011 -Current” |
EMBASE
Date range searched: 1974 to 6 March 2017.
Search date: 7 March 2017.
Search strategy
# | Searches |
1 | exp breast tumor/ |
2 | exp breast/ |
3 | exp neoplasm/ |
4 | 2 and 3 |
5 | (breast* adj5 (neoplasm* or cancer* or tumo?r* or carcinoma* or adenocarcinoma* or sarcoma* or dcis or ductal or infiltrat* or intraductal* or lobular or medullary)).ti. |
6 | (mammar* adj5 (neoplasm* or cancer* or tumo?r* or carcinoma* or adenocarcinoma* or sarcoma* or dcis or ductal or infiltrat* or intraductal* or lobular or medullar)).ti. |
7 | 1 or 4 or 5 or 6 |
8 | socioeconomics/ |
9 | quality adjusted life year/ |
10 | quality adjusted life.tw. |
11 | (qaly$or qald$or qale$or qtime$).tw. |
12 | disability adjusted life.tw. |
13 | daly$.tw. |
14 | health survey/ |
15 | (sf36 or sf 36 or short form 36 or shortform 36 or sf thirtysix or sf thirty six or shortform thirtysix or shortform thirty six or short form thirtysix or short form thirty six).tw. |
16 | (sf6 or sf 6 or short form 6 or shortform 6 or sf six or sfsix or shortform six or short form six).tw. |
17 | (sf12 or sf 12 or short form 12 or shortform 12 or sf twelve or sftwelve or shortform twelve or short form twelve).tw. |
18 | (sf16 or sf 16 or short form 16 or shortform 16 or sf sixteen or sfsixteen or shortform sixteen or short form sixteen).tw. |
19 | (sf20 or sf 20 or short form 20 or shortform 20 or sf twenty or sftwenty or shortform twenty or short form twenty).tw. |
20 | (euroqol or euro qol or eq5d or eq 5d).tw. |
21 | (hql or hqol or h qol or hrqol or hr qol).tw. |
22 | (hye or hyes).tw. |
23 | health$year$equivalent$.tw. |
24 | health utilit$.tw. |
25 | (hui or hui1 or hui2 or hui3).tw. |
26 | disutili$.tw. |
27 | rosser.tw. |
28 | quality of wellbeing.tw. |
29 | qwb.tw. |
30 | willingness to pay.tw. |
31 | standard gamble$.tw. |
32 | time trade off.tw. |
33 | time tradeoff.tw. |
34 | tto.tw. |
35 | or/8-34 |
36 | 7 and 35 |
37 | systematic review/ |
38 | meta-analysis/ |
39 | (meta analy* or metanaly* or metaanaly*).ti,ab. |
40 | ((systematic or evidence) adj3 (review* or overview*)).ti,ab. |
41 | (reference list* or bibliograph* or hand search* or manual search* or relevant journals).ab. |
42 | (search strategy or search criteria or systematic search or study selection or data extraction).ab. |
43 | (search* adj4 literature).ab. |
44 | (medline or pubmed or cochrane or embase or psychlit or psyclit or psychinfo or psycinfo or cinahl or science citation index or bids or cancerlit).ab. |
45 | cochrane.jw. |
46 | ((multiple treatment* or indirect or mixed) adj2 comparison*).ti,ab. |
47 | or/37-46 |
48 | 36 and 47 |
49 | limit 48 to yr=“2011 -Current” |
Web of Science Core Collection databases
Date range searched: 1900–search date.
Search date: 7 March 2017.
Science Citation Index Expanded
Date range searched: 1900–search date.
Search date: 7 March 2017.
Conference Proceedings Citation Index – Science
Date range searched: 1900–search date.
Search date: 7 March 2017.
Search strategy
# | Searches |
# 1 | TI=((breast* NEAR/5 (neoplasm* or cancer* or tumo?r* or carcinoma* or adenocarcinoma* or sarcoma* or dcis or ductal or infiltrat* or intraductal* or lobular or medullary))) |
# 2 | TI=((mammar* NEAR/5 (neoplasm* or cancer* or tumo?r* or carcinoma* or adenocarcinoma* or sarcoma* or dcis or ductal or infiltrat* or intraductal* or lobular or medullar))) |
# 3 | #2 OR #1 |
# 4 | TS=(qol or “quality of life” or “quality adjusted life” or qaly* or qald* or qale* or qtime* or “disability adjusted life” or daly*)OR TS=(sf36 or sf 36 or short form 36 or shortform 36 or sf thirtysix or sf thirty six or shorform thirtysix or shortform thirty six or short form thirtysix or short form thirty six) OR TS=(sf 6 or sf6 or short form 6 or shortform 6 or sf six or sfsix or shortform six or short form six) OR TS=(sf12 or sf 12 or short form 12 or shortform 12 or sf twelve or sftwelve or shortform twelve or short form twelve) OR TS=(sf16 or sf 16 or short form 16 or shortform 16 or sf sixteen or sfsixteen or shortfrom sixteen or short form sixteen) OR TS=(sf20 or sf 20 or short form 20 or shortform 20 or sf twenty or sftwenty or shortform twenty or short form twenty) OR TS=(euroqol or euro qol or eq5d or eq 5d or hql or hqol or h qol or hrqol or hr qol or disutilit* or rosser “quality of wellbeing” or qwb or “willingness to pay” or “standard gamble*” or “time trade off” or “time tradeoff” or tto) |
# 5 | #4 AND #3 |
# 6 | TS=(meta-analysis or meta analy* or metaanaly*) OR TS=(“review literature” or “literature review”) OR TS=(“systematic review*” or “systematic overview*”) OR TS=(cochrane or embase or psychlit or psyclit or psychinfo or psycinfo or cinahl or cinhal or science citation index or bids or cancerlit) OR TS=(“reference list*” or bibliograph* or hand-search* or “relevant journals” or “manual search*”) OR TS=((“selection criteria” or “data extraction”) and review) |
# 7 | #6 AND #5 |
Indexes=SCI-EXPANDED, CPCI-S Timespan=2011-2017 |
Health Technology Assessment Database: Centre for Reviews and Dissemination
Date range searched: 1995–2016.
Search date: 7 March 2017.
NHS Economic Evaluation Database: Centre for Reviews and Dissemination
Date range searched: 1995–2015.
Search date: 7 March 2017.
Search strategy
# | Searches |
1 | MeSH DESCRIPTOR Breast Neoplasms EXPLODE ALL TREES |
2 | MeSH DESCRIPTOR Neoplasms, Ductal, Lobular, and Medullary EXPLODE ALL TREES |
3 | MeSH DESCRIPTOR Breast EXPLODE ALL TREES |
4 | MeSH DESCRIPTOR Neoplasms EXPLODE ALL TREES |
5 | #3 AND #4 |
6 | (((breast* ADJ5 (neoplasm* or cancer* or tumor* or tumour* or carcinoma* or adenocarcinoma* or sarcoma* or dcis or ductal or infiltrat* or intraductal* or lobular or medullary)))):TI |
7 | (((mammar* ADJ5 (neoplasm* or cancer* or tumor* or tumour* or carcinoma* or adenocarcinoma* or sarcoma* or dcis or ductal or infiltrat* or intraductal* or lobular or medullar)))):TI |
8 | #1 OR #2 OR #5 OR #6 OR #7 |
9 | (#8) IN HTA FROM 2011 TO 2017 |
10 | (#8) IN NHSEED FROM 2011 TO 2017 |
EuroQol 5-Dimensions and breast cancer
Epub Ahead of Print, In-Process & Other Non-Indexed Citations, Ovid MEDLINE(R) Daily and Ovid MEDLINE(R)
Date range searched: 1946 to present.
Search date: 10 July 2017.
Search strategy
# | Searches |
1 | exp Breast Neoplasms/ |
2 | exp mammary neoplasms/ |
3 | exp “Neoplasms, Ductal, Lobular, and Medullary”/ |
4 | exp breast/ |
5 | exp neoplasms/ |
6 | 4 and 5 |
7 | (breast* adj5 (neoplasm* or cancer* or tumo?r* or carcinoma* or adenocarcinoma* or sarcoma* or dcis or ductal or infiltrat* or intraductal* or lobular or medullary)).ti. |
8 | (mammar* adj5 (neoplasm* or cancer* or tumo?r* or carcinoma* or adenocarcinoma* or sarcoma* or dcis or ductal or infiltrat* or intraductal* or lobular or medullar)).ti. |
9 | 1 or 2 or 3 or 6 or 7 or 8 |
10 | (euroqol or euro qol or eq5d or eq 5d).tw. |
11 | 9 and 10 |
EMBASE
Date range searched: 1974 to 7 July 2017.
Search date: 10 July 2017.
Search strategy
# | Searches |
1 | exp breast tumor/ |
2 | exp breast/ |
3 | exp neoplasm/ |
4 | 2 and 3 |
5 | (breast* adj5 (neoplasm* or cancer* or tumo?r* or carcinoma* or adenocarcinoma* or sarcoma* or dcis or ductal or infiltrat* or intraductal* or lobular or medullary)).ti. |
6 | (mammar* adj5 (neoplasm* or cancer* or tumo?r* or carcinoma* or adenocarcinoma* or sarcoma* or dcis or ductal or infiltrat* or intraductal* or lobular or medullar)).ti. |
7 | 1 or 4 or 5 or 6 |
8 | (euroqol or euro qol or eq5d or eq 5d).tw. |
9 | 7 and 8 |
Web of Science Core Collection databases
Date range searched: 1900–search date.
Search date: 10 July 2017.
Science Citation Index Expanded
Date range searched: 1900–search date.
Search date: 10 July 2017.
Conference Proceedings Citation Index – Science
Date range searched: 1900–search date.
Search date: 10 July 2017.
Search strategy
# | Searches |
# 1 | TI=((breast* NEAR/5 (neoplasm* or cancer* or tumo?r* or carcinoma* or adenocarcinoma* or sarcoma* or dcis or ductal or infiltrat* or intraductal* or lobular or medullary))) |
# 2 | TI=((mammar* NEAR/5 (neoplasm* or cancer* or tumo?r* or carcinoma* or adenocarcinoma* or sarcoma* or dcis or ductal or infiltrat* or intraductal* or lobular or medullar))) |
# 3 | #2 OR #1 |
# 4 | TOPIC: (euroqol or euro qol or eq5d or eq 5d) |
# 5 | #4 AND #5 |
Appendix 3 Table of excluded studies with rationale
Type of exclusion | Reason for exclusion | References |
---|---|---|
Population exclusions |
|
40176,210–248 |
Intervention exclusions |
|
27249–275 |
Comparator exclusions |
|
3276–278 |
Outcome exclusions |
|
146279–424 |
Study type exclusions |
|
34121,425–457 |
Other reasons for exclusions |
|
2458,459 |
|
128460–586 |
Appendix 4 Summary of the clinical review
This appendix provides a summary of results for all included tests, ordered by type of evidence. For the sake of clarity, this section focuses on LN0 and LN+ subgroups only, DRFI/DRFS outcomes and key points. Full descriptions and discussions of the evidence base are reported in Chapter 2, Report Supplementary Material 1–10, and Appendix 5, and should be read in conjunction with this summary to obtain a full understanding. The derivation cohorts are excluded from the summary (i.e. three US cohorts for oncotype DX,49 TransATAC46 for IHC4 and IHC4+C, TransATAC and NSABP B-14 pooled44 for RSPC, van ‘t Veer et al. 587 for MammaPrint, van de Vijver et al. 79 for Prosigna and Filipits et al. 117 for EndoPredict), except in the case of IHC4+C, as only the derivation data reported numerical values.
Risk classification
Lymph node negative
Among studies of LN0 patients receiving endocrine monotherapy, percentages categorised as high risk ranged from 9% to 33% across all five tests (Table 57): 9–33% for oncotype DX (three studies46,49,50,52,60,61), 29% for MammaPrint (one study91), 15–20% for Prosigna/ROR-PT (three studies46,104,105,112), 27% for EPClin (one study46,118–120), 9% for IHC4+C (derivation cohort46) and not reported for IHC4. Within studies with variable endocrine therapy and chemotherapy use, percentages categorised as high risk were similar to the above for oncotype DX (25–28%), but higher for MammaPrint (33–73%); this may reflect the selection of higher-risk patients for MammaPrint studies (some not ER+, some required chemotherapy).
Test | Number of studies with DRFS/DRFI | Population | Nodal status | ET/chemotherapy | Percentage of patients per group | Percentage with 10-year DRFS/DRFI risk | Significantly prognostic for DRFS/DRFI? | Additional value over clinicopathological factors or tests?a | Chemotherapy benefit? | ||||
---|---|---|---|---|---|---|---|---|---|---|---|---|---|
Low risk | Intermediate risk | High risk | Low risk | Intermediate risk | High risk | ||||||||
LN0, all ET, no chemotherapy | |||||||||||||
Oncotype DX | 346,49,52,60,61 | ER+; HER2+/– | LN0 | All ET; no chemotherapy | 48–64 | 20–27 | 9–33 | 93–97 | 86–100 | 70–77 | Yes (3 of 4 studies, NR in 1) | Yes (3 studies) | Weakb |
MammaPrint | 191 | ER+; HER2 NR | LN0 | All ET; no chemotherapy | 71 | – | 29 | 93 | 85 | NR | NR | ||
Prosigna/ROR-PT | 346,104,105,112 | Most ER+; HER2– | LN0 | All ET; no chemotherapy | 48–55 | 30–32 | 15–20 | 95–97 | 87–93 | 69–85 | Yes (3 of 3 studies) | Yes vs. CTS and NPI (2 studies) | N/A |
EPClin | 146,118–120 | ER+; HER2– | LN0 | All ET; no chemotherapy | 73 | – | 27 | 94 | 80 | Yes (1 study) | Yes vs. CTS and NPI (1 study) | N/A | |
IHC4 | 2 cohorts126 | ER+; HER2– NR | LN0 | All ET; no chemotherapy | NR | NR | NR | NR | NR | NR | NR | Yes (2 cohorts) | N/A |
IHC4+C | 146 (derivation) | ER+; 95% HER2– | LN0 | All ET; no chemotherapy | 70 | 21 | 9 | 96 | 82 | 77 | Yes (1 study) | Yes vs. CTS and NPI (1 study) | N/A |
LN0, variable ET/chemotherapy | |||||||||||||
Oncotype DX | 250,58 | ER+; HER2+/– | LN0 | 75–100% ET; 79–100% chemotherapy | 49–51 | 21–26 | 25–28 | 9650 | 8950 | 8850 | Yes (1 of 1 study) | NR | NR |
Oncotype DX RSPC | 144 | ER+; HER2– NR | LN0 | All ET; 64% chemotherapy | NR | NR | NR | NR | NR | NR | Yes (1 analysis) | Yes (derivation set) | No (1 study) |
MammaPrint | c779,84,86,88,91,95,96 | 70–100% ER+; HER2 NR | LN0 | 0–25% ET/chemotherapy | 27–67 | – | 33–73 | 80–90 | 50–71 | Yes (4 of 7 studies, 1 not significant, NR in 2) | Yes (pooled study, 2 cohorts, others NR) | Not statistically significant (pooled LN0/LN+)b | |
IHC4 | 2125,127 | ER/HER2 varies | Some ET/chemotherapy | Clinical cut-off points not used | NR | NR | NR | Yes (some analyses non-significant) | NR | N/A | |||
LN+, all ET, no chemotherapy | |||||||||||||
Oncotype DX | 146 | ER+; HER2– | LN1–3 | All ET; no chemotherapy | 57 | 32 | 11 | 82 | 75 | 67 | Yes (1 study) | No vs. CTS and NPI | Weakb |
Prosigna/ROR-PT | 346,104,105,112 | Most ER+; HER2– | LN1–3 (most) | All ET; no chemotherapy | 4–25 | 27–34 | 48–62 | 100–100 | 81–94 | 71–78 | Yes or borderline (3 studies) | Yes vs. CTS, no vs. NPI | NR |
EPClin | 146,118–120 | ER+; HER2– | LN1–3 | All ET; no chemotherapy | 24 | – | 76 | 95 | – | 72 | Yes (1 study) | Yes vs. CTS and NPI at 10 years (not 5 years) | NR |
IHC4 | 2 cohorts126 | ER+; HER2– NR | LN+ | All ET; no chemotherapy | NR | NR | NR | Mixed (1 yes, 1 no) | NR | ||||
IHC4+Cd | 146 | ER+; HER2– | LN1–3 | All ET; no chemotherapy | 28 | 34 | 38 | 96 | 75 | 67 | Yes (1 study) | No vs. CTS and NPI | NR |
LN+, variable ET/chemotherapy | |||||||||||||
Oncotype DX | 351,52,56,59 | ER+; HER2+/– | LN+ | 74–100% ET/chemotherapy | 36–39 | 30–34 | 30–31 | 81e | 65e | 59e | Yes | Yes | N/A |
MammaPrint | 285,89 | 80% ER+; 84% HER2 or NR | LN1–3; LN > 3, 26% | Some ET/chemotherapy | 38–41 | – | 59–62 | 79–91 | 54–76 | Yes (2 of 2 studies) | Borderline (1 study) | Not statistically significant (pooled LN0/LN+)b | |
Prosigna/ROR-PT | 1108,109 | ER+; HER2– | LN > 3, 36% | All ET; all chemotherapy | 19 | 56 | 26 | 92 | 74 | 66 | Yes (1 study) | NR | NR |
EPClin | 1108,109 | ER+; HER2– | LN > 3, 36% | All ET; all chemotherapy | 13 | – | 87 | 100 | 72 | Yes (1 study) | NR | NR | |
IHC4 | 2123,136 | HR+; HER2– | LN+ | ET varies; 100% chemotherapy | Clinical cut-off points not used | No clinical groups | NR | Mixed (1 yes, 1 no) | NR |
Lymph node positive
Three tests (Prosigna/ROR-PT, EPClin and IHC4+C) categorised far more LN+ than LN0 patients as high risk among studies of endocrine monotherapy (see Table 57): 48–62% for Prosigna/ROR-PT (three studies46,104,105,112), 76% for EPClin (one study46,118–120) and 38% for IHC4+C (one study46). Conversely, oncotype DX categorised similar percentages of LN+ and LN0 patients as high risk (11% for LN+; one study46). For MammaPrint, there were no LN+ endocrine monotherapy studies, but in studies with variable endocrine therapy and chemotherapy use, 59–62% were high risk (two studies85,89); this was similar to LN0 studies.
For tests with three categories, how many patients would be prescribed chemotherapy would depend on how intermediate patients are handled (see Table 57).
Prognostic performance and additional prognostic value
Oncotype DX
Seven reanalyses of RCTs37,46,49–56 and four retrospective cohort studies57–60 were included (total n = 4929). The 10-year DRFI rates for LN0 low-risk patients were 93–97% (with endocrine monotherapy); for intermediate-risk patients they were somewhat higher (86–100%). LN+ patients were generally at higher risk of recurrence than LN0 patients in both low and intermediate categories (10-year DRFI for LN+ was < 85% for low-risk patients and ≤ 75% for intermediate-risk patients). Unadjusted analyses indicated that oncotype DX was prognostic (statistically significant differences between low-risk and high-risk groups) across various recurrence outcomes regardless of lymph node status, although HRs between intermediate-risk and high- or low-risk groups were not always statistically significant. Oncotype DX provided additional prognostic information over most commonly used clinicopathological variables (age, grade, size and nodal status) regardless of lymph node status, and over CTS and NPI in LN0 (but not LN+) patients, but analyses used a 50-point or 1-point difference rather than categories defined by the 18–30 cut-off points.
Oncotype DX RSPC
One study44 derived the RSPC score in a meta-analysis of two RCT data sets (LN0/LN+; n = 1735) and validated it in another (LN0; n = 625), which included 233 patients used to derive the oncotype DX Breast Recurrence Score. Based on the derivation analysis set, the oncotype DX RSPC algorithm (oncotype DX plus age, tumour size and grade) appeared to provide additional prognostic information over oncotype DX and over clinicopathological variables, and was able to classify more patients into a low-risk category than oncotype DX while maintaining a roughly equivalent rate of distant recurrence in the low-risk group. In the validation cohort, RSPC had prognostic value in univariate analyses (no adjusted analysis was reported). However, RSPC has only been validated in one independent cohort that included patients who were used to derive one of its constituent parts (oncotype DX Breast Recurrence Score), and has not been tested in premenopausal or LN+ patients.
MammaPrint
The prognostic value of MammaPrint is based on nine retrospective analyses (total n = 1805), four pooled analyses (n = 964; including six of the nine series above) and one reanalysis of a RCT (n = 538). Studies were variable in terms of nodal status, ER status and receipt of endocrine therapy and chemotherapy. MammaPrint was statistically significantly prognostic for 10-year DRFS in almost all unadjusted analyses of LN0 and LN+ patients. For LN0 patients, 10-year DRFS/DRFI rates for low-risk patients ranged from 80% to 90% (with varying rates of endocrine therapy and chemotherapy use), while the reanalysis of a RCT reported 10-year DRFS of 93% with endocrine monotherapy and 83% without endocrine therapy or chemotherapy. For LN+ patients, 10-year DRFS rates in low-risk patients ranged from 79% to 91% (with varying rates of endocrine therapy and chemotherapy use). In terms of additional prognostic value, MammaPrint was statistically significantly prognostic for 10-year DRFS/DRFI in multivariable analyses adjusted for clinicopathological risk tools (AOL and NPI) and various combinations of clinicopathological variables in LN0/LN+ and LN0 cohorts, while adjusted analyses in LN+ cohorts were statistically significant or borderline significant.
Prosigna/risk of recurrence based on Prediction Analysis of Microarray 50 subtype information plus proliferation score plus tumour size
Based on six reanalyses of RCTs and two retrospective analyses of prospective cohorts (total n = 9118), Prosigna/ROR-PT was statistically significantly prognostic for unadjusted analyses of 10-year DRFS/DRFI in LN0 and LN+ patients. The 10-year DRFS/DRFI rates for low-risk patients were 95% to 97% in three studies of LN0 patients (endocrine monotherapy), and in LN+ patients these were 100% in two studies (endocrine monotherapy) and 92% in one study (all endocrine therapy and chemotherapy). For intermediate-risk patients, 10-year DRFS/DRFI rates were 87% to 93% for LN0 and 81% to 94% for LN+ (endocrine monotherapy). Prosigna/ROR-PT added prognostic information over clinicopathological variables or CTS/CLP/NPI in three studies; this was statistically significant in LN0 patients and either significant or borderline significant in LN+ patients.
EndoPredict and EndoPredict Clinical
Based on three reanalyses of RCTs (total n = 3135) in ER+ HER2– endocrine-treated patients, EPClin was statistically significantly prognostic for unadjusted analyses of 10-year DRFS/DRFI in LN0 and LN+ patients. The 10-year DRFS/DRFI rates for low-risk patients were approximately 95% in LN0 and LN+ patients receiving endocrine therapy only. EPClin added statistically significant information over CTS/NPI in LN0 and LN+ patients in TransATAC, while in two further studies, the EndoPredict score added statistically significant information over clinicopathological variables in mixed LN0/LN+ and LN+ patients (no data for EPClin).
IHC4
The IHC4 has been validated in five reanalyses of RCTs and six retrospective cohort studies (total n = 13,434) and provides statistically significant prognostic information consistently in unadjusted analyses in LN+/LN0, LN0 and LN+ groups. However, most studies used quartiles or tertiles to define risk groups, which are specific to each cohort and do not allow conclusions to be drawn about which cut-off points should be used in clinical practice and how these would perform. Many used laboratory methods that differed from the derivation study methodology. Only one validation study126 used the cut-off points from the original analysis,25 and provides second and third validation cohorts (BCS and TEAM). IHC4 had additional prognostic value over clinicopathological factors in some studies. Test methodologies did not appear to have an impact on the statistical significance of results, but concerns remain about the conduct of the test in laboratories other than that used to derive the score.
IHC4+C
The IHC4+C had prognostic value in one validation cohort (Nottingham) in which statistical significance was maintained after adjustments for clinicopathological factors.
Microarray studies
Microarray studies are defined here as those that applied a test algorithm to either in silico data (microarray gene expression data held on a database) or a de novo microarray assessment. These studies support conclusions from studies using the commercial versions of the assays in suggesting that oncotype DX, MammaPrint and EndoPredict can discriminate between high- and low-risk patients regardless of LN status.
Outcomes in low-risk and intermediate-risk groups
Lymph node negative
Among studies of LN0 patients receiving endocrine monotherapy, the 10-year DRFS/DRFI rates in low-risk groups were similar across all five tests (see Table 57): 93% to 97% for oncotype DX (four studies46,49,50,52,60,61), 93% for MammaPrint (one study91), 95% to 97% for Prosigna/ROR-PT (three studies46,104,105,112), 94% for EPClin (one study46,118–120) and 96% for IHC4+C (one study46). Intermediate-risk groups for oncotype DX, Prosigna/ROR-PT and IHC4+C had worse DRFS/DRFI rates than low-risk groups (EPClin and MammaPrint do not have intermediate-risk groups). Many studies of MammaPrint included some ER– patients, did not treat all patients with endocrine therapy, and treated some with chemotherapy; for these studies, 10-year DRFS/DRFI rates in low-risk groups were 80% to 90% (seven studies79,84,86,88,91,96).
Lymph node positive
Among studies of LN+ patients receiving endocrine monotherapy (see Table 57), 10-year DRFS/DRFI rates in low-risk groups were less favourable for oncotype DX (82%; one study46) than for Prosigna/ROR-PT (100%; two studies46,104,105), EPClin (95%; one study46,118–120) or IHC4+C (96%; one study46). There were no studies of MammaPrint in this population. Intermediate-risk patients had lower DRFS/DRFI than low-risk patients for oncotype DX (75%, one study46), Prosigna/ROR-PT (81% to 94%, two studies46,104,105) and IHC4+C (75%, one study46). For MammaPrint, the only LN+ data were in populations that included some ER– patients, did not treat all patients with endocrine therapy, and treated some with chemotherapy; 10-year DRFS/DRFI rates in low-risk groups were 79% to 91% (two studies85,89).
Chemotherapy benefit
Evidence of chemotherapy benefit was only assessed for oncotype DX, oncotype DX RSPC and MammaPrint. There was no chemotherapy benefit evidence for EndoPredict or EPClin, Prosigna/ROR-PT, IHC4 or IHC4+C.
Oncotype DX and oncotype DX RSPC
Analyses were reported in five studies. 50,53,61,64–67,70 Two were reanalyses of RCTs (one LN0,50,61 one LN+,53 total n = 1018) in which patients were randomised to endocrine monotherapy, or endocrine therapy plus chemotherapy. Three were observational studies64–67,70 (total approximately 44,000 with some double-counting, two LN0,64,65,67,70 one LN+/LN066) in which patients were treated in accordance with usual practice and their oncotype DX score. The two reanalyses of RCTs suggest that benefit from chemotherapy is highest in oncotype DX high-risk patients. Unadjusted interaction tests between oncotype DX risk group and chemotherapy benefit were mainly statistically significant. Adjusted interaction tests were borderline significant in the NSABP-B20 cohort (significant in HER2– patients), whereas in SWOG-8814 they were significant when adjusted for some clinicopathological variables individually, but not when adjusting for ER determined by Allred status. However, part of the NSABP-B20 cohort was the derivation cohort for oncotype DX and this may bias results in favour of observing an interaction. The RSPC algorithm (oncotype DX plus age, tumour size and grade) showed a non-significant interaction test between chemotherapy benefit and RSPC risk group,44 indicating that the incorporation of clinicopathological factors may reduce prediction of chemotherapy benefit, and therefore if chemotherapy decisions are based on an informal consideration of clinicopathological factors alongside the oncotype DX score, this may reduce the predictive ability of oncotype DX in clinical practice. Three observational cohort studies were at high risk of confounding; one reported a statistically significant interaction test adjusted for limited clinical factors. If predictive ability were assumed, it is unclear below which exact cut-off point patients could avoid chemotherapy (although one study suggests that this is a recurrence score of 20), as chemotherapy benefit is uncertain in the intermediate-risk group. Although the ongoing RCT TAILORx will address the issue of whether or not low-risk and intermediate-risk patients can avoid chemotherapy, it is unclear to what extent it will address the question of whether or not the test can predict chemotherapy benefit. The EAG considers that there remains uncertainty surrounding whether or not oncotype DX is associated with a predictive benefit of chemotherapy (i.e. a difference in relative effect by genomic risk group) and, if so, that there is uncertainty in the likely magnitude of this predictive effect within the clinical subgroups considered in this appraisal.
MammaPrint
Prediction of chemotherapy benefit for MammaPrint was reported in a pooled analysis of six non-randomised series (n = 541; half LN0, half LN1–3) in which patients were treated in accordance with usual practice. The effect of chemotherapy versus no chemotherapy was statistically significant in the MammaPrint high-risk group but not in the low-risk group in unadjusted analyses for 5-year DRFS and BCSS and in adjusted analyses for 5-year BCSS. However, the interaction test between chemotherapy treatment and risk group (for 5-year BCSS) was non-significant (p = 0.45). A further pooled analysis of two of the above series, restricted to LN1–3 patients, also reported a statistically non-significant interaction between chemotherapy treatment and risk group for 10-year BCSS (p = 0.95). The evidence for the ability of MammaPrint to predict chemotherapy benefit is therefore extremely limited; although unadjusted analyses suggest a greater effect of chemotherapy in high-risk groups, adjusted analyses were only reported for one outcome, and the non-significant interaction tests suggest no statistically significant difference in effect of chemotherapy between risk groups.
Clinical utility
Clinical utility is defined in this assessment as the impact of tests used prospectively in clinical practice on recurrence/survival outcomes. Studies assessing prospective use of tests were only available for oncotype DX and MammaPrint, and only one RCT had reported in full (MINDACT for MammaPrint). There was no clinical utility evidence for EndoPredict or EPClin, Prosigna/ROR-PT, IHC4, IHC4+C or oncotype DX RSPC.
Oncotype DX
Without the highest level of evidence (RCT of treatment guided by a test vs. treatment guided by usual practice), it is not possible to conclude whether or not patient outcomes would be affected by the use of the test in a clinical setting. In LN0 patients, the use of the test in clinical practice appears to result in low rates of chemotherapy use in low-risk patients (2% to 12%), with acceptable outcomes (5-year DRFS/DRFI/IDFS of 96% to 99.6%). Rates of chemotherapy use increased with increasing risk category, and were generally higher in LN+ patients; only one study reported 5-year DRFS/DRFI/IDFS for LN+ patients, which was 97% (7% received chemotherapy). It was not possible to determine whether or not patients in intermediate- and high-risk categories had better outcomes than low-risk patients as a result of using oncotype DX, owing to the observational nature of the studies.
MammaPrint
Two studies reported evidence relating to the clinical utility of MammaPrint. MINDACT is a RCT of MammaPrint versus clinical practice. This study randomised patients with discordant MammaPrint and mAOL risks to chemotherapy or no chemotherapy. For patients who were high-clinical, low-MammaPrint risk, 5-year DMFS was 95.9% with chemotherapy and 94.4% without chemotherapy, a non-significant absolute difference of 1.5% (p = 0.267). This raises the possibility of avoiding chemotherapy in these patients. In patients who were low-clinical, high-MammaPrint risk, 5-year DMFS was 95.8% with chemotherapy and 95.0% without chemotherapy, an absolute difference of 0.8%. This could be interpreted as showing that MammaPrint may not be useful in this group as it would increase chemotherapy rates without improving outcomes. However, the comparator was mAOL, and it is unclear whether or not the same would be true for other clinical risk scores.
RASTER is a prospective observational study in which patients were treated in accordance with MammaPrint plus usual clinical practice (LN0) or in accordance with usual clinical practice (LN+). The 5-year DRFI for LN0 patients was 97.0% for low-risk patients (15% had chemotherapy) and 91.7% for high-risk patients (81% received chemotherapy). The 10-year DRFI for LN0 patients was 93.7% for low-risk patients and 86.8% for high-risk patients. The DRFI rates in the MammaPrint low-risk group may be considered sufficiently low for these patients to avoid chemotherapy.
Decision impact
Decision impact studies assess how decisions to use or not to use chemotherapy change pre and post use of the test. Only decision impact studies from the UK and Europe were included, because other countries may have very different rates of chemotherapy use. The percentage of patients with any change in treatment recommendation or decision (either to or from chemotherapy) among UK studies was 29% to 49% across four oncotype DX studies, 37% in one EndoPredict study and 27% in one IHC4+C study. Ranges across European (non-UK) studies were 5% to 70% for oncotype DX, 38% to 41% for EndoPredict, 14% to 41% for Prosigna and 13% to 51% for MammaPrint. The net change in the percentage of patients with a chemotherapy recommendation or decision (patients changing to chemotherapy minus those changing to no chemotherapy) among UK studies was a reduction of 8% to 23% across four oncotype DX studies, an increase of 1% in one EndoPredict study and a reduction of between 2% and 26% in one IHC4+C study (unclear owing to category definitions). Net changes across European (non-UK) studies were a reduction of 0% to 64% for oncotype DX, a reduction of 13% to 26% for EndoPredict, a reduction of 2% to an increase of 9% for Prosigna and a reduction of 31% to an increase of 8% for MammaPrint.
Concordance
Concordance is defined in this review as the degree to which tests assign the same patients to the same risk groups. Concordance analyses do not report long-term outcomes. A full review of these data was beyond the scope of this review and, instead, the OPTIMA Prelim study588 was included as a key example of concordance between tests and included oncotype DX, MammaPrint, Prosigna and IHC4. The authors concluded, that although tests assigned similar proportions of patients to low/intermediate- and high-risk categories, test results for an individual patient could differ markedly depending on which test was used.
Anxiety and health-related quality of life
Six studies (seven publications)159,164,166,174–177 reported outcomes relating to anxiety (including worry and distress) and HRQoL. For oncotype DX (two studies, n = 286),174,176 EndoPredict (one study, n = 149)159 and Prosigna (two studies, n = 398),163,166 all studies had a pre–post test design, whereas MammaPrint compared patients subgrouped in accordance with their clinical risk, MammaPrint risk, whether or not they were assigned to chemotherapy and whether or not the MammaPrint test result was missing. 177 Across tests, and when reported, state anxiety decreased post test and total FACT-G scores generally stayed the same. However, without a comparator group it is not possible to tell if anxiety would have reduced post treatment decision regardless of how the decision was made. Emotional and functional well-being in FACT-G improved in one study,166 and FACT-B scores improved for some subgroups in one study. 177
Time-to-test results
One study181 of 263 US patients reported that the percentage having a delay of ≥ 42 days from surgery to chemotherapy initiation was 31% for patients for whom an oncotype DX test was ordered, compared with 20% for other patients. In another study, the median handling time was 3 working days (range 0–11 days), and 59% of tests were conducted within 3 days. 161
Appendix 5 Results: all tests compared with each other
This appendix provides an overview of three types of studies that allow some form of comparison between tests:
-
Studies reporting more than one test – these are studies in which two or more of the tests were conducted and patient outcomes were reported, such that the prognostic performance of two or more tests in the same cohort can be compared. Very few studies conduct formal comparisons between tests.
-
Microarray studies – these are studies in which the commercial version of the tests was not conducted; rather, test algorithms were applied to genetic profiles obtained using microarray techniques. Mostly, these are publicly available in silico (electronic database) genetic profiles, complete with patient outcome data. As with the studies that report more than one test, the comparisons provided are not always formal.
-
Concordance in risk categorisation between tests – focusing on the OPTIMA Prelim study.
Studies reporting more than one test
Prognostic performance: studies assessing multiple tests
Few studies assessed multiple tests in the same cohort. This section of the report focuses on how the tests compare with each other in terms of prognostic performance. Evidence is often limited and formal statistical comparisons are often lacking.
Study designs: studies assessing multiple tests
Data were reported for six cohorts (Table 58). Four studies were reanalyses of RCTs (TransATAC;46 ABCSG 6 and ABCSG 8118–120 and ABCSG 8 only;104,105 GEICAM 9906;108,109 and WSG PlanB73,74,77). The most comprehensive analysis in terms of the number of tests compared was the translational research analysis of UK-based patients from the ATAC589 trial (TransATAC; see Report Supplementary Material 1), which assessed four tests: EndoPredict, Prosigna, oncotype DX and IHC4+C. Analyses were reported across 10 publications,25,36–44 but none reported only ER+, HER2–, LN0–3 patients. In this section of the report, we use the reduced TransATAC data set (only including patients with a result for all four tests), whereas we have used the data from the full analysis set (where all patients with the relevant test were included) in the sections relating to each of the tests individually (see Chapter 2). A pooled analysis of 1702 patients from the ABCSG 6 and ABCSG 8 trials assessing EndoPredict (EndoPredict and EPClin) was reported by Dubsky et al. 119,120 plus subgroup analyses submitted to NICE by Myriad Genetics,117,118 and an analysis of 1397 patients from ABCSG 8 only assessed Prosigna (Gnant et al. 104 and Filipits et al. 105). Because these two analyses have a large overlap (the majority of patients are from ABCSG 8), they are used here to compare EndoPredict and Prosigna. Finally, 555 patients from the Spanish GEICAM 9906 trial were analysed for EndoPredict (EndoPredict and EPClin) and Prosigna by Martin et al. 108,109 WSG PlanB73,74,77 was a reanalysis of RCT data from Germany, but has limitations in its use for assessing prognostic performance (discussed in the next paragraph).
Study (first author and year) | Cohort(s) | Number of patients | Country | Study design | Test | Details of test | Cut-off points | Population | Nodal status | ET/chemotherapy |
---|---|---|---|---|---|---|---|---|---|---|
Sestak 2017 (data requested)46 | TransATAC | 774 | UK | R-RCT | EPClin |
FFPE RT-qPCR |
3.3 |
ER+ HER2– Postmenopausal 100% female |
LN0, 76% LN1–3, 24% |
ET 5 years No chemotherapy |
Onoctype DX |
FFPE Genomic Health |
18–30 | ||||||||
Onoctype DX RSPC |
FFPE Genomic Health |
10 year DR risk < 10%, 10–20%, > 20% | ||||||||
Prosigna |
FFPE NanoString Technologies nCounter |
LN0: 41–60 LN+: 16–40 |
||||||||
IHC4+C |
FFPE Cuzick et al. 201125 |
10–20 | ||||||||
Dubsky 2013,119,120 Myriad Genetics118 | ABCSG 6 and ABCSG 8 | 1702 (all) | Austria | R-RCT |
EndoPredict EPClin |
FFPE RT-qPCR |
5 3.3 |
ER+ HER2– Postmenopausal Stage I–II 100% female |
LN0, 68% LN1–3, 27% LN > 3, 5% |
ET 5 years No chemotherapy |
Gnant 2014,104 Filipits 2014105 | ABCSG 8 | 1397 | Prosigna |
FFPE nCounter |
LN0: 40–60 LN1–3: 15–40 LN > 3: all high |
ER+ HER2– Postmenopausal 100% female |
LN0, 71%a LN1–3, 26%a LN > 3, 3%a |
|||
Martin 2016,108 Martin 2014109 | GEICAM 9906 | 555 | Spain | R-RCT |
EndoPredict EPClin |
FFPE RT-qPCR |
5 3.3 |
ER+ HER2– 46% postmenopausal Stage II–III 100% female |
All LN+ LN1–3, 64% LN > 3, 36% |
Adjuvant chemotherapy (FEC/FEC-P) ET 5 years |
Prosigna | RT-qPCR, then microarray | 18–65 | ||||||||
Russell 201657 | University of South Florida; Morton Plan Hospital | 135 | USA | Observational, RPWT | Onoctype DX | NR | NR |
100% ER+ HER2– NR Menopausal NR Female NR |
NR | NR – RPWT |
MammaPrint | NR | |||||||||
Nitz 2017,77 Gluz 201673,74 | WSG PlanB | 2642 | Germany | R-RCT | Onoctype DX |
NR Genomic Health |
25th–75th percentile |
HR+ HER2– Premenopausal/postmenopausal 100% female High clinical riskb |
LN0–3 LN0 58.8% LN1–3 41.2% |
RS < 12, ET monotherapy; RS ≥ 12, chemotherapy plus ETc |
IHC4 |
PE IHC4 Prat et al. 2013590 Cuzick et al. 201125 |
25th–75th percentile | ||||||||
Gong 201658 | SYSMH; CCSYU; 3rd HNC | 153 | China | Observational, RPWOT | Onoctype DX |
FFPE Multiplex branched-DNA liquid chip technology (SurExam, Guangzhou, China) |
NR |
100% HR+ 100% HER2– 61% postmenopausal % female NR Non-metastatic |
LN0 |
100% ET 79% chemotherapy |
IHC4 |
IHC4 Cuzick et al. 201125 |
25th–75th percentile |
The three remaining studies (Russell et al. ,57 WSG PlanB73,74,77 and Gong et al. ;58 see Table 58) all had limitations. Russell et al. 57 was an observational study of oncotype DX and MammaPrint in which patients were treated in accordance with MammaPrint results, and is therefore confounded as a prognostic study as chemotherapy treatment is likely to have differed across risk groups. However, as there were no other data that compared MammaPrint with other tests, except from microarray studies (see Appendix 5 and Report Supplementary Material 10), it has been included as the next available level of evidence. Two studies (WSG PlanB73,74,77 and Gong et al. 58) that both have limitations were included because they compared oncotype DX with IHC4, and the only other data (apart from microarray studies) that compare oncotype DX with IHC4 are from the IHC4 derivation cohort (TransATAC). WSG PlanB73,74,77 was a reanalysis of RCT data from Germany and was included as a clinical utility study for oncotype DX (see Chapter 2, Clinical utility: oncotype DX) as patients were not treated with chemotherapy when the recurrence score was < 12, and as a prognostic study for IHC4 (see Section 2.7.2). Gong et al. 58 is an observational study in which patients were treated in accordance with usual practice and it was not clear if this included the test result, and the assay used was not the commercial version of oncotype DX.
As the TransATAC analysis is key to this assessment and compares the most in-scope tests (n = 4), to simplify the write-up we have structured this section of the report around the TransATAC data and compared other data with these, or used other data to provide comparative data when TransATAC data are lacking. The section contents are:
-
TransATAC46 comparing oncotype DX, EPClin, Prosigna and IHC4+C.
-
EndoPredict compared with EPClin (n = 2 studies, ABCSG 6 plus ABCSG 8; and GEICAM 9906). 108,109,118–120
-
EPClin compared with Prosigna (n = 3 studies, TransATAC; GEICAM 9906; ABCSG 6 plus ABCSG 8 or ABCSG 8). 42,104,105,108,109,118–120
-
Oncotype DX compared with MammaPrint (n = 1 study57). The limitations of Russell et al. 57 are discussed in the following section.
-
Oncotype DX compared with IHC4 or IHC4+C (n = 3 studies46,58,73,74,77). The limitations of WSG PlanB73,74,77 and Gong et al. 58 are discussed in the following section.
Patients and treatments: studies assessing multiple tests
Patient characteristics and details of the treatments received are presented in Table 58. Six of the seven data sets consisted of, or had analyses available for, ER+, HER2– patients,46,58,73,74,77,104,105,108,109,118–120 whereas Russell et al. 57 consisted of all ER+ patients, but did not report the proportion who were HER2–. 57 In terms of nodal status, one study was in LN0 patients only,58 one study was in LN+ patients only108,109 and one did not report nodal status. 57 Three data sets included node-negative and node-positive patients (TransATAC, ABCSG 6 plus ABCSG 8 and WSG PlanB104,105,118–120). In GEICAM 9906,108,109 36% of participants had more than three positive nodes; in ABCSG 6 plus ABCSG 8,118–120 5% had more than three positive nodes. In WSG PlanB, patients were at clinically high risk, defined as LN+ or LN0 with a risk factor (CpT2, grade 2/3, high uPA/PAI-1, < 35 years). 73,74,77 Patients in all analyses received 5 years of endocrine therapy, apart from those in the study by Russell et al. ,57 in which this was not reported, and Gong et al. ,58 in which 100% of participants received endocrine therapy, but the duration was not reported. Patients in the GEICAM 9906 analysis108,109 also received adjuvant chemotherapy; Russell et al. 57 did not report how many patients received chemotherapy; WSG PlanB73,74,77 patients with a recurrence score of ≥ 12 received chemotherapy; and 79% of patients in Gong et al. 58 received chemotherapy.
Tests and comparators: studies assessing multiple tests
Details of the tests conducted and the cut-off points applied are presented in Table 58. All data sets that included EndoPredict and Prosigna assessed EndoPredict as marketed, using RT-qPCR and standard cut-off points for risk groups (5 for EndoPredict and 3.3 for EPClin). In two analyses (TransATAC and ABCSG 6 plus ABCSG 8104,105), Prosigna was assessed using the nCounter device and cut-off points of 40 and 60 (LN0) or 15 and 40 (LN1–3), while GEICAM 9906108,109 used a ‘research-based non-standardised’ PAM50 ROR-PT assay, using RT-qPCR then microarray rather than nCounter, with cut-off points of 18 and 65 (LN+). Russell et al. 57 did not report how oncotype DX and MammaPrint were obtained. WSG PlanB73,74,77 ordered oncotype DX from Genomic Health and conducted IHC4 tests according to Prat et al. 590 and Cuzick et al. ,25 and used 25th to 75th percentiles as cut-off points for oncotype DX and IHC4. Gong et al. 58 conducted oncotype DX assays using SurExam (Guangzhou, China) and IHC4 according to Cuzick et al. ,25 also using 25th to 75th percentiles as cut-off points.
Comparators in TransATAC39,46 included the CTS score and NPI. ABCSG 6 plus ABCSG 8118–120 compared AOL with EndoPredict.
Quality assessment: studies assessing multiple tests
A summary of the quality of the studies is presented in Table 59. Two data sets (TransATAC and ABCSG 8 or 6 + 8)41,46,104,105,118–120 were reanalyses of RCTs in which no patients received chemotherapy and all received adjuvant endocrine therapy. Two (GEICAM and WSG PlanB)73,74,77,108,109 were reanalyses of RCTs in which some patients received chemotherapy, and two57,58 were observational studies in which patients were either treated in accordance with routine practice but it was not clear if the test results were known,58 or were treated in accordance with routine practice including a test result (MammaPrint). 57 None of the studies reported including all relevant patients, meaning that there is a risk of bias and the generalisability of the cohort to the decision problem is uncertain. Test assessors were blind to patient outcomes in four studies. 46,73,74,77,104,105,108,109 All used standardised outcomes. Two studies58,108,109 used assays that were not the same as the commercially marketed version of the test: Prosigna not using nCounter in one study;108,109 oncotype DX performed by SurExam and the IHC4 process was not clear in one study. 58
Study (first author and year) | Cohort(s) | Derivation or validation? | Study design appropriate? | All eligible patients included? | Blinding (of test assessors to outcomes)? | Outcome definition standardised or a priori? | Applicability: patient spectrum | Applicability: test as per decision problem? |
---|---|---|---|---|---|---|---|---|
Sestak 2017 (data request)46 | TransATAC | V | Yes, R-RCT, no chemotherapy | No; InT, FT | Yes | Yes | Yes | Yes |
Dubsky 2013,119,120 Myriad Genetics118 | ABCSG 6 plus ABCSG 8 | V | Yes, R-RCT, no chemotherapy | UC | UC | Yes | N, includes 5% LN > 3 | Yes |
Gnant 2014,104 Filipits 2014105 | ABCSG 8 | V | Yes, R-RCT, no chemotherapy | No; InT, MS, TF | Yes | Yes | Yes (for subgroup analysis) | Yes |
Martin 2016,108 2014109 | GEICAM 9906 | V | No, R-RCT, adjuvant chemotherapy | No (reason NR) | Yes | Yes | N (36% LN > 3) | No, Prosigna via RT-qPCR then microarray |
Nitz 2017,77 Gluz 2016,73,74 | WSG PlanB | V | No, some chemotherapy | No, MS | Yes | Yes | Yes, but high risk | Yes |
Russell 201657 | University of South Florida; Morton Plan Hospital | V | No, cohort study, usual practice (some chemotherapy) | No; InT, sent for test | UC | Yes | N; InT | Yes |
Gong 201658 (n = 611) | SYSMH; CCSYU; 3rd HNC | V | No, some chemotherapy | No; InT; MD | UC | Yes | N, InT, MD, chemotherapy | No – oncotype DX algorithm, but used SurExam assay |
Results: studies assessing multiple tests
Tables 60–63 present the data for all patients (node positive or node negative) and separate data for node-positive and node-negative patients.
Study (first author and year) | Cohort(s), design, country | Population | Nodal status | ET/chemotherapy | Test | Percentage of patients per group | Percentage risk | DRFI/DMFS/DRFS:a HR (95% CI) | |||||||
---|---|---|---|---|---|---|---|---|---|---|---|---|---|---|---|
0–5 years | 0–10 years | ||||||||||||||
Low | Intermediate | High | Low | Intermediate | High | Low | Intermediate | High | |||||||
Node negative and node positive | |||||||||||||||
Sestak 2017 (data request)46 (reduced data set)b | TransATAC, R-RCT, UK | ER+ HER2–, (n = 774) |
|
|
EPClin | 61 | – | 39 | – | – | – | – | – | – | 0–10 years: 4.65 (2.98 to 7.24) |
Oncotype DX | 62 | 28 | 10 | – | – | – | – | – | – | 0–10 years:
|
|||||
Prosigna | 43 | 30.5 | 26.5 | – | – | – | – | – | – | 0–10 years:
|
|||||
IHC4+C | 57 | 26 | 17 | – | – | – | – | – | – | 0–10 years:
|
|||||
Dubsky 2013,119,120 Myriad Genetics118 | ABCSG 6 plus ABCSG 8, R-RCT, Austria | ER+ HER2–, (n = 1702) |
|
|
EndoPredict | 49 | – | 51 | NR | – | NR |
0–5 years: 2.80 (1.81 to 4.34); p < 0.001 5–10 years: 3.28 (1.48 to 7.24); p = 0.002 |
|||
EPClin | 63 | – | 37 | 95.3 | – | NR |
0–5 years: 4.82 (3.12 to 7.44); p < 0.001 0–10 years: 5.11 (3.48 to 7.51); p < 0.001 5–10 years: 6.25 (2.72 to 14.36); p < 0.001 |
||||||||
Gnant 2014,104 Filipits 2014105 | ABCSG 8, R-RCT, Spain | ER+ HER2–, (n = 1397) |
|
Prosigna | 35 | 32 | 33 | 96.6 | 91.1 | 79.9 | 5–15 years: | ||||
Nitz 2017,77 Gluz 201673,74 | WSG PlanB, Germany | HR+ HER2–, (n = 2642) |
|
RS < 12 ET; RS ≥ 12, chemotherapy and ETd | Oncotype DX | 17e | 58e | 21e | 93.6d | 94.3d | 84.2d | 0–5 years: 2.33 (1.73 to 3.14); p < 0.001 | |||
IHC4 | NR | NR | NR | NR | NR | NR | 0–5 years: 2.04 (1.47 to 2.83); p < 0.001 | ||||||||
Russell 201657 | University of South Florida, Morton Plan Hospital, USA | ER+, NR HER2–, (n = 135) | NR | NR | Oncotype DX | 53 | 26 | 21 | Log-rank 0–5 years:
|
||||||
MammaPrint | 63 | 72 | Log-rank, 0–5 years: p = 0.032 | ||||||||||||
Node negative | |||||||||||||||
Sestak 2017 (data request)46 (reduced data set)b | TransATAC, R-RCT, UK | ER+ HER2–, (n = 591) | LN0 |
|
EPClin | 73 | – | 27 | 97.6 | – | 91.2 | 93.4 | – | 77.9 |
0–5 years: 3.76 (1.67 to 8.46) 0–10 years: 3.88 (2.31 to 6.52) |
Oncotype DX | 63 | 26 | 10 | 98.8 | 92.1 | 86.8 | 94.1 | 83.3 | 72.8 | 0–5 years:
|
|||||
Oncotype DX RSPC | 73 | 19 | 9 | 98.3 | 91.4 | 86.2 | 93.8 | 80.2 | 70.5 | 0–5 years:
|
|||||
Prosigna | 54 | 30 | 16 | 98.4 | 95.4 | 88.1 | 97.0 | 85.9 | 67.6 | 0–5 years:
|
|||||
IHC4+C | 66 | 23 | 11 | 98.4 | 93.2 | 85.7 | 94.7 | 79.8 | 74.6 | 0–5 years:
|
|||||
Gnant 2014,104 Filipits 2014105 | ABCSG 8, R-RCT, Austria | ER+ HER2–, (n = 984) | LN0 |
|
Prosigna | 48 | 32 | 20 | 96.5 | 90.0 | 84.7 | 5–15 years: | |||
Gong 201658 | SYSMH, CCSYU, 3rd HNC, China | ER+ HER2–, (n = 153) | LN0 |
|
Oncotype DX | 49 | 26 | 25 | 0–10 years C-index (AUC): 0.685 (0.540 to 0.830) | ||||||
IHC4 | 29 | 48 | 23 | 0–10 years C-index (AUC): 0.602 (0.436 to 0.767) | |||||||||||
Node positive | |||||||||||||||
Sestak 2017 (data request)46 (reduced data set)b | TransATAC, R-RCT, UK | ER+ HER2–, (n = 183) | LN1–3 |
|
EPClin | 23 | – | 77 | 97.7 | – | 86.7 | 94.4 | – | 69.7 |
0–5 years: 5.89 (0.79 to 44.15) 0–10 years: 6.58 (1.59 to 27.27) |
Oncotype DX | 57 | 32 | 11 | 95.1 | 82.0 | 79.7 | 80.6 | 70.9 | 62.0 | 0–5 years:
|
|||||
Prosigna | 8 | 32 | 60 | 100.0 | 91.1 | 86.8 | 100.0 | 79.3 | 69.3 | 0–5 years:
|
|||||
IHC4+C | 27 | 34 | 39 | 98.0 | 88.5 | 83.8 | 95.2 | 71.6 | 64.3 | 0–5 years:
|
|||||
Gnant 2014,104 Filipits 2014105 | ABCSG 8, R-RCT, Austria | ER+ HER2–, (n = 413) |
|
Prosigna | 4 | 34 | 62 | 100 | 93.6 | 76.2 | 5–15 years:
|
||||
Martin 2016,108 2014109 | GEICAM 9906, R-RCT, Spain | ER+ HER2–, (n = 536) |
|
|
EndoPredict | 25 | – | 75 | 93 | – | 69 | 0–10 years: 4.7 (CI NR), p < 0.0001 | |||
EPClin | 13 | – | 87 | 100 | – | 71 | 0–10 years: not estimable; p < 0.0001 | ||||||||
ROR-PT (research) | 19 | 56% | 26 | 92 | 74 | 66 | 0–10 years:
|
Study (first author and year) | Cohort(s), design, country | Population | Nodal status | ET/chemotherapy | Test | Percentage of patients per group | OS at 5 years | OS at 10 years | HR, low vs. high (95% CI) | ||||||
---|---|---|---|---|---|---|---|---|---|---|---|---|---|---|---|
Low | Intermediate | High | Low | Intermediate | High | Low | Intermediate | High | |||||||
Node negative and node positive | |||||||||||||||
Sestak 201746 (reduced data set)a | TransATAC, R-RCT, UK | ER+ HER2–, (n = 774) |
LN0, 76% LN1–3, 24% |
|
EPClin | 61 | – | 39 | – | – | – | – | – | – | 0–10 years: 2.15 (1.65 to 2.80) |
Oncotype DX | 62 | 28 | 10 | – | – | – | – | – | – | 0–10 years:
|
|||||
Prosigna | 43 | 30.5 | 26.5 | – | – | – | – | – | – | 0–10 years:
|
|||||
IHC4+C | 57 | 26 | 17 | – | – | – | – | – | – | 0–10 years:
|
|||||
Node negative | |||||||||||||||
Sestak 201746 (reduced data set)a | TransATAC, R-RCT, UK | ER+ HER2–, (n = 591) | LN0 |
|
EPClin | 73 | – | 27 | 92.8 | – | 88.9 | 79.6 | – | 63.3 |
0–5 years: 1.56 (0.87 to 2.79) 0–10 years: 2.06 (1.46 to 2.89) |
Oncotype DX | 63 | 26 | 10 | 94.4 | 87.7 | 85.2 | 80.7 | 69.2 | 55.8 | 0–5 years:
|
|||||
Oncotype DX RSPC | 73 | 19 | 9 | 94.5 | 87.3 | 84.1 | 80.7 | 69.4 | 50.5 | 0–5 years:
|
|||||
Prosigna | 54 | 30 | 16 | 93.4 | 92.7 | 84.1 | 83.6 | 72.7 | 51.7 | 0–5 years:
|
|||||
IHC4+C | 66 | 23 | 11 | 94.9 | 85.3 | 85.7 | 82.2 | 63.1 | 57.3 | 0–5 years:
|
|||||
Node positive | |||||||||||||||
Sestak 201746 (reduced data set)a | TransATAC, R-RCT, UK | ER+ HER2–, (n = 183) | LN1–3 |
|
EPClin | 23 | – | 77 | 95.3 | – | 81.4 | 72.9 | – | 57.8 |
0–5 years: 4.28 (1.02 to 18.03) 0–10 years: 1.99 (1.02 to 3.91) |
Oncotype DX | 57 | 32 | 11 | 90.4 | 77.6 | 75.0 | 66.8 | 58.1 | 44.4 | 0–5 years:
|
|||||
Prosigna | 8 | 32 | 60 | 100.0 | 87.9 | 80.8 | 90.0 | 70.1 | 52.9 | 0–5 years:
|
|||||
IHC4+C | 27 | 34 | 39 | 94.0 | 85.3 | 77.5 | 77.4 | 62.9 | 49.1 | 0–5 years:
|
|||||
Martin 2016,108 2014109 | GEICAM 9906, R-RCT, Spain | ER+ HER2–, (n = 536) |
LN1–3, 64% LN > 3, 36% |
|
EndoPredict | 25 | 75 | 92 | 6 | 0–10 years: 3.9 (2.0 to 7.5); p < 0.0001 | |||||
EPClin | 13 | 87 | 99 | 69 | 0–10 years: 19.4 (2.7 to 138.7); p < 0.0001 |
Study (first author and year) | Cohort(s), design, country | Population | Nodal status | Outcome | Test or comparatora | Likelihood ratio χ2 | Increase in likelihood ratio χ2 over CTS/CLPa |
---|---|---|---|---|---|---|---|
Node negative and node positive | |||||||
Sestak 2017 (data request)46 (reduced data set)b | TransATAC, R-RCT, UK |
|
|
DRFI at 10 years | EPClin | 69.31 (p < 0.0001) |
Over CTS: 24.39 (p < 0.0001) Over NPI: 22.17 (p < 0.0001) |
Oncotype DX | 26.94 (p < 0.0001) |
Over CTS: 15.22 (p = 0.0001) Over NPI: 11.89 (p = 0.0006) |
|||||
Prosigna | 61.47 (p < 0.0001) |
Over CTS: 26.30 (p < 0.0001) Over NPI: 23.91 (p < 0.0001) |
|||||
IHC4+C | 75.30 (p < 0.0001) |
Over CTS: 20.07 (p < 0.0001) Over NPI: 22.84 (p < 0.0001) |
|||||
Gnant 2014,104 Filipits 2014105 | ABCSG 8, R-RCT, Austria |
|
|
DRFS at 10 years | Prosigna | Over CLP: 29.94 (p < 0.0001) | |
Node negative | |||||||
Sestak 2017 (data request)46 (reduced data set)b | TransATAC, R-RCT, UK |
|
LN0 | DRFI at 10 years | EPClin | 40.60 (p < 0.0001) |
Over CTS: 15.22 (p = 0.0001) Over NPI: 17.00 (p < 0.0001) |
Oncotype DX | 22.78 (p < 0.0001) |
Over CTS: 10.64 (p = 0.001) Over NPI: 8.82 (p = 0.003) |
|||||
Oncotype DX RSPC | 24.30 (p < 0.0001) |
Over CTS: 5.10 (p = 0.02) Over NPI: 8.71 (p = 0.003) |
|||||
Prosigna | 50.77 (p < 0.0001) |
Over CTS: 23.71 (p < 0.0001) Over NPI: 25.54 (p < 0.0001) |
|||||
IHC4+C | 48.55 (p < 0.0001) |
Over CTS: 17.14 (p < 0.0001) Over NPI: 21.92 (p < 0.0001) |
|||||
Gnant 2014,104 Filipits 2014105 | ABCSG 8, R-RCT, Austria |
|
LN0 | DRFS at 10 years | Prosigna | Over CLP: 20.32 (p < 0.0001) | |
Node positive | |||||||
Sestak 2017 (data request)46 (reduced data set)b | TransATAC, R-RCT, UK |
|
LN1–3 | DRFI at 10 years | EPClin | 12.91 (p < 0.001) |
Over CTS: 7.36 (p = 0.007) Over NPI: 5.57 (p = 0.02) |
Oncotype DX | 4.75 (p = 0.023) |
Over CTS: 3.56 (p = 0.06) Over NPI: 2.14 (p = 0.10) |
|||||
Prosigna | 8.51 (p = 0.004) |
Over CTS: 4.39 (p = 0.04) Over NPI: 2.71 (p = 0.09) |
|||||
IHC4+C | 12.60 (p < 0.001) |
Over CTS: 3.08 (p = 0.08) Over NPI: 2.45 (p = 0.10) |
|||||
Gnant 2014,104 Filipits 2014105 | ABCSG 8, R-RCT, Austria |
|
DRFS at 10 years | Prosigna | Over CLP: 17.45 (p = 0.0002) |
Study (first author and year) | Cohort(s), design, country | Population | Nodal status | Outcome | Test or comparatora | C-index (AUC) | Increase in C-index (AUC) over clinicopathological factorsa | Multivariable Cox proportional hazards model (adjusted for clinicopathological factorsa): HR (95% CI) |
---|---|---|---|---|---|---|---|---|
Node negative and node positive | ||||||||
Dubsky 2013,119,120 | ABCSG 6 plus ABCSG 8, R-RCT, Austria |
|
|
DMFS at 0–5 years | EndoPredict | 1.20 (1.10 to 1.31); p < 0.001 | ||
DMFS at 5–10 years | EndoPredict | 1.28 (1.10 to 1.48); p = 0.001 | ||||||
EPClin | 0.786 | |||||||
EndoPredict plus AOL | 0.765 | EndoPredict plus AOL vs. AOL: p < 0.001 | ||||||
EndoPredict plus clinicopathological factors | 0.716 | EndoPredict plus clinicopathological factors vs. clinicopathological factors: p < 0.001 | ||||||
AOL | 0.674 | |||||||
Clinicopathological factors | 0.644 | |||||||
Gnant 2014,104 Filipits 2014105 | ABCSG 8, R-RCT, Austria |
|
|
DRFS at 10 years | Prosigna | 0.720 | NR |
HR (intermediate vs. low) 2.15 (1.21 to 3.81); p = 0.009 HR (high vs. low) 4.26 (2.44 to 7.43); p < 0.0001 |
CLP | 0.688 | |||||||
Nitz 201773,74,77 (n = 2642) | WSG PlanB |
|
|
IDFSc | Oncotype DX | HR (25th to 75th percentile) 1.73 (1.21 to 2.47); p = 0.001 | ||
IHC4 | HR (25th to 75th percentile) NS | |||||||
Node negative | ||||||||
Gnant 2014,104 Filipits 2014105 | ABCSG 8, R-RCT, Austria |
|
|
DRFS at 10 years | Prosigna | 0.692 | NR | |
CLP | 0.639 | |||||||
Node positive | ||||||||
Gnant 2014,104 Filipits 2014105 | ABCSG 8, R-RCT, Austria |
|
DRFS at 10 years | Prosigna | 0.743 | NR | ||
CLP | 0.667 | |||||||
Martin 2016,108 2014109 | GEICAM 9906, R-RCT, Spain |
|
|
DMFS at 10 years | EPClin | 0.693 | Adding EPClin to ROR-PT plus clinicopathological factors: p < 0.001 | |
EndoPredict plus clinicopathological factorsa | 0.672 | EndoPredict plus clinicopathological factors vs. clinicopathological factors: p = 0.0018 | ||||||
EndoPredict | 0.657 | 1.1 (1.0 to 1.2); p = 0.003 | ||||||
Clinicopathological factorsa | 0.654 | |||||||
ROR-PT (research-based) | 0.644 | Adding ROR-PT to EPClin plus clinicopathological factors: p = 0.567 |
Prognostic performance
Distribution of patients by risk group, event rates (distant recurrence/relapse-free interval/distant metastasis-free survival/distant recurrence/relapse-free survival) and hazard ratios (unadjusted analyses)
This section reports unadjusted analyses. Adjusted analyses, which show whether or not the test has prognostic value over clinicopathological variables, are reported in Additional prognostic value.
TransATAC data
In the TransATAC cohort (see Table 60),42,46 the proportion of patients categorised as low risk was similar for oncotype DX, EPClin and IHC4+C (62%, 61% and 57%, respectively), and Prosigna placed the fewest patients in this group (43%). In the LN0 subgroup, the proportion in the low-risk group was generally higher (63% for oncotype DX, 73% for EPClin, 66% for IHC4+C, 54% for Prosigna and 73% for oncotype DX RSPC) than in the LN+ group (57%, 23%, 27% and 8%, respectively; oncotype DX RSPC not assessed in LN+). Notably, oncotype DX categorised a high proportion as low risk in both subgroups (63% and 57%, respectively), and Prosigna always reported the smallest proportion (54% and 8%, respectively). The 5-year event rates in low-risk LN0 patients were largely similar across tests, ranging from 97.6% (EPClin) to 98.8% (oncotype DX), but at 10 years Prosigna low-risk patients had the best DRFI rates (97.0%) with the other four tests having similar rates (93.4% to 94.7%). In LN+ patients, event rates in low-risk patients were more variable, with DRFI rates ranging from 95.1% (oncotype DX) to 100% (Prosigna) at 5 years, and 80.6% (oncotype DX) to 100% (Prosigna) at 10 years. Notably, oncotype DX consistently had the worst survival rates in low-risk patients at both time points, whereas Prosigna had the best. However, only 8% of patients were assigned low-risk status by Prosigna, whereas 57% were assigned low-risk status by oncotype DX. IHC4+C and EPClin assigned fewer patients (27% and 23%, respectively) to the low-risk group than oncotype DX, but more than Prosigna, and had event rates of 95.2% and 94.4%, respectively. 46
In LN0–3 patients, the HRs for 10-year DRFI between risk groups were all statistically significant, with HRs for low vs. high risk ranging from 4.41 (oncotype DX) to 12.40 (Prosigna). In LN0 patients, there was not a consistent pattern regarding whether HRs were greater at 0–5 years than at 0–10 years. All were statistically significant except the analysis comparing low- with high-risk patients for Prosigna at 5 years [2.91 (95% CI 0.95 to 8.89)]. The greatest HR was for the analysis of low- versus high-risk patients for oncotype DX [13.07 (95% CI 3.93 to 43.41)], and the lowest was for Prosigna at 5 years [2.91 (95% CI 0.95 to 8.89)]. When comparing LN0 with LN+ HRs, both EPClin and IHC4+C tests reported lower HRs in the LN0 subgroup than in the LN+ subgroup at 10 years, whereas oncotype DX reported higher HRs in the LN0 subgroup than in the LN+ subgroup. 46
EndoPredict versus EndoPredict Clinical
Results are presented in Table 60. Similar proportions of low-risk patients as seen in the TransATAC cohort were reported for EPClin in ABCSG 6 plus ABCSG 8 [63% LN0–3 (additional analyses were provided in confidence by the company but cannot be reported here)]. When comparing EndoPredict with EPClin in the ABCSG 6 plus ABCSG 8 cohort, EndoPredict placed fewer patients in the low-risk category [49% for LN0–3 (additional analyses were provided in confidence by the company but cannot be reported here)] than EPClin (63%). 118–120 Only DRFS rates for EPClin were reported, which for low-risk patients at 5 years were 95.3%. In contrast, in the LN+ study GEICAM 9906, EndoPredict placed more patients in the low-risk category (25%) than EPClin (13%), but event rates were lower in EPClin low-risk groups (100%) than in EndoPredict (93%). HRs for EPClin were higher than for EndoPredict [e.g. 0- to 5-year HR for low vs. high 4.82 (EPClin) and 2.80 (EndoPredict)]. 108,109
Prosigna versus EndoPredict Clinical
Results are presented in Table 60. For LN0–3 cohorts, data from ABCSG 6 plus ABCSG 8118–120 were consistent with TransATAC:46 Prosigna/ROR-PT placed a smaller proportion of patients in the low-risk group than EPClin in both cohorts (TransATAC and ABCSG 6 plus ABCSG 8/ABCSG 8),104,105,118–120 with 43% versus 61% (TransATAC) and 35% versus 63% (ABCSG trials), respectively. Additional analyses were provided in confidence by the company but cannot be reported here. This was also true in LN0 subgroups [54% vs. 73% (TransATAC)]. In LN+ subgroups, there were 8% versus 23% in TransATAC, although in the GEICAM 9906 data set,108,109 the direction was reversed, with 18% versus 13%, respectively. In LN0, patients were better in Prosigna/ROR-PT than EPClin (97%46 and 93.4%) in TransATAC. 105 In GEICAM 9906 (LN+), the direction of the event rates were reversed, at 92% and 100% at 10 years, respectively.
Oncotype DX versus MammaPrint
Results are presented in Table 60. Only one study reported data for both tests. 57 MammaPrint assigned a larger proportion of patients (63%) to the low-risk category than oncotype DX (53%) in the observational study by Russell et al. 57 Event rates were not reported, and only p-values for log-rank tests given, where both tests showed a statistically significant difference in DRFS at the p < 0.05 level for high- versus low-risk group comparisons.
Oncotype DX versus IHC4 and IHC4+C
Results are presented in Table 60. Two studies reported oncotype DX and IHC4 analyses [WSG PlanB73,74,77 (LN0–3 only) and Gong et al. 58 (LN0 only)], and both used quartiles to define boundaries for risk categories, making the comparisons of proportions in risk categories and event rates in risk categories of little relevance to the decision problem. For IHC4 only, Gong et al. reported C-indices (AUC; which analyse IHC4 and oncotype DX as continuous variables) in LN0 patients, which indicate that the two tests have similar prognostic performance [oncotype DX 0.685 (95% CI 0.540 to 0.830) and IHC4 0.602 (95% CI 0.436 to 0.767)].
TransATAC46 (LN0–3, LN0, LN+; see Table 60) reported oncotype DX and IHC+C (rather than IHC4 only), and reported similar proportions of patients in the low-risk group in LN0–3 patients (62% and 57%, respectively) and LN0 patients (63% and 66%, respectively) but not in LN+ patients (57% and 27%, respectively). In LN0 patients, 10-year DRFI was similar between the two tests in low-risk patients (94.1% and 94.7%, respectively). 46 HRs for low- versus high-risk patients were very similar at 5.83 compared with 6.06, respectively. 46 In LN+ patients, 10-year DRFI was better for IHC4+C (95.2%) than for oncotype DX (80.6%). 46
Impact of menopausal status
Patients were subgrouped in accordance with menopausal status (premenopausal or postmenopausal), in GEICAM 9906. 108,109 For EndoPredict, event rates in the low-risk groups were similar in premenopausal and postmenopausal patients (93% and 92%, respectively), although HRs were somewhat different, at 6.7 (p < 0.0001) and 3.3 (p = 0.069), respectively. For EPClin, DRFS rates in the low-risk groups were identical (100%). HRs between groups were not reported, but between-group differences were statistically significant.
Overall survival
Data relating to OS are reported in Table 61. Only TransATAC46 and GEICAM 9906108,109 report OS. For 0–10 years in LN0–3 groups,46 HRs are all statistically significant and for low-risk versus high-risk group comparisons range from 2.15 (EPClin) to 3.42 (Prosigna). In LN0 patients, HRs comparing low- with high-risk groups range from 2.06 (EPClin) to 3.64 (Prosigna). In LN+ groups, however, the low- to high-risk groups show more variation, ranging from 1.99 (EPClin) to 9.02 (Prosigna) in TransATAC, and 19.38 for EPClin in GEICAM 9906.
Additional prognostic value
This section reports adjusted analyses, which indicate the additional prognostic value of IHC4 over clinicopathological factors. The clinicopathological factors adjusted for vary from study to study, and are detailed in the footnotes to the tables.
Likelihood ratios
In TransATAC,46 additional prognostic value was assessed via increases in likelihood ratio χ2 for 10-year DRFI, for each test plus NPI or CTS, over NPI or CTS only (see Table 62). In LN0 patients, increases in likelihood ratio χ2 over CTS and NPI were statistically significant for all tests (p-values range from p = 0.02 to p < 0.0001). Prosigna, EPClin and IHC4+C all showed greater increases (range 15.22 to 25.54) than oncotype DX Breast Recurrence Score or oncotype DX RSPC (range 5.10 to 10.64; see Table 62). However, for LN+ patients, increases in likelihood ratio χ2 were much more modest (range 2.14 to 7.36 across all tests; greatest increase for EPClin), and were borderline statistically significant for all tests (p-values range from 0.10 to 0.007).
In ABCSG-8,104 likelihood ratios also showed a statistically significant increase for Prosigna over the CLP (same variables as CTS) in node-negative patients (p < 0.0001) and node-positive patients (p = 0.0002).
C-indices (area under the curve)
In node-positive patients in GEICAM 9906,108,109 the C-index was higher for EPClin (0.693) and EndoPredict (0.657) than for the research-based ROR-PT (0.644) (see Table 63), although the lack of p-values and/or CIs mean that it is unclear whether or not the difference in C-indices were statistically significant. Adding EPClin to ROR-PT plus clinical variables increased the statistical significance of the test of the C-index (C-indices not reported; p < 0.001). Conversely, adding ROR-PT to EPClin plus clinical variables did not increase the statistical significance of the test of the C-index (p = 0.567) (see Table 63), although this finding should be interpreted with caution owing to the non-standard ROR-PT assay.
In ABCSG 6 plus ABCSG 8, a C-index for EPClin was only reported for a mixed node-negative and node-positive population (including 5% with more than three positive nodes) and for years 5–10 (no data for years 0–5). 119 In this period, the C-index statistically significantly increased when adding EndoPredict to a combination of clinical variables or to AOL (both p < 0.001; see Table 63). In the ABCSG 8 analysis of Prosigna,104 C-indices were numerically higher for Prosigna (0.720) than for the CLP (0.688), but any statistical significance of the difference was not reported.
Multivariable Cox proportional hazards models
Both ABCSG 6 plus ABCSG 8118–120 and GEICAM 9906108,109 used multivariable analyses and showed that EndoPredict was an independent prognostic parameter for 10-year DMFS/DRFS after adjustment for clinical variables (see Table 63), and ABCSG 8104 showed a similar finding for Prosigna.
Discussion: studies assessing multiple tests
Few studies reported data from multiple tests and no study reported all comparisons of interest to the decision problem. Of most relevance to the decision problem was the TransATAC analysis,46 as this includes patients from the UK, analyses four of the five tests, reports ER+, HER2–, LN0–3 patients only, and provides change in likelihood ratios, which allows comparisons between tests to be made. However, the TransATAC data also has limitations: it is the derivation set for IHC4 and is therefore likely to be subject to some overfitting and overestimation of prognostic performance, only menopausal patients were recruited and MammaPrint was not tested. It is also only a single cohort and ideally all comparisons would be available in multiple independent cohorts. Data from other cohorts also have limitations: ABCSG 6 plus ABCSG 8118–120 only evaluated Prosigna for a proportion of patients (ABCSG 8);104,105 WSG PlanB recruited only high-risk patients, and patients were treated with chemotherapy in accordance with oncotype DX score;73,74,77 Russell et al. 57 was an observational study and reported only very limited study characteristics and analyses, Gong et al. 58 used non-standard test methods for oncotype DX and IHC4, and was conducted in a population of a different ethnic composition to the decision problem population; and GEICAM 9906108,109 included a high proportion of LN > 4 patients (36%) and used a non-standard ROR-PT assay.
As the data comparing the tests with each other are limited, so are the conclusions that can be drawn. Broad observations include that, generally speaking, the more patients who are placed in a low-risk category, the poorer the event-free survival for that group. For example, in LN0 patients in TransATAC,46 EPClin categorised 73% as low risk and these patients had a 10-year DRFI of 93.4%, and Prosigna categorised 54% as low risk and these patients had a 10-year DRFI of 97%. This effect was more pronounced in LN+ patients in TransATAC, among whom oncotype DX categorised 57% as low risk and these patients had a 10-year DRFI of 80.6%, and Prosigna categorised 8% as low risk and these patients had a 10-year DRFI of 100%. Another broad observation is that the tests generally perform differently in LN+ and LN0 patients. In TransATAC, both EPClin and IHC4+C tests reported lower HRs in the LN0 subgroup than in the LN+ subgroup at 10 years (EPClin LN0 HR 3.88 vs. LN+ HR 6.58; IHC4+C LN0 6.06 vs. LN+ 9.57), whereas oncotype DX reported higher HRs in the LN0 subgroup than the LN+ subgroup (oncotype DX LN0 HR 5.83 vs. LN+ HR 2.77). Data from other cohorts generally supported these broad observations.
In terms of how much additional prognostic information the tests provide over clinicopathological variables or algorithms (e.g. NPI, AOL, CTS), most data came from TransATAC,46 in which increases in likelihood ratio χ2 over CTS or NPI were statistically significant in LN0 patients across all tests (with Prosigna showing the greatest increase) and borderline significant for all tests in LN+ (with EPClin showing the greatest increase). One analysis108 suggested that EPClin could provide additional information over ROR-PT (plus clinicopathological variables), whereas ROR-PT could not provide additional information over EPClin (plus clinicopathological variables), but this was limited by the use of a non-standard version of ROR-PT. 108,109
Microarray studies
Microarray studies are defined, for the purposes of this review, as any study that applied a test algorithm (e.g. oncotype DX, MammaPrint) to either in silico data [microarray gene expression data held electronically, usually accessed from the National Centre for Biotechnology Information (NCBI) Gene Expression Omnibus (GEO)]68 or to a de novo microarray assessment conducted for the purpose of the study. These studies differ from studies that used the commercially offered assays in that the agreement between microarray and commercial assays is unknown, and, for this reason, the generalisability of the findings to the decision problem is also unknown.
It should be noted that some of the early MammaPrint studies were conducted using a 25,000-gene microarray platform, until the mini-array specific to the 70 MammaPrint genes was developed (see Chapter 2, Development: MammaPrint). To minimise heterogeneity between studies, MammaPrint studies conducted after the development of the mini-array that used wider microarray data are included here as ‘microarray studies’ rather than alongside studies using the mini-array (see Chapter 2, Results: MammaPrint).
Given the limitations of these studies in terms of analytic validity and owing to time constraints, we have conducted a rapid review rather than a full systematic review. This section of the report differs from other sections in that:
-
No quality assessment of studies has been conducted.
-
Data were not checked by a second reviewer.
It should also be noted that, owing to time and expertise constraints, the EAG was not able to fully consider the following factors:
-
The degree to which the same cohorts of patients are included in multiple studies. There is likely to be considerable overlap.
-
The quality of the methodology used to conduct the microarray analyses.
-
The cut-off points used across the studies.
-
The proportion of ER+ and HER2– patients in each cohort.
-
The proportion receiving endocrine therapy or chemotherapy in each cohort.
-
The ethnic composition of the cohorts used.
Further general limitations of the studies as a whole include:
-
A lack of clarity as to the characteristics of the patients.
-
A lack of clarity as to whether patients were treated with endocrine therapy or chemotherapy.
-
A lack of clarity as to whether patients were treated in accordance with a protocol or in accordance with routine practice, and whether or not the exclusion of patients who were treated would therefore lead to spectrum bias.
Some of this information may have been obtainable by reference to the GEO, or to the primary publications relating to each cohort, but due to time constraints these data were not sought.
Although acknowledging the considerable limitations of these studies and the review methodology, microarray studies hold some value as they report data on more than one test. This is important as there are very few studies using the commercial versions of the assays that report data for more than one test (see Appendix 5; Studies reporting more than one test). Specifically, there are few studies that report data for MammaPrint compared with any other test, meaning that it is difficult to assess the relative merits of this test compared with others.
For this reason, the review of these studies will focus on the information provided relating to the prognostic performance and additional prognostic value of the tests in comparison with each other, rather than on absolute values provided for individual tests, which may not be generalisable. It is of course entirely possible that such comparisons between tests are not generalisable, but given the lack of data comparing the commercial tests, the information provided has some value to the decision problem.
Microarray studies
A total of 18 studies591–608 reported data from microarray analyses (Table 64). Of these, five reported only data for one test (three reported oncotype DX593,599,600 and two reported MammaPrint592,607); the results of these studies are presented in Report Supplementary Material 10 but are not considered further. Of the remaining 13 studies, six591,594,596–598,602 reported seven cohorts of data from single institutions, five595,596,601,604,608 reported pooled in silico data from multiple cohorts, three603,605,608 reported data from METABRIC (Molecular Taxonomy of Breast Cancer International Consortium) (a UK–Canada data set), one606 analysed TRANSBIG [Translating molecular knowledge into early breast cancer management: building on the BIG (Breast International Group) network for improved treatment tailoring] data (an international collaboration of 22 countries) and one analysed four previously reported cohorts605 in addition to METABRIC.
First author, year, number of patients | Cohorts | Country | Tests | Population | Nodal Status | ET/chemotherapy | ||||
---|---|---|---|---|---|---|---|---|---|---|
Oncotype DX | EndoPredict | MammaPrint | Prosigna | Other tests | ||||||
Oncotype DX vs. MammaPrint vs. EndoPredict | ||||||||||
Finetti 2014,595 n = 1229 | 33 publicly available gene expression data sets from NCBI GEO database | NR | Yes | Yes | Yes |
|
NR (n = 1,299) 58% LN0 (% LN > 3 NR) (n = 3,074) |
NR | ||
Zhao 2014608 a) n = 912 a-i) n = 692 b) n = 996 |
a) GSE6532, GSE3494, GSE1456, GSE7390, GSE2603, E-TABM-158 b) METABRIC cohort |
Yes | Yes | Yes | Excludeda |
a) ER+ 76%, HER2– 85%a-i) ER+ 100%, HER2– NR b) ER+ NR, HER2– NR |
a) LN0 67% (LN > 3 NR) a-i) NR b) NR |
NR | ||
Oncotype DX vs. MammaPrint studies | ||||||||||
Ahn 2013591 a) n = 186 b) n = 82 |
Gananam Severance Hospital (1997–2007) | Korea | Yes | Yes |
100% ER+ 12% HER2+ a) all patients b) subset with RS 19–30 |
a) 47.8% LN+ (% LN > 3 NR) b) 43.9% LN+ (LN > 3 NR) |
a) 84% ET, 13% chemotherapy b) 94% ET, 82% chemotherapy |
|||
Fan 2006594 Microarray: a) n = 295 b) Subgroup n = 225 |
NKI (derivation cohort for MammaPrint) | The Netherlands | Yes | Yes | a) 77% ER+
|
a) LN0, 51% LN1–3, 36% LN > 3, 13% b) NR |
a) 14% ET, 37% chemotherapy b) NR |
|||
Jonsdottir, 2014,597 n = 94 | NR | Norway | Yes | Yes | a) ER+ NR
|
LN0 100% (% LN > 3 NR) |
a) 14% ET, 11% chemotherapy a-i) NR |
|||
Li 2009,598 n = 27 | Fudan University Cancer Hospital | China | Yes | Yes | HR+ NR, 70% HER2– | LN0 56% (% LN > 3 NR) | ET NR, 100% chemotherapy | |||
Győrffy 2015596 a) n = 3534 b) n = 325 |
a) 25 data sets from GEOb b) University Hospitals (Frankfurt and Hamburg) |
a) NR b) Germany |
Yes | Yes |
a) 83.1% ER+, 84.4% HER2+ NR Subgroup: 100% ER+, HER2– b) 81.1% ER+, HER2– NR Subgroup: i) 100% ER+; HER2– NR |
a) LN+ 30.8% b) LN+ 39.4% (LN > 3 NR) Subgroup: ER+, LN0 |
a) ET NR, 19% chemotherapy Subgroups: i) ER+, HER2–, untreated ii) ER+, HER2– treated b) ET and chemotherapy NR Subgroup: i) NR |
|||
Prat 2012,601 n = 594/1380 a) n = 339 b) n = 171 |
GSE17705, GSE6532, GSE12093, GSE1456, MDACC133 | NR | Yes | Yes | Excludeda |
ER+, HER2– NR (n = 549) 100% ER+ HER2– NR (n = 1380) |
NR (n = 549) LN0 47% (% LN > 3 NR) (n = 1380) a) LN0 100% b) LN+ 100% |
ET 100%, chemotherapy 0% | ||
Tobin 2014602 a) n = 253 b) n = 159 |
a) Uppsala cohort b) Stockholm cohort (Karolinska Hospital) |
Sweden | Yes | Yes |
HR+ NR HER2– NR Subgroup: a-i) ER+ 100% |
a) LN0 63% b) LN0 59% |
a) ET 58%, chemotherapy 11% b) ET 72%, chemotherapy 19% |
|||
Vollan 2015,603 n = 1412 | METABRIC | International | Yes | Yes | ER+ 100%, HER2– NR | NR | NR | |||
Xu 2017604 a) n = 917 |
a) METABRIC/Bioconductor data sets: GSE11121, GSE7390, GSE3494, GSE2990, Breast Cancer NKI | International | Yes | Yes | Excludeda | NPI | ER+ 100%, HER2– NR | LN0 100% | NR | |
Ou Yang 2014605 i) LN0 subgroup ii) ER+ subgroup a) n = 1981 (1037;1526) b) n = 216 (125; 134) c) n = 393 (250; 348) d) n = 115 (64; 66) e) n = 236 (158; 201) |
a) METABRIC b) Loi (GSE6532) c) Buffa (GSE22219) d) Wang (GSE19615) e) Miller (GSE3494) |
International; NR | Yes | Yes | Excludeda |
ER+: a) 77% b) 62% c) 89% d) 57% e) 85% Subgroup: i) ER+ NR ii) ER+ 100% HER2– NR |
LN0: a) 52% b) 58% c) 64% d) 56% e) 67% Subgroup: i) LN0 100%, ii) LN0 NR |
NR | ||
Yin, 2014,606 n = 198 | TRANSBIG GSE7390 | France, Sweden, UK | Yes | Yes |
AOL NPI |
ER+ NR, HER2– NR | LN0 100% | ET 0%, chemotherapy 0% |
All studies reported data on oncotype DX and MammaPrint, and two595,608 also reported data on EndoPredict. For the most part, only HRs for recurrence/survival rates between test risk groups were reported, which give an indication of the test’s association with an outcome, but these do not allow conclusions to be drawn about the prognostic ability of one test versus another. These data are presented in Table 65, and C-index (AUC) data are presented in Table 66, and data that provide direct comparisons of the prognostic performance of one test compared with another are presented in Table 67.
First author, year, number of patients | Cohorts | Population | Nodal status | ET/chemotherapy | Percentage of patients per group | Outcome | Test | Outcomes, HR (95% CI) unless stated otherwise | ||||
---|---|---|---|---|---|---|---|---|---|---|---|---|
Low | Inter | High | 0–5 years | 0–10 years | 5–10 years | |||||||
Oncotype DX and MammaPrint | ||||||||||||
LN0/LN+ | ||||||||||||
Fan 2006594 a) n = 295 |
NKI (derivation cohort for MammaPrint) | a) 77% ER+, HER2 NR | a) LN0, 51%, LN1–3, 36%, LN > 3, 13% | a) 14% ET, 37% chemotherapy | 24 | 11 | 65 | RFS | Oncotype DX | – | NR, p < 0.001 | – |
39 | – | 61 | RFS | MammaPrint | – | NR, p < 0.001 | – | |||||
24 | 11 | 65 | OS | Oncotype DX | – | NR, p < 0.001 | – | |||||
39 | – | 61 | OS | MammaPrint | – | NR, p < 0.001 | – | |||||
Győrffy 2015596 a) n = 3534 |
a) 25 data sets from GEO | a) 83.1% ER+, 84.4% HER2+ NR | a) LN+/LN0, LN+ 30.8% | a) ET NR, 19% chemotherapy | – | – | – | RFS | Oncotype DX | 2.55 (2.21 to 2.94; p < 0.001) | ||
– | – | – | MammaPrint | 3.40 (2.47 to 4.68; p < 0.001) | ||||||||
Győrffy 2015596 a-i) n = 672 |
Subgroups a-i and ii: 100% ER+, HER2– | a-i) Untreated | – | – | – | Oncotype DX | 2.82 (2.04 to 3.90; p < 0.001) | |||||
– | – | – | MammaPrint | 3.07 (1.87 to 5.04; p < 0.001) | ||||||||
Győrffy 2015596 a-ii) n = 1316 |
a-ii) Treated | – | – | – | Oncotype DX | 2.47 (2.14 to 3.49; p < 0.001) | ||||||
– | – | – | MammaPrint | 3.01 (1.85 to 4.90; p < 0.001 | ||||||||
Győrffy 2015596 b) n = 325 |
b) University Hospitals (Frankfurt and Hamburg) | b) ER+, HER2– NR | b) LN+/LN0 (LN > 3 NR) | b) ET and chemotherapy NR | – | – | – | Oncotype DX | 2.65 (1.73 to 4.07; p < 0.001) | |||
– | – | – | MammaPrint | 1.91 (1.05 to 3.50; p = 0.0322) | ||||||||
Tobin, 2014602 a) n = 253 |
a) Uppsala cohort | HR+ NR, HER2– NR | a) LN0 63% | a) ET 58%, chemotherapy 11% | 37 | 19 | 44 | BCSS | Oncotype DX |
21-year follow-up: HR continuous NR; p = 0.004 Intermediate vs. low: HR NR; p = 0.018 High vs. low: HR NR; p = 0.001 High/intermediate vs. low: 2.57 (1.43 to 4.62) |
||
51 | – | 49 | MammaPrint |
21-year follow-up: HR continuous NR; p = 0.005 High vs. low: 1.96 (1.21 to 3.17) |
||||||||
Tobin, 2014602 b) n = 159 |
b) Stockholm cohort (Karolinska Hospital) | HR+ NR, HER2– NR | b) LN0 59% | b) ET 72%, chemotherapy 19% | 31 | 16 | 53 | Oncotype DX |
Follow-up NR: HR NR; p = 0.006 High/intermediate vs. low: 2.87 (1.43 to 5.75) |
|||
48 | – | 52 | MammaPrint |
Follow-up NR: HR NR; p < 0.001 High vs. low: 4.61 (2.12 to 10.03) |
||||||||
Vollan, 2015603 n = 1412 |
METABRIC | ER+ 100%, HER2– NR | NR | NR | – | – | – | BCSS | Oncotype DX |
Follow-up NR Intermediate vs. low: 1.23 (0.91 to 1.68; p = 0.179) High vs. low: 2.35 (1.64 to 3.36; p < 0.001) |
||
– | – | – | MammaPrint |
Follow-up NR High vs. low: 1.25 (0.95 to 1.64; p = 0.11) |
||||||||
LN0 | ||||||||||||
Jonsdottir, 2014597 n = 94 |
NR – Norway | a) ER+ NR, 85% HER2– | LN0 100% | a) 14% ET, 11% chemotherapy | 19 | 45 | 36 | DRFS | Oncotype DX |
14-year HR Intermediate vs. low: 1.2 (0.3 to 4.4) High vs. low: 1.8 (0.5 to 6.5), p = 0.522 Rates: low, 83%; intermediate, 79%; high, 68% |
||
48 | – | 52 | MammaPrint |
14-year HR 1.6 (0.7 to 3.6; p = 0.287) Rates: low, 80%; high, 71% |
||||||||
Győrffy 2015596 b-i) n = 113 |
b) University Hospitals (Frankfurt and Hamburg) | Subgroup b-i: 100% ER+; HER2– NR | Subgroup b-i: ER+, LN0 | NR | – | – | – | RFS | Oncotype DX |
Oncotype DX Sensitivity 0.80 (0.76 to 0.82) Specificity 0.55 (0.53 to 0.58) Accuracy: 0.64 (0.62 to 0.65) MammaPrint Sensitivity 0.98 (0.96 to 0.98) Specificity 0.14 (0.12 to 0.16) Accuracy: 0.47 (0.46 to 0.47) |
||
Prat 2012601 a) n = 339 |
GSE17705, GSE6532, GSE12093, GSE1456, MDACC133 | 100% ER+, HER2– NR | a) LN0 100% | ET 100%, chemotherapy 0% | 14 | 19 | 67 | DRFS | Oncotype DX | Rates:
|
DRFS censored at 8.5 years Continuous: 1.97, p < 0.0001 High vs. Low: 3.79, p < 0.0023 |
|
40 | – | 60 | MammaPrint | Rates:
|
DRFS censored at 8.5 years Continuous: 1.42, p < 0.005 High vs. Low: 2.6, p = 0.0054 |
|||||||
Xu 2017604 a) n = 917 |
METABRIC/Bioconductor data sets: GSE11121, GSE7390, GSE3494, GSE2990, breastCancerNKI | ER+ 100%, HER2– NR | LN0 100% | NR | – | – | – | RFS | Oncotype DX | 15 years: 2.7 (95% CI NR; p < 0.001) | ||
– | – | – | MammaPrint | 15 years: 2.5 (95% CI NR; p < 0.001) | ||||||||
– | – | – | NPI | 15 years: 2.6 (95% CI NR; p < 0.001) | ||||||||
LN+ | ||||||||||||
Prat 2012601 b) n = 171 |
GSE17705, GSE6532, GSE12093, GSE1456, MDACC133 | 100% ER+, HER2– NR | b) LN+ 100% (% LN > 3 NR) | ET 100%, chemotherapy 0% | 8 | 12 | 80 | DRFS | Oncotype DX | Rates:
|
DRFS censored at 8.5 years Continuous: 1.51, p = 0.01 High vs. Low: 4.67, p = 0.01 |
|
31 | – | 69 | MammaPrint | Rates:
|
DRFS censored at 8.5 years Continuous: 1.26, p = 0.06 High vs. Low: 2.12, p = 0.03 |
|||||||
Oncotype DX and MammaPrint and EndoPredict | ||||||||||||
Finetti 2014595 n = 1229 |
33 publicly available gene expression data sets from NCBI GEO database | NR | LN+/LN0 | NR | – | – | – | DRFS | Oncotype DX |
Median follow-up: 7.8 years Intermediate vs. low: 1.82 (1.44 to 2.3; p < 0.001) High vs. low: 2.05 (1.59 to 2.63; p < 0.001) |
||
– | – | – | MammaPrint |
Median follow-up: 7.8 years 1.5 (1.21 to 1.85; p = 0.0002) |
||||||||
– | – | – | EndoPredict |
Median follow-up: 7.8 years 1.88 (1.52 to 2.32; p < 0.001) |
||||||||
Zhao 2014608 a-i) n = 692 |
a) GSE6532, GSE3494, GSE1456, GSE7390, GSE2603, E-TABM-158 | a-i) ER+ 100%, HER2– NR |
a) LN0 67% (LN > 3 NR) a-i) NR |
– | – | – | Oncotype DX | 1.79 (1.55 to 2.07; p < 0.0001) | 0.65 (0.26 to 1.61; p = 0.3535) | 1.06 (0.78 to 1.43; p = 0.7311) | ||
– | – | – | MammaPrint | 1.70 (1.43 to 2.03; p < 0.0001) | 1.06 (0.57 to 1.96; p = 0.8468) | 1.16 (0.87 to 1.55; p = 0.3054) | ||||||
– | – | – | EndoPredict | 1.97 (1.66 to 2.33; p < 0.0001) | 1.02 (0.55 to 1.91; p = 0.9462) | 1.13 (0.83 to 1.53; p = 0.4393) | ||||||
Zhao 2014608 b) n = 996 |
b) METABRIC cohort | b) ER+ NR, HER2– NR | b) NR | – | – | – | Oncotype DX | 1.94 (1.69 to 2.24; p < 0.0001) | 1.19 (0.86 to 1.65; p = 0.2856) | 1.11 (0.89 to 1.38; p = 0.3481) | ||
– | – | – | MammaPrint | 1.99 (1.63 to 2.41; p < 0.0001) | 1.21 (0.87 to 1.68; p = 0.2545) | 1.11 (0.89 to 1.38; p = 0.3514) | ||||||
– | – | – | EndoPredict | 1.96 (1.64 to 2.33; p < 0.0001) | 1.13 (0.82 to 1.55; p = 0.4593) | 1.29 (1.04 to 1.59; p = 0.0183 |
First author, year, number of patients | Cohorts | Population | Nodal status | ET/chemotherapy | Percentage of patients per group | Outcome | Test | Outcomes | ||
---|---|---|---|---|---|---|---|---|---|---|
Low | Intermediate | High | ||||||||
Unique cohorts | ||||||||||
Oncotype DX vs. MammaPrint | ||||||||||
LN0/LN+ | ||||||||||
Li 2009,598 n = 27 |
Fudan University Cancer Hospital | HR+ NR, 70% HER2– | LN0 56% | ET NR, 100% chemotherapy | – | – | – | DFS | Oncotype DX | 5-year C-index (AUC): 0.59; sensitivity 68%; specificity 50.0% |
– | – | – | MammaPrint | 5-year C-index (AUC): 0.691; sensitivity 72%; specificity 66.2% | ||||||
Studies drawing from more than one data source, with multiple overlaps between studies | ||||||||||
Oncotype DX vs. MammaPrint studies | ||||||||||
LN0/LN+ | ||||||||||
Ahn 2013591 b) n = 82 |
Gananam Severance Hospital |
100% ER+ 12% HER2+ b) subset with RS 19–30 |
b) 43.9% LN+ (LN > 3 NR) | b) 94% ET, 82% chemotherapy | OS | MammaPrint vs. Oncotype DX |
10 years Oncotype DX intermediate-risk (RS 19–30) group K–M curve: MammaPrint low vs. high: HR NR, p = 0.013 C-index (AUC) MammaPrint: 0.844 |
|||
Prat 2012601 n = 1380 |
GSE17705, GSE6532, GSE12093, GSE1456, MDACC133 | 100% ER+, HER2– NR | LN0 47% (% LN > 3 NR) | ET 100%, chemotherapy 0% | DRFS | Oncotype DX vs. MammaPrint |
8.5 years Increase in LR χ2 of oncotype DX over MammaPrint: 14.4, p < 0.001 Increase in LR χ2 of MammaPrint over oncotype DX: 9.2, p = 0.002 |
|||
Tobin 2014602 a) n = 253 |
a) Uppsala cohort | a-i) ER+ 100% | NR | NR | BCSS | Oncotype DX |
13 years C-index (AUC): 0.68 |
|||
MammaPrint |
13 years C-index (AUC): 0.81 |
|||||||||
Tobin 2014602 b) n = 159 |
b) Stockholm cohort (Karolinska Hospital) | HR+ NR, HER2– NR | b) LN0 59% | b) ET 72%, chemotherapy 19% | 31 | 16 | 53 | BCSS | Oncotype DX | 14.5 years C-index (AUC): 0.72, p = NR |
48 | – | 52 | MammaPrint | 14.5 years C-index (AUC): 0.76, p = NR | ||||||
Ou Yang 2014605 n = 1981 a-ii) n = 1526 |
a) METABRIC | ER+ 100%, HER2– NR | NR | – | – | – | BCSS | Oncotype DX | 0- to 10-year C-index (AUC): 0.657, p = NR | |
– | – | – | MammaPrint | 0- to 10-year C-index (AUC): 0.612, p = NR | ||||||
Ou Yang 2014605 b-ii) n = 134 |
b) Loi (GSE6532) | ER+ 100%, HER2– NR | NR | – | – | – | Oncotype DX | 0- to 10-year C-index (AUC): 0.640, p < 0.05 | ||
– | – | – | MammaPrint | 0- to 10-year C-index (AUC): 0.606, p < 0.05 | ||||||
Ou Yang 2014605 c-ii) n = 348 |
c) Buffa (GSE22219) | ER+ 100%, HER2– NR | NR | – | – | – | Oncotype DX | 0- to 10-year C-index (AUC): 0.727, p < 0.05 | ||
– | – | – | MammaPrint | 0- to 10-year C-index (AUC): 0.647, p < 0.05 | ||||||
Ou Yang 2014605 d-ii) n = 66 |
d) Wang (GSE19615) | ER+ 100%, HER2– NR | NR | – | – | – | Oncotype DX | 0- to 10-year C-index (AUC): 0.435, p < 0.05 | ||
– | – | – | MammaPrint | 0- to 10-year C-index (AUC): 0.372, p < 0.05 | ||||||
Ou Yang 2014605 e-ii) n = 201 |
e) Miller (GSE3494) | ER+ 100%, HER2– NR | NR | – | – | – | Oncotype DX | 0- to 10-year C-index (AUC): 0.645, p < 0.05 | ||
– | – | – | MammaPrint | 0- to 10-year C-index (AUC): 0.650, p < 0.05 | ||||||
LN0 | ||||||||||
Tobin, 2014602 a) n = 253 |
a) Uppsala cohort | HR+ NR, HER2– NR | a) LN0 63% | a) ET 58%, chemotherapy 11% | 37 | 19 | 44 | BCSS | Oncotype DX | 13-year C-index (AUC): 0.73 |
51 | – | 49 | MammaPrint | 13-year C-index (AUC): 0.84 | ||||||
Prat 2012601 a) n = 610 |
GSE17705, GSE6532, GSE12093, GSE1456, MDACC133 | 100% ER+, HER2– NR | a) LN0 100% | ET 100%, chemotherapy 0% | DRFS | Oncotype DX vs. MammaPrint | 8.5-year C-index (AUC):
|
|||
Xu 2017604 a) n = 917 |
METABRIC/bioconductor data sets: GSE11121, GSE7390, GSE3494, GSE2990, breastCancer NKI | ER+ 100%, HER2– NR | LN0 100% | NR | – | – | – | RFS | Oncotype DX | 15-year C-index (AUC): 0.68 (estimate off graph) |
– | – | – | MammaPrint | 15-year C-index (AUC): 0.71 (estimate off graph) | ||||||
– | – | – | NPI | 15-year C-index (AUC): 0.68 (estimate off graph) | ||||||
Ou Yang 2014605 n = 1981 a-i) n = 1037 |
a) METABRIC | NR | LN0 100% | NR | – | – | – | BCSS | Oncotype DX | 0- to 10-year C-index (AUC): 0.650, p = NR |
– | – | – | MammaPrint | 0- to 10-year C-index (AUC): 0.641, p = NR | ||||||
Ou Yang 2014605 b-i) n = 125 |
b) Loi (GSE6532) | NR | LN0 100% | NR | – | – | – | Oncotype DX | 0- to 10-year C-index (AUC): 0.635, p < 0.05 | |
– | – | – | MammaPrint | 0- to 10-year C-index (AUC): 0.604, p < 0.05 | ||||||
Ou Yang 2014605 c-i) n = 250 |
c) Buffa (GSE22219) | NR | LN0 100% | NR | – | – | – | Oncotype DX | 0- to 10-year C-index (AUC): 0.681, p = NS | |
– | – | – | MammaPrint | 0- to 10-year C-index (AUC): 0.628, p < 0.05 | ||||||
Ou Yang 2014605 d-i) n = 64 |
d) Wang (GSE19615) | NR | LN0 100% | NR | – | – | – | Oncotype DX | 0- to 10-year C-index (AUC): 0.665, p < 0.05 | |
– | – | – | MammaPrint | 0- to 10-year C-index (AUC): 0.674, p = NS | ||||||
Ou Yang 2014605 e-i) n = 158 |
e) Miller (GSE3494) | NR | LN0 100% | NR | – | – | – | Oncotype DX | 0- to 10-year C-index (AUC): 0.608, p = NS | |
– | – | – | MammaPrint | 0- to 10-year C-index: 0.604, p = NS | ||||||
LN+ | ||||||||||
Prat 2012601 b) n = 699 |
GSE17705, GSE6532, GSE12093, GSE1456, MDACC133 | 100% ER+, HER2– NR | b) LN+ 100% (% LN > 3 NR) | ET 100%, chemotherapy 0% | DRFS | Oncotype DX vs. MammaPrint | 0- to 10-year C-index (AUC):
|
|||
Oncotype DX vs. MammaPrint vs. EndoPredict | ||||||||||
Zhao 2014608 a) n = 912 |
a) GSE6532, GSE3494, GSE1456, GSE7390, GSE2603, E-TABM-158 | a) ER+ 76%, HER2– 85% | NR | – | – | DRFS | Oncotype DX |
Follow-up year NR for C-index analysis C-index (AUC): 0.648 (95% CI 0.63 to 0.67) PVE: 4.05 |
||
– | – | – | MammaPrint |
C-index (AUC): 0.612 (95% CI 0.60 to 0.63) PVE: 4.76 |
||||||
– | – | – | EndoPredict |
C-index (AUC): 0.648 (95% CI 0.63 to 0.67) PVE: 4.78 |
Study first author and year | Cohorts | Population | Nodal status | ET/chemotherapy | Outcome | Test or comparison | Likelihood ratio χ2 | Increase in likelihood ratio χ2 over clinicopathological factorsa | Other analyses |
---|---|---|---|---|---|---|---|---|---|
LN+/LN0 or NR | |||||||||
Ahn 2013591 b) n = 82 |
Gananam Severance Hospital |
100% ER+, 12% HER2+ b) Subset with RS 19–30 |
b) 43.9% LN+ (LN > 3 NR) | b) 94% ET, 82% chemotherapy | OS | MammaPrint vs. oncotype DX |
Oncotype DX intermediate (RS 19–30) risk group Adjusted HRa of MammaPrint: 10.19 (95% CI 1.05 to 99.01; p = 0.045) |
||
Fan 2006594 a) n = 295 |
NKI (derivation cohort for MammaPrint) | a) 77% ER+, HER2 NR |
a) LN0, 51% LN1–3, 36% LN > 3, 13% |
a) 14% ET, 37% chemotherapy | RFS | Oncotype DX |
Adjusted HRa Intermediate vs. low: 1.81 (95% CI 0.70 to 4.68, p = 0.22) High vs. low: 4.27 (95% CI 2.05 to 8.92; p = 0.001) |
||
MammaPrint | Adjusted HR:a 3.44 (95% CI 1.98 to 5.99; p < 0.001) | ||||||||
OS | Oncotype DX |
Adjusted HRa Intermediate vs. low: 1.81 (95% CI 0.39 to 8.27; p = 0.45) High vs. low: 6.14 (95% CI 1.84 to 20.4; p = 0.003) |
|||||||
MammaPrint | Adjusted HR:a 4.71 95% CI (2.02 to 11.00; p < 0.001) | ||||||||
Fan 2006594 b) Subgroup n = 225 |
b) 100% ER+ | b) NR | b) NR | RFS | Oncotype DX |
Adjusted HRa Intermediate vs. low: 0.82 (95% CI 0.27 to 2.46; p = 0.72) High vs. low: 2.59 (95% CI 1.44 to 4.65; p = 0.001) |
|||
MammaPrint | Adjusted HR:a 3.88 (95% CI 2.15 to 7.02; p < 0.001) | ||||||||
OS | Oncotype DX |
Adjusted HRa Intermediate vs. low: 1.42 (95% CI 0.27 to 7.50; p = 0.68) High vs. low: 4.95 (95% CI 1.82 to 13.4; p = 0.002) |
|||||||
MammaPrint | Adjusted HR:a 5.47 (95% CI 2.13 to 14.1, p < 0.001) | ||||||||
Zhao 2014608 a-i) n = 692 |
a) GSE6532, GSE3494, GSE1456, GSE7390, GSE2603, E-TABM-158 | a-i) ER+ 100%, HER2– NR |
a) LN0 67% (LN > 3 NR) a-i) NR |
NR | DRFS | Oncotype DX | 43.6, p < 0.0001 | 23.1, p < 0.0001a | |
MammaPrint | 36.0, p < 0.0001 | 21.5, p < 0.0001a | |||||||
EndoPredict | 53.6, p < 0.0001 | 31.4, p < 0.0001a | |||||||
LN0 | |||||||||
Yin 2014606 n = 198 |
TRANSBIG GSE7390 | ER+ NR, HER2– NR | LN0 100% | ‘Systemically untreated patients’b | DRFS | Oncotype DX | 13.734, p = 0.004 | ||
MammaPrint | 3.038, p = 0.986 | ||||||||
AOL | 3.325, p = 0.601 | ||||||||
NPI | 6.823, p = 0.131 | ||||||||
OS | Oncotype DX | 13.286, p = 0.002 | |||||||
MammaPrint | 0.221, p = 0.647 | ||||||||
AOL | 0.377, p = 0.551 | ||||||||
NPI | 3.658, p = 0.16 |
Prognostic performance in microarray studies
Categorisation
Only four studies594,597,601,602 reported the numbers of patients in each risk category, and these only included oncotype DX and MammaPrint (see Table 65). In LN+/LN0 cohorts for oncotype DX, the proportions were 24%, 31% and 37% low-risk patients, 11%, 16% and 19% intermediate-risk patients and 44%, 53% and 65% high-risk patients. In LN0 groups, the proportions were 14% and 19% low-risk patients, 19% and 45% intermediate-risk patients and 67% and 36% high-risk patients. For MammaPrint, the proportions were 39%, 48% and 51% low-risk patients, 61%, 52% and 49% high-risk patients in LN+/LN0 patients, and similar proportions in LN0 patients (40% and 48% low risk and 60% and 52% high risk).
Hazard ratios
Nine studies594–597,601–604,608 reported HR data (see Table 65). Data for oncotype DX and MammaPrint were reported in four studies594,596,602,603 with a mix of LN+/LN0 patients, LN0 only patients were reported in four studies596,597,601,604 and LN+ patients in one study. 601 Two studies595,608 reported HRs for oncotype DX, MammaPrint and EndoPredict.
Oncotype DX versus MammaPrint: LN+/LN0
Six studies594–596,602,603,608 reported data for both oncotype DX and MammaPrint in a mixed LN+/LN0 cohort (see Table 65; seven cohorts/pooled cohorts analysed, including the two that also report EndoPredict HRs). Across various outcome measures, including DRFS, RFS, OS and BCSS, all reported statistically significant HRs between test risk groups for both tests, apart from Vollan et al. ,603 in which the HR for BCSS for MammaPrint was not significant [HR 1.25 (95% CI 0.95 to 1.64; p = 0.11)], and Zhao et al. ,608 which reported HRs at 5 and 10 years, and the 10-year HRs were not statistically significant. As both Zhao et al. and Vollan et al. used the METABRIC cohort, and Vollan et al. did not report the length of follow-up, it is possible the statistically non-significant result was for 10 or more years of follow-up. Oncotype DX had higher HRs in three studies (HR 2.65 vs. 1.91, 2.57 vs. 1.96 and 2.05 vs. 1.5 for oncotype DX vs. MammaPrint, respectively)595,596,602 and MammaPrint HRs were higher in two studies (3.40 vs. 2.82 and 4.61 vs. 2.87, for MammaPrint vs. oncotype DX, respectively). 596,602 Whether the HR was higher in oncotype DX or MammaPrint did not appear to depend on whether the tests were analysed categorically or as continuous variables.
Oncotype DX versus MammaPrint: lymph node negative
Three studies597,601,604 reported data for oncotype DX and MammaPrint in LN0 patients (see Table 65). Neither test was statistically significant in Jonsdottir et al. ,597 (oncotype DX, p = 0.522; MammaPrint, p = 0.287) when DMFS was measured at 14 years. HRs were statistically significant [HR 2.7 (95% CI not reported; p < 0.001) and HR 2.5 (95% CI not reported; p < 0.001), respectively] in Xu et al. 604 when RFS was measured at 15 years and in Prat et al. 601 (HR 1.97, p < 0.0001, and 1.42, p < 0.005, respectively; 95% CIs not reported), when outcomes were censored at 8.5 years. NPI was also measured in Xu et al. ,604 with a HR a little higher than MammaPrint and a little lower than oncotype DX, at 2.6 (p < 0.001).
Oncotype DX versus MammaPrint: lymph node positive
Only one study601 reported results in a subgroup of LN+ patients (see Table 65). Both oncotype DX and MammaPrint had statistically significant HRs [4.67 (95% CI not reported; p = 0.01) and 2.12 (95% CI not reported; p = 0.03), respectively].
Oncotype DX versus MammaPrint versus EndoPredict: lymph node positive/negative
Two studies595,608 reported two pooled analyses of 33 cohorts595 and six cohorts,608 and one analysis using METABRIC data (see Table 65). 608 These cohorts are likely to contain some of the same patients. All three tests reported statistically significant HRs for DRFS at time points of < 10 years, but an analysis of 0- to 5-year, 5- to 10-year and 0- to 10-year HRs in Zhao et al. 608 only reported statistically significant HRs in the period 0–5 years, for all three tests. Oncotype DX high versus low HR was the highest in the Finetti et al. 595 analysis [HR 2.05 (95% CI 1.59 to 2.63; p < 0.001)] compared with a HR for MammaPrint of 1.5 (95% CI 1.21 to 1.85; p = 0.0002) and a HR for EndoPredict of 1.88 (95% CI 1.52 to 2.32; p < 0.001), although when all tests were analysed as continuous variables in Zhao et al. ,608 the HR was highest for EndoPredict [1.97 (95% CI 1.66 to 2.33; p < 0.0001)] compared with MammaPrint [1.70 (95% CI 1.43 to 2.03; p < 0.0001)] and oncotype DX [1.79 (95% CI 1.55 to 2.07; p < 0.0001)].
C-index (area under the curve) and other comparative data
Data relating to C-indices and other outcomes are presented in Table 66.
Oncotype DX and MammaPrint: lymph node positive/negative
Pairs of C-indices (AUC) for oncotype DX and MammaPrint were reported in three studies598,602,605 in LN+/LN0 patients (for eight cohorts). Outcomes included DRFS, DFS, OS and BCSS. The C-index ranged from 0.372605 to 0.84,591,602 indicating a wide range of fit. Notably, the worst fit was reported for an analysis in Ou Yang et al. 605 of cohort GSE19615, when oncotype DX had a C-index of 0.435 (p < 0.05) and MammaPrint had a C-index of 0.372 (p < 0.05), both indicating that the test was worse than chance alone at categorising patients into risk groups. Apart from these data, C-indices for oncotype DX ranged from 0.59598 to 0.73602 and for MammaPrint from 0.606 to 0.84. Oncotype DX had a higher C-index in four cohorts (METABRIC, GSE6532, GSE22219 and GSE19615),605 whereas MammaPrint had a higher C-index in three (Fundan University, the Uppsala cohort and the Stockholm cohort). 598,602 p-values were only reported in one study605 (four out of five cohorts) and were all statistically significant. 95% CIs were not reported in any analyses, meaning that it was not possible to determine if the C-indices were substantially different from each other.
One further study591 reported data (see Table 66) that explored the prognostic value of MammaPrint in a group of patients with intermediate risk oncotype DX. MammaPrint still had prognostic value in this group, with a statistically significant difference between risk groups (HR not reported, p = 0.013) and a C-index of 0.844, indicating that MammaPrint was able to further discriminate between patients with and without OS events.
A further study601 reported increases in likelihood ratio χ2 for oncotype DX (low-/intermediate-risk group vs. high-risk group) over MammaPrint and vice versa (see Table 66). This showed that the likelihood ratio χ2 increased by 14.4 units (p < 0.001) when oncotype was added to MammaPrint, and by 9.2 (p = 0.002) when MammaPrint was added to oncotype DX, indicating that both tests had added prognostic value over the other, but oncotype DX added a little more.
Oncotype DX and MammaPrint: lymph node negative
Pairs of C-indices (AUC) for oncotype DX and MammaPrint were reported in four studies601,602,604,605 (for eight cohorts, two of which were pooled analyses). C-indices for oncotype DX ranged from 0.608 to 0.71 and for MammaPrint from 0.604 to 0.81. p-values were only reported in one study605 (five cohorts) and were not always statistically significant, possibly due to smaller sample sizes in these subgroup analyses than in the full LN+/LN0 cohorts. Oncotype DX had a higher C-index in five cohorts (Prat et al. 601 and four of the cohorts reported in Ou Yang et al. 605), and MammaPrint had a higher C-index in three (Tobin et al. , Xu and GSE19615 from Ou Yang et al. ). 602,604,605
Oncotype DX and MammaPrint: lymph node positive
One study601 reported the C-index for LN+ patients. This was 0.64 for oncotype DX and 0.61 for MammaPrint.
Additional prognostic value in microarray studies
Oncotype DX, MammaPrint and EndoPredict in lymph node-positive/-negative patients
One study594 reported a multivariable analysis including oncotype DX and MammaPrint separately alongside ER status, tumour grade, nodal status, age, tumour size and treatment (endocrine therapy, chemotherapy or both) in patients with mixed nodal status (see Table 67). The cohort used was the derivation cohort for MammaPrint (and there may therefore be some overfitting of the model, resulting in overestimation of the prognostic performance for MammaPrint) and a subgroup of ER+-only patients. Tests were analysed as categorical rather than as continuous variables. All high versus low HRs were statistically significant, although the intermediate versus low analyses (oncotype DX only) were not. High versus low HRs were higher for oncotype DX than for MammaPrint, although this is perhaps to be expected as oncotype DX high versus low comparisons do not account for the intermediate patients, and MammaPrint has only two categories; the analyses are therefore not comparable.
One study reported a multivariable analysis in oncotype DX intermediate patients (see Table 67), and MammaPrint was shown to have additional prognostic value in this subgroup of patients (adjusted for tumour size, nodal status, PR and chemotherapy treatment) with a HR of 10.19 (95% CI 1.05 to 99.01; p = 0.045). 591
One study608 reported likelihood ratio χ2 and differences in likelihood ratio χ2 for oncotype DX, MammaPrint and EndoPredict (see Table 67). EndoPredict had the highest likelihood ratio χ2, at 53.6 (p < 0.0001), compared with 43.6 (oncotype DX) and 36.0 (MammaPrint) (both p < 0.0001). In an analysis that adjusted for nodal status, grade and tumour size, the difference in likelihood ratio χ2 over these clinicopathological variables was also highest for EndoPredict (31.4 vs. 23.1 and 21.5, respectively, all p < 0.0001), indicating that all these tests have prognostic value over these clinical factors, and EndoPredict appears to perform best.
Oncotype DX and MammaPrint versus Nottingham Prognostic Index and Adjuvant! Online in lymph node negative patients
One study reported data for LN0 patients (see Table 67). The increase in likelihood ratio χ2 over clinicopathological variables was reported for oncotype DX, MammaPrint, NPI and AOL. For DMFS, oncotype DX had the highest increase, at 13.734 (p = 0.004), compared with MammaPrint (3.038, p = 0.986), AOL (3.325, p = 0.601) and NPI (6.823, p = 0.131), and was the only test to report a statistically significant change. Results were similar for OS.
Discussion: microarray studies
Data from microarray studies have been included in this report to provide additional information relating to the comparative prognostic value of the tests, as comparative data from studies using the commercial versions of the tests are limited in number (see Appendix 5, Studies reporting more than one test). In particular, comparisons between MammaPrint and other tests (specifically oncotype DX and EndoPredict) were made in microarray studies but rarely in the studies using the commercial tests. However, these data should be interpreted with caution because of the unknown comparability of microarray studies and the commercial versions.
Data relating to HRs for outcomes between test risk groups support the data from studies using the commercial assays that show a statistically significant difference between test risk categories for outcomes such as DRFS, DFS, OS and BCSS for oncotype DX, MammaPrint and EndoPredict (no microarray studies were identified assessing Prosigna or IHC4). One study did not report statistically significant HRs at ≥ 10 years. However, conversely, three studies reported statistically significant HRs at ≥ 10 years,594,602,604 suggesting that the assumption of proportional hazards may not hold in all cohorts, and the tests are likely to be more often accurate at 0–5 years than at time points beyond that. HRs were generally statistically significant in LN+/LN0 cohorts, LN0 cohorts and LN+ cohorts, although the evidence base for the LN0 cohorts and LN+ cohorts was limited and one study did not report a statistically significant HR in a LN0 cohort, which may have been due to a small sample size (n = 94) or follow-up duration (14 years). 597 No study reported HRs in LN+/LN0, LN0 and LN+ patients separately, so it is difficult to draw any conclusions about whether HRs differ in accordance with lymph node status.
C-indices (AUC) were generally good for all tests, and did not appear to differ in accordance with lymph node status. Conclusions that can be drawn from the data reporting C-indices were limited by the non-reporting of 95% CIs, meaning that it was not possible to tell whether the tests were substantially better or worse than each other. One further problem with determining the superiority of tests was that oncotype DX has three risk categories (high, intermediate and low) whereas MammaPrint and EndoPredict have only two (high and low); C-index analyses represent the prognostic potential of the test, but do not indicate which cut-off points should be used, what clinical decisions should be made for intermediate-risk patients or what the long-term clinical outcomes would be for patients treated in accordance with the test as commercially marketed. One study showed that MammaPrint could further categorise oncotype DX intermediate-risk patients into high- and low-risk patients, with an excellent C-index of 0.844. However, without seeing the overall performance of MammaPrint in this cohort, it is not possible to conclude that MammaPrint outperforms oncotype DX. For this reason, it is difficult to draw any conclusions about superiority given these differences in categories and the clinical significance in terms of treatment options.
As in previous sections of this report, it can be argued that the true value of the test lies in how much additional prognostic information is provided over and above clincial factors. The one study608 to report such data across three tests (oncotype DX, MammaPrint, EndoPredict) reported likelihood ratio χ2 and change in likelihood ratio χ2 in analyses adjusted for clinicopathological variables suggesting that EndoPredict had the greatest additional value, followed by oncotype DX, then MammaPrint, which agrees with the analyses reported in TransATAC. One study606 (not adjusted for clinicopathologial variables) in LN0 patients reported that only oncotype DX had a statistically significant change in likelihood ratio χ2 whereas AOL, NPI and MammaPrint did not, which supports the order of prognostic performance reported in TransATAC.
Conclusions: microarray studies
Microarray studies support conclusions from studies using the commercial versions of the assays in suggesting that oncotype DX, MammaPrint and EndoPredict can discriminate between high- and low-risk patients regardless of LN status (data limited to mixed LN+/LN0 patients for EndoPredict); the utility of the intermediate-risk group in oncotype DX is uncertain; the additional prognostic performance of the tests over clinicopathological variable is less certain for MammaPrint, although the order of superiority appears similar to that reported by TransATAC, namely EndoPredict, then oncotype DX, then MammaPrint, although the evidence base is limited.
OPTIMA Prelim: a study of concordance between tests
Concordance between tests
Concordance is defined in this review as the degree to which tests assign the same patients to the same risk groups. They do not report long-term outcomes. They are distinct from decision impact studies, in which patients are actually assigned to treatment or not based on the test result and clinician and patient preference.
In accordance with the scope21 and the protocol,610 we did not conduct a systematic review of concordance. Instead, we present a summary of one high-quality, highly relevant study [the Optimal Personalised Treatment of early breast cancer usIng Multi-parameter Analysis preliminary (OPTIMA Prelim) study]588 conducted in the UK.
OPTIMA Prelim: methods
The OPTIMA Prelim study (ISRCTN42400492) was a feasibility phase of Optimal Personalised Treatment of early breast cancer usIng Multi-parameter Analysis (OPTIMA). 611 OPTIMA is an ongoing trial that aims to test the effectiveness of multiparameter testing in identifying a subgroup of patients (among those who would ordinarily be offered chemotherapy) who will not respond to chemotherapy and can therefore avoid it and move more quickly to more appropriate treatments (i.e. endocrine therapy and radiotherapy). OPTIMA Prelim was designed to help select which of six available tests [oncotype DX, MammaPrint, Prosigna, IHC4, MammaTyper (BioNTech Diagnostics GmbH, Mainz, Germany), and NexCourse Breast by Aqua (IHC4 automated quantitative immunofluorescence-AQUA)(NexCourse BreastTM, Genoptix Inc. Carlsbad, CA, USA)] to use in the trial. Here, we only report data for the four in-scope tests (oncotype DX, MammaPrint, Prosigna and IHC4). Three clinical prognostic scores were also used, namely AOL, NPI and Predict, but these were only compared with each other.
The OPTIMA Prelim study selected women who would routinely be offered chemotherapy, specifically women aged ≥ 40 years with ER+, HER2– early-stage breast cancer with either 1–9 positive lymph nodes or a tumour of ≥ 30 mm if node negative. Women were randomised to test-directed therapy or standard treatment (chemotherapy followed by endocrine therapy). Patients in the test-directed arm received oncotype DX testing and those with a recurrence score of ≤ 25 received endocrine monotherapy.
OPTIMA Prelim: results
Results are presented in Table 68. A total of 313 patients from 35 UK hospitals were recruited and randomised; 302 patients received multiple tests. Eleven patients were excluded: four withdrew consent, one was ineligible and six had insufficient tissue for all tests to be conducted.
Test | Percentage tested | Percentage in risk category | Kappa statistic (95% CI) | ||||
---|---|---|---|---|---|---|---|
Low | Intermediate | High | MammaPrint | Prosigna (L/I) | IHC4 (L/I) | ||
Oncotype DX | 99.7 | 54 | 28 | 18 | 0.40 (0.30 to 0.5) | 0.44 (0.3 to 0.5) | 0.53 (0.4 to 0.7) |
MammaPrint | 98.9 | 61 | 39 | – | 0.53 (0.4 to 0.6) | 0.33 (0.2 to 0.4) | |
Prosigna (L/I) | 99.0 | 36 | 29 | 35 | – | – | 0.39 (0.3 to 0.5) |
IHC4 (L/I) | 85.1 | 24 | 48 | 28 | – | – | – |
Nottingham Prognostic Index, Predict and Adjuvant! Online
With NPI, patients were at high (21%), intermediate (75%) and low (4%) risk. All patients with an NPI of ≤ 3.4 had tumours of ≥ 30 mm. Predict and AOL predict a risk for patients depending on whether they take only endocrine monotherapy or take chemotherapy and endocrine therapy; the difference between the Predict and AOL median predicted 10-year OS within each treatment type ranged from 6.2% to 8.4%.
Oncotype DX, MammaPrint, Prosigna and IHC4, MammaTyper, NexCourse Breast by Aqua
Results for all tests were available from 236 patients (78%). IHC4 could not be determined for 45 patients (15%); one patient did not have enough tissue for oncotype DX testing, and three Prosigna and seven MammaPrint tests were unobtainable.
Out of the four in-scope tests, MammaPrint assigned the most patients to the low-risk category (61%), although when low and intermediate categories were treated as one category for the three tests that have three risk groups (oncotype DX, Prosigna and IHC4), oncotype DX assigned the most to the low/intermediate category (82%) and MammaPrint assigned the least (61%) (see Table 68).
Kappa statistics indicated modest agreement between tests, ranging from 0.33 (95% CI 0.3 to 0.5) between MammaPrint and IHC4 and 0.53 (95% CI 0.4 to 0.7) between MammaPrint and Prosigna, and 0.53 (95% CI 0.4 to 0.7) between oncotype DX and IHC4 (see Table 68). Data are not reported for the four in-scope tests alone, but across all five tests (that have risk groups rather than intrinsic subtypes, i.e. oncotype DX, MammaPrint, Prosigna, IHC4 and IHC4-AQUA), 61% of tumours gave no consensus, and only 119 tumours (39%) were uniformly classified as either low/intermediate or high by all five tests. Of these, 93 (31%) were low/intermediate risk by all tests and 26 (8%) were high risk by all tests. An exploratory analysis using high/intermediate- versus low-risk patients also showed only moderate agreement.
The authors report a number of further analyses that demonstrate that no tests appeared to be more in agreement than others, and that there were no statistically significant differences in clinicopathological variables between concordant and discordant patients. Disagreement spanning two categories (i.e. between low and high risk) was not infrequent. Agreement was not better at the extremes of the ranges of the tests (i.e. the very low- and very high-risk tumours).
Conclusions
The authors concluded that, although tests assigned similar proportions of patients to low/intermediate- and high-risk categories, test results for an individual patient could differ markedly depending on which test was used.
Appendix 6 Review of existing economic analyses published since National Institute for Health and Care Excellence Diagnostics Guidance 10
Cost-effectiveness review: methods
Systematic searches were undertaken to identify existing economic evaluations of tumour profiling tests to guide treatment decisions in people with early-stage breast cancer. Only those studies that were published since the previous appraisal of tumour profiling tests (NICE DG1020) were considered to be potentially relevant for inclusion in the review; a review and a critical appraisal of economic analyses published prior to this date is available in Ward et al. 1 The review was undertaken solely with the purpose of exploring methodological choices and their potential relevance to the current decision problem, rather than to assess the results of published economic evaluations or the potential sources of bias that might affect these.
A comprehensive search was undertaken to systematically identify economic evaluations of the five tumour profiling tests (EndoPredict, oncotype DX, MammaPrint, IHC4 and Prosigna) and reviews of economic evaluations of tumour profiling tests for breast cancer.
Literature searching for economic evaluation studies was undertaken in March 2017 in the following electronic databases:
-
MEDLINE Epub Ahead of Print, In-Process & Other Non-Indexed Citations: via Ovid, 1946 to present
-
EMBASE: via Ovid, 1974 to present
-
Health Technology Assessment Database: CRD, 1995 to 2016
-
NHS Economic Evaluation Database: CRD, 1995 to March 2015
-
Science Citation Index Expanded: Web of Science, 1900 to present
-
Conference Proceedings Citation Index – Science: Web of Science, 1990 to present.
The search strategies comprised MeSH or Emtree Thesauri terms and free-text synonyms for (1) ‘tumour profiling tests’ and ‘breast cancer’ and (2) ‘breast cancer’ only. Searches for oncotype DX, MammaPrint, IHC4 and Prosigna were limited by publication date from 2011 (the cut-off date for the previous appraisal), whereas no date limits were applied to EndoPredict. Searches were translated across databases and were not limited by language. The search strategies are presented in Appendix 2. Search filters designed to identify economic evaluations and reviews were used in MEDLINE and other databases, where appropriate. Reference and citation searching of included papers was undertaken.
In order to be considered potentially relevant for inclusion in the review, studies were required to meet all of the following criteria:
-
full economic evaluations comparing tumour profiling for breast cancer tests against other tests and/or current practice
-
published in English
-
available in full-text format (studies that were available in abstract form only were excluded from the review)
-
relevant to the populations included within the final NICE scope. 21
Cost-effectiveness review results: summary of studies identified
A total of 294 potentially includable studies (including potential duplicates) were identified by the searches. Of these, 59 studies were deemed to be potentially eligible for inclusion in the review and full texts were obtained, when available. A total of 26 unique studies met the inclusion criteria and were included in the review. The scope and methodological approaches adopted within the included studies are summarised in Tables 69 and 70, respectively.
First author and year | Population | Age | Intervention | Comparator | Country | Perspective | Time horizon | Discount rate |
---|---|---|---|---|---|---|---|---|
Bargalló-Rochaa (2015)430 | HR+, LN0 or LN1–3 early-stage breast cancer | Baseline age 55.5 years | Oncotype DX | Current standard of care | Mexico | Instituto Mexicano del Seguro Social perspective | 40 years | 5% |
Holta (2013)141 | LN0 or pNImi, ER+ breast cancer in the UK | Mean age 60.55 years | Oncotype DX | Conventional diagnostic procedures (including AOL and NPI) | UK | NHS | 30 years | 3.5% |
Davidsona (2013)478 | ER+ LN0 breast cancer | Mean age 53 years | Oncotype DX | Conventional diagnostic procedures | Canada | Canadian health-care system | Lifetime (up to maximum age of 100 years) | 5% |
Jahn (2015)618 | ER+ and/or PR+, HER2/neu negative, and LN0 breast cancer | Baseline age 50 years | Oncotype DX | AOL score | Austria | Societal perspective in line with the Austrian health-care system | Lifetime | 5% |
Kondo (2011)440 | ER+ early-stage breast cancer | Baseline age 45 years | Oncotype DX | St Gallen criteria | Japan | Societal | Lifetime (with assumptions about maximum survival after 10 1-year cycles) | 3% |
Lamond (2012)362 | Early-stage, endocrine-sensitive breast cancer undergoing adjuvant chemotherapy or no chemotherapy | Median age 50 years | Oncotype DX | Current practice (population-based study) | Canada | Canadian health-care system perspective | 25 years | 3% |
Paulden (2013)619 | LN0, ER+ and/or PR+ (HER2–/neu) early-stage breast cancer patients who are candidates for adjuvant chemotherapy | Baseline age 50 years | Oncotype DX | AOL | Canada | Ontario Ministry of Health and Long-Term Care | Lifetime | 5% |
Reeda (2013)620 | LN0, ER+ breast cancer | Baseline age 55 years | Oncotype DX | No RS-guided strategy | USA | US health-system perspective and societal perspective | Lifetime | 3% |
Blanka (2015)433 | ER+, HER2–breast cancer | Median age appears to be 64 years | EndoPredict (EPClin) ± three guidelines | Three guidelines (German S3, St Gallen, NCCN) | Germany | German health-care system | Lifetime (50 years) | 3% |
Bonastre (2014)434 | LN0 early-stage breast cancer. Subgroup analysis of ER+ patients | Patients aged < 61 years | MammaPrint | AOL, chemotherapy for all | France | French National Insurance Scheme | 10 years | 4% |
Retèla (2012)621 | Early, operable, LN0, ER+ breast cancer | Baseline age 50 years | MammaPrint | Clinical-pathological guidelines (such as AOL) | The Netherlands | Dutch health-care perspective | 20 years | 4% costs, 1.5% health outcomes |
Retèla (2012)622 | Early, LN0 breast cancer | Not reported | MammaPrint; oncotype DX | AOL | The Netherlands | Dutch health-care perspective | 20 years | 4% costs, 1.5% health outcomes |
Retèla (2013)623 | Early LN0 ER+ breast cancer after local therapy | Baseline age 50 years | MammaPrint 70G-FFT; MammaPrint 70G-PAR | AOL | The Netherlands | Societal perspective | 20 years | 4% costs, 1.5% health outcomes |
Retèla (2013)624 | Reflective of RASTER population | Mean age 48 years | MammaPrint | AOL | The Netherlands | Dutch health-care perspective | 20 years | 4% costs, 1.5% health outcomes |
Hall (2012)438 | LN+, ER+ early-stage breast cancer | Baseline age 60 years | Oncotype DX | Standard care (chemotherapy for all) | UK | NHS | Lifetime (up to maximum age of 100 years) | 3.5% |
Hannouf (2012)499 | Early-stage ER+/PR+ axilliary LN0 breast cancer | Starting age unclear | Oncotype DX | Current practice (population-based study) | Canada | Canadian public health-care system | Lifetime | 5% |
Hannouf (2014)500 | Postmenopausal women with early-stage ER+/PR+ axillary LN+ breast cancer | Mean age 61 years | Oncotype DX | Current practice | Canada | Canadian public health-care system | Lifetime | 5% |
Kondo (2012)625 | HR+, LN0, HER2– early-stage breast cancer | Baseline age 55 years | MammaPrint | St Gallen criteria | Japan | Societal | 10 years | 3% |
Mislicka (2014)626 | Early-stage, LN0, ER+ breast cancer | Not reported | Mammostrat | Oncotype DX | USA | Third-party payer perspective | 10 years | 3% |
Stein (2016)207 | ER+, HER2– early-stage breast cancer patients | Median age 58 years | Oncotype DX; MammaPrint/Bluetest; Prosigna | Chemotherapy for all | UK | NHS | Lifetime (up to maximum age of 100 years) | 3.5% |
Tiwana (2013)627 | Women who are LN0, ER+ and/or PR+, HER2/neu-negative early-stage breast cancer, who are candidates for adjuvant chemotherapy | 50 years | Oncotype DX | AOL | Canada | Not reported – appears to be payer perspective | Lifetime | 5% |
Vanderlaana (2011)628 | Minimally LN+, early-stage breast cancer | Mean age 62 years | Oncotype DX | Current care (US NCCN guidelines) | USA | US payer (managed care) perspective | 30 years | 3% |
Wong (2012)629 | Women with LN+ HR+ breast cancer (one to three nodes) | Reflective of RxPONDER630 | Oncotype DX | Current care (US NCCN guidelines) | USA | Payer | Lifetime (40 years) | 3% |
Ward (2013)1 | Women with ER+ LN0, and HER2– early-stage breast cancer | Mean age 58.3 years | Oncotype DX, IHC4, MammaPrint and Mammostrat (Mammostrat, Clarient Diagnostic Services, Inc, Aliso Viejo, CA, USA) | Current clinical practice | UK | NHS and PSS | Lifetime (up to age of 100 years) | 3.5% |
Yanga (2012)631 | LN0, ER+ breast cancer | Not reported | Oncotype DX | MammaPrint | USA | Third party payer | 10 years | 3% |
Yamauchia (2014)247 | Women with ER+, LN0 (including micrometastases) ESBC who were eligible for treatment with adjuvant chemotherapy after having undergone surgery for primary tumour removal and lymph node dissection | Mean age 49.8 years | Oncotype DX | No RS guided strategy | Japan | Societal | Lifetime | 3% |
First author and year | Model approach | Cycle length | Model type | Does model claim predictive benefit for test? | Assumptions on chemotherapy use | Long-term health states |
---|---|---|---|---|---|---|
Bargalló-Rocha (2015)430 | Markov | 1 year | Classification to LR, IR, HR | Yes – RRR only in the high-risk group, based on Paik50 | Proportion of all groups receive chemotherapy | 3 states:
|
Holt (2013)141 | Markov | 1 year | Classification to LR, IR, HR | Yes – RRRs in intermediate and high risk based on Paik50 | Change in chemotherapy use informed by decision conflict analysis (changes applied to all three risk groups) | 3 states:
|
Davidson (2013)218 | Markov | 1 year | Classification to LR, IR, HR | Yes – different RRRs between risk groups | Proportion of all groups receive chemotherapy | 5 states:
|
Jahn (2015)618 | DES | N/A | Sequential use of AOL and oncotype DX – eight test strategies considered | Yes | Chemotherapy provided to proportion of patients in all groups except AOL low risk and oncotype DX low risk | DES includes:
|
Kondo (2011)440 | Markov | Unclear – appears to be 1 year | Reclassification based on use of assay | Yes | Half of cases with no definitive indication undergo adjuvant chemotherapy and only cases with high RS undergo chemotherapy after the use of the assay based on the results of Japanese validation study | 5 states:
|
Lamond (2012)362 | Markov | 1 month | Classification to LR, IR, HR | Yes – only in low risk and high risk | For no test, based on Canadian population-based study; for test, based on RS. Usage in intermediate group assumed to be the same in both groups | 10 states:
|
Paulden (2013)619 | Markov | Appears to be monthly | Reclassification based on use of assay | Yes | Different regimens assumed for different risk groups. Different proportions of patients assumed to receive chemotherapy in accordance with risk group (estimated by linear regression) | 5 states:
|
Reed (2013)620 | Markov | 6 months | Classification based on RS | Yes – different RRR assumed in each risk group | No low risk get chemotherapy, all intermediate risk and high risk get chemotherapy | 3 states:
|
Blank (2015)433 | Markov | 1 year | Based on sensitivity and specificity of test/guideline | No – same treatment effect applied to all groups irrespective of risk | No chemotherapy for low-risk patients | 3 states:
|
Bonastre (2014)434 | EEACT with partitioned survival | Unclear | Unclear | No – authors state that there is no evidence to support predictive benefit for MammaPrint | For MammaPrint and AOL, only high-risk patients were assumed to receive chemotherapy. For the all chemotherapy comparator, all patients receive chemotherapy irrespective of risk | 4 states:
|
Retèl (2012)621 | Markov | 1 year | Based on sensitivity and specificity of test/guideline | Unclear | Chemotherapy used only in high-risk patients in accordance with treatment guidelines | 4 health states:
|
Retèl (2012)622 | Markov | 1 year | Based on sensitivity and specificity of test/guideline | Unclear | High and intermediate groups combined – both assumed to receive ET plus chemotherapy | 4 health states:
|
Retèl (2013)623 | Markov | 1 year | Based on sensitivity and specificity of test/guideline | Unclear | Chemotherapy used only in high-risk patients in accordance with treatment guidelines | Not reported but based on previous 4-state model reported by Retèl et al.621 (see above) |
Retèl (2013)624 | Markov | Not reported, but likely to be 1 year | Based on sensitivity and specificity of test/guideline | Unclear | Not reported but likely to be same as other Retèl studies | 4 health states:
|
Hall (2012)438 | Decision tree and modified Markov model | Not reported | Classification to LR or HR | Unclear – data contained within the appendices appear to suggest that predictive benefit is modelled | All high-risk patients receive chemotherapy | 6 health states:
|
Hannouf (2012)499 | Markov | 1 month | Classification to LR, IR, HR with separate Markov nodes for chemotherapy plus ET vs. ET only (accounting for chemotherapy-related AEs) | Unclear – appears to assume predictive benefit | Model assumes that 50% of intermediate-risk patients receive chemotherapy | ET-only model – 4 states:
|
Hannouf (2014)500 | Markov | 1 month | Classification to LR, IR, HR with separate Markov nodes for chemotherapy plus ET vs. ET alone (accounting for chemotherapy-related AEs) | Unclear – appears to assume predictive benefit | Model assumes that 50% of intermediate-risk patients receive chemotherapy | ET-only model – 5 states:
|
Kondo (2012)625 | Markov | 1 year | Classification to LR, HR | No | Chemotherapy applied to HR, ET only for low-risk patients | 5 states:
|
Mislick (2014)626 | Markov | 1 year | Classification to LR, IR, HR | Yes – for both Mammostrat and oncotype DX | 80% HR assumed to receive chemotherapy; 10% low risk assumed to receive chemotherapy; 50% intermediate risk assumed to receive chemotherapy | 3 states:
|
Stein (2016)207 | Decision tree and modified Markov model | 1 year | Classification to LR or HR | Separate analyses undertaken including predictive benefit and assuming constant benefit across risk groups | All high-risk patients receive chemotherapy | 7 health states:
|
Tiwana (2013)627 | Not reported – appears to be Markov | Not reported | Classification to low–low risk, low–intermediate risk, low–high risk, low–none risk, intermediate–low risk, intermediate–intermediate risk intermediate–high risk, intermediate–none risk, high–low risk, high–intermediate risk, high–high risk or high–none risk. Based on a model constructed for the Ontario Heath Technology Assessment Committee | Yes – different recurrence rates modelled between groups and tests | Based on usage reported in Asad et al.632 |
Not reported – appears to include 3 states: relapse-free distant metastases dead |
Vanderlaan (2011)628 | Appears to be Markov | Not reported | Classification to LR or HR. Original source model provided by Cedar Associates based in California, USA | No – same recurrence rates for all HR patients | 71% of women in usual care assumed to receive chemotherapy treatment | 3 states:
|
Wong (2012)629 | Decision tree with partitioned survival approach to determine sojourn time | Not reported | For patients whose treatment decision was based on US NCCN criteria classification to LR or HR. For patients whose treatment was based on the oncotype DX test results classification to LR, IR or HR | Yes – different treatment effects applied for each risk category | ≈55% of women assumed to receive chemotherapy | Not clearly reported – appears to be 3 states:
|
Ward (2013)1 | Markov | 6 months | Classification to risk/prognosis group | No | Baseline chemotherapy use (without test) based on English cancer registry data. Use of chemotherapy conditional on test based on unpublished data | 4 states:
|
Yang (2012)631 | Markov | 1 year | Classification to LR or HR using AOL and reclassification probabilities from the literature | Yes – different risk reductions applied between HR and LR | 90% of patients who were high risk in accordance with both AOL and oncotype DX/MammaPrint received chemotherapy, 90% of patients who were at low risk in accordance with both AOL and oncotype DX/MammaPrint did not receive chemotherapy. For patients who experienced a conflicting result between AOL and oncotype DX/MammaPrint, 50% of the subpopulation received chemotherapy | 3 states:
|
Yamauchi (2014)247 | Markov | Unclear – appears to be 1 year | Classification to LR, IR, HR | Yes – different risk reductions applied between risk groups | Based on empirical study (Yamauchi et al.247) | 3 states:
|
The models reported within the included studies were developed to assess the cost-effectiveness of tumour profiling tests across a variety of different countries including the UK, the USA, Canada, Mexico, Japan, Austria, Germany, France and the Netherlands. Most studies compared oncotype DX (18 studies) or MammaPrint (eight studies) against comparators such as AOL, the St Gallen guidelines, standard practice or other conventional diagnostic tools. One included study (Blank et al. 433) compared EndoPredict against a comparator that comprised a combination of three different guidelines. There was variation between the analyses with respect to the patient populations evaluated, their disease type and other patient characteristics. The models included populations with initial ages (when reported) ranging from 45 years to 64 years.
Across the breadth of included studies, there was a high level of consistency in terms of the general modelling approach and structure, and several studies were based on a previously published model. The majority of the included models adopted a Markov or hybrid decision tree–Markov approach, with discrete nodes applied to estimate long-term costs and outcomes for patients assigned to different test risk classification categories. Two studies adopted a partitioned survival approach. One further study used a discrete event simulation approach. The structure of the model used in one study was not reported. The time horizons used in the economic models ranged from 10 years to the patient’s remaining lifetime, with cycle lengths (when reported) ranging from 1 month to 1 year. Most of the models that evaluated oncotype DX against current practice assumed that the test was associated with a predictive benefit of chemotherapy.
Most of the included studies that adopted a Markov structure included a common set of three health states: (1) alive and recurrence-free, (2) alive with distant recurrence and (3) dead. However, several models also included other health states, such as local recurrence, disease-free after local recurrence, distant recurrence with response to treatment, distant recurrence with no response to treatment, progression of disease after distant recurrence, CHF, chronic myeloid leukaemia, AML/MDS, and febrile neutropenia and chemotherapy-induced nausea and vomiting. One model, which was reported across two studies,499,500 used different health states for patients receiving endocrine therapy only (remission, local recurrence, distant recurrence and dead) and for patients receiving chemotherapy plus endocrine therapy (remission with chemotherapy, remission without chemotherapy, local recurrence, distant recurrence and dead).
Although many of the models identified by the review adopted a similar modelling approach, none included all of the relevant tests listed in the final NICE scope. 21 For this reason, none of the existing models included in the review was considered to be suitable for the current appraisal.
Appendix 7 Review and critical appraisal of economic analyses provided by test manufacturers
Economic analyses were provided by the manufacturers of oncotype DX (Genomic Health) and MammaPrint (Agendia) and the chief investigator of the EndoPredict (Myriad Genetics) decision impact study. 62,94,159,182 The fully executable health economic models developed for the analyses of oncotype DX and MammaPrint were made available to the EAG; the model referred to in the draft EndoPredict cost-effectiveness paper was not provided to the EAG. These three analyses are detailed and critically appraised in the following sections.
Agendia cost-effectiveness report: MammaPrint versus current practice
Agendia submitted a model, which was critiqued by the EAG as part of the assessment process, but it cannot be reported here as Agendia withdrew permission to reproduce the model.
Genomic Health dossier: oncotype DX versus current practice
The Genomic Health dossier made available to NICE and the EAG includes a cost-effectiveness report detailing the methods and results of a de novo health economic evaluation of oncotype DX versus current practice for early-stage breast cancer in the UK. 62 The fully executable economic model was also made available to the EAG for scrutiny.
Genomic Health model scope
According to the Genomic Health dossier,62 the model was based on the previous analysis reported by Ward et al. 1 The company’s base-case model evaluates the cost-effectiveness of oncotype DX versus current practice in ER+ LN0 patients. The model also allows for the evaluation of oncotype DX versus MammaPrint, EndoPredict and Prosigna as secondary analyses. The base case includes ER+, LN0 early-stage breast cancer patients, with the option to evaluate LN+ patients as a secondary analysis. Cost-effectiveness results are expressed in terms of the incremental cost per QALY gained. Health outcomes and costs are discounted at a rate of 3.5% per annum. Costs are valued at 2016 prices and reflect a NHS and PSS perspective.
Genomic Health model structure
The company’s model is referred to as a Markov model in the Genomic Health dossier, but is more accurately described as a hybrid decision tree–Markov model. The decision tree portion of the model incorporates the decision to give adjuvant chemotherapy or not. Within the oncotype DX group, this probability is driven by the oncotype DX Breast Recurrence Score, and in the comparator group, this probability is driven by current clinical practice as recorded in the pre-oncotype DX chemotherapy decision in the NHS England oncotype DX Access Scheme Data set. 183
The Markov component of the model includes three health states: (1) recurrence free, (2) distant recurrence and (3) dead. The model adopts a 30-year time horizon and a 6-month cycle length. The age of patients on entry into the model is 58.9 years, based on the mean age of patients in the NHS England Access Scheme Database. 183 Patients can die from breast cancer or from other causes. The model assumes that oncotype DX is predictive of chemotherapy benefit, hence different treatment effects are applied in accordance with the low, intermediate and high recurrence score groups (applied to the oncotype DX group only). Health utilities are assigned to the recurrence-free and distant recurrence states. A chemotherapy-related disutility is applied during each cycle for those who receive chemotherapy in either the test or no-test group. A further QALY loss is applied for women who experience local recurrence. Separate health utility values are applied for patients who develop AML and for those in the final 3 months of life prior to death due to breast cancer. As the model does not contain separate health states for these two states, the health utility values for patients in the recurrence-free and distant recurrence health states are adjusted to account for the lower health utility values for patients with AML and for those dying from breast cancer.
The costs used in the Genomic Health model were based on Ward et al. ;1 these were uplifted to current values using the HCHS pay and prices inflation index. 195 According to the Genomic Health dossier, all patients are assumed to receive endocrine therapy, based on the following assumptions:
-
tamoxifen for 5 years (40% of patients)
-
anastrozole for 5 years (20% of patients)
-
letrozole for 5 years (20% of patients)
-
tamoxifen for 2 years plus exemestane for 3 years (20% of patients)
-
tamoxifen for 5 years followed by letrozole for a further 3 years (half of patients completing tamoxifen for 5 years received an additional 3 years of letrozole) (10% of patients).
The model assumes that adjuvant chemotherapy consists of six cycles of FEC75 (5 fluorouracil, epirubicin and cyclophosphamide). The cost of chemotherapy is included as a once-only cost and includes the costs of drug acquisition, administration, monitoring and an echocardiogram for 25% of patients undergoing chemotherapy (total chemotherapy cost = £4678). The model includes costs associated with the following short-term AEs: anaemia (1.4%), thrombocytopenia (0.3%), neutropenic infection (1.6%), nausea/vomiting (24.2%) and stomatitis (4%). This cost is applied as a once-only cost of £315 for women receiving adjuvant chemotherapy. A proportion of women receiving chemotherapy (0.46%) are assumed to subsequently develop AML; this is included as a once-only cost of £13,123. Half of the annual cost of distant recurrence (half of £9316) is applied to patients in the distant recurrence health state during each cycle. A once-only cost (£16,127) of treating local recurrence is applied to 10.5% of patients entering the distant recurrence state. The model also includes a cost of £4608 to reflect end-of-life costs for women who die as a consequence of their breast cancer.
The Genomic Health model makes the following structural assumptions:
-
The results of the oncotype DX test are assumed to be predictive of the benefit deriving from subsequent chemotherapy use. Conversely, a common relative ROR is applied to all patients in the current practice group.
-
Survival following distant recurrence is assumed to be 3.3 years (based on Thomas et al. 186).
-
A HRQoL decrement associated with AEs is applied during every model cycle for the remaining lifetime of patients who receive adjuvant chemotherapy.
-
The costs of short-term AEs are included only in the first model cycle.
-
AML is included as a long-term complication of chemotherapy.
-
All patients are assumed to receive endocrine therapy.
Evidence sources used to inform the Genomic Health model
Table 71 summarises the evidence sources used to inform the Genomic Health model.
Parameter | Source | EAG comments |
---|---|---|
10-year risk of distant recurrence on endocrine therapy | Dowsett et al.37 | Estimates presented in the Genomic Health dossier and model do not match the estimates in the Dowsett et al.37 paper |
Oncotype DX recurrence score classification | NHS England Access Scheme Database183 | Risk classification probabilities and risk of distant recurrence are not derived from the same source. This may produce a bias due to differences in the distribution of prognostic variables for patients within each recurrence score category between the two sources. Risk reclassification is applied incorrectly in the model |
Relative risk reduction associated with chemotherapy | Paik et al.50 | This is applied incorrectly within the standard care group in a way that suggests that the same patient receiving the same treatment accrues a different level of benefit if they are tested with oncotype DX |
Probability patient receives chemotherapy | NHS England Access Scheme Database183 | This source reflects the LN0 ‘intermediate-risk’ group only |
Health utilities | Ward et al.1 | Health losses due to chemotherapy-related AEs are applied incorrectly |
Probability of short-term AEs during the first 6 months | Ward et al.1 | – |
Probability of local recurrence | Ward et al.1 | – |
Probability of AML | Ward et al.1 | – |
Other-cause mortality rates | ONS190 | – |
Oncotype DX cost | Genomic Health62 | – |
All other costs | Ward et al.1 | – |
Probability of receiving chemotherapy under current practice and with oncotype DX
Data from the NHS England Access Scheme on the use of oncotype DX from 2015/16183 were used to model the levels of chemotherapy use resulting from the use of oncotype DX and from the use of standard practice. These data were provided as AiC and cannot be reported here.
Risk of distant recurrence
The 10-year risk of distant recurrence in accordance with oncotype DX Breast Recurrence Score was taken from Dowsett et al. ;37 the proportion of patients in each recurrence score category is common to both modelled groups (Table 72). Based on the assumptions employed in the model reported by Ward et al. ,1 the ROR is tapered to be 50% of the estimated risk during years 11–15 and 0% thereafter. The RR of distant recurrence for chemotherapy versus no chemotherapy is taken from Paik et al. 50 Within the oncotype DX group, it is assumed that the oncotype DX test is predictive of chemotherapy benefit. As shown in Table 72, the RR applied is dependent on the oncotype DX risk group, with the largest treatment effect applied in the high recurrence score group. In contrast, within the standard care group, the model assumes that the RR associated with chemotherapy is constant across all patients.
Node-positive patients
The Genomic Health dossier includes a secondary analysis that explores the use of oncotype DX in ER+ LN+ patients. The main differences between this analysis and the base-case LN0 analysis are summarised in Table 73. The proportion of patients receiving chemotherapy in the oncotype DX group was based on Loncaster et al. ,145 which resulted in a 69.2% reduction in chemotherapy following the use of the test. It should be noted that unlike the base case, the analysis in the LN+ population did not use DRFS to estimate chemotherapy benefit; instead, DFS rates were derived from Albain et al. 53 (this same approach is used within the EAG’s sensitivity analyses). The dossier states that only RRs for chemotherapy that were statistically significant were used; if this statement was accurate, this would result in a RR of 1.0 for the low recurrence score and intermediate recurrence score group and 0.59 in the high recurrence score group. However, the Genomic Health model inputs do not reflect this: all reported RRs were used, irrespective of whether or not they were associated with a statistically significant difference (see Table 73).
Population | Percentage receiving chemotherapy | 10-year cumulative probability of distant recurrence (no chemotherapy) (%) | RR with chemotherapy | ||
---|---|---|---|---|---|
Current practice group | Oncotype DX group | Current practice | Oncotype DX | ||
Low RS | 100 | 7.5 | 40 | 0.72 | 1.02 |
Intermediate RS | 100 | 63.2 | 51 | 0.72 | 0.72 |
High RS | 100 | 83.3 | 57 | 0.72 | 0.59 |
Comparison of oncotype DX and other tests (MammaPrint, EndoPredict score, EndoPredict Clinical and Prosigna)
In order to estimate the proportion of patients receiving chemotherapy in the comparator group, data on concordance between oncotype DX and the comparator tests were used to recategorise patients in the NHS England Access Scheme Database. 183 The proportions of patients receiving chemotherapy in each oncotype DX Breast Recurrence Score group are shown in Table 74. For MammaPrint, concordance data from Shivers et al. ,401 a US study with 135 patients, were used. For EndoPredict, data from Varga et al. ,410 a small study of 24 patients in Germany and Switzerland, were used. For Prosigna, a US study of 52 patients was used. 462 For MammaPrint, EndoPredict and Prosigna, it was assumed that 100% of high-risk and 0% of low-risk patients in the comparator group would receive chemotherapy, and, for Prosigna, 50% of the intermediate-risk group were assumed to receive chemotherapy. With the exception of the comparator test cost, all other parameters were held at the base-case values.
Oncotype DX RS group | Proportion of patients | Proportion of patients receiving chemotherapy by test (%) | |||
---|---|---|---|---|---|
MammaPrint | EndoPredict score | EPClin | Prosigna | ||
Low RS | Confidential information has been removed | 29 | 40 | 27 | 26 |
Intermediate RS | Confidential information has been removed | 51 | 80 | 50 | 38 |
High RS | Confidential information has been removed | 86 | 100 | 67 | 50 |
Results of the Genomic Health model
The Genomic Health deterministic base-case analysis indicates that oncotype DX produces positive health gains [0.03 life-years gained (LYGs) and 0.07 QALYs] at an additional cost of (confidential information has been removed); this corresponds to an ICER of (confidential information has been removed) per QALY gained. The results are driven by an overall reduction in chemotherapy levels in women with a low or intermediate oncotype DX Breast Recurrence Score (who benefit less from chemotherapy) and an increase in chemotherapy levels in those with a high oncotype DX Breast Recurrence Score (who benefit more from chemotherapy). The company’s probabilistic results indicate that the modelled estimates of incremental QALYs and costs are associated with considerable uncertainty. The cost-effectiveness plane generated using the Genomic Health model shows a wide dispersion of results, with a substantial number of samples being in the north-west quadrant (dominated) and the south-east quadrant (dominating). It should be noted that the cost-effectiveness plane presented in Figure 6 of the Genomic Health62 dossier appears very different from that generated by the EAG using the model; the reasons for this are unclear. The CEAC generated using the model suggests that the probability that oncotype DX produces more net benefit than current practice at WTP thresholds of £20,000 and £30,000 per QALY gained is approximately 0.51 and 0.52, respectively. The EAG has concerns regarding the robustness of the company’s probabilistic ICER as different model runs produced very different results, ranging from < £10,000 per QALY gained to > £170,000 per QALY gained.
The results for the LN+ population and for the LN0 population comparing oncotype DX with the other four tests are presented in Table 75. These analyses consistently indicate that, using mean values, oncotype DX dominates the comparators. As with the LN+ analysis, the cost-effectiveness plane presented in the Genomic Health62 dossier (Figure 6) shows a wide dispersion of results, with a large proportion of samples in the north-west (dominated) and south-east (dominating) quadrants.
Option | LYGs | QALYs | Costs | Incremental LYGs | Incremental QALYs | Incremental costs | ICER (per QALY gained) |
---|---|---|---|---|---|---|---|
Analysis 1: LN0 – comparison vs. standard care | |||||||
Oncotype DX | 12.82 | 10.50 | Confidential information has been removed | 0.03 | 0.07 | Confidential information has been removed | Confidential information has been removed |
Standard care | 12.80 | 10.43 | Confidential information has been removed | – | – | – | – |
Analysis 2: LN+ comparison vs. standard care | |||||||
Oncotype DX | 12.95 | 10.60 | Confidential information has been removed | –0.05 | 0.15 | Confidential information has been removed | Dominating |
Standard care | 13.00 | 10.44 | Confidential information has been removed | – | – | – | – |
Analysis 3: LN0 – comparison with MammaPrint | |||||||
Oncotype DX | 12.82 | 10.50 | £6319 | 0.02 | 0.07 | –£1272 | Dominating |
MammaPrint | 12.80 | 10.43 | £7590 | – | – | – | – |
Analysis 4: LN0 – comparison vs. EndoPredict score alone | |||||||
Oncotype DX | 12.82 | 10.50 | £6139 | 0.01 | 0.08 | –£762 | Dominating |
EndoPredict | 12.82 | 10.41 | £7081 | – | – | – | – |
Analysis 5: LN0 – comparison vs. EPClin score | |||||||
Oncotype DX | 12.82 | 10.50 | £6319 | 0.03 | 0.06 | –£532 | Dominating |
EndoPredict | 12.80 | 10.44 | £6850 | – | – | – | – |
Analysis 6: LN0 – comparison vs. Prosigna | |||||||
Oncotype DX | 12.82 | 10.50 | £6319 | 0.03 | 0.06 | –£655 | Dominating |
Prosigna | 12.79 | 10.44 | £6974 | – | – | – | – |
The company’s one-way sensitivity analyses (not shown) indicate that the model results are sensitive to changes in several parameter values including the time horizon, the discount rate, the disutility associated with chemotherapy and current levels of chemotherapy use.
The EAG notes that given that most of the probabilistic samples suggest that oncotype DX either dominates or is dominated by current practice, it is surprising that none of the DSAs indicate this result.
Critical appraisal of the Genomic Health model
The EAG has several concerns regarding the Genomic Health model (Box 1). In particular, the EAG identified a number of programming errors within the model. As a consequence, the EAG does not consider the results of the Genomic Health model to be robust. The EAG’s concerns are discussed in more detail in the following sections.
-
Use of inappropriate structural assumptions that bias in favour of oncotype DX.
-
Inappropriate application of chemotherapy-related disutility over remaining patient lifetime.
-
Risk classification probabilities and distant recurrence rates derived from separate sources.
-
Application of NHS England Access Scheme Database to all LN0 patients.
-
Model errors.
Use of inappropriate structural assumptions that bias in favour of oncotype DX
The Genomic Health model assumes that the oncotype DX test is predictive of chemotherapy benefit. As shown in Table 72, this results in the RR for distant recurrence being dependent on the oncotype DX Breast Recurrence Score category, with the greatest chemotherapy benefit being applied to the high recurrence score group. In the model, the standard care arm mirrors the oncotype DX arm in that patients are also split into the three oncotype DX Breast Recurrence Score categories. The difference between the arms is the proportion of patients in each risk group who go on to receive adjuvant chemotherapy. However, as shown in Table 72, the RR used in the standard care arm is constant across all three risk groups and is based on the crude average of the three RRs reported in the Paik et al. 50 study. The distribution of patients between the three recurrence score groups differs between the NHS England Access Scheme Database183 used in the Genomic Health model and the Paik et al. 50 study and, therefore, the crude mean RR from Paik et al. does not represent the average RR for the population in the Genomic Health model. Furthermore, as oncotype DX only identifies patients who may benefit from chemotherapy, the same RR of distant recurrence by recurrence score category should be applied to both the modelled oncotype DX and current practice groups (by recurrence score score), as each group has exactly the same patient distribution across recurrence score scores. If a patient is identified by oncotype DX as being high risk, the benefit they accrue from adjuvant chemotherapy should be identical to that accrued by the same patient who receives chemotherapy without the test.
Inappropriate application of chemotherapy-related disutility over remaining patient lifetime
The QALY decrement resulting from the use of chemotherapy is applied during every cycle for the remainder of the modelled patients’ lifetimes. The EAG considers it unlikely that patients would suffer the adverse effects of adjuvant chemotherapy years after they have completed their treatment. This represents a very pessimistic assumption that increases the overall reduction in QALYs associated with chemotherapy and overestimates the benefits associated with reducing overall chemotherapy use.
Risk classification probabilities and distant recurrence rates derived from separate sources
The risk of distant recurrence and the proportion of patients in each oncotype DX Breast Recurrence Score category were taken from two separate studies (Dowsett et al. 37 and the NHS England Access Scheme Database183). The use of separate sources for these inputs may produce confounding due to differences in the characteristics of patients within each recurrence score category between the two sources. In addition, the EAG notes that the risk of distant recurrence in the Genomic Health model does not match the 9-year risk of distant recurrence for LN0 patients presented in Dowsett et al. 37 or the Genomic Health62 dossier.
Application of NHS England Access Scheme Database to all lymph node-negative patients
The NHS England Access Scheme Database183 is only applicable to women who are at clinical intermediate-risk based on the NPI or other clinical indicators. However, it is unclear from the Genomic Health dossier whether the model applies only to this population or whether the model is intended to reflect costs and outcomes of the oncotype DX test across the whole LN0 population.
Model errors
The EAG identified an error in the company’s calculations relating to the proportion of patients in the low-, intermediate- and high-risk groups who receive chemotherapy. The correct proportions are presented in the model; however, these are not applied directly but are instead incorrectly adjusted when used to calculate the traces for the Markov nodes (confidential information has been removed). 183 This error leads to a substantial underestimate of the number of patients receiving chemotherapy in both the intermediate- and high-risk groups and has a significant impact on the model results.
In addition, the results reported for the node-positive patients in the Genomic Health model could not be replicated by the EAG using the data described in the Genomic Health dossier. In order to replicate the results, two different sets of data were required. For the risk of distant recurrence, the Dowsett et al. 37 used in the base-case analysis had to be selected (rather than the appropriate Albain et al. 53 study). In addition, the results in the dossier use Paik et al. 50 (rather than the appropriate Albain et al. 53 study).
The impact of correcting the major errors in the Genomic Health model is explored further through comparison with the EAG model in Chapter 3, Comparison between the Genomic Health model, the current External Assessment Group model and the previous External Assessment Group model (lymph node negative, clinical intermediate-risk subgroup).
EndoPredict draft cost-effectiveness paper (Myriad Genetics)182
Model scope
The chief investigator of the EndoPredict decision impact study159 made available a draft manuscript that outlines the methods and results of an economic analysis comparing of EPClin plus AOL versus AOL only in women with ER+, HER2– early-stage breast cancer, having had an intermediate-risk score using AOL. The EAG notes that the AOL risk interval is not explicitly defined. The executable model was not made available, hence the EAG was unable to verify whether it has been implemented appropriately.
The manuscript presents two sets of analyses: (1) a short-term cost-minimisation analysis of EPClin versus usual practice (including only chemotherapy acquisition costs and the costs of providing the EPClin test), and (2) a cost-effectiveness analysis of EndoPredict plus AOL versus AOL only from the perspective of the NHS over a lifetime horizon. Most of the details of the Myriad model182 were provided in confidence and cannot be reported here.
Results of the Myriad model
The cost-minimisation analysis suggests that EndoPredict led to a small but non-statistically significant increase in the mean per-patient cost of acquisition and provision of chemotherapy to the NHS of £149.
The cost-effectiveness analysis suggests that the ICER for EndoPredict plus AOL versus AOL is £26,836 per QALY gained. 182
The critical appraisal of the Myriad model was held as confidential and cannot be reported here.
Summary of economic evidence made available to the External Assessment Group
The EAG has concerns regarding the economic evidence for oncotype DX and EndoPredict that was made available to it. In particular, the Genomic Health model for oncotype DX includes a number of errors and, in the opinion of the EAG, unreasonable assumptions. The EAG did not receive a model for EndoPredict and, therefore, cannot comment fully on the reliability of the results presented. No economic evidence was provided by the manufacturers of Prosigna or IHC4. Agendia submitted a model, which was critiqued by the EAG as part of the assessment process, but it cannot be reported here as Agendia withdrew permission to reproduce the model.
Appendix 8 External Assessment Group model input parameter tables
Test (number of samples) | Proportion of patients with risk classificationa | ||
---|---|---|---|
Low | Intermediate | High | |
LN0 NPI ≤ 3.4 | |||
Oncotype DX (541) | 0.72 | 0.24 | 0.04 |
Prosigna (410) | 0.72 | 0.24 | 0.03 |
IHC4+C (510) | 0.88 | 0.11 | 0.01 |
EPClin (423) | 0.90 | – | 0.10 |
LN0 NPI > 3.4 | |||
Oncotype DX (284) | 0.50 | 0.31 | 0.19 |
Prosigna (253) | 0.27 | 0.38 | 0.35 |
IHC4+C (279) | 0.36 | 0.38 | 0.25 |
EPClin (254) | 0.47 | – | 0.53 |
LN1–3 | |||
Oncotype DX (219) | 0.57 | 0.32 | 0.11 |
Prosigna (192) | 0.08 | 0.32 | 0.60 |
IHC4+C (213) | 0.28 | 0.34 | 0.38 |
EPClin (198) | 0.24 | – | 0.76 |
Population | Proportion of patients with risk classification | |
---|---|---|
MammaPrint low risk | MammaPrint high risk | |
MINDACT ITT population (n = 6693) | 0.64 | 0.36 |
MINDACT mAOL clinical high-risk subgroup (n = 3370) | 0.46 | 0.54 |
MINDACT mAOL clinical high-risk subgroup (n = 3324) | 0.82 | 0.18 |
Population | 10-year DMFI (95% CI) | |||
---|---|---|---|---|
Oncotype DXa | Prosigna | IHC4+C | EPClin | |
LN0, NPI ≤ 3.4, low risk | 0.983 (0.963 to 0.992) | 0.986 (0.962 to 0.995) | 0.975 (0.954 to 0.987) | 0.971 (0.947 to 0.984) |
LN0, NPI ≤ 3.4, intermediate risk | 0.931 (0.867 to 0.965) | 0.933 (0.857 to 0.969) | 0.878 (0.747 to 0.943) | N/A |
LN0, NPI ≤ 3.4, high risk | 0.838 (0.577 to 0.945) | 0.636 (0.297 to 0.845) | 0.800 (0.204 to 0.969) | 0.870 (0.714 to 0.944) |
LN0, NPI > 3.4, low risk | 0.854 (0.776 to 0.907) | 0.923 (0.825 to 0.967) | 0.873 (0.787 to 0.926) | 0.848 (0.761 to 0.905) |
LN0, NPI > 3.4, intermediate risk | 0.798 (0.694 to 0.869) | 0.796 (0.687 to 0.870) | 0.788 (0.688 to 0.859) | N/A |
LN0, NPI > 3.4, high risk | 0.749 (0.598 to 0.851) | 0.699 (0.584 to 0.788) | 0.769 (0.645 to 0.855) | 0.774 (0.688 to 0.838) |
LN1–3, low risk | 0.818 (0.727 to 0.880) | 1 (N/A) | 0.961 (0.851 to 0.990) | 0.95 (0.811 to 0.988) |
LN1–3, intermediate risk | 0.754 (0.630 to 0.842) | 0.807 (0.679 to 0.889) | 0.758 (0.635 to 0.845) | N/A |
LN1–3, high risk | 0.686 (0.447 to 0.839) | 0.707 (0.604 to 0.788) | 0.672 (0.546 to 0.771) | 0.716 (0.629 to 0.785) |
Randomised group | Treatment | EAG group labelc | Number randomised before genomic correction | Number randomised after genomic correction | Number in groupa | Percentage of overall trial population | 5-year DMFS (%) | 5-year cumulative DMFS probability (%) | Distant metastases event rate (year) | 10-year DMFS probability for group | 6-month recurrence probability for group |
---|---|---|---|---|---|---|---|---|---|---|---|
mAOL low risk, MammaPrint low risk | Chemotherapy | A | 2634 | 2745 | 37 | 0.55 | 97.60 | 2.40 | 0.005 | 0.953 | 0.002 |
No chemotherapy | B | 2708 | 40.46 | 97.60 | 2.40 | 0.005 | 0.953 | 0.002 | |||
mAOL high risk, MammaPrint low risk | Chemotherapy | C | 1497 | 1550 | 793 | 11.85 | 95.90 | 4.10 | 0.008 | 0.920 | 0.004 |
No chemotherapy | D | 757 | 11.31 | 94.40 | 5.60 | 0.012 | 0.891 | 0.006 | |||
mAOL low risk, MammaPrint high risk | Chemotherapy | E | 690 | 592 | 296 | 4.42 | 95.80 | 4.20 | 0.009 | 0.918 | 0.004 |
No chemotherapy | F | 296 | 4.42 | 95.00 | 5.00 | 0.010 | 0.903 | 0.005 | |||
mAOL high risk, MammaPrint high risk | Chemotherapy | G | 1873 | 1806 | 1735 | 25.92 | 90.60 | 9.40 | 0.020 | 0.821 | 0.010 |
No chemotherapy | H | 71 | 1.06 | 90.60 | 9.40 | 0.020 | 0.821b | 0.010 |
Group | Age (years) | |||||
---|---|---|---|---|---|---|
55–75 | ≤ 75 | |||||
ACT | No ACT | Percentage | ACT | No ACT | Percentage | |
LN0, NPI ≤ 3.4 | 329 | 4248 | 7.19 | 964 | 6008 | 13.83 |
LN0, NPI > 3.4 | 1388 | 2081 | 40.01 | 3265 | 2897 | 52.99 |
LN1–3 | 1849 | 1099 | 62.72 | 4557 | 1526 | 74.91 |
Source | Population | Proportion of patients receiving adjuvant chemotherapy conditional on test risk classification | ||
---|---|---|---|---|
Low risk | Intermediate risk | High risk | ||
NHS England Access Database183 | LN0, intermediate clinical risk | 0.01 | 0.33 | 0.89 |
Holt et al.201 | LN0 or pN1mic | 0.07 | 0.59 | 0.91 |
Bloomfield et al.159 | Unclear | 0.07 | N/A | 0.77 |
Loncaster et al.145 | LN0 | 0.02 | 0.51 | 0.85 |
LN+ | 0.08 | 0.63 | 0.83 | |
UKBCG survey (three-level tests) | LN0, NPI ≤ 3.4 | 0.00 | 0.17 | 0.74 |
LN0, NPI > 3.4 | 0.04 | 0.41 | 0.92 | |
LN1–3 | 0.46 | 0.76 | 0.95 | |
UKBCG survey (two-level tests) | LN0, NPI ≤ 3.4 | 0.01 | N/A | 0.71 |
LN0, NPI > 3.4 | 0.15 | N/A | 0.92 | |
LN1–3 | 0.40 | N/A | 0.97 |
Test risk group | 10-year RR of distant recurrence: chemotherapy vs. no chemotherapy | ||
---|---|---|---|
EAG base case: oncotype DX, Prosigna, EPClin, IHC4+C, non-predictive (EBCTCG199) | EAG sensitivity analysis: oncotype DX, predictive benefit (Paik et al.50 and Albain et al.53) | EAG base case: MammaPrint MINDACT population, non-predictive (Cardoso et al.98) | |
LN0 subgroups (NPI ≤ 3.4 and NPI > 3.4) | |||
Low risk | 0.76 | 1.31a | – |
Intermediate risk | 0.76 | 0.61a | – |
High risk | 0.76 | 0.26a | – |
LN1–3 subgroup | |||
Low risk | 0.76 | 1.02a,b | – |
Intermediate risk | 0.76 | 0.72a,b | – |
High risk | 0.76 | 0.59a,b | – |
MINDACT ITT population | |||
MammaPrint low risk | – | – | 0.77 |
MammaPrint high risk | – | – | 0.77 |
MINDACT mAOL low risk | |||
MammaPrint low risk | – | – | 0.84 |
MammaPrint high risk | – | – | 0.84 |
MINDACT mAOL high risk | |||
MammaPrint low risk | – | – | 0.74 |
MammaPrint high risk | – | – | 0.74 |
Author | Publication type | Country | Population | Health state description | EQ-5D valuation for health state |
---|---|---|---|---|---|
Farkkila et al.204 | Abstract | Finland | 778 breast cancer patients aged 31–90 years in the Hospital District of Helsinki and Uusimaa | Baseline | 0.818 (SD 0.228) |
First year of remission | 0.860 (SD 0.178) | ||||
Following years after remission | 0.843 (SD 0.189) | ||||
Metastatic disease | 0.746 (SD 0.251) | ||||
Palliative patients | 0.514 (SD 0.300) | ||||
Lidgren et al.191 | Paper | Sweden | 361 consecutive breast cancer patients attending the breast cancer outpatient clinic at Karolinska University Hospital Solna for outpatient visits between April and May 2005 | First year after primary breast cancera | 0.696 (95% CI 0.634 to 0.747) |
First year after recurrence | 0.779 (95% CI 0.700 to 0.849) | ||||
Second and following years after primary breast cancer/recurrence | 0.779 (95% CI 0.745 to 0.811) | ||||
Metastatic disease | 0.685 (95% CI 0.620 to 0.735) | ||||
Naik et al.206 | Paper | Canada | 1759 ambulatory cancer survivors at the Princess Margaret Cancer Centre (mixed cohort with various cancer types, 282 patients with breast cancer) | Breast local/regional | 0.82 (SE 0.01) |
Breast distant/metastatic | 0.75 (SE 0.03) | ||||
Yousefi et al.205 | Paper | Iran | 163 patients with breast cancer who attended the breast cancer subspecialty clinic affiliated with the Breast Cancer Research Center (BCRC), in Tehran, Iran | First year after primary breast cancer | 0.674 (SD 0.201) |
First year after recurrence | 0.718 (SD 0.139) | ||||
Second and following years after primary breast cancer/recurrence | 0.730 (SD 0.221) | ||||
Metastatic disease | 0.552 (SD 0.227) |
Health state/event | Duration applied in model | Mean utility | SE | Source |
---|---|---|---|---|
Recurrence free | Indefinite | 0.824 | 0.018 | Lidgren et al.191 |
Distant metastases | Indefinite | 0.685 | 0.029 | |
Disutility distant metastases | Indefinite | –0.14 | 0.11 | Calculated using difference method |
Local recurrence | Once-only QALY loss applied on transition to distant recurrence state | –0.108 | 0.04 (assumed) | Campbell et al.193 |
Chemotherapy AEs | Once-only QALY loss applied in first cycle | –0.038 | 0.004 (assumed) | |
AML | Indefinite | 0.26 | 0.04 (assumed) | Younis et al.192 |
Test | Cost | Comments |
---|---|---|
Oncotype DX (excluding PAS) | £2580 | Tests carried out in Genomic Health laboratory in USA. Cost includes sample handling and customer service |
Prosignaa | £1970 | NanoString Technologies submitted a cost per Prosigna test based on conducting the test in a NHS laboratory, which included the laboratory costs (£240), the list price for Prosigna kits (£1650), cost of the nCounter System (£194,600) and was based on 2500 samples per lifetime of the nCounter System |
EndoPredicta | £1500 | Tests carried out in Myriad’s laboratory in Munich |
IHC4 | £203 | IHC4 submitted a document outlining the time and equipment necessary to conduct the test in 2014 prices. The total cost of the test (£198) was uplifted using the HCHS index195 |
MammaPrint | £2326 | Converted from Euros to UK Pounds Sterling assuming an exchange rate of 1.15 |
Regimen | Proportion of women receiving regimen | Costs | ||||||||
---|---|---|---|---|---|---|---|---|---|---|
Central line | Drug | Delivery | Supportive medicines | Medical oncology | Specialist nurse review | Blood tests | Toxicity | Total | ||
FEC100-T (3 + 3 cycles) | 0.25 | £18.17 | £306.84 | £1284.58 | £435.64 | £450.03 | £613.10 | £62.32 | £378.20 | £3548.88 |
TC (4 cycles) | 0.20 | £18.17 | £52.80 | £856.39 | £15.91 | £310.81 | £408.74 | £41.55 | £144.83 | £1849.19 |
FEC75 (6 cycles) | 0.45 | £18.17 | £346.38 | £1284.58 | £80.77 | £310.81 | £613.10 | £62.32 | £245.91 | £2962.05 |
FEC100-Pw (3 + 3 cycles) | 0.10 | £18.17 | £274.53 | £2569.16 | £435.89 | £450.03 | £613.10 | £124.64 | £378.20 | £4863.72 |
Endocrine therapy | Proportion of patients | Dosage (per day) | Product | Price per pack | Annual cost | Source |
---|---|---|---|---|---|---|
Tamoxifen | 0.40 | 20 mg | 30 × 20-mg tablet (various manufacturers) | £2.88 | £35.06 | BNF194 |
Anastrozole | 0.20 | 1 mg | 28 × 1-mg tablet (various manufacturers) | £1.08 (NHS Drug Tariff price) | £14.09 | |
Letrozole | 0.20 | 2.5 mg | 28 × 2.5-mg tablet (Alliance Healthcare) | £2.52 | £32.87 | |
Exemestane | 0.20 | 25 mg | 30 × 25-mg tablet (various manufacturers) | £5.71 (NHS Drug Tariff price) | £69.52 |
Test risk classification | Post-test chemotherapy probability | |
---|---|---|
Node negative | Node positive | |
Low risk | 0.05 | 0.36 |
High risk | 0.92 | 0.99 |
Test risk classification | Post-test chemotherapy probability |
---|---|
Low risk | 0.01 |
High risk | 0.87 |
Oncotype DX risk classification | Probability (no test) |
---|---|
Low risk | Confidential information has been removed |
Intermediate risk | Confidential information has been removed |
High risk | Confidential information has been removed |
Test risk classification | Classification probability | 10-year DMFI |
---|---|---|
LN0, NPI ≤ 3.4 | ||
Low risk | 0.89 | 0.978 |
Intermediate risk | 0.09 | 0.839 |
High risk | 0.02 | 0.635 |
LN0, NPI > 3.4 | ||
Low risk | 0.52 | 0.858 |
Intermediate risk | 0.30 | 0.817 |
High risk | 0.18 | 0.762 |
Test risk classification | Classification probability | 10-year DMFS |
---|---|---|
Low risk | 0.04 | 1.00 |
Intermediate risk | 0.34 | 0.94 |
High risk | 0.62 | 0.76 |
Test risk classification | Classification probability | 10-year DMFS |
---|---|---|
Low risk | 0.24 | 0.95 |
High risk | 0.76 | 0.72 |
Test risk classification | Classification probability | 10-year DMFS |
---|---|---|
Low risk | 0.71 | 0.93 |
High risk | 0.29 | 0.85 |
Appendix 9 External Assessment Group post-test chemotherapy use survey disseminated to UK Breast Cancer Group members
The following questionnaire was circulated via e-mail to members of the UKBCG.
Appendix 10 Modelled chemotherapy use with and without tumour profiling tests (External Assessment Group model)
Subgroup | Test | No test | Net change |
---|---|---|---|
Oncotype DX vs. current practice | |||
LN0 NPI ≤ 3.4 | 0.076 | 0.072 | 0.004 |
LN0 NPI > 3.4 | 0.273 | 0.430 | –0.157 |
LN1–3 | 0.337 | 0.627 | –0.290 |
IHC4+C vs. current practice | |||
LN0 NPI ≤ 3.4 | 0.030 | 0.072 | –0.042 |
LN0 NPI > 3.4 | 0.355 | 0.430 | –0.075 |
LN1–3 | 0.554 | 0.627 | –0.073 |
Prosigna vs. current practice | |||
LN0 NPI ≤ 3.4 | 0.075 | 0.072 | 0.003 |
LN0 NPI > 3.4 | 0.435 | 0.430 | 0.005 |
LN1–3 | 0.709 | 0.627 | 0.082 |
EPClin vs. current practice | |||
LN0 NPI ≤ 3.4 | 0.140 | 0.072 | 0.068 |
LN0 NPI > 3.4 | 0.438 | 0.430 | 0.008 |
LN1–3 | 0.603 | 0.627 | –0.024 |
MammaPrint vs. current practice | |||
MINDACT overall population | 0.319 | 0.466 | –0.148 |
mAOL high risk | 0.445 | 0.772 | –0.327 |
mAOL low risk | 0.191 | 0.159 | 0.033 |
Appendix 11 External Assessment Group cost-effectiveness acceptability curves
Cost-effectiveness acceptability curves
Oncotype DX versus current practice
Node-negative Nottingham Prognostic Index score of ≤ 3.4
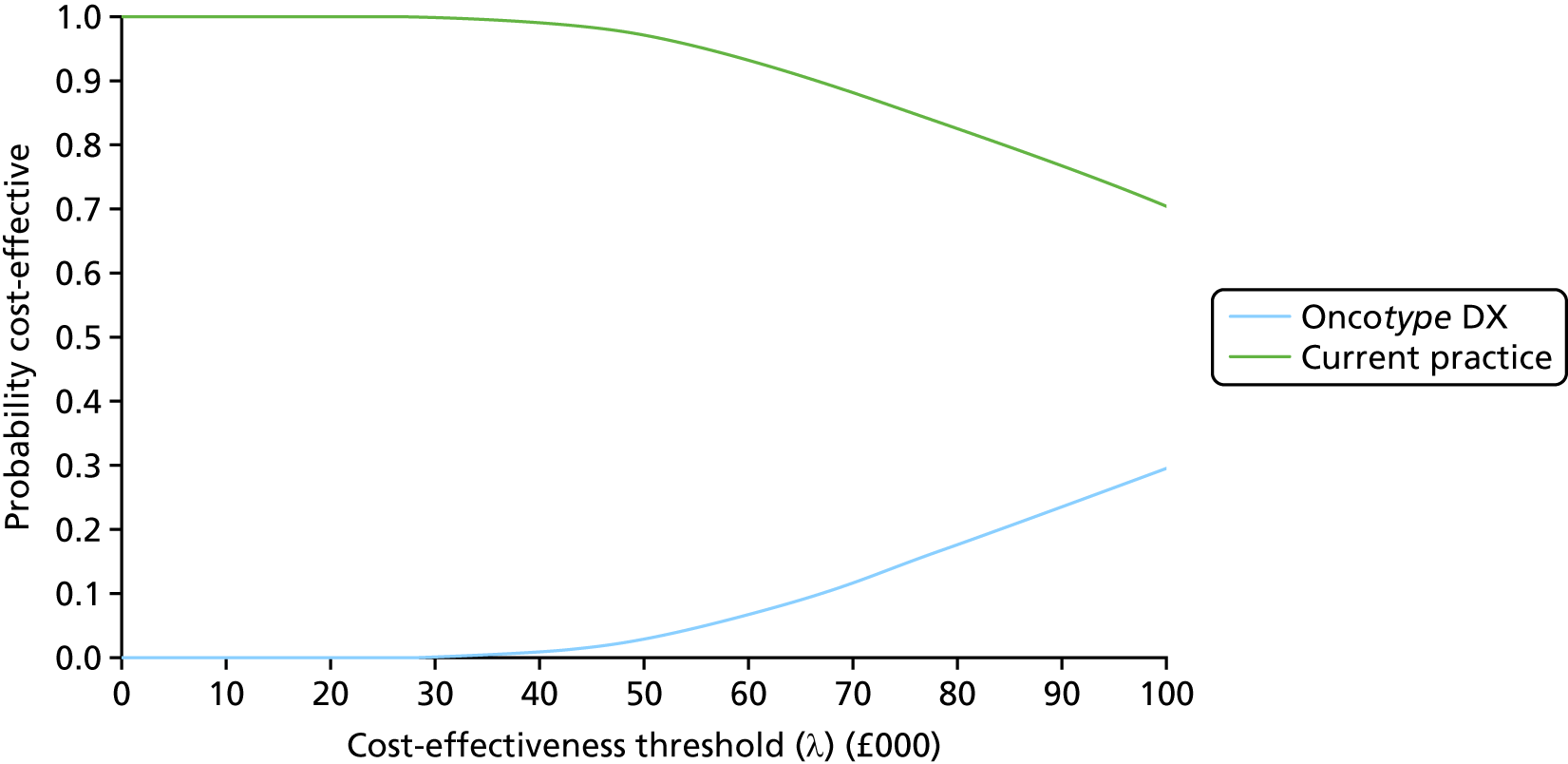
Node-negative Nottingham Prognostic Index score of > 3.4
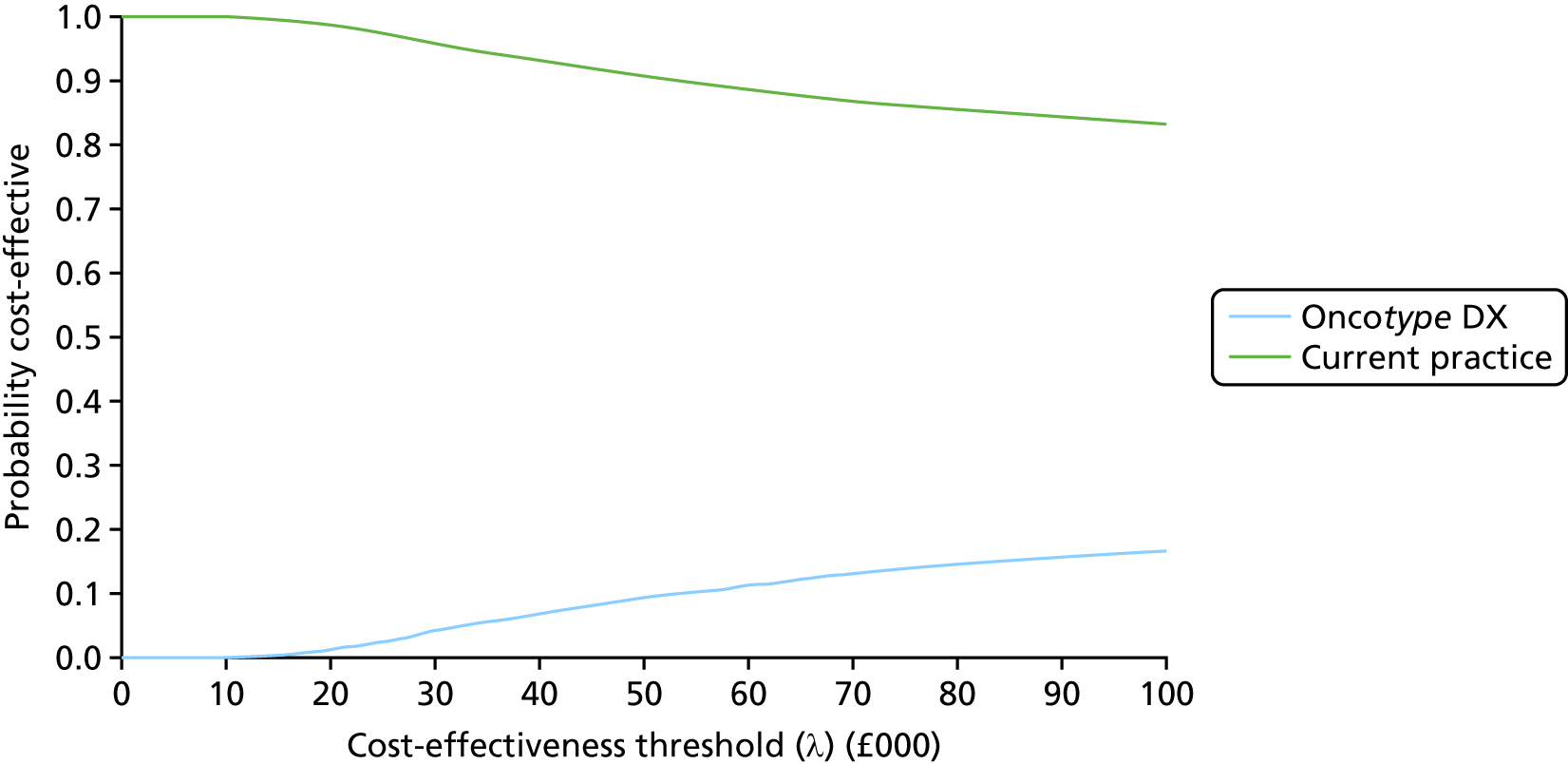
Node positive (one to three nodes)
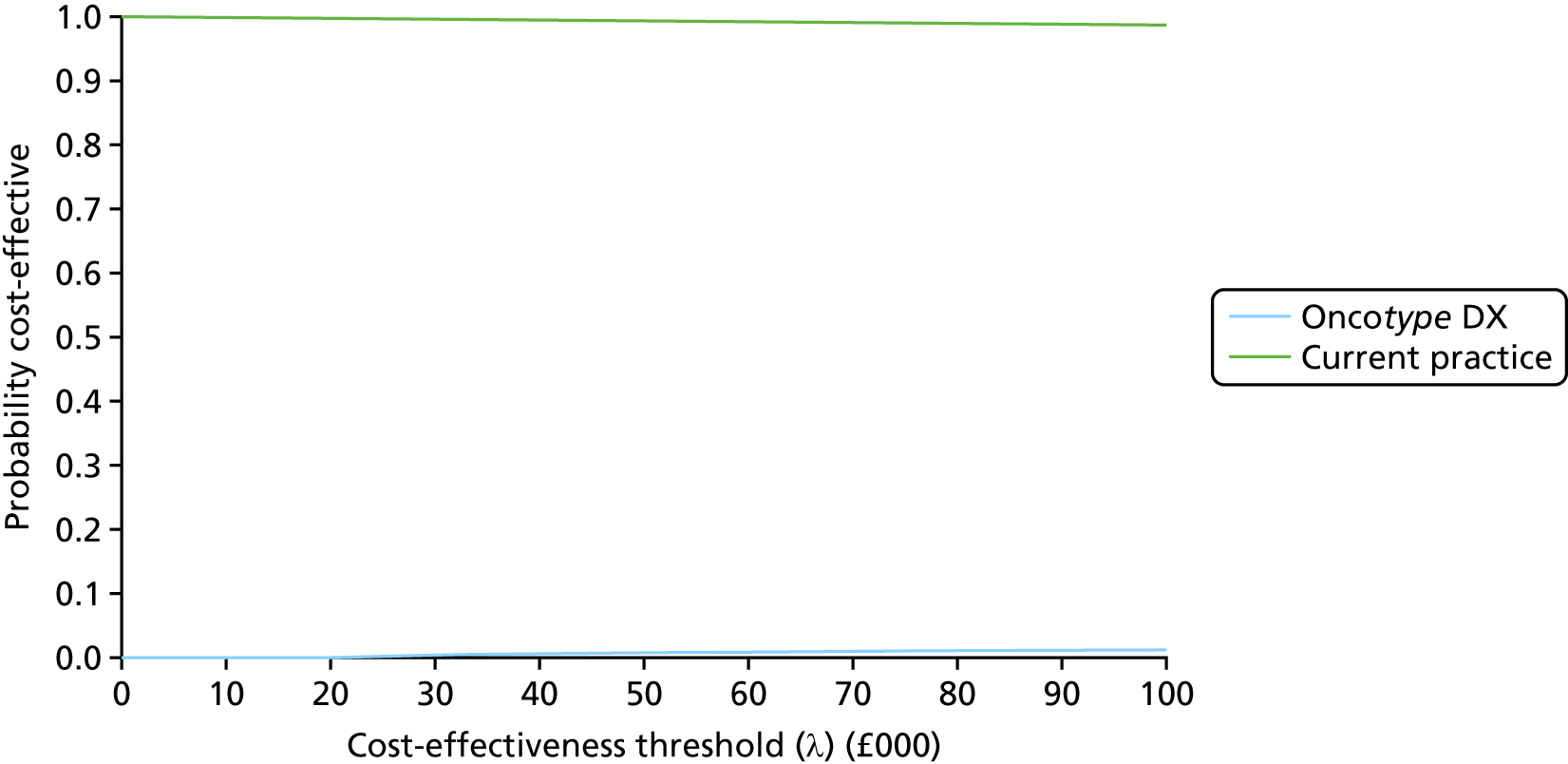
IHC4+C versus current practice
Node-negative Nottingham Prognostic Index score of ≤ 3.4
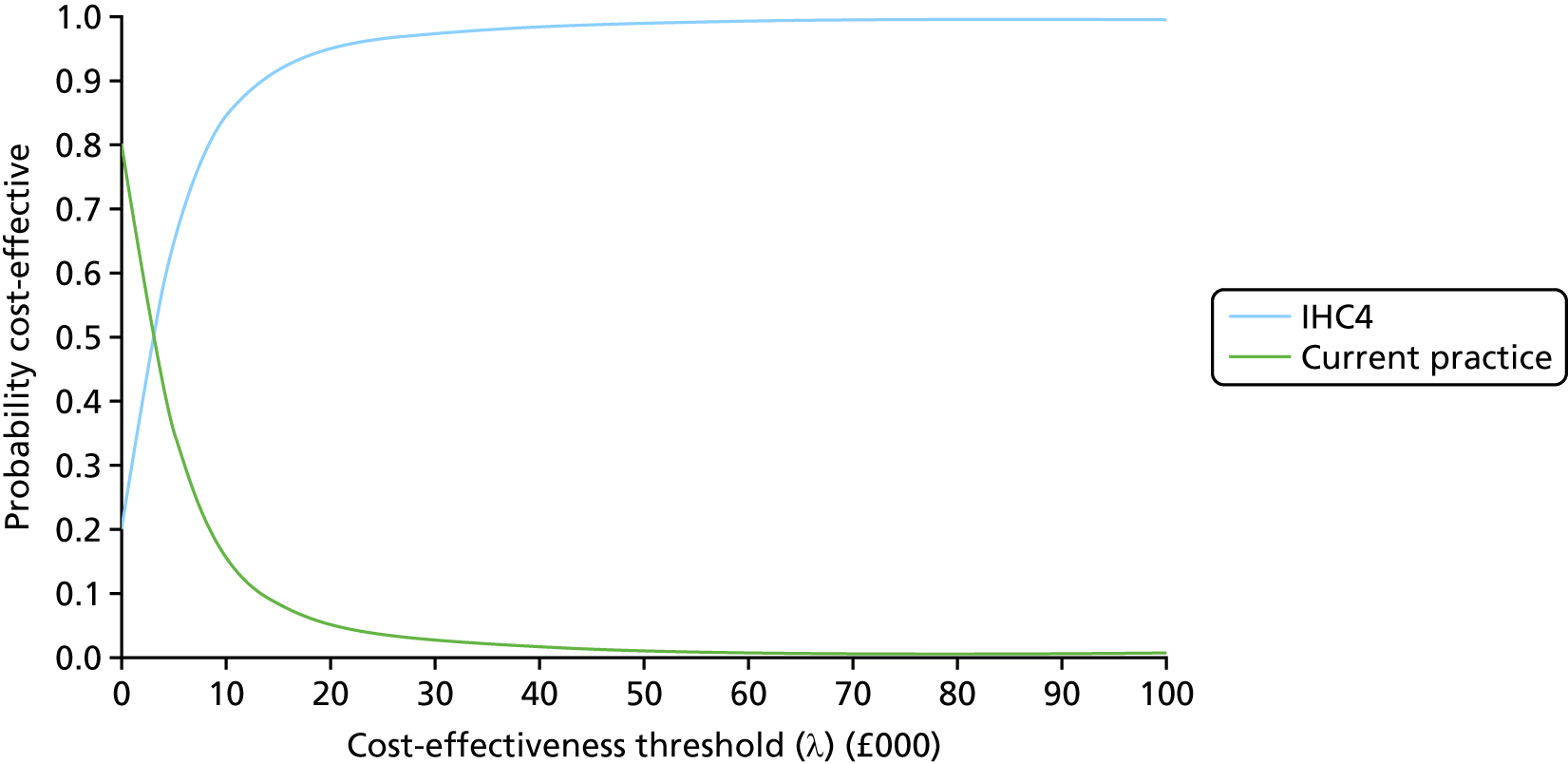
Node-negative Nottingham Prognostic Index score of > 3.4
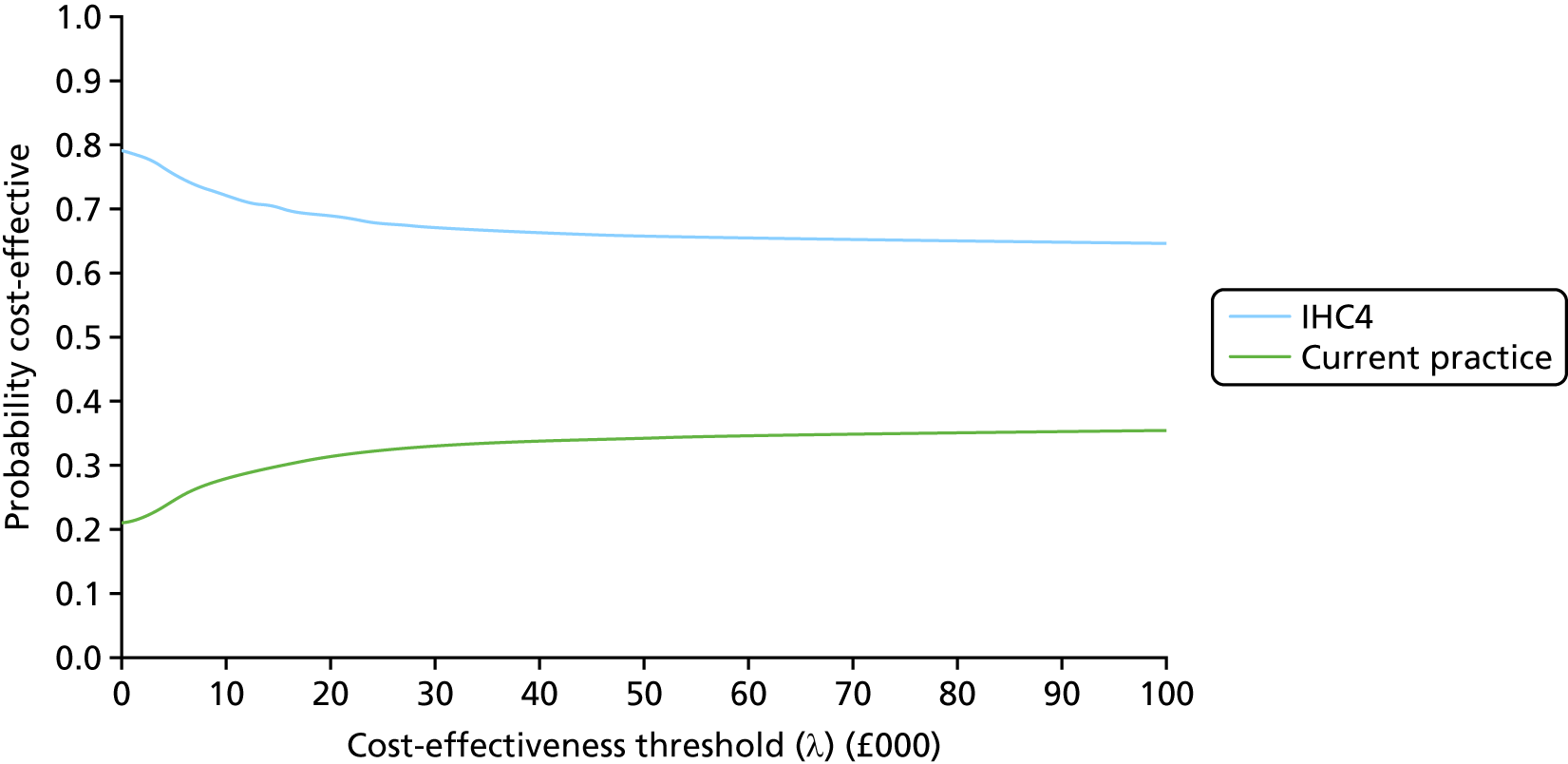
Node positive (one to three nodes)
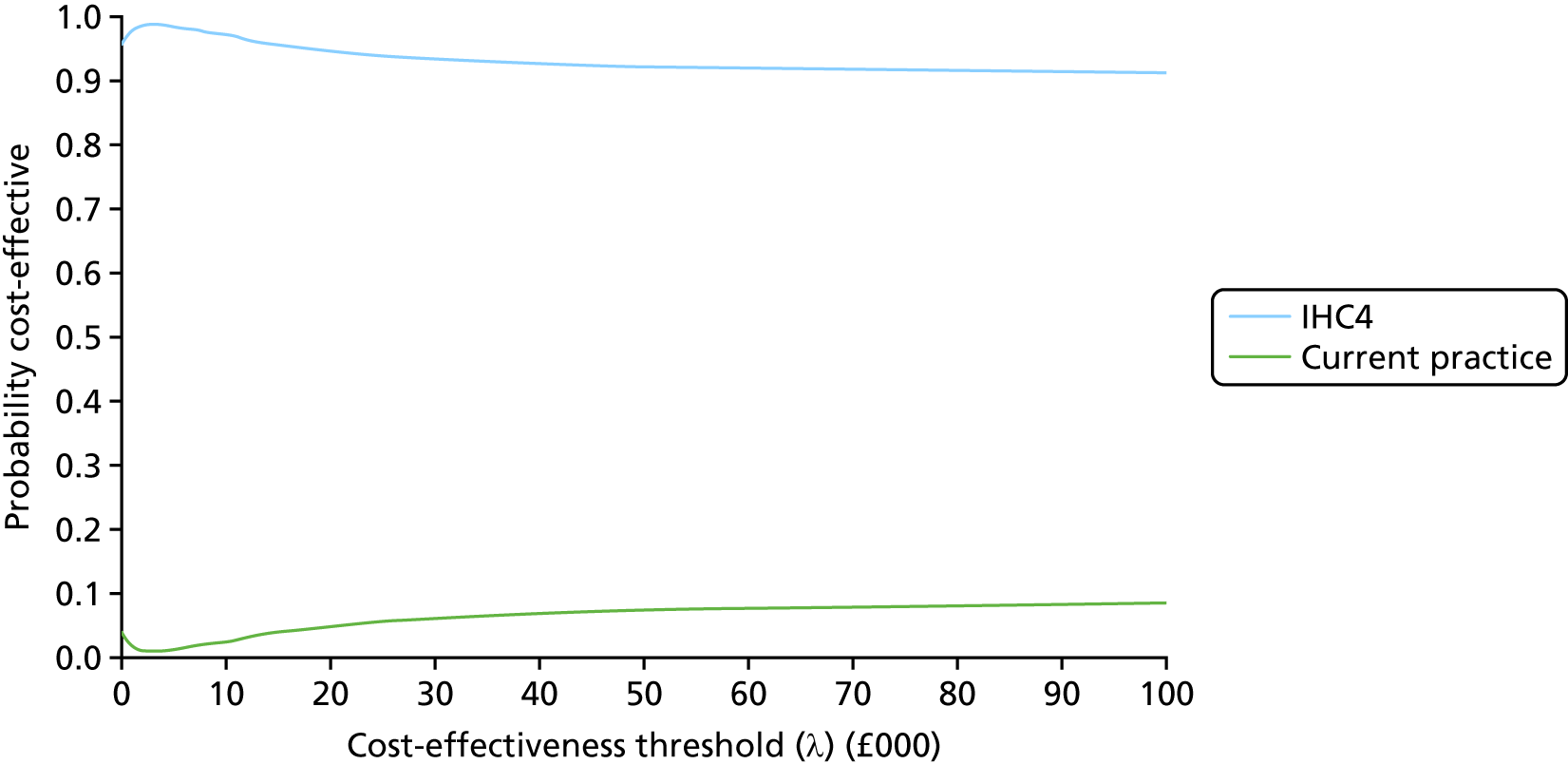
Prosigna versus current practice
Node-negative Nottingham Prognostic Index score of ≤ 3.4
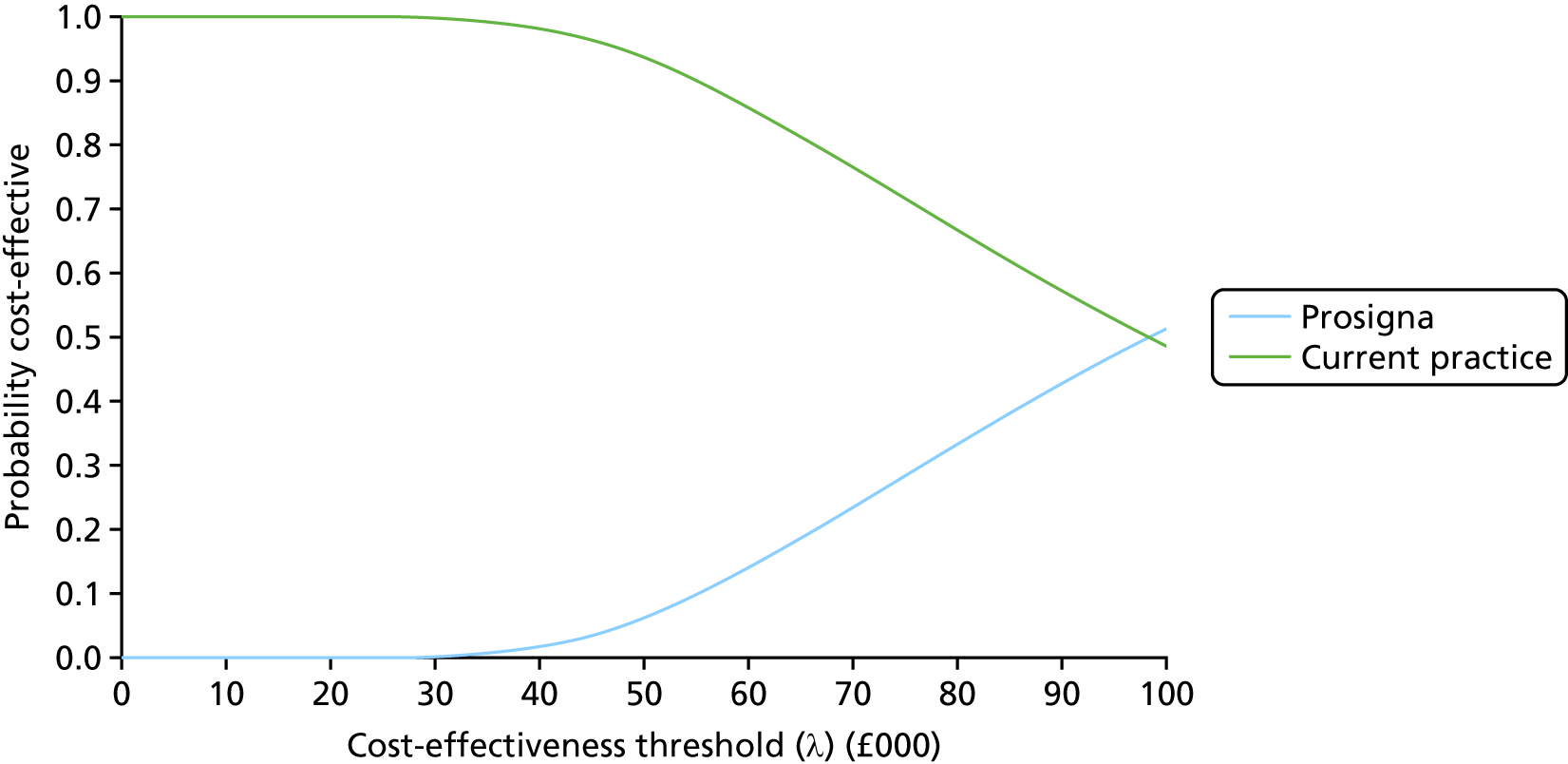
Node-negative Nottingham Prognostic Index score of > 3.4
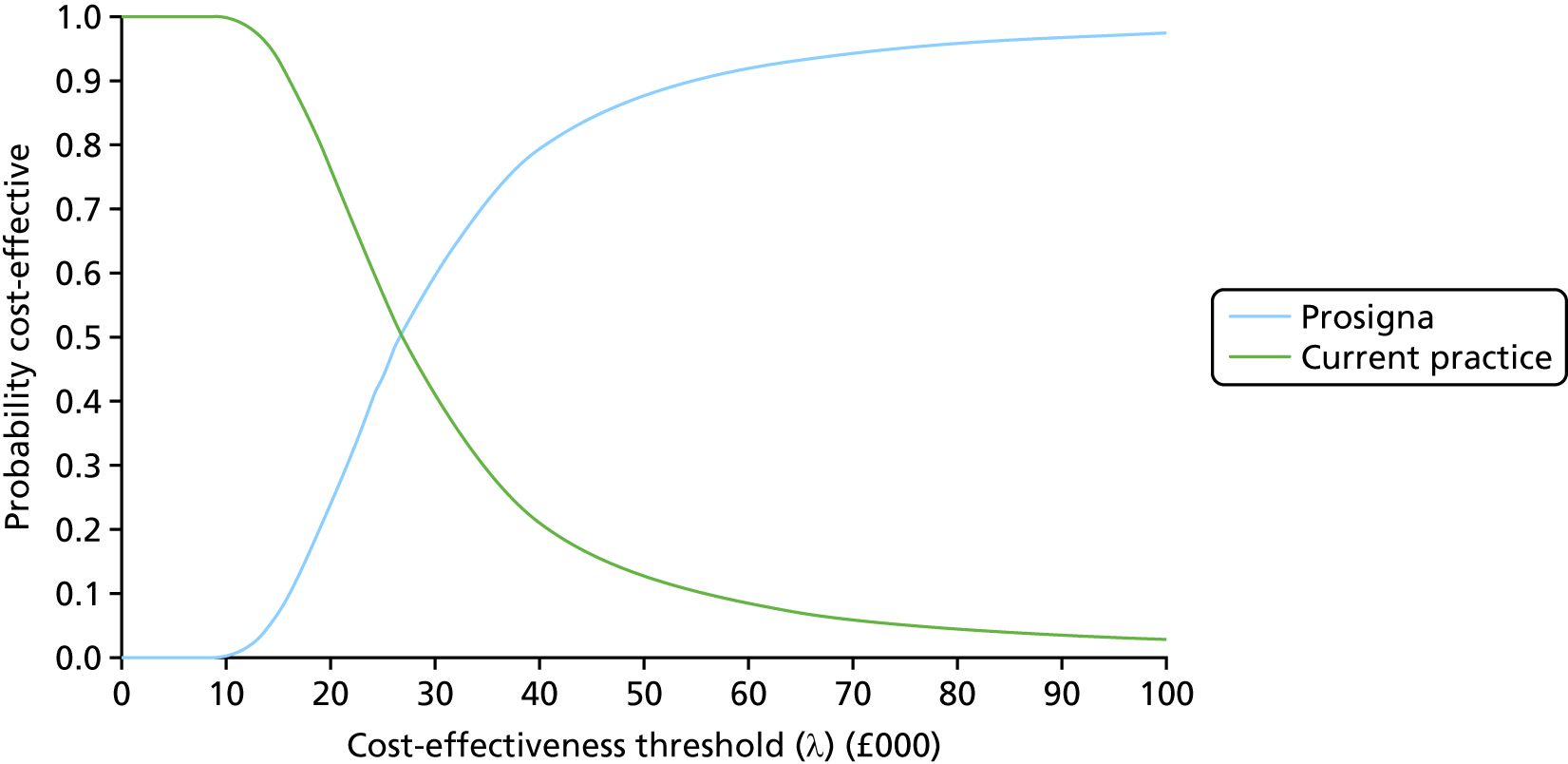
Node positive (one to three nodes)
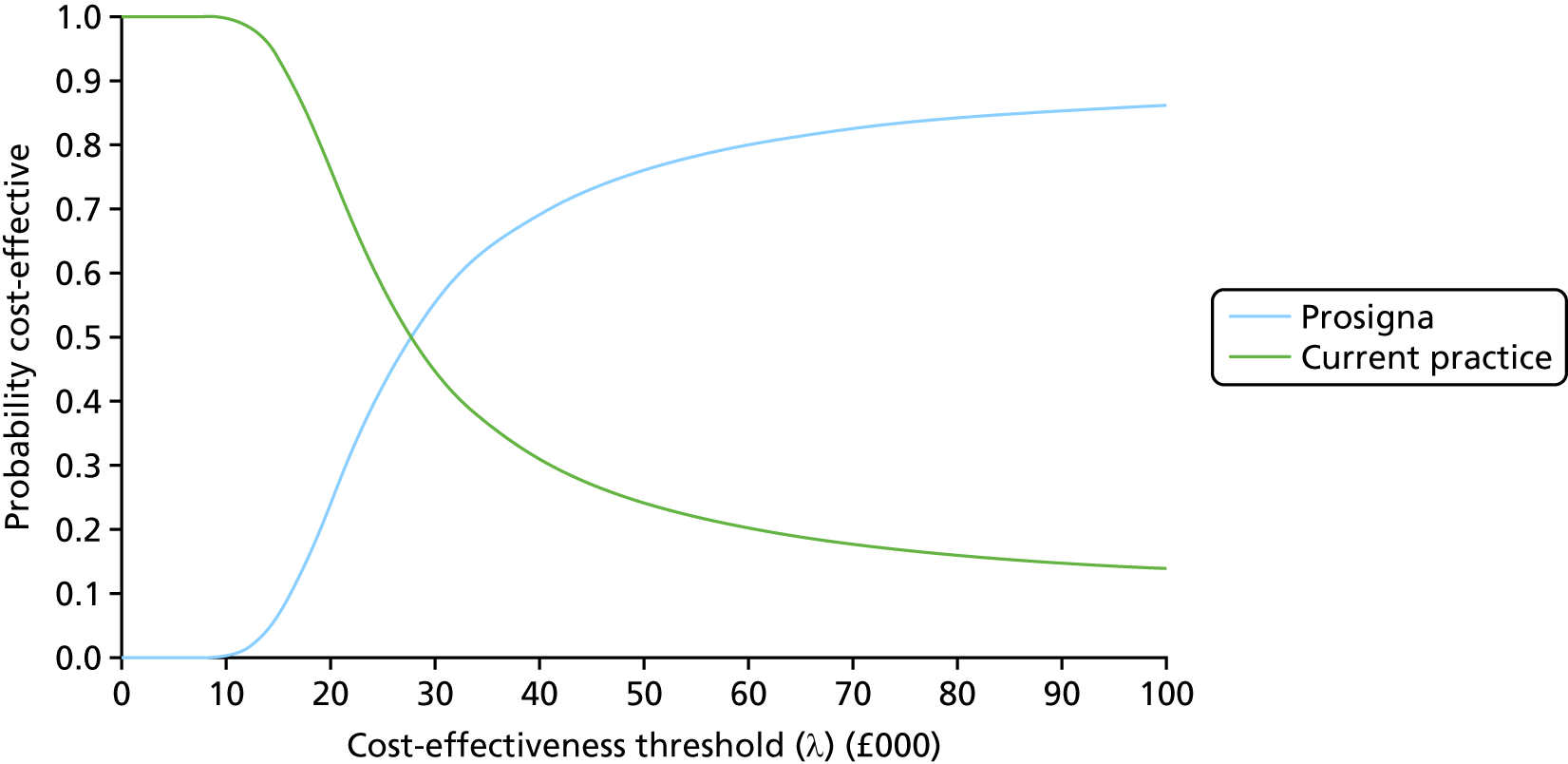
EndoPredict Clinical versus current practice
Node-negative Nottingham Prognostic Index score of ≤ 3.4
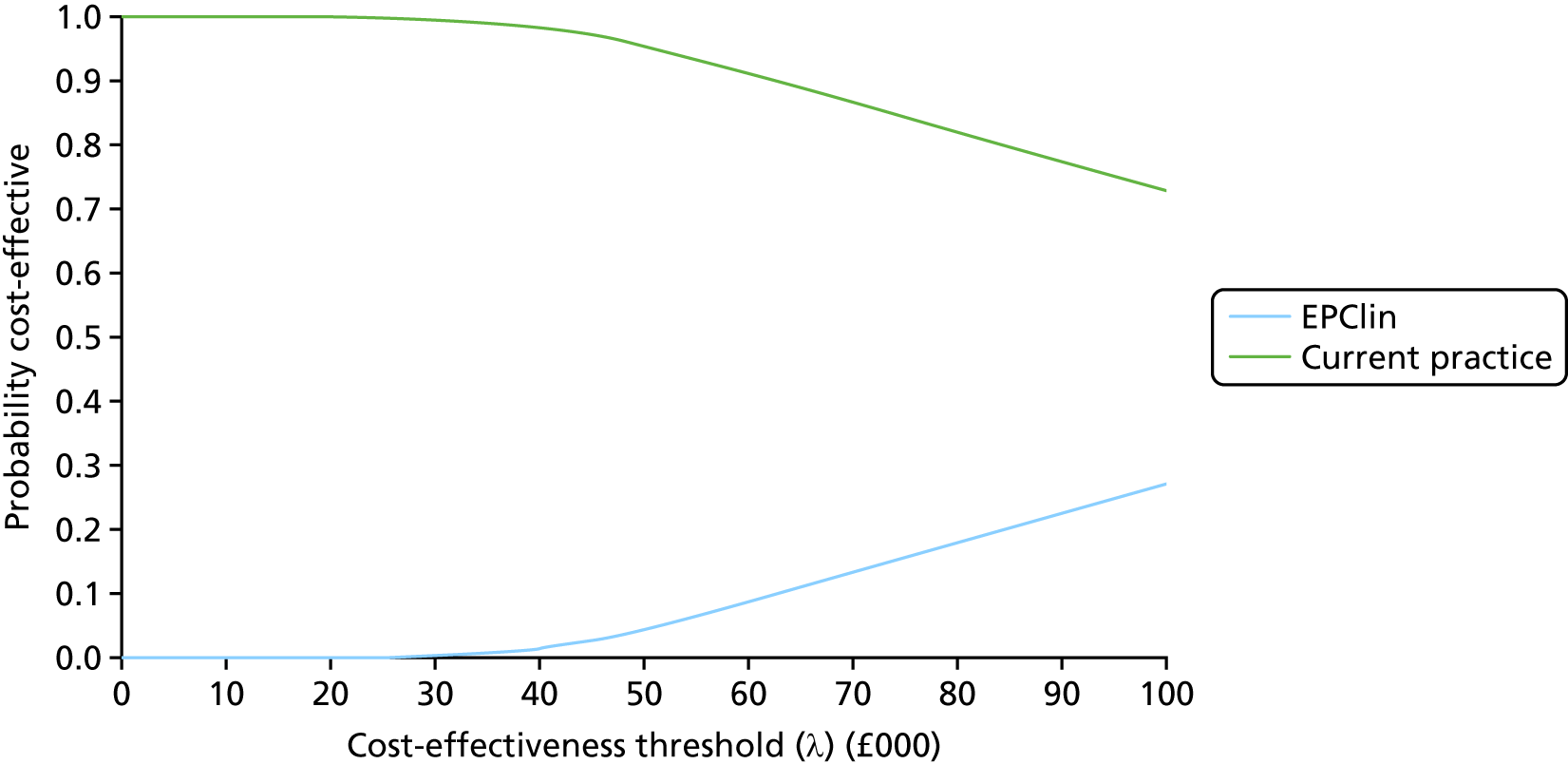
Node-negative Nottingham Prognostic Index score of > 3.4
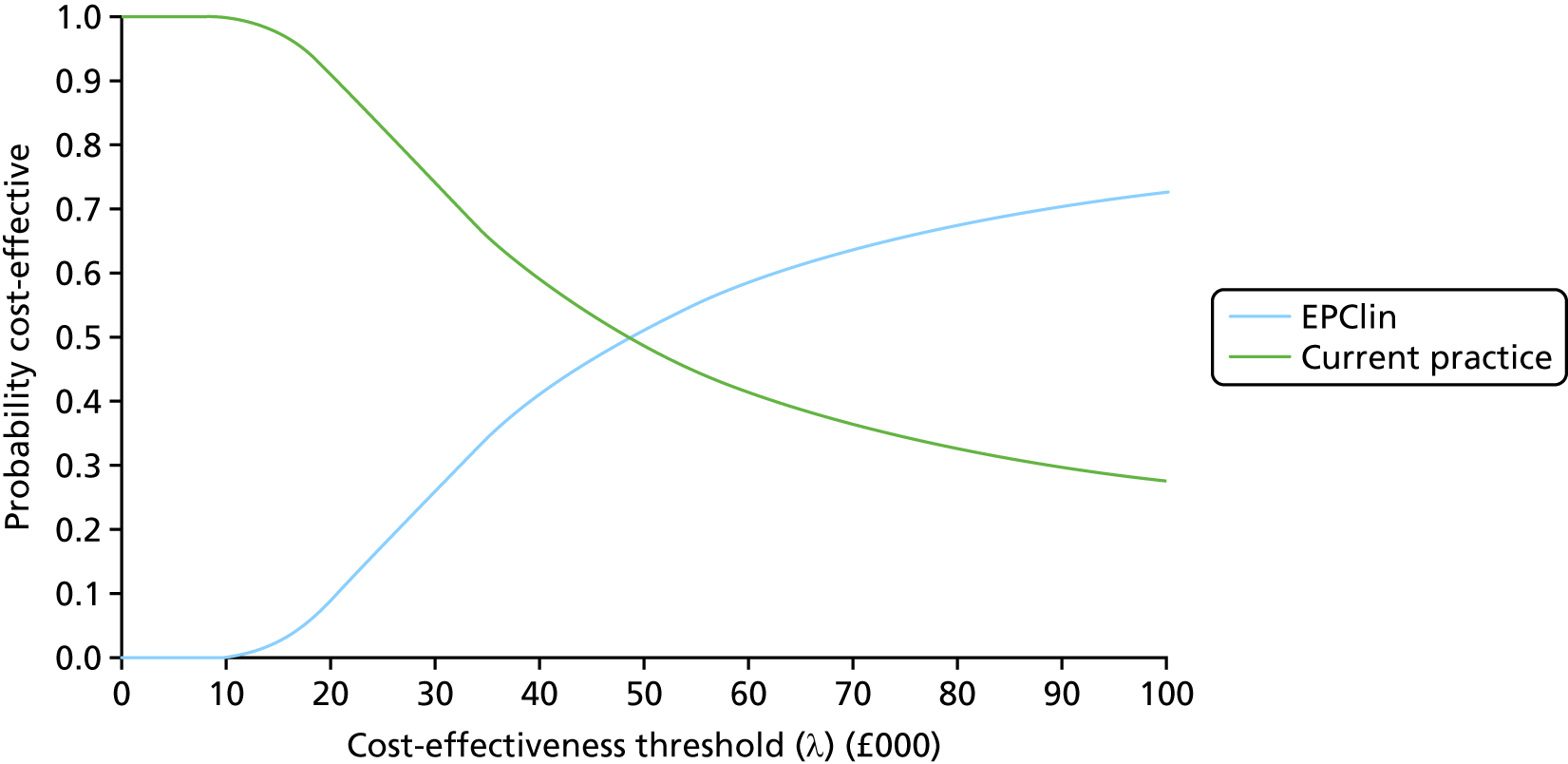
Node positive (one to three nodes)
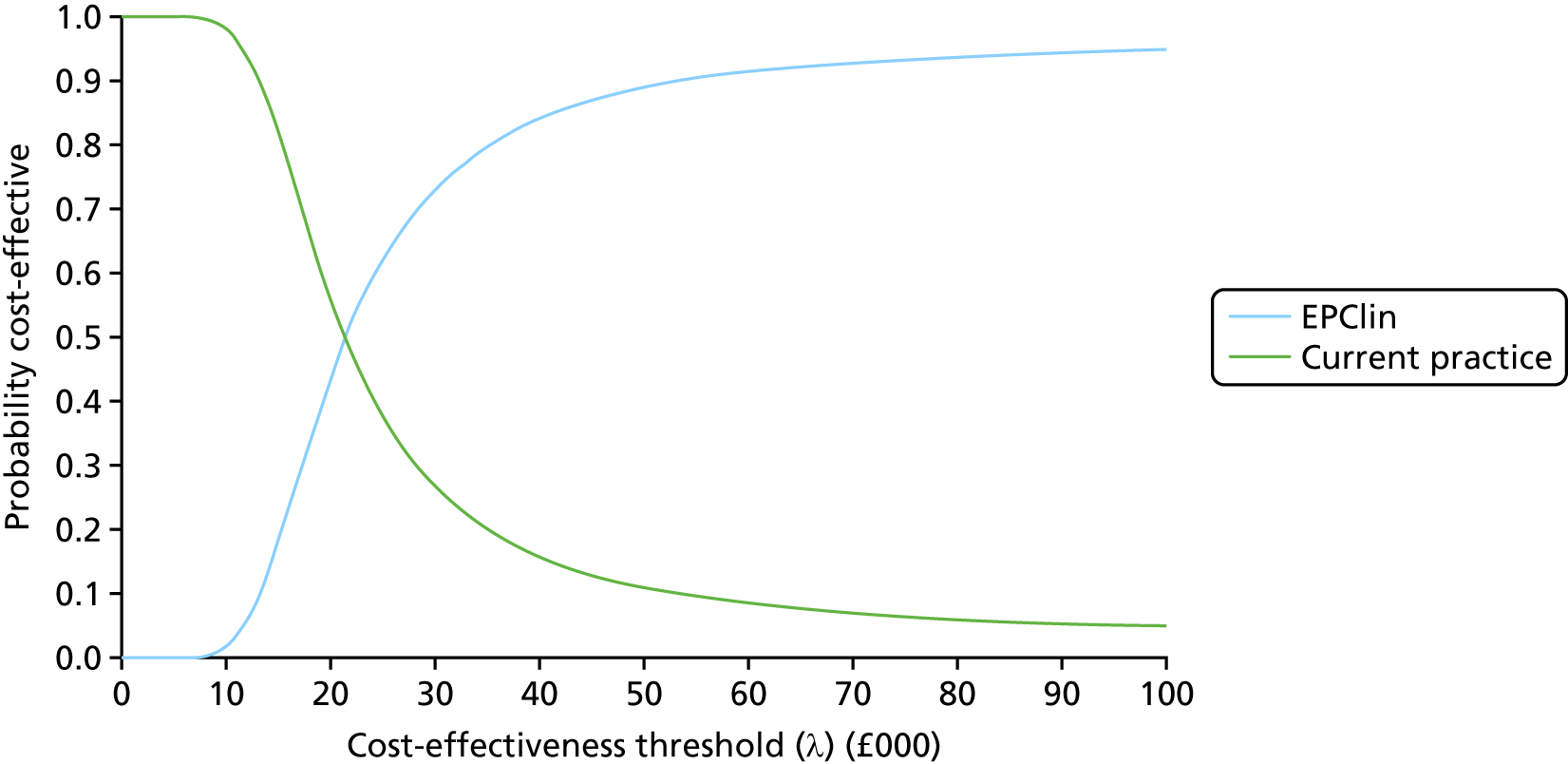
MammaPrint versus current practice
Overall MINDACT population
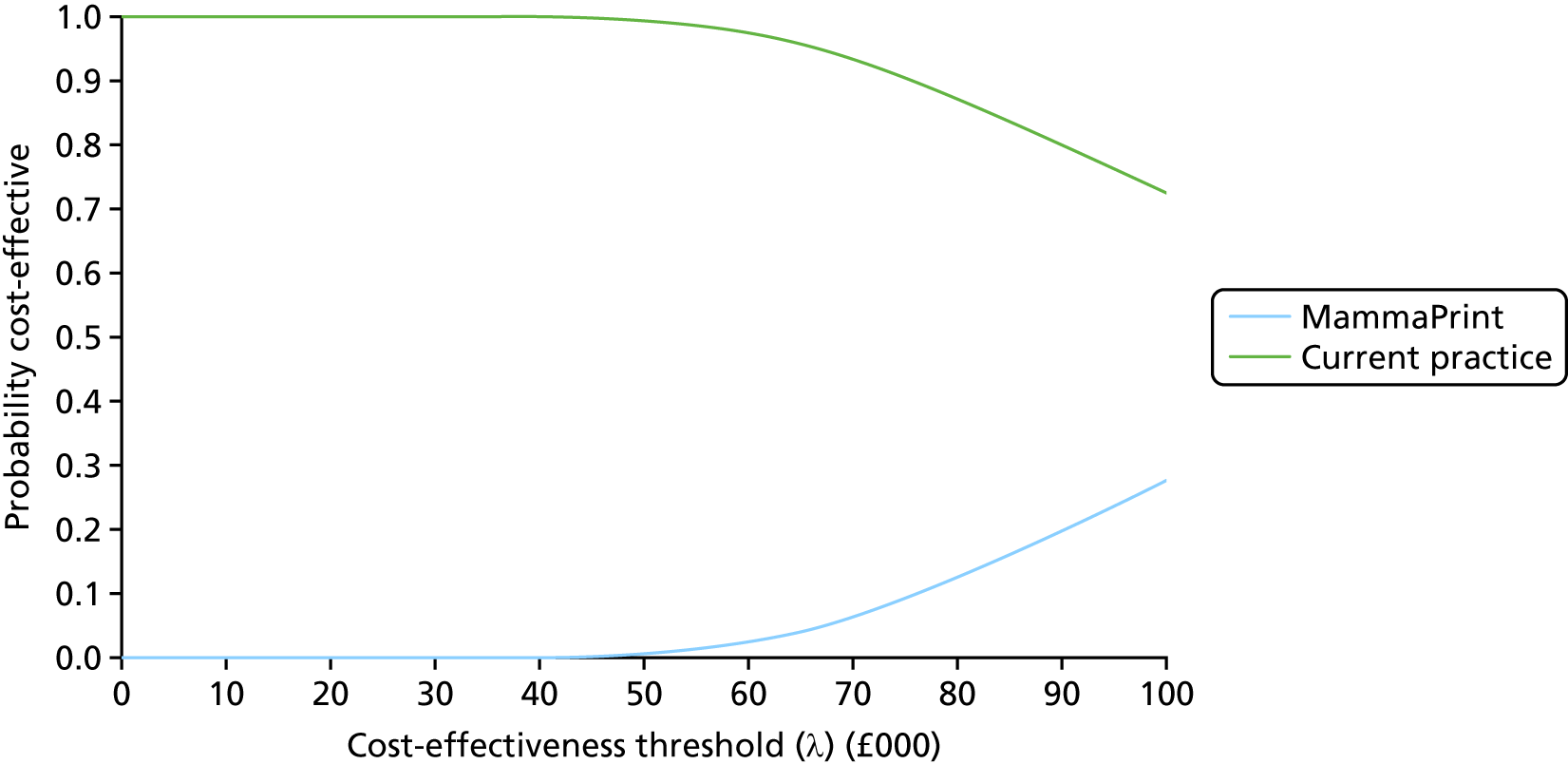
Modified Adjuvant! Online high-risk subgroup
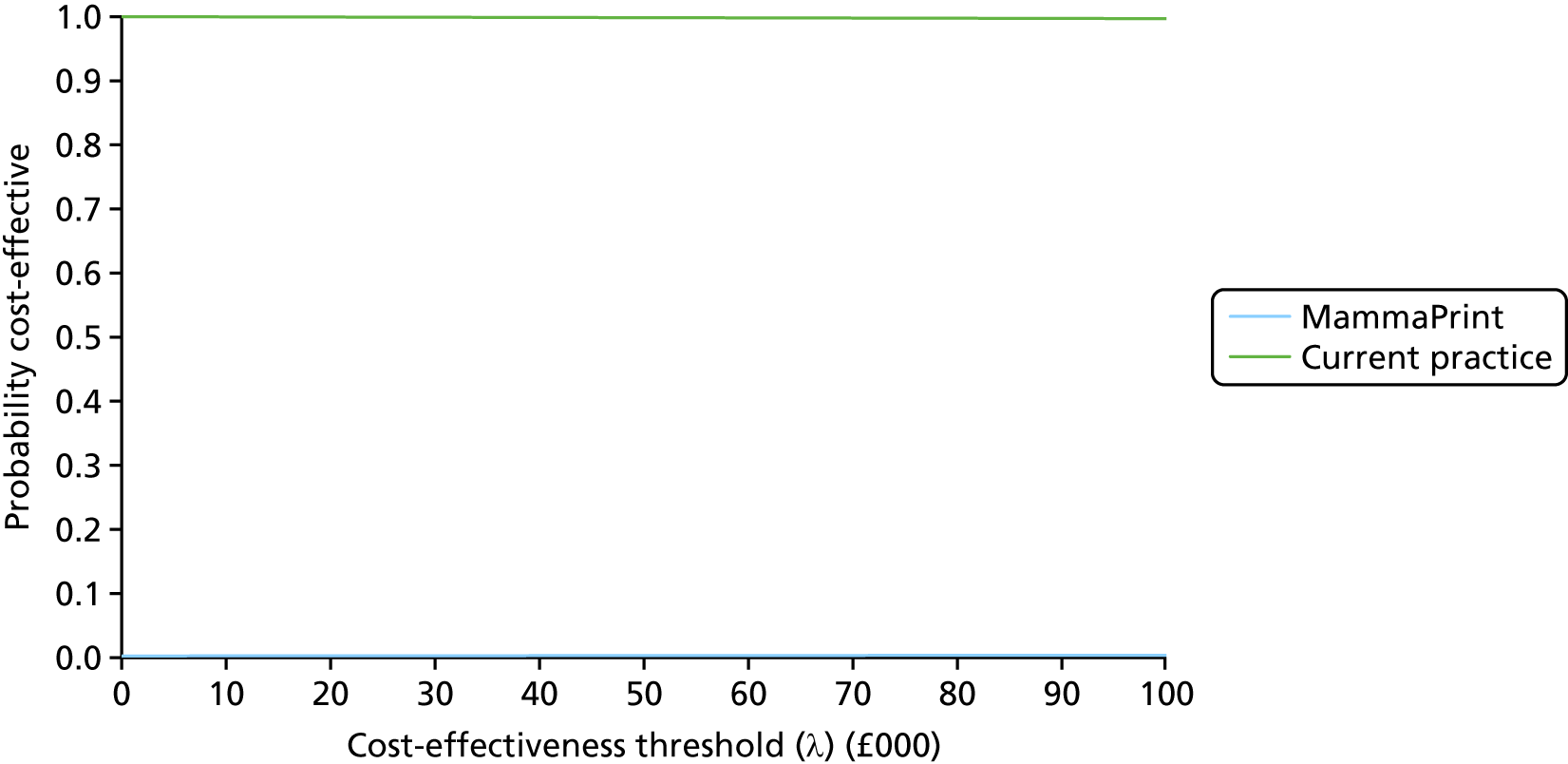
Modified Adjuvant! Online low-risk subgroup
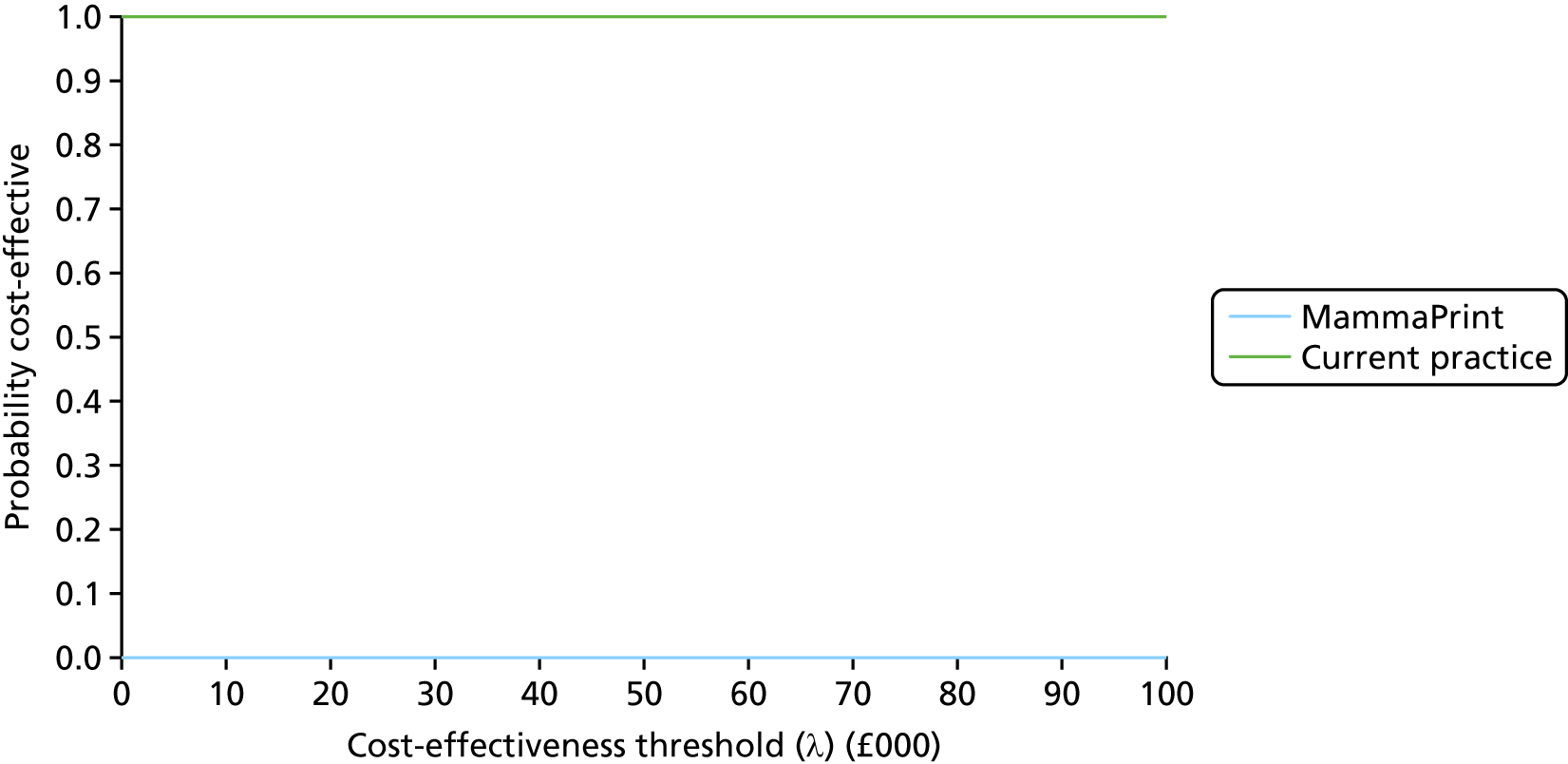
Appendix 12 Additional economic analyses undertaken after submission of the original External Assessment Group report
This appendix contains additional economic analyses undertaken after submission of the original EAG report.
Cost-effectiveness of chemotherapy by risk subgroup
During the consultation on the EAG report and the Diagnostic Consultation Document, it was suggested that the EAG model is predisposed to find giving chemotherapy to all patients a clinically effective and cost-effective use of resources. This interpretation of the model is inaccurate. In the interests of clarity, Table 95 presents the results of an analysis comparing 100% chemotherapy versus 0% chemotherapy using the EAG model. As shown in the table, the strategy involving the indiscriminate use of chemotherapy is dominated by the no-chemotherapy option for patients with a NPI of ≤ 3.4 (i.e. chemotherapy generates fewer QALYs at a greater cost). Chemotherapy appears to have a favourable clinical effectiveness and cost-effectiveness profile within the LN0, NPI > 3.4 and LN+ subgroups.
Subgroup | Option | QALYs | Costs | Incremental QALYs | Incremental costs | ICER |
---|---|---|---|---|---|---|
LN0, NPI ≤ 3.4 | 100% chemotherapy | 13.83 | £7454 | –0.04 | £3670 | Dominated |
No chemotherapy | 13.87 | £3784 | – | – | – | |
LN0, NPI > 3.4 | 100% chemotherapy | 12.85 | £11,700 | 0.27 | £2316 | £8449 |
No chemotherapy | 12.58 | £9384 | – | – | – | |
LN+ | 100% chemotherapy | 12.63 | £12,668 | 0.35 | £2011 | £5787 |
No chemotherapy | 12.28 | £10,658 | – | – | – |
Quality-adjusted life-year shortfall analysis to account for missing adverse events
In the light of the uncertainties associated with the analysis presented in Chapter 3, Independent economic evaluation, the EAG undertook a further analysis that presents the QALY shortfall associated with each test achieving an ICER of £20,000 and £30,000 per QALY gained, based on the deterministic version of the EAG model (Tables 96–101). Other things being equal, this additional analysis may further inform the appraisal committee’s deliberations around whether or not other factors that cannot be reliably quantified might have a sufficient impact on the ICERs of the tumour profiling tests to change the interpretation of the model results.
Oncotype DX (prognostic) | Subgroup | ||
---|---|---|---|
LN0, NPI < 3.4 | LN0, NPI > 3.4 | LN1–3 | |
Incremental QALYs | 0.01 | –0.02 | –0.07 |
Incremental costs | £1317 | £869 | £647 |
ICER | £120,144 | Dominated | Dominated |
QALY shortfall to achieve ICER of £20,000/QALY gained | 0.05 | 0.06 | 0.10 |
QALY shortfall to achieve ICER of £30,000/QALY gained | 0.03 | 0.04 | 0.09 |
Proportion of patients avoiding chemotherapy owing to testing | 0.00 | 0.16 | 0.29 |
Proportion of patients with unaccounted AEs (assumption based on consultation responses) | 0.25 | 0.25 | 0.25 |
Proportion of patients tested avoiding chemotherapy with unaccounted AEs | N/A – more get chemotherapy in test group | 0.04 | 0.07 |
QALY loss for patients avoiding chemotherapy with unaccounted AEs required to achieve shortfall at λ = £20,000/QALY | N/A – more get chemotherapy in test group | 1.49 | 1.44 |
QALY loss for patients avoiding chemotherapy with unaccounted AEs required to achieve shortfall at λ = £30,000/QALY | N/A – more get chemotherapy in test group | 1.12 | 1.29 |
Oncotype DX (predictive) | Subgroup | ||
---|---|---|---|
LN0, NPI < 3.4 | LN0, NPI > 3.4 | LN1–3 | |
Incremental QALYs | 0.04 | 0.27 | 0.09 |
Incremental costs | £1211 | –£364 | –£68 |
ICER | £34,245 | Dominating | Dominating |
QALY shortfall to achieve ICER of £20,000/QALY gained | 0.03 | N/A – ICER already below threshold | N/A – ICER already below threshold |
QALY shortfall to achieve ICER of £30,000/QALY gained | 0.01 | N/A – ICER already below threshold | N/A – ICER already below threshold |
Proportion of patients avoiding chemotherapy owing to testing | 0.00 | 0.16 | 0.29 |
Proportion of patients with unaccounted AEs (assumption based on consultation responses) | 0.25 | 0.25 | 0.25 |
Proportion of patients tested avoiding chemotherapy with unaccounted AEs | N/A – more get chemotherapy in test group | 0.04 | 0.07 |
QALY loss for patients avoiding chemotherapy with unaccounted AEs required to achieve shortfall at λ = £20,000/QALY | N/A – more get chemotherapy in test group | N/A – ICER already below threshold | N/A – ICER already below threshold |
QALY loss for patients avoiding chemotherapy with unaccounted AEs required to achieve shortfall at λ = £30,000/QALY | N/A – more get chemotherapy in test group | N/A – ICER already below threshold | N/A – ICER already below threshold |
IHC4+C | Subgroup | ||
---|---|---|---|
LN0, NPI < 3.4 | LN0, NPI > 3.4 | LN1–3 | |
Incremental QALYs | 0.01 | 0.01 | 0.05 |
Incremental costs | £22 | –£89 | –£269 |
ICER | £2752 | Dominating | Dominating |
QALY shortfall to achieve ICER of £20,000/QALY gained | N/A – ICER already below threshold | N/A – ICER already below threshold | N/A – ICER already below threshold |
QALY shortfall to achieve ICER of £30,000/QALY gained | N/A – ICER already below threshold | N/A – ICER already below threshold | N/A – ICER already below threshold |
Proportion of patients avoiding chemotherapy owing to testing | 0.04 | 0.08 | 0.07 |
Proportion of patients with unaccounted AEs (assumption based on consultation responses) | 0.25 | 0.25 | 0.25 |
Proportion of patients tested avoiding chemotherapy with unaccounted AEs | 0.01 | 0.02 | 0.02 |
QALY loss for patients avoiding chemotherapy with unaccounted AEs required to achieve shortfall at λ = £20,000/QALY | N/A – ICER already below threshold | N/A – ICER already below threshold | N/A – ICER already below threshold |
QALY loss for patients avoiding chemotherapy with unaccounted AEs required to achieve shortfall at λ = £30,000/QALY | N/A – ICER already below threshold | N/A – ICER already below threshold | N/A – ICER already below threshold |
Prosigna | Subgroup | ||
---|---|---|---|
LN0, NPI < 3.4 | LN0, NPI > 3.4 | LN1–3 | |
Incremental QALYs | 0.02 | 0.07 | 0.07 |
Incremental costs | £1891 | £1713 | £1967 |
ICER | £89,693 | £25,857 | £28,666 |
QALY shortfall to achieve ICER of £20,000/QALY gained | 0.07 | 0.02 | 0.03 |
QALY shortfall to achieve ICER of £30,000/QALY gained | 0.04 | N/A – ICER already below threshold | N/A – ICER already below threshold |
Proportion of patients avoiding chemotherapy owing to testing | 0.00 | –0.01 | –0.08 |
Proportion of patients with unaccounted AEs (assumption based on consultation responses) | 0.25 | 0.25 | 0.25 |
Proportion of patients tested avoiding chemotherapy with unaccounted AEs | N/A – more get chemotherapy in test group | N/A – more get chemotherapy in test group | N/A – more get chemotherapy in test group |
QALY loss for patients avoiding chemotherapy with unaccounted AEs required to achieve shortfall at λ = £20,000/QALY | N/A – more get chemotherapy in test group | N/A – more get chemotherapy in test group | N/A – more get chemotherapy in test group |
QALY loss for patients avoiding chemotherapy with unaccounted AEs required to achieve shortfall at λ = £30,000/QALY | N/A – more get chemotherapy in test group | N/A – more get chemotherapy in test group | N/A – more get chemotherapy in test group |
EPClin | Subgroup | ||
---|---|---|---|
LN0, NPI < 3.4 | LN0, NPI > 3.4 | LN1–3 | |
Incremental QALYs | 0.01 | 0.03 | 0.06 |
Incremental costs | £1686 | £1401 | £1185 |
ICER | £141,848 | £46,482 | £21,489 |
QALY shortfall to achieve ICER of £20,000/QALY gained | 0.07 | 0.04 | 0.00 |
QALY shortfall to achieve ICER of £30,000/QALY gained | 0.04 | 0.02 | N/A – ICER already below threshold |
Proportion of patients avoiding chemotherapy owing to testing | –0.07 | –0.01 | 0.02 |
Proportion of patients with unaccounted AEs (assumption based on consultation responses) | 0.25 | 0.25 | 0.25 |
Proportion of patients tested avoiding chemotherapy with unaccounted AEs | N/A – more get chemotherapy in test group | N/A – more get chemotherapy in test group | 0.01 |
QALY loss for patients avoiding chemotherapy with unaccounted AEs required to achieve shortfall at λ = £20,000/QALY | N/A – more get chemotherapy in test group | N/A – more get chemotherapy in test group | 0.69 |
QALY loss for patients avoiding chemotherapy with unaccounted AEs required to achieve shortfall at λ = £30,000/QALY | N/A – more get chemotherapy in test group | N/A – more get chemotherapy in test group | N/A – ICER already below threshold |
MammaPrint | Subgroup | ||
---|---|---|---|
MINDACT ITT | MINDACT high risk | MINDACT low risk | |
Incremental QALYs | 0.01 | –0.04 | 0.01 |
Incremental costs | £1757 | £1380 | £2415 |
ICER | £134,059 | Dominated | £399,182 |
QALY shortfall to achieve ICER of £20,000/QALY gained | 0.07 | 0.11 | 0.11 |
QALY shortfall to achieve ICER of £30,000/QALY gained | 0.05 | 0.09 | 0.07 |
Proportion of patients avoiding chemotherapy owing to testing | 0.15 | 0.33 | –0.03 |
Proportion of patients with unaccounted AEs (assumption based on consultation responses) | 0.25 | 0.25 | 0.25 |
Proportion of patients tested avoiding chemotherapy with unaccounted AEs | 0.04 | 0.08 | N/A – more get chemotherapy in test group |
QALY loss for patients avoiding chemotherapy with unaccounted AEs required to achieve shortfall at λ = £20,000/QALY | 2.03 | 1.39 | N/A – more get chemotherapy in test group |
QALY loss for patients avoiding chemotherapy with unaccounted AEs required to achieve shortfall at λ = £30,000/QALY | 1.23 | 1.11 | N/A – more get chemotherapy in test group |
Within each analysis, the QALY shortfall represents the additional number of incremental QALYs that would need to be accrued, given the currently quantified estimates of the incremental QALYs gained for the test and its incremental cost, in order for each test to achieve an ICER at a particular threshold (λ = £20,000 per QALY gained or λ = £30,000 per QALY gained). In health economic terms, this QALY shortfall is equivalent to net clinical benefit. The NICE Diagnostic Appraisal Committee may find it useful to consider whether the expected magnitude of the health losses avoided by reducing chemotherapy use via tumour profiling tests that are not captured in the EAG model is likely to be equal to or greater than this estimated QALY shortfall. It should be noted that this analysis is predicated on the commentators’ assumption that the adverse effects of chemotherapy have been underestimated in the EAG’s model. However, the EAG model suggests that with the exception of IHC4+C, all tests increase chemotherapy use in at least some subgroups (see Appendix 10); where this is the case, changing the balance of the net health gains and losses of chemotherapy will produce less favourable ICERs for the tumour profiling tests. It should also be noted that any potential underestimation of QALY losses only apply to those patients who would have received chemotherapy and who would have experienced associated late effects who now do not receive chemotherapy owing to the tumour profiling test result and thus avoid these late effects.
The QALY shortfall analysis operates as follows. As shown in Table 96, within the LN0 NPI > 3.4 group, oncotype DX (assuming prognostic benefit only) is estimated to lead to –0.02 QALYs and additional costs of £869 compared with no testing, hence it is expected to be dominated by no testing. In this subgroup, oncotype DX would need to make up a further 0.06 QALYs in order to achieve an ICER of £20,000 per QALY gained given its incremental cost [£869/(0.06 + –0.02) = £20,000]. Within this subgroup, the EAG model suggests that the probability of receiving chemotherapy is reduced by 16% owing to the use of oncotype DX. Assuming that 25% of these patients experience late effects of chemotherapy that are not accounted for within the EAG model, this means that 4% (0.16 × 0.25) of those forgoing chemotherapy will avoid late effects. The overall QALY shortfall of 0.06 QALYs and the probability of avoiding late effects of 0.04 means that each patient who would have experienced a late effect of chemotherapy would have had to have lost 1.49 QALYs (0.06/0.04) due to that AE in order for oncotype DX to be cost-effective at a threshold of £20,000 per QALY gained.
The results for this analysis are summarised in the following sections.
Oncotype DX (prognostic benefit assumed) (see Table 96)
-
For the LN0, NPI ≤ 3.4 subgroup, the analysis is not relevant as more patients receive chemotherapy in the test group.
-
For the LN0, NPI > 3.4 subgroup, each patient who avoids chemotherapy and avoids experiencing a late AE not quantified in the EAG model would have to save 1.49 QALYs due to the unquantified AE in order for oncotype DX to have an ICER of £20,000 per QALY gained. Assuming a threshold of £30,000 per QALY gained, the equivalent value is 1.12 QALYs per patient.
-
In the LN1–3 subgroup, each patient who avoids chemotherapy and avoids experiencing a late AE not quantified in the EAG model would have to save 1.44 QALYs due to the unquantified AE in order for oncotype DX to have an ICER of £20,000 per QALY gained. Assuming a threshold of £30,000 per QALY gained, the equivalent value is 1.29 QALYs per patient.
Oncotype DX (predictive benefit assumed) (see Table 97)
-
In the LN0, NPI ≤ 3.4 subgroup, the analysis is not relevant as more patients receive chemotherapy in the test group.
-
In the LN0, NPI > 3.4 subgroup, the analysis is not relevant as the test dominates.
-
In the LN1–3 subgroup, the analysis is not relevant as the test dominates.
IHC4+C (see Table 98)
-
In the LN0, NPI ≤ 3.4 subgroup, the analysis is not relevant as the ICER is already below £20,000 per QALY gained.
-
In the LN0, NPI > 3.4 subgroup, the analysis is not relevant as the test dominates.
-
In the LN1–3 subgroup, the analysis is not relevant as the test dominates.
Prosigna (see Table 99)
-
In the LN0, NPI ≤ 3.4 subgroup, the analysis is not relevant as the test increases chemotherapy use.
-
In the LN0, NPI > 3.4 subgroup, the analysis is not relevant as the test increases chemotherapy use.
-
In the LN1–3 subgroup, the analysis is not relevant as the test increases chemotherapy use.
EndoPredict Clinical (see Table 100)
-
In the LN0, NPI ≤ 3.4 subgroup, the analysis is not relevant as the test increases chemotherapy use.
-
In the LN0, NPI > 3.4 subgroup, the analysis is not relevant as the test increases chemotherapy use.
-
In the LN1–3 subgroup, the analysis is not relevant at a threshold of £30,000 per QALY gained as the ICER is below this. Each patient who avoids chemotherapy and avoids experiencing a late AE not quantified in the EAG model would have to save 0.69 QALYs due to the unquantified AE in order for EPClin to have an ICER of £20,000 per QALY gained.
MammaPrint (see Table 101)
-
In the MINDACT ITT subgroup, each patient who avoids chemotherapy and avoids experiencing a late AE not quantified in the EAG model would have to save 2.03 QALYs due to the unquantified AE in order for MammaPrint DX to have an ICER of £20,000 per QALY gained. Assuming a threshold of £30,000 per QALY gained, the equivalent value is 1.23 QALYs per patient.
-
In the MINDACT high-risk subgroup, each patient who avoids chemotherapy and avoids experiencing a late AE not quantified in the EAG model would have to save 1.39 QALYs due to the unquantified AE in order for MammaPrint to have an ICER of £20,000 per QALY gained. Assuming a threshold of £30,000 per QALY gained, the equivalent value is 1.11 QALYs per patient.
-
In the MINDACT low-risk subgroup, the analysis is not relevant as the test increases chemotherapy use.
Additional analyses of oncotype DX versus usual practice including chemotherapy benefit based on naive indirect comparisons of Study B20, Study B14 and TransATAC: lymph node-negative, Nottingham Prognostic Index > 3.4 subgroup
Table 102 presents estimated HRs for chemotherapy versus no chemotherapy based on naive indirect comparisons of Study B20,50 Study B1449 and TransATAC. 46
Oncotype DX risk group | 10-year DMFS | Estimated HR | |
---|---|---|---|
No chemotherapy (%) | Chemotherapy (%) | ||
B20 vs. B14 | |||
Low | 93.20 | 95.60 | 0.64 |
Intermediate | 85.70 | 89.10 | 0.75 |
High | 69.50 | 88.10 | 0.35 |
B20 vs. TransATAC | |||
Low | 94.90 | 95.60 | 0.86 |
Intermediate | 87.70 | 89.10 | 0.88 |
High | 77.20 | 88.10 | 0.49 |
Table 103 presents additional economic comparisons of oncotype DX versus usual practice including chemotherapy benefit based on naive indirect comparisons of Study B20,50 B1449 and TransATAC. 46 In each analysis, the modelled HR was calibrated against the estimates presented in Table 102. All analyses are based on the deterministic version of the EAG model.
Option | QALYs | Costs | Incremental QALYs | Incremental costs | ICER (per QALY gained) |
---|---|---|---|---|---|
Chemotherapy benefit based on indirect comparison of B20 and B14 | |||||
Oncotype DX | 12.82 | £10,664 | 0.03 | £682 | £24,334 |
No test | 12.79 | £9981 | – | – | – |
Chemotherapy benefit based on indirect comparison of B20 and TransATAC | |||||
Oncotype DX | 12.74 | £10,989 | 0.06 | £525 | £8150 |
No test | 12.68 | £10,465 | – | – | – |
List of abbreviations
- ABCSG
- Austrian Breast and Colorectal Cancer Study Group
- AE
- adverse event
- AiC
- academic in confidence
- AML
- acute myeloid leukaemia
- AOL
- Adjuvant! Online
- ASCO
- American Society of Clinical Oncology
- ATAC
- Arimidex, Tamoxifen, Alone or in Combination
- AUC
- area under the curve
- BCS
- Edinburgh Breast Conservation Series
- BCSS
- breast-cancer-specific survival
- BNF
- British National Formulary
- CALGB
- Cancer and Leukemia Group B
- cDNA
- complementary deoxyribonucleic acid
- CE
- Conformité Européenne
- CEAC
- cost-effectiveness acceptability curve
- CHF
- congestive heart failure
- CI
- confidence interval
- CLP
- clinical linear predictor
- CRD
- Centre for Reviews and Dissemination
- CTS
- clinical treatment score
- DBCG
- Danish Breast Cancer Cooperative Group
- DCIS
- ductal carcinoma in situ
- DFS
- disease-free survival
- DG
- diagnostics guidance
- DMFI
- distant metastasis-free interval
- DMFS
- distant metastasis-free survival
- DNA
- deoxyribonucleic acid
- DRFI
- distant recurrence/relapse-free interval
- DRFS
- distant recurrence/relapse-free survival
- DSA
- deterministic sensitivity analysis
- EAG
- External Assessment Group
- EBCTCG
- Early Breast Cancer Trialists’ Collaborative Group
- EPClin
- EndoPredict Clinical
- EQ-5D
- EuroQol 5-Dimensions
- ER
- oestrogen receptor
- ER–
- oestrogen receptor negative
- ER+
- oestrogen receptor positive
- FACT-B
- Functional Assessment of Cancer Therapy – Breast cancer
- FACT-G
- Functional Assessment of Cancer Therapy – General
- FFPE
- formalin fixed, paraffin embedded
- G-CSF
- granulocyte colony-stimulating factor
- GEICAM
- Grupo Español de Investigación en Cáncer de Mama
- GEO
- gene expression omnibus
- GEP
- gene expression profiling
- HCHS
- Hospital and Community Health Service
- HER2
- human epidermal growth factor receptor 2
- HER2–
- human epidermal growth factor receptor 2 negative
- HER2+
- human epidermal growth factor receptor 2 positive
- HR
- hazard ratio
- HR+
- hormone receptor positive
- HRQoL
- health-related quality of life
- HTA
- Health Technology Assessment
- ICER
- incremental cost-effectiveness ratio
- IDFS
- invasive disease-free survival
- IES
- Intergroup Exemestane Study
- IHC
- immunohistochemistry
- IHC4
- immunohistochemistry 4
- IHC4+C
- immunohistochemistry 4 plus clinical factors
- IPD
- individual patient data
- ITT
- intention to treat
- LN+
- lymph node positive
- LN0
- lymph node negative
- LN0–3
- zero to three positive lymph nodes
- LN1–3
- one to three positive lymph nodes
- LN1micro
- one lymph node micrometastasis
- LNmicro
- lymph node micrometastases
- LYG
- life-year gained
- mAOL
- Modified Adjuvant! Online
- MDS
- myelodysplastic syndromes
- MeSH
- medical subject heading
- MINDACT
- Microarray In Node-negative Disease may Avoid ChemoTherapy
- mRNA
- messenger ribonucleic acid
- NCBI
- National Centre for Biotechnology Information
- NCCN
- National Comprehensive Cancer Network
- NCIC
- National Cancer Institute of Canada
- NCRAS
- National Cancer Registration and Analysis Service
- NHS EED
- NHS Economic Evaluation Database
- NICE
- National Institute for Health and Care Excellence
- NPI
- Nottingham Prognostic Index
- NSABP
- National Surgical Adjuvant Breast and Bowel Project
- OPTIMA
- Optimal Personalised Treatment of early breast cancer usIng Multi-parameter Analysis
- OPTIMA Prelim
- Optimal Personalised Treatment of early breast cancer usIng Multi-parameter Analysis preliminary
- OR
- odds ratio
- OS
- overall survival
- PAI-1
- plasminogen activator inhibitor 1
- PAM50
- Prediction Analysis of Microarray 50
- PAS
- Patient Access Scheme
- PR
- progesterone receptor
- PR+
- progesterone receptor positive
- PRISMA
- Preferred Reporting Items for Systematic Reviews and Meta-Analyses
- PROBAST
- Prediction model study Risk Of Bias ASsessment Tool
- PSA
- probabilistic sensitivity analysis
- PSS
- Personal Social Services
- pT
- pathological tumour stage
- QALY
- quality-adjusted life-year
- RASTER
- MicroarRAy-prognoSTics-in-breast-cancER
- RCT
- randomised controlled trial
- RFI
- recurrence/relapse-free interval
- RFS
- recurrence/relapse-free survival
- RNA
- ribonucleic acid
- ROR
- risk of recurrence
- ROR-C
- risk of recurrence based on Prediction Analysis of Microarray 50 subtype information plus tumour size
- ROR-P
- risk of recurrence based on Prediction Analysis of Microarray 50 subtype information plus proliferation score
- ROR-PT
- risk of recurrence based on Prediction Analysis of Microarray 50 subtype information plus proliferation score plus tumour size
- ROR-S
- risk of recurrence based on Prediction Analysis of Microarray 50 subtype information
- ROR-T
- risk of recurrence based on Prediction Analysis of Microarray 50 subtype information plus tumour size
- RR
- relative risk
- RSPC
- recurrence score–pathology–clinical
- RT-PCR
- reverse transcription-polymerase chain reaction
- RT-qPCR
- reverse transcription-quantitative polymerase chain reaction
- ScHARR
- School of Health and Related Research
- ScHARR-TAG
- School of Health and Related Research Technology Assessment Group
- SE
- standard error
- SEER
- Surveillance, Epidemiology, and End Results
- STAI
- State–Trait Anxiety Inventory
- STO-3
- Stockholm Tamoxifen-3
- SWOG
- Southwest Oncology Group
- TAILORx
- Trial Assigning Individualized Options for Treatment (Rx)
- TC
- docetaxel and cyclophosphamide
- TEAM
- Tamoxifen vs Exemestane Adjuvant Multinational
- TransATAC
- Translational substudy of the Arimidex, Tamoxifen, Alone or in Combination
- TRANSBIG
- Translating molecular knowledge into early breast cancer management: building on the BIG (Breast International Group) network for improved treatment tailoring
- UKBCG
- UK Breast Cancer Group
- uPA
- urokinase plasminogen activator
- WSG
- West German Study Group
- WSG-AGO-Doc
- West German Study Group epirubicine and cyclophosphamide-Doc
- WTP
- willingness to pay
This monograph is based on the Technology Assessment Report produced for NICE. The full report contained a considerable number of data that were deemed confidential. The full report was used by the Appraisal Committee at NICE in their deliberations. The full report with each piece of confidential data removed and replaced by the statement ‘confidential information (or data) removed’ is available on the NICE website: www.nice.org.uk.
The present monograph presents as full a version of the report as is possible while retaining readability, but some sections, sentences, tables and figures have been removed. Readers should bear in mind that the discussion, conclusions and implications for practice and research are based on all the data considered in the original full NICE report.