Notes
Article history
The research reported in this issue of the journal was funded by the HTA programme as project number 14/32/01. The contractual start date was in December 2015. The draft report began editorial review in May 2019 and was accepted for publication in December 2019. The authors have been wholly responsible for all data collection, analysis and interpretation, and for writing up their work. The HTA editors and publisher have tried to ensure the accuracy of the authors’ report and would like to thank the reviewers for their constructive comments on the draft document. However, they do not accept liability for damages or losses arising from material published in this report.
Permissions
Copyright statement
© Queen’s Printer and Controller of HMSO 2021. This work was produced by Stock et al. under the terms of a commissioning contract issued by the Secretary of State for Health and Social Care. This issue may be freely reproduced for the purposes of private research and study and extracts (or indeed, the full report) may be included in professional journals provided that suitable acknowledgement is made and the reproduction is not associated with any form of advertising. Applications for commercial reproduction should be addressed to: NIHR Journals Library, National Institute for Health Research, Evaluation, Trials and Studies Coordinating Centre, Alpha House, University of Southampton Science Park, Southampton SO16 7NS, UK.
2021 Queen’s Printer and Controller of HMSO
Chapter 1 Introduction
Preterm birth (before 37 weeks) occurs in 7.1% of pregnancies in the UK (> 50,000 deliveries per annum) and the majority are the result of preterm labour. 1,2 Preterm birth remains the leading cause of neonatal morbidity and mortality,1 but timely interventions for women with preterm labour can improve neonatal outcomes.
Establishing a diagnosis of preterm labour, however, is challenging, and false-positive diagnoses are common. In a large randomised controlled trial (RCT), over 80% of women ‘diagnosed’ on clinical grounds with preterm labour had not given birth at 7 days post diagnosis. 3 Such diagnostic uncertainty means that a large proportion of women with symptoms of preterm labour are treated unnecessarily to ensure that treatment is given to the few women who do actually deliver preterm. Unnecessary interventions result in both a substantial economic burden to health services and potential adverse maternal and neonatal events.
Threatened preterm labour is the most frequently cited indication for maternal transfer, resulting in approximately 4.4 transfers per 1000 maternities according to a Scottish national study. 4 A qualitative study of women who experienced in utero transfer found that hospital admission and transfer had a substantial negative financial and emotional impact on their families. 5 Adverse effects particularly related to care of other children and dependents while the woman was in hospital, travel and accommodation costs for partners and family members near the destination hospital and employment issues for partners and family members. Antenatal steroids are frequently given to women with symptoms of preterm labour, as these decrease neonatal morbidity and mortality if birth occurs between 2 hours and 7 days after administration. 6 However, repeated doses of steroids may increase morbidity. In a recently reported 5-year follow-up trial of repeated doses of corticosteroids for women at risk of preterm birth, a subanalysis of the data suggested that children who had received multiple doses of corticosteroids but were born at term had a higher incidence of neurosensory disability than children in the comparator group who had received a single dose of corticosteroids and were born at term. 7 Maternal magnesium sulphate infusion in the hours immediately prior to birth can lower the risk of cerebral palsy in preterm neonates but is safe within only a narrow dosage range, and overdose can cause respiratory depression and cardiac arrest in the mother. 8 Tocolysis can also have serious adverse effects for both mother and baby. 9
Diagnostic tests for preterm labour are available and used in many units in the UK. 10 The most commonly used type of diagnostic test in the UK is for fetal fibronectin (fFN). This is available in the UK as a bedside test: Rapid fFN® (Hologic, Inc., Marlborough, MA, USA). Fetal fibronectin is a biochemical marker of preterm labour that can be measured in samples of cervicovaginal secretions collected at a speculum examination. An alternative approach (which can be combined with fFN testing) is to measure the cervical length using transvaginal ultrasonography, because the longer the cervix is, the less likely is preterm birth. 11 This approach is more commonly used in mainland Europe and the USA, but relies on specialist equipment and trained staff and is not routinely available throughout the UK. 10
As part of a report funded by the Health Technology Assessment (HTA) programme, Honest et al. 11 found that qualitative fFN (giving a positive or negative result based on a single threshold of 50 ng/ml) was potentially useful in the prediction of preterm birth at < 34 weeks’ gestation, with its main benefit relating to its high negative predictive value (i.e. its ability to rule out impending birth). A more recent review12 funded by the HTA programme found that qualitative fFN has moderate accuracy for predicting preterm birth, with overall sensitivity and specificity estimates of 76.7% and 82.7%, respectively, for birth within 7–10 days. These estimates suggest that qualitative testing on its own would not have the sensitivity to rule out preterm birth adequately; however, in a systematic review of clinical trials, no increase in neonatal morbidity or mortality was seen in association with false-negative fFN results. 12 The authors conclude that this observation is likely to relate to the multifactorial nature of assessment of the risk of preterm birth, whereas, in practice, fFN is just one component of the clinical assessment on which management decisions are based. 12
The current National Institute for Health and Care Excellence (NICE) guideline on preterm labour and birth13 includes recommendations about the management of women with symptoms of preterm labour. The recommendation is to use a test of preterm labour to guide management for women presenting with signs and symptoms of threatened preterm labour at ≥ 30 weeks’ gestation. Although the NICE-recommended test is transvaginal cervical length ultrasonography (its evaluation found this to have the most promising test accuracy), this is not routinely available in the UK and qualitative fFN is an accepted alternative. The NICE guideline13 recommends treatment for all women with threatened preterm labour on clinical assessment at < 30 weeks’ gestation without diagnostic testing, because this was found to be the more cost-effective strategy. However, the quality of evidence for tests of preterm labour was found to be generally low or very low, and further evaluation of tests was a research recommendation. 13
Although both HTA reviews11,12 and the NICE guideline13 evaluated the performance of qualitative fFN (positive or negative results), this test has recently been replaced in the UK with the Rapid fFN 10Q analyser system (Hologic, Inc.). The Rapid fFN 10Q provides a concentration of fFN (quantitative fFN) within 10 minutes and has the potential to be a more useful predictor of preterm birth. 12 However, there is little evidence published to date regarding its use, and recent NICE diagnostics guidance14 concludes that there is insufficient evidence to recommend the routine adoption of quantitative fFN at present. This NICE diagnostics guidance also found insufficient evidence to recommend the routine adoption of two other biochemical tests of preterm labour now available in the UK: Actim® Partus (Medix Biochemica Ab, Espoo, Finland), which measures phosphorylated insulin-like growth factor-binding protein 1 (phIGFBP-1), and PartoSure™ (Parsagen Diagnostics, Inc., Boston, MA, USA), which measures placental alpha microglobulin 1 (PAMG-1). Therefore, NICE guidance did not change and continues to recommend the use of fFN testing based on a single threshold if transvaginal ultrasonography is not available, but the need for further research was acknowledged. 14
The aim of the Quantitative fetal fibronectin to improve decision-making in women with symptoms of preterm birth (QUIDS) study was to determine the best way to use fFN testing for the prediction of preterm birth in women with symptoms of preterm labour in the NHS. We developed a prognostic model for preterm birth within 7 days, which included quantitative fFN and clinical characteristics, and assessed its performance and cost-effectiveness in comparison to other strategies for preterm birth prediction. We then validated the prognostic model and assessed its cost-effectiveness and acceptability in a multicentre prospective cohort study.
The QUIDS study also included two substudies: the Quantitative fetal fibronectin to improve decision-making in women with symptoms of preterm birth qualitative substudy (QUIDS qualitative) and the Quantitative fetal fibronectin to improve decision-making in women with symptoms of preterm birth substudy 2 (QUIDS2). At the outset of the study, we performed a parent and clinician consultation to determine what information parents and clinicians needed to help guide decision-making and the preferred presentation of any decision support (QUIDS qualitative). To enable an exploratory comparison of the prognostic performance of the three biochemical tests of preterm labour available in the UK, a subset of QUIDS participants donated samples for Actim® Partus (Medix Biochemica Ab, Aptoo, Finland) and PartoSure™ (Parsagen Diagnostics, Inc., Boston, MA, USA) testing (QUIDS2) in addition to providing samples for testing (quantitative fFN).
Chapter 2 Aims and conceptual design of the QUIDS study
Parts of this chapter are based on Stock et al. 15 © 2021 Stock et al. This is an open access article distributed under the terms of the Creative Commons Attribution License, which permits unrestricted use, distribution, and reproduction in any medium, provided the original author and source are credited.
Aims and objectives
The primary aim of the QUIDS study is to create an externally validated prognostic model for preterm birth within 7 days in women presenting with signs and symptoms of preterm labour.
Specific objectives relating to this are to:
-
determine the decisional needs of pregnant women with signs and symptoms of preterm labour, their partners and their caregivers (QUIDS qualitative; see Chapter 3)
-
perform an individual participant data (IPD)-level meta-analysis of data from existing efficacy studies of quantitative fFN to develop prognostic models using quantitative fFN and other clinical characteristics (development of the QUIDS prognostic model; see Chapter 4)
-
compare the performance and cost-effectiveness of these prognostic models to determine which have the most potential to be used in an NHS setting (see Chapter 5)
-
externally validate and, if necessary, refine (update) the QUIDS prognostic models using data collected in a prospective cohort study of women presenting with symptoms suggestive of preterm labour in UK hospitals (validation of the QUIDS prognostic model; see Chapter 6)
-
perform an economic evaluation of the QUIDS prognostic model, comparing it with other strategies for prediction of preterm birth, and explore the potential economic implications of using different thresholds of risk (percentage chance of birth within 7 days) predicted by the model to guide management decisions (economic evaluation of the QUIDS prognostic model; see Chapter 7)
-
assess the acceptability of the QUIDS prognostic model to women and clinicians, and to explore the acceptability of fFN testing and its effects on maternal anxiety (acceptability of fFN testing and effects on anxiety; see Chapter 8)
-
perform an exploratory comparison of the performance and cost-effectiveness of the three biochemical tests of preterm labour that are available in the UK: quantitative fFN, Actim Partus and PartoSure (QUIDS2; see Chapter 9)
-
determine an appropriate format to present the prognostic model (presentation of the prognostic model; see Chapter 10).
Health technologies being assessed
The QUIDS study evaluated the biochemical test of preterm labour quantitative fFN. In QUIDS2, we performed an exploratory comparison with the other two biochemical tests of preterm labour available in the UK: Actim Partus and PartoSure. All three tests are designed to be point-of-care tests that clinical staff can easily perform. Test reagents, specimen collection kits and sampling equipment can be stored at room temperature and can be kept in clinical areas where women with symptoms of preterm labour are assessed so that they can be conveniently accessed.
Quantitative fetal fibronectin
The QUIDS study evaluates the Rapid fFN 10Q system, which provides a concentration of fFN (ng/ml or invalid) from a vaginal swab sample within 10 minutes. 16 It is now the only commercially available fFN test system and replaces the TLiQ® system (Hologic, Inc.), which provided a qualitative fFN result (positive or negative) based on a threshold of 50 ng/ml.
Vaginal swab samples are analysed by lateral flow solid-phase immunochromatographic assay [the Rapid fFN Cassette Kit (Hologic, Inc.)] and interpreted in the Rapid fFN 10Q Analyzer (Hologic, Inc.). 16 A total volume of 200 µl of the sample is pipetted into the sample application well of the Rapid fFN cassette using a polypropylene or polyethylene pipette. 16 The sample flows from an absorbent pad across a nitrocellulose membrane via capillary action through a reaction zone containing murine monoclonal anti-fFN antibody conjugated to blue microspheres (conjugate). 16 The conjugate, embedded in the membrane, is mobilised by the flow of the sample. 16 The sample then flows through a zone containing goat polyclonal antihuman fibronectin antibody that captures the fibronectin–conjugate complexes. 16 The remaining sample flows through a zone containing goat polyclonal antimouse immunoglobulin-G antibody that captures unbound conjugate, resulting in a control line. 16 After 10 minutes of reaction time, the intensities of the test line and control line are interpreted with the Rapid fFN 10Q Analyzer and a printed result provided as a concentration in ng/ml (0–500 ng/ml) or as invalid. 16 The result is invalid if the test does not meet internal quality controls that are performed automatically with every test. 16 In the event of an invalid result, the test can be repeated with any remaining clinical specimen. A quality control can be performed by a reusable Rapid fFN 10Q QCette® (Hologic, Inc.), which verifies that the analyser performance is within specification. 16
Actim Partus
The Actim Partus test is a visually interpreted, qualitative immunochromatographic dipstick test that detects the presence of phIGFBP-1 (insulin-like growth factor-binding protein 1) in cervical secretions during pregnancy. 17 It gives a qualitative (positive or negative) result within 5 minutes. The lowest detectable amount of phIGFBP-1 in the extracted sample is approximately 10 µg/l. 17 Samples are taken using the Actim Partus test kit as per the manufacturer’s instructions. 17 The test kit comprises an Actim Partus dipstick in an aluminium foil pouch with desiccant, a sterile polyester swab and a tube of specimen extraction solution (bovine serum albumin, protease inhibitors and preservatives; 0.5 ml). 17 The sample is collected from the cervix using the sterile polyester swab during a speculum examination. 17 The swab should be left in the cervix for 10–15 seconds to allow it to absorb the secretions. 17 The sample is then placed into the provided specimen extraction solution and swirled vigorously for 10 seconds. 17 The swab is then pressed against the wall of the tube to remove any remaining liquid from the swab before it is discarded.
The test involves two monoclonal antibodies for human insulin-like growth factor-binding protein 1 (IGFBP-1):17 one is bound to the blue latex particles (the detecting label) and the other is immobilised on a carrier membrane to catch the complex of antigen and latex-labelled antibody and indicate a positive result. 17 When placed in the sample, the dipstick absorbs the liquid, which starts to flow up the dipstick. If the sample contains phIGFBP-1 it binds to the antibody labelled with latex particles. 17 The particles are then carried by the liquid flow and, if IGFBP-1 is bound to them, they bind to the catching antibody. 17 A blue line (test line) will appear in the result area if the concentration of phIGFBP-1 in the sample exceeds the detection limit of the test. 17 A second blue line (control line) confirms the correct performance of the test. 17 The yellow dip area of the dipstick is placed into the extracted sample and held until the liquid is seen to enter the result area. 17 The dipstick is then removed and placed on a horizontal surface. Test results will be reported as positive, negative or invalid. The presence of two lines (test line and control line) indicates a positive result, however strong the line is. A negative result is shown by only one line (control line); an invalid result is shown by either no lines or the sample line only (i.e. no control line).
PartoSure
The PartoSure test provides a qualitative result (positive or negative) within 5 minutes. It is a rapid, non-instrumented, qualitative immunochromatographic test for the in vitro detection of PAMG-1 in vaginal secretions of pregnant women. 18 The test employs monoclonal antibodies that are sufficiently sensitive to detect 1 ng/ml of PAMG-1. 18 Samples are taken using the PartoSure test kit as per the manufacturer’s instructions. 18 The test kit comprises a PartoSure test strip in a foil pouch with desiccant, a sterile flocked vaginal swab and a plastic vial with solvent solution (0.9% sodium chloride, 0.05% sodium azide and 0.01% triton X-100). 18 The swab is inserted into the vagina (between 5 cm and 7 cm) without speculum and withdrawn after 30 seconds. 18 The sample is then placed into the provided solvent vial and rinsed by rotating it for 30 seconds. 18 The swab is then removed and discarded.
For testing of the sample, the sample flows from an absorbent pad to a nitrocellulose membrane passing through a reactive area containing monoclonal anti-PAMG-1 antibodies conjugated to a gold particle. 18 The antigen–antibody complex flows to the test region where it is immobilised by a second anti-PAMG-1 antibody. 18 This event leads to the appearance of the test line. Unbound antigen–antibody complexes continue to flow along the test strip and are immobilised by a second antibody. 18 This leads to the appearance of the internal control line. The test strip is inserted into the sample and held there until either two lines are present or 5 minutes have elapsed. 18 The strip should then be placed on a horizontal surface to read the results. Test results are reported as positive, negative or invalid. The presence of two lines (test line and control line) indicates a positive result, however strong the line is. 18 A negative result is shown by only one line (control line); an invalid result is shown by either no lines or the sample line only (i.e. no control line). 18
Target population
The target population for the QUIDS study is pregnant women attending hospital with signs and symptoms of preterm labour. In the IPD meta-analysis, signs and symptoms of preterm labour were defined by the authors of contributing studies (see Table 3). In the prospective cohort study, signs and symptoms of preterm labour were described as any or all of back pain, abdominal cramping, abdominal pain, light vaginal bleeding, vaginal pressure, uterine tightenings and contractions (see Chapter 6, Methods).
A note on prognostic models and measures of model performance
We chose to develop a prognostic model in the QUIDS study based on the principle that predicting an outcome is usually poor when based on a single-factor or single-prognostic test, but can be improved when multiple factors are combined in a model. 19 A useful prognostic model will accurately predict an outcome to inform women and caregivers and to allow appropriate decision-making to improve outcomes and quality of care. 20 Prognostic models can also support clinical research into new interventions and allow stratified medicine approaches. 21,22
There are three main phases to creating a useful prognostic model: model development (including internal validation), external validation and investigation of impact in clinical practice. 20 In the QUIDS study, we aimed to develop and externally validate a model, and explore its potential clinical usefulness and cost-effectiveness. Investigating its implementation and impact in clinical practice was beyond the scope of this project but is a future research recommendation.
In the QUIDS study we used the following measures of prognostic model performance to describe and compare models.
Model discrimination
Model discrimination is the ability of a prognostic model to correctly differentiate between those with and those without the outcome of interest. 23 We present this as the c-statistic, which is identical to the area under the receiver operating characteristic curve (AUC). In the context of our prognostic model, it represents the chance that in two women, one with and one without spontaneous preterm birth within 7 days, the predicted risk will be higher for the woman with spontaneous preterm birth within 7 days than for the one without. A c-index of 0.5 represents no discriminative ability, whereas a c-index of 1.0 indicates perfect discrimination. 23
Model calibration
Model calibration is the degree of agreement between the risk predicted by the model and the actual risk observed. 23 For example, if a prognostic mode gives a 5% risk of preterm birth within 7 days, then approximately 5 out of 100 women with this predicted risk should give birth within 7 days. Calibration is less relevant at internal validation because you would expect that any model will give correct predictions for the cohort it is derived from.
We present the calibration slope as a measure of calibration, which is a measure of agreement between observed and predicted risk of the outcome across the full range of predicted values. A value of 1 suggests perfect calibration and a value much lower than 1 suggests overfitting of the model to the data. 23
We present calibration plots as a visual representation of the expected/observed number of events, which summarises the overall calibration of risk predictions from the model in the validation data. 23 The expected/observed number of events provides the ratio of the total number of women expected to have a spontaneous preterm birth within 7 days to the total number of women observed to have spontaneous preterm birth within 7 days, with an ideal value of 1. Values of < 1 indicate that the model is underpredicting the number of events in a population, and values of > 1 indicate that the model is overpredicting the number events in a population. 23 In the calibration plots that we present (see Figures 1–3 and 8–11), individuals are ranked by deciles of predicted probability of spontaneous preterm birth within 7 days and plotted on the x-axis. Observed outcome frequencies are plotted on the y-axis. The 45-degree lines represents perfect calibration. We also refer to ‘calibration in the large’, which represents the intercept of the calibration plot and is, thus, a measure of whether the predictions are systematically too low or too high.
Overall performance
Overall performance is a statistical representation of the distance between the predicted outcome and the actual outcome. 24 We express this using the Nagelkerke R2, which has a range from 0 to 1 and is a measure of how much of the variation is explained by the model. 24 It is not intuitive to interpret but can be used when comparing the performance of different models on the same data, with an aim of maximising the Nagelkerke R2.
Net benefit
Net benefit is a type of decision curve analysis and a measure of the potential clinical value of a prognostic model. 24 In a formal decision analysis, a single optimal decision threshold is calculated from the quantified harms and benefits of the use of a prognostic model. However, defining a single threshold may be difficult at a population level because harms and benefits from treatments may be difficult to quantify. 24 It may also be undesirable because the relative weight of harms and benefits are likely to vary across individuals and health-care settings, and the perception of benefits and harms are likely to be influenced by personal values and experience. For example, from a clinical perspective, the relative harm of incorrectly predicting a woman’s probability of spontaneous preterm birth is greater at earlier gestation than at later gestation (owing to the higher risk of complications of prematurity at early gestations, which are worsened by lack of treatment); thus, a lower-risk threshold may be recommended to indicate hospital admission and antenatal corticosteroid use in women at early gestation than women at late gestation. Alternatively, when admission to hospital is offered solely because there is a risk of preterm birth (which is likely to incur family disruption and personal cost),4 a woman may have a higher risk threshold before accepting transfer if she lives in a geographically remote location with other caring responsibilities than if she lives near to a neonatal unit and has other family support.
Net benefit analysis allows the harms (from ‘missing’ a case of preterm birth) and benefits (from avoiding unnecessary treatment) of the model to be considered across a range of risk thresholds for spontaneous preterm birth. The potential benefits from correct identification of women at low probability of spontaneous preterm birth within 7 days (‘true negatives’) are put on the same standardised scale as potential harms from unnecessary treatments (‘false negatives’) to allow direct comparison, and are presented for a range of risk thresholds. 25 This is akin to receiver operator characteristic (ROC) curves in that the full range of thresholds are included, rather than a single threshold for a sensitivity/specificity pairing. 24
In our analysis, the range of decision thresholds are for ‘ruling out’ treatment and assume that if the model gives a predicted risk at or below a threshold then no treatment will be given, and that above this threshold steps will be taken to ameliorate outcomes of preterm birth should it occur (i.e. standard or usual care). Approaches of ‘treat all’ or ‘treat none’ are presented alongside the model net benefit for comparison.
Chapter 3 QUIDS qualitative: establishing the decisional needs of parents and clinicians
Context
Clinicians and women face challenges in decision-making for preterm labour and birth. To the best of our knowledge, no research to date has focused on the decision-making experiences of women and clinicians during this time. Clinicians are required to make important decisions regarding clinical management of women with symptoms of preterm labour, despite prediction being challenging. 26 For women, qualitative evidence has indicated that they feel a sense of increased responsibility for their babies and themselves during a high-risk pregnancy, for example where threatened preterm labour is concerned. 27 Despite the emotional, social and financial burdens to women and their families associated with inpatient admission and in utero transfer for threatened preterm labour,5,27–29 evidence suggests that women are generally accepting of antenatal interventions to protect their babies. 28 Hence, both groups stand to benefit from a prognostic model that can improve the accuracy of preterm labour prediction.
The aim of this study was to determine the decisional and informational requirements of women and clinicians when considering preterm labour diagnosis and intervention. A secondary objective was to explore the experiences of women and clinicians receiving and providing preterm labour care. Findings were intended to influence the development of the QUIDS prognostic model and the subsequent decision support tool for clinical practice.
Methods
Design
This study adopted a qualitative, interpretive approach, using semistructured interviews and focus groups to explore the decisional requirements and experiences of participants. This enabled a focused investigation of the a priori aim, while encouraging participants to tell their own stories. Service users were involved in the development of the protocol and study resources. The study was carried out in three NHS tertiary referral centres in England and Scotland.
Participants and recruitment
Participants were purposively sampled to cover different personal and professional experiences of preterm labour and birth. Inclusion criteria were pregnant women at high risk of preterm birth or who had experienced threatened preterm labour, and postnatal women who had experienced preterm birth (at < 34 weeks’ gestation). Clinicians with experience of caring for women in preterm labour and making decisions about their care were eligible, including midwives and obstetricians. Exclusion criteria included being aged < 16 years and non-English speaking. Women were identified by staff in the maternity department and clinicians were identified by members of the research team. Verbal and written information was provided and informed, written consent was gained.
Data collection
Data were collected between January and May 2016 via semistructured interviews, using different topic guides for each group. Women were invited to attend a focus group and those unable to attend were interviewed individually, face to face, in a hospital setting or over the telephone. Individual interviews were preferred for clinicians to avoid dominating participant bias or false consensus and to enable flexibility to interview clinicians when they were available. Demographic details were collected prior to the interview. Interviews were audio-recorded and field notes were taken. The focus group was facilitated by two female researchers and all individual interviews were facilitated by one researcher. Researchers were not part of the clinical care team. Recapping and summarising were used to clarify meaning and avoid misinterpretation. Reflexivity and acknowledgement of personal bias were maintained by regular debriefing between researchers and written reflective accounts following interviews.
Data analysis
Data were analysed independently by three researchers using a framework approach. Analysis of women’s and clinicians’ data was conducted separately then brought together. Data were transcribed verbatim then checked for accuracy against the original recordings. Data were anonymised and labelled using a study identification number and, later, a pseudonym. One researcher analysed all of the data using NVivo version 11 (QSR International, Warrington, UK) and a large sample of the data was analysed separately by two researchers. Consensus was reached regarding meaning and the final framework confirmed by discussion.
The framework approach enabled the large number of data to be managed and interpreted within the focused primary and exploratory secondary aims of the study. 30 The approach to analysis was underpinned by the theory that knowledge is constructed by social interchange;31 hence, themes emerged based on participants’ emphasis rather than on the a priori aims. Following verbatim transcription of the interview recordings, the researchers became familiar with the data by reading the transcripts and field notes several times. Recurring characteristics were recognised and related to decisional and informational requirements and emergent themes. The data were coded then mapped into themes and subthemes according to the participants’ emphasis, creating a framework for each group. The frameworks were refined and interpreted based on the original transcripts. At all stages the transcripts were reviewed to ensure that the thematic framework reflected the original context. Having multiple analysts ensured that themes were interpreted directly from the data, thus minimising interpretation bias.
Separate ethics approval was granted for this part of the study by the North-West Liverpool East NHS Research Ethics Committee (reference number 15/NW/0945).
Results
A total of 40 individuals (22 women and 18 clinicians) consented to take part, 19 of whom were unable to commit a time or were uncontactable, and 21 (12 women and nine clinicians; identified throughout using pseudonyms) participated. Among the 12 women, two took part in a small focus group, three had individual face-to-face interviews and seven participated in a telephone interview. Six women were pregnant at the time of the interview and six were postnatal (Table 1). The women were from a range of ethnic groups. Seven women lived locally to the tertiary unit and five had transferred their care. Postcodes indicated that the women represented a range of social and economic backgrounds. Nine clinicians were interviewed over the telephone, comprising seven obstetricians and two midwives. The clinicians covered a range of professional experiences (Table 2).
Study ID (interview type) | Gestational age (weeks) or postnatal | Gravida | Parity | Ethnicity | Obstetric history (mid-trimester loss and/or preterm birth) | Proximity (miles) to tertiary referral unit (transferred or local care) |
---|---|---|---|---|---|---|
Arya (face to face) | 30+5 | 9 | 1 | British Indian | 2 mid-trimester losses at 19 and 20 weeks | 10 (transferred care) |
Beth (telephone) | 24+0 | 2 | 1 | Black African | Preterm birth at 27+2 weeks | 23 (transferred care) |
Clare (face to face) | 12+0 | 2 | 1 | White British | Preterm birth at 29+0 weeks | 16 (transferred care) |
Donna (telephone) | 20+2 | 2 | 0 | White British | Mid-trimester loss at 20+2 weeks | 2 (transferred care) |
Eva (focus group) | 28+6 | 3 | 1 | Bulgarian | Mid-trimester loss at 20 weeks and preterm birth at 23+2 weeks | 9 (local unit) |
Fran (focus group) | 28+0 | 1 | 0 | White British | Threatened preterm labour at 27+6 | 5 (local unit) |
Grace (face to face) | PN | 1 | 1 | Mixed white British and black Caribbean | Preterm birth at 24+4 weeks | Information not provided (local unit) |
Hatti (telephone) | PN | 1 | 1 | Pakistani | Preterm birth at 24+0 weeks | 4 (local unit) |
Isla (telephone) | PN | 4 | 4 | Black British | Preterm birth at 32+4 weeks | 6 (local unit) |
Jenny (telephone) | PN | 3 | 3 | White British | Preterm birth at 25+6 weeks | 30 (transferred care) |
Kara (telephone) | PN | 1 | 1 | White British | Preterm birth at 33+3 weeks | 10 (local unit) |
Lydia (telephone) | PN | 1 | 1 | White British | Preterm birth at 28+1 weeks | 15 (transferred care) |
Study ID | Job title | Experience | Unit type |
---|---|---|---|
Obs1 | Consultant obstetrician | > 3 years post consultant qualification | Tertiary referral centre with NICU; 8500 births (also has experience in working at units with LNU and SCBU) |
Obs2 | Specialist trainee, obstetrics and gynaecology | Year 2 of specialist training | Tertiary referral centre with NICU; 8500 births (also has experience working in a smaller unit with SCBU and has held a research post) |
Obs3 | Specialist trainee, obstetrics and gynaecology | Year 3 of specialist training | Tertiary referral centre with NICU; 8500 births |
MW1 | Midwife | 4 years qualified | Tertiary referral centre with NICU; 8000 births (also has experience working at another large unit with NICU) |
Obs4 | Clinical research fellow (preterm birth) | Specialist trainee year 4 equivalent | Tertiary referral centre with NICU; 8000 births (also has experience in working at a unit with LNU) |
Obs5 | Clinical research fellow (preterm birth) | Specialist trainee year 5 equivalent | Tertiary referral centre with NICU; 8000 births (also has experience in working at two units with LNU) |
MW2 | Midwife | 9 years qualified | Tertiary referral centre with NICU; 68,000 births |
Obs6 | Consultant obstetrician | 2 years post consultant qualification | Unit with LNU; 2800 births |
Obs7 | Consultant obstetrician | 9 years post consultant qualification | Tertiary referral centre with NICU; 6800 births |
Neonatal intensive care units (NICUs) are large intensive care units providing the whole range of medical, and sometimes surgical, neonatal care for their local population and for babies and their families referred from the neonatal network in which they are based (and other networks when necessary). Hence, in utero or ex utero transfer would be considered for resource or capacity reasons only from these units. Special care baby units (SCBUs) provide special care for their own local population; local neonatal units (LNUs) provide special care and high-dependency care and a restricted amount of intensive care. Hence, in utero or ex utero transfer may be considered more frequently from these units.
The themes ‘decision-making’, ‘communication’, ‘accessing care’ and ‘impact’ are explored here. These are presented inductively as they emerged, rather than being aligned to the a priori themes.
Decision-making
Prediction
Women and clinicians felt that predicting preterm birth accurately was essential for decision-making. Women spoke positively about the impact of the fFN test result on their care and how they felt. Clinicians reported using fFN test results confidently for ruling out preterm birth, but valued its contribution less in the presence of a positive result. Clinicians did not use the fFN result to make decisions in isolation; rather, it was used alongside their clinical judgement or ‘gut feeling’ (Obs6 and Obs3). All clinicians and most women agreed that a decision support tool using a prognostic model that improves the accuracy of prediction of preterm birth would be beneficial:
I’m not sure that at present we have a very good tool in being able to worry about the right group of women . . .
Obs7
. . . just knowing what was going to happen would have been a lot more reassuring for me.
Kara
Both groups felt that accurate prediction could inform care management decisions if preterm birth was considered likely.
Clinicians foresaw the benefit of reducing unnecessary clinical interventions:
I think what we’re missing is the overtreatment side of things. I think we can do more harm than good and we can’t lose that, focusing on these women who have high-risk preterm birth, and so I still think there’s a need for a tool that can really stratify out the women that will benefit.
Obs4
In addition, the women spoke of the chance that it would give them to prepare emotionally and practically, including processing the shock of a diagnosis before addressing their informational requirements.
Clinicians and some women felt that having an accurate predictive time scale of < 7 days (either 24 or 48 hours) would be useful. However, some women disagreed, stating that a short time scale of a matter of days would be shocking:
I think a week would be enough. I think if they were going to say ‘your baby’s going to come tomorrow’, you’d just, kind of, go into full-scale panic.
Fran
Clinicians acknowledged the importance of predictive time scales for certain clinical decisions, including timing antenatal corticosteroids, utero transfer, admission and allocation of resources. Clinicians felt that, although accurate prediction at very early gestation seems the most important, predictive time scales should be the same regardless of gestation and the size of and resources in the unit. Clinicians agreed that timescales of 2 weeks, 7 days, 48 hours and 24 hours would aid decision-making. Some women and clinicians could see value in having two predictive time scales: one shorter (24–48 hours) and one longer (1–2 weeks).
Women commented that their confidence in a result that was derived from the decision support tool would be enhanced if they understood how the prognostic model calculated their individual risk of preterm birth. Clinicians generally advocated using the decision support tool in front of women, where possible, to aid their understanding.
There was general agreement between the clinicians that an electronic or web-based format would be preferable for reliability, ease of use, ability to keep it up to date and ability to keep up with contemporary technology. Some clinicians suggested that a mobile application (app) would be preferable because mobile telephones are often at hand and can be accessed quickly. Some women were asked specifically about this format and the majority agreed that this was a good idea. They also commented that apps are familiar to most people, so their use would not seem out of place. Concern was expressed about confidentiality, especially if clinicians were using their personal mobile telephone as the device:
I don’t know how comfortable I would be with my information on someone’s phone, if that makes sense? I just, I don’t know. What if they lose their phone or someone steals it and all the information’s on there?
Kara
Use of this format would necessitate robust reassurances about confidentiality and data protection.
Women’s decision-making
Involvement in making decisions about their care was important to all women; however, they varied in the amount of control that they wanted over decisions. Some women voiced their frustration at not being given the opportunity to make decisions related to their care:
. . . no, it was just up to them. They didn’t even ask whether, what I wanted or, you know, what would I – we were just ‘no, we are going to follow our guidelines’.
Hatti
Conversely, some women were concerned that they would make the wrong decision, the shock of the situation reducing their ability to correctly understand the situation and other family commitments:
But should the decision have been mine? I don’t think so; I think it should be the clinician’s. I think for any number of reasons mums will make decisions that aren’t right.
Arya
Some women indicated that they did not feel that there was a ‘choice’ about care options when preterm birth was anticipated, an opinion that was also voiced by some clinicians. Both groups indicated that, because certain interventions were known to improve neonatal outcomes, there were no other realistic options. Hence, some women indicated that they would always follow the doctor’s recommendation but wished to be kept informed. Women were willing to accept care that they did not want or found scary, such as admission or in utero transfer, if they believed that it could keep their babies safe. An example of this was in women’s description of the discomfort and, in some cases, fear of the speculum examination. One woman (Fran) said that it was more painful than she expected or had been warned. Clinicians should be aware of this and ensure that they prepare women for the discomfort of the examination, especially as it can be carried out with only sterile water for lubrication. However, women still generally accepted having the speculum examination, having balanced the discomfort with the benefit of the information gained about their situation:
. . . being able to have an answer about what’s happening overrides the couple of minutes that it is uncomfortable and a little bit painful.
Fran
Clinicians acknowledged the difficulties in decision-making for women, especially at early gestations and when there is limited time. All clinicians felt that it was imperative that women were fully informed. Although recognising that women want to be involved in decisions, they questioned the extent to which this is possible given the options available. They understood the power of language and aimed to present treatment options in a manner that guides women to the recommended choice. Following many collective years’ clinical experience, clinicians could recall few occasions when women did not follow their recommendations in this context.
Clinicians’ decision-making
Clinicians described the complexity of decision-making, including the need to take account of information from many sources to diagnose preterm birth and manage subsequent care. This included presenting, medical and obstetric history, such as previous preterm birth or mid-trimester loss, and clinical assessment including observation, abdominal palpation and speculum examination findings. For some clinicians this also included cervical length measurement. Clinicians demonstrated reflexivity in decision-making, citing experiences that have shaped their practice. Decision-making was more complex at early gestations or when test results clashed with their clinical assessment, resulting in some junior clinicians feeling underconfident. In these scenarios junior clinicians valued the input of experienced, senior colleagues. Many clinicians were concerned about overtreatment but in general felt that this was less of a risk than undertreatment. This belief was exemplified by clinicians’ preference to ‘play it safe’ (Obs3) and ‘err on the side of caution’ (Obs4).
Communication
Communication between women and care providers permeated all narratives, emphasising its importance. Positive or negative experiences of communication appeared to influence women’s overall judgement of care.
Women valued the communication of information, particularly because they felt that they had little knowledge of preterm birth or mid-trimester loss prior to their experiences. Women listed numerous informational requirements. Clinicians recognised the challenge of providing the vast amount of complex information required at such a sensitive time.
Some women reported discovering that the information provided to them during their experiences was incomplete; this damaged the trust they held in caregivers. Woman wanted honesty even when the information was negative, such as a poor prognosis:
. . . nobody was actually saying to me that you’re dilated, the likelihood is your baby is going to be born soon and she is not going to live – which sounds brutal but that’s what a woman needs to know.
Donna
Despite this, women wanted clinicians to deliver information to them sensitively, balancing honesty with empathy.
Clinicians listed some terms that they do not use, which broadly corresponded with terms that the women highlighted as upsetting. There were not many terms that the women objected to, but where they did object they did so strongly owing to the upset and distress caused. These terms included ‘fetus’, ‘miscarriage’, ‘viable’ and ‘abortion’:
I didn’t want to hear that word [‘miscarriage’], you know, at the end of the day I know I wasn’t really far gone, I was only 5 months, but I was still 5 months pregnant, frightened and . . . You know, it was just not a word that I wanted to hear.
Hatti
These terms should not be used in the decision support tool, and clinicians should be cautious in using them in their discussions with women. Indeed, using the words the women themselves use is optimal, as they are less likely to be upsetting for the women.
The experiences of women indicate that technical jargon should be avoided in the decision support tool, or fully explained if there is no alternative. Clinicians reported being mindful of this when communicating with women and families and cited useful techniques to enhance women’s understanding, for example relating care to previous experiences:
I ask them if they’ve ever had a smear test done before – if they have, I say, ‘it will feel a bit like having a smear test done’.
Obs1
The women and clinicians agreed that verbal communication was the most appropriate for information and results provision, so that the discussion could be individualised and for the checking of understanding. However, both women and clinicians felt that written or interactive forms of communication were also helpful to enable women to revisit what they had been told, especially complex concepts such as preterm birth risk:
I sometimes think clinicians aren’t maybe very good at putting it simply. I think sometimes patient information leaflets, when people are sent home, thinking about actually how to put it in terms of maybe using words but also using visual aids sometimes can be more helpful.
Obs7
. . . to be told but also to have some information to go back to in case you forgot or you didn’t really understand it. I think it just makes it better.
Beth
The method of communicating results was also discussed and there was general agreement that, although verbal communication was essential, having the ability to print out results for notes and for the women would be valuable. Some element of reassurance was gained from seeing the result:
I mean, it was nice obviously just . . . ‘cause it did say ‘negative’ on the top, so just backed up what she was saying. Not that she would have lied, but . . . yeah.
Fran
One clinician suggested that the results printout could include robust, high-quality information to back up what the clinicians discuss with women verbally. This may include information about the prognostic model, what their level of risk means, recommended care for them, evidence-based prognostic information or signs and symptoms to look out for if they are considered low risk and discharged home. Some women also considered the experience of waiting for fFN results. The idea of using that time to provide women with information about the test and the decision support tool was rated positively. This could be in a written or an electronic/interactive format, and should be used in addition to face-to-face discussions with a clinician.
The decision support tool and any associated resources, such as a patient information leaflet or interactive videos and results printouts, offer an opportunity to provide robust and high-quality information in a format that women and clinicians will understand. Some clinicians provided examples of formats that are effective, such as visual analogue scales or infographics. Any written, interactive or visual information should be presented clearly and simply, without language or imagery that the women might find emotive or upsetting. Such resources should be considered adjuncts to verbal communication with a skilled and knowledgeable clinician.
Accessing and negotiating care
Expectations
Each story was unique, yet all women underpinned them with a description of their expectations of pregnancy. Mostly, those without a prior experience of preterm birth or mid-trimester loss did not know what to expect, or expected ‘normality’. Others described an instinctive feeling that something would go wrong, or specifically that they would not reach fullterm:
Do you know what? It was really weird because I don’t know if I had a hunch all along that something wasn’t quite right.
Jenny
Women with a previous experience were circumspect about their expectations, recognising that pregnancy does not always end with a full-term, healthy baby. For many this meant fear and the need to guard themselves against emotional trauma. They were aware from conception that their pregnancy was ‘high risk’, which meant that waiting for the regular monitoring that often started from 16 weeks was difficult. Some women valued the reassurance that they gained from this, whereas others felt that it was not enough and were disappointed and confused that more preventative treatment was not offered:
It is horrible because 2 weeks doesn’t seem long, but to wait 2 weeks in between appointments it’s, kind of, like I know anything can happen in that time, it doesn’t seem regular enough.
Donna
Seeking and receiving care
Women’s uncertainty about their signs and symptoms heightened anxiety and made the decision to seek care difficult. Even in cases with clear indications, such as vaginal bleeding or fluid loss, some women questioned their instincts. Many women recalled experiencing vague symptoms and struggled to describe how they felt, summarising that ‘something didn’t feel right’ (Arya and Donna). Often these same women recounted their failed attempts to access care after telephoning the maternity unit, because their descriptions had not caused enough concern to warrant a face-to-face review. These women felt dismissed, unwelcome and not listened to:
. . . then when I called them it was like ‘oh, it’s nothing to worry about, you’re 18 weeks, this kind of thing happens. Things are changing, that’s all it is. You’ve probably just weed yourself a little bit’. And I was like . . . no. ‘Yeah, you have.’. Okay then.
Arya
Most women felt able to cope with their experiences when they received regular monitoring, examinations and honest appraisals. Yet, women reported not feeling reassured following a telephone review; this was gained only following a physical check-up.
Women were particularly anxious when they had symptoms but were concerned about wasting clinicians’ time:
. . . the lady looked at my notes, and I could tell she was thinking, ‘oh, she’s here again’. I hadn’t seen this woman before, but like she read my notes . . . And I do think, people always think, I know, that you’re wasting their time. I think that’s why some people don’t bother coming.
Grace
Some women sought care on numerous occasions and felt that clinicians had ignored this ‘big picture’ when making care management decisions. On occasion, concerning symptoms were ‘normalised’ by clinicians, which resulted in women resetting their view of ‘normal’, and subsequently delaying or avoiding care:
Obviously that’s not normal but . . . once you see it like and you think everything is fine and everything medically looks OK, you do start to think ‘well, maybe I’m alright, maybe it is alright’.
Isla
In contrast, women with a prior experience easily accessed care. They were often expressly encouraged to call or attend for advice and reassurance. Interestingly, some still experienced anxiety from the tension between concern for well-being and being a burden:
Nobody ever made me feel like I was being a pain. I felt like I was, but they never made me feel that way.
Clare
Women with a prior history of preterm birth or mid-trimester loss experienced simultaneous and disparate levels of confidence in themselves: low or wavering confidence in their ability to reach fullterm and give birth to a healthy baby, yet high confidence in their ability to recognise signs and symptoms and successfully garner care, which had previously been so difficult. For example:
I have more confidence this year because, but also more fear because I know what I went through and having to go through it again makes me more scared.
Beth
Once women were under the care of doctors and midwives, some reported feeling ‘at their mercy’ (Donna). Having no say in their treatment was disempowering. Some women reported frustration and distress at not being listened to or treated like an individual:
I didn’t feel listened to in [the hospital] and felt very much just like a number and yeah, we have our protocols and our procedures and just need to follow those, and kind of get on with it.
Clare
Some women felt more prepared for preterm birth than others. Preparations that were considered helpful included consultations with the neonatologists about what to expect at their gestation, tours and explanations of the neonatal unit, labour preparation, and managing expectations around potential complications and length of stay.
All women described the speculum examination negatively. Surprise at the level of discomfort was expressed when women had not been accurately prepared for the examination by clinicians:
She said it wouldn’t hurt. She lied. Just because they can’t use anything . . . you know, it’s only water that they can use when they put the speculum in, so that was different. Yeah. A little bit more uncomfortable than what I thought, based on what she’d said.
Fran
However, most women were still willing to consent to the procedure if it was recommended. The only reason cited for refusing consent was concern that the speculum examination would precipitate labour.
Impact
How it feels
The short- and long-term impact of their experience of preterm birth or mid-trimester loss was a strong theme. Shock was experienced by many at the onset of symptoms, diagnosis, birth and seeing their baby for the first time, especially for those whose pregnancies had been ‘normal’. The emotion of their ordeal exacerbated the physical trauma. Universally, women felt that the emotional and psychological impact was the most severe and long lasting, especially for women who lost their babies:
The physical side of it is very traumatic but the aftermath of it is horrible, like, obviously like your mental health . . . nobody should ever lose their child.
Donna
Mourning their loss and the desire to have a baby took over some women’s lives. Other women explained how their traumatic preterm birth experience will prevent them from planning another baby.
For those who were pregnant again, their previous experience affected their current pregnancy. Worry, anxiety and the need for constant reassurance pervaded:
Yes, yes. Oh, my goodness, yes. I am very worried. I think about it all the time.
Beth
One woman demonstrated her hypervigilance by explaining that she ‘looks for everything’ (Eva). Coping strategies included living 1 day at a time, not looking too far into the future and focusing on the additional monitoring that was planned. Many women talked about reaching different milestones of pregnancy, including the gestation of their previous preterm birth or mid-trimester loss and other gestations that they associated with different outcomes for their babies:
I’m like, ‘oh, OK there you go’, I have passed 24 weeks, now I have to just get to 25 then 26 and it’s like I’m counting down to when [baby] was born and I’m telling myself at least if I pass when [baby] was born then at least that is going to be better.
Beth
Impact of care
Individual clinicians influenced how women felt about their experience. The women valued clinicians who were caring, friendly, conscientious and open to building a relationship, because this made them feel comfortable and relaxed:
And more open as well, because you go through an experience together, even though it’s that person’s job. If they like, you can build a relationship faster with someone, because I built one with that woman. And like I see her and say hello to her and stuff. Like that’s something built from just a few hours. So it can be done.
Grace
Women who trusted their clinicians also appeared to have more trust in their care plans and treatment. Those who spoke positively about their experiences expressed confidence that they would be listened to by clinicians and that the right recommendations would be made:
I’m just grateful that I am here and I’m getting the care I’m getting. And that I know I have complete confidence that if something happens they’re going to take care of me.
Arya
When women had confidence in their clinician they also spoke positively about the entire hospital. Elements of care considered positive included cohesion between care teams, continuity of carers, following agreed care plans and regular monitoring and attention. Negative aspects included changing or disregarding previous care plans without explanation and lack of continuity. When women had a negative experience in a particular hospital they reported feeling anxious about receiving care there again. Women’s overall perception of their experience seemed as closely linked to their judgement of the care they received as the outcome for them and their baby.
Discussion
In the context of informing a decision support tool, this study aimed to explore the decisional and informational requirements of women and clinicians in relation to preterm labour and their experiences. Considerations were highlighted regarding the content and format of the decision support tool. Findings supported the primary end point of the prognostic model being birth within 7 days. Furthermore, the test on which the prognostic mode is based (fFN test) was considered acceptable to women and clinicians, despite the discomfort and anxiety related to the speculum examination. A web-based format was desired, but was dependent on sufficient safeguards relating to data protection and confidentiality. Finally, synergistic benefits were considered, including incorporating high-quality patient information into results printouts.
Decision-making was a main theme for both groups. Women and clinicians felt that decision-making in preterm labour was dependent on accurate prediction, and the ability to predict more accurately was welcomed. Clinicians were concerned about avoiding either overtreatment or undertreatment, but accepted the need to overtreat to prevent poor outcomes. The women in this study wanted to be involved in the decision-making processes relating to their care, which reflects the findings of previous research. 32 The women were knowledgeable about preterm birth and reported active involvement during their experiences. However, involvement in decision-making related to their care did not always mean wanting control over decisions. Prior in-depth qualitative research exploring how women are involved in decision-making during a high-risk pregnancy found the same variance in women’s desired level of control. 27 One decision-making factor that was universal among the women was that they all made or accepted decisions that aimed to optimise their baby’s well-being. Exploring the women’s stories as a whole indicated that those who trusted their clinicians to keep their baby safe tended to be satisfied with accepting advice, whereas those who did not lamented not having more control. Where women received the level of control they desired they tended to be more positive about their experiences, a finding that is consistent with prior research. 27
Communication was generally verbal, and encompassed information provision and the development of relationships between women and clinicians. The way clinicians communicated shaped their practice and influenced women’s perceptions of their experiences. Experiences were negative for women when clinicians had not achieved a balance between providing an honest, accurate appraisal of the clinical situation and providing a sensitive and caring approach considering women’s vulnerability and worries. Reflective of previous research, this study found that women found certain terminology distressing. 32 Although the clinicians interviewed in this study were evidently mindful of this, women’s numerous examples of becoming distressed owing to terminology use indicates that some clinicians are not aware of the impact language can have.
The potential for a prognostic model and decision support tool to affect clinical outcomes and NHS resource allocation is dependent on timely use and appropriate decision-making. 5–8 Hence, women with symptoms of preterm birth must seek and access care at the right time. This study found that this can be difficult for women owing to uncertainty about their symptoms, a finding that is reflected elsewhere. 33–35 Symptoms can be vague, yet the women in this study and others have reported that their instinct was that something was ‘not right’. 33–35 However, the vague nature of symptoms meant that women struggled to articulate their concern over the telephone and, hence, did not manage to access care. Women felt anxious and unsure when to call back, because they did not feel reassured following a telephone conversation, only following face-to-face assessment. Anxiety, humiliation and frustration were also reported in other research. 35 More concerning, some women then normalised the symptoms that they felt and delayed seeking care when symptoms persisted. 35
Accessing care, however, was not a concern for women who had a prior experience of preterm birth or mid-trimester loss. They valued feeling welcome to attend for reassurance owing to significant anxiety cause by a previous preterm birth or mid-trimester loss. Women with a prior experience felt confident that they would recognise symptoms but, as in other research, felt the burden of responsibility to access face-to-face care appropriately. 35 This led women to feel hypervigilant and reduced their enjoyment of their pregnancies. 33,36
Women spoke animatedly, providing vivid descriptions when telling their stories, which demonstrated the emotional impact that their experiences had on subsequent pregnancies and family plans. The outcomes for the women in this research varied; however, even when women experienced trauma or loss, some spoke positively about their experience. Their tendency to do this was linked to their perceptions about the care that they received and the trust they had in caregivers.
The strengths of this study include that the participants were encouraged to tell their stories freely. Once participants had told their stories, the interview schedule was used to ask questions specific to the a priori aim related to the decision support tool. The theoretical underpinning of constructionism meant that interpretation of the resultant data was based on the emphasis that participants placed. Hence, topics raised by participants were just as likely to emerge as themes, as the topics defined a priori related to the decision support tool. The findings, therefore, represent what is important to women and clinicians regarding their informational and decisional needs. Therefore, unexpected and original findings emerged that are supported in some way by prior research. The women included in the study had a variety of experiences and clinical histories, which reflects the diversity that clinicians encounter in clinical practice. Clinicians had a variety of career lengths and experiences, reflective of the workforce. Nevertheless, saturation of themes was achieved. Despite viewing birth from a different perspective, clinicians’ and women’s themes reflected one another, indicating an awareness of women’s needs among the clinicians.
Limitations of the study include that the sample was small and self-selected. Women with a strong view of their care, or clinicians with an interest or confidence in preterm birth, may have been more inclined to participate than those who did not. Only two midwives participated, and no neonatologists, general practitioners or commissioners were included. Although the study was designed to understand the requirements of clinicians who make immediate decisions at the point of preterm birth diagnosis, it is acknowledged that the views of these other groups may have added valuable insight. Focus groups and face-to-face and telephone interviews were offered pragmatically to provide choice and flexibility to participants and optimise recruitment. These differences were acknowledged and accounted for during analysis. The trusts involved in recruitment were tertiary referral centres, with one linked district general hospital, which may have restricted the experiences of participants. However, some participants also had experiences in smaller hospitals and were asked about these specifically. Hence, factors specific to smaller units, including automatic in utero transfer below certain gestational ages, were considered. We were unable to recruit partners and no non-English speaking participants were included, which limits transferability of findings to these groups.
Decision-making for preterm labour care is a complex process for women and clinicians. Women wanted involvement in but differing levels of control over their care. Clinicians considered many factors when making decisions, and reported tending to ‘err on the side of caution’ (Obs4) in the case of uncertainty. Hence, the implementation of a prognostic-based decision support tool was positively viewed by participants, welcoming improved accuracy of prediction and decision support. Clearly, the priority of both groups was to improve outcomes for women and their babies. However, elements of care appeared to significantly influence women’s perceptions of their experiences, such as access to care, sensitive and honest communication from clinicians and achieving the desired level of control over decisions.
The QUIDS qualitative provided information to ensure that QUIDS remained relevant and focused on the needs of women and clinicians. This study supported the primary end point of birth within 7 days, which was used in the prognostic model designed and externally validated in the QUIDS study. Women and clinicians have provided insight that will shape the design of a decision support tool using the prognostic model, including a web-based format, to be used in conjunction with clinicians, women and their partners. Decision support development will be according to established guidance to ensure that it is of high quality. Any decision support developed would not replace face-to-face information provision and support but would supplement or enhance it. 37 We envisage that any tool would be implemented into practice with education and training for clinicians, enabling them to judge the validity of the tool and aid communication about the risk of preterm birth with women. Once developed, research will be required to explore the experiences of women and clinicians using the decision support tool in clinical practice. Furthermore, this substudy highlighted the challenge women with symptoms of preterm birth face in accessing care in a timely manner, which is worthy of future research.
Chapter 4 Development and internal validation of the QUIDS prognostic model: individual participant data meta-analysis
Parts of this chapter are based on Stock et al. 15 © 2021 Stock et al. This is an open access article distributed under the terms of the Creative Commons Attribution License, which permits unrestricted use, distribution, and reproduction in any medium, provided the original author and source are credited.
Context
In this chapter, we describe the development and internal validation of the QUIDS prognostic model, which includes quantitative fFN and other clinical characteristics (risk factors or prognostic factors) for the prediction of spontaneous preterm birth within 7 days in women presenting with signs and symptoms of preterm labour. The prognostic model is based on an analysis of IPD from existing prospective cohort studies in which quantitative fFN results and pregnancy outcome details were recorded. A health economic analysis was performed using an early-stage decision model based on the results of the IPD meta-analysis.
Methods
The QUIDS IPD meta-analysis is registered as PROSPERO CRD42015027590. The protocol was developed in accordance with the relevant guidelines for prognostic research, model development and validation38–40 and has been published. 41 The findings are reported in line with the Transparent Reporting of a multivariable prediction model for Individual Prognosis Or Diagnosis (TRIPOD) statement (see Appendix 1). 42
Primary end point
The primary end point, consistent with the findings of QUIDS qualitative (see Chapter 3), was the binary outcome of whether or not spontaneous preterm birth occurred within 7 days of quantitative fFN.
A secondary outcome was the binary outcome of whether or not spontaneous birth occurred within 48 hours of quantitative testing.
Inclusion criteria
We prespecified inclusion of prospective cohort studies or RCTs of women with signs and symptoms of preterm labour (as defined by investigators) that included quantitative fFN results determined by the Rapid fFN 10Q analyser system and pregnancy outcome data if the principal investigator (PI) was in agreement to collaborate and provide full data. 41
Exclusion criteria
We excluded studies in which fFN concentration was measured by an enzyme-linked immunosorbent assay (ELISA) and studies in which IPD were not available for meta-analysis. 41
Search strategy
When applying for funding for this study (April 2014), we completed a literature search for completed and ongoing cohort studies of quantitative fFN using search terms for quantitative fFN and preterm birth. The databases that we searched include MEDLINE, EMBASE, Cochrane Database of Systematic Reviews, Database of Abstracts of Reviews of Effects, HTA database, Cochrane Central Register of Controlled Trials and ClinicalTrials.gov. We also used general search engines, such as Google (Google Inc., Mountain View, CA, USA), and searched references of systematic reviews. We consulted preterm birth researchers and networks, for example Royal College of Obstetricians and Gynaecologists Clinical Study Groups, British Maternal Fetal Medicine Society (BMFMS), Preterm Birth International Collaborative (PREBIC) and the manufacturers of Rapid fFN (Hologic, Inc.) to capture all relevant studies. 41
Study manuscripts and/or protocols were screened by two researchers. We contacted the PIs of all eligible studies and invited them to participate. De-identified data were transferred and stored in a bespoke database on a secure server at the University of Edinburgh.
Data items and sample size
A prespecified set of factors thought to influence the probability of spontaneous preterm birth, as agreed by the experts on the project management group, were requested and considered for inclusion as predictors in the prognostic model. 41
These candidate predictors included fFN concentration (ng/ml), previous spontaneous preterm birth, nulliparity (no previous pregnancy of > 24 weeks), gestational age at fFN test (weeks), maternal age (years), ethnicity, body mass index (BMI) (kg/m2), smoking status, deprivation index, number of uterine contractions in set time period, cervical dilatation (cm), vaginal bleeding, previous cervical treatment for cervical intraepithelial neoplasia (CIN), cervical length (transvaginal cervical length measurement) (mm), singleton or multiple pregnancy and tocolysis. 41
We specified that only variables available in every study would be used for model development, and we planned to further refine the list of potential predictors by ranking them by probable clinical relevance as agreed by consensus in the project management team. 41
In model development, the number of predictor parameters that can be considered is limited by the number of events, with guidance (at the time of our study design) suggesting that at least 10 events are required for each predictor parameter. 43,44 We deemed it sensible to limit predictors for potential inclusion in our model using this rule of thumb. 41
Data cleaning
Study quality was assessed (MB and SJS) using a checklist modified, as recommended by Chang et al.,45 from the quality assessment of diagnostic accuracy studies 2 (QUADAS-2) assessment tool46 (see Appendix 2). Prior to analysis, data were checked for outliers, with nonsensical values removed, and missing data were identified. The characteristics of the population of the eligible studies were summarised using means and standard deviations (SDs), and medians and interquartile ranges (IQRs) for continuous variables, and using counts and percentages for categorical variables. 41 The baseline participants characteristics were summarised first by individual study and second for the entire database. A summary of the number of events, total participants and the median gestational age at birth for each of the studies was also presented to describe the events in the population. The percentage of missing data in the entire database was also presented by each candidate prognostic variable.
Missing data
Under the assumptions of a missing at random (MAR) mechanism, multiple imputation was used to impute missing values of IPD for the predictors included in the final model so as to avoid excluding participants from the analysis. 47 Multiple imputation was performed in R version 3.6.1 (The R Foundation for Statistical Computing, Vienna, Austria) using the ‘mice’ package. 48 Multiple imputation was performed for each original study separately, before the meta-analysis, to recognise clustering of participants within studies and retain any potential heterogeneity across studies. Rubin’s rules were used to combine parameter estimates across the analyses within each set of imputed IPD meta-analysis data sets.
As there was more than one predictor with missing data to be included in the model, multiple imputation by chained equations was used. This approach uses a set of imputation equations, including one for each of the predictors with missing data; all equations include all of the predictors of interest. Missing values for the first predictor are imputed by initially regressing the predictor on all other predictors and the outcome of interest and then drawing from the corresponding posterior predictive distribution of the predictor. 49 The second predictor with missing values is imputed in the same manner, but includes the imputed values of the first predictor in the regression model. The imputation is repeated for all predictors with missing values and this forms one cycle; cycles are repeated to stabilise the results and then the whole process is repeated to create a set of m imputed data sets. We performed 60 imputations, based on the rule of thumb that the number of imputed data sets should equal the largest proportion of incomplete data observed in individual study populations. 49
Model development for the primary outcome
As the outcomes of interest was binary (spontaneous preterm birth within 7 days), a logistic regression modelling framework was used to develop the models. 41 Only predictors from the predefined selection that were available in each study separately were used for inclusion in our prognostic model. 41
We wanted to minimise heterogeneity across studies to ensure that our final model had more robust and generalisable risk predictions across settings and populations. 23 Therefore, we began by assessing the heterogeneity of the predictor effects after full adjustment, using a two-stage approach. We fitted a model with all candidate predictors in each study (i.e. no variable selection) and performed a random effects meta-analysis of each predictor’s adjusted effect separately. Heterogeneity of the predictor effects was quantified using the estimated variance (τ2) and the I2 statistic. Predictors considered to have large heterogeneity in the prognostic effect across studies were removed to ensure that summary beta terms (adjusted log-odds ratios) in the model are meaningful (accurate) for individual populations. 50
For the model development we used a one-stage approach, combining all five data sets with a fixed-effect assumption. Estimation of effects was performed in R using the ‘glm’ function (binomial model). A separate intercept term per study was included in the model to account for clustering and to gauge how predictions may require tailoring to different populations (owing to differences in baseline risk). A backwards selection procedure was used to decide which of the candidate predictor variables should be included in the final prediction model (with a p-value of < 0.1 taken to warrant inclusion and prevent omission of important predictors). For categorical variables, we used the lowest p-value of any category (relative to the reference category) to indicate inclusion or exclusion. The model was refitted after dropping each individual predictor. The models were fitted in each of the imputed data sets and the parameter estimates combined using Rubin’s rules to form the prognostic model equation (see the ‘mice’ package48).
Continuous predictors were analysed on their continuous scale and non-linear associations with the outcome examined. The formulae [(quantitative fFN + 1)/100]0.5 and [(cervical length + 1)/10]0.5 were used to deal with non-linearity and zero values. We used the Multivariable Fractional Polynomial (MFP) package51 in R to identify non-linear terms for continuous variables. The package was applied to each of the multiply imputed data sets individually, with the pattern that optimally predicted the outcome variable in the majority of multiply imputed data sets being the one that was used.
Sensitivity analysis
A prognostic model that included tocolysis as a categorical variable (administered/not administered) was prespecified as a sensitivity analysis to explore any potential treatment effect of tocolysis in delaying birth. Tocolytics are often given to delay birth but have not been shown to delay birth beyond 48 hours. 52
Apparent model performance
After model development, the apparent performance of models was assessed by estimating their performance in the same data used to develop the model (using the means from the pooled imputed data sets). We calculated the overall fit (expressed by Nagelkerke R2) and the observed discrimination and calibration in the data set used to develop the model. The ability of models to discriminate between women with and women without spontaneous preterm birth within 7 days was determined by the AUC, also known as the c-statistic. Calibration was assessed for each tenth of predicted risk by calculating the ratio of predicted (expected) to observed probability of spontaneous preterm birth within 7 days, and visualised using a calibration plot with a non-parametric (locally weighted scatterplot smoothing) calibration curve. We also measured calibration across all participants by calculating the calibration slope and calibration in the large.
Internal validation and adjustment for overfitting
Apparent performance is likely to be optimistic because it is examined using the same data used for model development, for which there is likely to be overfitting. Therefore, internal validation was undertaken using a non-parametric bootstrap resampling technique. 53,54 Each modelling step was repeated in each of the bootstrap samples to obtain a new model based on each bootstrap sample. The apparent performance statistics (e.g. AUC and calibration slope) of each bootstrap model was compared with its performance in the original data set. The ‘optimism’ was the mean difference (across all bootstrap samples) between the apparent value in the bootstrap sample and the observed value in the original data set. This optimism estimate was then subtracted from the original model’s apparent performance to give an optimism-adjusted estimate of each measure of performance for the original model.
To adjust the model for overfitting, the optimism-adjusted calibration slope was used as a uniform shrinkage factor to shrink (penalise) the predictor effects (beta coefficients) of the original model. Then, while holding fixed the shrunken beta coefficients (via an offset term), the study-specific intercept terms were re-estimated to ensure that perfect overall calibration-in-the-large was maintained in each study separately. 50 This produced our final model containing the updated intercepts and the shrunken beta coefficients.
Calculation of net benefit
Net benefit was calculated as the proportion of true negatives minus the proportion of false negatives, weighted by the odds for high risk designation at the selected threshold. 25 We used the package ‘rmda’55 in R, using the means from the pooled imputed data sets to plot the net benefit across a range of thresholds for which a woman would be designated at high risk of preterm birth within 7 days. To compare the potential clinical value of including cervical length in a model, we plotted the net benefit of model A (clinical risk factors + quantitative fFN) against model C (clinical risk factors + quantitative fFN + cervical length) against strategies of ‘treat all’ and ‘treat none’. For comparison, we included diagnostic test accuracy results for cervical length only (based on a single threshold of 15 mm). At any given threshold, the preferred model is that with the higher net benefit. 25
Software
Data were cleaned in IBM Statistical Product and Service Solutions (SPSS) version 24 (IBM Corporation, Armonk, NY, USA). All analyses were done in R.
Results
Description of data
We identified a total of 10 studies of quantitative fFN that were potentially eligible. Four early data sets (in three publications) used ELISAs to determine the concentration of fFN56–58 and were excluded because the different method of analysis and earlier period of study would increase heterogeneity. Six studies fulfilled the eligibility criteria (at the time of study identification only one study59 had been published, but three studies60–62 have subsequently published; Table 3). Five PIs agreed to provide data [Mol, EUFIS (European Fibronectin Study) data;61 van Baaren, APOSTEL-1 (Alleviation of Pregnancy Outcome by Suspending of Tocolysis in Early Labour – 1) study data;60 Khalil, unpublished QFCAPS (Quantitative fetal fibronectin, Cervical length and Actim Partus for the prediction of Preterm birth in Symptomatic women) study data; Shennan, EQUIPP (Evaluation of Fetal Fibronectin with a Quantitative Instrument for the Prediction of Preterm Birth) study data;59 and David, unpublished UCLH/Whit (University College London Hospital/Whittington) study data]. The PI (Elovitz) of the sixth study [Screening to Obviate Preterm Birth (STOP)]62 indicated that data were available only after publication of the study, which occurred after completion of our analysis. The participating studies are from consultant-led maternity units in the UK (three studies)59 and mainland Europe (two studies). 60,61 All women in the included studies provided informed consent for participation in the clinical research and for their data to be used in subsequent analyses. The studies were rated as having a low risk of bias (see Appendix 2).
Variable | Study (authors) | |||||
---|---|---|---|---|---|---|
Included | Excluded | |||||
APOSTEL-1 (Bruijn et al.)60 | EUFIS (Bruijn et al.)61 | EQUIPP (Abbott et al.)59 | QFCAPS (Khalil et al.) | UCLH/Whit (David et al.) | STOP (Levine et al.)62 | |
Design | Prospective cohort study | Prospective cohort study | Prospective cohort study | Prospective cohort study | Prospective cohort study | Prospective cohort study |
Setting | 10 Dutch hospitals | 10 mainland European hospitals | Five UK centres | Two UK centres | Two UK centres | One US centre |
Dates | 2009–12 | 2012–14 | 2010–12 | 2012–16 | 2009–10 | 2013–15 |
Inclusion criteria | ||||||
Signs/symptoms of preterm labour |
|
|
|
|
|
|
Intact membranes | ✓ | ✓ | ✓ | ✓ | ✓ | ✓ |
Gestational age (weeks) | 24–34 | 24–34 | 18–36? | 24–35 | 22–35 | 22–34 |
Singleton/multiple pregnancy | Singleton and multiple | Singleton and twins | Singleton and twins | Singleton only | Singleton and twins | Singleton only |
Age (years) | All | All | All | ≥ 16 | All | All |
Exclusion criteria | ||||||
Cervical dilatation (cm) | > 3 | > 3 | > 3 | > 3 | > 3 | > 2 |
Other |
|
|
|
|
|
|
Primary outcome | Birth within 7 days of fFN test | Birth within 7 days of fFN test | Birth at < 34 weeks’ gestation | Birth within 7 days of fFN test | Birth within 7 days of fFN test | Birth at < 37 weeks’ gestation |
Table 4 shows the availability of the prespecified candidate predictors in each study. Only maternal age, BMI, ethnicity, smoking, nulliparity, multiple pregnancy, gestational age at assessment, previous spontaneous preterm birth before 34 weeks’ gestation, cervical length and fFN test results were available in each study and, therefore, these 10 candidate predictors were included in the model development. Tocolysis was included in sensitivity analysis to explore any potential treatment effect on delaying birth.
Predictor | Study (authors) | ||||
---|---|---|---|---|---|
APOSTEL-1 (Bruijn et al.)60 | EUFIS (Bruijn et al.)61 | EQUIPP (Abbott et al.)59 | QFCAPS (Khalil et al.) | UCLH/Whit (David et al.) | |
Age | ✓ | ✓ | ✓ | ✓ | ✓ |
BMI | ✓ | ✓ | ✓ | ✓ | ✓ |
Ethnicity | ✓ | ✓ | ✓ | ✓ | ✓ |
Smoking | ✓ | ✓ | ✓ | ✓ | ✓ |
Deprivation index | – | – | ✓ | ✓ | ✓ |
Nulliparity | ✓ | ✓ | ✓ | ✓ | ✓ |
Multiple pregnancy | ✓ | ✓ | ✓ | ✓ | ✓ |
Gestational age | ✓ | ✓ | ✓ | ✓ | ✓ |
Previous spontaneous preterm birth at < 34 weeks’ gestation | ✓ | ✓ | ✓ | ✓ | ✓ |
Previous cervical treatment for CIN | ✓ | – | ✓ | ✓ | ✓ |
Number of contractions | ✓ | ✓ | – | – | – |
Vaginal bleeding | ✓ | ✓ | – | – | – |
Cervical dilatation | ✓ | ✓ | – | – | – |
Cervical length | ✓ | ✓ | ✓ | ✓ | ✓ |
Qualitative fFN | ✓ | ✓ | ✓ | ✓ | ✓ |
Quantitative fFN | ✓ | ✓ | ✓ | ✓ | ✓ |
Tocolysis | ✓ | ✓ | ✓ | ✓ | ✓ |
Summary statistics for the baseline participant characteristics and available predictors in the IPD database are shown in Table 5. In total there were 139 events of spontaneous preterm birth within 7 days of fFN test among 1783 women with signs and symptoms of preterm labour; thus, there was an overall outcome proportion of 7.8%. There was a higher rate of spontaneous preterm birth within 7 days in the mainland European studies than in the UK studies. Given the 139 events, and the 10 events per predictor parameter rule of thumb, this suggested that about 14 predictor parameters could be considered. Because we had only 10 candidate predictors, this was appropriate and allowed us to consider non-linear trends.
Variable | Study (authors) | All | ||||
---|---|---|---|---|---|---|
APOSTEL-1 (Bruijn et al.)60 | EUFIS (Bruijn et al.)61 | EQUIPP (Abbott et al.)59 | QFCAPS (Khalil et al.) | UCLH/Whit (David et al.) | ||
Number of participants | 528 | 455 | 452 | 86 | 262 | 1783 |
Age (years), mean (SD) | 29.4 (5.3) | 29.5 (5.2) | 29.5 (6.0) | 30.0 (6.1) | 31.0 (6.1) | 29.7 (5.6) |
BMI (kg/m2), median (IQR) | 25.6 (23.5–28.1) | 25.7 (23.1–28.7) | 24.0 (21.2–28.8) | 24.6 (21.2–28.1) | 23.0 (21.0–27.8) | 24.8 (22.0–28.4) |
Ethnicity, n (%) | ||||||
White | 342 (64.8) | 352 (77.4) | 226 (50.0) | 58 (67.4) | 145 (55.3) | 1123 (63.0) |
South Asian | 8 (1.5) | 4 (0.9) | 25 (5.5) | 6 (7.0) | 30 (11.5) | 73 (4.1) |
East Asian | 6 (1.1) | 12 (2.6) | 10 (2.2) | 3 (3.5) | 12 (4.6) | 43 (2.4) |
African, Caribbean, Middle-Eastern | 63 (11.9) | 69 (15.2) | 159 (35.2) | 15 (17.4) | 57 (21.8) | 363 (20.4) |
Other | 23 (4.3) | 6 (1.3) | 32 (7.1) | 4 (4.7) | 2 (0.8) | 67 (3.8) |
Currently smoking, n (%) | 71 (13.4) | 41 (9.0) | 58 (12.8) | 11 (12.8) | 9 (3.4) | 190 (10.7) |
Nulliparity, n (%) | 288 (54.5) | 262 (57.6) | 200 (44.2) | 34 (39.5) | 140 (53.4) | 924 (51.8) |
Multiple pregnancy, n (%) | 85 (16.1) | 67 (14.7) | 20 (4.4) | 0 (0) | 14 (5.3) | 186 (10.4) |
Gestational age (weeks), median (IQR) | 29.4 (26.8–31.3) | 29.6 (26.7–31.6) | 29.2 (25.6–32.3) | 29.9 (27.3–33.0) | 29.0 (25.6–32.1) | 29.4 (26.4–31.7) |
Previous spontaneous preterm birth at < 34 weeks’ gestation, n (%) | 69 (13.1) | 39 (8.6) | 68 (15.0) | 7 (8.1) | 13 (5.0) | 196 (11.0) |
Cervical length (mm), mean (SD) | 25.0 (12.3) | 21.3 (9.5) | 26.9 (14.0) | 29.8 (9.0) | 14.2 (7.0) | 23.8 (11.5) |
Qualitative fFN: positive, n (%) | 199 (37.7) | 197 (43.3) | 105 (23.2) | 12 (14.0) | 35 (13.4) | 548 (30.7) |
Quantitative fFN (ng/ml), median (IQR) | 17.0 (4.0–112.5) | 34 (8.0–217) | 7.0 (3.0–43.8) | 4.0 (2.0–11.3) | 4.0 (2.0–16.3) | 11.0 (3.0–79.0) |
Tocolysis, n (%) | 345 (65.3) | 319 (70.1) | 36 (8) | 7 (8) | 10 (3.8) | 717 (40.2) |
Outcome: preterm birth | ||||||
< 7 days, n (%) | 70 (13.3) | 48 (10.5) | 14 (3.1) | 2 (2.3) | 5 (1.9) | 139 (7.8) |
< 48 hours, n (%) | 32 (6.1) | 24 (5.3) | 8 (1.8) | 2 (2.3) | 5 (1.9) | 71 (4.0) |
A summary of the percentage of missing data for each study, and across all studies, is presented by candidate predictor in Table 6. Multiple imputation was performed for missing data on all predictors excluding cervical length.
Variable | Study (author), percentage of missing data | All (n = 1783), percentage of missing data | ||||
---|---|---|---|---|---|---|
APOSTEL-1 (Bruijn et al.)60 (n = 528) | EUFIS (Bruijn et al.)61 (n = 455) | EQUIPP (Abbott et al.)59 (n = 452) | QFCAPS (Khalil et al.) (n = 86) | UCLH/Whit (David et al.) (n = 262) | ||
Age | 0.0 | 0.0 | 0.0 | 0.0 | 0.0 | 0.0 |
BMI | 57.4 | 44.4 | 0.4 | 0.0 | 8.4 | 29.7 |
Ethnicity | 16.3 | 2.6 | 0.0 | 0.0 | 6.1 | 6.4 |
Smoking | 8.1 | 9.2 | 0.7 | 0.0 | 8.0 | 6.1 |
Nulliparity | 0.0 | 0.0 | 0.0 | 0.0 | 0.0 | 0.0 |
Multiple pregnancy | 0.0 | 0.0 | 0.0 | 0.0 | 0.0 | 0.0 |
Gestational age | 0.0 | 0.0 | 0.0 | 0.0 | 0.0 | 0.0 |
Previous spontaneous preterm birth at < 34 weeks’ gestation | 0.0 | 0.0 | 0.0 | 0.0 | 0.0 | 0.0 |
Cervical length | 0.0 | 0.0 | 75.9 | 0.0 | 85.5 | 31.8 |
Qualitative fFN | 0.0 | 0.0 | 0.0 | 0.0 | 0.0 | 0.0 |
Quantitative fFN | 0.0 | 0.0 | 0.0 | 0.0 | 0.0 | 0.0 |
There were high levels of missing data for cervical length in two of the UK studies (76% missing in EQUIPP,59 86% missing in UCLH/Whit), which reflects the fact that cervical length is not routinely available for assessment of women with symptoms of preterm labour in the UK despite being recognised as a predictor of spontaneous preterm birth. 13 With the agreement of the project management group and study steering committee, to explore the potential prognostic value of combining quantitative fFN with cervical length we decided on the following analysis strategy for the primary end point (spontaneous preterm birth within 7 days):
-
Primary analysis – prognostic models with quantitative fFN without cervical length as candidate predictor, based on data from all five studies (relevant to current UK practice, in which cervical length assessment is not consistently routinely available). Model A is the model with variable selection (most parsimonious model). Model B is the full model with all predictors forced into the model.
-
Secondary analysis (i) – prognostic models with cervical length as the candidate predictor, based on data from three studies with complete cases of cervical length (EUFIS,61 APOSTEL-160 and QFCAPS) [exploring the potential added value of including cervical length in the prognostic model but based on a mainly mainland European (and higher-risk) population]. Model C is the model with variable selection (most parsimonious model). Model D is the full model with all predictors forced into the model.
-
Secondary analysis (ii) – a prognostic model with cervical length as the candidate predictor, based on data from all five studies, with multiple imputation of cervical length and other missing participant data (exploring the potential added value of including cervical length in the prognostic model, including data from a UK population, but with added uncertainty from the multiple imputation of large numbers of missing data). Model E is the model with variable selection (most parsimonious model). Model F is the full model with all predictors forced into the model.
Heterogeneity of predictor effects
The meta-analysis of the candidate predictor effects for the primary analysis showed low to moderate heterogeneity of their adjusted log-odds ratios across studies (see Appendix 3). Only previous spontaneous preterm birth showed moderate heterogeneity (τ2 = 0.6 and I2 = 38%). The other values were all close to zero. Therefore, we did not exclude any of the candidate predictors in our primary analysis because of heterogeneity.
Cervical length showed high heterogeneity (I2 = 75%) across all the studies, probably reflecting the recognised higher-risk population in the mainland European studies (EUFIS61 and APOSTEL-160) and regional differences in clinical practice. A plan was made to explore the effect of cervical length in model development in the secondary analyses while recognising the inherent uncertainty resulting from population differences and imputation of data.
Prognostic models for spontaneous preterm birth within 7 days
Primary analysis: prognostic model based on quantitative fetal fibronectin and clinical risk factors (models A and B)
For multiple imputation of predictors, we based the number of imputed data sets on the largest proportion of incomplete cases observed in an individual study. The EUFIS study60 had 59.5% incomplete cases, so we created 60 imputed data sets. We performed the multiple imputation in each data set separately before merging them together. Because QFCAPS did not have any missing data, we replicated the data from this study 60 times to merge the multiple imputed data sets.
After merging the five data sets, a prognostic model with all available candidate predictors (quantitative, maternal age, BMI, ethnicity, smoking, nulliparity, multiple pregnancy, gestational age at assessment, previous spontaneous preterm birth before 34 weeks’ gestation) was fitted in each of the imputed data sets and the results were combined using Rubin’s rules. Predictor variables were dropped stepwise based on the largest p-value > 0.1. After every step, the MFP procedure was used within each imputation set to allow the selection of non-linear terms for continuous variables. The final list of predictors for the logistic model was quantitative fFN, smoking, ethnicity, nulliparity and multiple pregnancy. Quantitative fFN was transformed (square root) because of non-linearity.
Table 7 shows the logistic models before (model B) and after (model A) variable selection prior to adjustment for optimism. The more parsimonious model A identified that high quantitative fFN levels, South Asian ethnicity, nulliparity and multiple pregnancy were associated with increased probability of spontaneous preterm birth within 7 days. East Asian and African/Caribbean/Middle-Eastern and other ethnicity, and smoking, were associated with a reduced risk. The apparent AUC for the model before variable selection (averaged across all multiply imputed data sets) was 0.90 [95% confidence interval (CI) 0.88 to 0.93] and the Nagelkerke R2 was 0.39. For the final model after variable selection (model A) the AUC was 0.90 (95% CI 0.87 to 0.93) and the Nagelkerke R2 was 0.38, indicating very little additional benefit of the omitted variables.
Variable | Model including all variables (model B) | Model after variable selection (model A) | ||
---|---|---|---|---|
Intercept | 95% CI | Intercept | 95% CI | |
Study | ||||
1 (APOSTEL-1)60 | –7.849 | –11.24 to –4.45 | –5.019 | –6.43 to –3.60 |
2 (EUFIS)61 | –8.529 | –11.58 to –5.10 | –5.697 | –7.11 to –4.28 |
3 (EQUIPP)59 | –9.019 | –12.44 to –5.59 | –6.152 | –7.61 to –4.69 |
4 (QFCAPS) | –8.700 | –12.40 to –5.00 | –5.874 | –7.85 to –3.90 |
5 (UCLH/Whit) | –9.324 | –12.87 to –5.78 | –6.493 | –8.21 to –4.78 |
Variable | Model including all variables (model B) | Model after variable selection (model A) | ||
Beta | OR (95% CI) | Beta | OR (95% CI) | |
Quantitative fFNa | 2.033 | 7.64 (5.68 to 10.28) | 2.042 | 7.71 (5.74 to 10.34) |
Age | 0.024 | 1.02 (0.98 to 1.07) | – | – |
BMI | 0.018 | 1.02 (0.96 to 1.13) | – | – |
Smoking | –0.656 | 0.52 (0.24 to 1.13) | –0.729 | 0.48 (0.23 to 1.03) |
Ethnicity | ||||
White | Reference | – | Reference | – |
South Asian | 1.066 | 2.90 (0.93 to 9.10) | 1.020 | 2.77 (0.89 to 8.61) |
East Asian | –1.184 | 0.31 (0.04 to 2.49) | –1.087 | 0.34 (0.04 to 2.65) |
African, Caribbean, Middle-Eastern | –0.216 | 0.81 (0.42 to 1.54) | –0.224 | 0.80 (0.46 to 1.50) |
Other | –0.252 | 0.78 (0.20 to 3.00) | –0.330 | 0.72 (0.18 to 2.82) |
Nulliparity | 0.527 | 1.69 (1.06 to 2.71) | 0.394 | 1.483 (0.95 to 2.31) |
Multiple pregnancy | 0.852 | 2.34 (1.00 to 4.07) | 0.900 | 2.46 (1.44 to 4.20) |
Previous spontaneous preterm birth at < 34 weeks’ gestation | 0.427 | 1.53 (1.25 to 3.03) | – | – |
Gestational age at assessment | 0.031 | 1.031 (0.49 to 1.11) | – | – |
Apparent predictive performance | ||||
Nagelkerke R2 | 0.39 | 0.38 | ||
AUC (95% CI) | 0.90 (0.88 to 0.93) | 0.90 (0.87 to 0.93) |
Internal validation using a non-parametric bootstrap resampling technique resulted in a uniform shrinkage factor of 0.92, which suggested some slight overfitting during model development as expected. Table 8 shows the final model (model A) after shrinkage to adjust for overfitting. The optimism-adjusted AUC for this model was 0.89 (95% CI 0.87 to 0.93) and the optimism-adjusted Nagelkerke R2 was 0.39. Figure 1 shows the calibration plot for model A with predicted versus observed risk in the complete data set.
Variable | Final model after variable selection and adjustment for optimism (model A) | |
---|---|---|
Beta | OR (95% CI) | |
Study | ||
1 (APOSTEL-1)60 | –4.640 | |
2 (EUFIS)61 | –5.267 | |
3 (EQUIPP)59 | –5.688 | |
4 (QFCAPS) | –5.431 | |
5 (UCLH/Whit) | –6.003 | |
Quantitative fFNa | 1.888 | 6.61 (4.92 to 8.87) |
Age | – | – |
BMI | – | – |
Smoking | –0.674 | 0.51 (0.24 to 1.08) |
Ethnicity | ||
White | Reference | – |
South Asian | 0.943 | 2.57 (0.84 to 7.88) |
East Asian | –1.005 | 0.37 (0.05 to 2.77) |
African, Caribbean, Middle-Eastern | –0.207 | 0.81 (0.43 to 1.52) |
Other | –0.305 | 0.74 (0.19 to 2.82) |
Nulliparity | 0.364 | 1.44 (0.92 to 2.24) |
Multiple pregnancy | 0.832 | 2.30 (1.35 to 3.92) |
Previous spontaneous preterm birth at < 34 weeks’ gestation | – | – |
Gestational age at assessment | – | – |
Performance | ||
Nagelkerke R2 | 0.39 | |
AUC (95% CI) | 0.89 (0.87 to 0.93) |
FIGURE 1.
Calibration plot with predicted vs. observed risk for model A as applied to the complete data set.
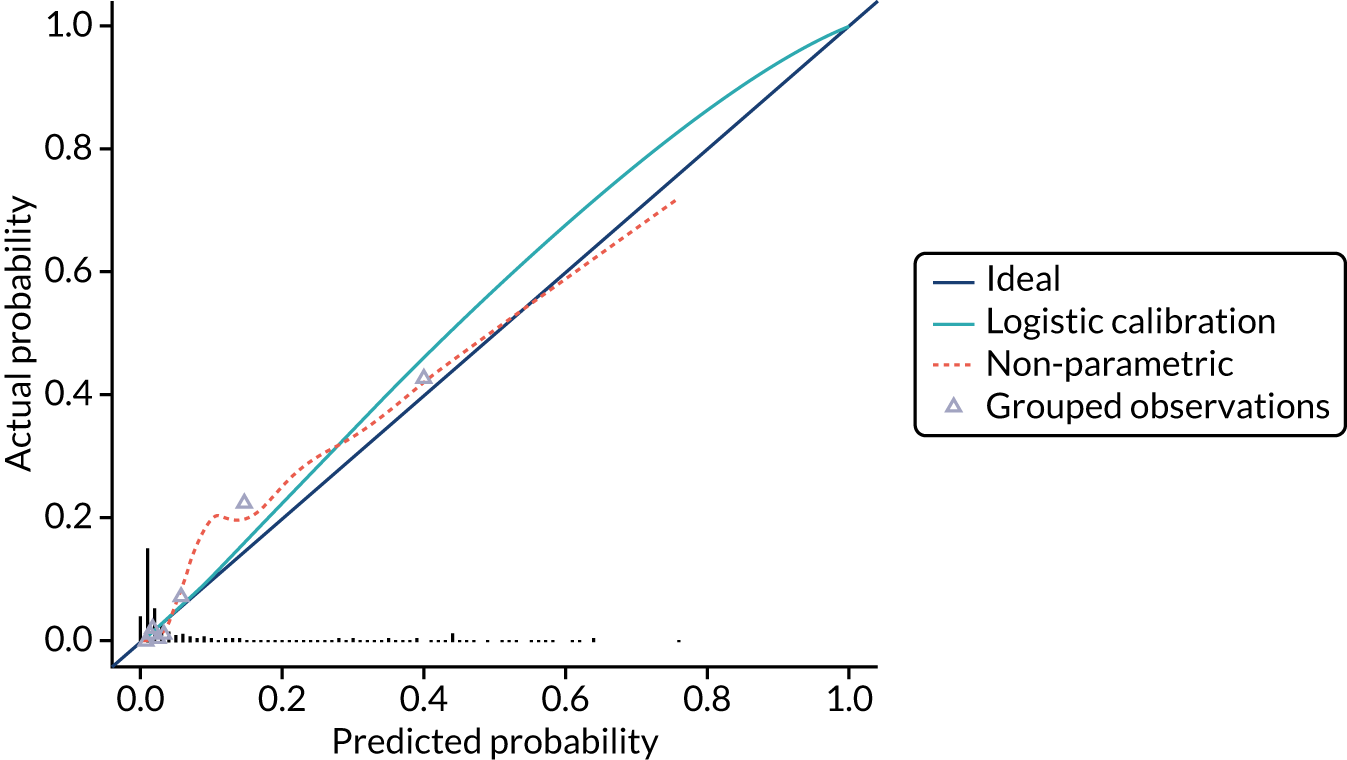
Secondary analysis (i): prognostic model based on quantitative fetal fibronectin and clinical risk factors with cervical length data from three European studies (models C and D)
The multiple imputed data sets (m = 60) from the three studies without missing values of cervical length (EUFIS,61 APOSTEL-160 and QFCAPS) were used to develop this model.
The same model development methods were used. The final list of predictors for this logistic model included quantitative fFN, cervical length, maternal age, smoking and gestational age at assessment. Quantitative fFN was not transformed in this model, hence the coefficients for quantitative fFN appear markedly different from those of models A and B.
Table 9 shows the logistic model before and after variable selection prior to adjustment for optimism. The final model identified that high quantitative fFN levels, high maternal age and high gestational age at assessment were associated with increased probability of spontaneous preterm birth within 7 days. Longer cervical length and smoking were associated with a reduced risk. The apparent c-statistic for the model before variable selection (averaged across all multiply imputed data sets) was 0.92 (95% CI 0.90 to 0.94) and the Nagelkerke R2 was 0.52. For the final model after variable selection (model C) the c-statistic was 0.92 (95% CI 0.89 to 0.94) and the Nagelkerke R2 was 0.51. Internal validation using a non-parametric bootstrap resampling technique resulted in a uniform shrinkage factor of 0.92. Table 10 shows the final model (model C) after adjustment for optimism. The apparent c-statistic for this model was 0.91 (95% CI 0.89 to 0.94) and the Nagelkerke R2 was 0.51. Figure 2 shows the calibration plot for model C with predicted versus observed risk in the complete data set.
Variable | Model including all variables (model D) | Model after variable selection (model C) | ||
---|---|---|---|---|
Intercept | 95% CI | Intercept | 95% CI | |
Study | ||||
1 (APOSTEL-1)60 | –2.932 | –7.23 to1.37 | –1.960 | –5.33 to 1.41 |
2 (EUFIS)61 | –3.535 | –7.85 to 0.78 | –2.615 | –6.00 to 0.77 |
4 (QFCAPS) | –4.267 | –8.89 to 0.36 | –3.382 | –7.26 to 0.49 |
Variable | Beta | OR (95% CI) | Beta | OR (95% CI) |
Quantitative fFN | 0.007 | 1.012 (1.010 to 1.015) | 0.007 | 1.012 (1.010 to 1.015) |
Cervical lengtha | –3.141 | 0.04 (0.02 to 0.09) | –3.195 | 0.04 (0.02 to 0.08) |
Age | 0.039 | 1.04 (0.99 to 1.09) | 0.047 | 1.05 (1.00 to 1.01) |
BMI | 0.013 | 1.01 (0.94 to 1.10) | – | – |
Smoking | –1.078 | 0.34 (0.12 to 0.95) | –1.076 | 0.34 (0.13 to 0.92) |
Ethnicity | ||||
White | Reference | – | Reference | – |
South Asian | 1.440 | 4.22 (0.72 to 24.80) | – | – |
East Asian | –0.686 | 0.50 (0.05 to 5.24) | – | – |
African, Caribbean, Middle-Eastern | –0.505 | 0.60 (0.23 to 1.58) | – | – |
Other | 0.119 | 1.13 (0.21 to 5.93) | – | – |
Nulliparity | –0.128 | 0.88 (0.49 to 1.60) | – | – |
Multiple pregnancy | 0.490 | 1.63 (0.85 to 3.14) | – | – |
Previous spontaneous preterm birth at < 34 weeks’ gestation | 0.328 | 1.39 (0.56 to 3.45) | – | – |
Gestational age at assessment (weeks) | 0.100 | 1.11 (1.00 to 1.22) | 0.100 | 1.11 (1.00 to 1.22) |
Performance | ||||
Nagelkerke R2 | 0.52 | 0.51 | ||
AUC (95% CI) | 0.92 (0.90 to 0.94) | 0.92 (CI 0.89 to 0.94) |
Variable | Model including all variables (model C) | |
---|---|---|
Intercept | 95% CI | |
Study | ||
1 (APOSTEL-1)60 | –1.805 | – |
2 (EUFIS)61 | –2.408 | – |
4 (QFCAPS) | –3.114 | – |
Variable | Beta | OR (95% CI) |
Quantitative fFN | 0.006 | 1.01 (1.01 to 1.01) |
Cervical lengtha | –2.942 | 0.05 (0.03 to 0.11) |
Age | 0.043 | 1.04 (1.00 to 1.10) |
BMI | – | – |
Smoking | –0.991 | 0.37 (0.14 to 0.99) |
Ethnicity | ||
White | Reference | Reference |
South Asian | – | – |
East Asian | – | – |
African, Caribbean, Middle-Eastern | – | – |
Other | – | – |
Nulliparity | – | – |
Multiple pregnancy | – | – |
Previous spontaneous preterm birth at < 34 weeks’ gestation | – | – |
Gestational age at assessment | 0.092 | 1.10 (0.99 to 1.21) |
Performance | ||
Nagelkerke R2 | 0.51 | |
AUC (95% CI) | 0.91 (0.89 to 0.94) |
FIGURE 2.
Calibration plot with predicted vs. observed risk for model C as applied to the complete data set.
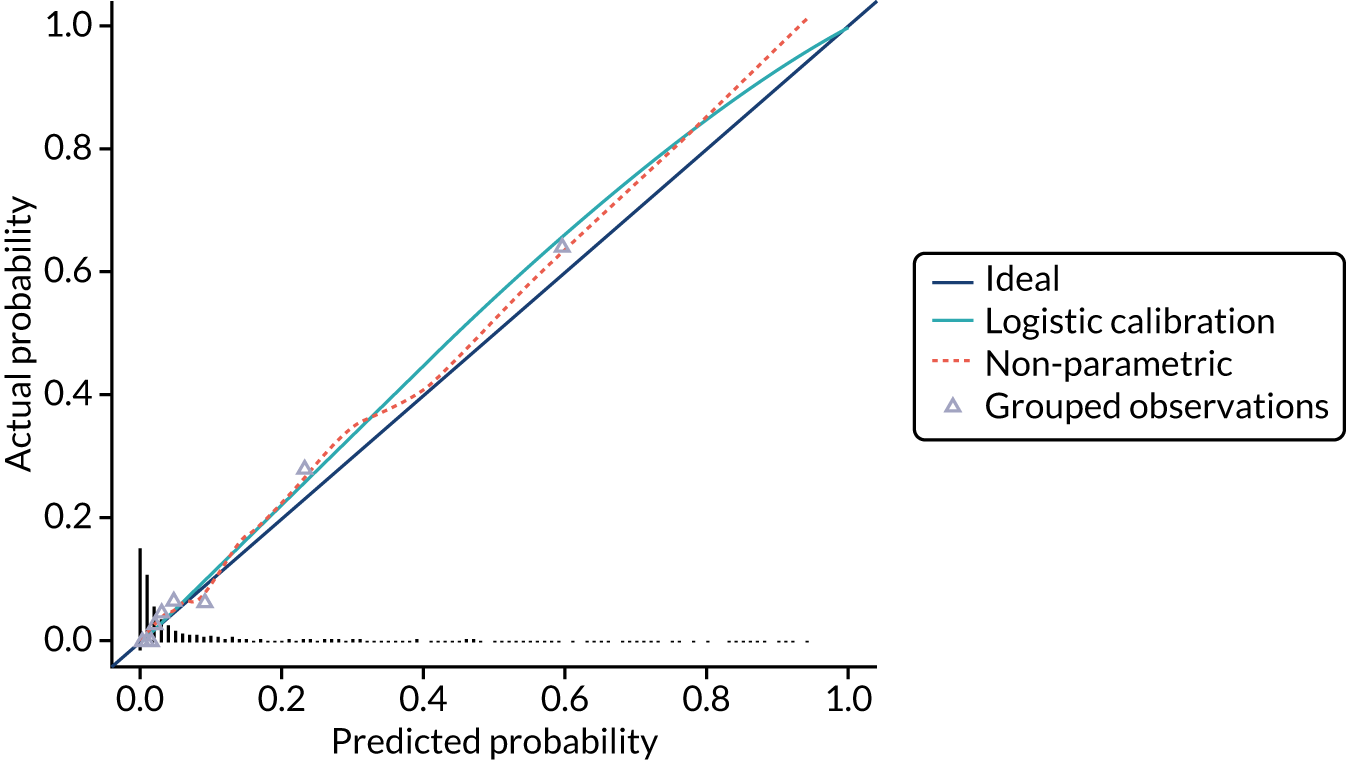
Secondary analysis (ii): prognostic model based on quantitative fetal fibronectin and clinical risk factors with cervical length data from three European studies (models E and F)
For this model we included all five studies, with imputation of missing values of cervical length in the two studies with missing data (EQUIPP59 and UCLH/Whit). However, in UCLH/Whit, cervical length was measured only in women at high risk of imminent birth (resulting in a shorter mean cervical length of 14 mm). As the missing cervical length data were not MAR in this study, multiple imputation was not feasible. Therefore, we first performed multiple imputation of missing data in all studies separately (m = 75 because of 75% incomplete cases in the EQUIPP study59), except for missing cervical length data in the UCLH/Whit study. The imputed data of all studies were then combined and a single imputation was performed for the completely missing cervical length data in UCLH/Whit study.
The same model development methods were used. The final list of predictors for this logistic model included quantitative fFN, cervical length, smoking, multiple pregnancy and gestational age at assessment. Cervical length was transformed because of non-linearity.
Table 11 shows the logistic model before and after variable selection prior to adjustment for optimism. The final model identified that high quantitative fFN levels, multiple pregnancy and high gestational age at assessment were associated with increased probability of spontaneous preterm birth within 7 days. Large cervical length and smoking were associated with a reduced risk. Quantitative fFN was not transformed in this model, hence the coefficients for quantitative fFN appear markedly different from those of models A and B.
Variable | Model including all variables (model G) | Model after variable selection (model F) | ||
---|---|---|---|---|
Intercept | 95% CI | Intercept | 95% CI | |
Study | ||||
1 (APOSTEL-1)60 | –3.751 | –7.58 to 0.07 | –1.609 | – |
2 (EUFIS)61 | –4.354 | –8.19 to –0.51 | –2.262 | – |
3 (EQUIPP)59 | –5.391 | –9.21 to –1.58 | –3.252 | – |
4 (QFCAPS) | –5.045 | –9.19 to –0.90 | –3.029 | – |
5 (UCLH/Whit) | –4.992 | –8.99 to –1.00 | –2.754 | – |
Variable | Beta | OR (95% CI) | Beta | OR (95% CI) |
Quantitative fFN | 0.006 | 1.01 (1.01 to 1.01) | 0.006 | 1.01 (1.01 to 1.01) |
Cervical lengtha | –2.807 | 0.06 (0.03 to 0.12) | –2.815 | 0.06 (0.03 to 0.12) |
Age | 0.031 | 1.03 (0.99 to 1.1) | – | – |
BMI | 0.014 | 1.01 (0.95 to 1.1) | – | – |
Smoking | –0.880 | 0.41 (0.17 to 0.99) | –1.005 | 0.37 (0.16 to 0.86) |
Ethnicity | ||||
White | Reference | – | – | – |
South Asian | 1.165 | 3.21 (0.83 to 12.33) | – | – |
East Asian | –0.696 | 0.50 (0.06 to 4.10) | – | – |
African, Caribbean, Middle-Eastern | –0.116 | 0.89 (0.44 to 1.82) | – | – |
Other | 0.108 | 1.11 (0.28 to 4.42) | – | – |
Nulliparity | 0.150 | 1.16 (0.68 to 1.98) | – | – |
Multiple pregnancy | 0.509 | 1.66 (0.92 to 3.01) | 0.560 | 1.75 (0.99 to 3.10) |
Previous spontaneous preterm birth at < 34 weeks’ gestation | 0.329 | 1.39 (0.65 to 2.95) | – | – |
Gestational age at assessment (weeks) | 0.101 | 1.11 (1.01 to 1.20) | 0.097 | 1.10 (1.01 to 1.10) |
Performance | Model including all variables (model G) | Model after variable selection (model F) | ||
Nagelkerke R2 | 0.47 | 0.47 | ||
AUC (95% CI) | 0.93 (0.91 to 0.95) | 0.93 (0.90 to 0.95) |
The apparent c-statistic for the model before variable selection (averaged across all multiply imputed data sets) was 0.93 (95% CI 0.91 to 0.95) and the Nagelkerke R2 was 0.47. For the final model, after variable selection the c-statistic was 0.93 (95% CI 0.90 to 0.95) and the Nagelkerke R2 was 0.47. Internal validation using a non-parametric bootstrap resampling technique resulted in a uniform shrinkage factor of 0.92. Table 12 shows the final model after adjustment for optimism. Figure 3 shows the calibration plot with predicted versus observed risk in the complete data set.
Variable | Model including all variables (model F) | |
---|---|---|
Beta | OR (95% CI) | |
Study | ||
1 (APOSTEL-1)60 | –1.484 | – |
2 (EUFIS)61 | –2.087 | – |
3 (EQUIPP)59 | –3.000 | – |
4 (QFCAPS) | –2.794 | – |
5 (UCLH/Whit) | –2.541 | – |
Quantitative fFN | 0.006 | 1.01 (1.01 to 1.01) |
Cervical lengtha | –2.597 | 0.07 (0.04 to 0.14) |
Age | – | – |
BMI | – | – |
Smoking | –0.927 | 0.40 (0.17 to 0.92) |
Ethnicity | ||
White | Reference | |
South Asian | – | – |
East Asian | – | – |
African/Caribbean/Middle-Eastern | – | – |
Other | – | – |
Nulliparity | – | – |
Multiple pregnancy | 0.517 | 1.68 (0.95 to 2.96) |
Previous spontaneous preterm birth at < 34 weeks’ gestation | – | – |
Gestational age at assessment | 0.089 | 1.09 (1.01 to 1.19) |
Performance measures | Model including all variables (model F) | |
Nagelkerke R2 | 0.48 | |
AUC (95% CI) | 0.93 (0.90 to 0.95) |
FIGURE 3.
Calibration plot with predicted vs. observed risk for model F as applied to the complete data set.
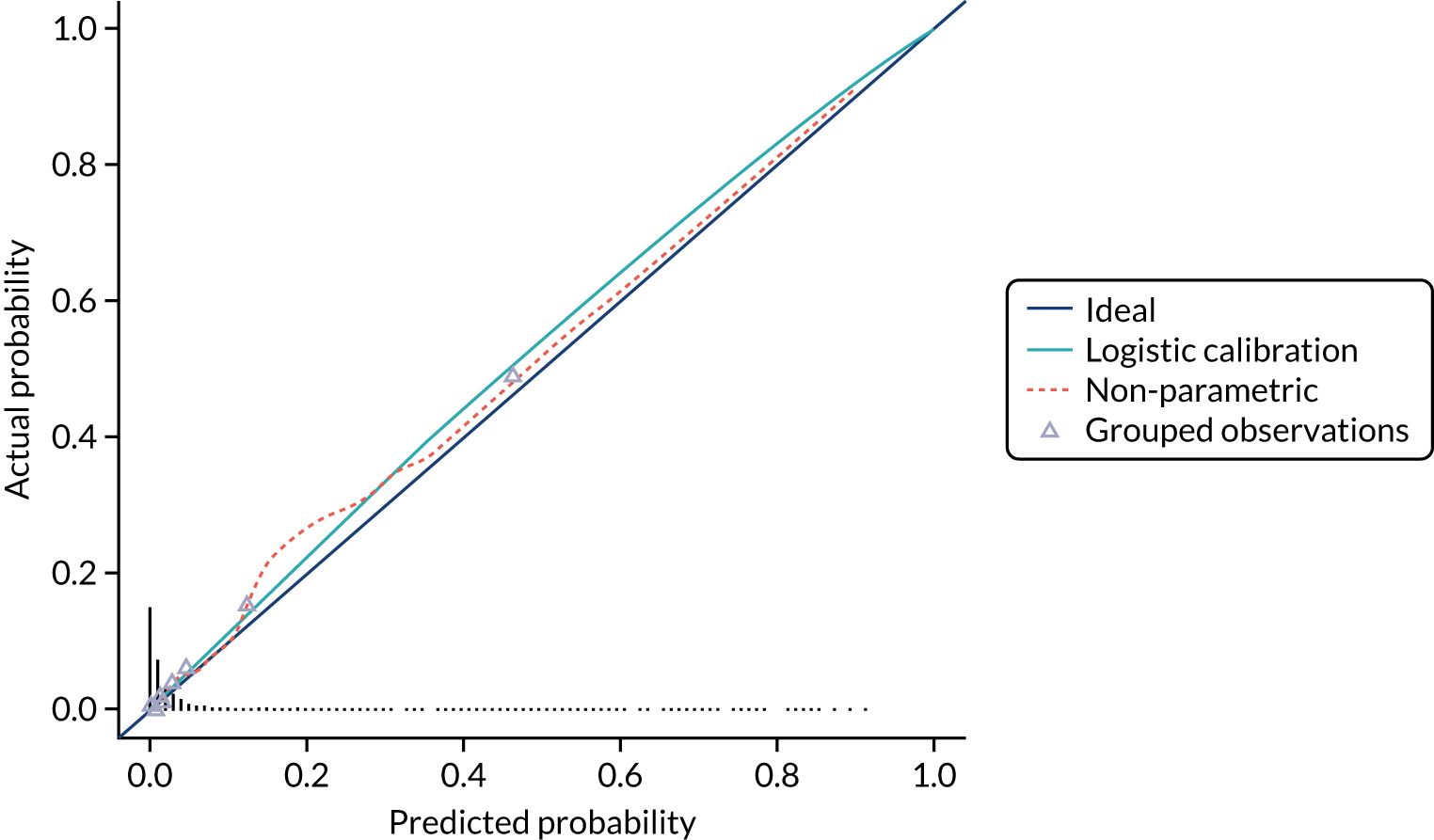
Net benefit analysis for prognostic model with and without cervical length
Figure 4 shows the net benefit curves for the means of the pooled imputation data set for model A (quantitative fFN + clinical risk factors), model C (quantitative fFN + clinical risk factors + cervical length) and cervical length only (at a single threshold of 15 mm, as currently recommended by NICE). 13 Model A and model C allow more women to be correctly identified as low risk up to a risk threshold of around 25%. Model A performs better than model C at risk thresholds below 5%, and model A and Model C perform similarly at thresholds above 5%, suggesting that there is limited clinical value in adding cervical length to the model. Cervical length (using a cut-off point of ≤ 15 mm) appears to have less clinical utility across all risk thresholds than the prognostic models based on multiple predictors.
FIGURE 4.
Net benefit curves for model A, model C and cervical length. Treat all (all) and treat none (none) are plotted for comparison. Cx, cervical length.
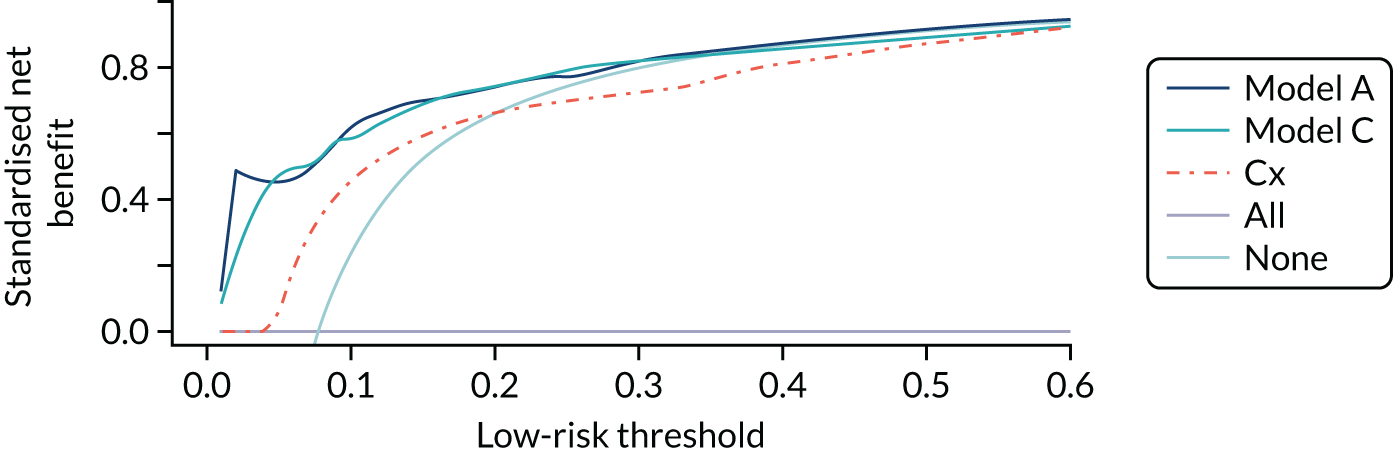
Sensitivity analysis for prognostic models for spontaneous preterm birth within 7 days
The results of the sensitivity analysis including tocolysis in models A and B are shown in the Appendix 4, Tables 36 and 37. This was performed to explore any potential confounding effect on time to birth resulting from administration of tocolysis (administration may be associated with other characteristics of the women and may delay birth by up to 48 hours). 52 If tocolysis is effective, it could be expected that tocolysis would be associated with a reduced risk of preterm birth within 7 days. However, the model showed that tocolysis was associated with a significantly increased probability of spontaneous preterm birth rather than having any association with delayed birth.
Prognostic models for spontaneous preterm birth within 48 hours
We had proposed to develop a model for spontaneous preterm birth within 48 hours if there were sufficient numbers of events. However, there were only 71 births within 48 hours in the IPD meta-analysis cohort. Therefore, we decided to combine the IPD meta-analysis cohort with the prospective cohort study for development of this model, recognising that using the maximal sample size is preferred. 63 This model will require further validation in future (see Chapter 6).
Discussion
In this chapter we describe development and internal validation of a number of prognostic models, including quantitative fFN and other risk factors, to predict spontaneous preterm birth within 7 days in women presenting with symptoms of preterm labour. Models were created with and without inclusion of cervical length using data from five European studies. All the models developed have excellent discrimination.
As cervical length is not routinely part of the assessment of women with threatened preterm labour in the UK,10 it was important that we developed a model without inclusion of cervical length that could be readily implemented in the UK should it be validated. Quantitative fFN dominated this model, being the strongest predictor of preterm birth. Certain clinical risk factors (smoking, ethnicity, nulliparity and multiple pregnancy) add prognostic value. Other candidate predictors (age, BMI, previous spontaneous preterm birth at < 34 weeks’ gestation and gestational age at assessment) were not found to be predictive of preterm birth in our model. We did include them in a second ‘all variable’ (nine-predictor) model, which we also validated in our prospective cohort study, and determined if there was any difference in performance and calibration between the two models.
We also developed models with cervical length to explore the added prognostic value if this measurement was available, and included it in the cost-effectiveness analysis (see Chapter 5). The predictive performance of these models in terms R2 and AUC was similar to the models without cervical length. However, the models that included cervical length were based on either (1) a data set with very high levels of imputed values or (2) data which predominantly come from outside the UK; thus, there is considerable uncertainty in the results. A net benefit analysis suggested that there was little clinical value from including cervical length in the prognostic model with quantitative fFN and clinical risk factors. Because cervical length measurement is rarely available in acute maternity services in the UK 24 hours per day (where and when women with threatened preterm labour present), we recognised from the outset that (1) we would be unlikely to be able to validate the models including cervical length within the prospective cohort data and (2) implementation of a model including cervical length would be difficult in the NHS. Indeed, the very low levels of cervical length recorded in the QUIDS prospective cohort study suggest that this was the case (see Chapter 6).
We could not include all of the prespecified candidate predictors in the prognostic model development because they were not universally recorded in the contributing studies. Variables such as previous cervical treatment for CIN, the number of contractions, presence or absence of vaginal bleeding, cervical dilatation and deprivation index may have the potential to improve the performance of the prognostic model. This will be explored in future analyses of prospective cohort study data (where these variables are recorded) and may be an area of future research.
The primary end point of the prognostic model was spontaneous preterm birth within 7 days of testing, as influenced by QUIDS qualitative (see Chapter 3), which included focus group consultation to determine the decisional needs of women, their partners and clinicians. It is also recognised as a clinically important end point because antenatal steroids (which significantly reduce morbidity and mortality in preterm babies) are most effective if birth occurs within 7 days of administration. 6 A secondary analysis using the end point of birth within 48 hours was also deemed important by women and clinicians. Because the number of events of births within 48 hours was smaller, we developed this secondary model from combining the IPD meta-analysis data set with the prospective cohort. This maximises the number of events and, thus, the precision of the model developed. 63 However, it will require further validation in the future.
Not all predictors in the model performed as expected. For example, smoking is recognised to be a risk factor for spontaneous preterm birth overall, but in our model it was associated with a reduced probability of spontaneous preterm birth in women who presented with symptoms. The reasons for this are unclear, but it may reflect an interaction between smoking and management decisions. For example, clinicians’ perception of smokers as being at ‘high risk’ of preterm birth may mean that they perform a fFN test in the presence of minor symptoms more readily in smokers than in non-smokers.
Strengths of this work include the detailed, prespecified and transparent protocol that was used for model development,41 in accordance with guidelines,38–40 including all identified available data and careful internal validation. A potential limitation is the number of missing data for certain variables; for example, 30% of BMI data were missing across all data sets. However, we addressed this using multiple imputation, which has been shown to be a valid technique for dealing with missing data in logistic regression models, resulting in less bias than excluding all women with missing data. 47
A sensitivity analysis with and without inclusion of tocolysis (which is given in an attempt to delay preterm birth) was performed to explore any potential confounding effects. Counterintuitively, the sensitivity analysis results showed that tocolysis was associated with an increased probability of spontaneous preterm birth within 7 days. This probably reflects the fact that women at highest risk of preterm birth were correctly identified and given tocolytics. Although the sensitivity analysis indicated that receipt of tocolysis could be considered as a predictor of preterm birth, we did not include it in our models because it was our prespecified intention to develop a model to guide treatment decisions (i.e. to be applied ‘upstream’ of the decision to start tocolysis).
In summary, we have used existing data on quantitative fFN to develop models for the prediction of preterm birth within 7 days in women presenting with signs and symptoms of preterm labour, which have excellent discrimination. In the following chapters we discuss the inclusion of this model in a cost-effectiveness analysis (see Chapter 5) and external validation of these in a UK population (see Chapter 6).
Chapter 5 Health economic analysis of prognostic models from individual participant data meta-analysis
Context
In this chapter, we describe the economic analyses that were undertaken based on the results of the IPD meta-analysis. A decision-analytic model was designed and populated based on published literature, and the analysis was undertaken in two parts based on the outcome data from the IPD meta-analyses. In part 1, the economic model was run using diagnostic test accuracy estimates generated from the IPD meta-analysis to compare the cost-effectiveness of qualitative fFN (UK routine practice) with that of quantitative fFN and cervical length measurement, both of which are relevant alternatives in the context of UK decision-making. Following the development of the prognostic models described in Chapter 4, part 2 of the economic analysis used the results from the most promising prognostic model(s) to provide an economic rationale for their inclusion in the cohort study, assessing the potential cost-effectiveness from the perspective of the NHS. Cervical length measurement is the recommended UK practice13 yet it is not routinely part of the assessment of women with threatened preterm labour in the UK. 10 Therefore, we wanted to consider both the prognostic model that included quantitative fFN and the clinical risk factors (model A; see Table 8) and a prognostic model that included quantitative fFN and the clinical risk factors and cervical length measurement. We chose to use model C (see Table 10) in this economic analysis because it avoids additional uncertainty caused by high levels of data imputation. The analysis explored the potential cost-effectiveness of each strategy to determine if it would be worthwhile including them in the validation cohort study.
Methods
The cost-effectiveness analysis was undertaken from the perspective of the NHS and Personal Social Services for cost year 2017/18, adhering to good practice guidelines64 and using NHS Reference Costs 2017/18. 65 The cost-effectiveness was assessed using a 7-day time horizon (in line with the primary outcome of birth within 7 days) to capture diagnostic accuracy, morbidity, mortality and costs to the NHS. Discounting was unnecessary given the 7-day time horizon. The outcomes of interest were cost and the incremental cost-effectiveness ratio (ICER) expressed as the cost per quality-adjusted life-day (QALD) based on a willingness-to-pay threshold of £55 per QALD gained [i.e. a willingness-to-pay threshold of £20,000 per quality-adjusted life-year (QALY) gained]. 66
Economic model
A literature review was undertaken to inform the model design and identify parameters. MEDLINE, EMBASE, the Cochrane Library and Paediatric Economic Database Evaluation were searched, on 17 January 2017 and with a date range of inception to January 2017, for all economic analyses that included the use of fFN testing in women with threatened preterm labour. Full details of the search strategy are given in Appendix 5, Tables 38–41, and Appendix 5, Figure 18.
A decision tree was developed to illustrate the clinical pathway for each prognostic strategy over the 7-day time horizon. The clinical pathway is the same for each of the three strategies (qualitative fFN, quantitative fFN and cervical length measurement); Figure 5 illustrates one branch of the decision tree (one strategy) for simplification. The decision tree shows the pathway for a pregnant woman presenting with preterm labour, incorporating the diagnostic test accuracy associated with each test (distinguishing between accurate and inaccurate prediction), short-term management and resultant neonatal outcomes (mortality, major morbidity, minor morbidity, full health and did not deliver) at 7 days post testing, and the quality of life and costs associated with these.
FIGURE 5.
Decision tree for alternative strategy pathways.
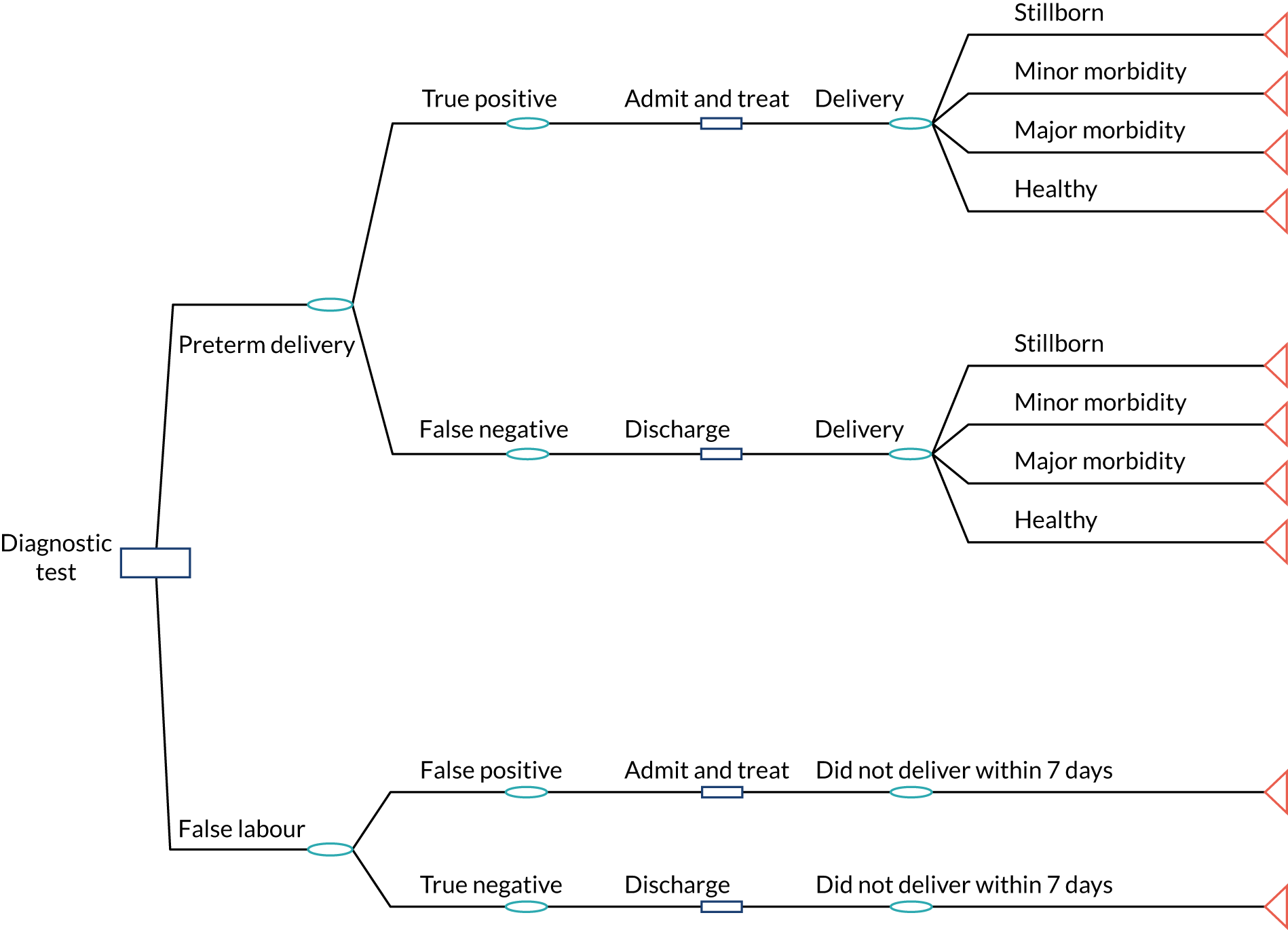
For each prognostic strategy the tree initiates with the true prevalence of preterm labour at the outset. Presentation of preterm labour that is identified as ‘positive’ by the strategy is diagnosed as preterm labour accurately (true positive) or inaccurately (false positive) and the pregnant woman is hospitalised and receives antenatal corticosteroids (admit and treat), which reduce the risk of neonatal morbidity and mortality. Presentation of preterm labour that is identified as ‘negative’ by the prognostic strategy is diagnosed as term labour accurately (true negative) or inaccurately (false negative) and the pregnant woman is not hospitalised and, therefore, does not receive antenatal corticosteroids. The model can allows for the possibility that a negative test result is over-ruled and the patient is admitted and treated and can also be run under a hypothetical ‘treat-all’ strategy in which everyone is admitted. The base-case analysis did not incorporate any over-ruling; this option to over-rule tests or treat all was explored in sensitivity analyses. The final destination in the decision tree is one of five possible states for preterm births: stillborn, minor morbidity, major morbidity, full health and did not birth within 7 days.
Given this structure, the model accounts for both the clinical and the economic impact of false-negative and false-positive results from the prognostic strategies. False negatives represent failures to treat women with risk-reducing antenatal corticosteroids, so infants associated with false-negative results will not receive antenatal corticosteroids and therefore have a greater probability of experiencing neonatal morbidity and mortality, incurring the associated costs and experiencing the associated quality-of-life and survival effects of these. False positives, on the other hand, will result in women being admitted to hospital unnecessarily, incurring the additional and unnecessary costs of hospitalisation, interhospital transfer and treatment. It is assumed that there are no quality-of-life side effects for receiving unnecessary treatment. This framework allows us to identify the correct diagnoses (true positives and true negatives) and capture the consequences of incorrect diagnoses [both false positives (women admitted and treated unnecessarily) and false negatives (women not admitted and not treated correctly)]; therefore, the alternative prognostic or diagnostic strategies that have the greatest predictive value will have the best outcomes in terms of improved morbidity and mortality outcomes and lower costs to the NHS.
Parameters for the economic model
The key parameters used to populate the economic model include the probabilities of the various neonatal outcomes (did not deliver, stillborn, minor morbidity, major morbidity and full health), admission to NICUs, morbidity risk reduction from antenatal corticosteroids, diagnostic test accuracy outcomes generated from (for part 1) the IPD meta-analysis and (for part 2) predicted prognostic outcomes from the prognostic models (model A, see Table 8, and model C, see Table 10), unit costs and health utilities. The full list of all parameters for the model, their values and sources is given in Appendix 6, Table 42.
Estimating effects
For part 1 of the analyses, the diagnostic test accuracy for the qualitative fFN, quantitative fFN and cervical length measurement strategies was calculated directly from the IPD meta-analyses results (the independent variables on diagnostic test accuracy from the tests in the IPD meta-analysis). For part 2 of the analyses, the most promising prognostic models from the IPD meta-analyses were used, that is the quantitative fFN prognostic model (model A; see Table 8) and the prognostic model including cervical length (model C; see Table 10). The predictive value was derived from these prognostic models, described in detail in Chapter 4. Table 13 summarises these two models.
Model | Included predictors | Predictive value, AUC (95% CI) | Further details |
---|---|---|---|
A | Quantitative fFN + clinical risk factors (smoking, ethnicity, null parity and multiple pregnancy) | 0.89 (0.87 to 0.93) | See Table 8 |
C | Quantitative fFN + clinical risk factors + cervical length | 0.91 (0.89 to 0.94) | See Table 10 |
The quantitative fFN prognostic model (model A) used the final multivariable logistic analysis after adjustment for optimism model, whereas the cervical length prognostic model data (model C) were derived from the final multivariable logistic analysis after variable selection involving three data sets. The results from the prognostic models (the result of the linear predictor) were transformed into a percentage probability of spontaneous preterm birth within 7 days. Cost-effectiveness results are presented considering alternative admit-to-hospital decision rules based on a range of probabilities (e.g. ≥ 2% ≥ 5%, ≥ 10% and ≥ 20%) of birth within 7 days.
The probabilities of the neonatal outcomes (stillbirth, morbidity and health) were obtained from the published literature. The health utility weights attached to these health states were derived from a sample of 4016 parents whose preferences for alternative health states for their children were estimated using the standard gamble technique. 67 The standard gamble technique is considered the ‘gold standard’ for measuring preferences.
The management strategies (hospital transfer and admission to intensive care) and relative risk reduction of mortality and morbidity from antenatal corticosteroids were also obtained from the literature.
Estimating costs
There are seven main cost areas of relevance: cost of testing, hospitalisation (length of stay), hospital transfer, treatment (corticosteroids and magnesium sulphate), birth, neonatal admission and cost of death. The unit costs are detailed in Appendix 6, Table 42. The equation below illustrates the main cost components in terms of the mean total cost per patient:
Resource use estimates were identified from the literature and verified by clinical experts on the QUIDS study project and valued in monetary terms for cost year 2016, using routine UK unit cost sources. 68–70
Estimating cost-effectiveness
Our analysis is split into two distinct sections: part 1 and part 2. The aim of part 1 was to estimate the cost-effectiveness of qualitative fFN, quantitative fFN and cervical length measurement as individual tests. This was included because the use of qualitative fFN and cervical length measurement is currently recommended in NICE clinical practice guidelines. 13 These analyses explore a cost-effectiveness rationale for inclusion in the prognostic models developed. The aim of part 2 was to estimate and compare the cost-effectiveness of two different prognostic models for potential external validation in the QUIDS prospective cohort study: one including quantitative fFN and clinical risk factors, and one including quantitative fFN and clinical risk factors and cervical length measurement.
For part 1, the cost-effectiveness was calculated comparing the three diagnostic tests – (1) qualitative fFN, (2) quantitative fFN and (3) cervical length – over the 7-day time horizon to capture diagnostic accuracy, morbidity, mortality and costs to the NHS. We also include a treat-all option in which all woman who present with signs and symptoms of preterm labour are admitted to hospital for treatment (which may include antenatal corticosteroid treatment and/or magnesium sulphate). Because cervical length measurement is not available in many UK hospitals, we first compared use of qualitative fFN (ng/ml; continuous variable), treat all and quantitative fFN (positive/negative; binary outcome); we then compared separately the use of qualitative fFN (positive/negative; binary outcome), treat all and cervical length measurement (mm; continuous variable). The alternative ‘risk thresholds’ used for quantitative fFN and cervical length measurement are different risks of spontaneous preterm birth within 7 days.
For part 2, the cost-effectiveness of the alternative prognostic model strategies was calculated, comparing prognostic models developed from the IPD analysis: (1) the model with quantitative fFN and clinical risk factors (model A) and (2) quantitative fFN and clinical risk factors and cervical length (model C). The analyses aimed to determine an economic rationale for which model to include in the validation cohort study. Models A and C had similar discriminatory performance; therefore, model A was compared with model C to determine whether or not it would be likely to be cost-effective to include cervical length measurement in a prognostic model.
Results are presented in terms of incremental cost per probability of correct prognosis, incremental cost per QALD and net monetary benefit (NMB), using a willingness-to-pay threshold of £54.79 per QALD, which is equivalent to the UK lower willingness-to-pay threshold of £20,000 per QALY. 66
Probabilistic sensitivity analysis (PSA) was undertaken using a 1000-iteration Monte Carlo simulation,71 and 95% credibility intervals for each strategy are reported. Beta distributions were used in the PSA to represent uncertainty surrounding all transition probability parameters, gamma distributions were used for costs and the relative risk of antenatal corticosteroids on mortality and morbidity were assigned a log-normal distribution. Cost-effectiveness acceptability curves (CEACs)72 were calculated for each model and a value-of-information (VOI) analysis was undertaken using the Sheffield Accelerated Value of Information tool. 73 VOI analysis allows estimation of the expected value of perfect information (EVPI), that is, the monetary value of reducing the uncertainty in your model to zero. We also estimated the value of reducing uncertainty in individual model parameters to estimate the contribution of individual parameters to overall decision uncertainty. For VOI we assumed that the alternative prognostic models would be used for a conservative time period of 5 years, and the effective population over that time period was calculated using the annual number of infants born preterm in the UK (61,000)74 extrapolated over that 5-year period and discounted at 3.5%.
Results
Part 1: economic analysis of alternative diagnostic tests available in the UK
Table 14 provides a breakdown of the results for qualitative fFN compared with alternative risk thresholds obtained from quantitative fFN. We compare incrementally the cost-effectiveness of each strategy to obtain the optimal treatment strategy in which qualitative fFN, quantitative fFN and treat all are available options. Table 15 presents our comparison of qualitative fFN, treat all and cervical length measurement at alternative risk thresholds to obtain the optimal treatment strategy in which these three treatment options are available.
Test strategy | Probability of correct diagnosis | Total cost (£) | Total QALD | Incremental QALD | Incremental cost (£) | ICER (QALD) (£) | Incremental NMB (QALD) (£) |
---|---|---|---|---|---|---|---|
Treat all | 0.0765 | 1695 | 6.141 | ||||
Qualitative fFN | 0.7515 | 709 | 6.140 | –0.0003 | –123 | 488,794 | 123 |
Quantitative fFN, risk thresholda | |||||||
≥ 2% | 0.6691 | 832 | 6.141 | –0.0007 | –863 | 1,248,476 | 862 |
≥ 5% | 0.8132 | 615 | 6.140 | –0.0003 | –94 | 318,558 | 93 |
≥ 10% | 0.8648 | 533 | 6.140 | –0.0005 | –82 | 162,624 | 82 |
≥ 20% | 0.8962 | 477 | 6.139 | –0.0008 | –56 | 74,354 | 56 |
≥ 30% | 0.9086 | 447 | 6.138 | –0.0008 | –30 | 35,386 | 29 |
≥ 40% | 0.9198 | 422 | 6.137 | –0.0006 | –25 | 41,753 | 24 |
Test strategy | Probability of correct diagnosis | Total cost (£) | Total QALD | Incremental QALD | Incremental cost (£) | ICER (QALD) (£) | Incremental NMB (QALD) (£) |
---|---|---|---|---|---|---|---|
Treat all | 0.0765 | 1695 | 6.141 | ||||
Qualitative fFN | 0.7515 | 709 | 6.140 | –0.0009 | –£986 | 1,045,159 | 986 |
Cervical length, risk thresholda | |||||||
≥ 2% | 0.4107 | 1338 | 6.136 | –0.005 | £629 | –132,490 | –630 |
≥ 5% | 0.5753 | 1084 | 6.135 | –0.001 | –£254 | 241,422 | 254 |
≥ 10% | 0.7259 | 855 | 6.134 | –0.001 | –£229 | 326,032 | 229 |
≥ 20% | 0.8681 | 625 | 6.132 | –0.008 | –£84 | 10,252 | 84 |
≥ 30% | 0.8980 | 569 | 6.131 | –0.001 | –£56 | 61,768 | 56 |
≥ 40% | 0.8990 | 553 | 6.130 | –0.001 | –£15 | 15,456 | 15 |
The probability of correct diagnosis at 7 days, mean costs and QALDs, incremental costs, incremental effects, ICERs and incremental NMB are presented in full in Tables 14 and 15.
The results in Table 14 show that, in terms of cost per QALD, quantitative fFN at a ≥ 2% risk threshold is associated with a reduction of 0.007 QALDs and a cost reduction of £863 compared with a treat-all strategy. Quantitative fFN at a ≥ 2% risk threshold extended dominates qualitative fFN and quantitative fFN at all other risk thresholds. Appendix 7 details the cost-effectiveness plane and CEACs (see Appendix 7, Figures 19 and 20). In terms of the cost-effectiveness plane, quantitative fFN at a ≥ 2% risk threshold is in the south-west and south-east quadrants, which indicates that this strategy is less costly than a treat-all strategy. Although there is uncertainty as to the distribution of QALDs, the cost-effectiveness plane suggests that quantitative fFN at a ≥ 2% risk threshold is either cost saving or cost-effective compared with a treat-all strategy. The CEAC suggests that, for low values of willingness to pay for QALD gains, quantitative fFN at a ≥ 2% risk threshold has a greater probability of being cost-effective than a treat-all strategy.
At the optimal threshold for admission to hospital using quantitative fFN (a ≥ 2% risk threshold), qualitative fFN has a cost saving of £123 per person, with a reduction of 0.0003 QALDs, resulting in an ICER of > £400,000 per QALD, which is well above the NICE-recommended threshold of £54 per QALD.
In terms of cervical length testing, the results suggest that the use of qualitative fFN is the most cost-effective strategy. Compared with a treat-all strategy, qualitative fFN is cost-effective and all other strategies (including all those involving the use of cervical length measurement) are extended dominated. The cost-effectiveness plane suggests that the use of qualitative fFN is associated with lower costs than a treat-all strategy, but with considerable uncertainty surrounding differences in QALDs. The CEAC suggests that, for very low values of willingness to pay for QALD gains, qualitative fFN has a greater probability of being cost-effective than a treat-all strategy. However, as willingness to pay for QALD gains increases, this reduces to a 50% probability for each strategy. Graphical outputs of the cost-effectiveness plane and CEAC are given in Appendix 7, Figures 22 and 23.
These results provide a clear economic rationale for exploring a prognostic model including the use of quantitative fFN. Cervical length measurement was dominated by qualitative fFN in our analysis, so there is a less convincing case for inclusion of this in a prognostic model. However, as cervical length is recommended for use in current NICE guidelines,13 we have also explored the potential cost-effectiveness of the use of cervical length measurement as part of a prognostic model.
The VOI analysis found that, when comparing quantitative fFN with treat all, the EVPI associated with using quantitative fFN at a ≥ 2% probability of spontaneous preterm birth was £1365 per person at risk in the UK. This is equivalent to 0.0.068 QALDs per person in decision uncertainty when valuing uncertainty on the QALD scale. When comparing qualitative fFN with treat all, the EVPI associated with using qualitative fFN at a ≥ 2% probability of spontaneous preterm birth was estimated at £1243 per person at risk in the UK, equivalent to 0.062 QALDs in decision uncertainty when valuing uncertainty on the QALD scale.
The expected value of perfect parameter information (EVPPI) information for both of these comparisons suggests that the majority of the value of reducing parameter uncertainty in our model would be generated from reducing uncertainty around the parameters relating to test accuracy (that is, the rate of true positives, false positives, false negatives and true negatives). The relative importance of health utilities, costs, probabilities of health states and test accuracy is presented visually in Appendix 7, Figures 21 and 24. The PSA and VOI results together suggest that there is significant uncertainty about which treatment strategy is likely to be cost-effective and, hence, there is considerable value is reducing this uncertainty.
Part 2: results from economic analysis of prognostic models (quantitative fetal fibronectin model versus cervical length model)
Tables 16 and 17 provide the results for models A and C, respectively, in terms of the probability of correct diagnosis at 7 days, mean costs and QALDs, incremental costs, incremental QALDs, ICERs and incremental NMB. The prognostic model results are presented at a range of alternative risk thresholds (probability of spontaneous preterm birth within 7 days), so that the optimal risk threshold to admit to hospital can be estimated.
Test strategy | Probability of correct diagnosis | Total cost (£) | Total QALD | Incremental QALD | Incremental cost (£) | ICER (QALD) (£) | Incremental NMB (QALD) (£) |
---|---|---|---|---|---|---|---|
Treat all | 0.0765 | 1695 | 6.141 | ||||
Qualitative fFN | 0.7515 | 709 | 6.140 | 0.000 | 59 | –280,449 | –59.02 |
Model A, risk thresholda | |||||||
≥ 2% | 0.7942 | 650 | 6.141 | –0.001 | –1045 | 1,425,674 | 1044.96 |
≥ 5% | 0.8721 | 523 | 6.140 | –0.001 | –127 | 131,173 | 126.90 |
≥ 10% | 0.8996 | 472 | 6.139 | –0.001 | –51 | 64,043 | 51.16 |
≥ 15% | 0.9125 | 442 | 6.138 | –0.001 | –29 | 38,831 | 29.37 |
≥ 20% | 0.9192 | 421 | 6.137 | –0.001 | –21 | 26,310 | 20.99 |
≥ 25% | 0.9232 | 398 | 6.136 | –0.001 | –23 | 18,749 | 22.81 |
Test strategy | Probability of correct diagnosis | Total cost (£) | Total QALD | Incremental QALD | Incremental cost (£) | ICER (QALD) (£) | Incremental NMB (QALD) (£) |
---|---|---|---|---|---|---|---|
Treat all | 0.0765 | 1572 | 6.504 | ||||
Model C, risk thresholda | |||||||
≥ 2% | 1377 | 6.136 | –0.37 | –194 | 528 | 174 | |
≥ 5% | 1056 | 6.136 | 0.00 | –321 | 3,960,865 | 320 | |
≥ 10% | 833 | 6.129 | –0.01 | –223 | 30,5453 | 222 | |
≥ 20% | 666 | 6.135 | 0.01 | –167 | –27,836 | 167 | |
≥ 30% | 598 | 6.134 | 0.00 | –69 | 212,348 | 59 | |
≥ 40% | 565 | 6.134 | 0.00 | –33 | 67,478 | 33 |
Table 16 shows that, in terms of cost per QALD, model A (quantitative fFN and clinical risk factors) at a ≥ 2% risk threshold is associated with considerably lower costs and just slightly lower QALDs than a treat-all strategy, and would be considered a cost-effective strategy. Model A at a ≥ 2% risk threshold extended dominates treat all and model A at all other risk thresholds. At the optimal threshold for admission to hospital (a ≥ 2% risk threshold), model A dominates treat all, with a cost saving of £1045 per person and a loss of 0.001 QALDs.
The results (see Table 17) suggest that the use of model C (cervical length and quantitative fFN and clinical risk factors) at a ≥ 5% risk threshold is cost-effective compared with model C at a ≥ 2% risk threshold. All other strategies are extendedly dominated. At the optimal threshold for admission to hospital (a ≥ 5% risk threshold), model C has an incremental cost reduction of £321 per person and no QALD loss compared with treat all. This results in an ICER of £3.96M, far greater than the accepted NICE threshold of £54 per QALD.
Model A at a ≥ 2% risk threshold dominates qualitative fFN, with a cost reduction of £59 and an additional 0.001 QALDs; therefore, we eliminated qualitative fFN as an option and compared model A with model C. To determine the most cost-effective choice of prognostic model between model A and model C, we compared the optimal strategy involving model A with the optimal strategy involving model C. Hence, we compared model A at a ≥ 2% risk threshold with model C at a ≥ 5% risk threshold. Model A was associated with an increase of 0.005 QALDs and a cost reduction of £406 compared with model C; hence, model A dominates model C and is the more cost-effective strategy.
The cost-effectiveness plane is illustrated in Figure 6 and shows the clear increase in costs associated with model C (all points are north of the horizontal axis, showing an increase in cost), but considerable uncertainty surrounds the difference in QALDs (all points cross the vertical axis, showing both QALD gains and QALD losses). The CEAC (see Appendix 7, Figure 25) suggests that, despite this uncertainty, at the NICE willingness-to-pay threshold for QALD gains (£54 per QALD, equivalent to £20,000 per QALY), model A has 100% probability of being cost-effective. Only once willingness to pay is > £1500 per QALD does the probability that model A is the optimal strategy reduce to almost 50%.
FIGURE 6.
Cost-effectiveness plane: model A at a ≥ 2% probability of spontaneous preterm birth compared with model C at a ≥ 5% probability of spontaneous preterm birth.
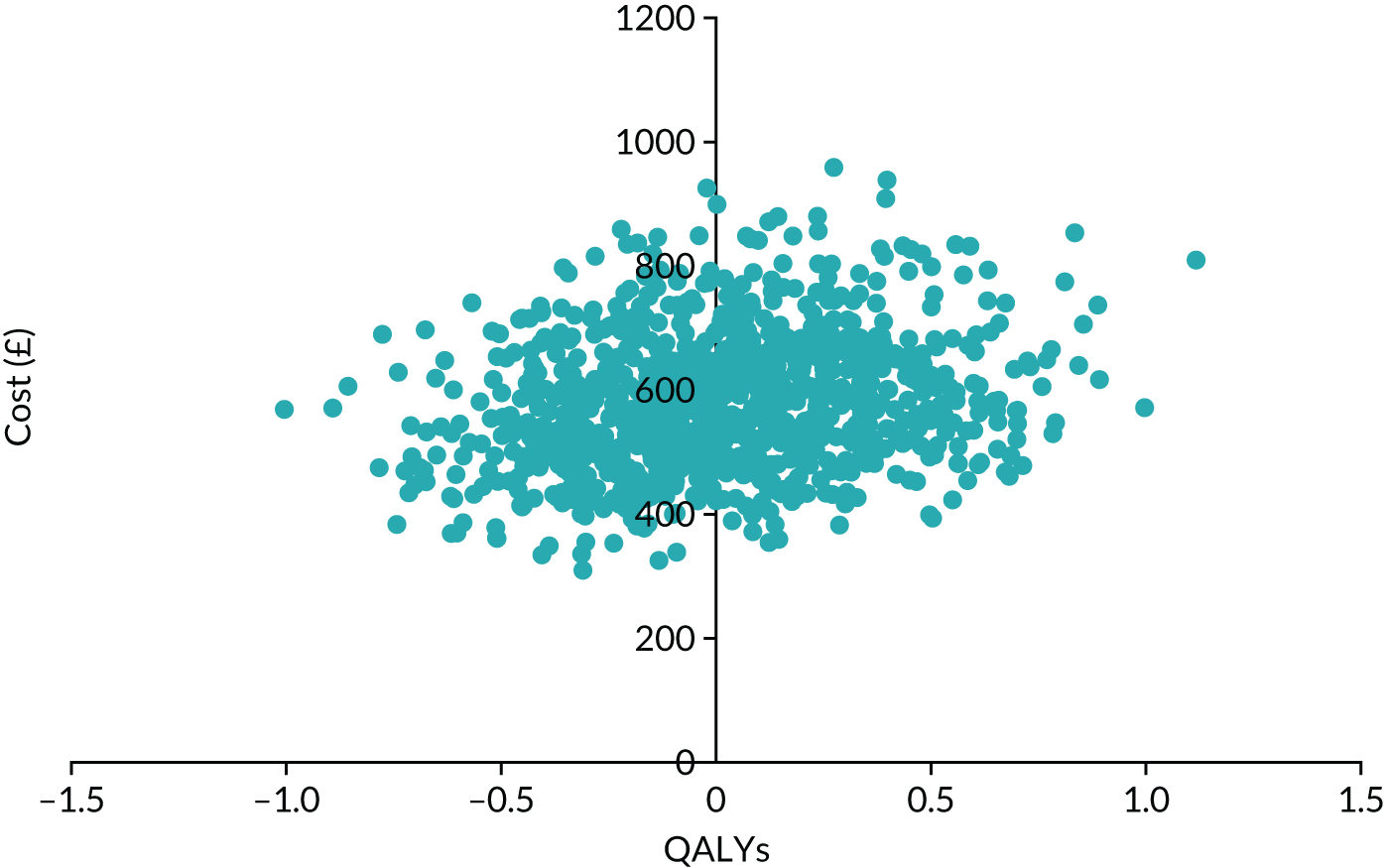
The VOI analysis found that the EVPI associated with the comparison between using model A at a ≥ 2% risk threshold with using model C at a ≥ 5% risk threshold is £1364 per person at risk in the UK. This is equivalent to 0.068 QALDs per person in decision uncertainty when valuing uncertainty on the QALD scale.
As with the individual test comparisons in part A, the EVPPI for both of these comparisons suggests that the key drivers of uncertainty in the model is related to test accuracy/prognostic value (that is, the rate of true positives, false positives, false negatives and true negatives). The relative importance of health utilities, costs, probabilities of health states, and test accuracy has been presented visually (see Figure 26). These findings show that further research into the prognostic accuracy of the test is potentially worthwhile if it were to cost less that £1364 per person.
Discussion
To our knowledge, this is the first IPD meta-analysis and cost-effectiveness analysis of alternative diagnostic strategies that include quantitative fFN for predicting spontaneous preterm birth in women with signs and symptoms of preterm labour. To our knowledge, it is also the first economic analysis of prognostic models for determining preterm labour, using predictive values for quantitative fFN and clinical risk factors (model A) and quantitative fFN and clinical risk factors and cervical length measurement (model C), developed in the IPD meta-analysis.
Strengths and weaknesses in relation to other studies
Over the 7-day time horizon, quantitative fFN had lower costs to the NHS and a greater probability of correct diagnosis than qualitative fFN, and also resulted in QALY gains. We found no previously published economic studies that included quantitative fFN. Previous studies have explored cost-effectiveness of cervical length measurement compared with usual care (risk factors and clinical judgement),13 or cost only and cost-effectiveness analyses of qualitative fFN,11,12,75,76 finding fFN testing to be cost saving in comparison with usual care. 12 Our findings corroborate this but have added new evidence that quantitative fFN is superior to qualitative fFN. The economic model adopts a structure similar to those used by other economic studies of tests for predicting preterm labour,11,12,75,76 capturing the diagnostic outcomes and the resultant clinical and economic impacts. Our literature search identified the same studies as those in a recent HTA report. 12 Inclusion of QALD in the model was of importance to account for the quality of life and mortality impacts of hospitalisation and receiving treatment to enable the analysis to capture the resultant management implications and resultant health impacts on women over the 7-day time horizon. A recent NICE overview of biomarker tests for predicting preterm labour77 also included QALY outcomes over a short time horizon and likewise found that moving from probability of diagnosis to inclusion of QALYs results in a reduction in QALYs with cost-savings for the NHS (results that reside in the south-west quadrant of the cost-effectiveness plane).
Strengths and weaknesses of the study
This study includes comprehensive and the most current research on quantitative fFN studies; limitations relate mainly to the primary data available for inclusion in our analyses. Our study excludes alternative biochemical tests that are now available (such as PartoSure and Actim Partus) because evidence on these was unavailable at the time of study commencement and they were outside the remit of the original QUIDS study. Our study has developed prognostic models for women with symptoms of preterm labour based on the six most promising prognostic strategies. These have been internally validated and have shown that model A, which includes quantitative fFN and clinical risk factors, is likely to be the most cost-effective strategy. We have found that inclusion of cervical length in a prognostic model in addition to quantitative fFN (model C) is unlikely to be cost-effective. However, there is some uncertainty about this because the data for this model come from studies performed outside the UK and in higher-risk populations. Therefore, it would also be reasonable to try to externally validate this model in a UK population.
Meaning of the study: implications for clinicians and policy-makers
Our study has several implications for clinical practice. Current NICE guidelines13 recommend the use of transvaginal ultrasonography cervical length measurement for predicting preterm birth in women at > 30 weeks presenting with signs and symptoms of preterm labour; however, a lack of specific equipment, skills and capacity around the clock means that this is rarely part of routine practice. Instead, qualitative fFN is the most commonly available test. 10 Our findings suggest that quantitative fFN in combination with clinical risk factors in a prognostic model (model A) is superior to also including cervical length (model C) in terms of probability of correct diagnosis and NMB outcomes, with a willingness to pay of £54 per QALD (equivalent to the £20,000/QALY threshold recommended by NICE). Model A was found to improve outcomes (0.005 QALD gains) compared with model C, with a cost saving of £406; hence, model A dominates model C and is the more cost-effective strategy.
Thus, it seems likely that use of the quantitative fFN prognostic model could replace the use of qualitative fFN and that efforts to implement 24-hour cervical length measurement are unnecessary. However, given these results and the uncertainty surrounding cervical length measurement included in our model, we concluded that it would be worthwhile to endeavour to include cervical length measurement in the prospective cohort study, where sites were able to do so, to strengthen the evidence base on cervical length measurement, its value within a prognostic model and the cost-effectiveness of this approach. Our findings from the economic analyses predict that a quantitative fFN prognostic model will improve predicative accuracy and reduce costs to the NHS compared with qualitative fFN.
Unanswered questions
Our analysis finds that quantitative fFN is superior to qualitative fFN, and a prognostic model of quantitative fFN and clinical risk factors is superior to a prognostic model of quantitative fFN and cervical length. The optimal choice between prognostic strategies that include quantitative fFN and cervical length measurement has considerable uncertainty and should be explored further in the UK clinical setting (i.e. in the validation cohort study). These findings provide a clinical and economic rationale for the prospective cohort study to validated the quantitative prognostic model and collect evidence on cervical length in the UK. A VOI analysis was undertaken and found that further research to reduce current uncertainty regarding the alternative prognostic strategies is worthwhile. In Chapter 6 we detail the results of this further research.
Chapter 6 Validation of the QUIDS prognostic model: prospective cohort study
Parts of this chapter are based on Stock et al. 15 © 2021 Stock et al. This is an open access article distributed under the terms of the Creative Commons Attribution License, which permits unrestricted use, distribution, and reproduction in any medium, provided the original author and source are credited.
Context
In this chapter, we describe a multicentre prospective cohort study that took place in 26 UK sites. The data collected were used to externally validate and refine the QUIDS prognostic models developed in Chapter 4, which include quantitative fFN and other clinical characteristics (risk factors) for the prediction of spontaneous preterm birth within 7 days in women presenting with signs and symptoms of preterm labour.
Methods
Ethics and registration
The study was conducted in accordance with the principles of Good Clinical Practice (GCP). The study was approved by the West of Scotland Research Ethics Committee (16/WS/0068). The study was registered with International Standard Registered Clinical/soCial sTudy Number (ISRCTN) ISRCTN41598423 and the National Institute for Health Research (NIHR) Central Portfolio Management System (CPMS31277), and the protocol has been published. 78
Population and eligibility
The prospective cohort study included women with signs and symptoms of preterm labour at 22+0 to 34+6 weeks’ gestation in whom admission, transfer or treatment for preterm labour was being considered. 78
Inclusion criteria at initial assessment were women:
-
at 22+0 to 34+6 weeks’ gestation (or earlier gestation if the fetus was considered potentially viable)78
-
with signs and symptoms of preterm labour including any or all of the following – back pain, abdominal cramping, abdominal pain, light vaginal bleeding, vaginal pressure, uterine tightenings and contractions78
-
for whom hospital admission, interhospital transfer or treatment (antenatal steroids, tocolysis or magnesium sulphate) was being considered owing to signs of preterm labour78
-
aged ≥ 16 years. 78
Additional inclusion criteria at speculum examination were:
-
cervical dilatation of ≤ 3 cm78
-
intact membranes78
-
no significant vaginal bleeding, as judged by the clinician. 78
Exclusion criteria were:
-
Contraindication to vaginal examination (e.g. placenta praevia). 78
-
Higher-order multiple pregnancy (triplets or more). 78
-
Moderate or severe vaginal bleeding. 78
-
Cervical dilatation of > 3 cm. 78
-
Confirmed rupture of membranes. 78
-
Sexual intercourse, vaginal examination or transvaginal ultrasonography in the preceding 24 hours. These factors may invalidate results. Women who meet these criteria were initially excluded from the study but could be included if still symptomatic after 24 hours, when fFN test accuracy would be considered to be restored. 78
Once it was established that a participant met the above criteria, the fFN swab was taken.
The broad inclusion criteria reflect current clinical practice and aimed to help ensure the generalisability of the results of the study for routine clinical care. We included women who reattended ≥ 7 days after initial recruitment with signs and symptoms of preterm labour, as well as women who remained symptomatic but undelivered 7 days later in whom repeat testing by the clinician was deemed to be appropriate. This is in line with manufacturer’s recommendations for fFN testing. These data have not been analysed in this QUIDS report (which has focused on predictive ability of the first quantitative fFN result), but may be used in future supplementary analyses. 78
Co-enrolment in other non-interventional studies was allowed; however, co-enrolment in trials of tocolytic treatments or other management strategies that might influence the timing of birth was not allowed because it may have affected the primary outcome of birth within 7 days of testing. Participation in the QUIDS study did not, however, preclude babies being subsequently involved in interventional trials. 78
Setting
The prospective cohort study took place in 26 consultant-led obstetric units in the UK (see Appendix 8, Table 43). More than 93% of pregnant women in the UK deliver in consultant-led units,1 and the vast majority of women with symptoms of preterm labour present to a consultant-led unit for assessment, either directly or following advice from their community midwife or general practitioner. The study did not include any community maternity units (staffed by midwives, with or without involvement of non-obstetric medical staff). Community maternity units cover only a very small proportion of the population and are located mainly in remote and rural areas. In the Perinatal Collaborative Transport Study (CoTS) of perinatal transfers in Scotland,4 which involved over 50,000 births, only 69 (0.13%) women were transferred to a consultant-led obstetric unit from a community maternity unit. Because of the small number of women cared for in community maternity units, their inclusion was not felt to be an efficient use of study resources. 78
Given that management of women with symptoms of preterm labour and interhospital transfer patterns depend partly on the level of available neonatal care and distance to transfer, we included a mixture of hospitals with different levels of neonatal care facilities in both rural and urban settings. 78 We included units with three different levels of neonatal care: SCBU (providing special care for their own local population), LNU (providing special care and high-dependency care and a restricted amount of intensive care) and NICU (larger intensive care units providing the whole range of medical, and sometimes surgical, neonatal care for their local population and for babies and their families referred from the neonatal network in which they are based, and other networks where necessary). The hospitals were chosen from different geographical settings (rural/urban) and different regions of the UK to help to ensure generalisability of findings. 78
Participant selection and enrolment
Women with signs and symptoms of preterm labour were identified on presentation to obstetric services. 78 A member of clinical staff, usually the doctor or midwife assessing the woman, identified potentially eligible participants, provided a participant information leaflet and invited consent. A suitably trained member of clinical staff (doctor or midwife) or research team was responsible for gaining consent from participants. 78
Posters and leaflets were situated in antenatal areas of participating hospitals to alert women that the study was taking place, and women were allowed as much time as possible to consider participation without unduly delaying further clinical assessment.
Screening for eligibility
The clinical likelihood of preterm birth is usually evaluated by history and examination. This includes abdominal palpation to assess the strength and frequency of uterine contractions. 78 If preterm labour is suspected, a vaginal speculum examination is performed in which the cervix is inspected for dilatation and evidence of vaginal bleeding and membrane rupture is assessed. 78 Swabs for fFN (or another biochemical test of preterm labour) are usually taken at this point, and cervical length ultrasonography is performed if the facilities are available at point of care.
Potential participants in the QUIDS study were identified after the initial assessment and provided with information about the study at this stage. A combined ‘screening and consent form’ was used as a self-screening tool for potentially eligible participants. Informed consent was provided before speculum examination. This approach meant that samples were collected at routine speculum examination, as they are in clinical practice, and participants avoided an additional vaginal examination. However, this approach meant that certain exclusion criteria could be applied only at speculum examination (e.g. vaginal bleeding or evidence of ruptured membranes), so a proportion of women were not be eligible for fFN testing after consent was given. These data are reported but not included in analysis. Women were also able to withdraw consent for use of their data at any time until the end of the study. 78
Study assessments and data collection
Study assessments are shown in Appendix 9, Table 44. Baseline demographics were collected on participants, including height and weight (at booking), information on medical history, obstetric history, estimated date of birth and presenting signs and symptoms. Samples for fFN analysis were taken with a fFN specimen collection kit, as per the manufacturer’s instructions. 16 The sample was run at a near-bedside Rapid fFN 10Q analyser specially adapted for the QUIDS study, which revealed a qualitative fFN result (positive/negative/invalid based on a threshold of 50 ng/ml) for clinicians to base clinical decision-making on, in accordance with local protocols. 78 The quantitative fFN result was masked from clinicians and stored as a three-letter code. 78 Samples were run as per the manufacturer’s instructions (described in Chapter 2). Hologic, Inc. offered training on sample collection and analysis to staff at sites participating in the study.
Screening data and data on quantitative fFN were collected on paper-based case report forms (CRFs), which were then inputted into a web-based electronic database by research staff. 78 All other data were collected from the participant records and recorded on the study database.
Data were collected on the following candidate predictors: fFN concentration, previous spontaneous preterm labour, gestation at fFN test, age, ethnicity, BMI, smoking, deprivation index (derived from postcode), number of uterine contractions in 10 minutes, cervical dilatation, vaginal bleeding, previous cervical treatment for CIN, cervical length (measured by transvaginal cervical length when available), singleton/multiple pregnancy, tocolysis and fetal sex. 78
Although we wanted to explore the added prognostic value of cervical length in our model validation, and we purposively selected sites that reported that facilities for cervical length were available, we did not make cervical length measurement mandatory. Doing so would have made the study extremely difficult to implement in the concurrent NHS setting. The majority of units do not have 24-hour availability of transvaginal ultrasonography and/or trained personnel to perform scans at point of care when women present with symptoms of preterm labour. Inclusion of cervical length could also have decreased recruitment rate (owing to the need for additional transvaginal ultrasonography examination) and would have required significant additional resources. Data on cervical length were thus, collected only when available.
Outcome and resource use data included78 gestational age at birth, date and time of birth, administration of treatments for preterm labour (steroids, antibiotics, tocolysis or magnesium sulphate), duration of hospital admission, hospital transfer, onset of labour [preterm prelabour rupture of membranes (PPROM); idiopathic preterm birth; medically indicated preterm birth (and indication)], place of birth (base hospital, other hospital or not in hospital), mode of birth, neonatal admission, neonatal complications, perinatal mortality, congenital anomaly, sex and birthweight.
Additional data were collected on alcohol use, employment group and education level, domestic violence, preterm labour symptoms and cervical dilatation.
At recruitment, participants were invited to complete an optional baseline State-Trait Anxiety Inventory (STAI) questionnaire and were provided with a second questionnaire to be completed 24–48 hours later (with a prepaid envelope to be returned by post). Acceptability of fFN testing and the decision support were assessed using follow-up interviews with a subgroup of participants (n = 30),78 which are detailed in Chapter 8.
Quality assessments
The Rapid fFN 10Q analyser has integrated quality control measures; records of these and staff training were kept. A precalibrated reusable quality control cassette was used to verify that analyser performance was within specification. Quality control checks were mandatory, that is a test sample would not be analysed if quality control had not been performed in the preceding 24 hours. Logs of results were stored on the machine and monthly paper logs of quality control tests were also kept. 78 Each fFN test has an internal quality control: a procedural control line that checks the threshold level of signal by the instrument. Sample flow detection ensures that the sample travels across the cassette properly and confirms absence of conjugate aggregation. All participating sites were requested to also enrol in the Wales External Quality Assurance Scheme (WEQAS) point-of-care quality assurance scheme. 69 The WEQAS provided a sample for analysis to each site bimonthly and provided confirmation of analyser performance and variability.
Sample size calculation
Our initial sample size calculation (n = 1602) was based on an anticipated event rate of between 6% and 12%. However, it quickly became apparent that our event rate was lower than estimated (stabilising at around 3%). Furthermore, new guidance emerged recommending a minimum of around 100 events and 100 non-events for prognostic model validation. 79 Therefore, we revised our sample size calculation during the study, aiming for 3000 participants, to obtain approximately 100 events of preterm birth within 7 days of testing.
Validation of the QUIDS prognostic model
A statistical analysis plan was drawn up and agreed prior to database lock and analysis.
Definitions
The primary end point mirrored that of the prognostic models developed in Chapter 4: spontaneous preterm birth within 7 days of the first recorded quantitative fFN in women with symptoms and signs of preterm labour. Spontaneous preterm birth within 7 days was defined as birth within 7 days of quantitative fFN (i.e. 7 × 24 hours from the time of quantitative fFN recorded on the study database) preceded by the spontaneous onset of contractions or spontaneous PPROM, with birth occurring at < 36 weeks’ gestation (women were eligible only if they had quantitative fFN at < 35 weeks’ gestation).
In women with twin pregnancies, the primary outcome pertained to the timing of the birth of the first twin. A prespecified subgroup analysis was performed including singletons only.
We excluded all medically indicated births within 7 days of quantitative fFN for the purposes of the primary analysis (because we could not determine if spontaneous birth would have happened within 7 days had medically indicated birth not taken place). However, we prespecified sensitivity analyses to include all preterm births within 7 days of quantitative fFN (i.e. spontaneous and medically indicated births within 7 days) because (1) in some cases it was difficult to determine if medically indicated birth had in fact been preceded by a spontaneous onset of labour and (2) the clinical and cost implications of predicting preterm birth within 7 days are similar regardless of the type of labour onset.
When quantitative fFN was performed multiple times, the first recorded quantitative fFN result was used in the model, mirroring model development. However, we prespecified sensitivity analyses to include all quantitative fFN results (i.e. considering each test as a separate episode).
Data cleaning
Prior to analysis, data were checked for outliers and missing data were identified. Descriptive statistics were used to summarise the data for women who were included in the cohort study. All events and non-events were verified by assessing the interval between quantitative fFN and birth. The time of the quantitative fFN and the time of birth recorded in the database were verified with source data for 50 women, with 100% concordance.
A number of women (n = 38) recruited from two centres (St Thomas’ Hospital and University College London) participated in QUIDS2 (see Chapter 9) but did not participate in the QUIDS study. For these women, quantitative fFN was performed as part of their routine clinical care and the result was not masked from their caregivers. These women did not contribute data to the QUIDS analysis.
Missing data
To maximise recruitment and our event rate, we increased the recruitment period and reduced the amount of time for follow-up data collection. Thus, we confirmed event status for all recruits after the close of the study (i.e. birth within 7 days of quantitative fFN: yes/no); however, because some pregnancies were ongoing, we did not have final birth dates or outcomes for all women and babies at the time of first database lock (14 January 2019). These data are being collected wherever possible and an updated database with birth and outcome details will be created for potential future analyses.
Multiple imputation by chained equations was used to impute missing values to avoid excluding participants from the analysis,47 as described in Chapter 4. Eight multiple-imputation data sets were created. Models were fitted to each data set and the estimates were pooled using Rubin’s rules into a single set of estimates and CIs.
Choice of models for external validation
A total of six prognostic models relating to the primary outcome were developed in the IPD meta-analysis, four of which included cervical length (see Chapter 4). Despite purposively sampling sites that reported facilities for cervical length measurement, the number of participants who had cervical length measured was very low (n = 98), and so we were unable to externally validate these models in the prospective cohort study data.
Two models were developed in the IPD meta-analysis using quantitative fFN and clinical risk factors without cervical length. Because both models had very similar discrimination, and the most parsimonious model is generally advantageous in clinical settings, we prespecified that the model including selection of variables would be used in our primary external validation (model A; see Table 8). We also externally validated the alternative model [which was developed without variable selection (model B; see Table 7)] for comparison.
External validation
The continuous variable fFN concentration was transformed (square root), as it was in the developed model (model A), because of non-linearity in the IPD-level-analysis data set. Zero values were dealt with by use of the following formula:
The models developed in Chapter 4 included individual intercepts for each study, with the application of these models requiring the user to choose a particular intercept or to use their own. To address this in model validation, we decided to perform a random effects meta-analysis of the intercepts from the five studies included in the IPD-level model development data set, and used this estimated pooled intercept as the intercept in the model equations externally validated the prospective cohort data.
Several measures of performance for the validated models were assessed using a methodology similar to that described in Chapter 4. Discrimination (how well the model distinguishes between women who have an event and women who do not have an event) was measured by the AUC. Nagelkerke R2 was used to assess overall goodness of fit. Calibration (agreement between the predicted probability of having an event and the number of observed events) was assessed using calibration-in-the-large and calibration plots with data plotted across tenths of predicted risk.
Recalibration
Because the outcome proportion was substantially lower in the validation data set than in the development data set, recalibration of the models was performed on the external validation data set using the following technique. A new covariate, LP1, was created for each participant in the prospective cohort study, which was the predicted logit risk score of spontaneous preterm birth in the model (i.e. the model excluding the intercept). The intercept was updated to correct calibration-in-the-large, thereby equating average predicted probability with the observed overall event rate using:
A logistic regression model was then fitted in the cohort study data set with the intercept, αnew, as the only free parameter and the linear predictor as an offset variable, where the slope of the linear predictor is fixed at 1. 54
Sensitivity analyses and subgroup analyses
We prespecified that our primary external validation would be performed on the most parsimonious model (i.e. the model after variable selection as opposed to the model including all candidate predictors: model A; see Table 8), developed from the IPD data set with the primary end point of spontaneous preterm birth within 7 days, using the first recorded quantitative fFN result.
The following, secondary, sensitivity analyses and subgroup analyses were undertaken:
-
secondary analyses
-
external validation of the model for spontaneous preterm birth within 7 days of the first recorded quantitative fFN result, which includes all potential candidate predictors (model B; see Table 7)
-
-
sensitivity analysis
-
external validation of the model for any preterm birth within 7 days of quantitative fFN
-
external validation of the model for spontaneous preterm birth within 7 days of the first recorded quantitative fFN in women with singleton pregnancy (i.e. excluding women with multiple pregnancy)
-
spontaneous preterm birth within 7 days of the first recorded quantitative fFN result using complete case analysis
-
-
net benefit analysis
-
performed as described in Chapter 4 for model A and model B.
-
Results
Women were recruited to the study between 22 June 2016 and 31 October 2018 inclusive, at 26 sites (see Appendix 8, Table 43).
A flow chart of study participants is shown in Figure 7. In total, 27 out of 2968 (0.91%) initial participants were found to be ineligible at speculum or were unable to have fFN testing completed for other reasons. A total of 17 out of 2941 (0.58%) fFN tests had an invalid result. A total of 2924 women were included in the final analysis data set.
FIGURE 7.
Flow chart of the QUIDS cohort study participants.
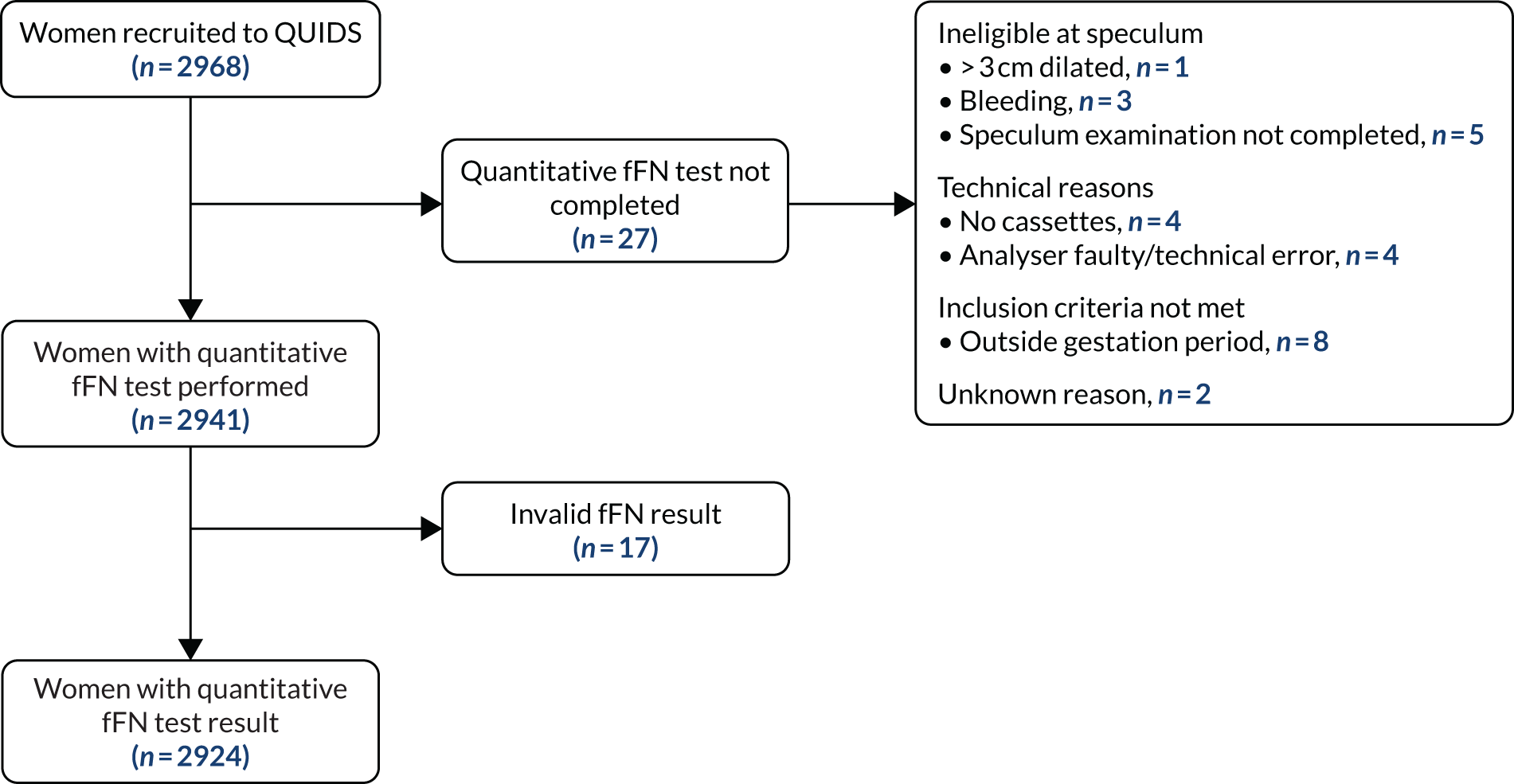
Summary statistics for the baseline participant characteristics are shown in Table 18, including the levels of missing data.
Variable | QUIDS prospective cohort study (N = 2924) | Missing, n (%) |
---|---|---|
Baseline characteristics | ||
Age (years), mean (SD) | 28.2 (5.7) | 31 (1.1) |
BMI (kg/m2), median (IQR) | 25.4 (22.2–30.2) | 51 (1.7) |
Ethnicity, n (%) | 42 (1.4) | |
White | 2544 (87.0) | |
South Asian | 165 (5.6) | |
East Asian | 7 (0.2) | |
African, Caribbean, Middle-Eastern | 98 (3.4) | |
Other | 68 (2.3) | |
Current smoker, n (%) | 608 (20.8) | 40 (1.4) |
Nulliparous, n (%) | 951 (32.5) | 171 (5.8) |
Multiple pregnancy, n (%) | 99 (3.4) | 29 (1.0) |
Gestation (weeks), median (IQR) | 31.0 (27.9–33.1) | 10 (0.3) |
Previous spontaneous preterm birth at < 34 weeks’ gestation, n (%) | 121 (4.1) | 179 (6.1) |
Qualitative fFN: positive, n (%) | 413 (14.1) | 1 (< 0.1) |
Quantitative fFN (ng/ml), median (IQR) | 7 (4–22) | 2 (< 0.1) |
Tocolysis, n (%) | 165 (5.6) | 78 (2.7) |
Cervical length measured, n (%) | 98 (3.4) | – |
Outcomes, n (%) | ||
Spontaneous preterm birth within 7 days of test | 85 (2.9) | – |
Any preterm birth within 7 days of test | 95 (3.2) | – |
Spontaneous preterm birth within 48 hours of quantitative fFN | 42 (1.4) | – |
Any preterm birth within 48 hours of quantitative fFN | 43 (1.5) | – |
In total, there were 85 events of spontaneous preterm birth within 7 days of fFN test in 2924 women presenting with signs and symptoms of preterm labour (2.9%).
Levels of missing data were < 2% for the majority of variables. A total of 171 (5.8%) women had a previous pregnancy recorded in the CRF but had provided no details regarding pregnancy outcome (miscarriage, termination of pregnancy or gestation at birth), so we were unable to determine whether or not these women were nulliparous. Data were thus recorded as missing. In addition, we could not determine whether or not there was a previous preterm birth in these women, along with 12 more women (total missing data, 183 cases; 6.3%).
For multiple imputation of predictors, we based the number of imputed data sets on the largest proportion of incomplete cases, which was 8%; therefore, eight imputed data sets were created. Appendix 10, Table 45, shows the characteristics of the QUIDS cohort study based on the pooled means of imputed data sets, alongside those of the QUIDS IPD analysis for comparison.
Validation of models for preterm birth within 7 days of test
Table 19 shows external validation of models A (after variable selection) and B (including all variables). The c-statistic for model A (pooled from the multiple-imputation data sets) was 0.89 (95% CI 0.85 to 0.93). The c-statistic for model B before variable selection (pooled from the multiple-imputation data sets) was the same at 0.89 (95% CI 0.85 to 0.93). Figures 8–11 show the calibration plots for models A and B before and after recalibration with updated intercepts. The majority of participants in the study had a very low risk of preterm birth within 7 days, and the majority of events happened in the highest decile of risk. The calibration plots indicate that the models were underfitted, with a higher proportion of observed events than expected events (see Figures 8 and 10). Recalibration with update of the intercept improved the calibration, as shown by a calibration-in-the-large close to 0 and a calibration slope close to 1 (see Figures 9 and 11).
Variable | Model B (all variables) | Model A (after variable selection and shrinkage) | ||
---|---|---|---|---|
Intercept | –8.672 | –5.352 | ||
Variable | Model B (all variables) | Model A (after variable selection and shrinkage) | ||
Beta | OR (95% CI) | Beta | OR (95% CI) | |
Quantitative fFN: [(quantitative fFN + 1)/100]0.5 | 2.033 | 7.64 (5.68 to 10.28) | 1.888 | 6.61 (4.92 to 8.87) |
Age (years) | 0.024 | 1.02 (0.98 to 1.07) | – | – |
BMI (kg/m2) | 0.018 | 1.02 (0.96 to 1.08) | – | – |
Smoking | –0.656 | 0.52 (0.24 to 1.13) | –0.674 | 0.51 (0.24 to 1.08) |
Ethnicity | ||||
White | Reference | Reference | ||
South Asian | 1.066 | 2.90 (0.93 to 9.10) | 0.943 | 2.57 (0.84 to 7.88) |
East Asian | –1.184 | 0.31 (0.04 to 2.49) | –1.005 | 0.37 (0.05 to 2.77) |
African, Caribbean, Middle-Eastern | –0.216 | 0.81 (0.42 to 1.54) | –0.207 | 0.81 (0.43 to 1.52) |
Other | –0.252 | 0.78 (0.20 to 3.00) | –0.305 | 0.74 (0.19 to 2.82) |
Nulliparity | 0.527 | 1.69 (1.06 to 2.71) | 0.364 | 1.44 (0.92 to 2.24) |
Multiple pregnancy | 0.852 | 2.34 (1.35 to 4.07) | 0.832 | 2.30 (1.35 to 3.92) |
Previous spontaneous preterm birth at < 34 weeks’ gestation | 0.427 | 1.53 (0.78 to 3.03) | – | – |
Gestational age (weeks) at assessment | 0.031 | 1.03 (0.96 to 1.11) | – | – |
Performance measures (before any recalibration) | ||||
AUC (95% CI) | 0.89 (0.85 to 0.93) | 0.89 (0.85 to 0.93) | ||
Calibration-in-the-large | 1.188 | 0.288 | ||
Calibration slope | 1.102 | 1.204 | ||
Performance measures after recalibration of the intercept | ||||
Intercept | –7.484 | –5.064 | ||
AUC (95% CI) | 0.89 (0.85 to 0.93) | 0.89 (0.85 to 0.93) | ||
Calibration-in-the-large | 1.14 × 10–14 | 6.42 × 10–14 | ||
Calibration slope | 1.102 | 1.2041 |
FIGURE 8.
Calibration plot with predicted vs. observed risk for model A (after variable selection) as applied to the prospective cohort study data (after multiple imputation; multiple imputation set 6 used to generate plot). LOWESS, locally weighted scatterplot smoothing.
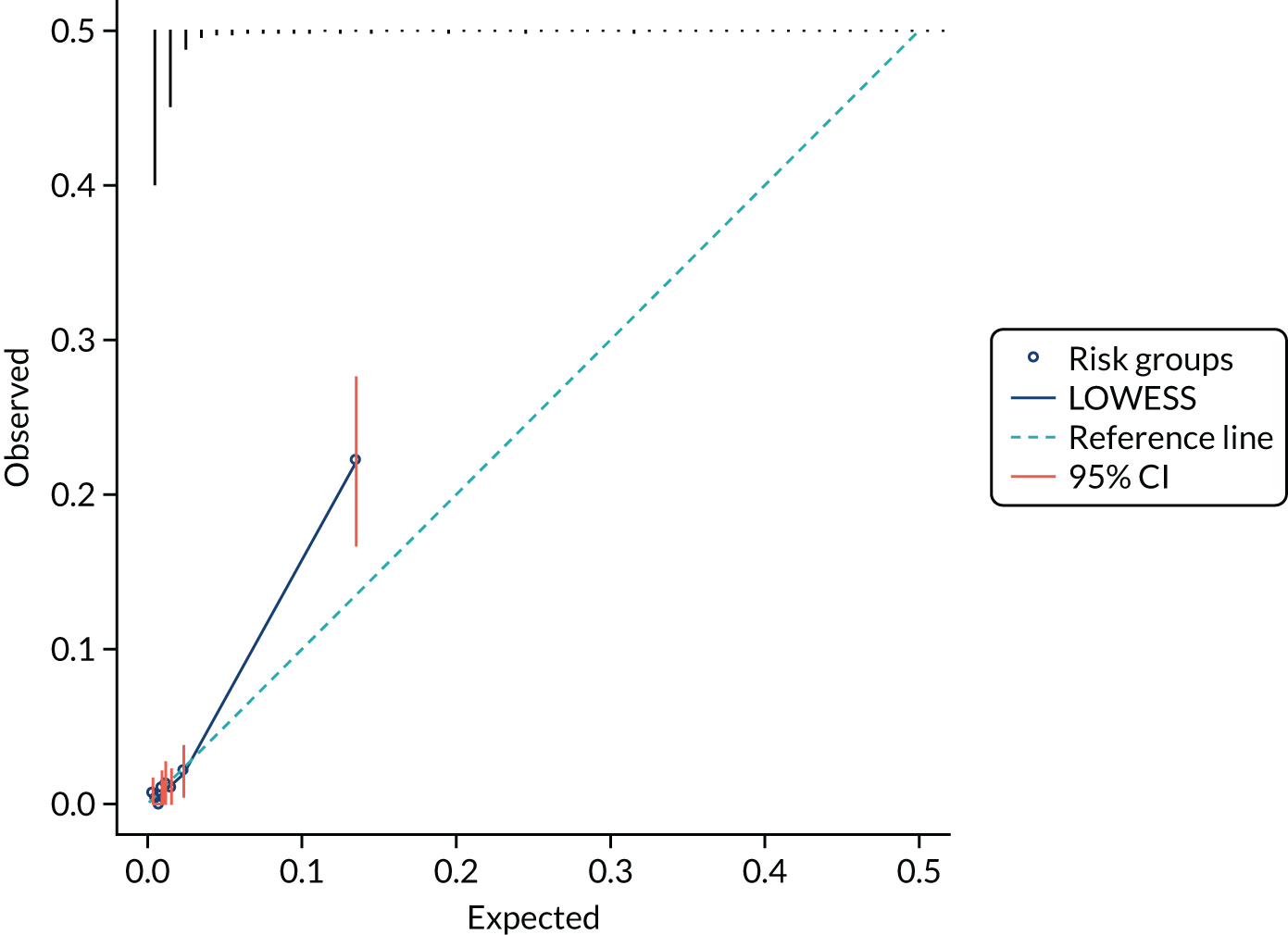
FIGURE 9.
Calibration plot with predicted vs. observed risk for model A (after variable selection) as applied to the prospective cohort study data (after multiple imputation; multiple imputation set 6 used to generate plot) after recalibration with updated intercept. LOWESS, locally weighted scatterplot smoothing.
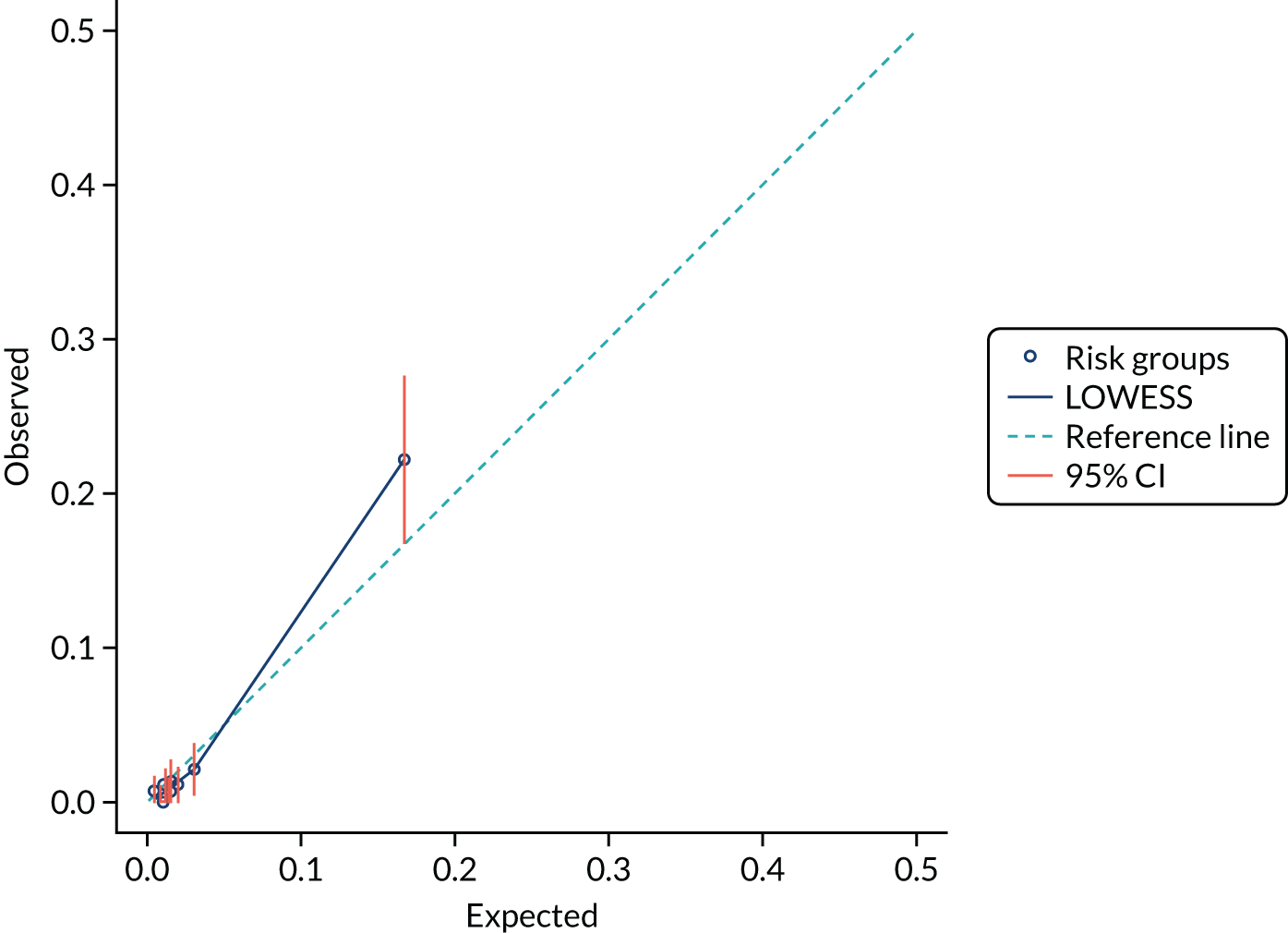
FIGURE 10.
Calibration plot with predicted vs. observed risk for model B (all variables) as applied to the prospective cohort study data (after multiple imputation; multiple imputation set 6 used to generate plot). LOWESS, locally weighted scatterplot smoothing.
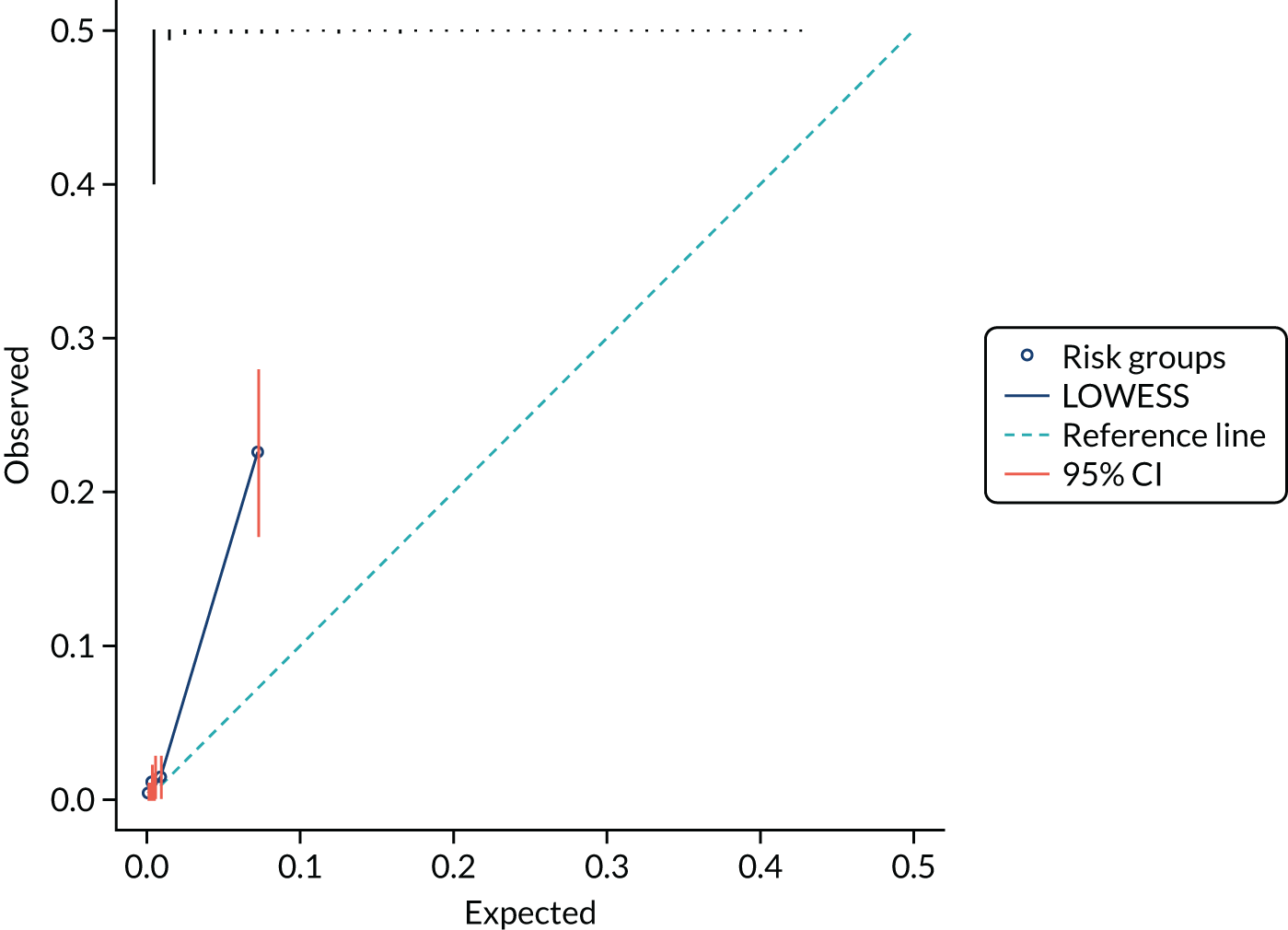
FIGURE 11.
Calibration plot with predicted vs. observed risk for model B (all variables) as applied to the prospective cohort study data (after multiple imputation; multiple imputation set 6 used to generate plot) after recalibration with updated intercept. LOWESS, locally weighted scatterplot smoothing.
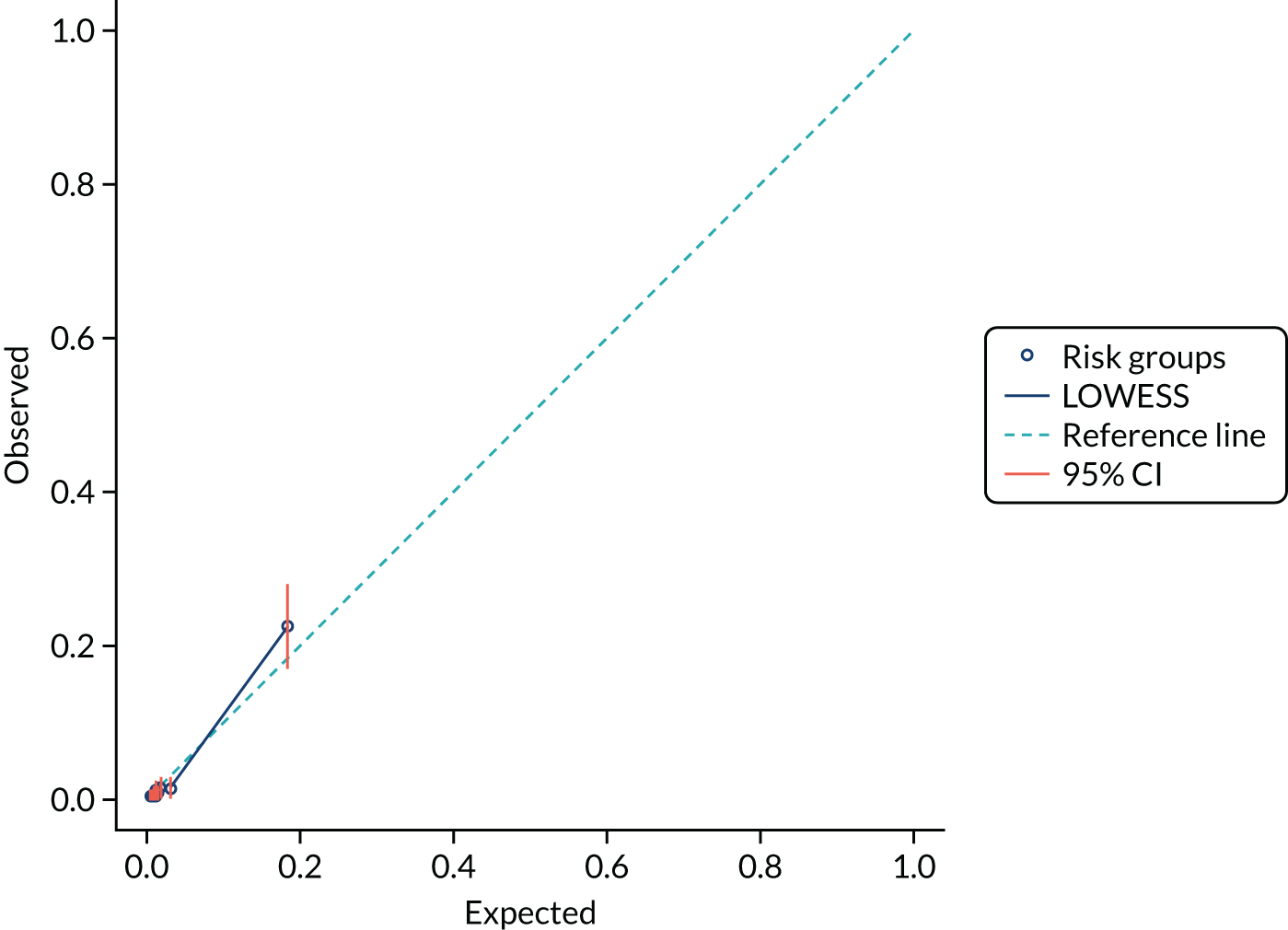
Sensitivity analyses
The model performance measures of models included in prespecified sensitivity analyses are presented in Appendix 11, Table 46, alongside those of the primary analysis for comparison. Model performance was similar across primary and sensitivity analyses.
Net benefit analysis
Figure 12 shows the net benefit of models A and B compared with treat-all and treat-none strategies. As would be expected, both models appear to have very similar net benefits, which are better than treat all or treat none up to a threshold of 20% risk.
FIGURE 12.
Net benefit curves for externally validated models A and B plotted across a range of thresholds used to identify women at low risk of preterm birth within 7 days. Treat all and treat none are plotted for comparison. All, treat all; none, treat none.
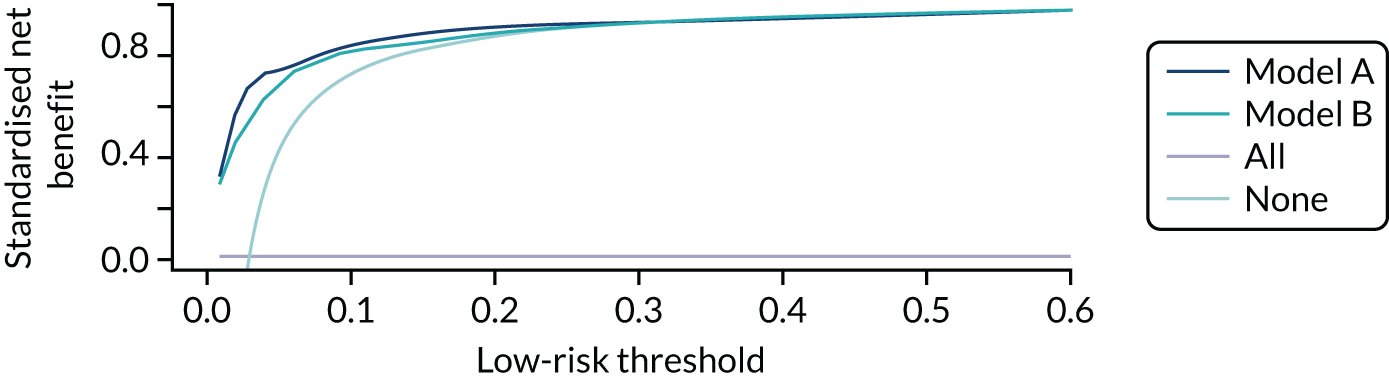
Development and internal validation of model for spontaneous preterm birth within 48 hours of test
We combined the original model development cohort with the prospective cohort in a new IPD meta-analysis for development and internal validation of a model for spontaneous preterm birth within 48 hours.
The baseline characteristics are shown in Table 20.
Predictor | Spontaneous preterm birth within 48 hours | ||
---|---|---|---|
Yes (N = 113; 2.4%) | No (N = 4594; 97.6%) | Yes or no (N = 4707; 100%) | |
Age (years), mean (SD) | 30.5 (5.6) | 28.7 (5.7) | 28.8 (5.7) |
BMI (kg/m2), median (IQR) | 24.3 (21.6–29.7) | 25.2 (22.1–29.7) | 25.2 (22.1–29.7) |
Ethnicity, n (%) | |||
White | 88 (77.9) | 3579 (77.9) | 3667 (77.9) |
South Asian | 5 (4.4) | 233 (5.1) | 238 (5.1) |
East Asian | 1 (0.9) | 49 (1.1) | 50 (1.1) |
African/Caribbean/Middle-Eastern | 12 (10.6) | 449 (9.8) | 461 (9.8) |
Other | 0 (0) | 135 (2.9) | 135 (2.9) |
Smoker, n (%) | 5 (4.4) | 793 (17.3) | 798 (17.0) |
Nulliparity, n (%) | 68 (60.2) | 1763 (38.4) | 1831 (38.9) |
Previous spontaneous preterm birth, n (%) | 13 (11.5) | 304 (6.6) | 317 (6.7) |
Multiple pregnancy, n (%) | 28 (24.8) | 257 (5.6) | 285 (6.1) |
Gestational age (weeks), median (IQR) | 31.1 (27.9–33.1) | 30.4 (27.1–32.7) | 30.4 (27.1–32.7) |
Qualitative fFN: positive, n (%) | 91 (80.5) | 870 (18.9) | 961 (20.4) |
Quantitative fFN (ng/ml), median (IQR) | 342.0 (84.0–500.0) | 8.0 (4.0–30.0) | 8.0 (4.0–33.5) |
Table 21 shows the logistic model after adjustment for optimism (uniform shrinkage factor of 0.93). The final model identified that high quantitative fFN levels, gestational age at assessment (weeks), age, smoking, nulliparity, multiple pregnancy and previous spontaneous preterm birth at < 34 weeks’ gestation were all predictors.
Test strategy | 48-hour model after variable selection and shrinkage | |
---|---|---|
Intercept | 95% CI | |
Study | ||
1 (APOSTEL-1)60 | –9.492 | –12.242 to –6.741 |
2 (EUFIS)61 | –10.019 | –12.818 to –7.220 |
3 (EQUIPP)59 | –10.080 | –12.890 to –7.271 |
4 (QFCAPS) | –9.380 | –12.580 to –6.179 |
5 (UCLH/Whit) | –9.851 | –12.803 to –6.899 |
6 (QUIDS cohort) | –9.941 | –12.761 to –7.122 |
Beta | OR (95% CI) | |
log[(quantitative fFN + 1)/100] | 0.980 | 2.665 (2.273 to 3.124) |
Gestational age (weeks) at assessment | 0.127 | 1.136 (1.055 to 1.222) |
Age (years) | 0.034 | 1.035 (0.996 to 1.075) |
Smoking | –1.430 | 0.239 (0.094 to 0.607) |
Nulliparity | 0.748 | 2.112 (1.304 to 3.422) |
Multiple pregnancy | 1.122 | 3.070 (1.815 to 5.191) |
Previous spontaneous preterm birth at < 34 weeks’ gestation | 0.690 | 1.994 (0.965 to 4.123) |
Apparent predictive performance | ||
Nagelkerke R2 | 0.27 | |
AUC | 0.90 (95% CI 0.87 to 0.93) |
Discussion
In this chapter, we describe a UK prospective cohort study and external validation of the prognostic models including quantitative fFN and clinical risk factors, developed from our IPD meta-analysis (see Chapter 4), to predict spontaneous preterm birth within 7 days in women presenting with symptoms of preterm labour.
Our primary aim was to validate the most parsimonious model (model A), which includes quantitative fFN and four other clinical predictors. We also validated model B, which includes quantitative fFN and eight clinical predictors. Both models had an identical c-statistic: 0.89 (95% CI 0.85 to 0.93). This is remarkably similar to the development cohort c-statistic of 0.89 (95% CI 0.87 to 0.93) (for model A) and 0.90 (95% CI 0.88 to 0.93) (for model B) and indicate excellent discrimination.
We used a random-effects meta-analysis of the intercepts of the five studies included in the model development cohort to estimate the intercept for external model validation. Using these intercepts, we found that model calibration was suboptimal, with calibration plots indicating underfitting of the models. Updating of the intercept for the UK prospective cohort improved the model calibration, and this is the final model we present. Although the models still appeared to slightly underestimate the risk of preterm birth within 7 days at the highest decile of risk, the lower bound of the 95% CI reached the calibration reference line.
We recognised that cervical length measurement is not routinely part of the assessment of women with threatened preterm labour in the UK10 despite current NICE guidelines,13 and this was supported in our study. Despite preferentially recruiting centres that reported that they offered cervical length measurement for women with signs and symptoms of preterm birth, only a small minority of women had cervical length measured (98 women; 3.4%). The model we have validated does not include cervical length and still appears to have good discrimination; thus, it can be readily implemented in the UK.
The majority of women participating in the QUIDS prospective cohort study were at low risk of preterm birth, with the majority of events occurring at the highest decile of risk, which equated to a ≈ 20% chance of preterm birth with 7 days. It is likely that clinicians would treat on a lower risk threshold than this.
The primary end point of the prognostic model was spontaneous preterm birth within 7 days of testing, as influenced by QUIDS qualitative (see Chapter 3), which included focus group consultation to determine the decisional needs of women, their partners and clinicians. A secondary analysis using the end point of birth within 48 hours was also deemed important by women and clinicians. We developed this secondary model by combining the IPD meta-analysis data set with the prospective cohort data set in a new meta-analysis, which is preferable to maximise the number of events. 63 This model perfomed excellently but needs further validation before clinical use.
Strengths of this work include that we used a published protocol,41 in accordance with guidelines. 38–40 Potential limitations include the number of events. We aimed to have at least 100 births within 7 days of testing, but the event rate was lower than anticipated and we had a higher proportion of medically indicated preterm births (a prespecified exclusion criterion in our analysis) than expected. Nevertheless, the rates of preterm birth are comparable with other similar studies, and to our knowledge this is the largest published study of quantitative fFN to date. A potential limitation is the number of missing data for certain variables. Although levels were generally low, parity and previous preterm birth had > 5% missing data. This was because of a problem with the database setup, in which it was possible to record a previous pregnancy without specifying gestational age; thus, we were unable to be sure of parity (pregnancy resulting in a live birth or stillbirth after 24 weeks’ gestation) in all cases. We have noted this for future studies. Multiple imputation was used to address missing data, which has been shown to be a valid technique for dealing with missing data in logistic regression models, resulting in less bias than excluding all women with missing data. 47
Another limitation is that the CIs are notably wide around risks associated with certain ethnicities. This limits the prognostic models use in non-white women. In future work, we would like to further refine estimates of different ethnicities as predictors of preterm birth.
Prespecified sensitivity analyses were performed and indicated stability of the model with very similar discriminatory performance across all sets of conditions. These did not indicate that any changes should be made in our model inclusion criteria.
Net benefit analysis showed similar clinical value of model A, which was more parsimonious, and model B, which contained more clinical risks. The traditional view is that the more parsimonious model is preferable in clinical settings, because, with fewer risk factors included, it is simpler to use. Nevertheless, it is possible that clinicians and women will have more confidence in a model that contains variables that they believe may influence preterm birth risk, even if those variables do not actually improve the model performance. An example of this is previous preterm birth, which is not prognostic of birth within 7 days in women with symptoms but is a risk factor for preterm birth overall. This will be explored in further qualitative work.
In summary, the models for spontaneous preterm birth within 7 days, based on clinical risk factors and quantitative fFN, that were developed in our IPD meta-analysis (see Chapter 4) were externally validated in a pragmatic prospective cohort study across a range of geographical settings. The models perform very well in terms of discrimination and, after updating the intercept for the UK data, have reasonable calibration. We propose use of the more parsimonious model (model A), which appears to have clinical value in helping to determine the management for women who present with symptoms of preterm labour, in particular reducing unnecessary treatment.
Chapter 7 Economic evaluation of the QUIDS prognostic model
Parts of this chapter are based on Stock et al. 15 © 2021 Stock et al. This is an open access article distributed under the terms of the Creative Commons Attribution License, which permits unrestricted use, distribution, and reproduction in any medium, provided the original author and source are credited.
Context
In this chapter, we describe the economic evaluation of the QUIDS prognostic model (which includes quantitative fFN and other clinical risk factors) compared with qualitative fFN for the prediction of spontaneous preterm birth within 7 days. As detailed in Chapter 6, the QUIDS prognostic model has been validated in 26 maternity units in the UK in a prospective cohort study. During the prospective cohort study we collected data on resource use associated with women presenting at a hospital setting with signs and symptoms of preterm labour. We then combined these resource use data with the prognostic model performance data derived from the cohort study, and used this to estimate the cost and health outcomes associated with a decision to treat at alternative thresholds of probability of spontaneous preterm birth within 7 days.
There were two primary aims to this economic analysis of the QUIDs cohort study:
-
Estimate the resource use for treatment of a woman with signs and symptoms of preterm labour based on data from the prospective cohort study.
-
Evaluate the cost-effectiveness of alternative prognostic strategies (i) over a 7-day time horizon reporting outcomes as incremental cost QALD and NMB and (ii) over a lifetime horizon to account for the longer-term cost and quality-of-life impacts. The key drivers for this are the cost and quality-of-life impacts from morbidity directly related to not getting steroid treatment for preterm labour. Outcomes of the lifetime analysis will be reported as incremental cost per QALY gained.
Methods
The economic evaluation was undertaken from the perspective of the UK NHS and Personal Social Services. The analysis was undertaken for cost year 2017/18 and was conducted in accordance with best practice guidelines. 64,66 The base-case economic evaluation used a decision-analytic model to assess the costs and health outcomes associated with the prognostic model (model A, which includes quantitative fFN plus clinical risk factors) compared with qualitative fFN over (1) a 7-day time period, in line with the primary study outcome, and (2) over a lifetime horizon to account for relevant morbidities associated directly with not receiving treatment (corticosteroids and magnesium sulphate) for preterm labour. The primary outcome of the QUIDS study was spontaneous preterm birth within 7 days of a fFN test. This is considered to be a clinically important time point6 and was indicated as being important to women in QUIDS qualitative (see Chapter 3). Resource use data from the cohort study were collected and the mean cost for a woman admitted to hospital with signs and symptoms of preterm labour over a 7-day time horizon was estimated. Discounting of costs and health outcomes was not necessary for the 7-day analysis, but for the lifetime analysis costs and outcomes were discounted at 3.5% as per guidelines. 66
Resource use and cost estimates
The following resource items were collected in the cohort study: maternal admission, neonatal admissions, complications, transfers and treatments given. The equation below illustrates the main cost components in terms of the total mean cost per patient:
Resource use data were collected via CRFs for women who were admitted to hospital with signs and symptoms of preterm labour. Data on subsequent birth in woman who were not admitted hospital following assessment were not captured. Appendix 12, Table 47, details the resource use items that were collected and recorded, the unit cost applied and the accompanying references. All unit costs were collected or converted into Great British pounds (£) for the price year 2017/18. Unit costs were collected from routine sources such as the British National Formulary,70 Personal Social Services Research Unit69 and NHS Reference Costs. 65 Some unit costs that are not available on the British National Formulary,70 such as the cost of a cervical length measurement, were obtained from published literature or from expert (e.g. the project management team) opinion.
Analysing costs
The mean resource use and cost for each patient included in the prospective cohort study was estimated. It is typical for health-care cost data to be distributionally skewed to the right with a long tail. 80 This is because there is a lower bound at zero (health-care costs cannot be less than zero) and because there are generally a small number of women who require substantially more medical services than the norm. Owing to the problems associated with health-care cost analysis, it is recommended that multivariable analysis of the difference in arithmetic mean of the cost be carried out. 80 We estimated a number of generalised linear models with different families and link functions and based our choice of final model on goodness of fit using the modified Park test.
Clinical outcomes
The prognostic model A (see Chapter 4) was validated in 26 maternity units across the UK. The data set included 3049 women. Following the exclusion of women who did not meet the eligibility criteria, the final data set was a cohort of 2924 women. The validation study generated data on quantitative fFN prognostic model performance, birth outcomes and demographic characteristics of the women involved. The cohort study results for the quantitative fFN prognostic model (the result of the linear predictor) were transformed into a percentage probability (between 0% and 100%) of spontaneous preterm birth within 7 days. Appendix 12, Table 48, provides all model parameters used in the cost-effectiveness analysis.
Cost-effectiveness analysis of cohort study: within 7 days
The base-case cost-effectiveness analysis compared the cohort study results for qualitative fFN with the quantitative fFN prognostic model results, reporting the incremental cost per QALD gained and NMB using a willingness-to-pay threshold per QALD of £54.79, equivalent to the UK threshold of £20,000 per QALY.
Model overview
The decision model is based on the same decision tree framework shown in Figure 5. Patient outcomes are generated via the proportion of women who enter into each of four possible states, in accordance with which diagnostic test or prognostic model is implemented:
-
correctly identified, correctly treated (true positive)
-
incorrectly identified, incorrectly not treated (false negative)
-
incorrectly identified, incorrectly treated (false positive)
-
correctly identified, correctly not treated (true negative).
The base-case analysis makes the following key assumptions:
-
Clinicians always follow the test results (i.e. test results are never over-ruled).
-
Minor neonatal morbidity is captured as 7 days of care in a lower level of neonatal care (SCBU). The cost of 7 days in this type of care is applied and the health utility associated with this is based on the quality of life of an infant suffering from respiratory distress syndrome.
-
Major neonatal morbidity is captured as 7 days of high-level neonatal care (NICU). The cost of this is the NHS cost of this level of care for 7 days and the health utility associated with this is based on the quality of life of an infant suffering from intraventricular haemorrhage (proxy for cerebral palsy).
-
The outcome of ‘did not deliver at 7 days’ is attributed the same ‘full health’ QALYs as those babies delivered in ‘full health’.
The outcomes associated with the quantitative prognostic model strategy are reported using alternative probability of spontaneous preterm birth thresholds (probability of spontaneous preterm birth) for a ‘treat’ decision rule. The decision to admit to hospital can be based on results of probability of spontaneous preterm birth within 7 days at risk thresholds of ≥ 2%, ≥ 5%, ≥ 10%, ≥ 20%, ≥ 30%, and so on. Because each admit threshold is associated with a unique set of outcomes (costs and QALYs), the most cost-effective threshold for the quantitative fFN prognostic model can be determined and compared against the qualitative fFN outcome in terms of the optimal incremental ICER and NMB.
Lifetime analysis: lifetime horizon
To capture the longer-term cost and health outcomes associated with infants who did or did not receive treatment, the 7-day outcomes were extrapolated over a lifetime horizon. The 7-day time horizon does not capture the costs and quality-of-life impacts of minor and major morbidity that may endure beyond 7 days. For the lifetime horizon, QALYs rather than QALDs are presented and the key assumptions are that:
-
Infants who are incorrectly not treated (i.e. false negatives) and experience major morbidity during the 7-day time horizon have lifetime cost and health implications. It is assumed that minor morbidity does not extend beyond 7 days.
-
The quality of life for major morbidity is represented by the health utility associated with intraventricular haemorrhage (proxy for cerebral palsy). Lifetime costs associated with lifetime care for women with cerebral palsy (£115,000 lifetime care) are incorporated. 77 Lifetime healthy utilities are based on an infant’s state in the model at 7 days (dead, minor morbidity, major morbidity or healthy). These utilities67 are extrapolated over an average lifespan and discounted to the present value. We do not capture the natural decreasing time profile of health utility over a lifetime because this is not known for infants with minor or major morbidity at 7 days.
Sensitivity analyses
One-way sensitivity analysis
The one-way sensitivity analysis assessed the impact on cost-effectiveness of varying key input parameters. The one-way sensitivity analysis was conducted on the lifetime model considering:
-
A 50% reduction in the cost of lifetime major morbidity from £115,000 to £57,500.
-
A reduction in mean lifetime utility related to major morbidity from 0.76 to 0.40. 81
-
Applying a discount rate to future costs and utilities of 1.5% rather than the standard 3.5%. This lower rate is recommended in evaluations of public health interventions and for those with very young women. 82
Probabilistic sensitivity analysis
Uncertainty around the parameter estimates used in our model was fully characterised and propagated through to the model results by conducting PSA. This was done via a 1000-iteration Monte Carlo simulation, defining distributions around the mean point estimates for each parameter. The PSA produced a distribution of NMB estimates that were then plotted on a cost-effectiveness plane to graphically represent uncertainty. 76 The CEACs present the uncertainty surrounding the probability that each strategy is cost-effective in terms of willingness to pay for QALYs gained. 76
Scenario analysis
Owing to low levels of data on cervical length from the cohort study (see Chapter 6), we report summary statistics for the use of cervical length measurement for the prognosis of spontaneous preterm birth within 7 days.
Results
Resource use estimates from cohort study
Table 22 provides a breakdown of the resource use and cost estimates relating to women admitted to hospital at risk of suspected preterm labour. These data were used in the final health economic model to estimate the cost-effectiveness of alternative prognostic strategies in a UK clinical setting. Full details of the assumptions made regarding resource use data are given in Appendix 13.
Mean resource use (days) (95% CIs), and/or per cent of total receiving treatment (95% CIs) | Mean cost (£) estimate per patient (95% CI) | |
---|---|---|
Maternal observations (N = 1372) | ||
Maternal admission | 2.43 (1.99 to 2.87) | 611 (501 to 722) |
Maternal hospital transfer | 6% (5% to 7%) | 56 (44 to 67) |
Corticosteroids | 37% (35% to 40%) | 8.32 (8 to 9) |
Betamethasone | 75% (67% to 75%) | 6 (5 to 6) |
Dexamethasone | 25% (21% to 29%) | 2 (1 to 2) |
Magnesium sulphate | 0.34 (0.14 to 0.54), 4% | 4 (2 to 6) |
Tocolytics | 0.42 (0.19 to 0.67), 10% | 0.04 (0.02 to 0.05) |
Nidedipine | 4.25 (1.70 to 6.80), 91% | 0.27 (0.01 to 0.04) |
Indometacin | 0% to 0% | 0 |
Glyceryl trinitrate | 4.25 (1.70 to 6.80), 6% | 0.01 (0.00 to 0.01) |
Atosiban | 0% to 0% | 0 |
Neonatal observations (N = 735) | ||
Neonatal admission | 11.56 (9.96 to 13.17) | 5163 (4060 to 6265) |
SCBU | 5.68 (4.85 to 6.52), 42% | 1848 (1578 to 2120) |
LNU | 1.70 (1.11 to 2.28), 15% | 870 (570 to 1170) |
NICU | 2.02 (1.44 to 2.60), 17% | 2900 (2069 to 3732) |
Neonatal hospital transfer | 9% (7% to 11%) | 103 (78 to 129) |
Complications | ||
CPAP | 1.63 (0.10 to 2.70), 22% | 340 (207 to 472) |
Intubation | 0.4 (0.20 to 0.59), 9% | 83 (43 to 123) |
Oxygen | 1.91 (0.93 to 2.89), 14% | 40 (19 to 60) |
Surfactant | 7% (6% to 9%), 5% | 14 (10 to 19) |
Surgery | 1% (1% to 2%), 2% | 75 (36 to 114) |
Cost-effectiveness results
Table 23 details the cost-effectiveness results of the cohort study comparing qualitative fFN with the quantitative prognostic tool over a 7-day time horizon. Table 24 details the results of the lifetime extrapolation, comparing qualitative fFN with the quantitative prognostic tool. In both tables, a scenario analysis of ‘treat all’ is included; this can be compared with the qualitative fFN results and an optimal quantitative fFN treatment threshold identified.
Test strategy | Probability of correct diagnosis | Total cost (£) | Total QALD | Incremental QALDs | Incremental cost (£) | ICER (QALD) (£) | Incremental NMB (QALD) (£) |
---|---|---|---|---|---|---|---|
Treat all | 0.0291 | 1182 | 6.149 | ||||
Model A, risk thresholda | |||||||
≥ 2% | 0.8338 | 316 | 6.148 | –0.0005 | –866 | 1,875,566 | 866 |
≥ 5% | 0.8765 | 267 | 6.148 | –0.0004 | –49 | 112,221 | 48 |
≥ 10% | 0.8912 | 249 | 6.147 | –0.0002 | –18 | 86,141 | 17 |
≥ 15% | 0.8977 | 239 | 6.147 | –0.0002 | –10 | 43,104 | 9 |
≥ 20% | 0.8977 | 234 | 6.147 | –0.0004 | –5 | 15,268 | 5 |
≥ 25% | 0.8988 | 230 | 6.147 | –0.0002 | –3 | 21,861 | 3 |
Qualitative fFN | 0.8745 | 275 | 6.146 | –0.0007 | 44 | –59,686 | –44 |
Total cost (£) | Total QALYs | Incremental QALYs | Incremental cost (£) | ICER (QALY) (£) | Incremental NMB (QALY) (£) | |
---|---|---|---|---|---|---|
Treat all | 1232 | 13.16 | ||||
QUIDS ≥ 2% risk | 371 | 13.1593 | –0.0006 | –840 | 1,400,000 | 827 |
QUIDS ≥ 5% risk | 328 | 13.1587 | –0.0006 | –57 | 95,000 | 45 |
QUIDS ≥ 10% risk | 313 | 13.1584 | –0.0003 | –7 | 23,333 | 2 |
QUIDS ≥ 15% risk | 305 | 13.1581 | –0.0003 | 3 | –10,000 | –10 |
QUIDS ≥ 20% risk | 304 | 13.1576 | –0.0005 | 2 | –4000 | –12 |
QUIDS ≥ 25% risk | 303 | 13.1574 | –0.0002 | –16 | 80,000 | 12 |
Qualitative fFN | 331 | 13.1513 | –0.0061 | 35 | –5738 | –157 |
The results in Table 23 show that, in terms of cost per QALD over a 7-day time horizon, the prognostic tool (model A: quantitative fFN and clinical risk factors) at a ≥ 5% risk threshold dominates qualitative fFN and the tool at all other risk thresholds. At a risk threshold of ≥ 2% the prognostic tool (model A) gives the greatest incremental NMB and is the optimal choice. Compared with a treat-all strategy, the prognostic tool at a ≥ 2% risk is associated with a reduction in QALDs of 0.0005 and a cost reduction of £866. In terms of the cost-effectiveness plane, this strategy is in the south-west quadrant, which indicates that the most cost-effective option is the strategy that produces the greatest cost reduction per unit of health lost, in this case admitting to hospital at a ≥ 2% risk threshold predicted by the prognostic tool. All other options here are extendedly dominated.
Comparing the optimal threshold for admission to hospital (a ≥ 2% risk threshold) against qualitative fFN, the prognostic tool was found to increase costs by £41 per patient with a QALD gain of 0.002.
The results in Table 24 show that, in terms of cost per QALY, the prognostic tool (model A: quantitative ffN and clinical risk factors) at a ≥ 2% risk threshold is a cost-effective strategy. Compared with a treat-all strategy, the tool at a ≥ 2% risk threshold is associated with a reduction in QALYs of 0.0006 and a cost reduction of £840. In terms of the cost-effectiveness plane, this strategy is in the south-west quadrant, which indicates that the most cost-effective option is the strategy that produces the greatest cost reduction per unit of health lost, in this case admitting at a threshold of ≥ 2% risk. Qualitative fFN and the prognostic tool at all other risk thresholds are extendedly dominated. In comparison with qualitative fFN, the prognostic tool (model A) at a ≥ 2% risk threshold has an incremental cost of £40 with 0.008 QALY gain, resulting in an ICER of £5000 per QALY over a lifetime horizon. This is highly cost-effective given the recommended NICE threshold of £20,000 per QALY.
Sensitivity analysis
One-way sensitivity analysis
The result of all three sensitivity analyses showed a small change in the overall NMB of the most cost-effective strategy but no change in the ranking of strategies or cost-effective decision. Full results are presented in Appendix 12.
Probabilistic sensitivity analysis
The PSA was run for the optimal quantitative fFN strategy (≥ 2% probability of spontaneous preterm birth) compared with treat all. This analysis found that the majority of data points occurred in the south-west and south-east quadrants of the cost-effectiveness plane (i.e. where a treatment rule based on a ≥ 2% probability is less costly), but may lead to a small increase or decrease in QALDs. Hence, the PSA demonstrates that there is significant uncertainty regarding which is the most cost-effective strategy. This is illustrated in Figure 13. The CEAC is presented in Appendix 12, Figure 27.
FIGURE 13.
Cost-effectiveness plane: incremental cost and QALDs of the quantitative fFN prognostic tool at a ≥ 2% risk threshold vs. treat all.
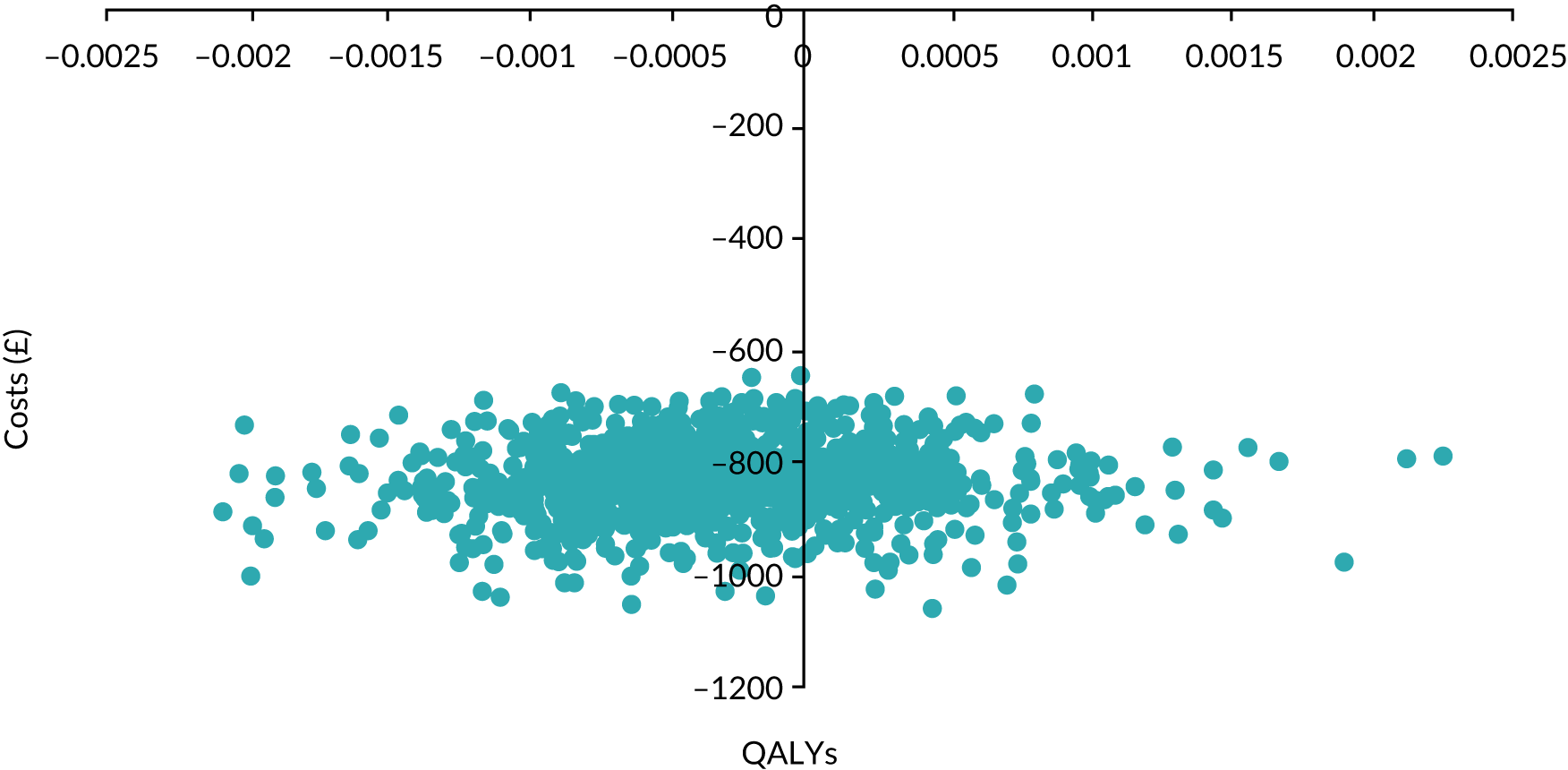
Figure 14 illustrates the results of the qualitative fFN and the various quantitative fFN risk thresholds plotted on a ROC curve. This depicts the trade-off between the sensitivity and the specificity of alternative treatment probability thresholds used in the cost-effectiveness analysis. The strategy for which the data point is closest to the top-left side of the ROC space is regarded as the most accurate. This would suggest that an admit strategy of ≥ 15% probability would be regarded as the most accurate strategy.
FIGURE 14.
The ROC plot for the quantitative fFN prognostic tool (at a ≥ 2% risk threshold).
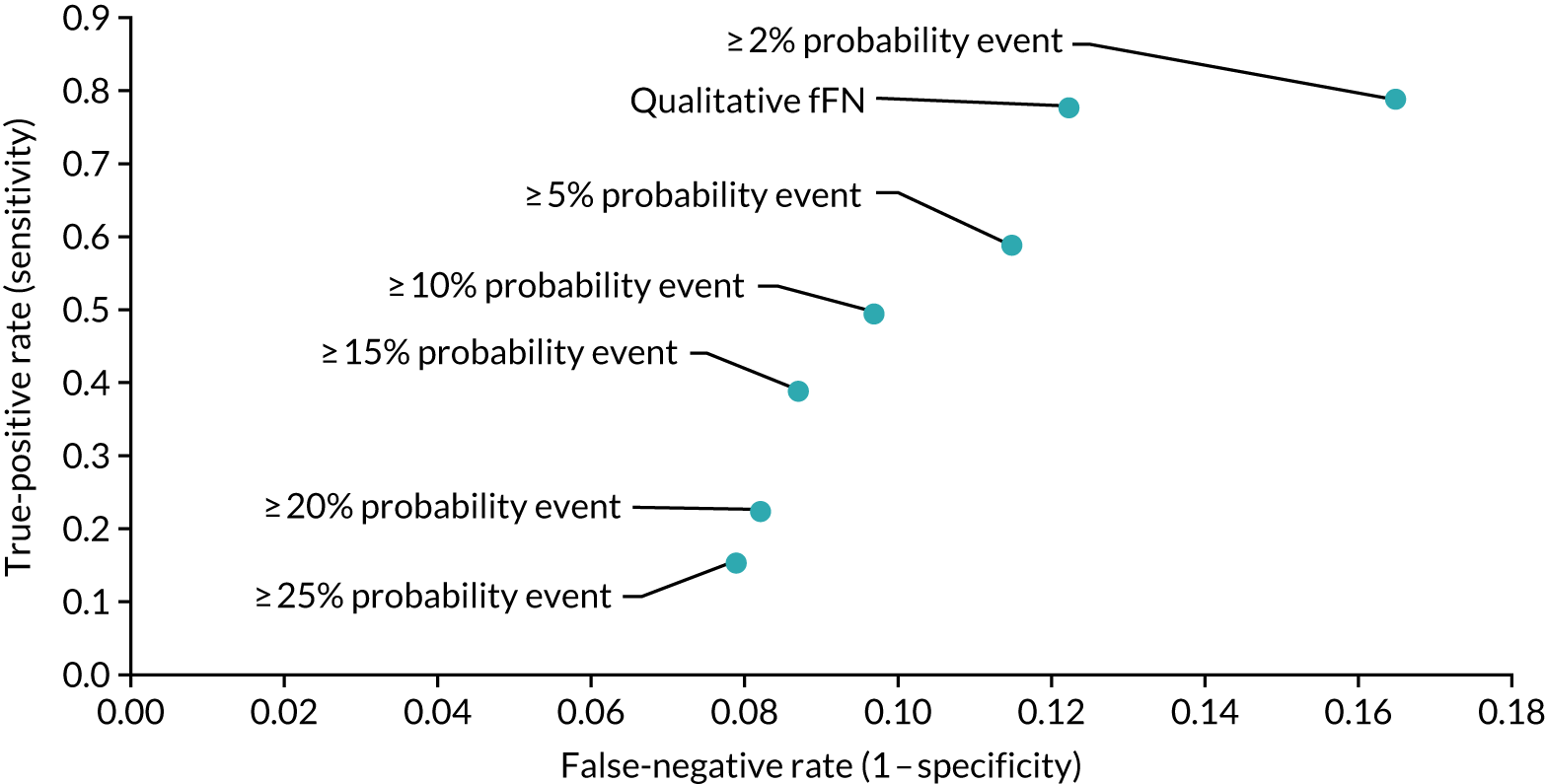
Figure 15 presents the NMB associated with each treatment threshold. However, in contrast to the ROC curve presented in Figure 14, this would suggest that a treatment threshold of ≥ 2% probability of spontaneous preterm birth is the optimal strategy. This is because the NMB approach considers both the costs and the quality of life consequences of false positive and false negatives. Under this approach, the results would suggest that a strategy which minimises false positives is likely to be the most cost-effective.
FIGURE 15.
The NMB at alternative admit probability thresholds.
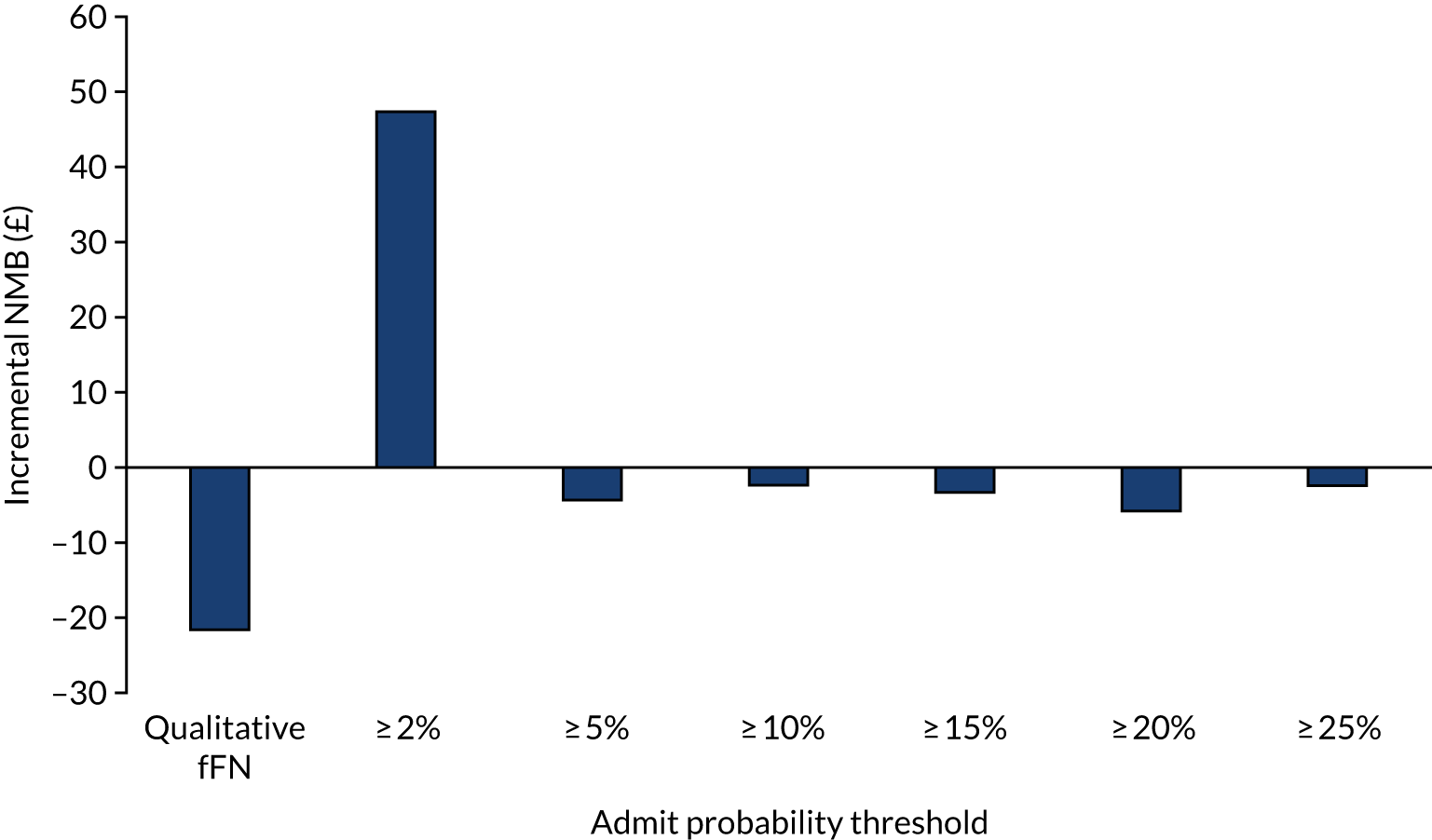
Scenario analysis
Among the 2924 women included in the final analysis, 93 women (3.18% of the population) had cervical length measured. Owing to the lack of data on cervical length measurement in the cohort study (which provides insight into the logistic and technical restrictions of using this as a diagnostic tool in clinical practice), statistical analysis of these data was limited. Indeed, it would have been inappropriate to undertake multiple imputation for the remaining 97% of the study sample.
Discussion
In this chapter, we have undertaken a cost-effectiveness analysis of the quantitative fFN prognostic tool developed in the QUIDS study. Using data on clinical outcomes and resource use from the cohort study (based on a UK population), we have shown that the QUIDS prognostic model is cost-effective compared with current clinical practice of qualitative fFN where cervical length measurement is unavailable. This should be considered alongside NICE guidelines,13 which currently recommend the use of qualitative fFN or cervical length measurement as individual tests (i.e. not as part of a prognostic model).
Using NMB (with a willingness-to-pay threshold of £55 per QALD), the ‘treatment decision rule’ of ≥ 2% probability of spontaneous preterm birth within 7 days was found to be the optimal strategy for a quantitative fFN prognostic tool. Among the 2924 women included in the cohort study, an additional 121 women would have been correctly classified as false positives and not admitted to hospital to be given unnecessary treatment compared with using a qualitative fFN prognostic tool. At a ≥ 2% risk threshold, only one less woman would have been sent home incorrectly and, hence, denied necessary treatment. Compared with a treat-all strategy, a ≥ 2% decision rule would lead to 18 additional false negatives but would prevent 2232 false positives.
Previous research has investigated the cost-effectiveness of individual prognostic tests (including qualitative fFN and cervical length measurement) and combinations of the two tests. 12,83 Both studies found that the use of a combination of qualitative fFN and cervical length measurement was the most cost-effective strategy. Watson et al. 84 developed a prognostic model that included quantitative fFN and found that this was a safe alternative to a treat-all strategy; however, the model was based on a small number of events and cost-effectiveness of alternative treatment strategies based on their model was not assessed.
Strength of this study
This study was based on clinical and resource use data gathered from women being treated in a UK clinical setting. To our knowledge, this is the first UK study in which the qualitative fFN and quantitative fFN prognostic tools have been validated in a large population (2924 women in 26 maternity units in the UK).
Limitations of this study
Resource use data were collected for woman admitted to hospital with signs and symptoms of preterm labour. Data on the subsequent birth of woman who were not admitted to hospital following assessment were not captured. It may be the case that, among the woman who were incorrectly sent home (false negatives), subsequent hospital admission and treatment costs may have been higher. This would have the impact of increasing the cost of resource use in the economic model. However, because the number of false negatives is small (1–2% across all admit strategies), the impact of any additional resource use for these women is unlikely to have much impact on the overall resource use and cost estimates.
Estimating the quality of life for infants is challenging. Our model required health utility estimates for infants born with minor or major morbidity. We chose as a proxy for these states severe respiratory distress syndrome and moderate cerebral palsy, respectively. Because it is not possible to elicit health utilities directly from infants, these utilities were elicited from parents using the standard-gamble preference-elicitation technique. To estimate preferences for public health spending, this technique should be applied to the general population rather than to any specific patient group (the parents, in this case). However, no such health utility values were identified in our review. Alternative utility estimates from other recent studies were included in the sensitivity analyses. 81
Women’s outcomes in the model are determined from the diagnostic test or prognostic model outcomes, which then feed into the economic decision tree model to determine the proportion of women who enter into each of four possible health states (death, minor morbidity, major morbidity or healthy), according to which prognostic strategy is implemented and, hence, whether or not the patient receives treatment. The trade-off between unnecessary treatment (false positives) and missed cases (false negatives) is of importance in driving the model outcomes, specifically how these are weighted with costs and utilities. Because there are only a relatively small number of women who are classified as false positives or false negatives [compared with > 80% of women who are correctly sent home (true negatives)], the actual difference between the proportion of women who enter into the minor or major morbidity states, depending on which prognostic strategy is used, is in fact very small. This means that the overall difference in cost-effectiveness between strategies is small.
Summary of principal findings
-
In comparison with qualitative fFN, the prognostic tool at a ≥ 2% risk threshold is highly cost-effective over a lifetime horizon. The results showed an incremental cost of £40 with a 0.008 QALY gain, resulting in an ICER of £5000 per QALY, which is highly cost-effective given the recommended NICE threshold of £20,000 per QALY.
-
Current NICE guidance recommends using qualitative fFN or cervical length measurement (as individual tests). Our study suggests that the use of quantitative fFN as part of a prognostic tool is highly cost-effective and should, therefore, be considered for use in clinical practice.
-
The use of the prognostic model validated at a treatment threshold of 2% probability of spontaneous preterm birth within 7 days dominated using qualitative fFN alone (i.e. it is more effective and less costly).
-
There is only a small difference in costs and QALDs across alternative admit probability threshold strategies.
-
This suggests that there remains uncertainty surrounding the optimal treatment probability threshold when using the prognostic model. This is driven by the uncertainty surrounding the true health and monetary cost of infants who are born preterm to mothers who are not given treatment (i.e. false negatives). Further research aimed at understanding the full cost and health outcome impact of preterm labour is warranted.
Chapter 8 Acceptability of fetal fibronectin testing and effects on anxiety
Context
Despite the widespread use of fFN testing to predict preterm birth, to date there is no evidence about clinician perspectives on its use and limited evidence relating to its impact on women. 85,86 Limiting women’s exposure to anxiety in pregnancy is worthwhile, given the known link between antenatal anxiety and preterm birth. 87 Hence, understanding the influence of clinical tests such as fFN tests on anxiety for a group of women who have symptoms of preterm labour is important. The aim of this aspect of the study, therefore, was to explore clinicians’ and women’s perceptions of the acceptability of fFN testing and a prognostic model and to seek to understand any influence of the test on maternal anxiety.
Methods
State-Trait Anxiety Inventory
The STAI assesses both state anxiety (situational anxiety) and trait anxiety (a person’s tendency to anxiety). At recruitment to the QUIDS prospective cohort study, participants were invited to complete an optional pre-test STAI questionnaire and provided with a second questionnaire to be completed 24–48 hours later (with a prepaid envelope to be returned by post). Pre- and post-test state and trait anxiety scores were compared (using Student’s t-test) and analysed using multiple logistic regression adjusting for qualitative fFN result, pre-test score and centre.
Sample and recruitment
We purposively sampled 30 women and 30 clinicians from a subset of trusts (n = 14) that had a high response rate to the STAI questionnaires88 as of January 2017. Women who had completed and returned both STAI questionnaires and had indicated an interest in being interviewed were sent a participant information sheet (PIS) by post and contacted by telephone. Clinicians were approached by research midwives or the local researcher, with the aim recruiting clinicians with a range of professional experience. Separate written consent was sought from all participants after they had been given time to read the PIS and the opportunity to ask questions.
Data collection
Data were collected via a semistructured interview conducted over the telephone. Separate structured topic guides were designed for women and clinicians; however, all participants were invited to speak freely about their experiences. Clinicians were also presented with a clinical scenario and asked to discuss their management plan based on clinical findings only, qualitative fFN, quantitative fFN and, finally, the QUIDS prognostic model result. All interviews were audio-recorded with consent and field notes were taken. Audio recordings were transcribed verbatim.
Data analysis
Interview data were analysed using a framework approach (see Chapter 3).
Results
State-Trait Anxiety Inventory
Among the 2924 participants in the QUIDS study, 1876 (64.16%) completed a baseline STAI questionnaire and 412 (14.1%) returned both a baseline and a post-test STAI form. Baseline characteristics of the women who did not complete either questionnaire, those who completed baseline questionnaire only and those who competed baseline and post-test questionnaires are shown in Table 25. The proportion of smokers was lower in the group that completed both pre-test and post-test questionnaires, and there was a higher proportion of white women in the group that completed both questionnaires. However, mean baseline scores were similar in all women who competed a baseline questionnaire, whether or not they completed the post-test questionnaire, and there were similar proportions of women who had a spontaneous preterm birth within 7 days across the groups.
Characteristic | No STAI at baseline (N = 636) | Baseline STAI only (N = 1876) | Baseline and posttest (N = 412) | Total (N = 2924) |
---|---|---|---|---|
Age (years) at screening, mean (SD) | 27.98 (5.70) | 28.02 (5.62) | 29.18 (5.69) | 28.18 (5.66) |
Missing, n (%) | 11 (1.73) | 17 (0.91) | 3 (0.73) | 31 (1.07) |
Smoker, n (%) | 132 (20.8) | 419 (22.3) | 57 (13.8) | 608 (20.8) |
Missing, n (%) | 12 (1.9) | 24 (1.3) | 4 (1.0) | 40 (1.4) |
Ethnicity, n (%) | ||||
White | 515 (81.0) | 1654 (88.2) | 375 (91.0) | 2544 (87.0) |
South Asian | 54 (8.5) | 100 (5.3) | 11 (2.7) | 165 (5.6) |
East Asian | 4 (0.6) | 2 (0.1) | 1 (0.2) | 7 (0.2) |
African/Caribbean/Middle-Eastern | 36 (5.7) | 56 (3.0) | 6 (1.5) | 98 (3.4) |
Other | 13 (2.0) | 39 (2.1) | 16 (3.9) | 68 (2.3) |
Missing, n (%) | 14 (2.2) | 25 (1.3) | 3 (0.7) | 42 (1.4) |
Nulliparous, n (%) | 206 (32.4) | 583 (31.1) | 162 (39.3) | 951 (32.5) |
Missing, n (%) | 83 (13.1) | 80 (4.3) | 8 (1.9) | 171 (5.8) |
BMI (kg/m2), median (IQR) | 25.7 (22.3–29.8) | 25.7 (22.4–30.5) | 24.3 (21.5–28.6) | 25.4 (22.2–30.2) |
Missing, n (%) | 16 (2.52) | 31 (1.65) | 4 (0.97) | 51 (1.78) |
Spontaneous preterm birth within 7 days of test, n (%) | 22 (3.5) | 53 (2.8) | 10 (2.4) | 85 (2.9) |
Qualitative fFN: positive, n (%) | 101 (15.9) | 238 (12.7) | 64 (15.5) | 403 (13.8) |
Baseline STAI state anxiety, mean (SD) | – | 41.65 (11.52) | 42.63 (12.06) | 41.83 (11.62) |
Missing,a n (%) | – | 34 (1.81) | 4 (0.97) | 38 (1.30) |
Baseline STAI trait anxiety, mean (SD) | – | 37.76 (9.94) | 37.52 (10.48) | 37.72 (10.04) |
Missing,a n (%) | – | 93 (4.96) | 12 (2.91) | 105 (3.61) |
Table 26 shows the pre- and post-test STAI scores for the 415 women who completed both questionnaires. As would be expected, STAI trait anxiety scores (which represent inherent tendency to anxiety) were similar at baseline and posttest, with a mean change of –0.12 points (SD 5.99 points), and qualitative fFN results did not influence these. In contrast, post-test STAI state anxiety scores (which represent situational anxiety) were lower than at baseline overall, with a mean change of –5.6 points (SD 11.74 points), which is considered a clinically significant reduction. 89 However, women who had a positive qualitative fFN result did not have a reduction in their state anxiety score, and positive qualitative fFN results were associated with greater state anxiety than negative qualitative fFN results [adjusted OR 4.33 (95% CI 1.67 to 6.99); adjusted for baseline test score and centre], with a mean score above the cut-off point of 39–40 points, which has been suggested to detect clinically significant symptoms. 89
Subscale | n | Baseline score (points), mean (SD) | n | Post-test score (points), mean (SD) | Change in score (points), mean (SD) | p-value | Adjusted OR (95% CI) | p-value |
---|---|---|---|---|---|---|---|---|
State anxiety | ||||||||
Overall | 408 | 42.63 (12.06) | 411 | 37.03 (11.89) | –5.60 (11.74) | 0.001 | ||
Qualitative fFN: negative | 333 | 42.25 (12.07) | 336 | 36.21 (11.43) | –6.05 (11.32) | 0.001 | Reference | |
Qualitative fFN: positive | 64 | 43.63 (11.96) | 64 | 41.20 (12.65) | –2.42 (13.56) | 0.266 | 4.329 (1.67 to 6.99) | 0.001 |
Trait anxiety | ||||||||
Overall | 400 | 37.52 (10.48) | 404 | 37.31 (11.10) | –0.12 (5.99) | 0.783 | ||
Qualitative fFN: negative | 330 | 37.52 (10.57) | 328 | 37.17(11.09) | –0.29 (5.71) | 0.677 | Reference | |
Qualitative fFN: positive | 61 | 37.74 (10.06) | 63 | 38.14 (10.39) | 0.65 (6.95) | 0.828 | 0.94 (–0.66 to 2.53) | 0.248 |
Acceptability
A total of 104 individuals from 14 NHS trusts across England, Scotland and Wales consented to take part in the acceptability interviews (32 women and 72 clinicians) and 61 participated [30 women and 31 clinicians (1 partial interview)] (Table 27). Forty-three individuals (two women and 41 clinicians) were unable to commit to a time for the interview or were uncontactable following consent. The trusts covered a range of geographical locations and included large tertiary-level maternity units and smaller district general hospitals.
Site identifier | Approached (includes contact by telephone or post) (n) | Consented (n) | Interviewed (n) |
---|---|---|---|
11 | 40 | 20 | 17 (1 partial) |
14/15 | 14 | 11 | 3 |
16 | 14 | 10 | 2 |
17 | 28 | 25 | 13 |
19 | 6 | 4 | 3 |
20/21 | 4 | 1 | 1 |
24/25 | 6 | 1 | 1 |
26 | 18 | 7 | 7 |
27/28 | 6 | 5 | 3 |
29 | 6 | 5 | 2 |
30 | 8 | 1 | 1 |
32 | 2 | 2 | 0 |
36 | 3 | 3 | 2 |
All sites | 165 | 104 | 61 (1 partial) |
Findings from women’s interviews
The women who participated in interviews had completed and returned the STAI questionnaires pre and post testing (Table 28).
Identifier | Parity | Gestational age at fFN test | fFN result | Eventa | Outcome | Baseline | Post test | ||
---|---|---|---|---|---|---|---|---|---|
State anxiety | Trait anxiety | State anxiety | Trait anxiety | ||||||
11001 | 0 + 1 | 33+4 | Pos | Yes | Preterm birth at 34 weeks’ gestation | 64 | ✗ | 65 | 48 |
11008 | 2 + 1 | 28+5 | Pos | No | Term birth | 50 | 41 | 36 | 37 |
11027 | 1 + 1 | 33+5 | Neg | No | Term birth | 32 | 40 | 43 | 34 |
11086 | 1 + 0 | 30+0 | Neg | No | Term birth | 37 | 44 | 28 | 30 |
11114 | 0 + 0 | 25+0 | Neg | No | Term birth | 47 | 32 | 52 | 28 |
11115 | 0 + 0 | 25+0 | Neg | No | Term birth | 39 | 29 | 43 | 32 |
11154 | 0 + 1 | 30+0 | Pos | Yes | Preterm birth at 30 weeks’ gestation | 69 | ✗ | 27 | 22 |
11185 | 0 + 0 | 33+0 | Pos | No | Term birth | 51 | 30 | 35 | 28 |
11195 | 2 + 0 | 26+4 | Pos | No | Term birth | 46 | 54 | 49 | 53 |
11196 | 1 + 1 | 28+2 | Neg | No | Term birth | 57 | 57 | 35 | 56 |
11197 | 0 + 0 | 33+6 | Neg | No | Term birth | 35 | 27 | 30 | 33 |
11201 | 1 + 0 | 30+3 | Neg | No | Term birth | 51 | 51 | 40 | 33 |
11245 | 1 + 1 | 34+0 | Pos | No | Term birth | 46 | ✗ | 45 | 42 |
11260 | 1 + 1 | 30+3 | Neg | No | Term birth | 23 | 23 | 20 | 20 |
11243 | 1 + 0 | 27+0 | Pos | No | Term birth | 58 | 45 | 31 | 33 |
16063 | 1 + 1 | 32+0 | Neg | No | Term birth | 42 | 30 | 40 | 35 |
17024 | 1 + 0 | 30+0 | Neg | No | Term birth | 60 | 23 | 20 | 22 |
19030 | 2 + 2 | 31+4 | Neg | No | Preterm birth 36 weeks’ gestation | 39 | 32 | 43 | 37 |
20005 | – | 34+3 | Neg | No | Term birth | 32 | 30 | 29 | 31 |
25013 | 1 + 0 | 30+5 | Neg | No | Preterm birth at 35 weeks’ gestation | 41 | 44 | 45 | 46 |
26007 | 2 + 0 | 33+1 | Pos | No | Term birth | 34 | 31 | 24 | 26 |
26071 | 1 + 1 | 27+1 | Neg | No | Term birth | 25 | 28 | 20 | 21 |
26087 | 0 + 0 | 26+5 | Neg | No | Term birth | 43 | 32 | 24 | 31 |
26139 | 1 + 4 | 26+4 | Neg | No | Term birth | 31 | 31 | 26 | 28 |
26172 | 2 + 0 | 26+4 | Pos | No | Preterm birth at 35 weeks’ gestation | 37 | 48 | 58 | 51 |
26203 | 0 + 0 | 30+0 | Neg | No | Term birth | 46 | 32 | 23 | 30 |
27007 | 1 + 0 | 32+1 | Pos | Yes | Preterm birth 31 weeks’ gestation | 27 | 34 | 40 | 34 |
27008 | 0 + 0 | 26+4 | Neg | No | Preterm birth at 36 weeks’ gestation | 47 | 42 | 42 | 42 |
28136 | 1 + 0 | 33+4 | Neg | No | Term birth | 28 | 31 | 22 | 30 |
30023 | 0 + 1 | 27+1 | Pos | Yes | Preterm birth at 27 weeks’ gestation | 62 | 36 | 57 | 38 |
Analysis of the women’s interviews revealed four main themes and five subthemes. The theme ‘anxiety and influencing factors’ ran across each of the other three themes, which were ‘deciding to access care’, ‘fFN testing’ and ‘impact of the fFN result’.
Anxiety and influencing factors
Anxiety, or a lack of it, was a theme that ran throughout the interviews. Some women reported feeling anxious at different time points during their experiences and some reported feeling calm throughout. This finding is also reflected in the results of the STAI questionnaires (see Table 26). Among those who did report feeling anxious, the main reason was concern for their baby. Women reported worrying about various morbidities and mortality:
You know, I thought the baby could have this or the baby could have that, or it could, you know, not survive.
11008: positive fFN, no event, term birth
However, some women explained that they did not feel anxious during their experiences because they felt that they were being cared for by the right professionals in the right place:
I wasn’t too overly stressed. I knew basically that I was in the best, safe possible hands and, you know, what will be will be, basically.
11195: positive fFN, no event, term birth
It is notable that this woman indicated high levels of state and trait anxiety at both time points in her STAI questionnaire (Table 29). This may demonstrate the effect of time on anxiety recall, especially when the pregnancy ended in a term birth without complication.
Identifier | Level of professional experience | Type of maternity unit |
---|---|---|
1102 | Specialist trainee year 5/clinical teaching fellow | Tertiary unit |
1104 | Specialist trainee year 2 | Tertiary unit |
1401 | Specialist trainee year 5 | Level 2 unit |
1402 | Trust doctor (registrar) | Level 2 unit |
1503 | Specialist trainee year 1 | District general hospital |
1606 | Consultant | District general hospital |
1701 | Specialist trainee year 4 | District general hospital |
1702 | Specialist registrar | District general hospital |
1703 | Foundation years doctor | District general hospital |
1704 | Middle grade | District general hospital |
1705 | Registrar | District general hospital |
1706 | Clinical fellow | District general hospital |
1707 | Consultant | District general hospital |
1708 | Specialist trainee year 6 | District general hospital |
1709 | Registrar | District general hospital |
1710 | Consultant | District general hospital |
1716 | Consultant | District general hospital |
1719 | Foundation years doctor | District general hospital |
1801 | Specialist trainee year 4 | Tertiary unit |
1802 | Specialist trainee year 4/clinical research fellow | Tertiary unit |
1803 | Specialist trainee year 6 | Tertiary unit |
1804 | Senior trainee | Tertiary unit |
1805 | Consultant | Tertiary unit |
1806 | Specialist trainee year 2 | Tertiary unit |
1902 | Consultant | Tertiary unit |
1903 | Consultant | Tertiary unit |
2601 | Specialist trainee year 4 | District general hospital |
2901 | Registrar | Level 2 unit |
2905 | Specialist trainee year 6 | Level 2 unit |
3602 | Foundation years doctor (obstetrics and gynaecology trainee) | Tertiary unit |
3603 | Specialist trainee year 2 | Tertiary unit |
By contrast, another woman linked her lack of anxiety to her generally laid-back nature:
I was like what will be will be, if it’s positive it’s positive, and if it’s not it’s not, you know. Then literally I’m one of those sort of go with the flow.
26007: positive fFN, no event, term birth
This matched her STAI results, which indicated state and trait anxiety scores below the mean for anxiety in pregnancy. 90
There appeared to be a link between women’s perception of their symptoms and women’s anxiety. For some women anxiety heightened their perception of symptoms, whereas for others anxiety resolved when symptoms did.
Anxiety commenced or was heightened when clinicians demonstrated concern about women’s symptoms. The invitation to attend hospital or in some cases introducing the concept of fFN testing to rule out preterm labour caused anxiety:
They suggested having this test and that was when it first really . . . it never occurred to me that I might be going into preterm labour. So that was maybe a bit shocking but I never really thought that I was.
26087: negative fFN, no event, term birth
Summary
Women’s anxiety was influenced by different factors, including symptoms, clinician responses and the women’s personality traits. This indicates that, although a degree of anxiety can be influenced by clinical care, a degree is related to factors outside the clinicians’ or women’s control. However, clinicians can be mindful of women’s anxiety and provide appropriate support, reassurance and information.
Deciding to access care
All women were invited to talk about their experience of having symptoms of preterm labour. Women talked about their experience of initial symptoms and their decision to contact maternity services. Symptoms included vague sensations that can be common to pregnancy – backache, abdominal tightening sensations, Braxton Hicks contractions, vaginal discharge or dampness and abdominal, vaginal or rectal pressure – and generalised feelings of being unwell, such as having an upset stomach and flu. For some women, symptoms were more clearly indicative of labour, such as contractions and having a ‘show’. The women who experienced more vague symptoms commonly talked about the feeling that ‘something wasn’t right’, indicating that they needed to contact maternity services:
I felt that something wasn’t quite right, like I felt that . . . yeah, I just felt something was different.
11001: positive fFN, event, preterm birth at 34 weeks’ gestation
A complicating factor in making the decision to contact maternity services was that women said that they were not expecting preterm labour and did not consider this to be the reason for their symptoms:
At that point it wasn’t something that even entered my head that it could be potentially going into labour.
11185: positive fFN, no event, term birth
Persistence or severity of symptoms was the driver for most women to contact maternity services. For some women, their previous experiences of childbirth helped them to decide when they needed to call. For some women the decision to contact maternity services was influenced by other people, who advised them to call.
Making contact and accessing face-to-face care was difficult for some women. Knowing who or where to call was sometimes unclear, especially ‘out of hours’:
I tried to phone the midwife, but they weren’t able to help me and they said I should phone the [NHS] and I phoned them. And they weren’t able to help me, ’cause I think it was over the weekend.
11008: positive fFN, no event, term birth
Once women contacted maternity services, some felt that they were being put off and encouraged to remain at home:
I just felt like it wasn’t normal but I felt like I was just getting told . . . I don’t know, I just kind of felt that I was getting . . . not that I was lying. I don’t know, I couldn’t really . . . I felt like, again, it was another trip to the hospital. That’s the only way I could put it, probably.
11027: negative fFN, no event, term birth
Some women felt that they needed to ‘fight their corner’ to be invited to attend for face-to-face care. However, others felt welcomed when they called maternity services.
The barrier to contacting maternity services was sometimes an internal struggle. Some women were worried about wasting clinicians’ time or being ‘dramatic’ about their experiences:
I just thought that maybe I was like being a bit . . . oh, what’s the word, not dramatic but, you know, oh, going for no reason, wasting their time I thought, do you know what I mean?
27007: positive fFN, event, preterm birth at 31 weeks’ gestation
Women with a prior childbirth experience found that negotiating access to care with clinicians over the telephone was easy because their judgement about whether or not they were in labour was believed readily.
Summary
As in the QUIDS qualitative interviews, some women spoke of the challenge of accessing maternity care with symptoms of preterm labour. This was owing to the vagueness of some women’s symptoms, and difficulties in making contact and negotiating with clinicians over the telephone. However, some women reported following their instinct that something was not right and feeling welcome to attend for face-to-face assessment. Others did not dwell on this aspect of their experience. Encouraging women to follow their instincts and giving clear information about how to contact maternity services at all times may reduce some of the challenges some women encounter.
Fetal fibronectin testing
Informed choice for the fetal fibronectin test
Women understood the fFN test and felt that they had been given sufficient information about it. Some women acknowledged the excellent negative predictive value but moderate positive predictive value of fFN testing;11,12 however, they were still happy to consent to the test because they considered the ability to rule out preterm labour valuable.
In general, women reported feeling positive about the fFN test. The main reason for this was that they wanted as much information as possible about what was happening to them:
I was quite happy to have it, because I would rather have known for sure.
11001: positive fFN, event, preterm birth at 34 weeks’ gestation
Some women felt anxious about the prospect of the fFN test. As previously reported, this was sometimes the result of an initial recognition that clinicians were concerned that they may be in preterm labour. Other women were anxious about the procedure and the results:
It [anxiousness] was a bit of both, really, it was the actual test just because no one really likes having anything like that done but also, I was worried about what the results were going to come back as.
26139: negative fFN, no event, term birth
Women balanced their anxiety about the process of the test and the result with their desire to know what was happening.
Having the fetal fibronectin test
The vast majority of the women reported that the experience of having the speculum examination to take the fFN swab was uncomfortable and unpleasant, but not painful. In general, the level of discomfort seemed to be the level that women expected, especially those who had experienced cervical screening (smear testing). However, a small number of women did report that the speculum examination was a very painful experience:
I remember it vividly . . . I’d had examinations like that before, but this one was just . . . it was very painful.
11001: positive fFN, event, preterm birth at 34 weeks’ gestation
Nevertheless, all women consented to the speculum examination because they felt that it was best for their baby:
So I think you just want to do what’s best for the baby – put your baby’s needs first, before your own.
11260: positive fFN, no event, term birth
Women reported waiting between 10 minutes and 2 hours for their fFN test results. Many were pleasantly surprised at how quickly the results were ready. However, others expressed that it felt like a long time. In some cases, this appeared to be exacerbated by concern about ongoing symptoms and what they indicated:
Yes and the time went very, very slowly.
26139: negative fFN, no event, term birth
The distraction provided by some women’s partners or other support was helpful to reduce anxiety. For women who were unsupported during their admission, attention from clinicians and support staff during their wait was welcomed.
Women were verbally informed of their fFN test result by their midwife or doctor. In general, women reported a good understanding of their results. The majority of women received a qualitative (positive or negative) result, whereas some were told a percentage risk interpreted from the quantitative fFN result. Some women liked the extra information gained from the quantitative fFN:
It was useful, because I think there is obviously still a risk, isn’t there. And so I did, sort of, take a few days to just rest.
27008: negative fFN, no event, preterm birth at 36 weeks’ gestation
However, some women appreciated the reassurance of having the positive or negative result, because it did not bring the potential worry of a borderline result:
To be honest, possibly not, because if it’s at the higher end of negative, then I think that’s slightly less reassuring. So I think, to be honest, black or white, positive and negative, would for me personally, is better.
26071: negative fFN, no event, term birth
Summary
All women anticipated benefits of agreeing to fFN testing, in particular that it would give them information about their situation. The fFN testing was associated with increased anxiety for some women; however, this appeared to be in relation to their overall situation and anticipation of the speculum examination and results, rather than in relation to the test itself. Most women were stoic about the speculum examination, although they found it uncomfortable and unpleasant. This highlights the need to manage women’s expectations and be sensitive to their fears and experiences. Women highlighted advantages and disadvantages to both qualitative and quantitative fFN results. The main disadvantages of either format could be addressed by clinicians providing high-quality information.
Impact of the fetal fibronectin test result
Immediate impact
Some women described the shock they experienced when they were given a positive fFN test result:
Well, at that point I just burst out crying. It was a bit of a shock that I came back as high risk, because obviously we hadn’t expected that.
11001: positive fFN, event, preterm birth at 34 weeks’ gestation
Understandably, the positive result increased feelings of anxiety for some women. However, other women took reassurance from the care that they were receiving and reported not feeling anxious. Women who had a positive result valued the chance for emotional and practical preparation for a preterm birth, even when preterm birth did not occur.
Some women who received a negative result reported feeling reassured:
I was over the moon, yeah, it was a massive relief; that was what gave me the peace of mind, I felt loads happier.
17024: negative fFN, no event, term birth
However, some women who had a negative result reporting feeling dissatisfied because they felt that they did not have a full understanding of their symptoms:
I was still curious as to . . . we didn’t really have an answer . . . a definitive answer as to why what happened happened, you know.
11086: negative fFN, no event, term birth
The majority of the women reported that they had confidence in their test result and felt that it was accurate:
Yeah, I had a total and utter confidence in that test.
27007: positive fFN, event, preterm birth at 31 weeks’ gestation
In general, women accepted their recommended care plan. Women who were discharged following a negative qualitative fFN result reported feeling comfortable to go home, reassured by the advice to contact the hospital again if symptoms returned or worsened:
It was just peace of mind, and I was quite happy just to go home and carry on as I was, as I were before.
11197: negative fFN, no event, term birth
Without the negative qualitative fFN result, one woman commented that she would have been anxious about returning home:
I think I would have been panicking. I think I would have just been on edge like wondering if I was in labour or not.
16063: negative fFN, no event, term birth
However, some women did comment that they would have felt more reassured if they had been offered follow-up contact with a clinician.
Women’s feelings about being admitted after a positive qualitative fFN test result were particularly variable and included concern that there was a reason for admission and reassurance that they were being looked after. Other women did not want to be admitted, felt that it was unnecessary or had competing priorities, such as other children at home, but agreed to it as they did not want to put their baby at risk:
I did think I could just go home. But I thought if I go home and something happens I would never forgive myself.
11243: positive fFN, no event, term birth
A small number of the women interviewed experienced in utero transfer owing to gestational age or their local unit being full, which increased their anxiety and caused concern.
Impact on the rest of pregnancy
The fFN result affected women’s feelings and actions during the rest of their pregnancy. Some women who had a positive fFN test result but did not go into preterm labour felt anxious throughout their pregnancy:
It definitely played on my mind afterwards. Each step that went by you would be thinking, it could happen this week or it could happen in the next couple of days.
11185: positive fFN, no event, term birth
A negative qualitative fFN result could reassure women that they were not in preterm labour. This enabled them to feel able to carry on with their daily activities, and provided some reassurance about future symptoms:
I felt a bit more reassured that I wasn’t going to go into labour soon. So, I could do things and not worry about it.
16063: negative fFN, no event, term birth
Reflections on fetal fibronectin testing
Universally, women said that they would have the test again if symptoms were to arise in a future pregnancy; indeed, some women said they would ask for it.
Women were generally accepting of the principle of the prognostic model, reflecting that the additional information may have helped to shape their interpretation of the result. However, some women were concerned that receiving a percentage risk may cause them more anxiety, particularly a low percentage risk compared with a negative test result. This reflected the concern of some clinicians about discussing results of the prognostic model with women:
If you had given me a percentage, even a one per cent or three per cent, I would have just panicked, thinking well I might be in that three per cent. So I think the fact that I was just told, you’re not going to, that almost reassured me and put it away from my mind.
11196: negative fFN, no event, term birth
Summary
As would be anticipated, receiving a positive result was shocking and anxiety-provoking for some women, whereas receiving a negative result was likely to be reassuring. Women with either result had confidence in its accuracy and were accepting of management plans for their care based on these. Those requiring admission or in utero transfer following a positive result were willing to follow these recommendations, despite preferring not to or having other priorities, for the sake of their babies’ well-being.
Overall, women’s perception of fFN testing was positive, highlighted by the universal agreement that they would have fFN testing in a future pregnancy. The advantages and disadvantages of being given a numerical or percentage risk of preterm birth were considered, and women were positive about the concept of using fFN testing within a prognostic model.
Findings from clinician interviews
Clinicians who participated in the acceptability interviews covered a range of professional experience in different types of maternity unit (see Table 28).
Analysis of the clinician interviews revealed three main themes, and nine subthemes. The main themes were ‘factors influencing use of fFN’, ‘fFN as a tool for preterm labour diagnosis’ and ‘prognostic model to aid decision-making’.
Factors influencing use of fetal fibronectin
Clinician experience
The clinicians’ experience of using fFN testing in clinical practice ranged from a few occasions to regular use spanning many years. All clinicians had experience of using qualitative fFN and some also had experience using quantitative fFN. For all clinicians the use of fFN testing featured prominently in their assessment of women with symptoms of preterm labour, which was expected given that all sites were participating in the QUIDS study.
Junior obstetricians working in triage, day/maternity assessment units, delivery suites or, occasionally, antenatal clinics used fFN testing most regularly. Senior clinicians’ experience of fFN test use included consulting on a clinical case without the opportunity to review the woman themselves. In these cases, the objective nature of the fFN result could increase their confidence in their junior colleagues’ clinical assessment:
So, I will be more confident of a GP’s [general practitioner’s] assessment that a patient is not in, you know, preterm labour, if they can give me objective test results.
1802
Clinicians’ experience and opinions of using quantitative fFN were variable, with some interpreting the result in a binary manner (positive or negative) and others finding the exact concentration a helpful feature for decision-making.
Clinician values and beliefs
Clinicians expressed that they ‘err on the side of caution’ (1402, 1701, 1702, 1704 and 1802) when caring for women with symptoms of preterm labour. Where there is doubt, clinicians develop proactive management plans given the potentially ‘devastating’ (1701) consequences of an unexpected preterm birth.
Clinicians also acknowledged the risks of ‘overtreating’, but felt that this was a better strategy than ‘undertreating’. Some clinicians felt that fFN testing can reduce the incidence of overtreating:
I like fibronectin because it targets your steroids.
2901
Concerns about medico-legal issues also influenced clinicians’ actions and made them more positively predisposed to the objective nature of fFN testing:
If the worst was to happen and she was to go home and deliver, then in sort of in your defence you can say, look, you know, there was a test, it was negative . . . it was just unfortunate as opposed to negligent.
1802
The decision to use fetal fibronectin
The clinicians acknowledged the difficulty of predicting preterm labour and reported using different strategies in collaboration, for example history-taking and observation. Clinicians reported using a range of assessment techniques and tools, including fFN testing. Despite one clinician acknowledging that national guidance recommends transvaginal ultrasonography assessment of cervical length as the first-line diagnostic test,13 this was rarely mentioned by the clinicians compared with fFN testing:
From my point of view, it [fFN testing] still features very highly in my own little protocol in my head with the preterm labour actually. Which it probably shouldn’t actually, I should get cervical screening in there more.
1710
Judicious use of fFN testing was described by some clinicians, including withholding the test where the fFN result would not have changed the clinical management plan.
The objectivity of fFN was considered beneficial, particularly for managing resources, such as justifying interventions with significant resource implications such as in utero transfer or supporting clinical decisions to discharge women.
Summary
The value clinicians placed on fFN testing differed depending on their experience, whether it was to aid their own decision-making or add objectivity to others’. For many it helped them balance their philosophical stance of ‘erring on the side of caution’ with their concern about overtreatment. Clinicians appeared satisfied to base their management plans for women with suspected preterm labour on a combination of history taking, clinical assessment and fFN testing.
Fetal fibronectin testing as a tool for preterm labour diagnosis
Confidence in fetal fibronectin testing
When asked about the best way to predict preterm labour, many clinicians referred to maternal history and indicated that this may override the fFN result. The importance of recognising a history of previous preterm labour was highlighted by some clinicians:
In my opinion actually I would check the history very seriously. If someone has had three previous preterm labours and they come in and fetal fibronectin tells me it’s negative or unlikely, I would take that with a pinch of salt.
1402
Nevertheless, clinicians appeared to like the objective nature of fFN testing:
But currently we know that there is no single predictor of preterm labour that is 100 per cent accurate, so QUIDS [fFN testing] is the most objective of all those things. So for me I would take QUIDS [fFN testing] above any other thing currently.
1702
In general, the consensus among clinicians was that preterm labour diagnosis must take account of a number of factors including history, direct observation, visualisation of the cervix and fFN test result if available.
All clinicians seemed aware of the negative-predictive value of fFN testing and, hence, felt confident to base management plans on a negative fFN result:
So, if it is a negative result I would very, very rarely admit a patient if they had a negative result purely for reasons of threatened preterm labour.
1802
Clinicians reported feeling less confident about their decisions when fFN testing was not possible, particularly the decision to discharge a woman.
Despite being aware that the positive predictive value of fFN testing is not as great as the negative predictive value, clinicians still reported that they act on the result in almost all cases:
I mean with a positive no one would ever dispute keeping . . . well, from our perspective that means transferring, so no one would ever dispute that.
1701
Management plans are influenced by fFN test results, demonstrating the confidence that clinicians place in them. However, clinicians made it clear that they would rely on their own clinical judgement over the fFN test result:
A test is a test, and the clinical situation can evolve, and so I think if you’ve got a strong history of previous preterm delivery, despite the negative fibronectin, if the patient is clinically presenting with palpable contractions and is distressed, I would never send someone like that home.
1716
In particular, if there was conflict between the clinician’s clinical judgement and a negative qualitative fFN result, some clinicians would tend to act with caution, following their clinical judgement.
Qualitative versus quantitative fetal fibronectin
A number of clinicians had experience using both qualitative and quantitative fFN. Some clinicians felt that quantitative fFN was a more useful test than qualitative in enabling them to make individualised decisions and management plans for women:
Whereas previously, you know, 49, the difference between 49 and 51 is probably negligible, but one is negative and one is positive, so you couldn’t necessarily discriminate between those two things. But, whereas with the quantitative one was more useful.
1802
Other clinicians were ambivalent, considering advantages and disadvantages to quantitative fFN. Some clinicians felt that the binary nature of the qualitative fFN was more reassuring and easier to interpret, whereas the percentage risk left doubt:
Even if it is like 3 per cent chance or like 6 per cent chance or whatever, according to whatever the table says, you know, or whatever the number comes up and all there is still that little window that is left behind and sending a patient, and the patient is not reassured.
1503
Clinicians considered the two types of fFN test from the women’s perspective, recognising the advantages and disadvantages of the extra information. Whereas some clinicians were concerned that women would not understand a percentage value, others felt that it was the duty of the clinician to ensure that there was understanding. This was considered something that clinicians are experienced in doing:
So you would hope you would be able to explain it well enough. We explain risk on a day-to-day basis, so you would hope that you would be able to explain it in enough detail.
2905
Summary
Clinicians appeared to have a high level of confidence in fFN test results. Clinicians used fFN testing in collaboration with clinical assessment and history taking, and a composite of all would influence management plans. The additional challenge for clinicians was when there was conflict between the clinical assessment and the fFN test result. However, in general, clinical judgement would over-rule fFN test results, in particular if this meant ‘erring on the side of caution’. Clinicians reported advantages and disadvantages about both qualitative and quantitative fFN results, as did women. To ensure that women benefit and are not disadvantaged by the additional information gained from the quantitative fFN result, clinicians are required to provide further explanation and support to interpret results.
Use of the prognostic model in clinical practice
The prognostic model in clinical scenarios
Clinicians were given clinical scenarios to discuss to understand how the information gained from clinical assessment, qualitative fFN, quantitative fFN and the prognostic model may influence clinical management plans (see Appendix 14). It should be noted that the version of the prognostic model used in the scenarios included ‘previous spontaneous preterm birth’ as a variable; hence, the impact of not including this was not explored specifically. The scenario discussions indicated that the additional information that clinicians gained from the prognostic model risk prediction did influence clinical management for some, although not for others, and not always in the same way. Some clinicians expressed that, although the prognostic model result did not change their management plan, certain nuances did change, for example how relaxed they felt about discharging the woman or with what level of urgency they arranged an in utero transfer. Responding to clinical assessment was still evident, indicating that the prognostic model did not influence clinical management alone; rather, it was used in conjunction with other evidence:
I’m just accepting of what the figure says, but . . . I don’t go 6 per cent, oh, that’s low, so let’s do something different. I’m still looking at the patient from a clinical perspective, knowing that, okay, well the predictive model says this, but actually her risk of preterm labour is zero or one.
1803
For some clinicians, receiving the prognostic model percentage risk made them more confident about their management planning, whereas others felt less confident about their plan. The level of confidence may be a result of the inexperience clinicians have in using and interpreting the prognostic model:
I think if it was a tool that we used more often, that you gain a certain confidence with, and yes, it may change my practice in the future, I guess.
1708
Reflecting on their experience using the prognostic model, some clinicians reported that the additional information it gave was useful. They liked that the model took account of individual factors for the woman to provide her with a risk relating just to her:
The more information you have, the more it adds to it, then the more information you can give the woman, and the more accurate you can make your planning.
1401
Other clinicians felt that the prognostic model result was not more useful than the fFN test result, reporting that they were unsure how to interpret the percentage risk:
I think if it was like 50 per cent then you’d be like, oh wow, that’s quite high, but then 6 per cent you could consider as quite high, but not massively high.
1706
Clinicians could anticipate possible advantages to the tool, including reducing admissions to hospital, unnecessary in utero transfers, targeting use of steroids and reassuring women and clinicians. Potential disadvantages included over-reliance on technology, increasing clinician time and paperwork, making clinicians overcautious and adding confusion if the clinical assessment, fFN test result and prognostic model were contradictory.
Predictive time scales
The current time scale of the prognostic model is preterm birth within 7 days of testing. Clinicians generally felt that this was a useful time scale to accurately target administration of steroids:
Seven days is fine, 7 days is fine because you want to get . . . the most important thing is to get the steroids on board.
1702
Some clinicians felt that having ‘two time scales would be helpful’ (1803): one 7-day time scale and another that was shorter (24–72 hours) was suggested.
Implementation of the prognostic model
When asked, the vast majority of clinicians stated that they would choose to use the prognostic model in clinical practice. However, some explained that this should not be a personal decision, rather, it would need to be decided locally, regionally or nationally:
We don’t use things personally . . . If we decide to something, we all use it in the department . . . And sometimes we check the nearby trusts and make sure they’re also using it and accepting it because it has to be at regional level, I think.
1606
Clinicians felt that co-ordinated, region-wide implementation of the prognostic model was important, given the anticipated utility of the prognostic model in helping to manage in utero transfers.
Having guidance about how to interpret the prognostic model risk prediction, including recommendations for clinical management at certain risk percentages, was discussed. Some expressed that the decision about how to interpret the prognostic model should not be individual, and that there should be guidance:
Definitely, there should be guidance. I don’t think the person should take responsibility of the outcome.
1606
As professionals, clinicians wanted detailed information about how the model was developed, because they felt that this would enhance their trust in it:
But knowing how the predictive model is constituted would help me better make a decision. That is not to say I need to know the nitty-gritty of it, but I just want to know what goes into it.
1709
Summary
The scenario discussions indicated that the prognostic model may influence clinicians’ management plans. Having used the model in a hypothetical case, most clinicians said that they found the additional information useful. Others felt that it was not more useful than the qualitative fFN test, because they did not have the experience in its use to feel confident about interpreting the result. In general, the 7-day predictive time scale was considered appropriate, and others felt that the addition of a shorter time scale would be useful. The clinician responses indicated clearly that the implementation of a prognostic tool into clinical practice would require thorough consideration of associated guidance and should be done in collaboration with clinicians.
Discussion
Our STAI findings suggest that there was a reduction in mean state anxiety score 24–48 hours post fFN test. Because all women had qualitative fFN test results disclosed, we are unable to determine whether this reduction in anxiety was associated with provision of qualitative fFN results or not. It is entirely possible that the reduction in the mean state anxiety score reflects the natural course of anxiety following presentation with symptoms of preterm labour. Nevertheless, because a previous study in women without symptoms of preterm labour85 has suggested that fFN results can be associated with an increase in anxiety, it was reassuring to see no evidence of this in our data. These findings are consistent with findings from a Canadian qualitative study. 86
The strengths of this part of the study include that pre- and post-test questionnaires were used, and results were adjusted for baseline anxiety scores and centre. We did not detect any change in trait anxiety following fFN testing, suggesting that the questionnaires performed as expected in this domain. A weakness in the study is the fact that only 14% of women in the study completed both questionnaires, and, because the baseline characteristics of the group that did complete both questionnaires were different from those who completed only a pre-test questionnaire or no questionnaires, the findings may not be generalisable. The findings are also descriptive because we had no comparison group with concealed or no fFN test results.
Further information on women’s anxiety was provided via qualitative interviews. Anxiety was a theme of the women’s interviews; however, not all women were anxious. This finding was reflected in the STAI questionnaire results, which indicated low levels of anxiety throughout for some women and anxiety at different time points or anxiety throughout for others. However, women’s self-report of their anxiety during interviews did not always match their scores, indicating that their levels of anxiety changed over time, or possibly resolution of anxiety because of a good pregnancy outcome. The interview results did not suggest that fFN testing directly affected anxiety for all women. Rather, they indicated that women had different levels of anxiety around the time of fFN testing, mainly related to their overall experience. A comparison with women undergoing clinical assessment for preterm labour without fFN testing would provide more certainty in this finding. This seems at odds with a previous finding that fFN testing increases anxiety to clinically significant levels in women with a positive result. 85 However, the women under investigation were different from the women in this study in important ways: participants in the previous study were all at high risk of preterm birth and were asymptomatic at the time of testing and, hence, would probably have a different anxiety profile. More closely reflecting the results of this study, a qualitative exploration of 17 symptomatic women undergoing fFN testing in Canada86 indicated that women felt anxiety around the time of testing and while awaiting results, but that results brought either relief or reassurance. Women receiving a negative result often reported relief, and those receiving a positive result had confidence in their clinicians’ management plans and felt reassured they were being cared for. However, in our study, some women with a positive result felt shock and anxiety, which for those with a false-positive result resulted in some degree of anxiety for the rest of their pregnancy. Parallels can be drawn with fFN testing and prenatal genetic testing, particularly given that their results can carry an unquantifiable risk for the fetus just as a positive fFN test result may or may not accurately predict preterm labour. In a qualitative investigation of parents’ experiences of being informed about the presence of certain genetic variants,91 parents reported feeling either shock or worry at the results. However, nearly all parents indicated that they would prefer to be told again. 91 Likewise, irrespective of receiving a positive or negative result, or of whether or not their positive result was accurate, all women in our study indicated that they would prefer to have fFN testing again in a future pregnancy.
Our analysis indicates that clinicians and women alike have high confidence in fFN test results, particularly negative results. Prior research suggests that women’s reassurance from a negative result may be enhanced by clinicians’ confidence. 86 Although clinicians and women were well aware of the uncertainty of a positive result, this did not affect the value they placed on this test. The negative effects on parents of diagnostic uncertainty, including dissatisfaction with care and low trust in the clinician, have been identified previously. 92 However, women in this study displayed high levels of confidence in clinicians, demonstrated by their universal acceptance of clinical management plans. Perhaps this is indicative of the quality of the pre-test counselling and information that clinicians provide to women to enable shared decision-making.
Overall, clinicians and women remained well disposed to the benefits of fFN testing, despite acknowledgement of the uncertainty inherent in a positive result. Clinicians appeared to frame their management decisions on two dichotomous philosophies of care: erring on the side of caution and concern about over-treatment. In practice, erring on the side of caution prevailed given clinicians’ concern about the impact of preterm birth in the wrong place or without intervention. Hence, the idea of a prognostic model that may increase the accuracy of the fFN result by incorporating individual factors was viewed with positive anticipation. Prognostic modelling to individualise risk prediction is well established in medicine and has been used for decades in obstetrics, including the Apgar score of neonatal well-being and the Bishop score to assess cervical readiness for induction of labour. One recent systematic review93 indicates that, although new prognostic models are frequently published, few are fully implemented into clinical practice. The clinicians interviewed expressed useful opinions about how the implementation of a prognostic model should be managed.
In summary, fFN testing appears acceptable to women and clinicians. Prognostic modelling to increase accuracy and individualise results is likely to be well received. Current good practice around information provision and shared decision-making can enhance women’s satisfaction with their experience. Implementation of a prognostic model into clinical practice must take account of women’s and clinicians’ informational needs and consider the need for guidance for interpretation.
Chapter 9 QUIDS2: exploratory comparison of the prognostic performance and cost-effectiveness of quantitative fetal fibronectin, Actim Partus and PartoSure
Context
Recent NICE diagnostics guidance14 concluded that there is insufficient evidence to recommend the routine adoption of quantitative fFN or either of the two other biochemical tests related to preterm labour now available in the UK: Actim Partus, which measures phIGFBP-1, and PartoSure, which measures PAMG-1.
The primary aim of QUIDS2 was to provide a preliminary comparison of the independent prognostic value of Actim Partus, PartoSure and quantitative fFN in women with signs and symptoms of preterm labour. Specific objectives were to evaluate the test accuracy of each of the three tests (Actim Partus, PartoSure and quantitative fFN) used in isolation to predict preterm birth within 7 days of testing and to compare the prognostic value of (1) Actim Partus and (2) PartoSure compared with quantitative fFN when adjusted for clinical risk factors.
Methods
Ethics and registration
The study was conducted in accordance with the principles of GCP. The study was approved by the West of Scotland Research Ethics Committee (17/WS/0081). The study was registered with ISRCTN ISRCTN41598423 and the NIHR Central Portfolio Management System (36026) and the protocol was made available on the QUIDS study website in October 2018. It is now available at https://argoshare.is.ed.ac.uk/content/340/ (accessed 20 August 2020).
Population and eligibility
The eligibility criteria were identical to the QUIDS prospective cohort study (see Chapter 6): women with signs and symptoms of preterm labour at 22+0 to 34+6 weeks’ gestation for whom admission, transfer or treatment for preterm labour was being considered.
Once it was established that a participant met the eligibility criteria, preterm birth test swabs were taken. The fFN swab was taken first (from the posterior fornix), followed by the Actim Partus swab (from the endocervix), followed by the PartoSure swab (from the low vagina once the speculum was removed). All tests were carried out as per the manufacturer’s instructions.
Setting
The prospective cohort study took place in 19 consultant-led obstetric units in the UK (see Appendix 15, Table 49). All but two of these units were already participating in QUIDS and recruited women for QUIDS2 alongside QUIDS. To boost recruitment towards the end of the study we also included two additional QUIDS2-only sites: large teaching hospitals in London that already used quantitative fFN.
Participant selection and enrolment
Similar to QUIDS, women with signs and symptoms of preterm labour were identified on presentation to obstetric services. A member of clinical staff, usually the doctor or midwife assessing the woman, identified potentially eligible participants, provided a participant information leaflet and invited consent. A suitably trained member of clinical staff (doctor or midwife) or the research team was responsible for gaining consent from participants. 78
Posters and leaflets were situated in antenatal areas of participating hospitals to alert women that the study was taking place, and women were allowed as much time as possible to consider participation without unduly delaying further clinical assessment.
Screening for eligibility
Potential participants in QUIDS2 were identified after the initial assessment on presentation to maternity services and provided with information about the study at this stage. Informed consent was provided before speculum examination. Samples were collected at routine speculum examination.
Study assessments and data collection
Study assessments were similar to those of the QUIDS study (see Appendix 8, Table 43), apart from sample collection. Samples for preterm birth tests were taken using specimen collection kits as per the manufacturer’s instructions. The fFN swab was taken from the posterior fornix, as per the QUIDS study. In 17 out of 19 QUIDS2 centres the fFN sample was run at a near-bedside Rapid fFN 10Q analyser, as per the QUIDS study, which revealed a qualitative fFN result (positive/negative/invalid based on a 50 ng/ml threshold) for clinicians to base clinical decision-making on, in accordance with local protocols. In the two QUIDS2-only centres, routine clinical analysers were used and quantitative fFN results revealed to clinicians and women.
Actim Partus samples were collected using a sterile polyester swab from the cervix during a speculum examination. 17 The swab was left in the cervix for 10–15 seconds to absorb the secretions and then placed into the provided specimen extraction solution and swirled vigorously for 10 seconds. The swab was then pressed against the wall of the tube to remove any remaining liquid from the swab before being discarded. 17 Samples were stored appropriately, as per the manufacturer’s instructions,17 until tested by the research team/nominated site staff not involved in direct clinical care of the woman. For testing, the yellow dip area of the dipstick was placed into the extracted sample and held until the liquid was seen to enter the result area. 17 The dipstick was then removed and placed on a horizontal surface.
PartoSure tests were taken using the PartoSure test kit, as per the manufacturer’s instructions, using a sterile flocked vaginal swab. 18 It was recommended that the swab was taken after removal of the speculum, with the swab inserted into the vagina (no more than 5–7 cm) and withdrawn after 30 seconds. 18 The sample was then placed into the provided solvent vial and rinsed by rotating for 30 seconds before being discarded. 18 Samples were again stored appropriately until testing by the research team/nominated site staff not involved in direct clinical care of the participant. For testing, the test strip was inserted into the sample and held there until either two lines were present or 5 minutes had elapsed. 18
For both Actim Partus and PartoSure, results were reported as positive, negative or invalid at 5 minutes, with the presence of two lines (test line and control) indicating a positive result, however strong the line was. A negative result was shown by only one line (the control line) and an invalid result was recorded if no lines were present or the sample line only (i.e. no control line) was present. QUIDS2 Actim Partus and PartoSure collection kits were stored in clinical areas and test strips were kept remotely and accessed only by the research team/nominated staff who were doing the testing of the samples, to help avoid disclosure of the results.
All other data were collected in same manner as in the QUIDS study.
Sample size calculation
From the outset, we acknowledged that there was uncertainty in the recruitment rate and event rate in this add-on study to the QUIDS study, and recruitment was constrained by the duration of the QUIDS study (with QUIDS2 commencing only in the final 12 months of the QUIDS study period). At the time of commencement an observed event rate of 3.5% was seen in the QUIDS study. We aimed to recruit approximately 500 women in the prospective cohort study and have 10–25 events (preterm labour within 7 days of test). If the true diagnostic test accuracy sensitivity of a test was 95%, the 95% CI around this would be expected to be ± 10.8%. (i.e. 84.2% to 100%) (see Appendix 16, Table 50).
Statistical analysis
A statistical analysis plan was drawn up and agreed prior to database lock and analysis. Definitions used were the same as those used in the QUIDS study.
Data cleaning
Prior to analysis, data were checked for outliers and missing data identified. Descriptive statistics were used to summarise the data for women included in the cohort study. All events and non-events were verified by assessing the interval between preterm birth test and birth.
Missing data
Missing data are described. Only complete-case analyses were included.
Analysis
Data were cleaned and descriptive analyses were undertaken to summarise the data for participants in the QUIDS2 cohort. Diagnostic test accuracy was calculated from two-by-two tables to provide test sensitivity, specificity, positive likelihood ratio (sensitivity/1 − specificity) and negative likelihood ratio (1− sensitivity/specificity) for Actim Partus and PartoSure alongside qualitative fFN for comparison.
To explore the potential value of including alternative tests in a prognostic model, we developed a prognostic model for spontaneous birth within 7 days of test, using data from women in the QUIDS study who were not co-participating in QUIDS2 based on clinical risk factors alone. We then assessed the incremental value of quantitative fFN, Actim Partus and PartoSure individually, fitting a logistic regression model that included the clinical risk factor model as a single covariate (using restricted cubic splines to allow flexibility), and with each of the three tests in turn as a second covariate in the model. Our focus was on whether or not each of the three tests added prognostic value, that is, whether or not the odds ratio for each test (after adjustment for the existing model) was important statistically. By expressing the clinical risk model as a single predictor, we minimised the number of covariates in the model, maximising the power of the study to detect differences given the very limited number of events.
Results
Recruitment for QUIDS2 is shown in Figure 16. Overall, 554 women were recruited to QUIDS2. Five women were ineligible at speculum examination and were excluded. Baseline characteristics for the remaining 549 women are presented in Table 30. Forty-eight women had one or more preterm birth test result missing (owing to invalid results or technical error), leaving 501 women who were included in the exploratory comparison of preterm birth test performance. A total of 462 women had complete data; levels of missing data are shown in Table 29. The outcome proportion (spontaneous preterm birth within 7 days of test) was lower than anticipated at 1.5%.
FIGURE 16.
Flow chart for the QUIDS2 study.
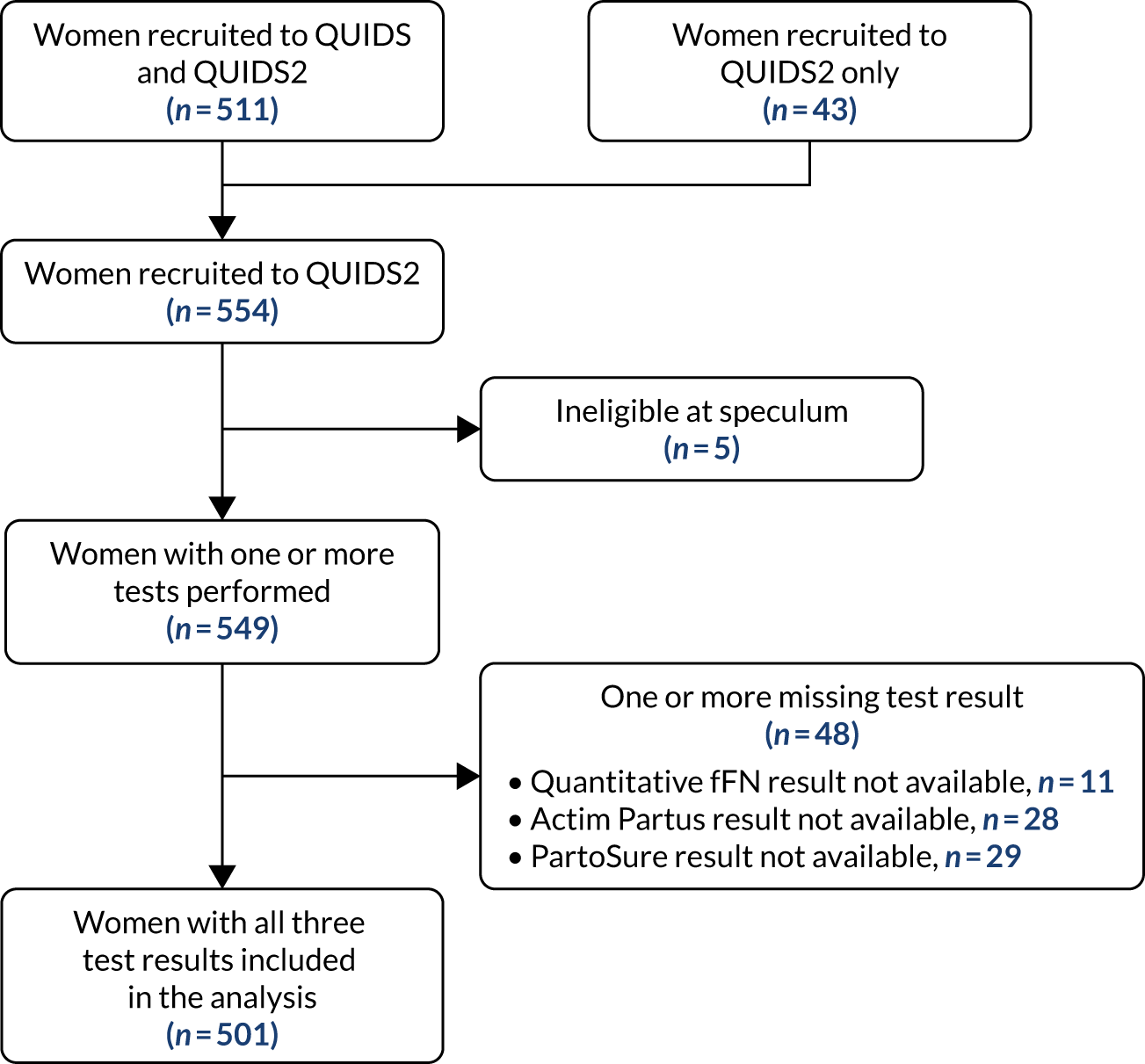
Variable | QUIDS2 (N = 549) | Missing, n (%) |
---|---|---|
Baseline characteristics | ||
Age (years), mean (SD) | 28 (24–32) | 13 (2.4) |
BMI (kg/m2), median (IQR) | 25.1 (22.1–30.0) | 10 (1.8) |
Ethnicity, n (%) | 8 (1.5) | |
White | 468 (85.2) | |
South Asian | 30 (5.5) | |
East Asian | 4 (0.7) | |
African, Caribbean, Middle-Eastern | 27 (4.9) | |
Other | 12 (2.2) | |
Current smoker, n (%) | 110 (20.0) | 5 (0.9) |
Nulliparous, n (%) | 184 (33.5) | 58 (10.6) |
Multiple pregnancy, n (%) | 22 (4.0) | 2 (0.4) |
Gestation (weeks), median (IQR) | 31.0 (28.0–33.0) | 2 (0.4) |
Previous spontaneous preterm birth at < 34 weeks’ gestation, n (%) | 29 (5.3) | 60 (10.9) |
Qualitative fFN: positive, n (%) | 99 (18.0) | 1 (< 1) |
Quantitative fFN (ng/ml), median (IQR) | 8 (4–30) | 1 (< 1) |
Actim Partus: positive, n (%) | 186 (33.9) | 28 (5.1) |
PartoSure: positive, n (%) | 26 (4.7) | 29 (5.3) |
Outcomes, n (%) | ||
Spontaneous preterm birth at < 7 days of test | 8 (1.5) | – |
Table 31 shows the diagnostic test accuracy for Actim Partus and PartoSure alongside qualitative fFN for comparison. Owing to the small number of events, the CIs surrounding the test performance results are very large, limiting comparison. PartoSure had the highest specificity, but this was at the expense of a low sensitivity, with only one out of seven preterm births being preceded by a positive PartoSure test.
Test | Sensitivity (95% CI) | Specificity (95% CI) | Positive likelihood ratio (95% CI) | Negative likelihood ratio (95% CI) |
---|---|---|---|---|
Qualitative fFN | 71.43 (29.04 to 96.33) | 83.0 (79.39 to 86.20) | 4.20 (2.53 to 6.98) | 0.34 (0.11 to 1.11) |
Actim Partus | 57.14 (18.41 to 90.10) | 64.57 (60.18 to 68.80) | 1.61 (0.84 to 3.10) | 0.66 (0.28 to 1.57) |
PartoSure | 14.29 (0.36 to 57.87) | 95.34 (93.10 to 97.03) | 3.07 (0.48 to 19.67) | 0.90 (0.66 to 1.22) |
A total of 2422 women participated in the QUIDS study but not in QUIDS2. Among these, 2252 had complete data. We used this cohort to develop a model for preterm birth within 7 days of testing, based on clinical predictors alone. The clinical predictors included were age, BMI, ethnicity, gestational age at assessment, multiple pregnancy, nulliparity, previous spontaneous preterm birth before 34 weeks’ gestation and smoking. We fitted the resulting model to the women who participated in QUIDS2 (n = 462; complete case analysis). The linear predictor of this model was calculated for each woman and included in the clinical model as a single variable, shown as ‘clinical risk model’ in Table 32.
Variable | Clinical risk model (n = 462; complete case analysis) | Clinical risk model plus quantitative fFN | Clinical risk model plus Actim Partus | Clinical risk model plus PartoSure | ||||||||
---|---|---|---|---|---|---|---|---|---|---|---|---|
Intercept (95% CI) | –3.139 (–6.296 to 0.018) | –4.564 (–7.867 to –1.261) | –3.483 (–6.798 to –0.169) | –3.648 (–6.964 to 15.341) | ||||||||
Variable | Beta | OR (95% CI) | p-value | Beta | OR (95% CI) | p-value | Beta | OR (95% CI) | p-value | Beta | OR (95% CI) | p-value |
Clinical risk linear predictor | 0.319 | 1.375 (0.556 to 3.401) | 0.491 | 0.087 | 1.091 (0.459 to 2.592) | 0.843 | 0.286 | 1.33 (0.542 to 3.271) | 0.532 | 0.206 | 1.229 (0.493 to 6.839) | 0.658 |
Preterm birth test result | – | – | – | 0.006 | 1.006 (1.002 to 1.010) | 0.005 | 0.545 | 1.724 (0.340 to 8.734) | 0.511 | 1.244 | 3.471 (0.347 to 25.717) | 0.289 |
Nagelkerke R2 | 0.0075 | 0.111 | – | 0.015 | – | 0.023 | – | |||||
Residual deviance | 62.625 | 56.492 | – | 62.197 | – | 61.733 | – | |||||
Difference in residual deviance | – | 6.133 | 0.013 | 0.428 | 0.513 | 0.891 | 0.345 |
Three new models were then created by combining the clinical risk model linear predictor with each of the three tests of preterm labour: quantitative fFN, Actin Partus and PartoSure. Only quantitative fFN showed added prognostic value, with a statistically significant odds ratio after adjustment for the existing model, and significant difference in residual deviance between the clinical risk model and the clinical risk model plus quantitative fFN. This supports the hypothesis that quantitative fFN has the best prognostic value or the three tests when included in a prognostic model with clinical risk factors.
The clinical risk model included eight clinical predictors [age, BMI, ethnicity, gestational age at assessment, multiple pregnancy (singleton vs. twins), nulliparity, previous spontaneous preterm birth before 34 weeks’ gestation and smoking]. The linear predictor of this model was calculated for each woman and included in the clinical model as a single variable.
Discussion
To the best of our knowledge, this study is the largest study of PartoSure to date and the only study in which the three tests for preterm birth that are available in the UK (quantitative fFN, Actim Partus and PartoSure) are directly compared in samples from the same women. Nevertheless, there is considerable uncertainty about the findings as a result of the small number of events.
We aimed to recruit as many women as possible to QUIDS2 within the constraints of the QUIDS recruitment period that remained once we had confirmation of funding and governance approvals for the study. We had anticipated that we would recruit around 500 participants and have around 25 events, sufficient to perform exploratory analysis comparing the three tests for preterm birth. However, the event rate in QUIDS2 was lower than that in the QUIDS study. The reasons for this are unclear but may relate to the fact that, in QUIDS2, to mask tests results from clinicians, staff not in the clinical care team were required to run the Actim Partus and PartoSure tests. Recruitment in many centres was, therefore, reliant on research staff, limiting recruitment to daytime hours.
The number of events within the QUIDS2 cohort was at the lowest bounds of the CI for our sample size estimate. With such a limited number of events, QUIDS2 was underpowered for comparison of test performance. However, the test accuracy data for qualitative fFN and Actim Partus are consistent with the findings of previous studies. 11 The sensitivity of PartoSure was low, with only one out of seven events identified by a positive PartoSure test. Even if the sensitivity of PartoSure was at the upper end of the CI, the false-negative rate would still be unacceptable (≈ 57%).
In an attempt to explore test performance in a prognostic model for birth within 7 days with only a limited number of events, we created a model based on clinical risk factors and expressed it as a single covariate. We then combined this with each test as an additional predictor. Only quantitative fFN significantly contributed to model performance. This suggests that it is the best of the three tests for inclusion in a prognostic model with clinical risk factors.
The strengths of this study include that tests were obtained from the same women, allowing direct comparison of test performance. However, a major weakness is the number of events, which limits interpretation.
In summary, in the QUIDS study we developed and validated a model using rigorous methodology. Our exploratory analysis in QUIDS2 provides no evidence that an alternative model based on a different test of preterm birth would be superior to a model using quantitative fFN.
Chapter 10 Presentation of the prognostic model to aid decision-making, implementation and future work
In the QUIDS study we developed a number of different prognostic models for the prediction of spontaneous preterm birth. Our primary analysis aimed to externally validate the most parsimonious model for prediction of spontaneous preterm birth within 7 days of testing. This model had excellent performance and good calibration (following updating of the intercept). The model was robust to a number of sensitivity analyses. However, the precision around the estimates for ethnicity were wide owing to small numbers of study participants in certain ethnicity categories. After consultation with the project management group and clinicians, we elected to collapse ethnicity into two categories: white and other. This is the model that we present for clinical use and its equation is provided below. It includes quantitative fFN and four clinical risk factors [current smoking (yes/no), nulliparity (yes/no), non-white ethnicity (yes/no) and multiple pregnancy (yes/no)]. The AUC for this model as presented is 0.89 (0.86 to 0.92), with a calibration slope of 1.24 and Nagelkerke R2 of 0.34:
We have developed a prototype risk predictor based on the QUIDS model for prediction of preterm birth within 7 days (Figure 17). We present the chance of birth as a percentage, which our qualitative work suggested was acceptable to women. A suggestion from clinicians is that it might be more appropriate to discuss the chance of remaining pregnant at 7 days (rather than the probability of spontaneous preterm birth). This is an easy conversion, obtained by subtracting the percentage from 100.
FIGURE 17.
Screenshot of decision support tool.
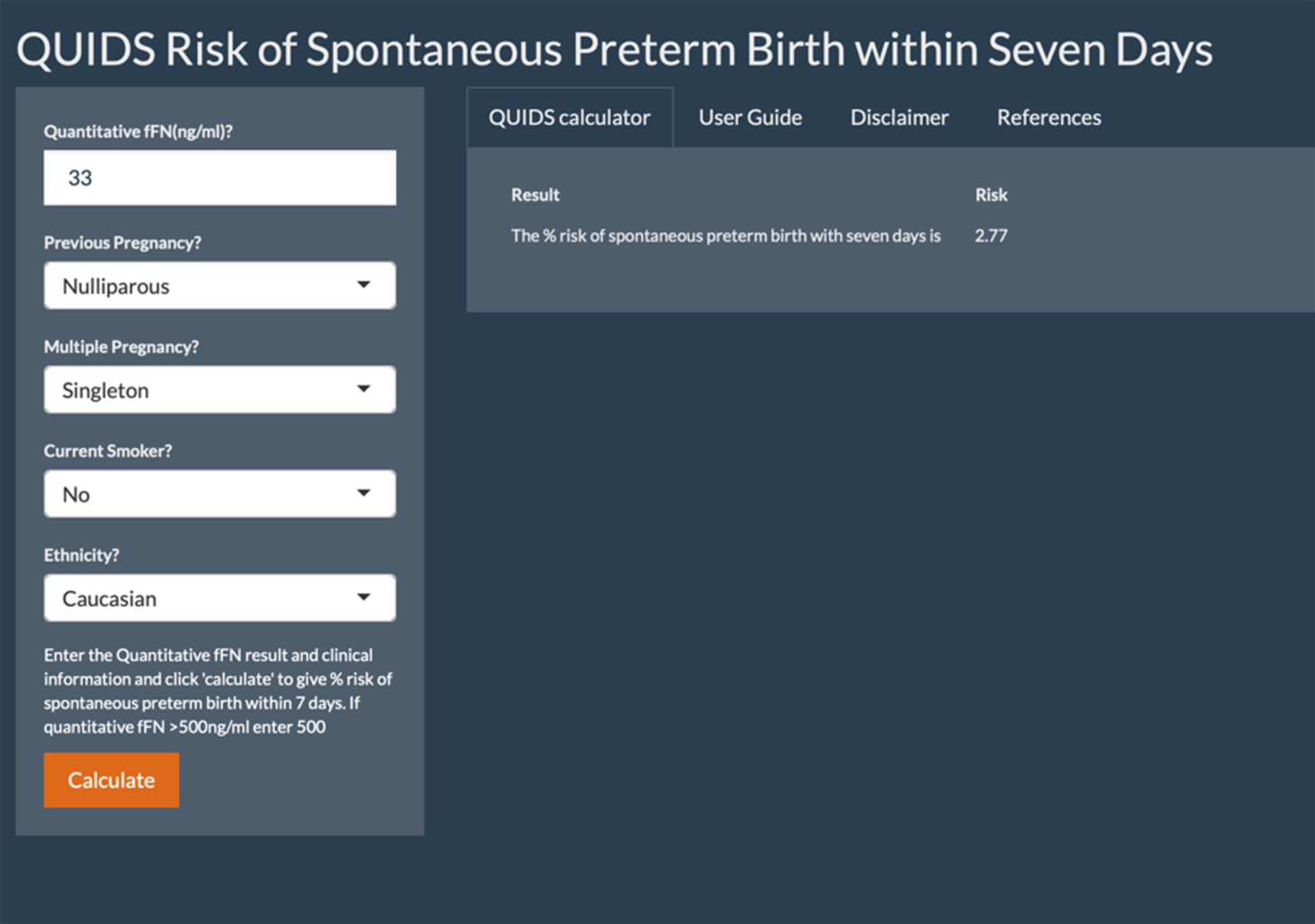
Our aim is to embed the decision support tool into electronic maternity records, to enable it to be used at the point of care and work, and to develop an app. This will allow ongoing data collection from users of the model, allowing further refinement and validation in future. For example, we could further refine estimates of different ethnicities as predictors of preterm birth.
It is generally believed that the simplest prognostic model is preferred in clinical practice. However, there was a suggestion in the qualitative interviews with clinicians (see Chapter 8) that a model that did not include risk factors, such as previous spontaneous preterm birth, may be over-ruled. Although inclusion of such additional risk factors did not improve the model performance from a statistical perspective, it may enhance women’s and clinicians’ confidence in the model. For this reason, a model with all prespecified clinical risk factors included may be preferable. In our secondary analysis, a model with all eight prespecified clinical risk factors forced into the model had near-identical discrimination as the more parsimonious model; in addition, after recalibration it had good calibration. It performed marginally less well on net benefit analysis. The downside of this model is that it is more complicated and there is more potential that missing data will mean that the model will not work. Initial consultation with clinicians on presentation of the QUIDS findings has suggested that the more parsimonious model is preferable. However, we will perform further work with women and clinicians at the implementation stage to determine if, in practice, a fuller model may be more acceptable.
Although we also developed a prognostic model for spontaneous preterm birth within 48 hours of testing, we believe that this should have further validation before implementation in the NHS. This is an aim of future work, and we anticipate that it can be performed on routinely collected data once the model is embedded in electronic health records. We also developed prognostic models that included cervical length. Inclusion of cervical length did not improve model performance and did not appear to be cost-effective, but there was uncertainty surrounding the data owing to the number of missing values and proportion of data from outside the UK. Cervical length measurement is not routinely available in the UK, so validating a model without this predictor was also pragmatic. Nevertheless, the models with cervical length could be validated in other settings in which cervical length is available in the future, for example the USA or mainland Europe.
In summary, we present a risk predictor for preterm birth in women with symptoms of preterm labour based on our externally validated QUIDS model. This presents, the chance of preterm birth within 7 days. The risk can easily be converted to the chance of remaining pregnant at 7 days by subtracting the percentage from 100. Details of alternative models are provided in Chapter 6.
Chapter 11 QUIDS public and parent involvement
We had one lay co-applicant, Susan Harper-Clarke. Throughout the project, Susan was a key member of the project management team and attended project management meetings. She led the review of parent-facing materials and advised on aspects of the study including recruitment, design of QUIDS2 and presentation of the decision support.
We had initially included another lay co-applicant with the intention that she would support Susan in the management group. However, she stopped responding to contact soon after the project commenced, and despite repeated efforts we were unable to re-engage.
Susan was supported by members of two parental advisory groups, one based at St Thomas’ Hospital and one at the University of Edinburgh. Members of these groups were consulted on an ad hoc basis regarding study processes and management. For example, they were consulted on the acceptability of additional sample collection for QUIDS2, concealing QUIDS2 test results and presentation of the decision tool. Although an initial intention was to create a specific QUIDS parental advisory group, we found it difficult to keep members engaged throughout the main part of the project (during which less parental involvement was required). We found ad hoc consultation from a wider group worked better in this case.
We had two lay members on the project steering committee: Zing Gardiner and Ben Wills. They contributed to project steering committee meetings and provided feedback on all aspects of the study, including dissemination. Zing attended training days and contributed to the programme for staff training, which was a strategy we found particularly useful and got good feedback from research sites.
Lay members were reimbursed for their time with honoraria in the form of vouchers.
We have strong links with the baby charity Tommy’s (https://www.tommys.org; accessed 16 May 2020). Tommy’s will be consulted on dissemination and implementation, and we anticipate using its large parental network to leverage this.
Chapter 12 Discussion and conclusions
Summary of findings
-
In QUIDS qualitative, women, their partners and clinicians indicated that birth within 7 days of testing would be the best end point for a prognostic model that predicts preterm birth in women who present with symptoms of preterm labour.
-
In the QUIDS IPD meta-analysis we used data from existing studies of quantitative fFN to develop six models for the prediction of spontaneous preterm birth within 7 days:
-
All models included quantitative fFN and clinical risk factors, and we developed models with and without cervical length measurement.
-
We found that all six models performed similarly in terms of discrimination, with AUCs of around 0.90.
-
Net benefit analysis suggested that little additional clinical benefit was likely from the inclusion of cervical length measurement in the prognostic model.
-
Cost-effectiveness analysis suggested that quantitative fFN dominates qualitative fFN (saves costs and improves QALDs) and that the quantitative fFN prognostic model was superior to those that included cervical length measurement in terms of probability of correct diagnosis and NMB outcomes with a willingness-to-pay threshold of £54 per QALD (equivalent to the £20,000-per-QALY threshold recommended by NICE).
-
-
We externally validated the prognostic models that included clinical risk factors and quantitative fFN in the QUIDS prospective cohort study in 26 NHS maternity units. The most parsimonious model (based on quantitative fFN and four clinical predictors) performed well and, after updating for the UK population, had an AUC of 0.89 (95% CI 0.84 to 0.93), calibration-in-the-large of 6.42 × 10–14 and calibration slope of 1.204. The model was stable across a number of prespecified sensitivity analyses.
-
The cost-effectiveness analysis found the prognostic tool to be highly cost-effective over a lifetime horizon and that a risk threshold of ≥ 2% is optimal.
-
In comparison with qualitative fFN, the prognostic tool has an additional cost of £3, with a 0.0015 QALY gain, resulting in an ICER of £2000 per QALY. This is highly cost-effective given the recommended NICE threshold of £20,000 per QALY.
-
An exploratory analysis of two alternative biochemical tests of preterm birth: PartoSure and Actim Partus. There was considerable uncertainty in test performance estimates owing to the small number of events, severely limiting interpretation of the results. However, there was no indication that either test would perform substantially better than quantitative fFN individually, as a diagnostic test or within a prognostic model.
Effectiveness and acceptability of the intervention
Our findings suggest that we have a robust model for prediction of preterm birth within 7 days in women who have signs and symptoms of preterm labour, which could have clinical benefit. Qualitative research embedded within the QUIDS study found that fFN testing is acceptable to women and clinicians and that the prognostic model is likely to be well received. Further work is required to see if the model is effective in clinical practice at reducing unnecessary treatments for preterm birth.
Strengths and limitations
Strengths of the study include the following:
-
Outcomes were clinician and women focused and were informed by a preceding qualitative study.
-
A rigorous methodology was used for model development and validation, adhering to prespecified published protocols and reporting guidelines.
-
The study was large.
Limitations of the study include the following:
-
The outcome proportion (spontaneous preterm birth within 7 days of test) was 2.9% in the validation study. This is in line with other studies, but having slightly fewer than 100 events is a limitation of model validation.
-
The outcome proportion in QUIDS2 was even lower and any comparison between quantitative fFN, Actim Partus and PartoSure can only be considered exploratory.
-
There were relatively low numbers of non-white participants.
-
The study was not designed to determine clinical effectiveness of the prognostic model.
Other methodological issues
-
We were unable to evaluate cervical length in the prospective cohort owing to small numbers of women having this investigation. In the QUIDS IPD meta-analysis we found that cervical length added little to the QUIDS model performance, with no likely clinical benefit seen on net benefit analysis. However, there was some uncertainty about the data included, because many data came from studies carried out in mainland Europe. Pragmatically, cervical length could not be widely, routinely offered in the UK without considerable training and investment in obstetric services, whereas the QUIDS model can be readily implemented.
-
The majority of women participating in the QUIDS study had a low risk of preterm birth within 7 days of testing, with the vast majority of events occurring in the highest decile of risk, but this still equated to a risk of around only 20%. The threshold for ruling out preterm birth using the QUIDS model is likely to be relatively low.
-
There was only a small difference in costs and QALYs across alternative admit probability threshold strategies, so uncertainty remains surrounding the optimal treatment threshold to use with the prognostic model from a health economic perspective. This is driven by the uncertainty surrounding the true health and monetary cost of infants who are born preterm to mothers who are not given treatment (i.e. false negatives).
Interpretation of results
The QUIDS model is a robust model to help guide management of women who present with symptoms and signs of preterm labour. By providing a personalised risk of impending birth, unnecessary treatments might be avoided and resources can be directed towards women and babies who are most in need. Interpretation of the level of risk is likely to depend on a number of factors, for example health-care setting, proximity to appropriate neonatal facilities, gestational age and the woman’s home circumstances. A relatively low ‘rule-out’ threshold is likely to be used to avoid false-negative results, but our net benefit analysis suggests that even this is likely to have clinical benefit by avoiding unnecessary treatments. An important point is that the model was validated within the setting of current clinical care. The model cannot replace clinical care, nor should it indicate absence of ongoing care, but is aimed to be used within current care provision.
Implications for health care
The QUIDS prognostic model including quantitative fFN and clinical risk factors showed excellent performance in the prediction of spontaneous preterm birth within 7 days of testing, and can be used to inform a decision support tool to help guide management decisions for women with threatened preterm labour. It is readily implementable within existing structures within the NHS. It is likely to have immediate benefit to women and babies and health services through avoidance of unnecessary admission and treatment.
Future research implications
It is our intention that the prognostic model will be embedded in electronic maternity records and an app, enabling ongoing data collection for further refinement and validation of the model.
Further research on implementation is warranted, for example to explore the preferred format of the model and to determine whether ‘full’ or ‘parsimonious’ versions of the model are more credible.
Other variables (such as previous cervical treatment for CIN, the number of contractions, presence or absence of vaginal bleeding, cervical dilatation and deprivation index and ethnicity category) may have potential to improve the prognostic performance of the prognostic model. Model refinement should be explored in future analyses.
External validation of the model for birth within 48 hours is required in another data set.
An evaluation of the effectiveness of using the prognostic tool is required and the impact on clinical care would be useful, for example through a stepped wedge or interrupted time series (quasi-experimental design).
Further research aimed at understanding the full cost and health outcome impact of preterm labour is warranted, particularly surrounding the true health and monetary cost of infants who are born preterm to mothers who are not given treatment (i.e. false-negatives).
Acknowledgements
We gratefully acknowledge the women who participated in the research study, the research team members at QUIDS sites (listed in Appendix 17) and all others who contributed to the screening, recruitment and outcome data collection.
We gratefully acknowledge the contribution of Ediri O’Brien (Senior Trial Co-ordinator, University of Liverpool) to the qualitative research and for covering Helen White’s period of maternity leave.
Many thanks to Angel Niven, the study administrator for the QUIDS project, and to Rebecca Lees and Mary Paterson for administrative help. Many thanks to Elspeth Horne for statistical advice and support and Laura Bonnett for support with R code.
We are particularly grateful to the members of the study steering committee, Philip Bennett (chairperson), Melissa Whitworth, Olivia Wu, Peter Blair, Zing Gardiner and Ben Wills.
We are also grateful to:
-
Hologic, Inc., for
-
providing adapted analysers at each site, allowing quantitative fFN values to be masked from clinicians
-
offering training and support to each site for fFN testing
-
allowing QUIDS sites to purchase tests at the lowest list price (NHS treatment cost).
-
-
Parsagen Diagnostics, Inc., for gifting PartoSure test kits for QUIDS2 and offering training and support to sites.
-
Medix Biochemica Ab for provided test kits at a reduced cost and offering training and support to sites.
These companies had no other involvement in the study design, implementation, analysis or interpretation of results.
Contributions of authors
Sarah J Stock (https://orcid.org/0000-0003-4308-856X) (Senior Clinical Lecturer and Honorary Consultant and Subspecialist in Maternal and Fetal Medicine) was the chief investigator. She conceived the study, co-wrote the original grant application, co-designed the study, co-wrote the protocol and analysis plan, performed some analyses, interpreted the study findings and led the drafting of the report.
Margaret Horne (https://orcid.org/0000-0001-7621-2462) (Statistician) co-wrote the statistical analysis plan and analysed the data for the external validation of the prognostic models and QUIDS2 data, and assisted with the drafting of the report.
Merel Bruijn (https://orcid.org/0000-0003-0788-4438) (Statistician) co-wrote the statistical analysis plan, led the IPD meta-analysis, led QUIDS model development and internal validation, and assisted with the drafting of the report.
Helen White (https://orcid.org/0000-0001-7823-7831) (Clinical Lecturer in Midwifery) led qualitative aspects of the study, wrote the QUIDS qualitative protocol and analysis plans, performed qualitative research and assisted with the drafting of the report.
Robert Heggie (https://orcid.org/0000-0001-7396-4773) (Research Assistant and Health Economist) performed health economic analyses and assisted with the drafting of the report.
Lisa Wotherspoon (https://orcid.org/0000-0003-4110-5493) (Study Manager) managed the study, trained staff, contributed to data collection, interpreted the trial findings and assisted with the drafting of the report.
Kathleen Boyd (https://orcid.org/0000-0002-9764-0113) (Senior Lecturer in Health Economics) was a co-applicant who co-wrote the original grant application, co-designed the study, co-wrote the protocol and health economic analysis plan, supervised health economic analyses and assisted with the drafting of the report.
Lorna Aucott (https://orcid.org/0000-0001-6277-7972) (Trial Unit Senior Statistician) provided data, supervised and performed analyses and assisted with the drafting of the report.
Rachel K Morris (https://orcid.org/0000-0003-1247-429X) (Reader in Maternal Fetal Medicine) was a co-applicant who co-wrote the original grant application, co-designed the study, contributed to protocol, interpreted the study findings and reviewed the report.
Jon Dorling (https://orcid.org/0000-0002-1691-3221) (Professor of Neonatal-Perinatal Medicine) was a co-applicant who contributed to the original grant application and design of the study, contributed to the protocol, interpreted the study findings and reviewed the report.
Lesley Jackson (https://orcid.org/0000-0001-8200-1987) (Neonatologist) was a co-applicant who contributed to the original grant application and design of the study, contributed to protocol and analysis plans, interpreted the study findings and reviewed the report.
Manju Chandiramani (https://orcid.org/0000-0002-8024-6339) (Obstetrician) was a co-applicant who contributed to the original grant application and design of the study, interpreted the study findings and reviewed the report.
Anna David (https://orcid.org/0000-0002-0199-6140) (Professor of Obstetrics and Maternal Fetal Medicine) provided data for the IPD meta-analysis, contributed to study protocols, interpreted the study findings and reviewed the report.
Asma Khalil (https://orcid.org/0000-0003-2802-7670) (Professor of Obstetrics and Maternal Fetal Medicine) was a co-applicant who provided data for the IPD meta-analysis, contributed to study protocols, interpreted the study findings and reviewed the report.
Andrew Shennan (https://orcid.org/0000-0001-5273-3132) (Professor of Obstetrics) was a co-applicant who helped conceive the study; contributed to the original grant application, protocol and analysis plan; provided data for the IPD meta-analysis; interpreted the study findings; and reviewed the report.
Gert-Jan van Baaren (https://orcid.org/0000-0002-7656-6081) (Obstetrician) provided data for the IPD meta-analysis, contributed to study protocols, interpreted the study findings and reviewed the report.
Victoria Hodgetts-Morton (https://orcid.org/0000-0001-9817-4313) (Clinical Research Fellow, Obstetrics) contributed to qualitative research, contributed to study protocols, interpreted the study findings and reviewed the report.
Tina Lavender (https://orcid.org/0000-0003-1473-4956) (Professor of Midwifery) was a co-applicant who oversaw all qualitative research aspects, co-wrote the original grant application, co-designed the study, co-wrote the protocol, interpreted the study findings and reviewed the report.
Ewoud Schuit (https://orcid.org/0000-0002-9548-3214) (Assistant Professor in Epidemiology) contributed to the statistical analysis plan, co-supervised IPD meta-analysis and model development and internal validation, contributed to interpretation and reviewed the report.
Susan Harper-Clarke (https://orcid.org/0000-0001-8170-4170) (PPI Representative) was a co-applicant who contributed to the original grant application and protocol, interpreted the study findings and reviewed the report.
Ben Mol (https://orcid.org/0000-0001-8337-550X) (Professor of Obstetrics and Gynaecology) was a co-applicant who helped conceive the study; contributed to the original grant application, protocol and analysis plan; provided data for the IPD meta-analysis; interpreted the study findings; and reviewed the report.
Richard D Riley (https://orcid.org/0000-0001-8699-0735) (Professor of Biostatistics) was a co-applicant who helped conceive the study; contributed to the original grant application, protocol and statistical analysis plans; provided training and support to analysis teams regarding prognostic research methodology; interpreted the study findings; and contributed to the report.
Jane Norman (https://orcid.org/0000-0001-6031-6953) (Dean for the Faculty of Health Sciences) was a co-applicant who helped conceive the study; contributed to the original grant application, protocol and statistical analysis plans; interpreted the study findings; contributed to the report; and provided mentorship and training to the chief investigator.
John Norrie (https://orcid.org/0000-0001-9823-9252) (Professor of Medical Statistics and Trial Methodology) was senior study statistician and a co-applicant who had overall responsibility for the analyses. He helped conceive the study, contributed to the original grant application, co-wrote the protocol and statistical analysis plans, interpreted the study findings and contributed to drafting the report.
Publications
Stock SJ, Wotherspoon LM, Boyd KA, Morris RK, Dorling J, Jackson L, et al. Quantitative fibronectin to help decision-making in women with symptoms of preterm labour (QUIDS) part 1: individual participant data meta-analysis and health economic analysis. BMJ Open 2018;8:e020796.
Stock SJ, Horne M, Brujin M, White H, Boyd KA, Heggie R, et al. Development and validation of a risk prediction model of preterm birth for women with preterm labour symptoms (the QUIDS study): a prospective cohort study and individual participant date meta-analysis. PLOS Med 2021;18:e1003686.
Data-sharing statement
On completion of our analyses and publication of related manuscripts, the anonymised, cleaned IPD data set (including QUIDS cohort data) will be made freely available through a recognised biorepository.
Patient data
This work uses data provided by patients and collected by the NHS as part of their care and support. Using patient data is vital to improve health and care for everyone. There is huge potential to make better use of information from people’s patient records, to understand more about disease, develop new treatments, monitor safety, and plan NHS services. Patient data should be kept safe and secure, to protect everyone’s privacy, and it’s important that there are safeguards to make sure that it is stored and used responsibly. Everyone should be able to find out about how patient data are used. #datasaveslives You can find out more about the background to this citation here: https://understandingpatientdata.org.uk/data-citation.
Disclaimers
This report presents independent research funded by the National Institute for Health Research (NIHR). The views and opinions expressed by authors in this publication are those of the authors and do not necessarily reflect those of the NHS, the NIHR, NETSCC, the HTA programme or the Department of Health and Social Care. If there are verbatim quotations included in this publication the views and opinions expressed by the interviewees are those of the interviewees and do not necessarily reflect those of the authors, those of the NHS, the NIHR, NETSCC, the HTA programme or the Department of Health and Social Care.
References
- Office for National Statistics . Gestation-Specific Infant Mortality in England and Wales 2012 2014. www.ons.gov.uk/peoplepopulationandcommunity/healthandsocialcare/causesofdeath/datasets/gestationspecificinfantmortalityinenglandandwalesreferencetables (accessed 19 September 2019).
- Norman JE, Morris C, Chalmers J. The effect of changing patterns of obstetric care in Scotland (1980–2004) on rates of preterm birth and its neonatal consequences: perinatal database study. PLOS Med 2009;6. https://doi.org/10.1371/journal.pmed.1000153.
- Kenyon SL, Taylor DJ, Tarnow-Mordi W. ORACLE Collaborative Group . Broad-spectrum antibiotics for spontaneous preterm labour: the ORACLE II randomised trial. ORACLE Collaborative Group. Lancet 2001;357:989-94. https://doi.org/10.1016/S0140-6736(00)04234-3.
- Macintyre-Beon C, Jackson L, Booth P, Cameron A. Perinatal Collaborative Transport Study. Edinburgh: NHS Quality Improvement Scotland; 2008.
- Wilson AM, MacLean D, Skeoch CH, Jackson L. An evaluation of the financial and emotional impact of in utero transfers upon families: a Scotland–wide audit. Infant 2010;6:38-40.
- Roberts D, Brown J, Medley N, Dalziel SR. Antenatal corticosteroids for accelerating fetal lung maturation for women at risk of preterm birth. Cochrane Database Syst Rev 2017;3. https://doi.org/10.1002/14651858.CD004454.pub3.
- Asztalos EV, Murphy KE, Willan AR, Matthews SG, Ohlsson A, Saigal S, et al. Multiple courses of antenatal corticosteroids for preterm birth study: outcomes in children at 5 years of age (MACS-5). JAMA Pediatr 2013;167:1102-10. https://doi.org/10.1001/jamapediatrics.2013.2764.
- Lumbiganon P. Magnesium Sulfate for Women at Risk of Preterm Birth for Neuroprotection of the Fetus: RHL Commentary (last revised: 1 July 2009). Geneva: WHO Reproductive Health Library; 2009.
- de Heus R, Mol BW, Erwich JJ, van Geijn HP, Gyselaers WJ, Hanssens M, et al. Adverse drug reactions to tocolytic treatment for preterm labour: prospective cohort study. BMJ 2009;338. https://doi.org/10.1136/bmj.b744.
- Stock SJ, Morris RK, Chandiramani M, Shennan AH, Norman JE. Variation in management of women with threatened preterm labour. Arch Dis Child Fetal Neonatal Ed 2015;100. https://doi.org/10.1136/archdischild-2014-307806.
- Honest H, Forbes CA, Durée KH, Norman G, Duffy SB, Tsourapas A, et al. Screening to prevent spontaneous preterm birth: systematic reviews of accuracy and effectiveness literature with economic modelling. Health Technol Assess 2009;13. https://doi.org/10.3310/hta13430.
- Deshpande SN, van Asselt AD, Tomini F, Armstrong N, Allen A, Noake C, et al. Rapid fetal fibronectin testing to predict preterm birth in women with symptoms of premature labour: a systematic review and cost analysis. Health Technol Assess 2013;17. https://doi.org/10.3310/hta17400.
- National Institute for Health and Care Excellence (NICE) . Preterm Labour and Birth. NICE Guideline (NG25) 2015.
- National Institute for Health and Care Excellence (NICE) . Biomarker Tests to Help Diagnose Preterm Labour in Women With Intact Membranes. Diagnostics Guidance (DG33) 2018.
- Stock SJ, Horne M, Brujin M, White H, Boyd KA, Heggie R, et al. Development and validation of a risk prediction model of preterm birth for women with preterm labour symptoms (the QUIDS study): a prospective cohort study and individual participant date meta-analysis. PLOS Med 2021;18. https://doi.org/10.1371/journal.pmed.1003686.
- Hologic, Inc . Rapid FFN® 10Q Cassette Kit n.d. www.hologic.com/sites/default/files/package-insert/AW-09189-002_004_02.pdf (accessed 19 September 2019).
- Medix Biochemica Ab . Actim® Partus: Instructions for Use n.d. www.medixbiochemica.com/wp-content/uploads/2017/06/Käyttöohje-Actim-Partus_AOACE31931_LR.pdf (accessed 19 September 2019).
- Parsagen Diagnostics, Inc . PartoSure™ Test n.d. www.accessdata.fda.gov/cdrh_docs/pdf16/P160052C.pdf (accessed 19 Septenber 2019).
- Riley RD, Hayden JA, Steyerberg EW, Moons KG, Abrams K, Kyzas PA, et al. Prognosis Research Strategy (PROGRESS) 2: prognostic factor research. PLOS Med 2013;10. https://doi.org/10.1371/journal.pmed.1001380.
- Steyerberg EW, Moons KG, van der Windt DA, Hayden JA, Perel P, Schroter S, et al. Prognosis Research Strategy (PROGRESS) 3: prognostic model research. PLOS Med 2013;10. https://doi.org/10.1371/journal.pmed.1001381.
- Hemingway H, Croft P, Perel P, Hayden JA, Abrams K, Timmis A, et al. Prognosis research strategy (PROGRESS) 1: a framework for researching clinical outcomes. BMJ 2013;346. https://doi.org/10.1136/bmj.e5595.
- Hingorani AD, Windt DA, Riley RD, Abrams K, Moons KG, Steyerberg EW, et al. Prognosis research strategy (PROGRESS) 4: stratified medicine research. BMJ 2013;346. https://doi.org/10.1136/bmj.e5793.
- Riley RD, Ensor J, Snell KI, Debray TP, Altman DG, Moons KG, et al. External validation of clinical prediction models using big datasets from e-health records or IPD meta-analysis: opportunities and challenges. BMJ 2016;353. https://doi.org/10.1136/bmj.i3140.
- Steyerberg EW, Vickers AJ, Cook NR, Gerds T, Gonen M, Obuchowski N, et al. Assessing the performance of prediction models: a framework for traditional and novel measures. Epidemiology 2010;21:128-38. https://doi.org/10.1097/EDE.0b013e3181c30fb2.
- Vickers AJ, Elkin EB. Decision curve analysis: a novel method for evaluating prediction models. Med Decis Making 2006;26:565-74. https://doi.org/10.1177/0272989X06295361.
- Alfirevic Z, Allen-Coward H, Molina F, Vinuesa CP, Nicolaides K. Targeted therapy for threatened preterm labor based on sonographic measurement of the cervical length: a randomized controlled trial. Ultrasound Obstet Gynecol 2007;29:47-50. https://doi.org/10.1002/uog.3908.
- Harrison MJ, Kushner KE, Benzies K, Rempel G, Kimak C. Women’s satisfaction with their involvement in health care decisions during a high-risk pregnancy. Birth 2003;30:109-15. https://doi.org/10.1046/j.1523-536X.2003.00229.x.
- Porcellato L, Masson G, O’Mahony F, Jenkinson S, Vanner T, Cheshire K, et al. ‘It’s something you have to put up with’ – service users’ experiences of in utero transfer: a qualitative study. BJOG 2015;122:1825-32. https://doi.org/10.1111/1471-0528.13235.
- Coster-Schulz MA, Mackey MC. The preterm labor experience: a balancing act. Clin Nurs Res 1998;7:335-59. https://doi.org/10.1177/105477389800700402.
- Smith J, Firth J. Qualitative data analysis: the framework approach. Nurse Res 2011;18:52-6. https://doi.org/10.7748/nr2011.01.18.2.52.c8284.
- Gergen KJ. Realities and Relationships: Soundings in Social Constructionism. Cambridge, MA: Harvard University Press; 1994.
- Côté-Arsenault D, Marshall R. One foot in-one foot out: weathering the storm of pregnancy after perinatal loss. Res Nurs Health 2000;23:473-85. https://doi.org/10.1002/1098-240X(200012)23:6<473::AID-NUR6>3.0.CO;2-I.
- O’Brien ET, Quenby S, Lavender T. Women’s views of high risk pregnancy under threat of preterm birth. Sex Reprod Healthc 2010;1:79-84. https://doi.org/10.1016/j.srhc.2010.05.001.
- Weiss ME, Saks NP, Harris S. Resolving the uncertainty of preterm symptoms: women’s experiences with the onset of preterm labor. J Obstet Gynecol Neonatal Nurs 2002;31:66-7. https://doi.org/10.1111/j.1552-6909.2002.tb00024.x.
- Palmer L, Carty E. Deciding when it’s labor: the experience of women who have received antepartum care at home for preterm labor. J Obstet Gynecol Neonatal Nurs 2006;35:509-15. https://doi.org/10.1111/j.1552-6909.2006.00070.x.
- Côté-Arsenault D, Donato KL, Earl SS. Watching & worrying: early pregnancy after loss experiences. MCN Am J Matern Child Nurs 2006;31:356-63. https://doi.org/10.1097/00005721-200611000-00005.
- Elwyn G, O’Connor A, Stacey D, Volk R, Edwards A, Coulter A, et al. Developing a quality criteria framework for patient decision aids: online international Delphi consensus process. BMJ 2006;333. https://doi.org/10.1136/bmj.38926.629329.AE.
- Moons KG, Royston P, Vergouwe Y, Grobbee DE, Altman DG. Prognosis and prognostic research: what, why, and how?. BMJ 2009;338. https://doi.org/10.1136/bmj.b375.
- Royston P, Moons KG, Altman DG, Vergouwe Y. Prognosis and prognostic research: developing a prognostic model. BMJ 2009;338. https://doi.org/10.1136/bmj.b604.
- Altman DG, Vergouwe Y, Royston P, Moons KG. Prognosis and prognostic research: validating a prognostic model. BMJ 2009;338. https://doi.org/10.1136/bmj.b605.
- Stock SJ, Wotherspoon LM, Boyd KA, Morris RK, Dorling J, Jackson L, et al. Quantitative fibronectin to help decision-making in women with symptoms of preterm labour (QUIDS) part 1: individual participant data meta-analysis and health economic analysis. BMJ Open 2018;8. https://doi.org/10.1136/bmjopen-2017-020796.
- Collins GS, Reitsma JB, Altman DG, Moons KG. Transparent Reporting of a multivariable prediction model for Individual Prognosis Or Diagnosis (TRIPOD). Ann Intern Med 2015;162:735-6. https://doi.org/10.7326/L15-5093-2.
- Vergouwe Y, Steyerberg EW, Eijkemans MJ, Habbema JD. Substantial effective sample sizes were required for external validation studies of predictive logistic regression models. J Clin Epidemiol 2005;58:475-83. https://doi.org/10.1016/j.jclinepi.2004.06.017.
- Vittinghoff E, McCulloch CE. Relaxing the rule of ten events per variable in logistic and Cox regression. Am J Epidemiol 2007;165:710-18. https://doi.org/10.1093/aje/kwk052.
- Chang SM, Matchar DB, Smetana GW, Umscheid CA. Methods Guide for Medical Test Reviews. Rockville, MD: Agency for Healthcare Research and Quality (US); 2012.
- Whiting PF, Rutjes AW, Westwood ME, Mallett S, Deeks JJ, Reitsma JB, et al. QUADAS-2: a revised tool for the quality assessment of diagnostic accuracy studies. Ann Intern Med 2011;155:529-36. https://doi.org/10.7326/0003-4819-155-8-201110180-00009.
- Sterne JA, White IR, Carlin JB, Spratt M, Royston P, Kenward MG, et al. Multiple imputation for missing data in epidemiological and clinical research: potential and pitfalls. BMJ 2009;338. https://doi.org/10.1136/bmj.b2393.
- cran.r-project.org . Package ‘mice’ 2020. https://cran.r-project.org/web/packages/mice/mice.pdf (accessed 15 April 2020).
- White IR, Royston P, Wood AM. Multiple imputation using chained equations: issues and guidance for practice. Stat Med 2011;30:377-99. https://doi.org/10.1002/sim.4067.
- Debray TP, Moons KG, Ahmed I, Koffijberg H, Riley RD. A framework for developing, implementing, and evaluating clinical prediction models in an individual participant data meta-analysis. Stat Med 2013;32:3158-80. https://doi.org/10.1002/sim.5732.
- cran.r-project.org . Package ‘mfp’ 2015. https://cran.r-project.org/web/packages/mfp/mfp.pdf (accessed 15 April 2020).
- Haas DM, Imperiale TF, Kirkpatrick PR, Klein RW, Zollinger TW, Golichowski AM. Tocolytic therapy: a meta-analysis and decision analysis. Obstet Gynecol 2009;113:585-94. https://doi.org/10.1097/AOG.0b013e318199924a.
- Riley RD vdWD, Croft P, Moons KGM. Prognosis Research in Healthcare: Concepts, Methods and Impact. Oxford: Oxford University Press; 2019.
- Steyerberg EW. Clinical Prediction Models: A Practical Approach to Development, Validation, and Updating. New York, NY: Springer; 2009.
- mdbrown.github.io . Risk Model Decision Analysis 2018. http://mdbrown.github.io/rmda/ (accessed 15 April 2020).
- Peaceman AM, Andrews WW, Thorp JM, Cliver SP, Lukes A, Iams JD, et al. Fetal fibronectin as a predictor of preterm birth in patients with symptoms: a multicenter trial. Am J Obstet Gynecol 1997;177:13-8. https://doi.org/10.1016/S0002-9378(97)70431-9.
- Lu GC, Goldenberg RL, Cliver SP, Kreaden US, Andrews WW. Vaginal fetal fibronectin levels and spontaneous preterm birth in symptomatic women. Obstet Gynecol 2001;97:225-8. https://doi.org/10.1097/00006250-200102000-00012.
- Gomez R, Romero R, Medina L, Nien JK, Chaiworapongsa T, Carstens M, et al. Cervicovaginal fibronectin improves the prediction of preterm delivery based on sonographic cervical length in patients with preterm uterine contractions and intact membranes. Am J Obstet Gynecol 2005;192:350-9. https://doi.org/10.1016/j.ajog.2004.09.034.
- Abbott DS, Radford SK, Seed PT, Tribe RM, Shennan AH. Evaluation of a quantitative fetal fibronectin test for spontaneous preterm birth in symptomatic women. Am J Obstet Gynecol 2013;208:122-6. https://doi.org/10.1016/j.ajog.2012.10.890.
- Bruijn M, Vis JY, Wilms FF, Oudijk MA, Kwee A, Porath MM, et al. Quantitative fetal fibronectin testing in combination with cervical length measurement in the prediction of spontaneous preterm delivery in symptomatic women. BJOG 2016;123:1965-71. https://doi.org/10.1111/1471-0528.13752.
- Bruijn MM, Kamphuis EI, Hoesli IM, Martinez de Tejada B, Loccufier AR, Kühnert M, et al. The predictive value of quantitative fibronectin testing in combination with cervical length measurement in symptomatic women. Am J Obstet Gynecol 2016;215:793.e1-8. https://doi.org/10.1016/j.ajog.2016.08.012.
- Levine LD, Downes KL, Romero JA, Pappas H, Elovitz MA. Quantitative fetal fibronectin and cervical length in symptomatic women: results from a prospective blinded cohort study. J Matern Fetal Neonatal Med 2019;32:3792-800.
- Steyerberg EW, Harrell FE. Prediction models need appropriate internal, internal-external, and external validation. J Clin Epidemiol 2016;69:245-7. https://doi.org/10.1016/j.jclinepi.2015.04.005.
- Husereau D, Drummond M, Petrou S, Carswell C, Moher D, Greenberg D, et al. Consolidated Health Economic Evaluation Reporting Standards (CHEERS) statement. Int J Technol Assess Health Care 2013;29:117-22. https://doi.org/10.1017/S0266462313000160.
- Department of Health and Social Care (DHSC) . NHS Reference Costs 2017 18 2008. https://improvement.nhs.uk/resources/reference-costs/#rc1718 (accessed 19 September 2019).
- National Institute for Health and Care Excellence (NICE) . Guide to the Methods of Technology Appraisal 2013. Process and Methods (PMG9) 2013. www.nice.org.uk/process/pmg9/resources/guide-to-the-methods-of-technology-appraisal-2013-pdf-2007975843781 (accessed 19 September 2019).
- Carroll AE, Downs SM. Improving decision analyses: parent preferences (utility values) for pediatric health outcomes. J Pediatr 2009;155:21-5. https://doi.org/10.1016/j.jpeds.2009.01.040.
- Department of Health and Social Care (DHSC) . NHS Reference Costs 2015–16 2016. https://assets.publishing.service.gov.uk/government/uploads/system/uploads/attachment_data/file/577083/Reference_Costs_2015-16.pdf (accessed 19 September 2019).
- Curtis L, Burns A. Unit Costs of Health and Social Care 2017. Canterbury: PSSRU, University of Kent; 2017.
- Joint Formulary Committee . British National Formulary n.d. www.medicinescomplete.com (accessed 19 September 2019).
- Briggs A. Economic evaluation and clinical trials: size matters. BMJ 2000;321:1362-3. https://doi.org/10.1136/bmj.321.7273.1362.
- O’Hagan A, McCabe C, Akehurst R, Brennan A, Briggs A, Claxton K, et al. Incorporation of uncertainty in health economic modelling studies. PharmacoEconomics 2005;23:529-36. https://doi.org/10.2165/00019053-200523060-00001.
- Strong M, Oakley JE, Brennan A. Estimating multiparameter partial expected value of perfect information from a probabilistic sensitivity analysis sample: a nonparametric regression approach. Med Decis Making 2014;34:311-26. https://doi.org/10.1177/0272989X13505910.
- Action Medical Research for Children . Premature Birth: Predicting Who’s At Risk 2016. https://action.org.uk/research/premature-birth-predicting-whos-risk (accessed 19 September 2019).
- Mozurkewich EL, Naglie G, Krahn MD, Hayashi RH. Predicting preterm birth: a cost-effectiveness analysis. Am J Obstet Gynecol 2000;182:1589-98. https://doi.org/10.1067/mob.2000.106855.
- Boyd KA, Briggs AH, Fenwick E, Norrie J, Stock S. Power and sample size for cost-effectiveness analysis: fFN neonatal screening. Contemp Clin Trials 2011;32:893-901. https://doi.org/10.1016/j.cct.2011.07.007.
- National Institute for Health and Care Excellence (NICE) . Biomarker Tests to Help Diagnose Preterm Labour in Woman With Intact Membranes. Diagnostics Guidance [DG33] 2018. www.nice.org.uk/guidance/dg33 (accessed 19 September 2019).
- Stock SJ, Wotherspoon LM, Boyd KA, Morris RK, Dorling J, Jackson L, et al. Study protocol: quantitative fibronectin to help decision-making in women with symptoms of preterm labour (QUIDS) part 2, UK Prospective Cohort Study. BMJ Open 2018;8. https://doi.org/10.1136/bmjopen-2017-020795.
- Collins GS, Ogundimu EO, Altman DG. Sample size considerations for the external validation of a multivariable prognostic model: a resampling study. Stat Med 2016;35:214-26. https://doi.org/10.1002/sim.6787.
- Glick HA, Doshi JA, Sonnad SS, Polsky D. Economic Evaluation in Clinical Trials. Oxford: Oxford University Press; 2014.
- Tonmukayakul U, Le LK, Mudiyanselage SB, Engel L, Bucholc J, Mulhern B, et al. A systematic review of utility values in children with cerebral palsy. Qual Life Res 2019;28:1-12. https://doi.org/10.1007/s11136-018-1955-8.
- National Institute for Health and Care Excellence (NICE) . Discounting of Health Benefits in Special Circumstances n.d. www.nice.org.uk/guidance/ta235/resources/osteosarcoma-mifamurtide-discounting-of-health-benefits-in-special-circumstances2 (accessed 19 September 2019).
- van Baaren GJ, Vis JY, Grobman WA, Bossuyt PM, Opmeer BC, Mol BW. Cost-effectiveness analysis of cervical length measurement and fibronectin testing in women with threatened preterm labor. Am J Obstet Gynecol 2013;209:436-8. https://doi.org/10.1016/j.ajog.2013.06.029.
- Watson HA, Carter J, Seed PT, Tribe RM, Shennan AH. The QUiPP App: a safe alternative to a treat-all strategy for threatened preterm labor. Ultrasound Obstet Gynecol 2017;50:342-6. https://doi.org/10.1002/uog.17499.
- Shennan A, Jones G, Hawken J, Crawshaw S, Judah J, Senior V, et al. Fetal fibronectin test predicts delivery before 30 weeks of gestation in high risk women, but increases anxiety. BJOG 2005;112:293-8. https://doi.org/10.1111/j.1471-0528.2004.00420.x.
- Peterson WE, Sprague AE, Reszel J, Walker M, Fell DB, Perkins SL, et al. Women’s perspectives of the fetal fibronectin testing process: a qualitative descriptive study. BMC Pregnancy Childbirth 2014;14. https://doi.org/10.1186/1471-2393-14-190.
- Rose MS, Pana G, Premji S. Prenatal maternal anxiety as a risk factor for preterm birth and the effects of heterogeneity on this relationship: a systematic review and meta-analysis. Biomed Res Int 2016;2016. https://doi.org/10.1155/2016/8312158.
- Spielberger CD. State–Trait Anxiety Inventory (Form Y). Palo Alto, CA: Mind Garden; 1983.
- Knight RG, Waal-Manning HJ, Spears GF. Some norms and reliability data for the State–Trait Anxiety Inventory and the Zung Self-Rating Depression scale. Br J Clin Psychol 1983;22:245-9. https://doi.org/10.1111/j.2044-8260.1983.tb00610.x.
- Gunning M, Denison F, Stockley C, Ho S, Sandhu H, Reynolds R. Assessing maternal anxiety in pregnancy with the State–Trait Anxiety Inventory (STAI): issues of validity, location and participation. J Reprod Infant Psychol 2010;28:266-73. https://doi.org/10.1080/02646830903487300.
- van der Steen SL, Riedijk SR, Verhagen-Visser J, Govaerts LC, Srebniak MI, Van Opstal D, et al. The psychological impact of prenatal diagnosis and disclosure of susceptibility loci: first impressions of parents’ experiences. J Genet Couns 2016;25:1227-34. https://doi.org/10.1007/s10897-016-9960-y.
- Bhise V, Meyer AND, Menon S, Singhal G, Street RL, Giardina TD, et al. Patient perspectives on how physicians communicate diagnostic uncertainty: an experimental vignette study. Int J Qual Health Care 2018;30:2-8. https://doi.org/10.1093/intqhc/mzx170.
- Kleinrouweler CE, Cheong-See FM, Collins GS, Kwee A, Thangaratinam S, Khan KS, et al. Prognostic models in obstetrics: available, but far from applicable. Am J Obstet Gynecol 2016;214:79-90. https://doi.org/10.1016/j.ajog.2015.06.013.
- Collins GS, Reitsma JB, Altman DG, Moons KG. Transparent reporting of a multivariable prediction model for individual prognosis or diagnosis (TRIPOD): the TRIPOD statement. BMJ 2015;350.
- Manuck TA, Rice MM, Bailit JL, Grobman WA, Reddy UM, Wapner RJ, et al. Preterm neonatal morbidity and mortality by gestational age: a contemporary cohort. Am J Obstet Gynecol 2016;215:103.e1-103.e14. https://doi.org/10.1016/j.ajog.2016.01.004.
- Cooke RW. Health, lifestyle, and quality of life for young adults born very preterm. Arch Dis Child 2004;89:201-6. https://doi.org/10.1136/adc.2003.030197.
- Parisaei M, Currie J, O’Gorman N, Morris S, David AL. Implementation of foetal fibronectin testing: admissions, maternal interventions and costs at 1 year. J Obstet Gynaecol 2016;36:888-92. https://doi.org/10.3109/01443615.2016.1168374.
- Dani C, Ravasio R, Fioravanti L, Circelli M. Analysis of the cost-effectiveness of surfactant treatment (Curosurf®) in respiratory distress syndrome therapy in preterm infants: early treatment compared to late treatment. Ital J Pediatr 2014;40. https://doi.org/10.1186/1824-7288-40-40.
- Public Health Scotland . Speciality Costs: Maternity 2018 n.d. www.isdscotland.org/Health-Topics/Finance/Costs/Detailed-Tables/Speciality-Costs/ (accessed 19 September 2019).
Appendix 1 The TRIPOD statement
Section/topic | Item | Development or validation?a | Checklist item | Page or chapter |
---|---|---|---|---|
Title and abstract | ||||
Title | 1 | D;V | Identify the study as developing and/or validating a multivariable prediction model, the target population, and the outcome to be predicted | i |
Abstract | 2 | D;V | Provide a summary of objectives, study design, setting, participants, sample size, predictors, outcome, statistical analysis, results, and conclusions | vii |
Introduction | ||||
Background and objectives | 3a | D;V | Explain the medical context (including whether diagnostic or prognostic) and rationale for developing or validating the multivariable prediction model, including references to existing models | Chapter 1 |
3b | D;V | Specify the objectives, including whether the study describes the development or validation of the model or both | Chapter 2 | |
Methods | ||||
Source of data | 4a | D;V | Describe the study design or source of data (e.g., randomised trial, cohort, or registry data), separately for the development and validation data sets, if applicable | Chapters 4 and 6 |
4b | D;V | Specify the key study dates, including start of accrual; end of accrual; and, if applicable, end of follow-up | Chapters 4 and 6 | |
Participants | 5a | D;V | Specify key elements of the study setting (e.g., primary care, secondary care, general population) including number and location of centres | Chapters 4 and 6 |
5b | D;V | Describe eligibility criteria for participants | Chapters 4 and 6 | |
5c | D;V | Give details of treatments received, if relevant | Chapters 4 and 6 | |
Outcome | 6a | D;V | Clearly define the outcome that is predicted by the prediction model, including how and when assessed | Chapter 4 |
6b | D;V | Report any actions to blind assessment of the outcome to be predicted | Not applicable | |
Pedictors | 7a | D;V | Clearly define all predictors used in developing or validating the multivariable prediction model, including how and when they were measured | Chapters 4 and 6 |
7b | D;V | Report any actions to blind assessment of predictors for the outcome and other predictors | Chapter 6 | |
Sample size | 8 | D;V | Explain how the study size was arrived at | Chapters 4 and 6 |
Missing data | 9 | D;V | Describe how missing data were handled (e.g., complete-case analysis, single imputation, multiple imputation) with details of any imputation method | Chapters 4 and 6 |
Statistical analysis methods | 10a | D | Describe how predictors were handled in the analyses | Chapter 4 |
10b | D | Specify type of model, all model-building procedures (including any predictor selection), and method for internal validation | Chapter 4 | |
10c | V | For validation, describe how the predictions were calculated | Chapter 6 | |
10d | D;V | Specify all measures used to assess model performance and, if relevant, to compare multiple models | Chapter 4 | |
10e | V | Describe any model updating (e.g., recalibration) arising from the validation, if done | Chapter 6 | |
Risk groups | 11 | D;V | Provide details on how risk groups were created, if done | Not done |
Development vs. validation | 12 | V | For validation, identify any differences from the development data in setting, eligibility criteria, outcome, and predictors | Chapter 6 |
Results | ||||
Participants | 13a | D;V | Describe the flow of participants through the study, including the number of participants with and without the outcome and, if applicable, a summary of the follow-up time. A diagram may be helpful | Chapters 4 and 6 |
13b | D;V | Describe the characteristics of the participants (basic demographics, clinical features, available predictors), including the number of participants with missing data for predictors and outcome | Chapters 4 and 6 | |
13c | V | For validation, show a comparison with the development data of the distribution of important variables (demographics, predictors and outcome) | Chapter 6 | |
Model development | 14a | D | Specify the number of participants and outcome events in each analysis | Chapter 4 |
14b | D | If done, report the unadjusted association between each candidate predictor and outcome | Not done | |
Model specification | 15a | D | Present the full prediction model to allow predictions for individuals (i.e., all regression coefficients, and model intercept or baseline survival at a given time point) | Chapter 4 |
15b | D | Explain how to the use the prediction model | Chapter 10 | |
Model performance | 16 | D;V | Report performance measures (with CIs) for the prediction model | Chapters 4 and 6 |
Model-updating | 17 | V | If done, report the results from any model updating (i.e., model specification, model performance) | Chapter 6 |
Discussion | ||||
Limitations | 18 | D;V | Discuss any limitations of the study (such as nonrepresentative sample, few events per predictor, missing data) | Chapter 12 |
Interpretation | 19a | V | For validation, discuss the results with reference to performance in the development data, and any other validation data | Chapter 12 |
19b | D;V | Give an overall interpretation of the results, considering objectives, limitations, results from similar studies, and other relevant evidence | Chapter 12 | |
Implications | 20 | D;V | Discuss the potential clinical use of the model and implications for future research | Chapter 10 |
Other information | ||||
Supplementary information | 21 | D;V | Provide information about the availability of supplementary resources, such as study protocol, Web calculator, and data sets | Chapter 10 |
Funding | 22 | D;V | Give the source of funding and the role of the funders for the present stud | viii |
Appendix 2 Assessment of risk of bias in studies included in the individual participant data meta-analysis
Test strategy | Study (authors) | ||||
---|---|---|---|---|---|
APOSTEL-1 (Bruijn et al.60) | EUFIS (Bruijn et al.61) | EQUIPP (Abbott et al.59) | QFCAPS (Khalil et al.) | UCLH/Whit (David et al.) | |
Participant selection | |||||
Was a consecutive or random sample of patients enrolled? | ✓ | ✓ | ✓ | ? | ? |
Was a case–control design avoided? | ✓ | ✓ | ✓ | ✓ | ✓ |
Did the study avoid inappropriate exclusions? | ✓ | ✓ | ✓ | ✓ | ✓ |
Could the selection of patients have introduced bias? | Low risk | Low risk | Low risk | Unclear | Low risk |
Index test | |||||
Were the index test results interpreted without knowledge of the reference standard? | ✓ | ✓ | ✓ | ✓ | ✓ |
If a threshold was used, was it pre-specified? | ✓ | ✓ | ✓ | ✓ | ✓ |
Could the conduct or interpretation of the index test have introduced bias? | Low risk | Low risk | Low risk | Low risk | Low risk |
Reference standard | |||||
Is the reference standard likely to correctly classify the target condition? | ✓ | ✓ | ✓ | ✓ | ✓ |
Were the reference standard results interpreted without knowledge of the results of the index test? | ✓ | ✓ | ✓ | ✓ | ✓ |
Could the reference standard, its conduct, or its interpretation have introduced bias? | Low risk | Low risk | Low risk | Low risk | Low risk |
Flow and timing | |||||
Was there an appropriate interval between index test(s) and reference standard? | ✓ | ✓ | ✓ | ✓ | ✓ |
Did all patients receive a reference standard? | ✓ | ✓ | ✓ | ✓ | ✓ |
Did all patients receive the same reference standard? | ✓ | ✓ | ✓ | ✓ | ✓ |
Were all patients included in the analysis? | ✓ | ✓ | ✓ | ✓ | ✓ |
Could the patient flow have introduced bias? | Low risk | Low risk | Low risk | Low risk | Low risk |
Overall | |||||
Risk-of-bias rating | Low risk | Low risk | Low risk | Low risk | Low risk |
Appendix 3 Results of meta-analysis for heterogeneity of predictor effects
Test strategy | Heterogeneity measures | |
---|---|---|
T (95% CI) | I2 (95% CI) | |
Age (years) | 0.0 (0.0 to 0.03) | 0.0 (0.0 to 89) |
BMI (kg/m2) | 0.0 (0.0 to 0.0) | 0.0 (0.0 to 26) |
Smoking | 0.12 (0.0 to 2.4) | 9.6 (0.0 to 68) |
Ethnicity | ||
White | – | – |
South Asian | 0.02 (0.0 to 6.4) | 0.57 (0.0 to 62) |
East Asian | 0.0 (0.0 to 0.0) | 0.0 (0.0 to 0.0) |
African/Caribbean/Middle-Eastern | 0.0 (0.0 to 2.1) | 0.0 (0.0 to 68) |
Other | 0.0 (0.0 to 0.0) | 0.0 (0.0 to 0.0) |
Nulliparity | 0.16 (0.0 to 1.2) | 25 (0.0 to 75) |
Multiple pregnancy | 0.0 (0.0 to 7.8) | 0.0 (0.0 to 94) |
Gestational age (weeks) | 0.0 (0.0 to 0.12) | 11 (0.0 to 91) |
Previous spontaneous preterm birth at < 34 weeks’ gestation | 0.58 (0.0 to 3.7) | 38 (0.0 to 80) |
Cervical length (mm) | 0.0 (0.0 to 0.09) | 75 (0.0 to 98) |
Quantitative fFN | 0.0 (0.0 to 0.0) | 26 (0.0 to 98) |
Appendix 4 Sensitivity analyses with tocolysis
Variable | Model including all variables | Model after variable selection | ||
---|---|---|---|---|
Beta | OR (95% CI) | Beta | OR (95% CI) | |
Intercept | ||||
Study | ||||
1 (APOSTEL-1)60 | 11.8 | – | –7.7 | – |
2 (EUFIS)61 | –12.5 | – | –8.4 | – |
3 (EQUIPP)59 | –11.7 | – | –7.6 | – |
4 (QFCAPS) | –11.8 | – | –7.8 | – |
5 (UCLH/Whit) | –12.3 | – | –8.2 | – |
Quantitative fFN: [(quantitative fFN + 1)/100]0.5 | 1.8 | 6.1 (4.5 to 8.3) | 1.8 | 6.4 (4.7 to 8.6) |
Age (years) | 0.03 | 1.0 (0.99 to 1.1) | – | – |
BMI (kg/m2) | 0.02 | 1.0 (0.96 to 1.1) | – | – |
Smoking | –0.62 | 0.54 (0.24 to 1.2) | –0.75 | 0.47 (0.22 to 1.0) |
Ethnicity | ||||
White | Reference | Reference | ||
South Asian | 1.2 | 3.2 (0.96 to 11) | 1.1 | 2.9 (0.88 to 9.4) |
East Asian | –1.4 | 0.26 (0.03 to 2.2) | –1.2 | 0.29 (0.03 to 2.4) |
African/Caribbean/Middle-Eastern | –0.35 | 0.70 (0.36 to 1.4) | –0.39 | 0.68 (0.35 to 1.3) |
Other | –0.33 | 0.72 (0.18 to 2.9) | –0.46 | 0.63 (0.16 to 2.6) |
Nulliparity | 0.42 | 1.5 (0.93 to 2.5) | – | – |
Multiple pregnancy | 0.64 | 1.9 (1.1 to 3.3) | 0.71 | 2.0 (1.2 to 3.5) |
Previous spontaneous preterm birth at < 34 weeks’ gestation | 0.50 | 1.6 (0.82 to 3.3) | – | – |
Gestational age (weeks) at assessment | 0.05 | 1.0 (0.97 to 1.1) | – | – |
Tocolysis | 2.1 | 8.1 (3.7 to 18) | 2.0 | 7.7 (3.5 to 17) |
Performance | Model including all variables | |||
Nagelkerke R2 | 0.44 | 0.44 | ||
AUC (95% CI) | 0.92 (0.90 to 0.94) | 0.92 (0.89 to 0.94) |
Variable | Model including all variables | |
---|---|---|
Beta | OR (95% CI) | |
Intercept | ||
Study | ||
1 (APOSTEL-1)60 | –7.0 | – |
2 (EUFIS)61 | –7.6 | – |
3 (EQUIPP)59 | –6.9 | – |
4 (QFCAPS) | –7.1 | – |
5 (UCLH/Whit) | –7.4 | – |
Quantitative fFN: [(quantitative fFN + 1)/100]0.5 | 1.7 | 5.4 (4.0 to 7.3) |
Smoking | –0.68 | 0.51 (0.24 to 1.09) |
Ethnicity | ||
White | Reference | |
South Asian | 0.96 | 2.6 (0.81 to 8.5) |
East Asian | –1.1 | 0.32 (0.04 to 2.6) |
African/Caribbean/Middle-Eastern | –0.36 | 0.70 (0.37 to 1.3) |
Other | –0.42 | 0.66 (0.17 to 2.6) |
Multiple pregnancy | 0.65 | 1.9 (1.1 to 3.3) |
Tocolysis | 1.9 | 6.4 (3.0 to 14) |
Performance measures | Model including all variables | |
Nagelkerke R2 | 0.43 | |
AUC (95% CI) | 0.92 (0.90 to 0.94) |
Appendix 5 Economic literature review for development of economic model
This section provides full details of the literature review undertaken to inform the economic model design and identify parameters:
-
population – studies including pregnant woman, with singleton or twin gestation, who have signs or symptoms of preterm labour before 37 weeks’ gestation
-
intervention – the use of qualitative or quantitative fFN before 37 weeks’ gestation in the treatment of woman with threatened preterm labour
-
comparator – usual care, without fFN testing, for the treatment of woman with threatened preterm labour
-
outcomes/inclusion criteria – any economic studies (cost-effectiveness, cost utility, cost–benefit and cost-consequence) that including the use of fFN testing in women with threatened preterm labour before 37 weeks’ gestation.
Search strategy
We searched MEDLINE, EMBASE, Cochrane Library (including the Database of Abstracts of Reviews of Effects, HTA and NHS Economic Evaluation Database) and Paediatric Economic Database Evaluation from inception to January 2017 on 17 January 2017. We included studies in the English language only. Tables 38–41 detail the search terms and used for each database.
# | Search | Results |
---|---|---|
1 | (f?etal adj2 fibronectin$).ti,ab,ot,hw. | 767 |
2 | ((oncofetal or oncofoetal) adj2 fibronectin$).ti,ab,ot,hw. | 129 |
3 | (ffn or onfn or fdc-6).ti,ab,ot,hw. | 410 |
4 | (tli system$ or (tli adj iq) or tliiq or quikcheck).ti,ab,ot,hw. | 15 |
5 | or/1-4 | 977 |
6 | fibronectins/ | 27,969 |
7 | (86088-83-7 or fibronectin$).ti,ab,ot,rn. | 36,436 |
8 | or/6-7 | 36,571 |
9 | exp Obstetric Labor, Premature/ | 35,414 |
10 | ((Pre term or preterm or premature or early or immature) adj5 (labo?r or birth$ or childbirth$ or deliver$ or partu$ or ruptur$)).ti,ab,ot,hw. | 68,679 |
11 | (PROM or PROM or PTB).ti,ab,ot. | 8014 |
12 | ((Short$ or reduced or multiple) adj4 gestation$).ti,ab,ot. | 5144 |
13 | or/9-12 | 77,295 |
14 | 5 or (8 and 13) | 1235 |
15 | economics/ | 143,980 |
16 | exp “costs and cost analysis”/ | 251,868 |
17 | economics, dental/ | 20,535 |
18 | exp “economics, hospital”/ | 603,737 |
19 | economics, medical/ | 20,211 |
20 | economics, nursing/ | 19,397 |
21 | economics, pharmaceutical/ | 7455 |
22 | (economic$ or cost or costs or costly or costing or price or prices or pricing or pharmacoeconomic$).ti,ab. | 647,321 |
23 | (expenditure$ not energy).ti,ab. | 24,822 |
24 | (value adj1 money).ti,ab. | 22 |
25 | budget$.ti,ab. | 23,213 |
26 | or/15-25 | 1,084,651 |
27 | ((energy or oxygen) adj cost).ti,ab. | 2443 |
28 | (metabolic adj cost).ti,ab. | 916 |
29 | ((energy or oxygen) adj expenditure).ti,ab. | 21,263 |
30 | or/27-29 | 24,006 |
31 | 26 not 30 | 1,080,245 |
32 | letter.pt. | 677,698 |
33 | editorial.pt. | 438,912 |
34 | historical article.pt. | 0 |
35 | or/32-34 | 1,116,610 |
36 | 31 not 35 | 1,009,585 |
37 | animals/not (animals/and humans/) | 433,885 |
38 | 36 not 37 | 995,070 |
39 | 14 and 38 | 127 |
40 | remove duplicates from 39 | 54 |
# | Search | Results |
---|---|---|
1 | (f?etal adj2 fibronectin$).mp. | 559 |
2 | ((oncofetal or oncofoetal) adj2 fibronectin$).mp. | 147 |
3 | (ffn or onfn or fdc-6).mp. | 324 |
4 | (tli system$ or (tli adj iq) or tliiq or quikcheck).mp. | 7 |
5 | or/1-4 | 765 |
6 | Fibronectin/ | 25,358 |
7 | (86088-83-7 or fibronectin$).mp. | 42,605 |
8 | or/6-7 | 42,605 |
9 | exp “premature labor”/ | 23,856 |
10 | ((Pre term or preterm or premature or early or immature) adj5 (labo?r or birth$ or childbirth$ or deliver$ or partu$ or ruptur$)).mp. | 61,505 |
11 | (PROM or PROM or PTB).mp. | 5858 |
12 | ((Short$ or reduced or multiple) adj4 gestation$).mp. | 4395 |
13 | or/9-12 | 68,684 |
14 | 5 or (8 and 13) | 874 |
15 | health-economics/ | 0 |
16 | exp economic-evaluation/ | 74,552 |
17 | exp health-care-cost/ | 58,869 |
18 | exp pharmacoeconomics/ | 2816 |
19 | or/15-18 | 121,830 |
20 | (econom$ or cost or costs or costly or costing or price or prices or pricing or pharmacoeconomic$).ti,ab. | 566,466 |
21 | (expenditure$ not energy).ti,ab. | 22,252 |
22 | (value adj2 money).ti,ab. | 1238 |
23 | budget$.ti,ab. | 21,156 |
24 | or/20-23 | 589,052 |
25 | 19 or 24 | 623,381 |
26 | letter.pt. | 962,103 |
27 | editorial.pt. | 424,974 |
28 | note.pt. | 0 |
29 | or/26-28 | 1,386,989 |
30 | 25 not 29 | 603,462 |
31 | (metabolic adj cost).ti,ab. | 1053 |
32 | ((energy or oxygen) adj cost).ti,ab. | 3212 |
33 | ((energy or oxygen) adj expenditure).ti,ab. | 20,929 |
34 | or/31-33 | 24,351 |
35 | 30 not 34 | 598,147 |
36 | 14 and 35 | 53 |
37 | animal/or animal experiment/ | 6,734,998 |
38 | (rat or rats or mouse or mice or murine or rodent or rodents or hamster or hamsters or pig or pigs or porcine or rabbit or rabbits or animal or animals or dogs or dog or cats or cow or bovine or sheep or ovine or monkey or monkeys).mp. | 7,020,706 |
39 | or/37-38 | 7,020,706 |
40 | exp human/or human experiment/ | 18,009,599 |
41 | 39 not (39 and 40) | 4,870,347 |
42 | 36 not 41 | 53 |
43 | remove duplicates from 42 | 53 |
# | Search | Results |
---|---|---|
1 | (fetal or foetal) near/2 (fibronectin*) | 116 |
2 | (oncofetal or oncofoetal) near/2 (fibronectin*) | 1 |
3 | (ffn or onfn or fdc-6) | 41 |
4 | (tli system* or tli iq or tliiq or quikcheck) | 18 |
5 | (#1 OR #2 OR #3 OR #4) | 137 |
6 | MeSH descriptor Fibronectins, this term only | 0 |
7 | (86088-83-7 or fibronectin*) | 446 |
8 | (#6 OR #7) | 446 |
9 | MeSH descriptor Obstetric Labor, Premature explode all trees | 12 |
10 | ((Pre term or preterm or premature or early or immature) near/5 (labor or labour or birth* or childbirth* or deliver* or partu* or ruptur*)) | 6672 |
11 | (PROM or PROM or PTB) | 566 |
12 | (Short* or reduced or multiple) near/4 (gestation*) | 499 |
13 | (#9 OR #10 OR #11 OR #12) | 7170 |
14 | (#5 OR (#8 AND #13)) | 158 |
15 | Remove duplicates from 14 | 48 |
# | Search | Results |
---|---|---|
1 | Fibronectin | 2 |
2 | Fibronectins | 2 |
3 | ffn | 1 |
4 | onfn | 0 |
5 | fdc-6 | 0 |
6 | Total before deduplication | 5 |
7 | Total | 2 |
Results
Our search identified the following: MEDLINE, 54 records; EMBASE, 53 records; Cochrane Library, 48 records; Paediatric Economic Database Evaluation, 2 records. After de-duplication and exclusion criteria were applied, five papers were found to meet all inclusion criteria. The search strategy output in presented in a Preferred Reporting Items for Systematic Review and Meta-Analyses (PRISMA) diagram in Figure 18.
FIGURE 18.
The PRISMA flow diagram for literature review.
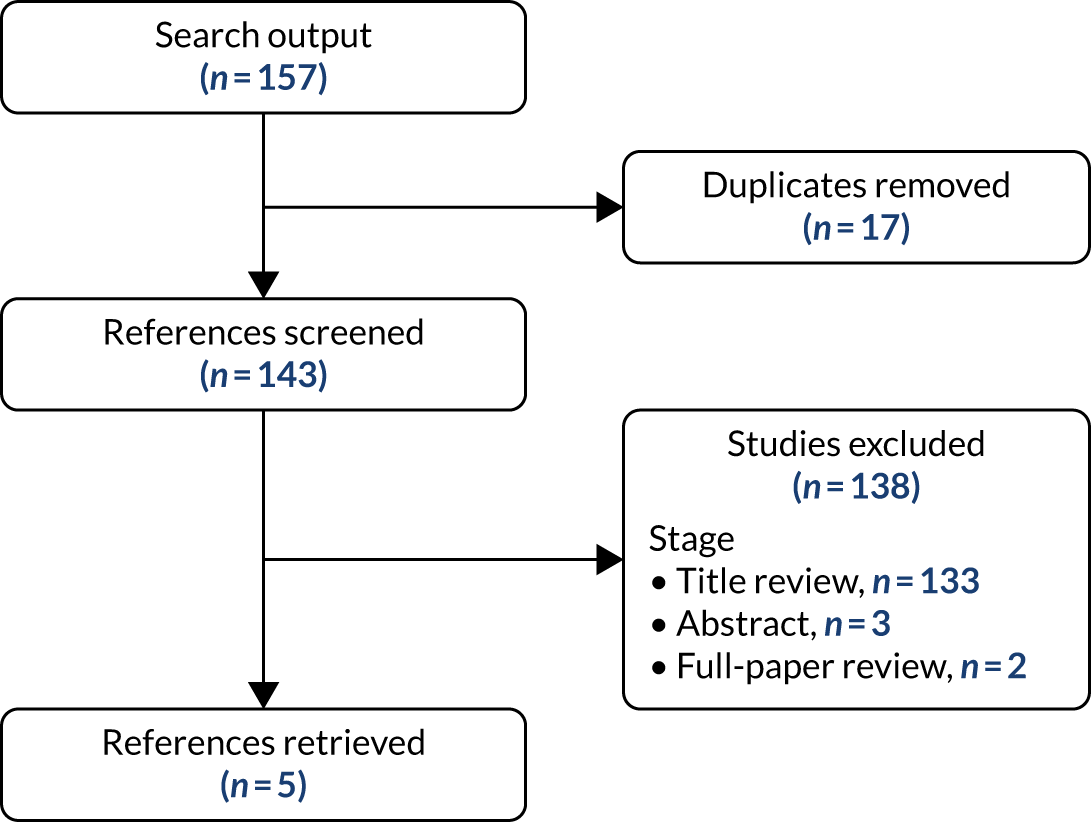
Appendix 6 Economic model parameters
Treatment effect | Value | SE | Probability distribution | Reference |
---|---|---|---|---|
Relative risk reduction of corticosteroids on mortality | 0.69 | 0.058 | Log-normal | 6 |
Relative risk reduction of corticosteroids on morbidity | 0.66 | 0.036 | Log-normal | 6 |
Neonatal outcomes | Value | SE | Probability distribution | Reference |
Probability of death | 0.01 | 0.001 | Beta | 95 |
Probability of major morbidity | 0.08 | 0.008 | Beta | 95 |
Probability of minor morbidity | 0.38 | 0.038 | Beta | 95 |
Probability of healthy | 0.53 | 0.053 | Beta | 95 |
Test/model performance at alternative admit thresholds | Sensitivity | Specificity | Probability distribution | Reference |
Qualitative fFN | 0.88 | 0.74 | NA | IPD meta-analysis |
Quantitative fFN | ||||
≥ 2% | 0.92 | 0.65 | NA | IPD meta-analysis |
≥ 5% | 0.83 | 0.81 | NA | IPD meta-analysis |
≥ 10% | 0.74 | 0.88 | NA | IPD meta-analysis |
≥ 20% | 0.61 | 0.92 | NA | IPD meta-analysis |
≥ 30% | 0.47 | 0.95 | NA | IPD meta-analysis |
≥ 40% | 0.37 | 0.97 | NA | IPD meta-analysis |
Cervical length | ||||
≥ 2% | 0.98 | 0.34 | NA | IPD meta-analysis |
≥ 5% | 0.85 | 0.54 | NA | IPD meta-analysis |
≥ 10% | 0.77 | 0.72 | NA | IPD meta-analysis |
≥ 20% | 0.57 | 0.91 | NA | IPD meta-analysis |
≥ 30% | 0.46 | 0.95 | NA | IPD meta-analysis |
≥ 40% | 0.34 | 0.97 | NA | IPD meta-analysis |
≥ 50% | 0.27 | 0.99 | NA | IPD meta-analysis |
≥ 60% | 0.20 | 1.00 | NA | IPD meta-analysis |
Prognostic model A | ||||
≥ 2% | 0.91 | 0.78 | NA | IPD meta-analysis |
≥ 5% | 0.75 | 0.88 | NA | IPD meta-analysis |
≥ 10% | 0.61 | 0.92 | NA | IPD meta-analysis |
≥ 15% | 0.48 | 0.95 | NA | IPD meta-analysis |
≥ 20% | 0.35 | 0.97 | NA | IPD meta-analysis |
≥ 25% | 0.14 | 0.99 | NA | IPD meta-analysis |
Prognostic model C | ||||
≥ 2% | 1.00 | 0.13 | NA | IPD meta-analysis |
≥ 5% | 0.99 | 0.29 | NA | IPD meta-analysis |
≥ 10% | 0.92 | 0.40 | NA | IPD meta-analysis |
≥ 20% | 0.86 | 0.48 | NA | IPD meta-analysis |
≥ 30% | 0.83 | 0.51 | NA | IPD meta-analysis |
≥ 40% | 0.78 | 0.52 | NA | IPD meta-analysis |
≥ 50% | 0.71 | 0.54 | NA | IPD meta-analysis |
≥ 60% | 0.62 | 0.56 | NA | IPD meta-analysis |
≥ 70% | 0.49 | 0.56 | NA | IPD meta-analysis |
≥ 80% | 0.41 | 0.57 | NA | IPD meta-analysis |
Health utilities | Value | SE | Probability distribution | Reference |
Healthy | 0.88 | 0.05 | Beta | 96 |
Utility of minor morbidity | 0.83 | 0.21 | Beta | 67 |
Utility of major morbidity | 0.76 | 0.23 | Beta | 67 |
Utility of death | 0 | NA | NA | Assumption |
Unit cost parameter | Cost (£) | SE (£) | Probability distribution | Reference |
Magnesium sulphate | 362.00 | 72.40 | Gamma | 14 |
Corticosteroids | 4.46 | 0.88 | Gamma | 12 |
Maternal admission | 1325.00 | 265.00 | Gamma | 97 |
Maternal transfer | 337.00 | 67.40 | Gamma | 83 |
Neonatal transfer | 965.00 | 193.00 | Gamma | 14 |
Minor morbidity | 4081.00 | 75.51 | Gamma | 65 |
Major morbidity | 10,038.00 | 5.38 | Gamma | 65 |
Qualitative fFN test | 65.00 | 13.00 | Gamma | 14 |
Quantitative fFN test | 65.00 | 13.00 | Gamma | 14 |
Cervical length measurement | 75.00 | 15.00 | Gamma | Expert opinion |
Appendix 7 Individual participant data economic analyses results
Part 1: probabilistic sensitivity analysis results
FIGURE 19.
Cost-effectiveness plane for quantitative fFN at ≥ 2% probability of spontaneous preterm birth vs. treat all.
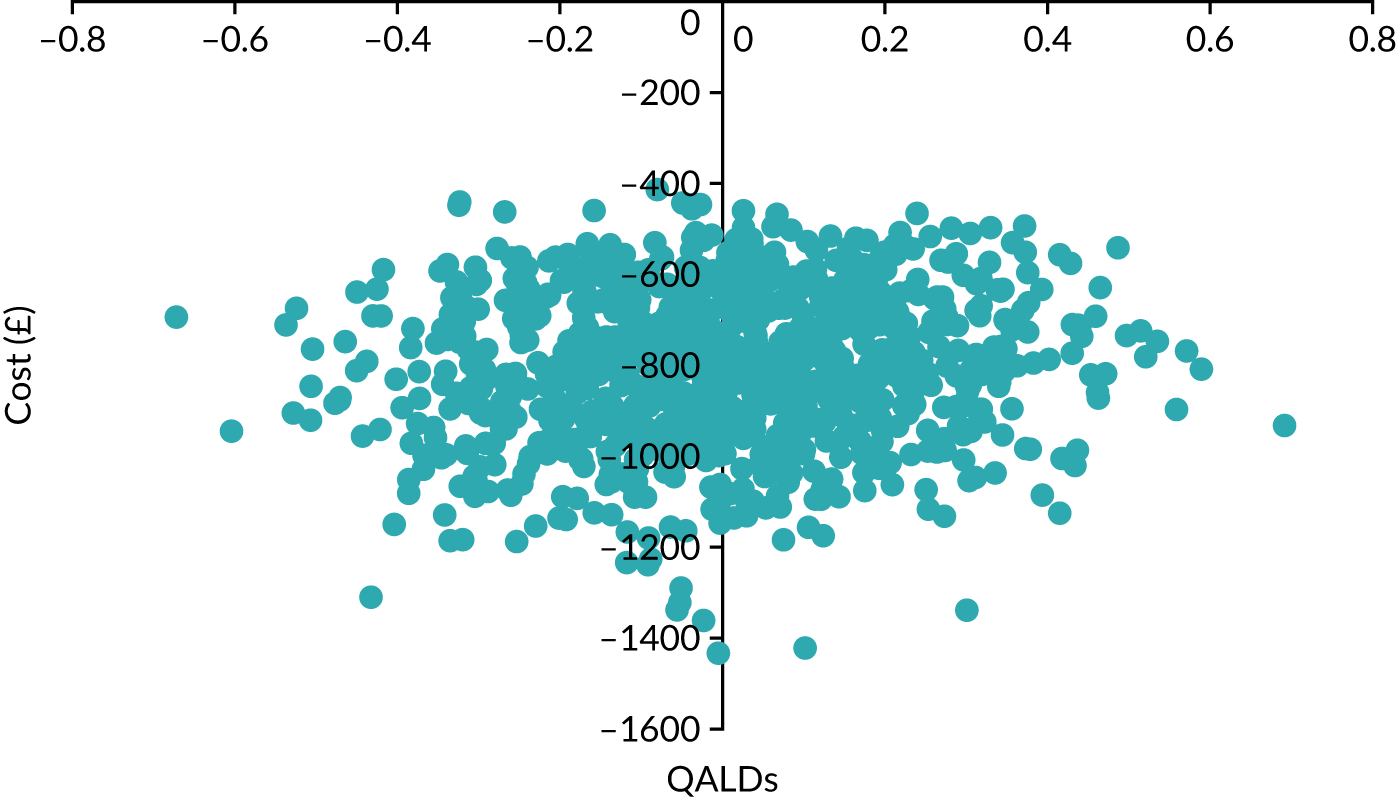
FIGURE 20.
Cost-effectiveness acceptability curve for quantitative fFN at ≥ 2% probability of spontaneous preterm birth vs. treat all.
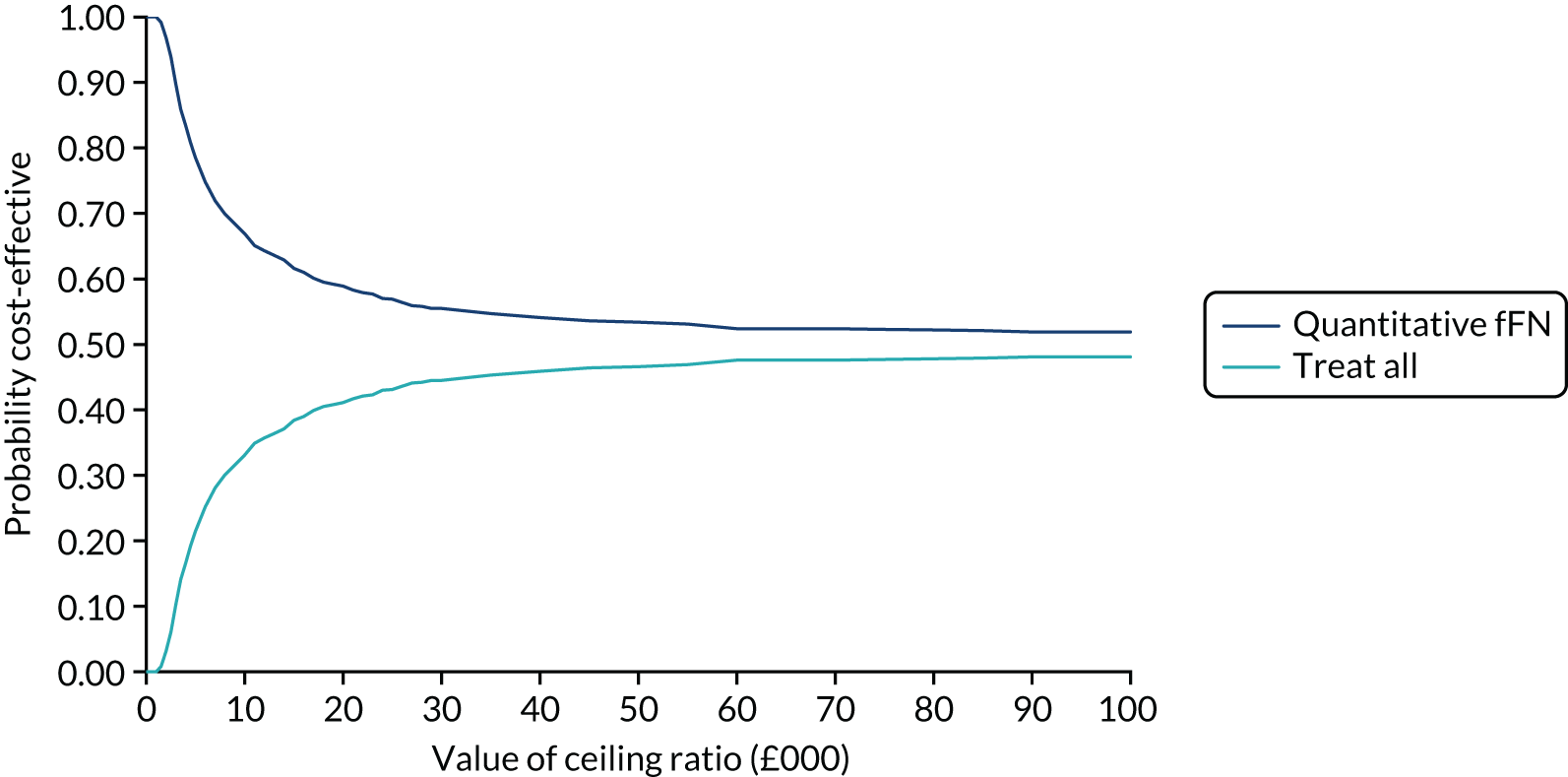
FIGURE 21.
Expected value of perfect parameter information for quantitative fFN at ≥ 2% probability of spontaneous preterm birth vs. treat all.
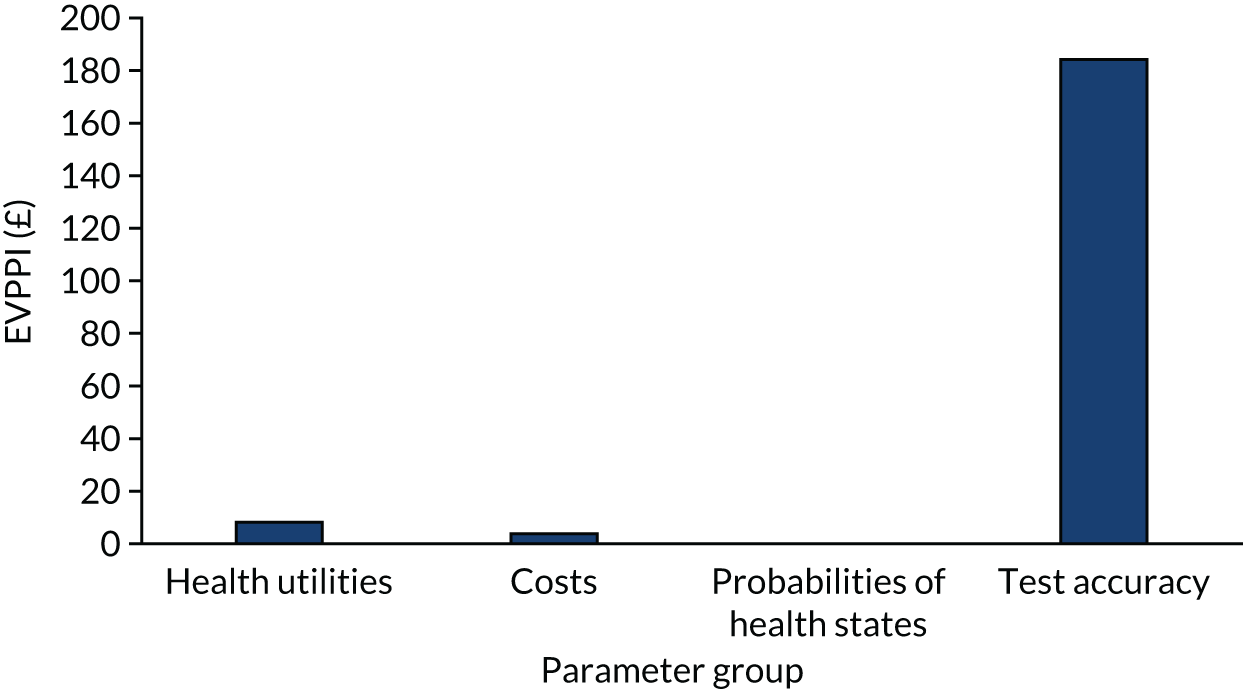
FIGURE 22.
Cost-effectiveness plane for qualitative fFN vs. treat all.
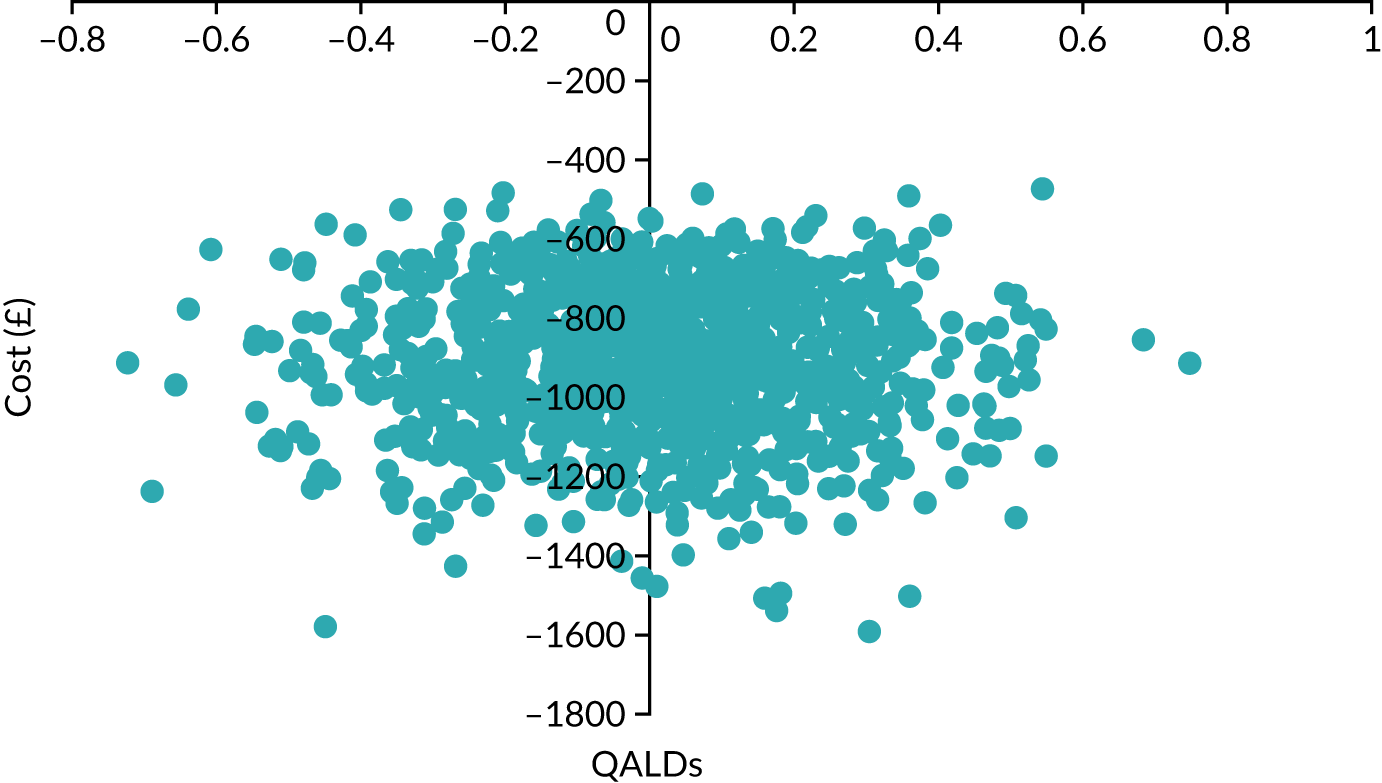
FIGURE 23.
Cost-effectiveness acceptability curve for qualitative fFN vs. treat all.
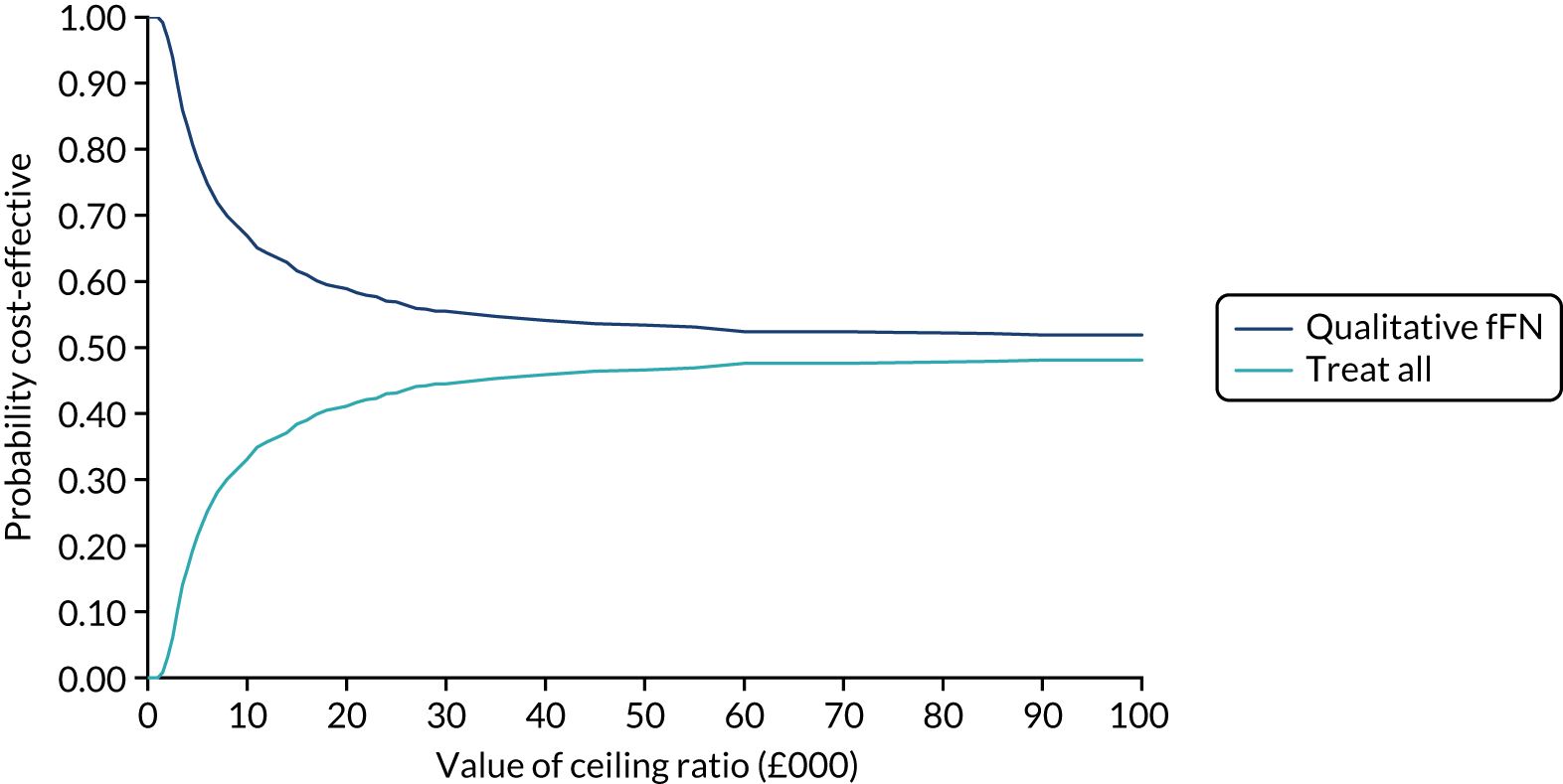
FIGURE 24.
Expected value of perfect parameter information for qualitative fFN vs. treat all.
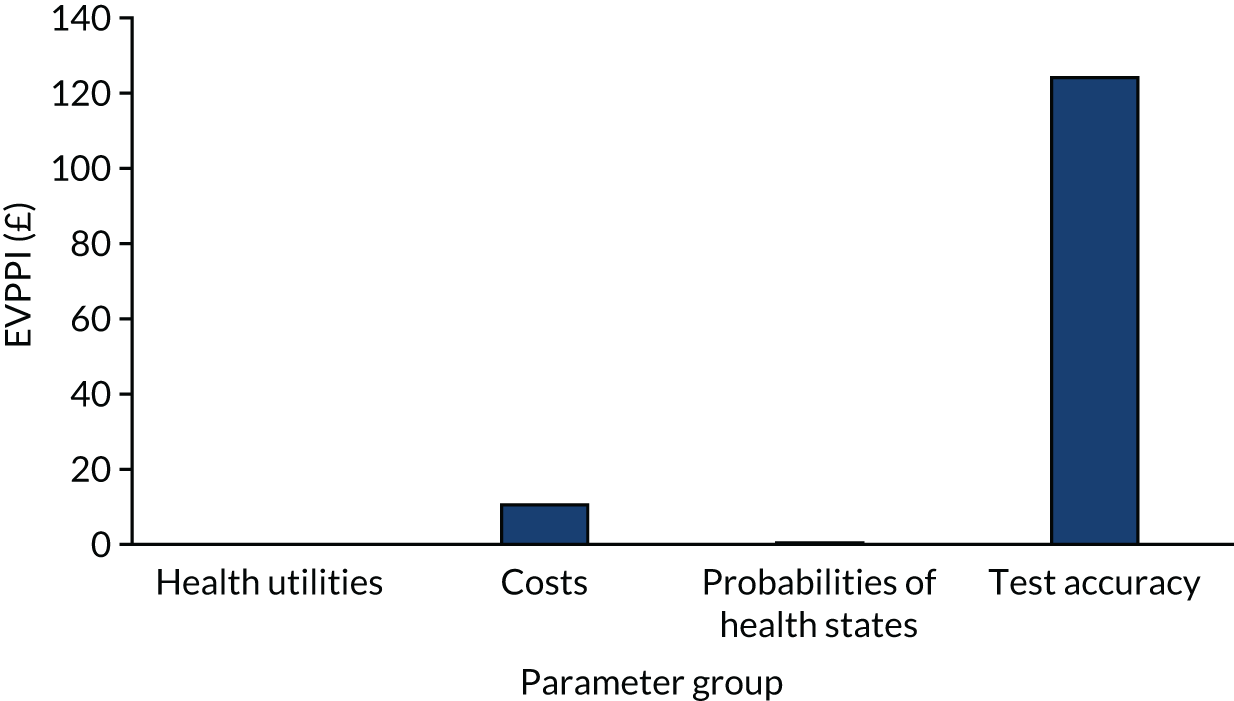
Part 2: probabilistic sensitivity analysis results
FIGURE 25.
Cost-effectiveness acceptability curve for model A at ≥ 2% probability of spontaneous preterm birth vs. model C at ≥ 5% probability of spontaneous preterm birth.
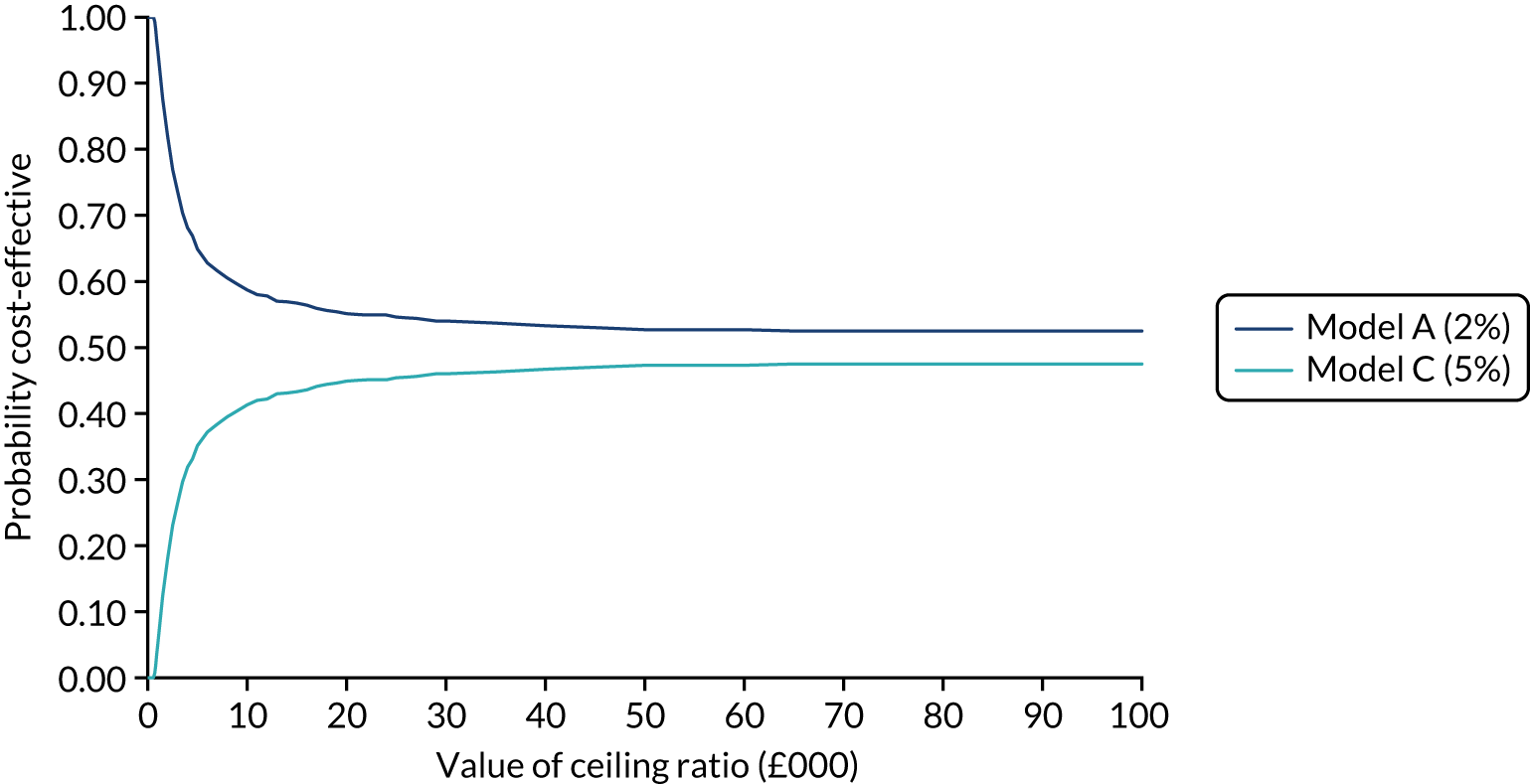
FIGURE 26.
Expected value of perfect parameter information for model A at ≥ 2% probability of spontaneous preterm birth vs. model C at 5% probability of spontaneous preterm birth.
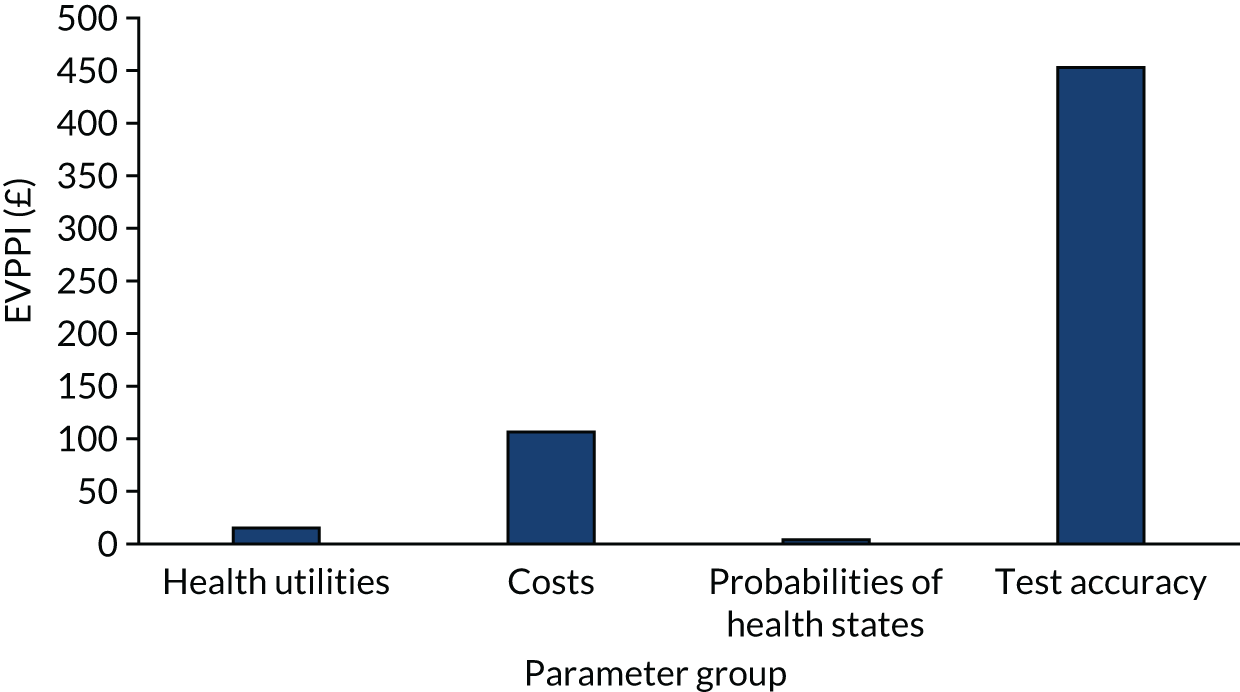
Appendix 8 Sites included in the QUIDS multicentre prospective cohort study
Name | Postcode | PI | Deliveries per annum | Neonatal care level |
---|---|---|---|---|
Bedford Hospital | MK42 9DJ | Mrs Sarah Reynolds | 2691 | SCBU |
Birmingham City Hospital | B18 7QH | Dr Maheshwari Srinivasan | 5073 | LNU |
Birmingham Heartlands Hospital | B9 5SS | Dr Mani Malarselvi | 5535 | NICU |
Birmingham Women’s Hospital | B15 2TG | Dr R Katie Morris | 6770 | NICU |
Borders General Hospital | TD6 9BS | Dr Alex Viner | 966 | LNU |
Darlington Memorial Hospital | DL3 6HX | Dr Shilpi Mittal | 1787 | SCBU |
Hinchingbrooke Hospital | PE29 6NT | Dr Sangeeta Pathak | 2108 | LNU |
King’s Mill Hospital | NG17 4JL | Dr Jyothi Rajeswary | 2815 | LNU |
Nevill Hall Hospital | NP7 7EG | Dr Anurag Pinto | 1763 | SCBU |
Princess of Wales Hospital | CF31 1RQ | Mr Marsham Moselhi | 2000 | LNU |
Queen Alexandra Hospital | PO6 3LY | Mr Saumitra Sengupta | 5182 | NICU |
Queen Elizabeth Hospital (Gateshead) | NE9 6SX | Mr Vaideha Deshpande | 1616 | SCBU |
Queen Elizabeth University Hospital | G51 4TF | Dr Stewart Pringle | 5129 | NICU |
Queen’s Hospital | RM7 0AG | Dr Chineze Otigbah | 7388 | LNU |
Royal Gwent Hospital | NP20 2UB | Dr Anurag Pinto | 3248 | LNU |
Royal Infirmary of Edinburgh | EH16 4SA | Dr Shona Cowan | 6057 | NICU |
Royal London Hospital | E1 1BB | Mr Matthew Hogg | 4097 | NICU |
Singleton Hospital | SA2 8QA | Mr Marsham Moselhi | 2861 | NICU |
South Tyneside District Hospital | NE34 0PL | Mr Umo Esen | 1228 | SCBU |
St George’s Hospital | SW17 0QT | Professor Asma Khalil | 4642 | NICU |
St Richard’s Hospital | PO19 6SE | Mr Attila Vecsei | 2454 | LNU |
St Thomas’ Hospital | SE1 7EH | Professor Andy Shennan | 5541 | NICU |
Stoke Mandeville Hospital | HP21 8AL | Miss Aparna Reddy | 4950 | LNU |
University College Hospital | NW1 2BU | Dr Davide Casagrandi | 5939 | NICU |
University Hospital of North Durham | DH1 5TW | Dr Shilpi Mittal | 2654 | LNU |
University Hospital of North Tees | TS19 8PE | Mr Steve Wild | 2699 | NICU |
Whipps Cross University Hospital | E11 1NR | Mr Matthew Hogg | 4292 | SCBU |
Worthing Hospital | BN11 2DH | Mr Attila Vecsei | 2197 | LNU |
Appendix 9 The QUIDS study assessments
Visit | Attendance with signs and symptoms preterm labour | |||
---|---|---|---|---|
Screening and recruitment | 24–48 hours | 1–6 months | Birth | |
Inclusion/exclusion criteria | ✓ | |||
PIS | ✓ | |||
Consent form | ✓ | |||
Demographics | ✓ | |||
Obstetric history | ✓ | |||
Symptoms and signs | ✓ | |||
Quantitative fFN (ng/ml) | ✓ | |||
Cervical length scan (if available) | ✓ | |||
STIA questionnaire | ✓ | ✓ | ||
Birth details | ✓ | |||
Neonatal outcomes | ✓ | |||
Qualitative acceptability questionnaires (subgroup, n = 30) | ✓ |
Appendix 10 Baseline characteristics for individual participant data analysis data set and prospective cohort study
Baseline characteristic | QUIDS IPD data set (N = 1783) | QUIDS cohort study (N = 2924) |
---|---|---|
Age (years), mean (SD) | 29.7 (5.6) | 28.2 (5.6) |
BMI (kg/m2), mean (IQR) | 24.8 (22.0–28.4) | 25.4 (22.2–30.2) |
Ethnicity, n (%) | ||
White | 1206 (67.6) | 2578 (88.2) |
South Asian | 78 (4.4) | 169 (5.7) |
East Asian | 46 (2.6) | 8 (0.3) |
African/Caribbean/Middle-Eastern | 381 (21.4) | 100 (3.4) |
Other | 72 (4.0) | 69 (2.4) |
Current smoker, n (%) | 208 (11.7) | 614 (21.0) |
Nulliparous, n (%) | 924 (51.8) | 1030 (35.2) |
Multiple pregnancy, n (%) | 186 (10.4) | 100 (3.5) |
Gestational age (weeks), median (IQR] | 29.4 (26.4–31.7) | 31.0 (27.9–33.1) |
Previous spontaneous preterm birth at < 34 weeks’ gestation, n (%) | 196 (11.0) | 174 (6.0) |
Cervical length (mm), mean (SD) | 23.8 (11.2) | |
Qualitative fFN: positive, n (%) | 548 (30.7) | 413 (14.1) |
Quantitative fFN (ng/ml), median (IQR) | 11 (3–79) | 7 (4–22) |
Tocolysis, n (%) | 717 (40.2) |
Appendix 11 Sensitivity analyses
Model A (variable selection) | Primary analysis | Sensitivity analyses | ||
---|---|---|---|---|
Performance measure | Any preterm birth | Singletons only | Complete case | |
Discrimination | ||||
AUC C-statistic: point estimate | 0.89 | 0.88 | 0.90 | 0.89 |
95% CI | 0.85 to 0.93 | 0.83 to 0.92 | 0.85 to 0.94 | 0.85 to 0.94 |
Calibration | ||||
Calibration-in-the-large | 0.2884 | 0.4227 | 0.2418 | 0.303 |
Calibration slope | 1.2041 | 1.1731 | 1.2747 | 1.211 |
Recalibrated intercept | –5.0637 | –4.9294 | –5.4849 | –5.423 |
Model B (variable selection) | Primary analysis | Sensitivity analyses | ||
Performance measure | Any preterm birth | Singletons only | Complete case | |
Discrimination | ||||
AUC C-statistic: point estimate | 0.89 | 0.88 | 0.90 | 0.89 |
95% CI | 0.85 to 0.93 | 0.83 to 0.92 | 0.85 to 0.94 | 0.85 to 0.93 |
Calibration | ||||
Calibration-in-the-large | 1.1878 | 1.3288 | 1.1496 | 1.206 |
Calibration slope | 1.1023 | 1.0746 | 1.1634 | 1.100 |
Recalibrated intercept | –7.4839 | –7.3430 | –7.5221 | –7.817 |
Appendix 12 Economic analysis methods
Test strategy | Unit recorded in study | Unit cost (£) | Source |
---|---|---|---|
Maternal admissiona | Hours (and minutes) | 449.00 per day | 65 |
Corticosteroidsb | |||
Betamethasone | Per dose | 11.30 | 70 |
Dexamethasone | Per dose | 8.70 | 70 |
Magnesium sulphateb | Hours (and minutes) | 7.70 | 70 |
Tocolyticsb | |||
Nidedipine | Hours (and minutes) | 0.05 | 70 |
Indometacin | Hours (and minutes) | 0.15 | 70 |
Glyceryl trinitrate | Hours (and minutes) | 0.02 | 70 |
Atosiban | Hours (and minutes) | 9.21 | 70 |
Other | Hours (and minutes) | 0.05 | 70 |
Neonatal admissiona | |||
SCBU | Hours (and minutes) | 583.00 per day | 65 |
LNU | Hours (and minutes) | 920.00 per day | 65 |
NICU | Hours (and minutes) | 1434.00 per day | 65 |
Hospital transfer | Per transfer | 965.00 | 14 |
Complications | |||
CPAP | Hours (and minutes) | 208.00 per day | 98 |
Intubation | Per treatment | 208.00 | 98 |
Oxygen | Hours (and minutes) | 18.90 per day | 98 |
Surfactant | Per treatment | 216.00 | 98 |
Surgery | Per treatment | 3945.00 | 99 |
Treatment effect | Value | SE | Probability distribution | Reference |
---|---|---|---|---|
Relative risk reduction of corticosteroids on mortality | 0.69 | 0.058 | Log-normal | 6 |
Relative risk reduction of corticosteroids on morbidity | 0.66 | 0.036 | Log-normal | 6 |
Neonatal outcomes | Value | SE | Probability distribution | Reference |
Probability of death | 0.01 | 0.001 | Beta | 95 |
Probability of major morbidity | 0.08 | 0.008 | Beta | 95 |
Probability of minor morbidity | 0.38 | 0.038 | Beta | 95 |
Probability of healthy | 0.53 | 0.053 | Beta | 95 |
Test performance at alternative admit thresholds | Sensitivity | Specificity | Probability distribution | Reference |
Model A | ||||
≥ 2% | 0.79 | 0.84 | NA | Cohort study |
≥ 5% | 0.59 | 0.89 | NA | Cohort study |
≥ 10% | 0.49 | 0.90 | NA | Cohort study |
≥ 15% | 0.39 | 0.91 | NA | Cohort study |
≥ 20% | 0.22 | 0.92 | NA | Cohort study |
≥ 25% | 0.15 | 0.92 | NA | Cohort study |
Health utilities | Value | SE | Probability distribution | Reference |
Healthy | 0.88 | 0.08 | Beta | 96 |
Utility of minor morbidity | 0.83 | 0.21 | Beta | 67 |
Utility of major morbidity | 0.76 | 0.23 | Beta | 67 |
Utility of death | 0 | NA | NA | Assumption |
Analysis
The cost-effectiveness of alternative prognostic strategies was evaluated by its ICER, which was calculated as follows:
where ΔCosts is the difference in total costs between alternative prognostic strategies and ΔQALY is the difference in utility between alternative prognostic strategies. This ICER can be compared against a societal willingness to pay for a 1-QALY gain (of £20,000, in line with NICE reference case for cost per QALY). 66 Because we are considering QALYs a 7-day time horizon, we will represent our results in terms of QALD, assuming a willingness to pay for a 1-QALD gain of £55 per day.
The cost-effectiveness of the alternative prognostic strategies may also be converted to the NMB where there are multiple comparators. The NMB is a measure of the health benefit, expressed in monetary terms, which incorporates the cost of the new strategy, the health gain obtained and the societal willingness-to-pay threshold for health gains (£20,000). The NMB is expressed using the following formula:
where E is effectiveness, WTP is the willingness-to-pay threshold and C is cost. The NMB approach is recommended when comparing more than one intervention and provides a clear decision rule (i.e. if NMB > 0, the new strategy is cost-effective). Results can also be presented incrementally as the incremental NMB.
Results
Sensitivity analysis
One-way sensitivity analysis
-
The cost of lifetime major morbidity was varied from £115,000 to £57,500 (50% reduction). The strategy with the highest NMB (admit/no admit based on a ≥ 2% risk threshold) was unchanged.
-
The utility of lifetime major morbidity was reduced from 0.76 to 0.40. This had the result of changing the strategy with the highest NMB (admit/no admit based on a ≥ 2% risk threshold) from £827 to £822. There was no change in which strategy had the highest NMB.
-
A lower discount rate of 1.5% was applied to future costs and utilities, which may be more appropriate (than a rate of 3.5%) to better reflect the lifetime outcomes for very young children. This had the result of changing the strategy with the highest NMB (admit/no admit based on a ≥ 2% risk threshold) from £827 to £810. There was no change in which strategy had the highest NMB.
Probabilistic sensitivity analysis
Figure 27 presents the CEAC, which illustrates the probability of the prognostic model (model A at a ≥ 2% risk threshold) being cost-effective across a range of willingness-to-pay thresholds.
FIGURE 27.
Cost-effectiveness plane for incremental cost and QALDs of quantitative fFN at a ≥ 2% risk threshold vs. treat all over a lifetime time horizon.
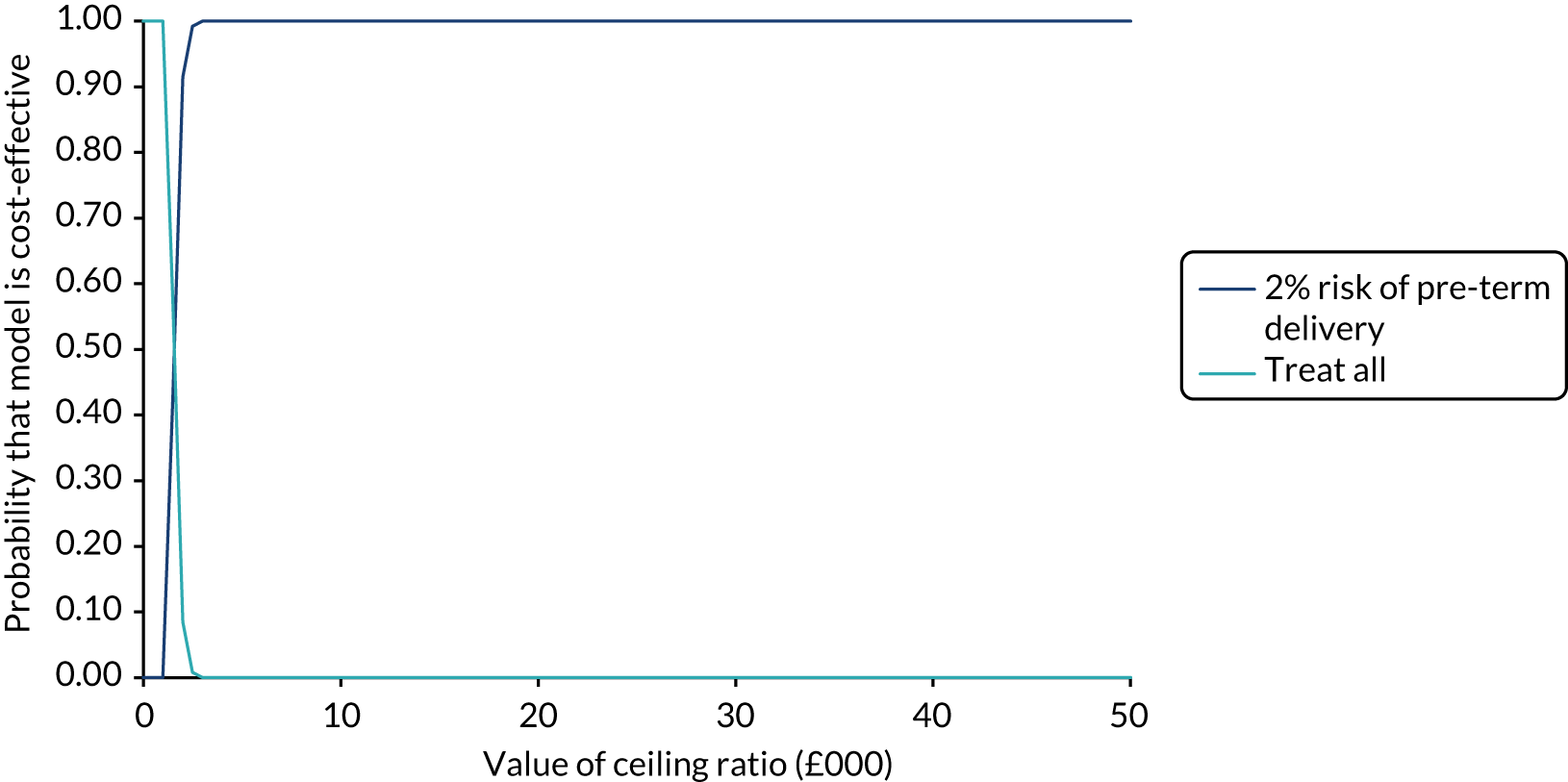
Appendix 13 Cost analysis details on the cohort study resource use data
Assumptions and missing data
Neonatal resource use
-
Defining the population: patients are counted as having a neonatal admission if they have a date of admission, date of discharge or reason for admission given.
-
Duration of stay: duration of stay in neonatal ward is estimated by subtracting the date of admission from the date of discharge if the patient is counted as having a neonatal admission. If date of admission is present but date of discharge is not, it is checked if the patient died in the neonatal ward; if so, the date of death is regarded as the date of discharge. If date of discharge is present but there is no date of admission, and if a reason for admission is present but there is no date of admission/discharge, we impute the median length of stay for a patient in the neonatal ward.
-
Cost of stay: if the patient is recorded as having a neonatal admission, their time spent in the relevant level of care is multiplied by the unit cost for that level of care. If the level of care is not given, the duration of stay is multiplied by the mean cost of care in a neonatal setting.
-
Transfers: if a patient is recorded as having a neonatal admission and an infant transfer, they are recorded as incurring a mean transfer cost.
-
Cost of stay when transferred: if a patient is recorded as having a neonatal admission and being transferred, the level of care to which they are transferred is recorded and multiplied by the relevant unit cost of care. If a patient is recorded as having a neonatal admission and being transferred but the level of care to which they were transferred is not recorded, we impute median length of stay for patients who are transferred and attach to this the mean cost of care in a neonatal setting.
-
Continuous positive airway pressure (CPAP): if a patient is recorded as having a neonatal admission, they are recorded as receiving CPAP if they have a CPAP start date or stop date or a CPAP of 1 (yes). For these patients, the duration of CPAP is estimated as the time of CPAP stop minus CPAP start.
-
Intubation: same as CPAP.
-
Oxygen support: same as CPAP.
-
Surgery: if a patient is recorded as having a neonatal admission and surgery, we attach to this patient a ‘surgical paediatrics’ episode cost.
Maternal resource use
-
Defining the population: patients are counted as having a maternal admission if they have a date of admission, date of discharge or type of admission is given.
-
Duration of stay: duration of stay in maternal ward is estimated by subtracting the date of admission from the date of discharge if the patient is counted as having a maternal admission. If date of admission is present, but date of discharge is not (or if the date of discharge is present, but the date of admission is not), and type of admission is present, patients are given the median length of stay in the ward recorded for their type of admission.
-
Cost of stay: if the patient is recorded as having a maternal admission, their time spent in the relevant level of care is multiplied by the unit cost for that level of care. If the level of care is not given, the duration of their stay is multiplied by the mean cost of care in a maternal setting.
-
Delivery cost: if the patient is recorded as having a maternal admission, their delivery cost is estimate based on the type of delivery recorded for the patient.
-
Transfers: if a patient is recorded as having a maternal admission and a transfer, they are recorded as incurring a mean transfer cost.
-
Corticosteroids: if a patient is recorded as having a maternal admission and as receiving corticosteroids, it is checked whether they received one or two doses and which type of corticosteroids they received. Unit costs for the type of corticosteroid and doses given are then attached accordingly.
-
Magnesium sulphate: if a patient is recorded as having a maternal admission and as receiving magnesium sulphate, the duration of time on magnesium sulphate is attached to the relevant unit cost.
-
Tocolytics: if a patient is recorded as having a maternal admission and as receiving tocolytics (1) before this assessment or (2) after this assessment, the duration of time spent on tocolytics is multiplied by the unit cost for the type of tocolytic given.
Appendix 14 Prognostic model scenarios
At interview, clinicians were given detailed information about the QUIDS prognostic model, including how it was developed and the individual variables included, and had the opportunity to ask questions. They were then provided with one (or two) of three different clinical scenarios relating to women presenting to maternity services with symptoms of preterm labour (see Scenario A, Scenario B and Scenario C). Clinicians were then asked to discuss their clinical impression and management plan at four different time points in the scenario: following initial clinical examination, following qualitative fFN result, following quantitative fFN result and following the prognostic model result. A high proportion of clinicians did not provide a clinical management plan following addition of the quantitative fFN result because they were not experienced in interpreting this value.
Clinical management plans varied between clinicians and often altered slightly following the addition of more information throughout the scenario. For scenario A, the addition of fFN led all clinicians who had not already done so (7/11 clinicians) to recommend admission and antenatal corticosteroids. Following the prognostic model risk prediction, those who had not already done so (4/11 clinicians) now included consideration of tocolytics, magnesium sulphate and in utero transfer if indicated. For scenario B, the majority of clinicians planned to monitor the woman following the clinical assessment only, whereas following fFN testing those who had not already done so (10/12 clinicians) were more likely to step up their management, often recommending admission, steroids and consideration of tocolysis and in utero transfer. Following the prognostic model risk prediction, the majority of clinicians recommended the same management plan, whereas a few (3) reduced their recommended intervention, including recommending discharge, or delaying or omitting steroids. For scenario C, clinicians varied between recommending admission and commencing steroid administration (7) and observing the woman following clinical assessment only (4). The addition of fFN results reassured some to discharge the woman (4). Following the prognostic model risk prediction, some more clinicians recommended discharge or decided to hold off recommending steroids (4), whereas one clinician changed their recommendation from discharge to admission.
Scenario A
-
Woman A attends maternity triage/the delivery suite with symptoms of preterm labour at 30 weeks’ gestation. She is contracting three times in every 10 minutes, appears to be in a lot of discomfort and is requesting analgesia. The contractions palpate moderate in strength and are clearly picking up on the cardiotocograph. Her urinalysis result is normal: no abnormalities detected. She has no vaginal bleeding. On speculum examination her cervix appears closed and there is no evidence of or history of PPROM. She is 35 years old and a para 0, with a BMI of 24 kg/m2, and the pregnancy is a singleton pregnancy. She is a non-smoker and her ethnicity is South Asian.
-
Her qualitative fFN result was positive.
-
Her quantitative fFN result was 303 ng/ml.
-
The risk prediction for delivery within 7 days based on the fFN result and all clinical factors in the predictive model was 46%.
Scenario B
-
Woman B attends the delivery suite with symptoms of preterm labour at 31 weeks’ gestation. She is contracting irregularly, one or two times in every 10 minutes. Although she is not in an awful lot of pain, and what pain she has is predominantly in her back, she is very distressed and anxious. The contractions palpate mild in strength and are showing on the cardiotocograph. Her urinalysis result is normal: no abnormalities detected. She has no vaginal bleeding. On speculum examination her cervix appears closed and there is no evidence or history of PPROM. She is 33 years old and a para 0, with a BMI of 24 kg/m2, and the pregnancy is a singleton pregnancy. She is a non-smoker and her ethnicity is white.
-
Her fFN result was positive.
-
Her quantitative fFN result was 98 ng/ml.
-
The risk prediction for delivery within 7 days based on the fFN result and all clinical factors in the predictive model was 6%.
Scenario C
-
Woman C attends maternity triage with symptoms of preterm labour at 32 weeks’ gestation. She is contracting twice in every 10 minutes, and appears to be in moderate discomfort. The contractions palpate moderate in strength and are picking up on the cardiotocograph. Her urinalysis result is normal: no abnormalities detected. She has no vaginal bleeding. On speculum examination her cervix appears closed and there is no evidence or history of PPROM. She is 34 years old and a para 0, with a BMI of 20, and a singleton pregnancy. She is a non-smoker and her ethnicity is African.
-
Her qualitative fFN result is negative.
-
The quantitative fFN result was 49 ng/ml.
-
The risk prediction for delivery within 7 days based on the fFN result and all clinical factors in the predictive model was 3%.
Prompts
Prompts after each additional piece of information: what is your clinical judgement at this stage? What do you consider her risk of preterm birth to be? What is your plan of care? How would you explain these to woman [A/B/C]?
Appendix 15 The QUIDS2 sites
Name | Postcode | PI | Births per annum | Neonatal care level |
---|---|---|---|---|
Birmingham Heartlands Hospital | B9 5SS | Dr Mani Malarselvi | 5535 | NICU |
Birmingham Women’s Hospital | B15 2TG | Dr R Katie Morris | 6770 | NICU |
Darlington Memorial Hospital | DL3 6HX | Dr Shilpi Mittal | 1787 | SCBU |
Hinchingbrooke Hospital | PE29 6NT | Dr Sangeeta Pathak | 2108 | LNU |
Nevill Hall Hospital | NP7 7EG | Dr Anurag Pinto | 1763 | SCBU |
Queen Alexandra Hospital | PO6 3LY | Mr Saumitra Sengupta | 5182 | NICU |
Queen Elizabeth Hospital (Gateshead) | NE9 6SX | Mr Vaideha Deshpande | 1616 | SCBU |
Queen Elizabeth University Hospital | G51 4TF | Dr Stewart Pringle | 5129 | NICU |
Royal Gwent Hospital | NP20 2UB | Dr Anurag Pinto | 3248 | LNU |
Royal Infirmary of Edinburgh | EH16 4SA | Dr Shona Cowan | 6057 | NICU |
Singleton Hospital | SA2 8QA | Mr Marsham Moselhi | 2861 | NICU |
South Tyneside District Hospital | NE34 0PL | Mr Umo Esen | 1228 | SCBU |
St George’s Hospital | SW17 0QT | Professor Asma Khalil | 4642 | NICU |
St Thomas’ Hospital | SE1 7EH | Professor Andy Shennan | 5541 | NICU |
Stoke Mandeville Hospital | HP21 8AL | Miss Aparna Reddy | 4950 | LNU |
University College Hospital | NW1 2BU | Dr Davide Casagrandi | 5939 | NICU |
University Hospital of North Durham | DH1 5TW | Dr Shilpi Mittal | 2654 | LNU |
University Hospital of North Tees | TS19 8PE | Mr Steve Wild | 2699 | NICU |
Whipps Cross University Hospital | E11 1NR | Mr Matthew Hogg | 4292 | SCBU |
Appendix 16 Sample size calculations for QUIDS2
Sensitivity (%) | Event rate (%) | Achieved sample size | N = 500 | ||
---|---|---|---|---|---|
N = 350 | N = 400 | N = 450 | |||
75 | 3.0 | 26.2 | 24.5 | 23.1 | 21.9 |
3.5 | 24.2 | 22.7 | 21.3 | 20.3 | |
4.0 | 22.7 | 21.2 | 20.0 | 19.0 | |
80 | 3.0 | 24.2 | 22.6 | 21.3 | 20.2 |
3.5 | 22.4 | 21.0 | 19.8 | 18.7 | |
4.0 | 21.0 | 19.6 | 18.5 | 17.5 | |
85 | 3.0 | 21.6 | 20.2 | 19.1 | 18.1 |
3.5 | 20.0 | 18.7 | 17.6 | 16.7 | |
4.0 | 18.7 | 17.5 | 16.5 | 15.6 | |
90 | 3.0 | 18.1 | 17.0 | 16.0 | 15.2 |
3.5 | 16.8 | 15.7 | 14.8 | 14.1 | |
4.0 | 15.7 | 14.7 | 13.9 | 13.1 | |
95 | 3.0 | 13.2 | 12.3 | 11.6 | 11.0 |
3.5 | 12.2 | 11.4 | 10.8 | 10.2 | |
4.0 | 11.4 | 10.7 | 10.1 | 9.6 | |
99 | 3.0 | 6.0 | 5.6 | 5.3 | 5.0 |
3.5 | 5.6 | 5.2 | 4.9 | 4.7 | |
4.0 | 5.2 | 4.9 | 4.6 | 4.5 |
Appendix 17 Additional QUIDS team members
Name | Site | Designation |
---|---|---|
Shona Cowan | Royal Infirmary of Edinburgh | PI |
Morag Dalton | Royal Infirmary of Edinburgh | Research midwife |
Alex Viner | Borders General Hospital | PI |
Brian Magowan | Borders General Hospital | Obstetrician |
Joy Dawson | Borders General Hospital | Data manager |
Shilpi Mittal | University Hospital of North Durham | PI |
Vicki Atkinson | University Hospital of North Durham | Research midwife |
Jacqui Jennings | Darlington Memorial Hospital | Research midwife |
Umo Essen | South Tyneside District Hospital | PI |
Judith Ormonde | South Tyneside District Hospital | Research midwife |
Vaideha Deshpande | Queen Elizabeth Hospital (Gateshead) | PI |
Christine Moller-Christensen | Queen Elizabeth Hospital (Gateshead) | Research midwife |
Phern Adams | Birmingham Women’s Hospital | Lead research midwife |
Nicola Farmer | Birmingham Women’s Hospital | Midwife |
Cody Allen | Birmingham Women’s Hospital | Midwife |
Mani Malarselvi | Birmingham Heartlands Hospital | PI |
Lucy O’Leary | Birmingham Heartlands Hospital | Research midwife |
Lucy Sheppard | Birmingham Heartlands Hospital | Research nurse |
Anurag Pinto | Royal Gwent Hospital/ Nevill Hall Hospital | PI |
Emma Mills | Royal Gwent Hospital/ Nevill Hall Hospital | Lead research and innovation midwife |
Tracy James | Royal Gwent Hospital/ Nevill Hall Hospital | Research midwife |
Kelly Griffiths | Royal Gwent Hospital/ Nevill Hall Hospital | Midwife |
Becky Westbury | Royal Gwent Hospital/ Nevill Hall Hospital | Midwife |
Patricia Jarvis | Royal Gwent Hospital/ Nevill Hall Hospital | Midwife |
Yaa Acheampong | St George’s Hospital | Research midwife |
Daniella Hake | St George’s Hospital | Research midwife |
Nessa Muhidun | St George’s Hospital | Clinical trial administrator |
Jyothi Rajeswary | King’s Mill Hospital | PI |
Katie Slack | King’s Mill Hospital | Research nurse |
Caroline Moulds | King’s Mill Hospital | Research nurse |
Sarah Shelton | King’s Mill Hospital | Research nurse |
Mandy Gill | King’s Mill Hospital | Research nurse |
Attila Vecsei | St Richard’s Hospital/Worthing Hospital | PI |
Emma Meadows | St Richard’s Hospital | Research midwife |
Viv Cannons | Worthing Hospital | Research midwife |
Sangeeta Pathak | Hinchingbrooke Hospital | PI |
Tara Pauley | Hinchingbrooke Hospital | Research midwife |
Christie Oakes | Hinchingbrooke Hospital | Research midwife |
Kimberley Morris | Hinchingbrooke Hospital | Research midwife |
Charlotte Clayton | Hinchingbrooke Hospital | Research midwife |
Marsham Moselhi | Princess of Wales Hospital/Singleton Hospital | PI |
Sharon Jones | Princess of Wales Hospital/Singleton Hospital | Lead research midwife |
Helen Worrell | Princess of Wales Hospital/Singleton Hospital | Research midwife |
Eve Watkins | Princess of Wales Hospital/Singleton Hospital | Research midwife |
Maria Nash | Princess of Wales Hospital/Singleton Hospital | Research midwife |
Sian Phillips | Princess of Wales Hospital/Singleton Hospital | Research midwife |
Cath Jones | Princess of Wales Hospital/Singleton Hospital | Research midwife |
Claire Vaughan Hughes | Princess of Wales Hospital/Singleton Hospital | Research midwife |
Rhian Love | Princess of Wales Hospital/Singleton Hospital | Research midwife |
Andrea Hill | Princess of Wales Hospital/Singleton Hospital | Research midwife |
Rhian Lewis | Princess of Wales Hospital/Singleton Hospital | Research midwife |
Steve Wild | University Hospital North Tees | PI |
Sharon Gowan | University Hospital North Tees | Research midwife |
Alison Samuels | University Hospital North Tees | Research midwife |
Aparna Reddy | Stoke Mandeville Hospital | PI |
Julie Tebbutt | Stoke Mandeville Hospital | Lead research midwife |
Sarah Reynolds | Bedford Hospital | PI |
Carina Gaplin | Bedford Hospital | Research co-ordinator |
Marina Iaverdino | Bedford Hospital | Research midwife |
Stewart Pringle | Queen Elizabeth University Hospital | PI |
Therese McSorely | Queen Elizabeth University Hospital | Research nurse |
Kirsteen Paterson | Queen Elizabeth University Hospital | Research midwife |
Maheshwari Srinivasan | Birmingham City Hospital | PI |
Sarah Potter | Birmingham City Hospital | Research midwife |
Sarah Figg | Birmingham City Hospital | Research midwife |
Lavinia Henry | Birmingham City Hospital | Research midwife |
Matthew Hogg | Royal London Hospital | PI |
Zoi Vardavaki | Royal London Hospital | Research midwife |
Alice Rossi | Royal London Hospital | Research midwife |
Matthew Hogg | Whipps Cross University Hospital | PI |
Prudence Jones | Whipps Cross University Hospital | Senior research midwife |
Sujatha Thamban | Whipps Cross University Hospital | PI |
Saumitra Sengupta | Queen Alexandra Hospital | PI |
Zoe Garner | Queen Alexandra Hospital | Research midwife |
Amanda Hungate | Queen Alexandra Hospital | Clinical trial administrator |
Berni Edge | Queen Alexandra Hospital | Midwife |
Layla Toomer | Queen Alexandra Hospital | Midwife |
Kay Andrews | Queen Alexandra Hospital | Midwife |
Faith Hagger | Queen Alexandra Hospital | Midwife |
Chineze Otigbah | Queen’s Hospital | PI |
Anne-Marie McGregor | Queen’s Hospital | Research midwife |
Elsie Uwegba-Obatarhe | Queen’s Hospital | Advanced midwifery practitioner |
Agnieszka Glazewska-Hallin | St Thomas’ Hospital | Obstetrician |
Alexandria Fry | St Thomas’ Hospital | Research midwife |
Giorgia Dalla Valle | St Thomas’ Hospital | Research midwife |
Davide Casigrande | University College Hospital | PI |
Clara Cantalapiedra Calvete | University College Hospital | Lead senior research midwife |
Rebecca Daley | University College Hospital | Research midwife |
Natasha Baker | University College Hospital | Research midwife |
Caroline Ramsey | University College Hospital | Research midwife |
Amos Tetteh | University College Hospital | Obstetrician |
Eirini Vaikouski | University College Hospital | Obstetrician |
Rita Sarquis | University College Hospital | Co-investigator |
Foteini Emmanouella Bredaki | University College Hospital | Investigator |
Chandrima Biswas | Whittington Health NHS Trust | Obstetrician |
Ora Jesner | Whittington Health NHS Trust | Obstetrician |
List of abbreviations
- APOSTEL-1
- Alleviation of Pregnancy Outcome by Suspending of Tocolysis in Early Labour – 1
- app
- mobile application
- AUC
- area under the receiver operating characteristics curve
- BMI
- body mass index
- CEAC
- cost-effectiveness acceptability curve
- CI
- confidence interval
- CIN
- cervical intraepithelial neoplasia
- CPAP
- continuous positive airway pressure
- CRF
- case report form
- ELISA
- enzyme-linked immunosorbent assay
- EQUIPP
- Evaluation of Fetal Fibronectin with a Quantitative Instrument for the Prediction of Preterm Birth
- EUFIS
- European Fibronectin Study
- EVPI
- expected value of perfect information
- EVPPI
- expected value of perfect parameter information
- fFN
- fetal fibronectin
- GCP
- Good Clinical Practice
- HTA
- Health Technology Assessment
- ICER
- incremental cost-effectiveness ratio
- IGFBP-1
- insulin-like growth factor-binding protein 1
- IPD
- individual participant data
- IQR
- interquartile range
- ISRCTN
- International Standard Registered Clinical/soCial sTudy Number
- LNU
- local neonatal unit
- MAR
- missing at random
- MFP
- Multivariable Fractional Polynomial
- NICE
- National Institute for Health and Care Excellence
- NICU
- neonatal intensive care unit
- NIHR
- National Institute for Health Research
- NMB
- net monetary benefit
- PAMG-1
- placental alpha microglobulin 1
- phIGFBP-1
- phosphorylated insulin-like growth factor-binding protein 1
- PI
- principal investigator
- PPROM
- preterm prelabour rupture of membranes
- PREBIC
- Preterm Birth International Collaborative
- PRISMA
- Preferred Reporting Items for Systematic Review and Meta-Analyses
- PSA
- probabilistic sensitivity analysis
- QALD
- quality-adjusted life-day
- QALY
- quality-adjusted life-year
- QFCAPS
- Quantitative fetal fibronectin, Cervical length and Actim Partus for the prediction of Preterm birth in Symptomatic women
- QUADAS-2
- quality assessment of diagnostic accuracy studies 2
- QUIDS
- Quantitative fetal fibronectin to improve decision-making in women with symptoms of preterm birth
- QUIDS qualitative
- Quantitative fetal fibronectin to improve decision-making in women with symptoms of preterm birth qualitative substudy
- QUIDS2
- Quantitative fetal fibronectin to improve decision-making in women with symptoms of preterm birth substudy 2
- RCT
- randomised controlled trial
- ROC
- receiver operator characteristic
- SCBU
- special care baby unit
- SD
- standard deviation
- STAI
- State–Trait Anxiety Inventory
- STOP
- Screening to Obviate Preterm Birth
- TRIPOD
- Transparent Reporting of a multivariable prediction model for Individual Prognosis Or Diagnosis
- UCLH/Whit
- University College London Hospital/Whittington
- VOI
- value of information
- WEQAS
- Wales External Quality Assurance Scheme Point of Care Quality Assurance Scheme