Notes
Article history
The research reported in this issue of the journal was funded by the HTA programme as project number 14/49/94. The contractual start date was in September 2015. The draft report began editorial review in May 2019 and was accepted for publication in October 2019. The authors have been wholly responsible for all data collection, analysis and interpretation, and for writing up their work. The HTA editors and publisher have tried to ensure the accuracy of the authors’ report and would like to thank the reviewers for their constructive comments on the draft document. However, they do not accept liability for damages or losses arising from material published in this report.
Declared competing interests of authors
Neil M Davies, Amy E Taylor, Taha Itani and Marcus R Munafò report a grant from the Global Research Awards for Nicotine Dependence, which is an Independent Competitive Grants Programme supported by Pfizer Inc. (New York, NY, USA), the maker of varenicline. Marcus R Munafò reports grants from Rusan Pharma Ltd (Mumbai, India), and non-financial support from GlaxoSmithKline (Brentford, UK) outside the submitted work. Richard M Martin was a member of the Independent Scientific Advisory Committee of the Medicines and Healthcare products Regulatory Agency, which approves applications for Clinical Practice Research Datalink studies.
Permissions
Copyright statement
© Queen’s Printer and Controller of HMSO 2020. This work was produced by Davies et al. under the terms of a commissioning contract issued by the Secretary of State for Health and Social Care. This issue may be freely reproduced for the purposes of private research and study and extracts (or indeed, the full report) may be included in professional journals provided that suitable acknowledgement is made and the reproduction is not associated with any form of advertising. Applications for commercial reproduction should be addressed to: NIHR Journals Library, National Institute for Health Research, Evaluation, Trials and Studies Coordinating Centre, Alpha House, University of Southampton Science Park, Southampton SO16 7NS, UK.
2020 Queen’s Printer and Controller of HMSO
Chapter 1 Introduction
Smoking is the major avoidable cause of preventable morbidity and mortality in the UK and internationally. 1,2 Smoking is also the principal cause of health inequalities and is responsible for most of the difference in healthy life expectancy between the richest and poorest in our society3 and those with and without mental health problems. 4 Studies have estimated that smoking-related illnesses cost the NHS approximately £5B per year. 5 In 1974, 45.6% of the population smoked; by 2016, this had fallen to 16.1% (Figure 1).
FIGURE 1.
Proportion of the adult population in Great Britain who smoke, by age. Estimates from the Annual Population Survey and published by the Office for National Statistics. 6
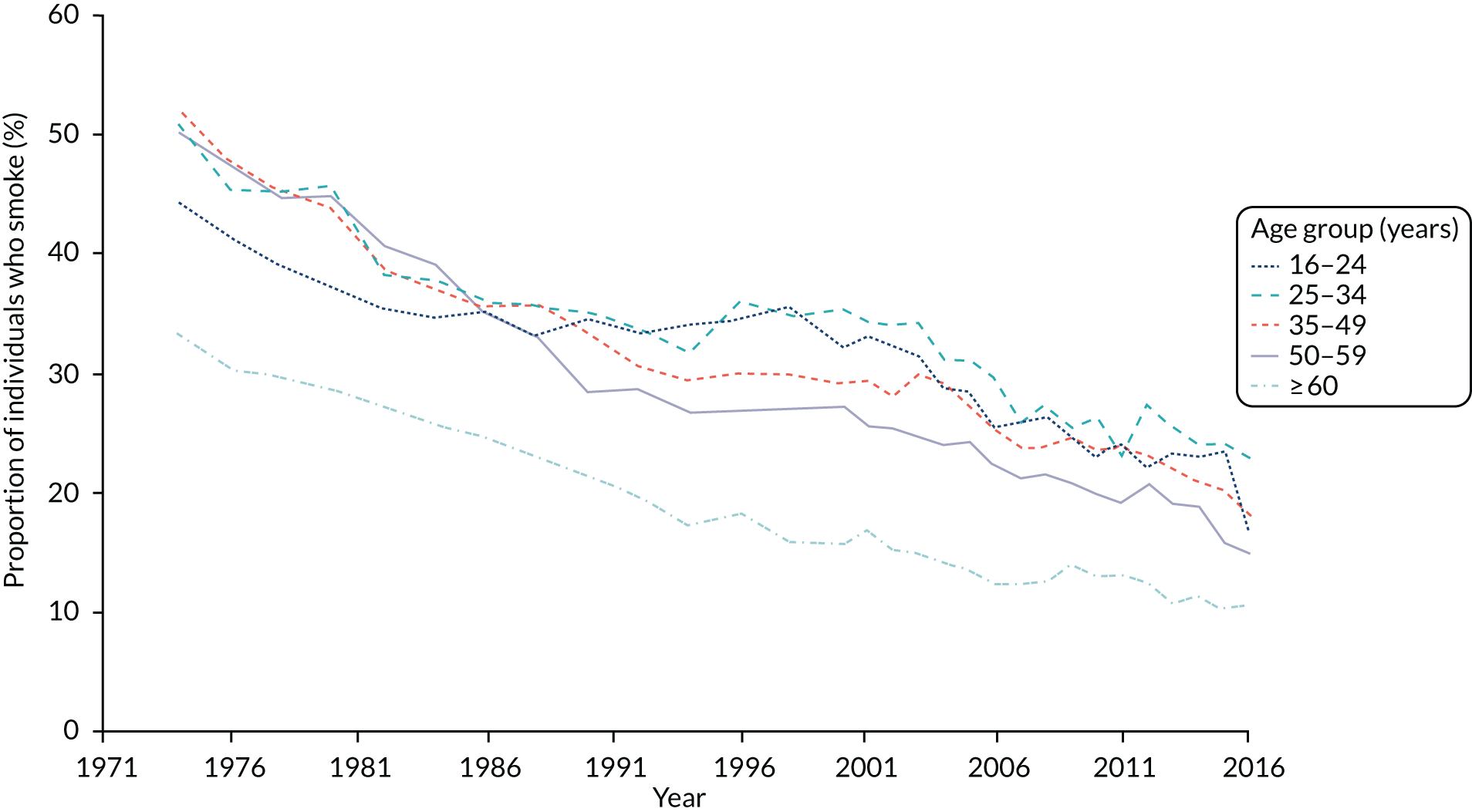
The main form of smoking cessation treatment is nicotine replacement therapy (NRT), such as nicotine patches and gums. Pharmaceutical treatments for nicotine addiction include bupropion and varenicline; however, bupropion is rarely used. 7 Varenicline, a nicotinic receptor partial agonist, has been available in the UK from 2006. Varenicline has been shown to be the most effective smoking cessation medicine for short-term abstinence in randomised controlled trials (RCTs). 7 However, there is relatively little evidence for its long-term effectiveness and impact on clinical outcomes that are relevant to health-care providers.
In addition, concerns have been raised that smokers taking varenicline have a higher risk of adverse events, including suicide, self-harm and cardiovascular events, than with other smoking cessation interventions. 8 This led the Food and Drug Administration (FDA) to issue black box warnings about the possible adverse effects of varenicline between July 2009 and December 2016. 9 There have been large randomised studies comparing the effectiveness and safety of varenicline with NRT, such as (EAGLES Evaluating Adverse Events in a Global Smoking Cessation Study). 10 However, much of the evidence about the potential adverse effects of varenicline comes from observational studies that are prone to residual confounding. Furthermore, the experimental evidence about the effects of prescribing varenicline on health outcomes has limited follow-up.
The rationale for this study
We addressed this gap in the literature by investigating the adverse and beneficial effects of prescribing varenicline and NRTs using three statistical approaches to overcome confounding: multivariable adjusted, propensity score matched and instrumental variable regression. We investigated the effects of varenicline on all-cause mortality and smoking abstinence, and on all-cause primary and secondary care use because smoking increases morbidity and imposes major costs on health-care services. 11 We examined the differences in smoking cessation medication effectiveness by socioeconomic position (SEP) because a recent systematic review reported that UK stop-smoking services might be helping to reduce inequalities in smoking prevalence by preferentially targeting smokers of lower SEP. 12
Chapter 2 Methods
Parts of this text have been reproduced from Davies et al. 13 Published by the BMJ Publishing Group Limited. For permission to use (where not already granted under a licence) please go to http://www.bmj.com/company/products-services/rights-and-licensing/. This is an Open Access article distributed in accordance with the terms of the Creative Commons Attribution (CC BY 4.0) license, which permits others to distribute, remix, adapt and build upon this work, for commercial use, provided the original work is properly cited. See: http://creativecommons.org/licenses/by/4.0/.
Research objectives
-
To investigate the long-term smoking abstinence of patients prescribed either varenicline or NRTs in the Clinical Practice Research Datalink (CPRD) and whether or not the effects differ by area-level deprivation.
-
To investigate the causal effects of prescribing smoking cessation therapies on:
-
all-cause mortality and death due to chronic lung disease, lung cancer, coronary heart disease, pneumonia, cerebrovascular disease, diabetes and external causes
-
all-cause and incident cause-specific hospitalisation (specifically chronic lung disease, lung cancer, coronary heart disease, pneumonia, cerebrovascular disease, diabetes and external causes)
-
incident primary care diagnosis of respiratory illness
-
incident primary care diagnosis of myocardial infarction
-
incident primary care prescription of a hypnotic or anxiolytic
-
the frequency of attendance at a general practice or a hospital
-
weight in kg
-
incident registration of (1) any malignant neoplasm and (2) lung malignancy.
-
Study design
This was a prospective longitudinal cohort study of electronic medical records of patients prescribed smoking cessation treatments in primary care. The CPRD’s Independent Scientific Advisory Committee approved this study (protocol number 15_107). The protocol has been published. 13
Data extraction
We extracted data from patients aged ≥ 18 years who were prescribed smoking cessation treatment (see British National Formulary, chapter 4.10.214) in ‘up to standard’ primary care centres contributing to the CPRD after 1 September 2006, when varenicline became available in the UK. ‘Up to standard’ practices are those that provided consistent data to the CPRD. Inclusion criteria included having an ‘acceptable’ record and having at least 1 year of registration at their practice before their first smoking cessation prescription to allow for high-quality assessment of confounders. The CPRD defines patients’ data as acceptable if they have valid information, including information regarding age and sex. 15 The CPRD linked data from the Office for National Statistics (ONS) mortality records and the Hospital Episode Statistics for around half of the practices in the study. We defined the health outcomes mainly from these linked data sets. For this reason, we restricted the evaluation of the health impact of smoking cessation treatments to linked practices. Figure 2 shows a flow chart of the inclusion and exclusion of patients for the effectiveness and morbidity and mortality aspects of the study.
Intervention
The intervention was the first prescription of either smoking cessation treatment in the eligible period. We compared the effect of prescribing varenicline with the effect of prescribing NRT. We focus on the first recorded treatment because subsequent treatment decisions are likely to be highly non-random. We extracted the date of the first prescription from the patients’ records.
Follow-up
Follow-up started on the date that each patient received their first prescription and ended when they had an event, died or left the practice.
Outcomes
We assessed all outcomes at 3, 6, 9, 12, 24 and 48 months after the first prescription. We took the date of diagnosis from the first record that indicated an outcome after the first prescription. We excluded patients who were prevalent cases at the time of their first prescription from these analyses.
Smoking cessation
We defined smoking cessation using GP records that indicated the patients’ smoking status. Each patient had multiple records that stated their smoking status, which can be indicated as current, former or never smoker. GPs were incentivised to record their patients’ smoking status as part of the Quality and Outcomes Framework. 18 We defined smoking cessation as the status indicated by the most recent record within each follow-up period. If patients had no record that indicated their smoking status but were prescribed smoking cessation medication, we assumed that they had relapsed. We conducted sensitivity analyses to investigate whether or not our results were affected when we assumed that all unobserved smokers relapsed.
Mortality
We defined mortality using the linked ONS mortality data. This data set gave the date and primary causes of death. We investigated the following secondary outcomes: mortality due to chronic lung disease [International Statistical Classification of Diseases and Related Health Problems, Tenth Revision19 (ICD-10) codes = J40–44], lung cancer (ICD-10 code = C34), coronary heart disease (ICD-10 codes = I21–25), pneumonia (ICD-10 codes = J12–18), cerebrovascular disease (ICD-10 codes = I60–69), diabetes (ICD-10 codes = E10–14) and external causes (ICD-10 codes = V01–Y98).
Hospitalisation
We identified episodes of hospitalisation using the linked Hospital Episode Statistics data set, which provides the date and cause of hospitalisation for all linked patients. We investigated both all-cause hospitalisation and cause-specific hospitalisation using the codes described above for mortality.
Primary care diagnosis and attendance
Primary care diagnoses of myocardial infarction, chronic obstructive pulmonary disease (COPD) and depression, and prescriptions of hypnotics and anxiolytics were identified using validated code lists. The frequency that each patient attended primary care was identified from their records.
Weight
The patients’ weight in the months following their initial prescription was identified using their electronic medical records.
Potential confounders
The potential confounders included the following variables measured at baseline: age; body mass index (BMI); alcohol drug misuse, defined using Read codes; SEP measured by the Index of Multiple Deprivation (IMD); an indicator for having more than five primary care consultations in the year before first prescription; indicators for year of prescription; any previous prescriptions of hypnotics; any previous prescriptions of antipsychotics; any previous prescriptions of antidepressants; diagnoses of self-harm; diagnoses of myocardial infarction; diagnoses of COPD; any psychiatric disease; or any serious comorbidity (a non-zero Charlson Comorbidity Index). We accounted for missing data by using imputation by chained equations, because some patients were missing BMI and SEP data.
Statistical methods
We estimated the effects of varenicline and NRT using three statistical methods: multivariable-adjusted logistic regression, propensity score matched regression and instrumental variable regression. We did not use Cox survival models because the proportional hazards assumption did not hold. Our basic adjusted models adjust for sex, year of first prescription and age. Our fully adjusted results also adjust for all confounders described above. We used propensity score and the covariates described above to match patients who were treated with varenicline to other similar patients prescribed NRT using one-to-one matching with no calliper. There was a complete overlap in the propensity scores between smokers who were prescribed varenicline and who were prescribed NRT. The propensity score depends on similar assumptions to multivariable-adjusted regression (conditional exchangeability). Both approaches could suffer from residual confounding. We addressed residual confounding using instrumental variable analysis.
Instrumental variable analysis requires a variable, the ‘instrument’ that is defined by the following three assumptions: (1) the instrument associates with the exposure of interest, (2) the instrument does not associate with unobserved confounding factors and (3) the instrument affects the outcome only via the exposure. 20 A widely used instrument in pharmacoepidemiology is physicians’ prescribing preferences for different medications. 21 We cannot directly measure GPs’ preferences, but we can use their prescriptions to previous patients as a proxy for their preferences. We investigated whether or not the instrumental variable assumptions held by (1) testing the association of their prior prescriptions to their previous seven patients with their subsequent prescriptions and (2) investigating the association with potential confounders. We estimated the effects of varenicline and NRT using additive structural models for binary outcomes and two-stage least squares for continuous outcomes.
All standard errors account for the clustering of patients at the practice level. We published all analytic scripts used to generate the data to GitHub. 22,23
Changes to the protocol
Survival analysis
Our original protocol proposed to use survival analysis to estimate hazard ratios of events. However, there was evidence that the proportional hazards assumption was violated; therefore, we dichotomised the outcomes instead.
Definition of mortality and hospitalisation outcomes
Peer reviewers of Davies et al. 16 noted that our original definition of our cause-specific outcomes was relatively general (diseases of the respiratory system, ICD-10 = J00–99 and cardiovascular disease, ICD-10 = I00–52) and recommended reporting more specific causes of death. As a result, we amended our protocol to include the following eight outcomes defined by ICD-10 code: (1) chronic lung disease (ICD-10 = J40–44), (2) lung cancer (ICD-10 = C34), (3) coronary heart disease (ICD-10 = I21–25), (4) pneumonia (ICD-10 = J12–18), (5) cerebrovascular disease (ICD-10 = I60–69), (6) diabetes (ICD-10 E10–14) and (7) external causes (ICD-10 V01–Y98). We used the same set of codes to define cause-specific mortality and hospital admission.
Cancer registry outcomes
Our protocol originally intended to use data from the National Cancer Registry. However, unfortunately, the CPRD was not able to provide access to this data set. To overcome this, we revised our protocol to use data from primary and secondary care to identify cancer cases. These alternative sources of information are reliable indicators of cancer diagnosis date and type. 24
Chapter 3 The effectiveness of varenicline for smoking cessation
Parts of this text have been reproduced from Taylor et al. 17 © The Author 2017. Published by Oxford University Press on behalf of the International Epidemiological Association. This is an Open Access article distributed under the terms of the Creative Commons Attribution License (http://creativecommons.org/licenses/by/4.0/), which permits unrestricted reuse, distribution, and reproduction in any medium, provided the original work is properly cited.
In this chapter, we estimate the effects of prescribing varenicline or NRT on patients’ likelihood of smoking cessation using multivariable-adjusted, propensity score and instrumental variable regression. Varenicline has been demonstrated to be efficacious in RCTs and network meta-analysis of RCTs. However, these trials had < 1 year of follow-up and may not have been representative of the population seen in primary care.
Population characteristics
Between 1 September 2006 and the end of follow-up, 1,875,980 smoking cessation prescriptions were issued to 287,079 patients. Of these, 220,136 patients met our eligibility criteria (see Figure 2 for a flow chart of exclusion and inclusion of prescriptions and patients into the study). Patients who were prescribed NRT received patches, gum, oral or nasal spray, inhaler, lozenges or microtabs, and 23% received more than one type of NRT at their first prescription. The proportion of current smokers who were prescribed NRT or varenicline in primary care has fallen between 2007 and 2015 (Figure 3). Nationally, the number of NRT prescriptions issued fell by 65% from 110,608 in February 2013 to 38,303 in January 2018. Prescriptions of varenicline fell by 59% from 71,551 in February 2013 to 29,645 in January 2018. 25
FIGURE 3.
The per cent of smokers prescribed NRT or varenicline between 2007 and 2015 in the CPRD.
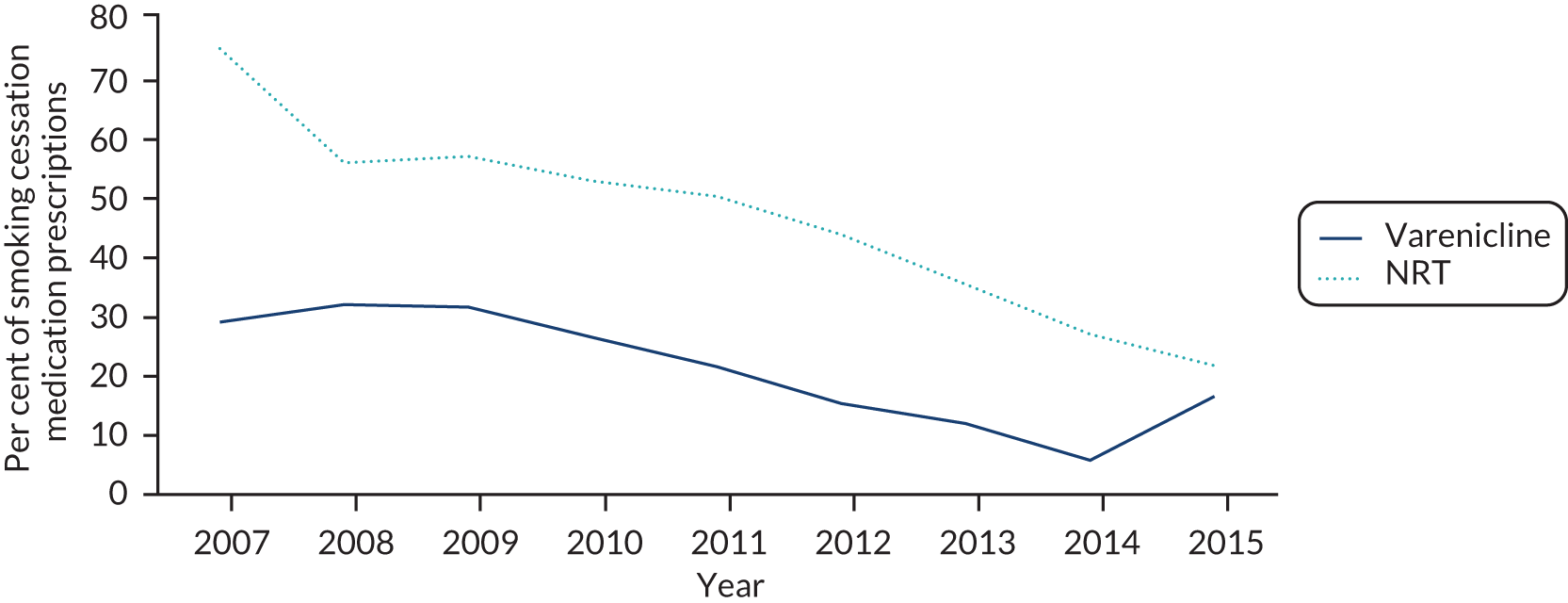
Table 1 shows the baseline characteristics of the patients who were prescribed varenicline and NRT. Patients who were prescribed varenicline were healthier in almost every way. They were younger, visited their GP less frequently, had fewer comorbidities, were less likely to have misused alcohol or drugs, were less likely to have a diagnosis of bipolar disorder, depression, a neurotic disorder, self-harm, a rare mental disorder, or were less likely to be prescribed antidepressants, antipsychotics, hypnotics/anxiolytics or other psychotropic medications. There were few differences by BMI or length of prior registration.
Characteristic | NRT (N = 149,526) | Varenicline (N = 70,610) | Whole sample (N = 220,136) |
---|---|---|---|
Age at time of their first prescription (years),a mean (SD) | 46.4 (15.4) | 44.5 (13.2) | 45.8 (14.9) |
Sex (female), % (n) | 53.7 (80,348) | 50.2 (35,466) | 52.6 (115,814) |
IMD score, median | 12 | 12 | 12 |
Number of GP visits 1 year prior to first prescription,a mean (SD) | 7.9 (7.4) | 6.3 (6.1) | 7.4 (7.0) |
BMI (kg/m2),a,b mean (SD) | 26.4 (6.4) | 26.5 (5.9) | 26.4 (6.1) |
Year of first prescription | 2009 | 2010 | 2009 |
Days of history,a mean (SD) | 3158.7 (1892.1) | 3283.9 (1976.6) | 3198.9 (1920.5) |
Comorbidity ever (Charlson Comorbidity Index), % (n) | 37.6 (56,274) | 31.9 (22,523) | 35.8 (78,797) |
Alcohol misuse ever, % (n) | 8.3 (12,422) | 6.0 (4199) | 7.6 (16,621) |
Drug misuse ever, % (n) | 3.1 (4595) | 1.9 (1357) | 2.7 (5952) |
Bipolar disorder ever, % (n) | 1.0 (1464) | < 1.0 (160) | < 1.0 (1624) |
Depression ever, % (n) | 35.0 (52,233) | 29.2 (20,615) | 33.1 (72,848) |
Neurotic disorder ever, % (n) | 24.7 (36,921) | 20.1 (14,189) | 23.2 (51,110) |
Self-harm ever, % (n) | 10.6 (15,903) | 8.7 (6169) | 10.0 (22,072) |
Other rare mental disorder ever, % (n) | 6.9 (10,343) | 4.0 (2832) | 6.0 (13,175) |
Antidepressant prescription ever, % (n) | 50.1 (74,921) | 43.1 (30,435) | 47.9 (105,356) |
Antipsychotic prescription ever, % (n) | 20.0 (29,873) | 14.8 (10,459) | 18.3 (40,332) |
Hypnotics/anxiolytics prescription ever, % (n) | 21.1 (31,513) | 17.6 (12,415) | 20.0 (43,928) |
Other psychotropic medication, % (n) | < 1.0 (473) | < 1.0 (120) | < 1.0 (593) |
Differences in smoking cessation rates by treatment prescribed
Patients prescribed varenicline were more likely to be recorded as a former smoker at all follow-up periods (Figure 4). Three months after the first prescription, 18.8% and 14.1% of patients prescribed varenicline and NRT, respectively, were recorded as a former smoker. These proportions increased to 32.3% and 28.4%, respectively, by 4 years after the first prescription. Figure 5 and Table 2 illustrate the relative difference in quit rates between the two treatments over time. Patients prescribed varenicline were 47% [95% confidence interval (CI) 42% to 52%] more likely to quit after 3 months than patients prescribed NRT. The relative difference in quit rates fell over time; patients prescribed varenicline were 23% (95% CI 21% to 26%) more likely to have quit after 4 years than patients prescribed NRT. These differences slightly attenuated, but remained detectable, after multiple imputation and adjustment for a full range of confounders.
FIGURE 4.
The proportion of patients who quit, by medication prescribed, at 3, 6 and 9 months and 1, 2 and 4 years after exposure (n = 220,136). Reproduced from Taylor et al. 17 © The Author 2017. Published by Oxford University Press on behalf of the International Epidemiological Association. This is an Open Access article distributed under the terms of the Creative Commons Attribution License (http://creativecommons.org/licenses/by/4.0/), which permits unrestricted reuse, distribution, and reproduction in any medium, provided the original work is properly cited.
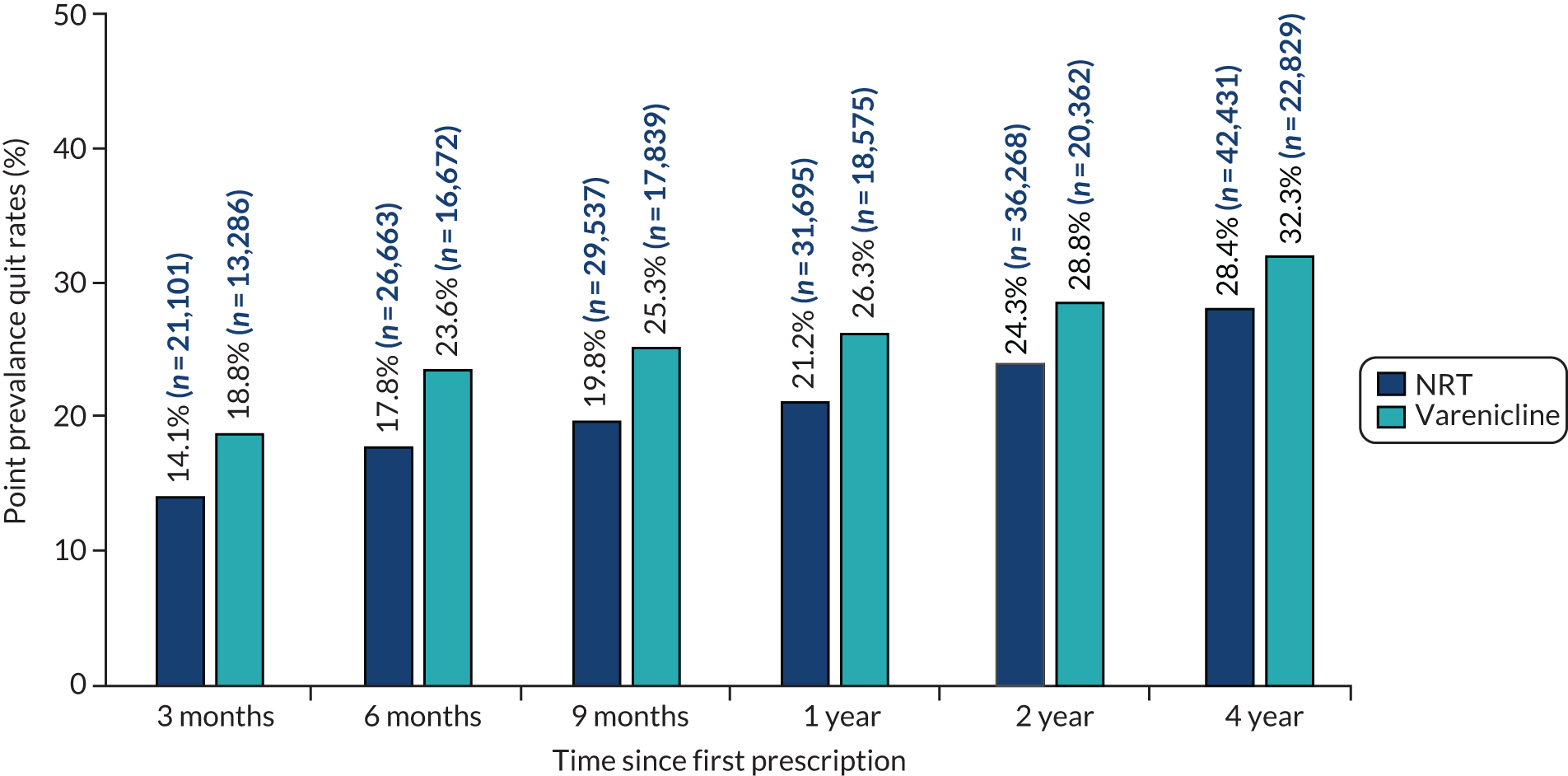
FIGURE 5.
Patients prescribed varenicline were more likely to be non-smokers 3, 6 and 9 months and 1, 2 and 4 years after their first prescription than those prescribed NRT. Fully multivariable-adjusted logistic regression model using multiply imputed data: odds ratios and 95% CIs presented. The difference in smoking cessation rates peaks at 6 months and declines over the following 3.5 years (n = 220,136). Reproduced from Taylor et al. 17 © The Author 2017. Published by Oxford University Press on behalf of the International Epidemiological Association. This is an Open Access article distributed under the terms of the Creative Commons Attribution License (http://creativecommons.org/licenses/by/4.0/), which permits unrestricted reuse, distribution, and reproduction in any medium, provided the original work is properly cited.
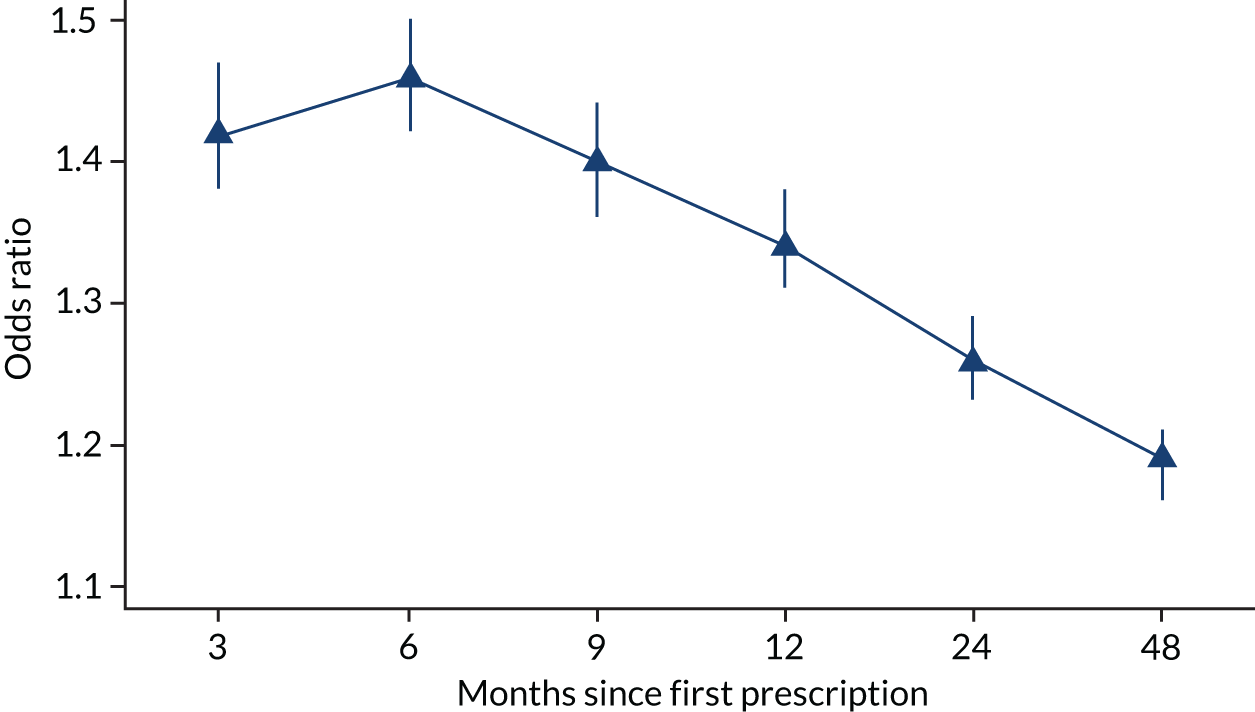
Model | Odds ratio (95% CI) | |||||
---|---|---|---|---|---|---|
3 months | 6 months | 9 months | 1 year | 2 years | 4 years | |
Partial adjustedb | 1.47 (1.42 to 1.52) | 1.50 (1.46 to 1.55) | 1.44 (1.4 to 1.48) | 1.38 (1.35 to 1.42) | 1.30 (1.27 to 1.33) | 1.23 (1.21 to 1.26) |
Fully adjustedc | 1.42 (1.38 to 1.47) | 1.46 (1.42 to 1.50) | 1.40 (1.36 to 1.44) | 1.34 (1.31 to 1.38) | 1.26 (1.23 to 1.29) | 1.19 (1.16 to 1.21) |
In almost every way we could measure, patients who were prescribed varenicline were different from those who were prescribed NRT. As a result, we were concerned that our multivariable results might suffer from residual confounding. Therefore, we estimated the effects of the medications using propensity score and instrumental variable methods. The patients’ characteristics were well balanced across the propensity score (see Report Supplementary Material 1, Figures S1 and S2). The covariate balance tests presented in Report Supplementary Material 1, Figure S3, suggest that the measured covariates were more balanced across the instrument than across the actual prescription. The effects of prescribing varenicline estimated using the propensity score and instrumental variable analysis were consistent with the multivariable-adjusted regression above (see Report Supplementary Material 1, Tables S1 and S2).
Heterogeneity in the effect of varenicline by socioeconomic position
Patients from more deprived areas were 9% (95% CI 8% to 10%) less likely to be prescribed varenicline after controlling for age and sex. Patients from areas in the least deprived quintile were slightly more likely to have quit between 3 and 12 months after their first prescription. However, these differences could be attributable to chance (p > 0.05). The longer-term (after 2 and 4 years) differences in relative quit rates by treatment were similar in areas of high and low deprivation (Figure 6). Therefore, there was little evidence that the effects of varenicline and NRT differed across socioeconomic groups. There was insufficient statistical power to detect treatment effect heterogeneity using instrumental variable analysis (see Report Supplementary Material 1, Tables S3 and S4). However, instrumental variable point estimates suggested that varenicline increased the likelihood of smoking cessation at all follow-up periods.
FIGURE 6.
The effectiveness of varenicline stratified by SEP. Partially adjusted odds ratios and 95% CIs for the association of prescription of varenicline vs. NRT and smoking cessation at 3, 6 and 9 months and 1, 2 and 4 years after exposure, by the level of deprivation as measured by the IMD. IMD is an increasing measure of neighbourhood disadvantage. Reproduced from Taylor et al. 17 © The Author 2017. Published by Oxford University Press on behalf of the International Epidemiological Association. This is an Open Access article distributed under the terms of the Creative Commons Attribution License (http://creativecommons.org/licenses/by/4.0/), which permits unrestricted reuse, distribution, and reproduction in any medium, provided the original work is properly cited.
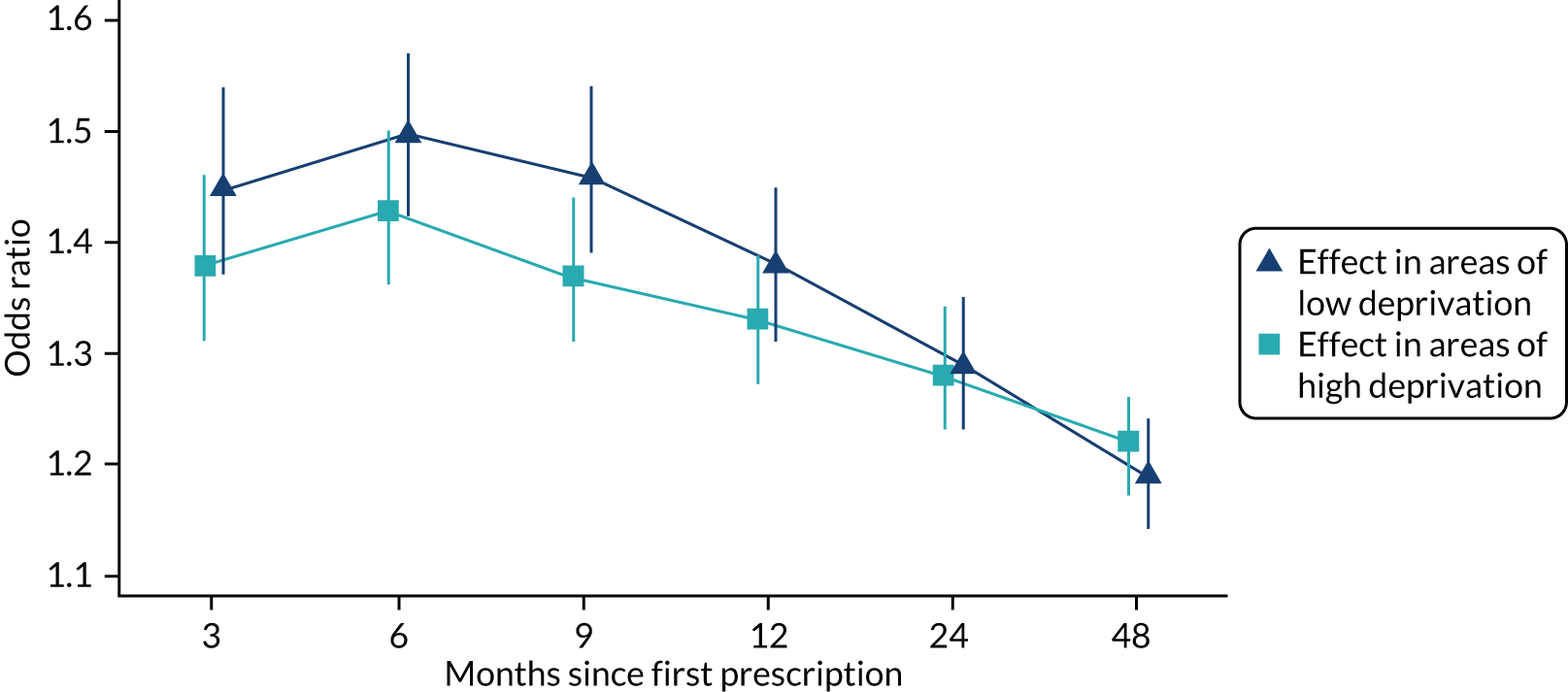
Sensitivity analyses
Missing data
Patients prescribed varenicline and NRT had similar numbers of missing data. After 2 years, 20.3% of patients had no new smoking status records (see Report Supplementary Material 1, Table S5). The patients who were missing smoking status records were more likely to be younger and male, were less likely to visit the GP and had fewer comorbidities (see Report Supplementary Material 1, Table S6). We investigated whether or not the missing smoking status records affected our results using multiple imputation. The multiply imputed results were similar to our main results, which assumed that patients with no smoking status records in the follow-up period were continuing smokers (see Report Supplementary Material 1, Table S7). 28
Varenicline versus nicotine replacement monotherapy versus multitherapy
Patients who were prescribed varenicline were more likely to have ceased smoking after 2 years than those who were prescribed any NRT [odds ratio (OR) 1.30, 95% CI 1.27 to 1.33]. Patients who were prescribed varenicline were more likely to have ceased smoking after 2 years than patients who were prescribed NRT monotherapy defined by route of administration (OR 1.32, 95% CI 1.29 to 1.36). Patients who were prescribed varenicline were more likely to have ceased smoking after 2 years than patients who were prescribed NRT multitherapy (OR 1.23, 95% CI 1.19 to 1.27). Patients who were prescribed NRT multitherapy were more likely to have ceased smoking after 2 years than those prescribed NRT monotherapy (OR 1.08, 95% CI 1.05 to 1.12). After adjustment for the same set of covariates as we included in the primary analysis, the OR of cessation at 2 years comparing varenicline with NRT multitherapy was 1.22 (95% CI 1.17 to 1.28). The fully adjusted OR for cessation at 2 years comparing varenicline with NRT monotherapy was 1.23 (95% CI 1.19 to 1.28). After adjustment, there was little evidence of differences in quit rates between those prescribed NRT monotherapy and NRT multitherapy (OR 1.01, 95% CI 0.97 to 1.05). These results suggest that patients who were prescribed varenicline were more likely to be non-smokers after 2 years than patients who were prescribed either NRT monotherapy or NRT multitherapy.
Treatment compliance
On average, patients who were prescribed varenicline were issued 2.8 [standard deviation (SD) 1.6] prescriptions for varenicline in the 3 months following their first eligible prescription, and received an average of 107.5 (SD 120.7) tablets; 49.7% (35,076/70,610) of these patients received a full course of varenicline (i.e. ≥ 12 weeks) (see British National Formulary, chapter 4.10.214). Patients who were initially prescribed NRT were issued 2.5 (SD 2.2) prescriptions for NRT on average during the 3 months after their first eligible prescription. Patients who were issued a single varenicline prescription only were less likely to cease smoking after 2 years than those who were prescribed any NRT (OR 0.59, 95% CI 0.57 to 0.62). Patients who were prescribed at least two varenicline prescriptions were more likely to cease smoking after 2 years than those prescribed any NRT (OR 1.63, 95% CI 1.59 to 1.67). Patients prescribed two or more varenicline prescriptions in the 2 months following their first prescription were more likely to cease smoking than those who were issued a single varenicline prescription (OR 2.80, 95% CI 2.67 to 2.92). After adjustment for the same set of covariates as we included in the primary analysis, the difference in cessation between patients issued a single varenicline prescription and those issued NRT increased (OR 0.55, 95% CI 0.51 to 0.58). The difference in cessation at 2 years between patients prescribed two or more varenicline prescriptions and any NRT attenuated (OR 1.51, 95% CI 1.46 to 1.57). The adjustment did not meaningfully alter the difference between patients prescribed two or more varenicline prescriptions and those prescribed a single varenicline prescription (OR 2.79, 95% CI 2.61 to 2.97). These results suggest that patients who were prescribed two or more varenicline prescriptions, who have a longer duration of treatment, were less likely to be smokers after 2 years than those prescribed either NRT monotherapy or NRT multitherapy.
Multiple quit attempts
Patients who were prescribed varenicline were more likely to quit smoking than patients who were prescribed NRT who had previously had any smoking cessation prescriptions (OR 1.27, 95% CI 1.22 to 1.31). After adjustment for the same set of covariates as included in the primary analysis, the association in patients previously prescribed smoking cessation prescription remained similar (OR 1.27, 95% CI 1.22 to 1.33). Patients who had never previously been prescribed smoking cessation treatment who were prescribed varenicline were more likely to cease smoking than those who were prescribed NRT (OR 1.32, 95% CI 1.29 to 1.36). This association attenuated after adjustment for the covariates included in the primary analysis (OR 1.23 95% CI 1.19 to 1.29). Patients who had previously received a smoking cessation prescription were less likely to quit (OR 0.90, 95% CI 0.88 to 0.92) than those who had never received a smoking cessation prescription. This difference attenuated after adjustment (OR 0.93, 95% CI 0.91 to 0.96). These results suggest that patients prescribed varenicline are more likely to have ceased smoking after 2 years, irrespective of whether or not they had previously been prescribed smoking cessation therapy. Patients who had previously received smoking cessation therapies were less likely to quit.
Comparison with other studies
Figure 7 compares our results with the previously published results from RCTs. Our estimates of the effectiveness of varenicline compared with NRT at 6 months were similar to, and considerably more precise than, the results estimated via the large RCT reported by Anthenelli et al. 10 and a network meta-analysis conducted by Cahill et al. 7 Combined, the studies suggested that, on average, patients prescribed varenicline were 44% (95% CI 33% to 55%) more likely to quit smoking after 6 months.
FIGURE 7.
Random-effects meta-analysis comparing effect estimates (OR and 95% CI) from existing studies examining the effect of varenicline vs. NRT for smoking cessation at 6- to 12-month follow-up. Reproduced from Taylor et al. 17 © The Author 2017. Published by Oxford University Press on behalf of the International Epidemiological Association. This is an Open Access article distributed under the terms of the Creative Commons Attribution License (http://creativecommons.org/licenses/by/4.0/), which permits unrestricted reuse, distribution, and reproduction in any medium, provided the original work is properly cited.
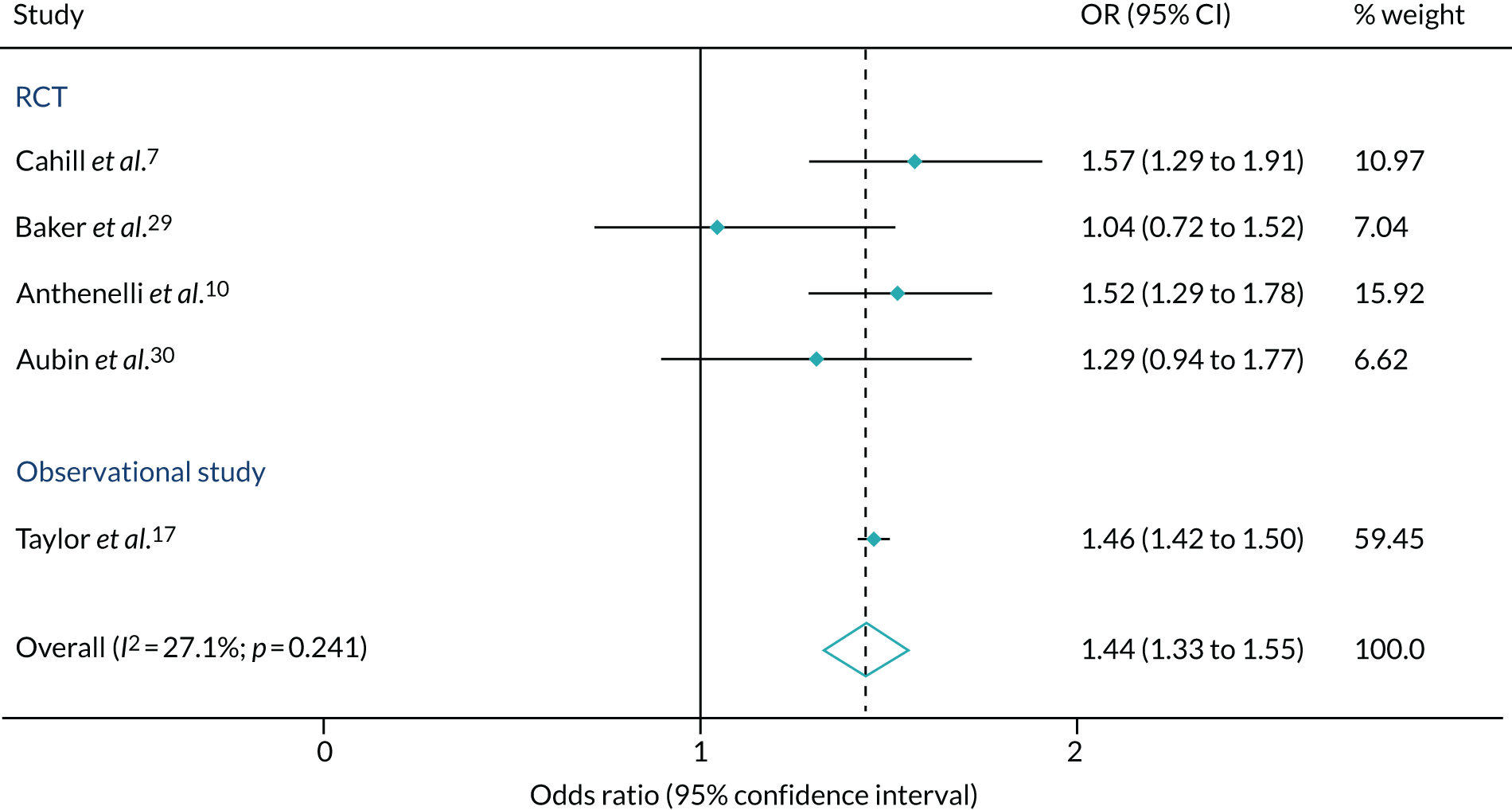
Chapter 4 The effects of prescribing varenicline on longer-term health outcomes
Parts of this text have been reproduced from Davies et al. 16 © 2018 The Authors. Addiction published by John Wiley & Sons Ltd on behalf of Society for the Study of Addiction. This is an open access article under the terms of the Creative Commons Attribution License, which permits use, distribution and reproduction in any medium, provided the original work is properly cited.
Although randomised trials are the gold standard for demonstrating efficacy, they are underpowered to detect the effect of treatments on rare secondary outcomes and have a limited follow-up. For smoking cessation treatments, follow-up is generally ≤ 1 year. In this chapter, we investigate the effect of varenicline on health outcomes between 3 and 48 months after the first prescription. In the results presented below, we focus on the instrumental variable analyses because the specification tests suggested that the multivariable-adjusted and propensity score regression results may suffer from residual confounding. We present the results for the propensity score analysis in Report Supplementary Material 1, Tables S9–S11.
Population characteristics
Of the 287,079 patients who were prescribed smoking cessation treatments during the study period, 126,718 patients met inclusion criteria for assessing the health outcomes. Of these patients, most (84,976, 67.1%) were prescribed NRT and the remainder (41,742, 32.9%) were prescribed varenicline. Most (73%) of the patients who were prescribed varenicline were issued an additional prescription of varenicline in the 3 months following their first prescription. However, only 20% of patients were issued ≥ 151 tablets within the 3-month treatment window. This is the minimum number of tablets patients needed to adhere to a full course of treatment. As with the effectiveness analysis presented in Chapter 3, patients in this restricted sample who were prescribed varenicline, and had linked Hospital Episode Statistics and ONS mortality data, were healthier in almost every way that we could measure (Table 3).
Characteristic | NRT (N = 84,976) | Varenicline (N = 41,742) |
---|---|---|
Male, n (%) | 39,285 (46.2) | 20,928 (50.1) |
Median age (years) (SD) | 46 (24) | 44 (19) |
BMI (kg/m2), mean (SD) | 25.5 (7.1) | 25.7 (6.6) |
Misuses alcohol, n (%) | 6199 (7.3) | 2086 (5.0) |
Misuses drugs, n (%) | 2484 (2.9) | 747 (1.8) |
Least deprived fifth of patients, n (%) | 11,209 (13.2) | 6427 (15.4) |
Most deprived fifth of patients, n (%) | 20,896 (24.6) | 9177 (22.0) |
Median number of primary care visits in prior year (SD) | 6 (8) | 5 (6) |
Prescribed before 2009, n (%) | 38,568 (45.4) | 10,422 (25.0) |
Previous prescription of, n (%) | ||
Hypnotics/anxiolytic | 17,419 (20.5) | 7114 (17.0) |
Antipsychotic | 16,918 (19.9) | 5975 (14.3) |
Antidepressant | 42,450 (50.0) | 17,895 (42.9) |
Statins | 16,534 (19.5) | 6217 (14.9) |
Antihypertensive | 17,608 (20.7) | 6974 (16.7) |
Diabetic medication | 7798 (9.2) | 2710 (6.5) |
Previous diagnosis of, n (%) | ||
Self-harm | 8846 (10.4) | 3614 (8.7) |
Myocardial infarction | 2624 (3.1) | 708 (1.7) |
COPD | 6837 (8.0) | 2516 (6.0) |
Chronic disease (Charlson Comorbidity Index) | 32,517 (38.3) | 13,277 (31.8) |
Any psychiatric disease | 41,275 (48.6) | 17,005 (40.7) |
Follow-up time (years), mean (SD) | 4.56 (2.07) | 3.72 (1.84) |
Specification tests of the instrumental variable assumptions
The physicians’ previous prescriptions were strongly associated with their prescriptions to their subsequent patients. A doctor who issued varenicline to their previous patient was 24.5% (95% CI 23.3% to 25.9%) more likely to prescribe varenicline to their subsequent patients. The instrument was the number of varenicline prescriptions issued to the physicians’ previous seven patients who were receiving smoking cessation treatment. Hence, the physicians’ prescribing behaviours explain a substantial proportion of the variation in treatment rates. The partial F-statistic, a measure of instrument strength, ranged from 4045 to 7775. 31 A value of the partial F-statistic ≥ 10 suggests that the analysis is unlikely to suffer from weak instrument bias. 32 This value of the F-statistic does not guarantee that the estimates will be unbiased, or will be sufficiently powered to detect effect sizes of interest. However, it does mean that the coverage of the estimates (CIs) should be correct. Values of the F-statistic < 10 can result in overly precise CIs and over-rejection of the null hypothesis. This problem is a particular concern in analyses with more than one instrument. This statistic indicates that the instrument was very strong. Furthermore, the potential confounders were more balanced across the instrument than across the patients’ prescriptions (Figure 8 and also see Report Supplementary Material 1, Table S8). These results mean that even after taking account of the strength of the physicians’ preferences (the instrument), the bias for the instrumental variable analysis (attributable to all of the observed potential confounders except BMI, the number of prior GP visits and deprivation) was likely to be lower than for the patients’ prescriptions. The estimated instrumental variable bias terms for BMI, number of GP visits and deprivation were similar to the linear regression bias terms.
FIGURE 8.
The bias terms for linear and instrumental variable regression. These plots indicate the bias caused by omitting a single observed covariate from a multivariable-adjusted or instrumental variable regression analysis. This analysis treats the omitted covariate as an ‘outcome’ and the exposure or instrumental variable (prior prescription) as the dependent variable. Relative linear regression and instrumental variable bias component terms are indicated by squares (▪) and triangles (▲) respectively. 95% CIs are plotted. The patients’ actual prescriptions more strongly associated with the measured covariates than the proposed instrument, even after taking into account the instrument strength. This statistic suggests that the instrumental variable estimates are likely to be less biased than the linear regression estimates. CIs allow for clustering between physicians. We adjusted each bias term except sex for year, sex and age and no other covariates. The instrumental variable was defined using seven prior prescriptions. Reproduced from Davies et al. 16 © 2018 The Authors. Addiction published by John Wiley & Sons Ltd on behalf of Society for the Study of Addiction. This is an Open Access article distributed in accordance with the terms of the Creative Commons Attribution (CC BY 4.0) license, which permits others to distribute, remix, adapt and build upon this work, for commercial use, provided the original work is properly cited. See: http://creativecommons.org/licenses/by/4.0/. The figure includes minor additions and formatting changes to the original text.
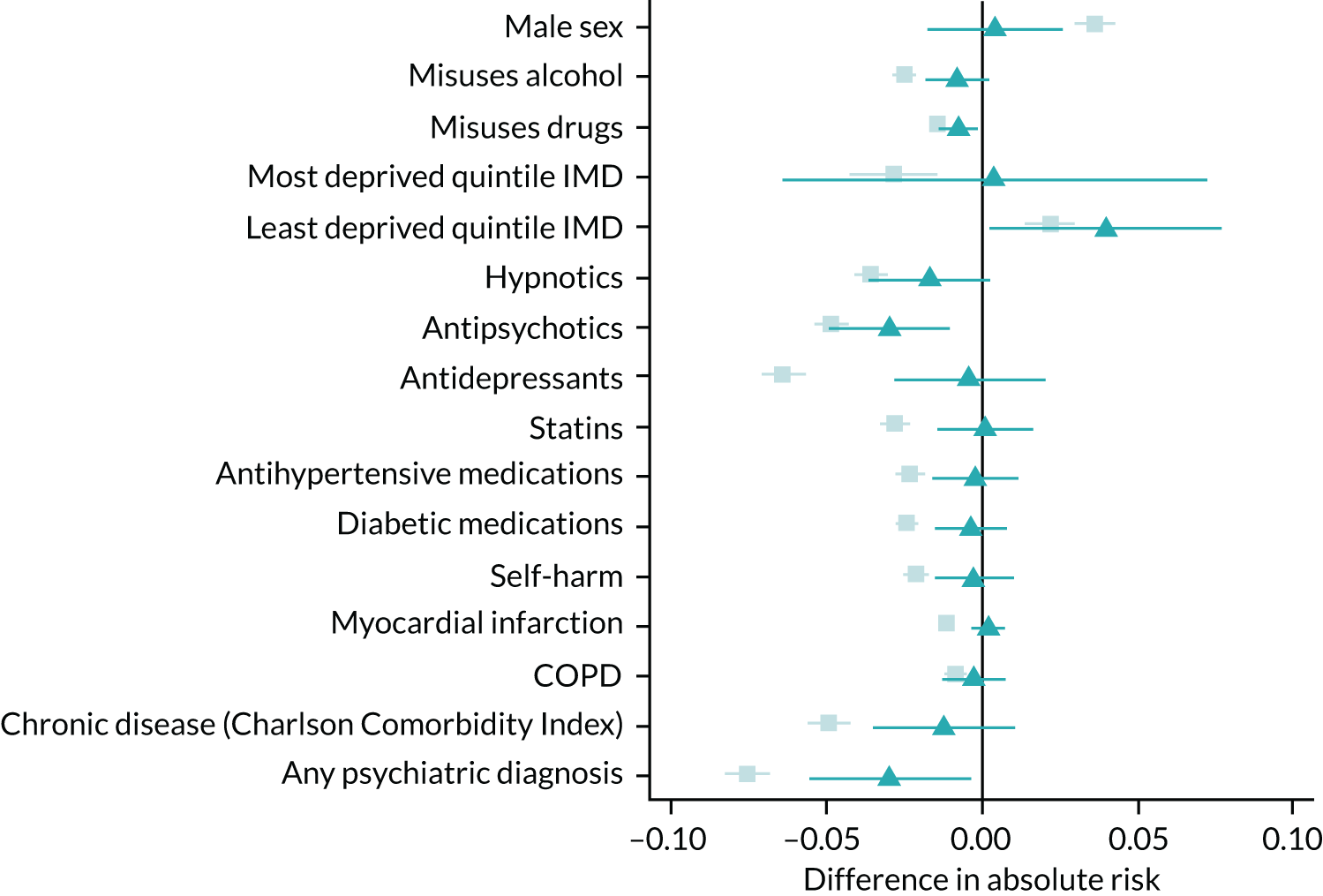
The effects of varenicline on mortality and morbidity after 2 years
Patients prescribed varenicline had slightly higher rates of all-cause mortality than those who were prescribed NRT (risk difference per 100 patients treated = 0.67, 95% CI –0.11 to 1.46) (Figure 9). From the instrumental variable analyses, there were few detectable differences in cause-specific mortality, all-cause or cause-specific hospitalisation, or primary care diagnoses of myocardial infarction or COPD (see Figure 9). Our results suggest that positive or negative effects > 2.5 per 100 patients treated are unlikely for all-cause hospitalisation. Varenicline is unlikely to cause positive or negative effects > 1 per 100 patients on the other outcomes, including a lung cancer diagnosis.
FIGURE 9.
The effects of prescribing varenicline vs. NRT on mortality and morbidity in the 2 years following the first prescription. The estimates are calculated from instrumental variable regression. CIs allow for clustering between physicians. Instrumental variable results use seven prior prescriptions and adjust for the year of first prescription, sex and age. Reproduced with modification Davies et al. 16 © 2018 The Authors. Addiction published by John Wiley & Sons Ltd on behalf of Society for the Study of Addiction. This is an Open Access article distributed in accordance with the terms of the Creative Commons Attribution (CC BY 4.0) license, which permits others to distribute, remix, adapt and build upon this work, for commercial use, provided the original work is properly cited. See: http://creativecommons.org/licenses/by/4.0/. The figure includes minor additions and formatting changes to the original text.
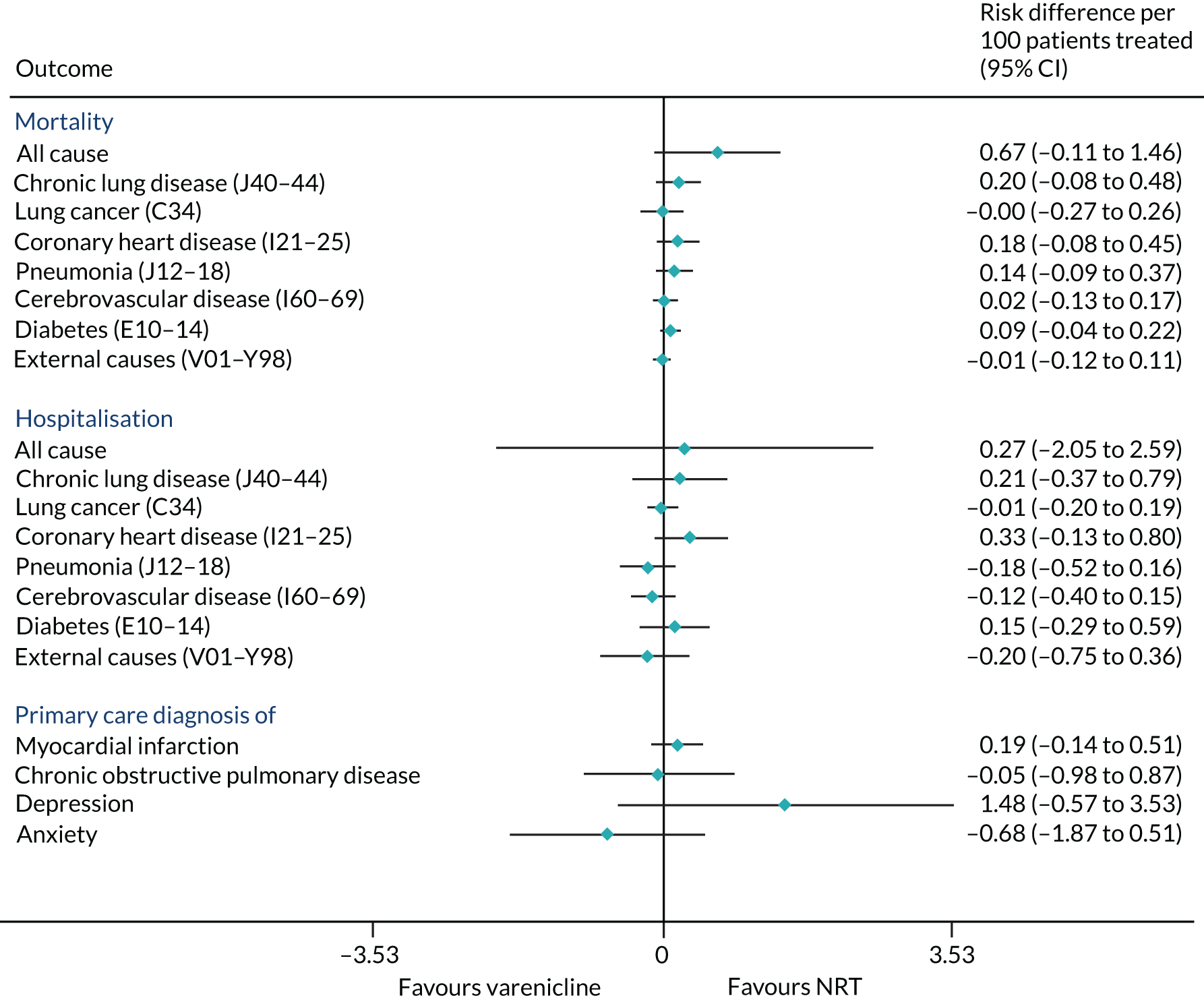
Consistent with the broader literature, the multivariable-adjusted results suggested that after 2 years, patients who were prescribed varenicline had substantially lower mortality and morbidity than patients who were prescribed NRT (Figure 10). Patients who were prescribed varenicline had lower rates of all-cause and cause-specific mortality, except lung cancer (Figure 11). After fully adjusting for all the baseline confounders listed in Table 3, the estimates modestly attenuated towards the null; however, substantial differences remained. The multivariable-adjusted estimates systematically differed from the instrumental variable estimates of the effect of varenicline after 2 years on all-cause mortality and hospital admission (pHausman = 4.14 × 10–9 and 6.35 × 10–8 respectively). The Hausman test is a test for the difference between the ordinary least squares and instrumental variable estimates. There were systematic differences in estimated effects on cause-specific mortality: chronic lung disease, coronary heart disease, pneumonia and diabetes (pHausman = 0.0006, = 0.005, = 0.001 and = 0.04 respectively). The instrumental variable results were inconsistent with the reduction in risk of hospitalisation for coronary heart disease estimated via multivariable adjustment (pHausman = 0.006). The effects of varenicline on other cause-specific hospitalisation, weight and the number of GP visits were similar for both multivariable-adjusted regression and instrumental variable regression.
FIGURE 10.
The effect of prescribing varenicline on (a) all-cause, (b) chronic lung disease-related (ICD-10 J40–44), (c) lung cancer (ICD-10 C34), (d) coronary heart disease (ICD-10 I21–25), (e) pneumonia-related (ICD-10 J12–18) and (f) cerebrovascular disease-related (ICD-10 I60–69) mortality in the 4 years following first prescription as indicated via ONS mortality records. Linear regression is indicated by squares (▪), the solid line adjusts for basic confounders, the dashed line adjusts for all confounders listed in Table 3, and the instrumental variable results are indicated by triangles (▲). 95% CIs are indicated. CIs allow for clustering between physicians. The multivariable-adjusted results adjust for all the covariates listed in Table 1. Instrumental variable results use seven prior prescriptions. Reproduced from Davies et al. 16 © 2018 The Authors. Addiction published by John Wiley & Sons Ltd on behalf of Society for the Study of Addiction. This is an Open Access article distributed in accordance with the terms of the Creative Commons Attribution (CC BY 4.0) license, which permits others to distribute, remix, adapt and build upon this work, for commercial use, provided the original work is properly cited. See: http://creativecommons.org/licenses/by/4.0/. The figure includes minor additions and formatting changes to the original text.
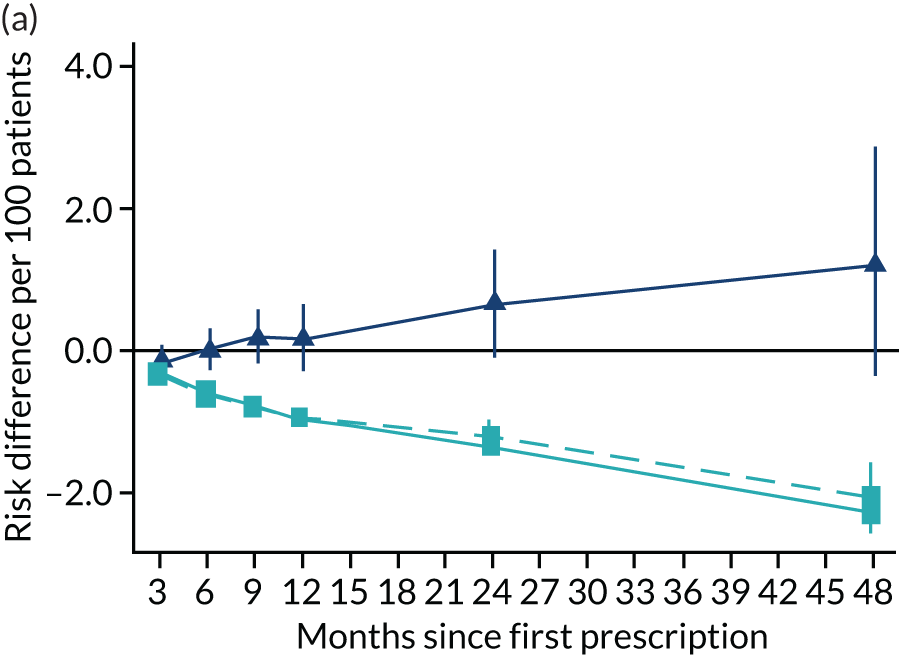
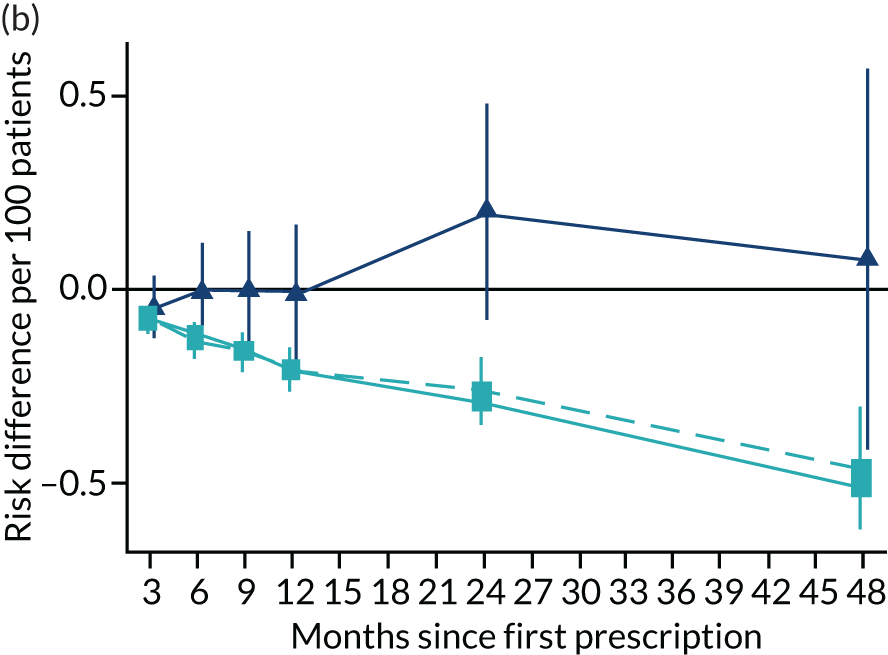
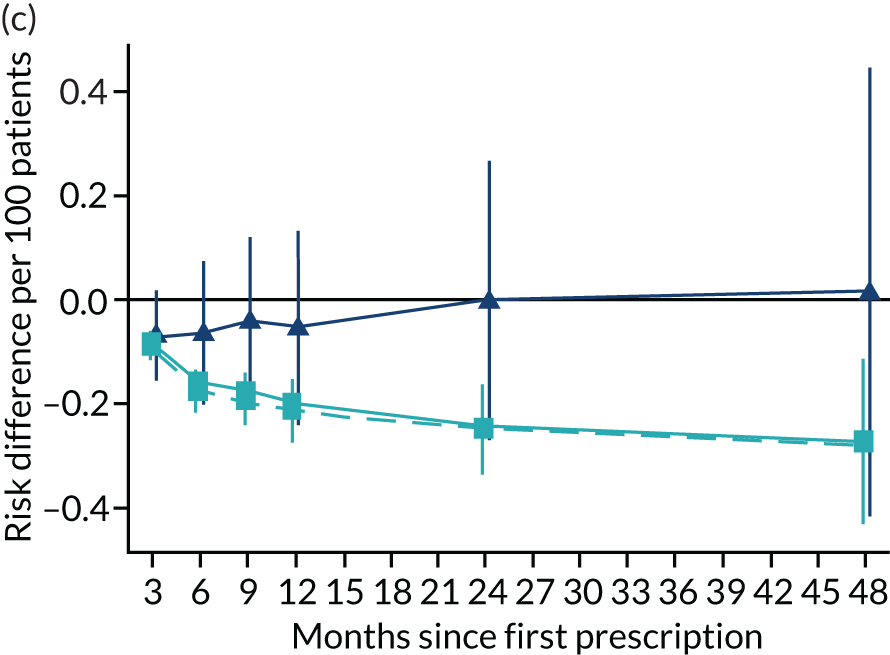
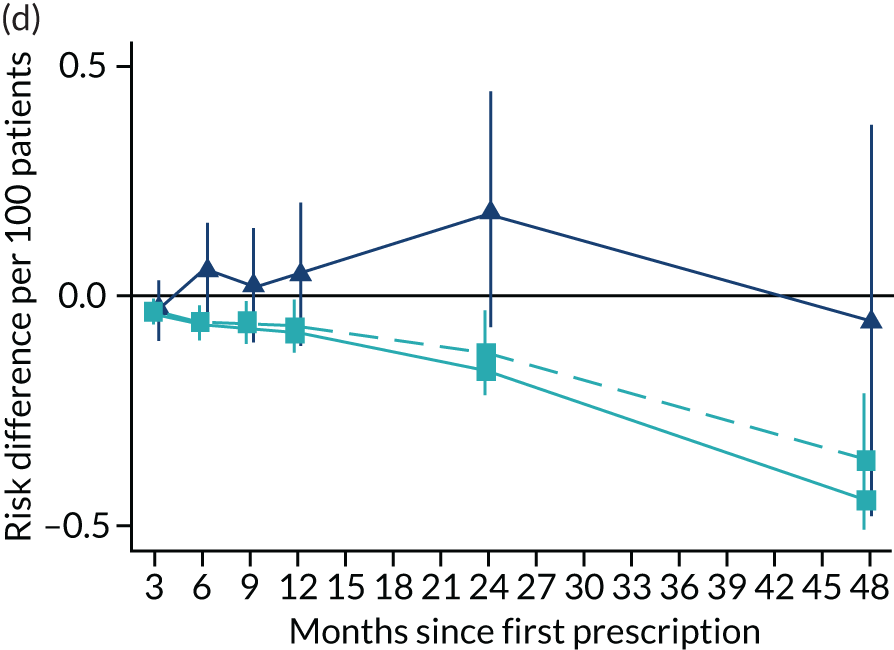
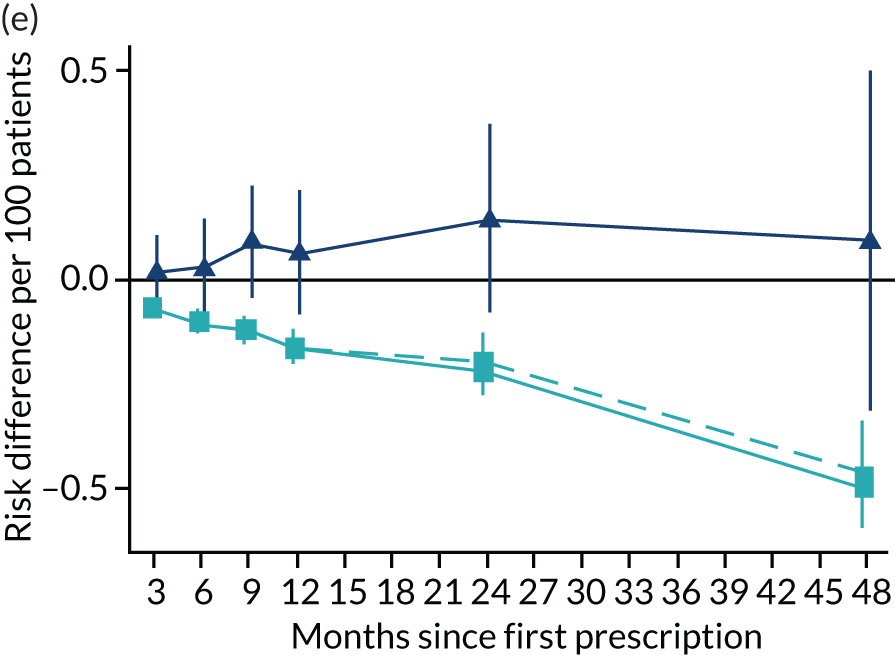
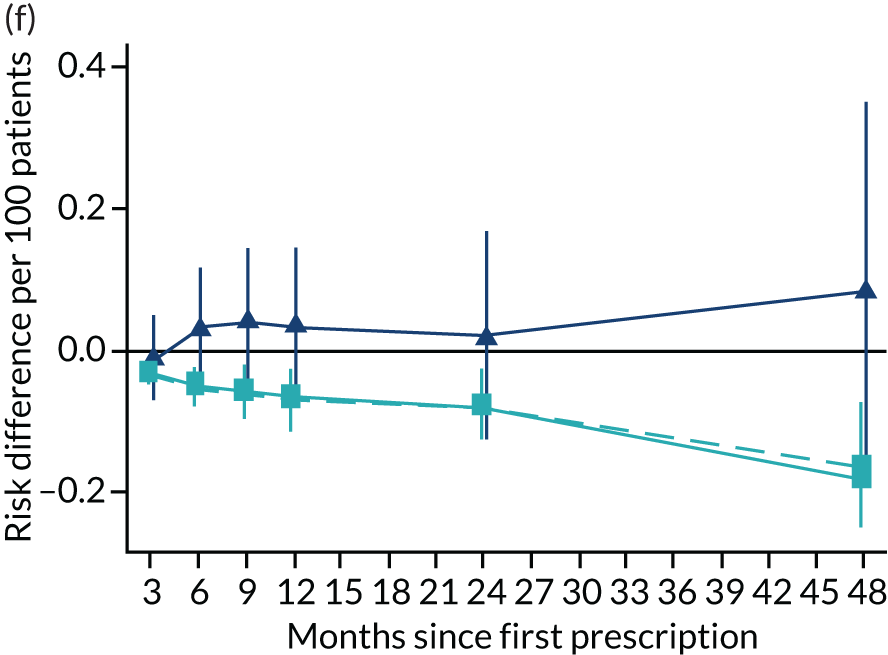
FIGURE 11.
The effect of prescribing varenicline on (a) all-cause, (b) chronic lung disease-related (ICD-10 J40–44), (c) lung cancer (ICD-10 C34), (d) coronary heart disease (ICD-10 I21–25), (e) pneumonia-related (ICD-10 J12–18) and (f) cerebrovascular disease-related (ICD-10 I60–69) hospital inpatient admission in the 4 years following first prescription as indicated via ONS death records. Linear regression is indicated by squares (▪), the solid line adjusts for basic confounders, the dashed line adjusts for all confounders listed in Table 3 and the instrumental variable results are indicated by triangles (▲). 95% CIs are indicated. CIs allow for clustering between physicians. The fully multivariable-adjusted results adjust for all the covariates listed in Table 1. Instrumental variable results use seven prior prescriptions. Reproduced from Davies et al. 16 © 2018 The Authors. Addiction published by John Wiley & Sons Ltd on behalf of Society for the Study of Addiction. This is an Open Access article distributed in accordance with the terms of the Creative Commons Attribution (CC BY 4.0) license, which permits others to distribute, remix, adapt and build upon this work, for commercial use, provided the original work is properly cited. See: http://creativecommons.org/licenses/by/4.0/. The figure includes minor additions and formatting changes to the original text.
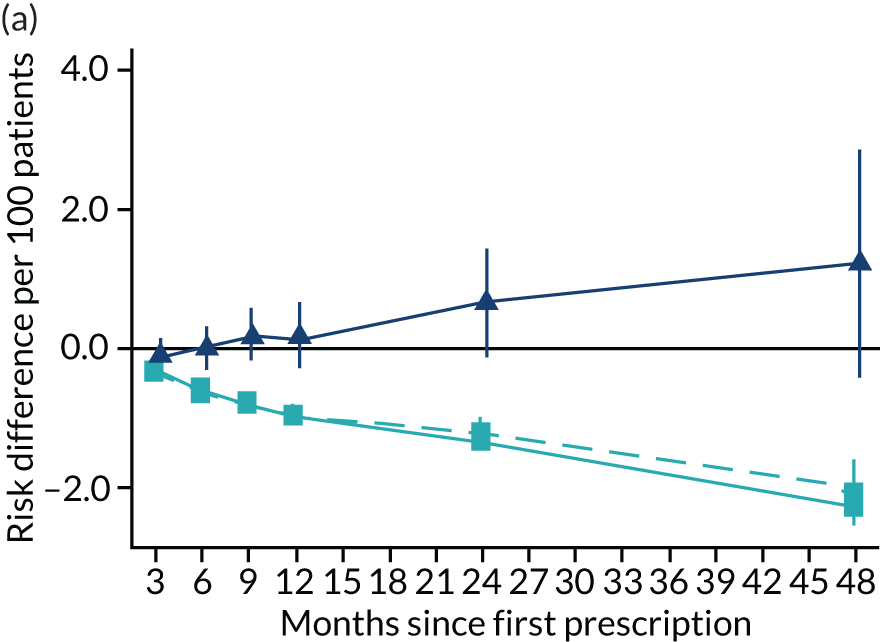
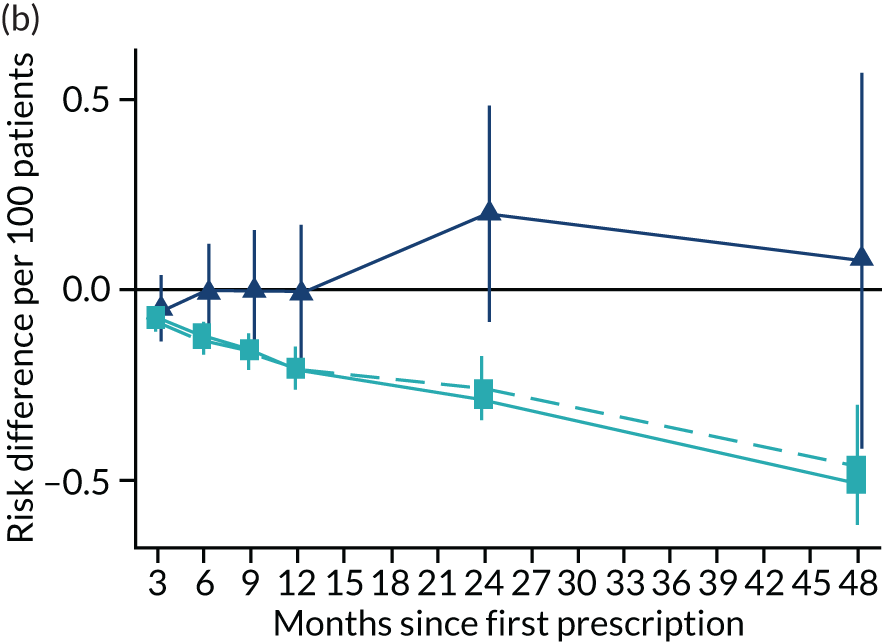
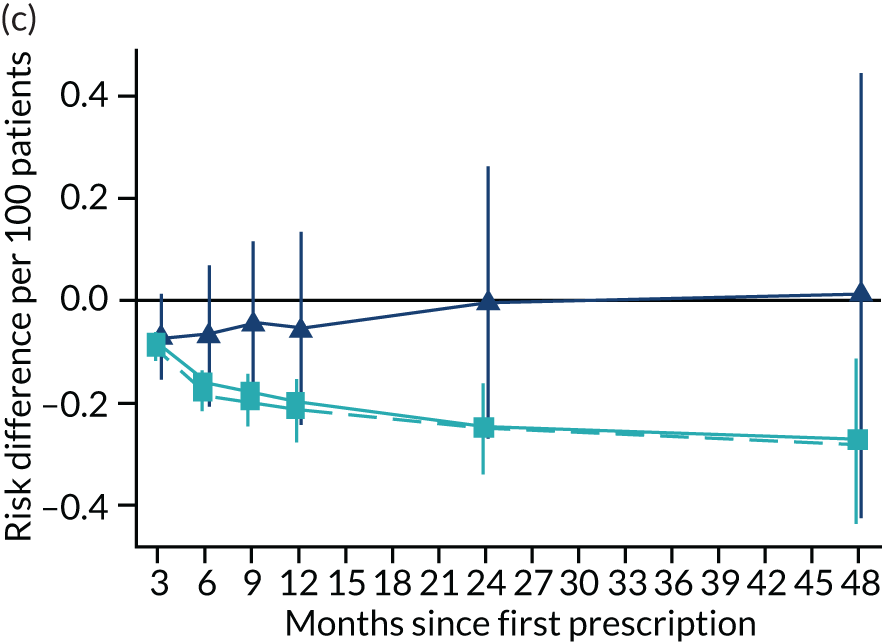
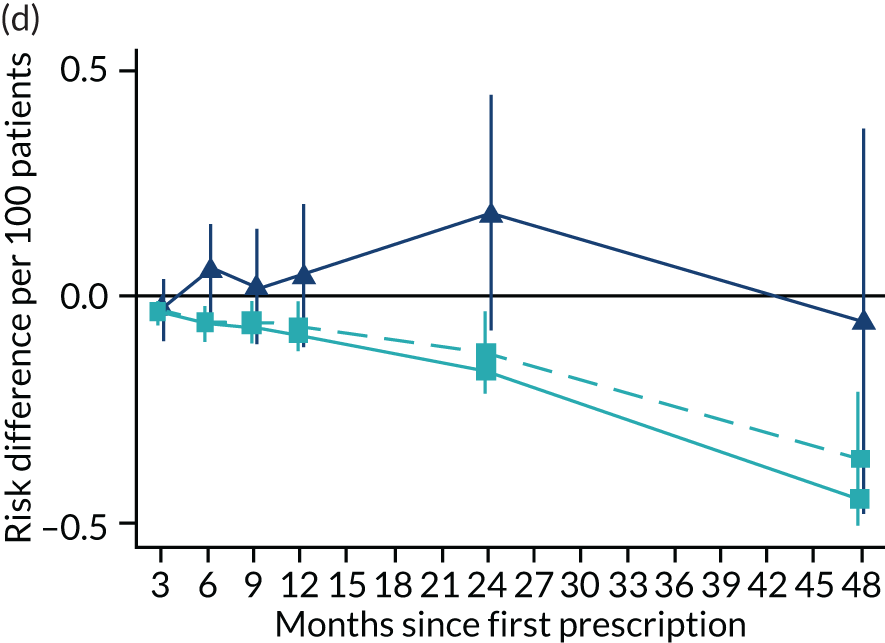
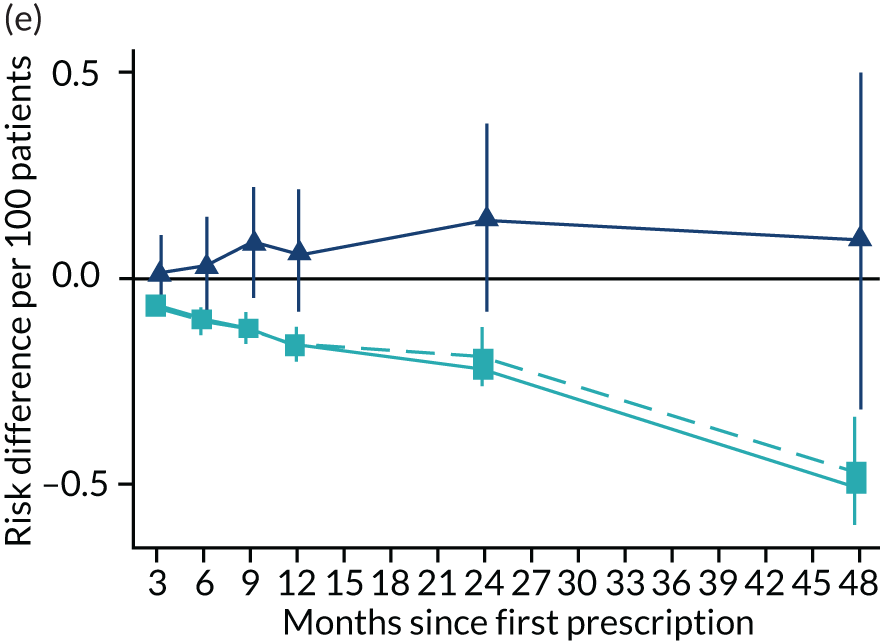
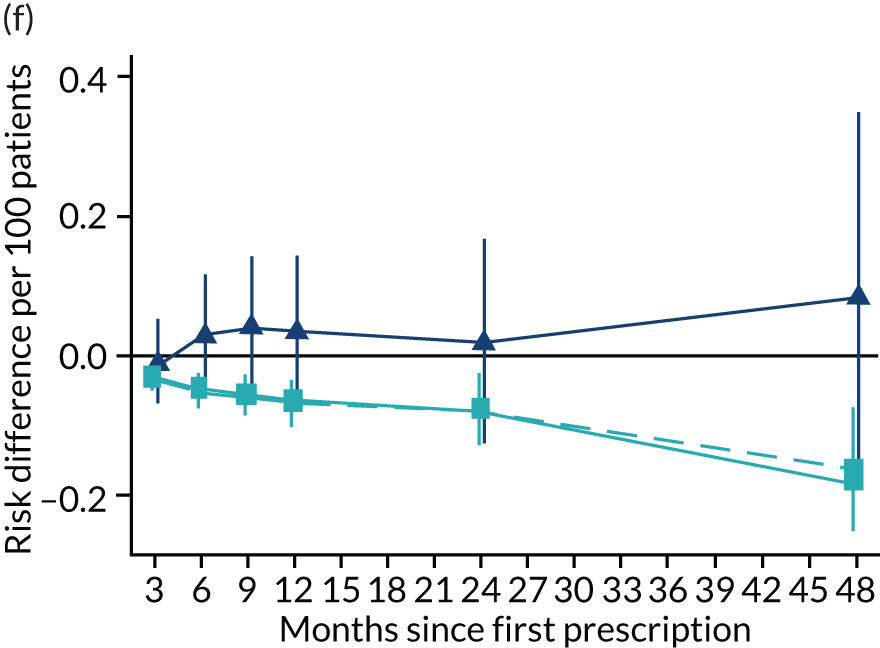
The effects of varenicline versus nicotine replacement therapy over time
Figure 10 illustrates the effects of prescribing varenicline versus NRT on rates of all-cause and cause-specific mortality over time. The navy line indicates the effects as estimated via instrumental variables and the solid light blue line indicates the minimally adjusted multivariable-adjusted regression. The dashed light blue line indicates the fully adjusted multivariable-adjusted regression estimates. The instrumental variable estimates provide little evidence that varenicline affects mortality at any follow-up period. The partially adjusted multivariable estimates suggest that all-cause and cause-specific mortality risks generally drop linearly after the first prescription. The exception to this is lung cancer mortality, which drops more quickly in months 3–6 than months 6–48. Adjustment for the full range of covariates reported in Table 1 resulted in little change in the estimates. One explanation of the difference between the instrumental variable and multivariable-adjusted results is that the latter suffer from residual confounding.
Figure 11 illustrates the effects of prescribing varenicline over 4 years on all-cause and cause-specific hospitalisation. The instrumental variable estimates suggest a modest reduction in all-cause admissions in the 3–9 months following the first prescription. There is little difference in rates of hospitalisation between months 12 and 24, and an imprecise positive effect at 48 months. The instrumental variable estimates of the effect of varenicline are too imprecise to distinguish them from the null hypothesis or the multivariable-adjusted estimates. The partially adjusted multivariable estimates of the effect on all-cause hospitalisation suggest a substantial protective effect that peaks 12 months after the first prescription, before modestly attenuating by 48 months. The cause-specific estimates for chronic lung disease, pneumonia and cerebrovascular disease suggest a protective effect. There was little evidence of a difference in the rates of admission for lung cancer or (after full adjustment) for coronary heart disease. These differences attenuated towards the null after adjustment but remained detectable.
The instrumental variable estimates suggest that prescribing varenicline caused an increase in weight, on average 1.14 kg (95% CI 0.09 to 2.20 kg) after 2 years; this effect was detectable across the entire follow-up period (Figure 12). The partially multivariable-adjusted results suggested a similar increase in weight across the entire follow-up period. The fully adjusted results were mildly attenuated towards the null. There were little differences between the instrumental variable and multivariable-adjusted estimates (pHausman for all follow-up periods > 0.01). The instrumental variable estimates suggest that prescribing varenicline caused a 19.16% (95% CI 11.17% to 27.15%) reduction in the number of primary care attendances. These estimates were consistent with the partially multivariable-adjusted estimates, but these effects largely attenuated after adjusting for all the baseline covariates listed in Table 3.
FIGURE 12.
Effect of prescribing varenicline on (a) weight (kg) and (b) frequency of attendance to primary care in the 4 years following initial prescription. Linear regression is indicated by squares (▪), the solid line is adjusted for basic confounders, the dashed line adjusts for all confounders listed in Table 3 and the instrumental variable results are indicated by triangles (▲). 95% CIs are indicated. Reproduced from Davies et al. 16 © 2018 The Authors. Addiction published by John Wiley & Sons Ltd on behalf of Society for the Study of Addiction. This is an Open Access article distributed in accordance with the terms of the Creative Commons Attribution (CC BY 4.0) license, which permits others to distribute, remix, adapt and build upon this work, for commercial use, provided the original work is properly cited. See: http://creativecommons.org/licenses/by/4.0/. The figure includes minor additions and formatting changes to the original text.
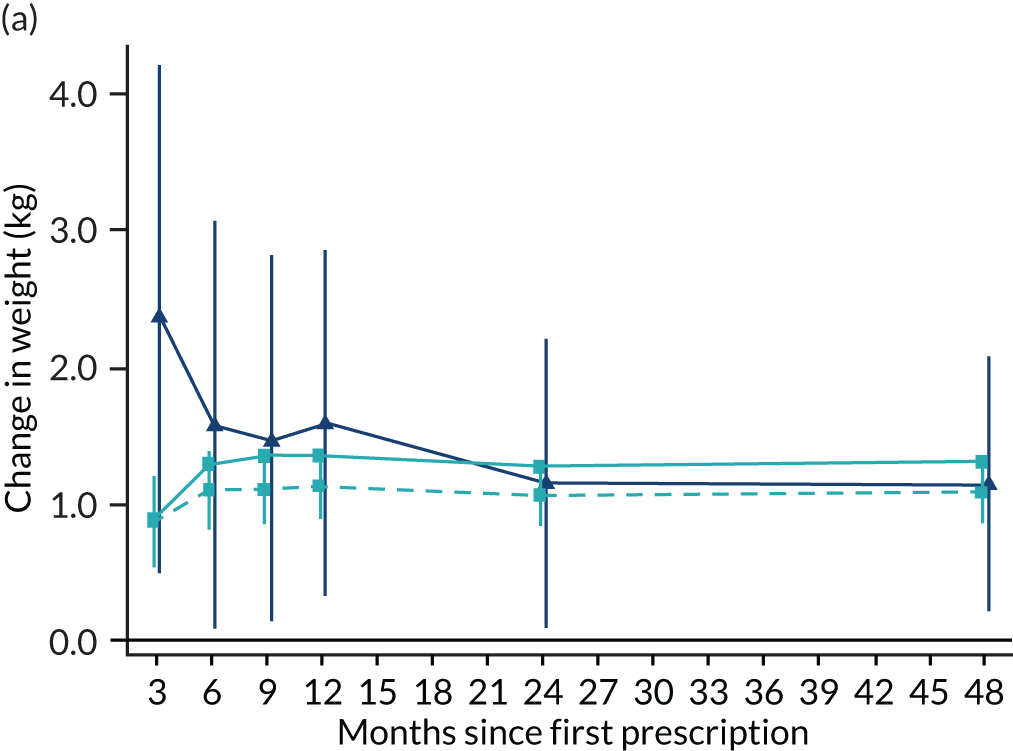
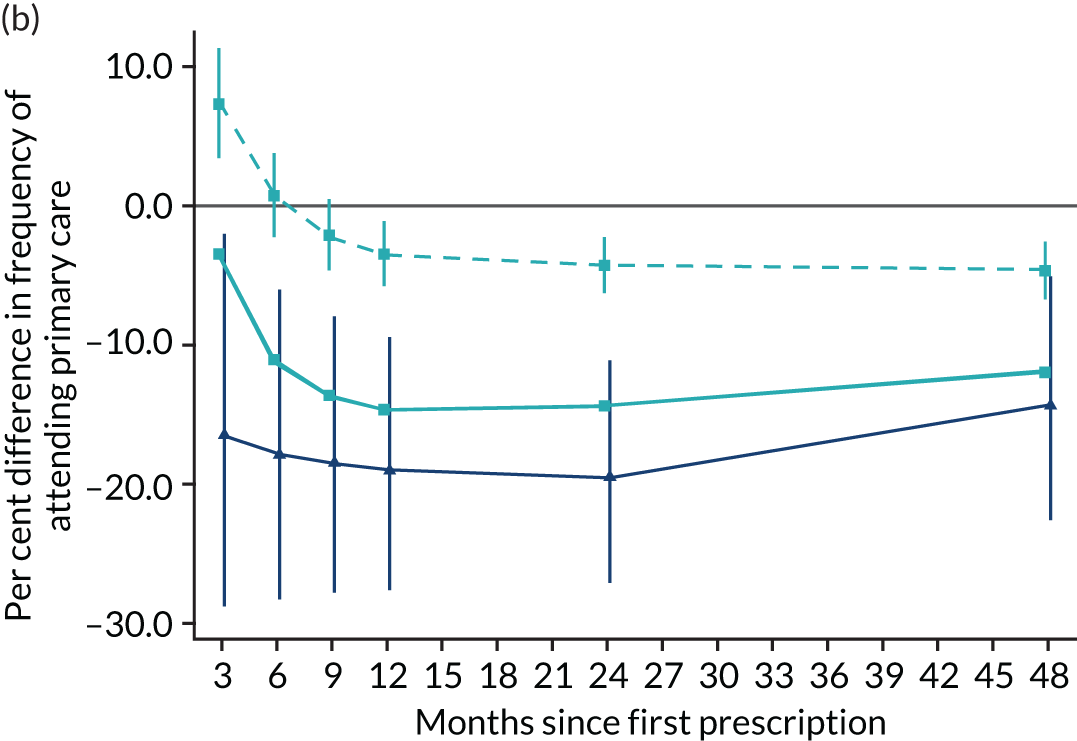
Figure 13 illustrates the effects of varenicline on diabetes-related mortality, diabetes-related hospital admission, myocardial infarction and COPD. For diabetes-related mortality, the instrumental variable analysis provides little evidence of an effect in the year after the first prescription, but shows a rapid increase in risk by 24 and 48 months. The partially and fully adjusted multivariable-adjusted regression results suggest that prescribing varenicline reduces the risk of diabetes-related mortality. There was little evidence from either the instrumental variable or the multivariable-adjusted regression that prescribing varenicline affected likelihood of diabetes-related hospital admission. The instrumental variable point estimates suggested an increased likelihood of primary care diagnosis of myocardial infarction and COPD in months 12–48 after the first prescription for myocardial infarction. However, these effects were imprecise and consistent with the null and the protective effects implied by the multivariable-adjusted estimates. The instrumental variable analysis suggested that varenicline caused an increase in the risk of a diagnosis of COPD after 48 months, but little evidence of an effect at other follow-up periods. The multivariable-adjusted estimates implied a reduction in risk of COPD at all follow-up periods; these differences attenuated after adjustment for baseline confounders. For primary care diagnosis of depression, the instrumental variable analysis provides little evidence of an effect in any period after first prescription. The fully adjusted multivariable-adjusted regression results suggest that prescribing varenicline is associated with a lower risk of depression in the year after the first prescription, but little evidence of differences after ≥ 2 years. For primary care prescription of hypnotics or anxiolytics, the instrumental variable analysis provided little evidence of an effect at any point after the first prescription. The fully adjusted multivariable-adjusted regression analysis suggests that patients prescribed varenicline had a lower risk of being prescribed hypnotics or anxiolytics in the 2 years following the first prescription of smoking cessation therapy, but there was little difference in risk after 4 years.
FIGURE 13.
Effect of prescribing varenicline on diabetes-related (ICD-10 E10–14) (a) mortality and (b) inpatient admission, (c) primary care diagnosis of myocardial infarction, (d) COPD, (e) primary care diagnosis or prescription for depression, and (f) prescription of hypnotics or anxiolytics in the 4 years following initial prescription. Linear regression is indicated by squares (▪), the solid line adjusts for basic confounders, the dashed line adjusts for all confounders listed in Table 1 and the instrumental variable results are indicated by triangles (▲). 95% CIs are indicated. CIs allow for clustering between physicians. The fully multivariable-adjusted results adjust for all the covariates listed in Table 1. Instrumental variable results use seven prior prescriptions. Reproduced from Davies et al. 16 © 2018 The Authors. Addiction published by John Wiley & Sons Ltd on behalf of Society for the Study of Addiction. This is an Open Access article distributed in accordance with the terms of the Creative Commons Attribution (CC BY 4.0) license, which permits others to distribute, remix, adapt and build upon this work, for commercial use, provided the original work is properly cited. See: http://creativecommons.org/licenses/by/4.0/. The figure includes minor additions and formatting changes to the original text.
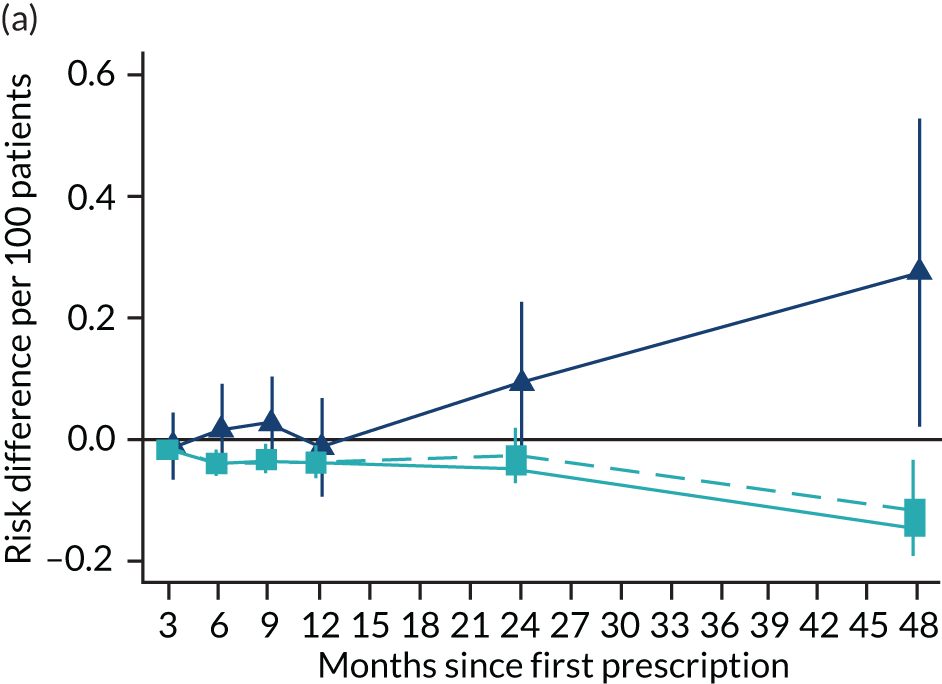
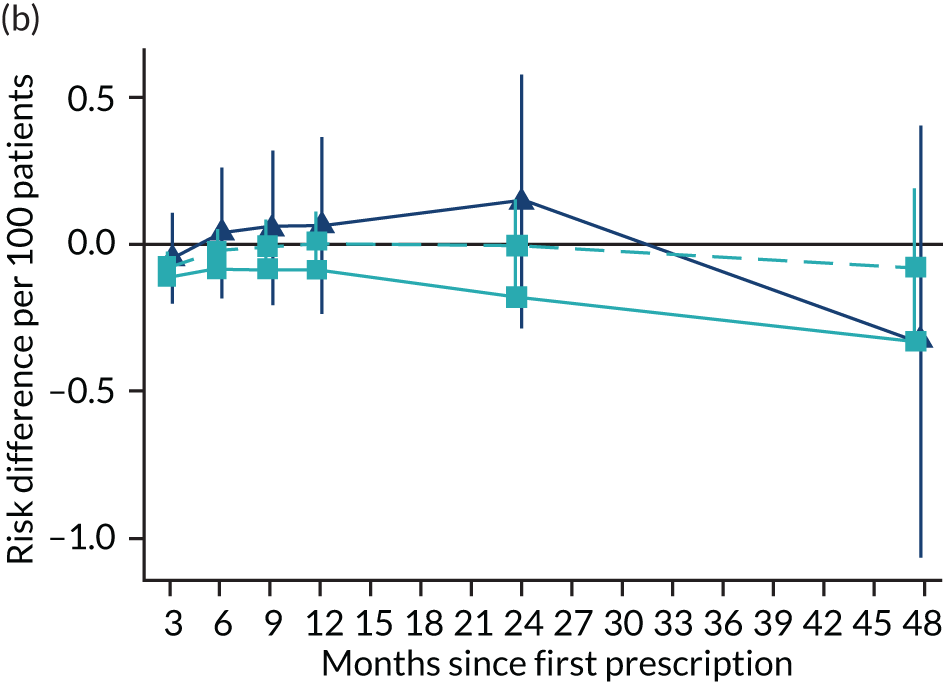
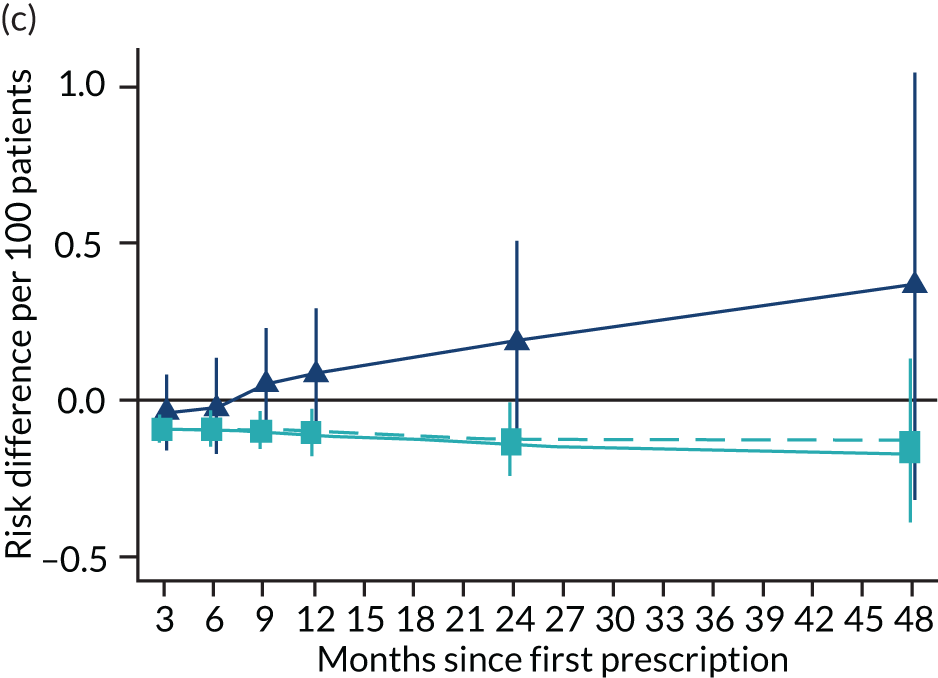
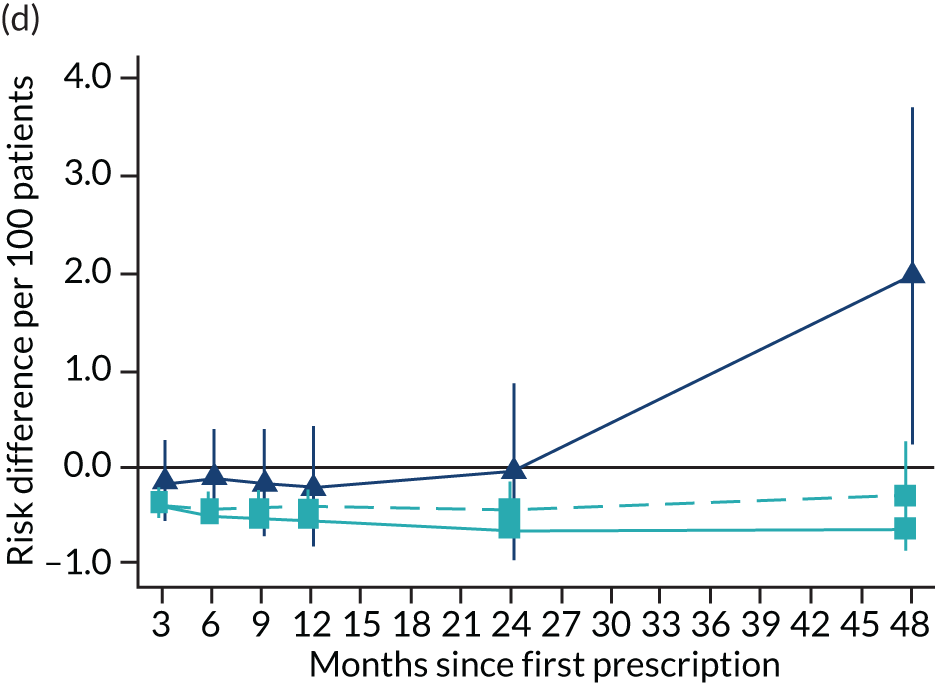
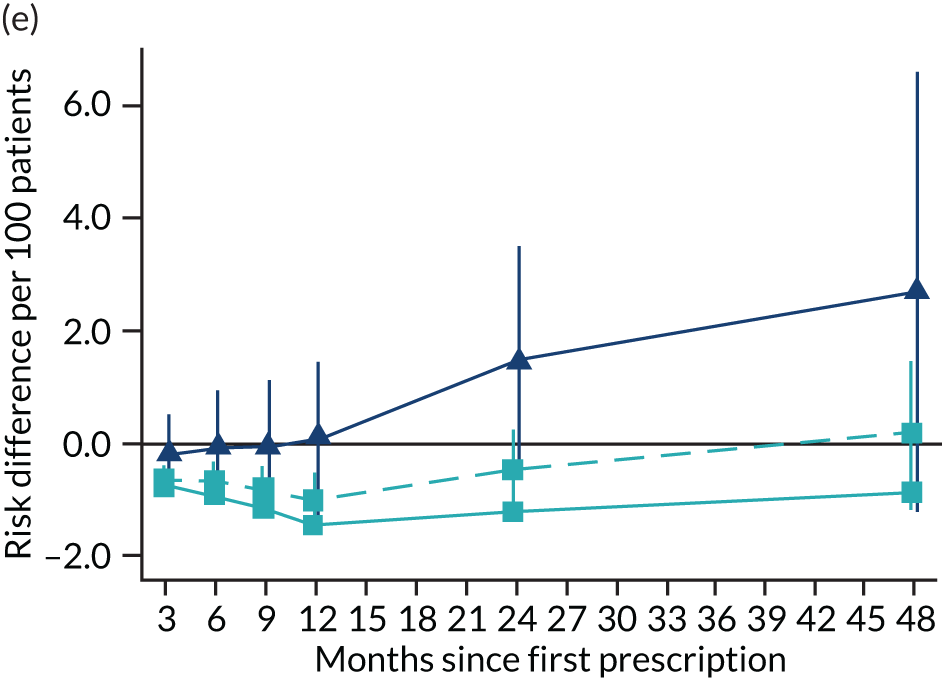
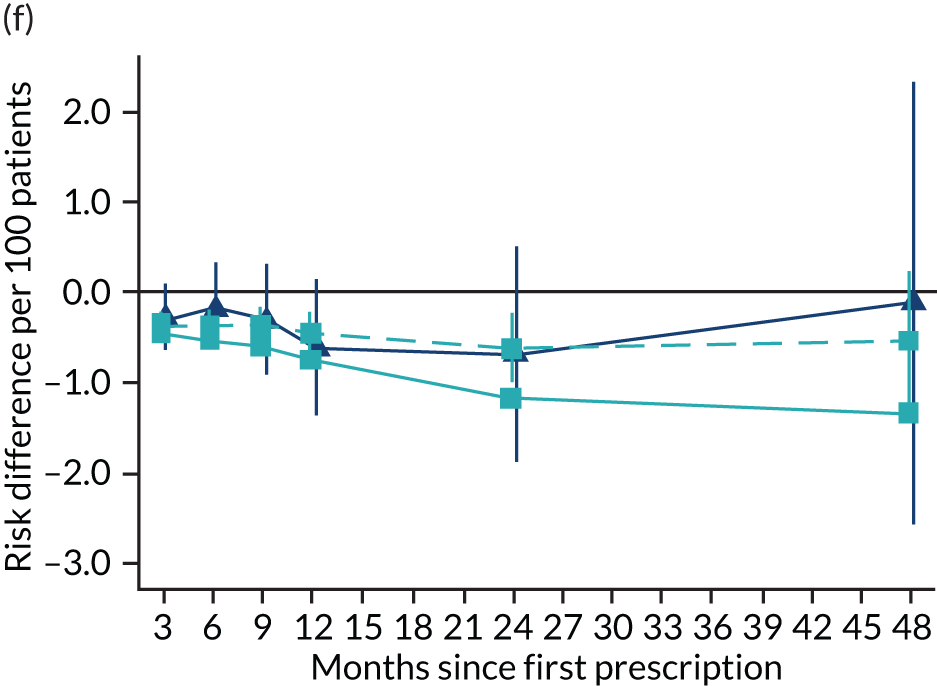
Figure 14 compares the experimental estimates reported in the literature with our estimates from the CPRD. We compared the estimates 1 year after the first prescription for comparability with the trials, which had limited follow-up. Mills et al. 34 conducted a network meta-analysis of data from RCTs. We assumed an incidence rate of 0.2% to convert the risk ratios they report to the risk difference scale. They found little evidence of a difference in risk of major cardiovascular events. However, their estimates were relatively imprecise and consistent with the null. The CATS trial (Cardiac Assessments Following Different Treatments of Smoking Cessation Medications in Subjects With and Without Psychiatric Disorders)33 reported the frequency of major adverse cardiovascular outcome but was less precise than the network meta-analysis above and again consistent with the null. Both estimates are also consistent with our multivariable-adjusted and instrumental variable estimates. We included Mills et al. 34 in this figure because it was a large network meta-analysis on the cardiovascular risk caused by varenicline, and we included the CATS trial in this figure because it was a large RCT published after Mills et al. 34
FIGURE 14.
The effect of varenicline compared with NRT estimated using RCTs and observational studies. The Mills et al. 34 estimate assumes an incidence rate of 0.2% in those allocated to NRT. 34 The CATS trial estimates are taken from reported proportions of major adverse cardiovascular events in the varenicline and NRT arms of the trial. The asymmetric CIs on the Mills et al. 34 result are because we converted the risk ratios to risk differences. Adapted from Davies et al. 16 © 2018 The Authors. Addiction published by John Wiley & Sons Ltd on behalf of Society for the Study of Addiction. This is an Open Access article distributed in accordance with the terms of the Creative Commons Attribution (CC BY 4.0) license, which permits others to distribute, remix, adapt and build upon this work, for commercial use, provided the original work is properly cited. See: http://creativecommons.org/licenses/by/4.0/. The figure includes minor additions and formatting changes to the original text.
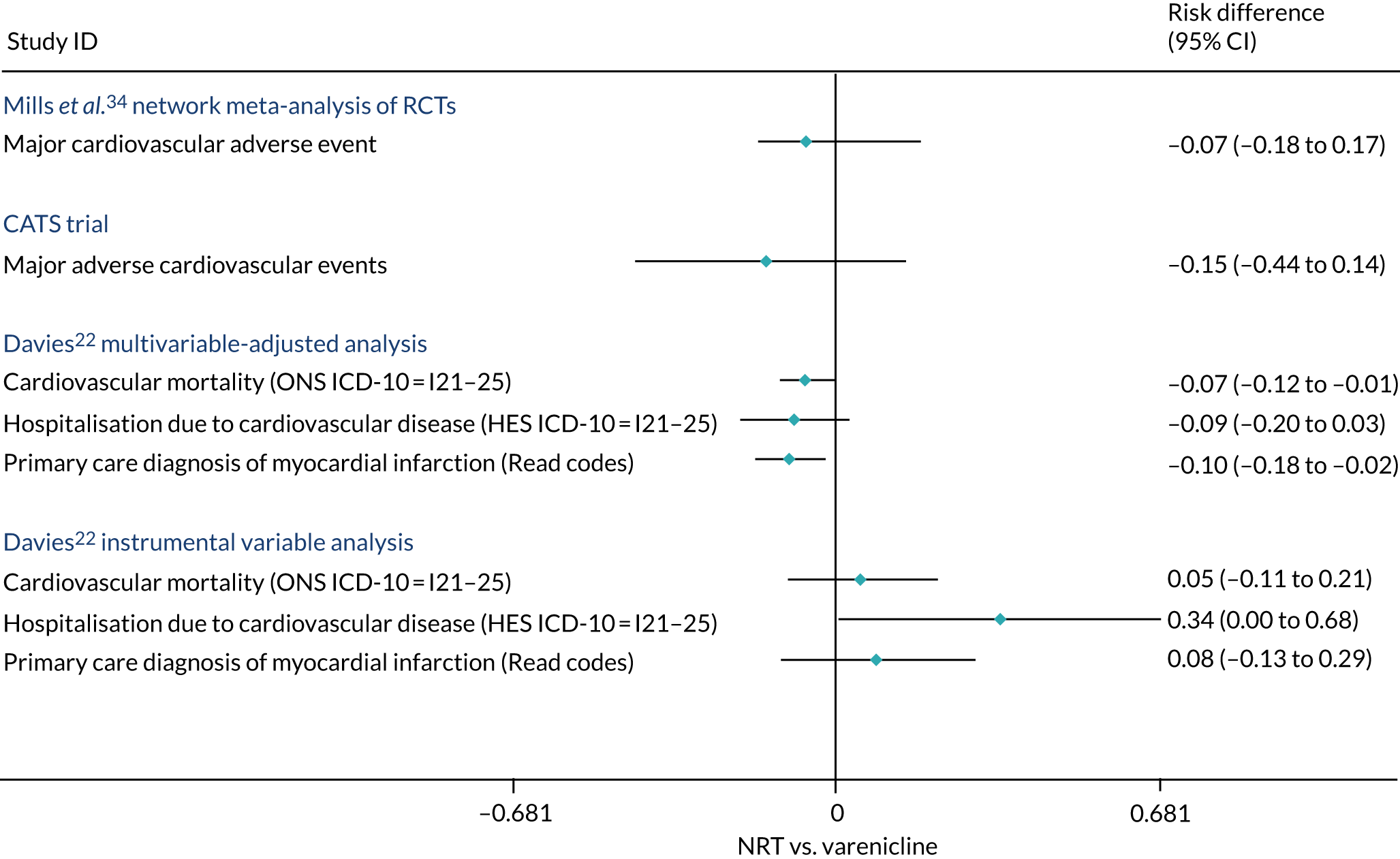
Chapter 5 Prescribing of smoking cessation products in patients recently diagnosed with cancer
There is limited evidence about the prescribing of smoking cessation products to patients recently diagnosed with cancer. In this chapter, we investigate the rate of smoking in patients diagnosed with cancer, the prevalence of prescribing smoking cessation products to patients and the effectiveness of smoking cessation in this population. In the results presented below, we focus on the conventional analysis for two reasons. First, we found little evidence of bias in the effectiveness analysis for the full sample using conventional methods. Second, the sample of patients recently diagnosed with cancer is much smaller than the full sample. Therefore, the statistical power is much lower.
Population characteristics
Of the 220,136 patients prescribed smoking cessation products sampled in the effectiveness analysis in Chapter 3, 2965 had a diagnosis of cancer in the year before their smoking cessation prescription. A smaller proportion of patients diagnosed with cancer (20.9%) were prescribed varenicline than non-cancer patients (32.2%). On average, patients diagnosed with cancer were older than the general population (59 years vs. 46 years), were more likely to be male and had more comorbidities, but were otherwise similar (Table 4).
Characteristic | Any incident cancer (N = 2965) | No incident cancer (N = 217,171) | ||||
---|---|---|---|---|---|---|
NRT (N = 2345) | Varenicline (N = 620) | Total | NRT (N = 147,181) | Varenicline (N = 69,990) | Total | |
Age (years) at time of first prescription, mean (SD) | 59.6 (12.3) | 56.7 (12.2) | 59.02 (12.3) | 46.2 (15.5) | 44.4 (13.2) | 45.6 (14.8) |
Sex (male), n (%) | 1195 (51.0) | 313 (50.5) | 1508 (50.9) | 67,983 (46.2) | 34,831 (49.8) | 102,814 (47.3) |
Multiple deprivation score (IMD), mediana | 12 | 12 | 12 | 12 | 12 | 12 |
Number of GP visits 1 year prior to first prescription, mean (SD) | 11.8 (9.0) | 11.1 (8.6) | 11.7 (8.8) | 7.8 (7.3) | 6.2 (6.0) | 7.3 (7.0) |
BMI (kg/m2), mean (SD)a | 25.8 (5.3) | 26.2 (5.5) | 25.9 (5.4) | 26.5 (5.7) | 26.5 (5.4) | 26.5 (5.6) |
Year of first prescription, median | 2009 | 2010 | 2010 | 2009 | 2010 | 2009 |
Days of history, mean (SD) | 3662.8 (1996.2) | 3323.5 (2012.4) | 3591.8 (2004) | 3150.7 (1889.3) | 3283.5 (1976.3) | 3193.5 (1918.8) |
Comorbidity ever (Charlson Comorbidity Index), n (%) | 2050 (87.4) | 492 (79.4) | 2542 (85.7) | 54,224 (36.8) | 22,031 (31.5) | 76,255 (35.1) |
Alcohol misuse ever, n (%) | 1318 (13.6) | 66 (10.7) | 384 (13.0) | 12,678 (8.6) | 4354 (6.2) | 17,032 (7.8) |
Drug misuse ever, n (%) | 49 (2.1) | 13 (2.1) | 62 (2.1) | 4546 (3.1) | 1344 (1.9) | 5890 (2.7) |
Self-harm ever, n (%) | 218 (9.3) | 52 (8.4) | 270 (9.1) | 15,719 (10.7) | 6128 (8.8) | 21,847 (10.1) |
Ever anxiety and stress-related disorders, n (%) | 663 (28.3) | 156 (25.2) | 819 (27.6) | 41,109 (27.9) | 16,266 (23.2) | 57,375 (26.4) |
Other behavioural/neurological disorder ever, n (%) | 112 (4.8) | 20 (3.2) | 132 (4.5) | 8259 (5.6) | 2751 (3.9) | 11,010 (5.1) |
Ever depression, n (%) | 930 (39.7) | 224 (36.1) | 1154 (38.9) | 60,347 (41.0) | 24,289 (34.7) | 84,636 (39.0) |
Ever antidepressants, n (%) | 1205 (51.4) | 303 (48.9) | 1508 (50.9) | 73.778 (50.1) | 30,152 (43.1) | 103,930 (47.9) |
Ever antipsychotics, n (%) | 501 (21.4) | 97 (15.7) | 598 (20.2) | 27,183 (18.5) | 9255 (13.2) | 36,438 (16.8) |
Ever hypnotics/anxiolytics, n (%) | 1116 (47.6) | 267 (43.1) | 1383 (46.6) | 55,871 (38.0) | 23,535 (33.6) | 79,406 (36.6) |
Ever mood stabilisers, n (%) | 149 (6.4) | 16 (2.6) | 165 (5.6) | 7089 (4.8) | 1706 (2.4) | 8795 (4.1) |
We extracted information on the number of patients in the entire CPRD who were diagnosed with cancer each year. We used this information to calculate the proportion of incident cancer cases who were current smokers. The proportion of patients with incident diagnoses of cancer was steady between 9.9% and 12.1% between 2007 and 2012 (Figure 15). Since 2012, the proportion of these patients who smoke has steadily dropped to 6.5% in 2015. In 2007, patients with a recent diagnosis of cancer were as likely to be prescribed NRT as those without a diagnosis, whereas they were slightly less likely to be prescribed varenicline. Prescribing of NRT fell over the period, but less quickly for those recently diagnosed with cancer (Figure 16). Prescribing of varenicline fell after 2012 for people with and without a recent cancer diagnosis. By 2015, patients with a cancer diagnosis were more likely to be prescribed varenicline than those without a cancer diagnosis.
FIGURE 15.
The proportion of incident cancer patients in primary care who smoke, from 2007 to 2015.
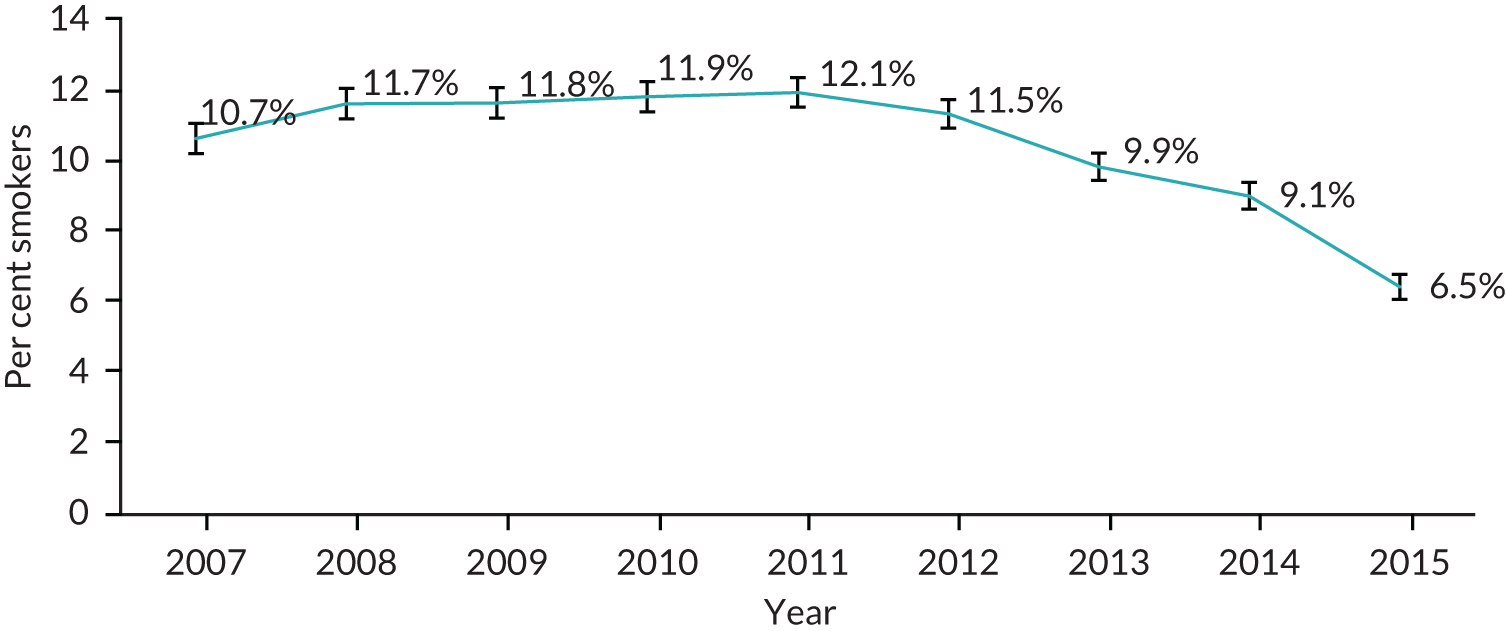
FIGURE 16.
Prescription prevalence of varenicline or NRT in primary care, from 2007 to 2015, in smokers with any incident cancer diagnosis.
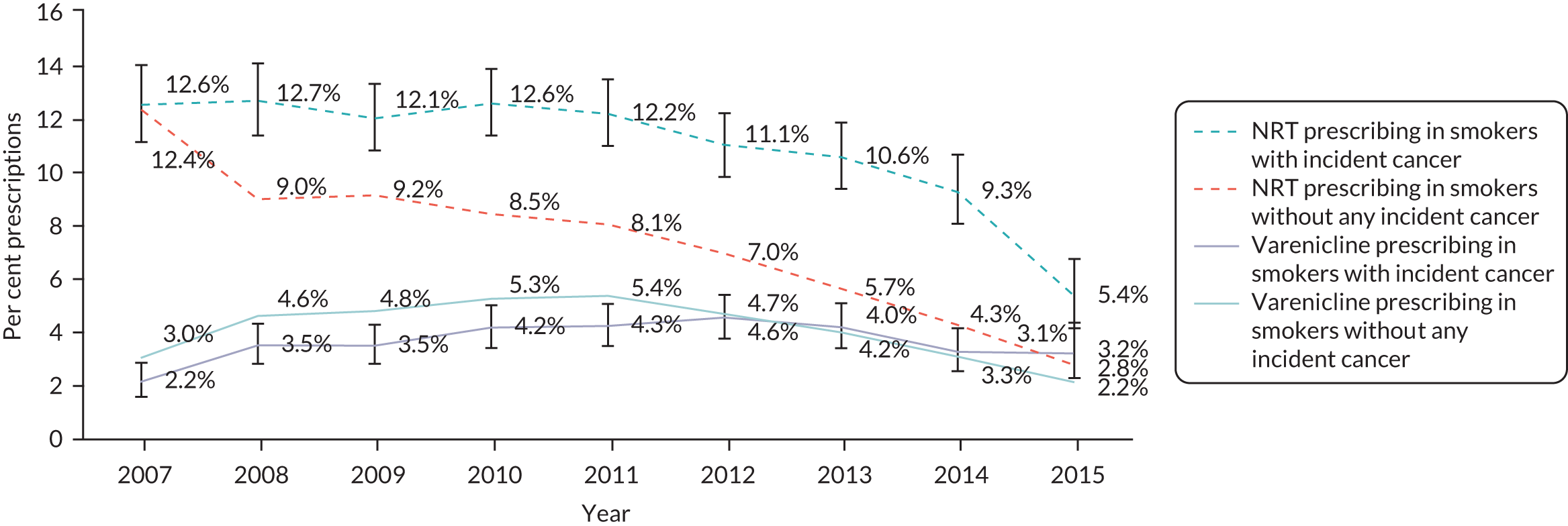
Patients who were diagnosed with lung cancer were substantially more likely to be prescribed NRT than varenicline across the entire period; however, prescribing rates have fallen since 2010 (Figure 17). In contrast, prescribing rates for varenicline in this sample have increased from 2.4% in 2007 to 5.6% in 2015.
FIGURE 17.
Prescription prevalence of varenicline or NRT in primary care, from 2007 to 2015, in smokers with an incident lung cancer diagnosis.
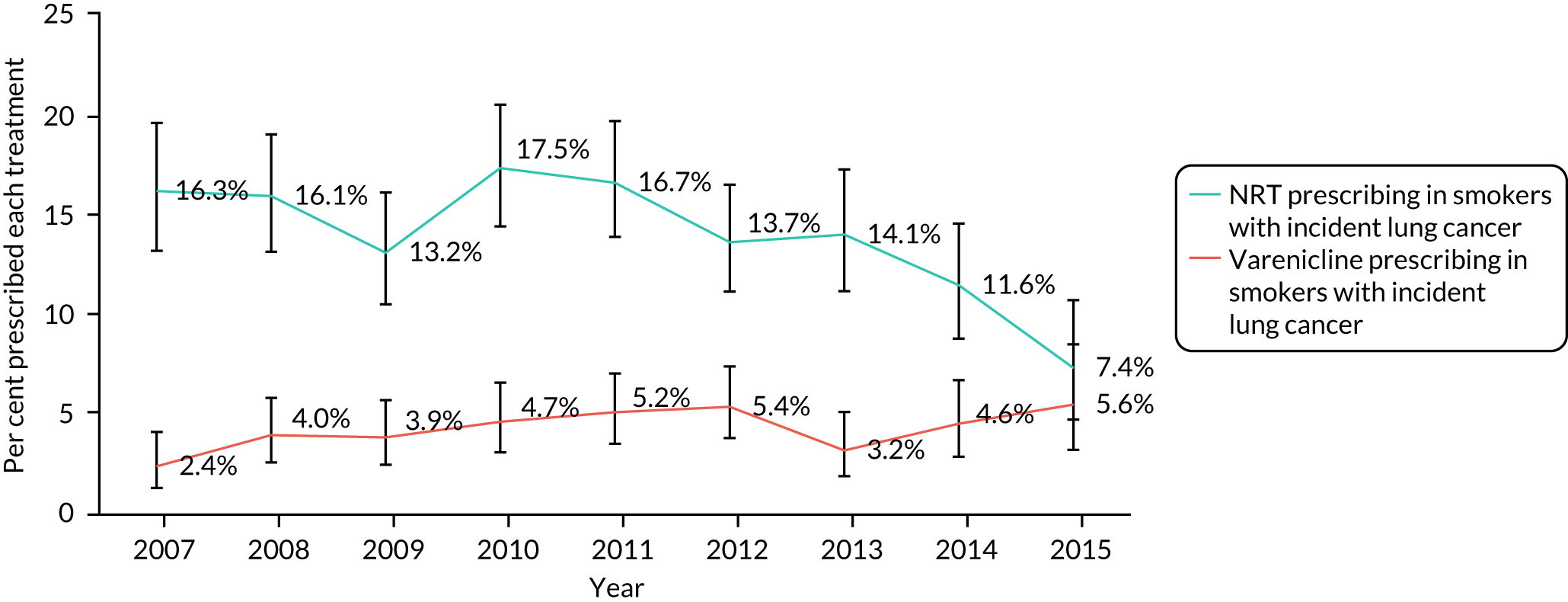
Patients recently diagnosed with cancer were more likely to be abstinent 2 years after their first prescription than patients without a diagnosis (Figure 18). A total of 32.7% and 29.8% of those prescribed varenicline and NRT, respectively, were abstinent 2 years after their first prescription. However, these figures are much more imprecise than the equivalent figures for the whole sample. They are too imprecise to test whether or not there is a difference in effectiveness in this population compared with the whole population.
FIGURE 18.
Percentage of patients with an electronic medical record indicating smoking cessation at 2-year follow-up, by exposure, and incident cancer status.
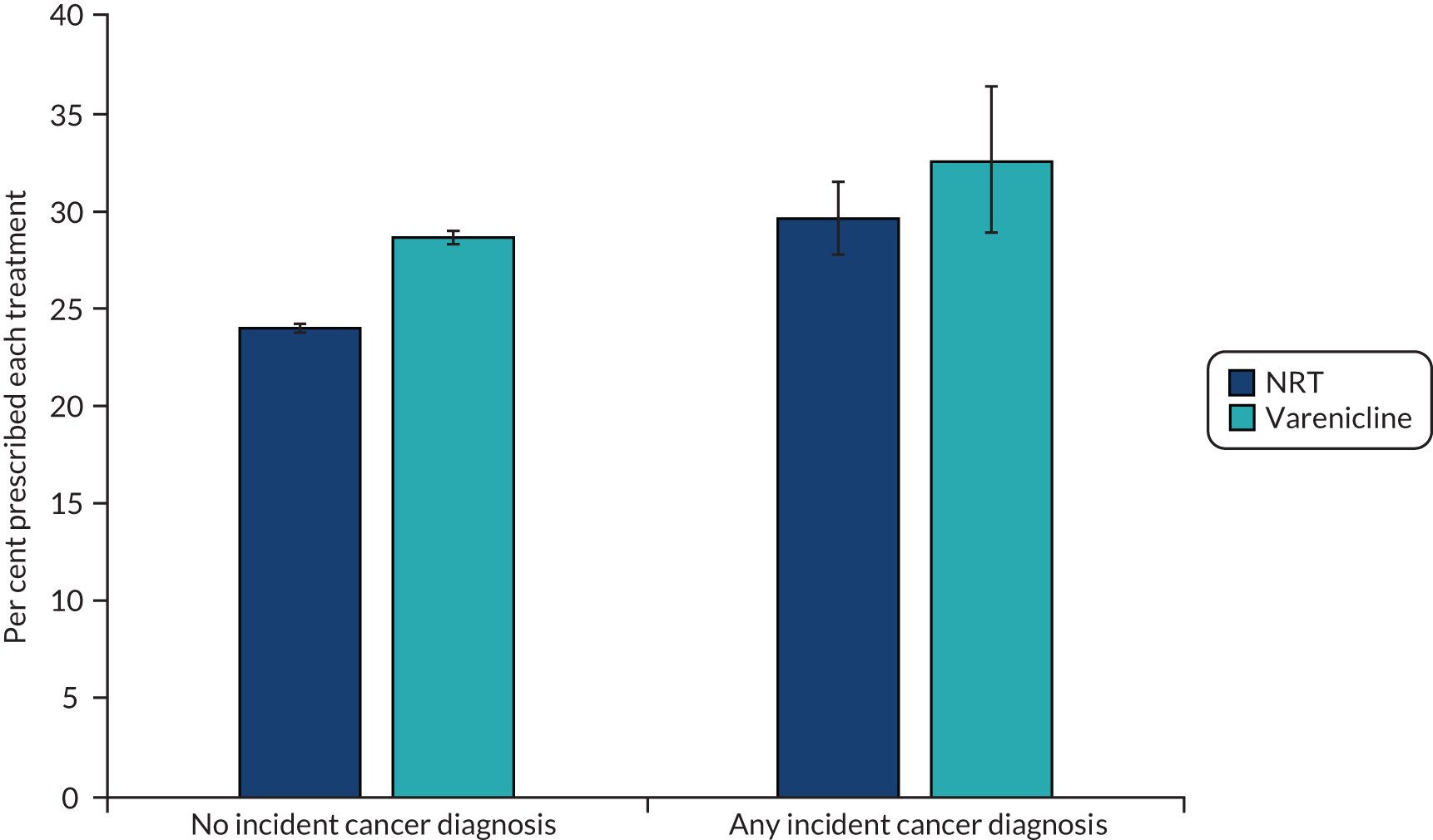
Chapter 6 Discussion
Aims
We aimed to investigate the effect of prescribing varenicline on smoking abstinence, all-cause and cause-specific mortality and hospitalisation, a broad range of health outcomes and whether or not the effects of varenicline differed by SEP.
Summary of the risks and the benefits
Effectiveness
Randomised controlled trials have demonstrated that varenicline is more efficacious than NRT for smoking cessation. Our results demonstrate that varenicline is more effective in the general population when prescribed as part of patient usual care. Our estimates suggested that 4.76 (95% CI 2.77 to 6.74) additional patients would be expected to quit within 2 years per 100 patients prescribed varenicline rather than NRT. These estimates are highly consistent with results from RCTs. 10 There was little evidence of large differences in effectiveness by SEP.
Health outcomes
Patients prescribed varenicline had lower all-cause and cause-specific mortality; however, they were healthier than those prescribed NRT in almost all ways we could measure at baseline. Therefore, the multivariable-adjusted results are likely to suffer from a degree of residual confounding. We addressed this using instrumental variable analysis. Our instrumental variable results found little evidence that varenicline caused large reductions in mortality. Similarly, smokers who were prescribed varenicline had lower rates of all-cause and most types of cause-specific hospital admission than those prescribed NRT. However, our instrumental variable results for hospitalisation were imprecise and consistent with the null and the multivariable-adjusted results. All analytic methods suggested that, on average, patients prescribed varenicline gained around 1 kg in weight and attended primary care less frequently. There was some evidence from the instrumental variable analysis that prescribing varenicline increased the risk of diabetes-related mortality.
Public and patient involvement
Aim
To get feedback on our study from the patients and the public.
Methods
On 30 September 2015, we presented our plans for the research project to the public advisory panel of the Elizabeth Blackwell Institute. The panel members gave feedback about outcomes of most interest to the public and suggested possible avenues for dissemination. On 6 October 2015, we presented our plans for the research project to the UK Centre for Tobacco and Alcohol Studies Smokers Panel in Nottingham. This interactive session involved a group of around 30 smokers, ex-smokers and health-care professionals. We presented details of the outcomes we planned to study, the data we planned to use and the analysis we expected to run. This presentation provided an opportunity to discuss with smokers what they knew about varenicline and where they got information on side effects. The panel was interested in more evidence about the effects of varenicline in everyday clinical care, and possible side effects of varenicline. On 5 June 2016, we presented our findings to the UK Centre for Tobacco and Alcohol Studies panel.
Study results
These presentations had a major impact on how we presented our results in the published papers. In particular, it was clear that it was difficult for panel members to interpret multiple estimates from the same study. Furthermore, providing the results on an intuitive scale helped make the results from the study more accessible. We integrated this feedback into our presentation of the results in the published papers.
Discussion and conclusions
The findings from the patient and public involvement helped inform our study and changed the way we presented results, particularly around risk.
Strengths and limitations
A strength of our study is that we used the CPRD, which includes data from > 4.4 million patients actively registered at general practices across the UK. 15 These patients are likely to be representative of patients prescribed smoking cessation medications in the UK. These patients are also more likely to be representative of the population than participants recruited to RCTs. Furthermore, compliance with treatment regimen is likely to be more representative of real-world compliance seen in primary care. This fact means that our estimates of the effects of prescribing are likely to better reflect the effects of prescribing smoking cessation medications in primary care. A drawback of this approach is that they are likely to underestimate the effect of complying with treatment. The parameter is perhaps of most interest to patients, who have the largest effect on compliance. Physicians, who have less influence over compliance, may be more interested in the effect of issuing prescriptions for varenicline or NRT.
Where possible, we have used validated code lists to define the events used in this study. These lists ensure that the events are well defined and have high sensitivity and specificity for detecting events. For other outcomes, we constructed the code lists by using expert clinical review of search terms and Read terms. We have uploaded the code lists used in this study to a repository for transparency and to enable other researchers to reuse our code lists (https://github.com/nmdavies/varenicline-effectiveness).
The CPRD accurately records smoking status and GPs had financial incentives to record the smoking status of their patients. Furthermore, they were incentivised to repeatedly ask smokers and former smokers about their current smoking status. We used these records to determine patients’ smoking status in the years after their first prescription.
Most of the data were complete. However, there were missing values of IMD and BMI. We addressed this using multiple imputation for the multivariable-adjusted and propensity score regression models. The imputation suggested that missing values of these covariates had little impact on our findings. This similarity is partially because most of our covariate data and all of our prescription data were complete. In a sensitivity analysis, we also investigated whether or not our results were robust to using a multiple imputation model for smoking status after the first prescription. We found little evidence that our results differed when we used multiple imputation.
We retrospectively analysed patients’ electronic medical records. This approach means that we had a substantial follow-up period for each patient, both before they first received a smoking cessation prescription (which we used to define baseline covariates) and after their first prescription (which we used to define outcomes).
A major limitation of observational studies is residual confounding. Confounding can occur if there are baseline differences between patients given different treatments, in this case varenicline and NRT. Patients who were prescribed varenicline were healthier in almost every way that we could measure, although they were more likely to be male. We used multivariable-adjusted regression to control for these differences. This approach assumes that the measured differences account for all differences between patients given one treatment and another. This assumption is strong: that conditional on the measured covariates, the patients were as good as randomised. Although we used a comprehensive set of covariates, these variables are unlikely to have measured all differences between patients prescribed NRT and varenicline. We addressed this limitation using propensity score matching and instrumental variable analysis. The propensity score matching depends on similar assumptions to multivariable regression (i.e. no residual confounding) but can help address imbalance where there are groups of patients who were very unlikely to be given one of the treatments. Instrumental variable analysis can estimate the causal effect in the presence of unmeasured confounding of the treatment outcome association if the instrumental variable assumptions hold. The multivariable-adjusted and instrumental variable estimates for the effectiveness analysis were similar, suggesting that they are unlikely to be due to residual confounding. In contrast, for a number of the safety outcomes, there were substantial differences between the multivariable-adjusted and instrumental variable analysis. These differences in the results may indicate residual confounding.
We drew our sample from the general population of patients who were prescribed smoking cessation medications in primary care. We included all smoking cessation treatments except bupropion. However, very few patients received prescriptions for bupropion so this is unlikely to have caused selection bias in our results. 35 Furthermore, studies have found that bupropion is less effective than other treatments and it is increasingly rarely prescribed. 7
A significant limitation of our study is that we had little information on medication adherence or over-the-counter use of NRT. We did not model treatment adherence or treatment duration. In this study, we were aiming to replicate as closely as possible the intention-to-treat estimate in a RCT of prescribing NRT or varenicline. Treatment adherence decisions are likely to be highly non-random and may be related to the strength of addiction. This issue makes it difficult to estimate the causal effect of adhering to treatment. Our instrumental variable affected prescribing decisions rather than adherence. Varenicline is a prescription-only medicine. Therefore, we are likely to have complete information about the amount of varenicline issued to our sample. However, individuals in our sample are likely to have previously attempted to quit smoking using over-the-counter NRT. This could bias our multivariable-adjusted estimate, for example if individuals prescribed varenicline were more strongly addicted and had previously attempted to quit more frequently. Our instrumental variable estimates could be biased, for example, if individuals who attend physicians preferring varenicline have attempted to quit more frequently.
Finally, we estimated the effect of issuing prescriptions for NRT or varenicline, not the effect of actually taking NRT or varenicline. This estimate is perhaps most relevant for clinicians who have limited influence over treatment adherence. The effect of actually taking the medications is the estimate most of interest to patients. However, we cannot directly address this hypothesis using the models described in this project.
Areas of uncertainty
Our results provide relatively little evidence about the effects of smoking cessation medication on outcomes after 4 years. Our follow-up is much longer than RCTs, which typically follow patients for 1 year. However, varenicline became available only in 2006, so the maximum possible follow-up in our study was around 9 years. The follow-up for the vast majority of patients, who were prescribed varenicline after 2006, is much less than this. Future studies could use (1) a new extract of CPRD data and (2) instrumental variable survival models to make more efficient use of these data. 27
We had limited power to investigate whether or not the effects of these medications differed in subgroups (e.g. older patients, patients with type 2 diabetes or patients diagnosed with cancer). A larger sample of data could provide far more precise estimates of the effects of these drugs. The CPRD covers only around 12% of the population. Therefore, a population sample could potentially provide results that were 2.7 times as precise as the estimates presented in this study. We had limited information about cancer stage and type. The information in the cancer registry could provide valuable evidence about the effects of smoking cessation by cancer type and stage.
However, it is reassuring that large experimental studies and real-world observational studies suggest that varenicline is both efficacious and effective in everyday clinical care. There was little evidence of treatment effect heterogeneity.
We had limited power to detect effects on important clinical outcomes, particularly with the instrumental variable analysis. The multivariable-adjusted analysis suggested that patients prescribed varenicline had better outcomes very shortly after their first prescription. However, the instrumental variable estimates suggested that residual confounding may drive these apparent benefits on clinical outcomes. There is little experimental data on the long-term effects of smoking cessation. Therefore, most evidence about the long- and medium-term effects of smoking cessation comes from observational studies, most of which use methods similar to multivariable-adjusted regression. 1 Our results suggest that multivariable-adjusted regression may suffer from bias. Future studies could increase the precision of the results by using a larger sample, or survival analysis to make more efficient use of the data.
Chapter 7 Conclusions
Implications for service provision
Our results are consistent with large randomised trials and meta-analyses of smaller trials, suggesting that varenicline is the most efficacious and effective method of smoking cessation. 7,10 There may be a need to update clinical guidance in the light of recent evidence.
Suggested research priorities
Trends in smoking cessation prescriptions
More evidence is needed about why prescribing of NRT and varenicline has fallen over the last decade. One explanation may be because of increased use of e-cigarettes and over-the-counter use of NRT. Alternatively, it may be because of changes to the funding and provision of addiction services. Our results suggest that this decline is not due to the general decline in smoking rates because the rate at which smokers receive NRT or varenicline prescriptions has fallen.
Long-term follow-up
Varenicline has only been available in the UK since 2006. This means that we have only a limited observational follow-up, which, in our case, is a maximum of 9 years. Many of the health benefits of smoking cessation are likely to accrue much later in life. It could be informative to prospectively follow up patients treated with varenicline to compare their outcomes with patients treated with NRT for much longer follow-up periods (10–20 years). It would be of methodological interest to compare prospective estimates with retrospective estimates derived from cross-sectional and cohort studies.
Survival analysis with instrumental variables
Recent methodological papers have described estimators for using instrumental variables with survival outcomes. Using survival outcomes could substantially increase the power of the study and could be more informative than the additive structural mean models used in this report. Furthermore, it would be informative to have more information about how these estimators perform on an applied example with a large data set.
Comparing instrumental variable and multivariable-adjusted regression
One of the most significant limitations to observational analyses of electronic medical records is residual confounding. Outside RCTs, patients are rarely treated at random. Researchers are rarely able to measure all indications for treatment, which can result in bias. Instrumental variable analysis can provide an alternative source of evidence based on different assumptions. 36 However, instrumental variable analysis can provide contradictory evidence to multivariable-adjusted regression using the same data. During this project, we developed some tools that can potentially be used to assess the relative plausibility of different methods. 37 These methods use measured covariates to assess the relative bias of instrumental variable and linear regression estimates using intuitive graphic plots. However, more research is needed to guide clinicians and regulators about when estimates from different methods should be used and preferred.
Acknowledgements
This work was carried out using the computational facilities of the Advanced Computing Research Centre [www.bris.ac.uk/acrc/ (accessed 13 January 2020)] and the Research Data Storage Facility of the University of Bristol [www.bris.ac.uk/acrc/storage/ (accessed 13 January 2020)].
A Cancer Research UK programme grant [C18281/A19169] (the Integrative Cancer Epidemiology Programme) supports RMM. A Norwegian Research Council Grant number 295989 supports NND.
Funding
The Medical Research Council (MRC) and the University of Bristol support the MRC Integrative Epidemiology Unit (MC_UU_12013/6, MC_UU_12013/9). The MRC (MR/N01006X/1) and National Institute for Health Research (NIHR) Health Technology Assessment programme funded the research described in this paper. Amy E Taylor, Marcus R Munafò and Gemma MJ Taylor are members of the UK Centre for Tobacco and Alcohol Studies, a UK Clinical Research Collaboration Public Health Research: Centre of Excellence. Funding from the British Heart Foundation, Cancer Research UK, Economic and Social Research Council, MRC and NIHR, under the auspices of the UK Clinical Research Collaboration, is gratefully acknowledged. A Cancer Research UK programme grant (C18281/A19169) (the Integrative Cancer Epidemiology Programme) supports Richard M Martin. No funding body has influenced data collection, analysis or its interpretations. This study is based in part on data from the CPRD obtained under licence from the UK Medicines and Healthcare products Regulatory Agency. The funders had no role in the design and conduct of the study; collection, management, analysis and interpretation of the data; or preparation, review or approval of the manuscript for submission.
The Nottingham UK Centre for Tobacco and Alcohol Studies Smokers’ Panel, Elizabeth Blackwell Patient and Public Advisory Group, Professor Ann McNeill, Dr Lisa Szatkowski, and the Study Steering Committee, Professor Robert West, Dr Rhian Daniel and Dr Emily Herrett. Dr Gemma Taylor and Dr Neil Davies had full access to all of the data in the study and take responsibility for the integrity of the data and the accuracy of the data analysis.
Contributions of authors
Dr Neil M Davies (https://orcid.org/0000-0002-2460-0508) contributed to study conceptualisation, study design, data cleaning, data analysis, interpretation of results and the writing of the manuscript. He also had full access to all of the data in the study and takes responsibility for the integrity of the data and the accuracy of the data analysis.
Dr Amy E Taylor (https://orcid.org/0000-0003-1853-0563) and Dr Kyla H Thomas (https://orcid.org/0000-0001-5418-4034) contributed to study conceptualisation, study design, interpretation of results, data cleaning and the writing of the manuscript.
Dr Gemma MJ Taylor (https://orcid.org/0000-0003-2185-0162) contributed to study design, data cleaning, data analysis, interpretation of results and the writing of the manuscript.
Dr Taha Itani (https://orcid.org/0000-0002-8973-1867) conducted analysis and contributed to the writing of the manuscript.
Dr Tim Jones (https://orcid.org/0000-0002-1199-8668) extracted the data and contributed to the writing of the manuscript.
Professor Richard M Martin (https://orcid.org/0000-0002-7992-7719), Professor Marcus R Munafò (https://orcid.org/0000-0002-4049-993X) and Professor Frank Windmeijer (https://orcid.org/0000-0002-4232-2783) contributed to study conceptualisation, study design, interpretation of results and writing of the manuscript.
This publication is the work of the authors, who serve as guarantors for the contents of this paper.
Publications
Davies NM, Taylor G, Taylor AE, Thomas KH, Windmeijer F, Martin RM, Munafò MR. What are the effects of varenicline compared with nicotine replacement therapy on long-term smoking cessation and clinically important outcomes? Protocol for a prospective cohort study. BMJ Open 2015;5:e009665.
Taylor GMJ, Taylor AE, Thomas KH, Jones T, Martin RM, Munafò MR, et al. The effectiveness of varenicline versus nicotine replacement therapy on long-term smoking cessation in primary care: a prospective cohort study of electronic medical records. Int J Epidemiol 2017;46:1948–57.
Davies NM, Thomas KH, Taylor AE, Taylor GMJ, Martin RM, Munafò MR, Windmeijer F. How to compare instrumental variable and conventional regression analyses using negative controls and bias plots. Int J Epidemiol 2017;46:2067–77.
Davies NM, Taylor GMJ, Taylor AE, Jones T, Martin RM, Munafò MR, et al. The effects of prescribing varenicline on two-year health outcomes: an observational cohort study using electronic medical records: varenicline and 2-year health outcomes. Addiction 2018;113:1105–16.
Taylor GMJ, Itani T, Thomas KH, Rai D, Jones T, Windmeijer F, et al. Prescribing prevalence, effectiveness, and mental health safety of smoking cessation medicines in patients with mental disorders. Nicotine Tob Res 2019;ntz072.
Data-sharing statement
This observational cohort study used electronic medical records from the CPRD. Conditions attached to the use of these data means that the authors cannot share them further; however, they can be requested directly from CPRD via kc@cprd.com. The statistical code used to produce these results can be accessed from https://github.com/nmdavies/. Any further queries should be submitted to the corresponding author in the first instance.
Patient data
This work uses data provided by patients and collected by the NHS as part of their care and support. Using patient data is vital to improve health and care for everyone. There is huge potential to make better use of information from people’s patient records, to understand more about disease, develop new treatments, monitor safety, and plan NHS services. Patient data should be kept safe and secure, to protect everyone’s privacy, and it is important that there are safeguards to make sure that it is stored and used responsibly. Everyone should be able to find out about how patient data are used. #datasaveslives You can find out more about the background to this citation here: https://understandingpatientdata.org.uk/data-citation.
Disclaimers
This report presents independent research funded by the National Institute for Health Research (NIHR). The views and opinions expressed by authors in this publication are those of the authors and do not necessarily reflect those of the NHS, the NIHR, NETSCC, the HTA programme or the Department of Health and Social Care. If there are verbatim quotations included in this publication the views and opinions expressed by the interviewees are those of the interviewees and do not necessarily reflect those of the authors, those of the NHS, the NIHR, NETSCC, the HTA programme or the Department of Health and Social Care.
References
- Peto R, Darby S, Deo H, Silcocks P, Whitley E, Doll R. Smoking, smoking cessation, and lung cancer in the UK since 1950: combination of national statistics with two case-control studies. BMJ 2000;321:323-9. https://doi.org/10.1136/bmj.321.7257.323.
- Jha P, Peto R. Global effects of smoking, of quitting, and of taxing tobacco. N Engl J Med 2014;370:60-8. https://doi.org/10.1056/NEJMra1308383.
- Department of Health and Social Care . Smoking Kills: A White Paper on Tobacco 1998. http://webarchive.nationalarchives.gov.uk/+/www.dh.gov.uk/en/Publicationsandstatistics/Publications/PublicationsPolicyAndGuidance/DH_4006684 (accessed 20 June 2014).
- Ratschen E, Britton J, McNeill A. The smoking culture in psychiatry: time for change. Br J Psychiatry 2011;198:6-7. https://doi.org/10.1192/bjp.bp.110.081372.
- Allender S, Balakrishnan R, Scarborough P, Webster P, Rayner M. The burden of smoking-related ill health in the UK. Tob Control 2009;18:262-7. https://doi.org/10.1136/tc.2008.026294.
- Office for National Statistics . Adult Smoking Habits in the UK. n.d. www.ons.gov.uk/peoplepopulationandcommunity/healthandsocialcare/healthandlifeexpectancies/bulletins/adultsmokinghabitsingreatbritain/2016 (accessed 19 July 2017).
- Cahill K, Stevens S, Perera R, Lancaster T. Pharmacological interventions for smoking cessation: an overview and network meta-analysis. Cochrane Database Syst Rev 2013;5. https://doi.org/10.1002/14651858.CD009329.pub2.
- Thomas KH, Martin RM, Davies NM, Metcalfe C, Windmeijer F, Gunnell D. Smoking cessation treatment and risk of depression, suicide, and self harm in the Clinical Practice Research Datalink: prospective cohort study. BMJ 2013;347.
- US FDA . Press Announcements, FDA: Boxed Warning on Serious Mental Health Events to Be Required for Chantix and Zyban n.d. www.fda.gov/NewsEvents/Newsroom/PressAnnouncements/ucm170100.htm (accessed 23 September 2014).
- Anthenelli RM, Benowitz NL, West R, St Aubin L, McRae T, Lawrence D, et al. Neuropsychiatric safety and efficacy of varenicline, bupropion, and nicotine patch in smokers with and without psychiatric disorders (EAGLES): a double-blind, randomised, placebo-controlled clinical trial. Lancet 2016;387:2507-20. https://doi.org/10.1016/S0140-6736(16)30272-0.
- Centers for Disease Control and Prevention . Smoking-attributable mortality, years of potential life lost, and productivity losses – United States, 2000–2004. MMWR Morb Mortal Wkly Rep 2008;57.
- Brown T, Platt S, Amos A. Equity impact of population-level interventions and policies to reduce smoking in adults: a systematic review. Drug Alcohol Depend 2014;138:7-16. https://doi.org/10.1016/j.drugalcdep.2014.03.001.
- Davies NM, Taylor G, Taylor AE, Thomas KH, Windmeijer F, Martin RM, et al. What are the effects of varenicline compared with nicotine replacement therapy on long-term smoking cessation and clinically important outcomes? Protocol for a prospective cohort study. BMJ Open 2015;5. https://doi.org/10.1136/bmjopen-2015-009665.
- Joint Formulary Committee . British National Formulary (online) n.d. www.medicinescomplete.com (accessed January 2020).
- Herrett E, Gallagher AM, Bhaskaran K, Forbes H, Mathur R, van Staa T, et al. Data Resource Profile: Clinical Practice Research Datalink (CPRD). Int J Epidemiol 2015;44:827-36. https://doi.org/10.1093/ije/dyv098.
- Davies NM, Taylor GMJ, Taylor AE, Jones T, Martin RM, Munafò MR, et al. The effects of prescribing varenicline on two-year health outcomes: an observational cohort study using electronic medical records: varenicline and 2-year health outcomes. Addiction 2018;113:1105-16. https://doi.org/10.1111/add.14146.
- Taylor GMJ, Taylor AE, Thomas KH, Jones T, Martin RM, Munafò MR, et al. The effectiveness of varenicline versus nicotine replacement therapy on long-term smoking cessation in primary care: a prospective cohort study of electronic medical records. Int J Epidemiol 2017;46:1948-57. https://doi.org/10.1093/ije/dyx109.
- NICE . Quality and Outcomes Framework Indicators. Standards &Amp; Indicators n.d. www.nice.org.uk/standards-and-indicators/qofindicators?categories=3910&page=1 (accessed 26 April 2018).
- World Health Organization . International Statistical Classification of Diseases and Related Health Problems 2004.
- Hernán MA, Robins JM. Instruments for causal inference: an epidemiologist’s dream?. Epidemiology 2006;17:360-72. https://doi.org/10.1097/01.ede.0000222409.00878.37.
- Brookhart MA, Wang PS, Solomon DH, Schneeweiss S. Evaluating short-term drug effects using a physician-specific prescribing preference as an instrumental variable. Epidemiology 2006;17:268-75. https://doi.org/10.1097/01.ede.0000193606.58671.c5.
- Davies NM. Varenicline Safety 2016. https://github.com/nmdavies/varenicline-safety (accessed January 2020).
- Davies NM. Varenicline Effectiveness 2016. https://github.com/nmdavies/varenicline-effectiveness (accessed January 2020).
- Dregan A, Moller H, Murray-Thomas T, Gulliford MC. Validity of cancer diagnosis in a primary care database compared with linked cancer registrations in England. Population-based cohort study. Cancer Epidemiol 2012;36:425-9. https://doi.org/10.1016/j.canep.2012.05.013.
- OpenPrescribing n.d. https://openprescribing.net/ (accessed 28 March 2018).
- Sterne JA, White IR, Carlin JB, Spratt M, Royston P, Kenward MG, et al. Multiple imputation for missing data in epidemiological and clinical research: potential and pitfalls. BMJ 2009;338. https://doi.org/10.1136/bmj.b2393.
- Martinussen T, Vansteelandt S, Tchetgen Tchetgen EJ, Zucker DM. Instrumental variables estimation of exposure effects on a time-to-event endpoint using structural cumulative survival models. Biometrics 2017;73:1140-9. https://doi.org/10.1111/biom.12699.
- West R, Hajek P, Stead L, Stapleton J. Outcome criteria in smoking cessation trials: proposal for a common standard. Addiction 2005;100:299-303. https://doi.org/10.1111/j.1360-0443.2004.00995.x.
- Baker TB, Piper ME, Stein JH, Smith SS, Bolt DM, Fraser DL, et al. Effects of nicotine patch vs. varenicline vs. combination nicotine replacement therapy on smoking cessation at 26 weeks: a randomized clinical trial. JAMA 2016;315:371-9. https://doi.org/10.1001/jama.2015.19284.
- Aubin H-J, Bobak A, Britton JR, Oncken C, Billing CB, Gong J, et al. Varenicline versus transdermal nicotine patch for smoking cessation: results from a randomised open label trial. Thorax 2008;63:717-24. https://doi.org/10.1136/thx.2007.090647.
- Davies NM, Smith GD, Windmeijer F, Martin RM. Issues in the reporting and conduct of instrumental variable studies: a systematic review. Epidemiology 2013;24:363-9. https://doi.org/10.1097/EDE.0b013e31828abafb.
- Staiger D, Stock J. Instrumental variables regression with weak instruments. Econometrica 1997;65:557-86. https://doi.org/10.2307/2171753.
- ClinicalTrials.gov . Study To Evaluate Cardiac Assessments Following Different Treatments Of Smoking Cessation Medications In Subjects With And Without Psychiatric Disorders. Study Results–ClinicalTrials.Gov n.d. https://clinicaltrials.gov/ct2/show/NCT01574703 (accessed 12 August 2017).
- Mills EJ, Thorlund K, Eapen S, Wu P, Prochaska JJ. Cardiovascular events associated with smoking cessation pharmacotherapies clinical perspective: a network meta-analysis. Circulation 2014;129:28-41. https://doi.org/10.1161/CIRCULATIONAHA.113.003961.
- Swanson SA, Robins JM, Miller M, Hernán MA. Selecting on treatment: a pervasive form of bias in instrumental variable analyses. Am J Epidemiol 2015;181:191-7. https://doi.org/10.1093/aje/kwu284.
- Lawlor DA, Tilling K, Davey Smith G. Triangulation in aetiological epidemiology. Int J Epidemiol 2016;45:1866-86. https://doi.org/10.1093/ije/dyw314.
- Davies NM, Thomas KH, Taylor AE, Taylor GMJ, Martin RM, Munafò MR, et al. How to compare instrumental variable and conventional regression analyses using negative controls and bias plots. Int J Epidemiol 2017;46:2067-77. https://doi.org/10.1093/ije/dyx014.
List of abbreviations
- BMI
- body mass index
- CI
- confidence interval
- COPD
- chronic obstructive pulmonary disease
- CPRD
- Clinical Practice Research Datalink
- FDA
- Food and Drug Administration
- GP
- general practitioner
- ICD-10
- International Statistical Classification of Diseases and Related Health Problems, Tenth Revision
- IMD
- Index of Multiple Deprivation
- MRC
- Medical Research Council
- NIHR
- National Institute for Health Research
- NRT
- nicotine replacement therapy
- ONS
- Office for National Statistics
- OR
- odds ratio
- RCT
- randomised controlled trial
- SEP
- socioeconomic position
Notes
-
Supplementary results
Supplementary material can be found on the NIHR Journals Library report project page (www.journalslibrary.nihr.ac.uk/programmes/hta/144994/#/documentation).
Supplementary material has been provided by the authors to support the report and any files provided at submission will have been seen by peer reviewers, but not extensively reviewed. Any supplementary material provided at a later stage in the process may not have been peer reviewed.