Notes
Article history
This issue of the Health Technology Assessment journal series contains a project commissioned by the MRC–NIHR Methodology Research Programme (MRP). MRP aims to improve efficiency, quality and impact across the entire spectrum of biomedical and health-related research. In addition to the MRC and NIHR funding partners, MRP takes into account the needs of other stakeholders including the devolved administrations, industry R&D, and regulatory/advisory agencies and other public bodies. MRP supports investigator-led methodology research from across the UK that maximises benefits for researchers, patients and the general population – improving the methods available to ensure health research, decisions and policy are built on the best possible evidence.
To improve availability and uptake of methodological innovation, MRC and NIHR jointly supported a series of workshops to develop guidance in specified areas of methodological controversy or uncertainty (Methodology State-of-the-Art Workshop Programme). Workshops were commissioned by open calls for applications led by UK-based researchers. Workshop outputs are incorporated into this report, and MRC and NIHR endorse the methodological recommendations as state-of-the-art guidance at time of publication.
The authors have been wholly responsible for all data collection, analysis and interpretation, and for writing up their work. The HTA editors and publisher have tried to ensure the accuracy of the authors’ report and would like to thank the reviewers for their constructive comments on the draft document. However, they do not accept liability for damages or losses arising from material published in this report.
Permissions
Copyright statement
Copyright © 2021 French et al. This work was produced by French et al. under the terms of a commissioning contract issued by the Secretary of State for Health and Social Care. This is an Open Access publication distributed under the terms of the Creative Commons Attribution CC BY 4.0 licence, which permits unrestricted use, distribution, reproduction and adaption in any medium and for any purpose provided that it is properly attributed. See: https://creativecommons.org/licenses/by/4.0/. For attribution the title, original author(s), the publication source – NIHR Journals Library, and the DOI of the publication must be cited.
2021 French et al.
Chapter 1 Introduction
Measuring people can affect their behaviour, their emotions and the data that they provide about themselves. 1–3 This phenomenon is sometimes known as measurement reactivity (MR). 1 Randomised controlled trials (RCTs) always include measurements of trial outcomes and commonly include further measurements as part of process evaluations. Measurements include self-reports (e.g. via questionnaires and interviews), objective measurements of behaviour (e.g. via accelerometers) and clinical markers (e.g. blood pressure or body scans that estimate body fat). Measurement techniques used in trials are typically treated as though they are inert (i.e. have no impact on participants). The usual methods of conduct and analysis of trials implicitly assume that the taking of measurements does not affect subsequent outcome measurements or interact with the trial intervention and that any effects of measurement-taking will be the same in each experimental group and, hence, are unlikely to bias treatment comparisons. 1,2,4 Any effects on participants are therefore ignored and not considered as a potential source of bias in trials (i.e. incorrect estimates of intervention effects or their standard errors). The present report aims to promote awareness of when trial measurements can produce bias and to provide recommendations to prevent such bias.
The phenomenon of MR is related to the broader term ‘Hawthorne effect’,5 which is used to refer to research participants changing their behaviour in response to being observed. The Hawthorne effect appeared in a research publication 65 years ago5 and the term is in widespread use, although it has been the subject of little empirical research. 6 It has been suggested that the Hawthorne effect is an umbrella term for a number of discrete phenomena, including MR, and it is proposed that more precise terms are needed to develop understanding of research participation effects and how they may lead to bias. 7 In the present document, the term MR is used to mean changes (in individual trial participants as well as in others such as health-care professionals) that would not occur in the absence of measurement.
There are few areas of research where there is sufficient evidence to be entirely confident that MR is present. The main exceptions are (1) the question–behaviour effect (QBE)3,8–11 and (2) pedometers. 12,13 The evidence in both of these areas is summarised in Boxes 1 and 2. In addition, there is evidence from randomised studies showing that people who complete questionnaires about the consequences of health conditions have higher anxiety levels than people who have not completed such questionnaires. 18,19 Furthermore, when people complete questionnaires about anxiety for the first time, they score more highly than when they are measured subsequently. 18,20,21 Other measurement procedures widely employed to assess outcomes in RCTs (e.g. assessing body weight) are also used as intervention techniques in their own right because they are seen to be effective at producing behaviour change. 22
Several systematic reviews, including a meta-analytic synthesis of 104 question–behaviour studies across 51 published and unpublished papers, found evidence that measuring a variety of behaviours via questionnaires can affect the subsequent performance of those behaviours. 3,8–11 Much of this evidence derives from studies in which people who were asked to complete a questionnaire about their behaviour, or attitudes or beliefs about that behaviour, showed changes in that behaviour relative to a no-questionnaire control group. Systematic reviews have consistently provided evidence of small effects on objective and subjective measures of behaviour, but there is considerable heterogeneity in the effects. Individual primary studies in the reviews have generally shown that some risk of bias and publication bias may be present, although it does not appear that bias can fully account for the effects observed. 8,9,14
A systematic review of eight RCTs and 18 observational studies found that providing people with pedometers produced an increase in physical activity, particularly when pedometers are provided in conjunction with goal-setting12 and when research participants can access step count readings. 13 Given this, pedometers are sometimes used as part of interventions15 and are often used as tools to measure outcomes. These two purposes for which pedometers are being used has flagged up that measurement is not always inert in trials and that greater consideration is needed for pedometers when used solely as measurement tools.
Although use of pedometers can produce changes in people’s behaviour, it is unclear to what extent their use causes bias in trials; however, there are plausible reasons to think that it does. The mechanism by which the provision of pedometers produces an increase in physical activity is that pedometers allow participants to self-monitor their behaviour. 1,16 The use of self-monitoring is a key component of many behaviour change interventions. 17 Therefore, the use of pedometers as a measurement tool could result in both trial arms receiving assistance in self-monitoring their behaviour when this was intended in only one arm. This would be likely to reduce the observed effect of an intervention that was designed to promote self-monitoring to increase physical activity, relative to the true effect that would be observed without the use of pedometers.
The present report considers the challenges associated with MR for all kinds of RCTs, especially in the context of behaviour change, public health and health services research. 2 The focus on trials is because of the central importance of trials evidence for health-care decision-making. The present recommendations are designed to apply when measurement is used as a method of assessment that produces unintended effects rather than when it has been used as an intended intervention. The report is structured as follows.
In summary, there are multiple and diverse empirical studies showing that measurement may produce changes in the people being measured. By contrast, there is little direct evidence regarding how much of a problem MR poses for bias in trials because there has been little research directly addressing this issue. 4,23 As a consequence, MR has generally been ignored in discussions of how to reduce bias in trials. Given this, MR is not adequately addressed in existing guidelines for designing, reporting [e.g. Consolidated Standards of Reporting Trials (CONSORT)24] and appraising trials (e.g. risk-of-bias frameworks). 25 In the present document we rely on indirect evidence regarding the likely consequences of measurement in producing bias. That is, drawing on the existing evidence regarding where measurement affects research participants, we have produced scenarios where we think it plausible that bias may be produced. The procedure by which these recommendations were developed is described in more detail in Chapter 3, involving systematic reviewing and consultation with experts as part of the MEasurement Reactions In Trials (MERIT) study. It should be noted that, given the limited direct evidence, many of the recommendations are – in the terminology of the Grading of Recommendations Assessment, Development and Evaluations(GRADE) – ‘motherhood statements’, in that to propose the opposite would not be reasonable. 26
In Chapter 2 we spell out how measurement affecting people can produce bias.
In Chapters 3 and 4 we discuss some issues for researchers to consider in relation to MR as a potential source of bias. We include a list of RCT features that should act as ‘red flags’ for researchers to consider as indicating that MR or risk of bias due to MR may be present.
In Chapter 5 we identify future research that is needed to develop a stronger evidence base on the extent of bias in trials due to MR.
The main aims of the present recommendations are to help researchers more systematically consider MR as a potential threat to the validity of trial decision-making and to select appropriate strategies to minimise this potential bias. We aim to highlight the current state of evidence regarding the extent of MR and how it can lead to bias so that researchers can select strategies that are proportionate and mindful of the many other forms of bias that need to be prevented in trials. Finally, we hope to raise awareness of the ways in which trial evidence can be affected by MR and how MR might be better understood in the future.
Chapter 2 Measurement reactivity and risk of bias
Parts of this chapter have been reproduced with permission from French et al. 27 This is an Open Access article distributed in accordance with the terms of the Creative Commons Attribution (CC BY 4.0) license, which permits others to distribute, remix, adapt and build upon this work, for commercial use, provided the original work is properly cited. See: https://creativecommons.org/licenses/by/4.0/. The text below includes minor additions and formatting changes to the original text.
This section is concerned with describing mechanisms by which MR can lead to bias. Bias has been defined as ‘systematic deviation of results or inferences . . . leading to results or conclusions that are systematically (as opposed to randomly) different’. 28 It is important to note that MR may or may not lead to bias in trials: the existence of MR in a trial does not necessarily mean that the intervention effect estimate is biased. We describe six scenarios in which MR may produce bias. These may seem in some ways closely related to each other, although they are conceptually distinct, with bias being produced via different mechanisms in each scenario. Being aware of the distinctions between them should help develop understanding of the nature of MR and the associated bias. The six scenarios are:
-
different measurement protocols across trial arms
-
contamination
-
interactions between measurement and intervention
-
dilution bias
-
other inadvertent intervention effects
-
effects on other forms of bias such as attrition or information bias.
Different measurement protocols across trial arms
Bias may arise when different measurement protocols are used across randomised trial arms, with one trial arm being measured more than, or differently from, another. If measurement has an impact on trial outcomes, then greater disparities in measurement protocols will produce greater bias. For example, participants in the experimental condition may be asked to complete process measures to assess mechanism, more frequent momentary assessments of behaviour or treatment response and/or ongoing measurements using technology (e.g. a digital application), whereas participants in the control condition are not asked to complete such measures. Such practices may be found widely in eHealth, mental health and other areas in which psychosocial and behaviour change interventions are evaluated. Ongoing measurements, for example for fidelity assessment or intervention development feedback purposes, carry the potential to serve as reinforcers, reminders or boosters of intervention effects, and thus can exaggerate the apparent effects of interventions. This entails bias when these measurements are not defined as part of the intervention to be assessed.
Contamination
Contamination refers to the inadvertent exposure of a non-experimental control group to intervention content that is an integral part of an effective experimental group treatment. For instance, if a pedometer were one component of a multicomponent intervention to promote walking, then its use as a research measure is intrinsically problematic because the non-intervention control group also has access to part of the intervention. If the intervention component in question is actually inert (i.e. it is not effective in producing change in measured outcomes), then bias would not result. It will often be the case that it is unknown whether or not a particular component will produce change, and so vigilance should be exercised when intervention content and outcome assessment are closely related. Similarities between the contents of research measurements and interventions also provide prima facie grounds for concern about bias being induced by contamination. This is because they may exert their effects via similar or the same mechanisms. In this situation, estimates of effectiveness are likely to be biased towards the null because both intervention and control groups are exposed to similar content.
Interactions between measurement and intervention
If MR is present, then the risk of bias needs to be considered. Research measurements and interventions, even when they are very distinct and there is no overlap in content, may exert their effects via similar mechanisms. For example, pedometers may be effective intervention tools because they produce effects by promoting self-monitoring of behaviour. Thus, research procedures other than pedometers (e.g. regular body weight weighing) that also stimulate participant self-monitoring may interfere with comparisons between randomised groups. That is, although the intervention tool (i.e. pedometers) and measurement tool (i.e. weighing) take different formats, they both may promote self-monitoring and hence the control group may be exposed to content that underpins the anticipated effect of the intervention. In this example the biasing effect will be similar to that of contamination (i.e. towards the null). There are other circumstances in which it goes in the opposite direction.
This scenario illustrates a wider point about how randomisation may not always safeguard against bias due to MR, making it difficult to distinguish true change in outcomes arising from the intervention from change due to a combination of intervention and measurement. 4 This is true even in the absence of contamination. If there are similar levels of reactivity between experimental groups in a trial, it might be considered that the true effects of interventions are safeguarded by randomisation, but this does not take into account the possibility that measurements might interact with interventions to either strengthen or weaken the observed effects and, therefore, lead to biased estimates of effect (see Appendix 2). For example, research measurement could prepare experimental group participants to be more receptive to an intervention by prompting contemplation of the reasons for behaviour change. 4 Similarly, measurement may also obstruct or diminish the means by which interventions produce their effects (e.g. if it creates or reinforces negative views towards the intervention target).
These examples suggest interaction effects between measurement and intervention on trial outcomes. When measurement invites thinking about barriers to successfully performing physical activity (e.g. resulting in problem-solving on the part of the participant) and when barriers to anticipation and problem-solving are not an intended part of the intervention, it would be surprising if this had no relevance to the effects of the intervention. Alternatively, a food diary may draw attention to the elements of a dietary intervention that are being tested in the experimental arm and, therefore, enhance the effects of intervention components. Such interactions between measurement and intervention could be widespread in the case of interventions whose effects rely on behaviour change, but this possibility has received little empirical attention.
Dilution bias
Dilution bias refers to the situation in which MR has an impact on both arms in a two-arm trial and interferes with the estimation of effect sizes through restrictions on the possible range of measured outcomes. 4 For example, there may be a finite limit to the distance walked that a walking intervention can reasonably stimulate. The more pedometers or other measurement procedures unintentionally stimulate the behaviour that is the target of the intervention, the less scope there is for the intervention to be more effective than the control. This situation will also arise for other behaviours or targets for health interventions that are susceptible to measurement reactions. Consideration of the likely maximum effects on the extent of change possible following intervention is therefore needed to appreciate this particular risk of bias. When measurement reactions account for change and there are finite limits to how much change is possible, MR may lead to dilution bias, making it less likely that intervention effects will be identified. 4
Other inadvertent intervention effects
There are both clinical and research practices associated with measurement that can lead to bias when MR is present. Sharing measurement data that are surprising or that can have an impact in other ways could prima facie be regarded as particularly likely to be produce reactions to measurement. For example, the process of collecting measurement data taken during the course of a trial may alter the care provided by health-care professionals, which may lead to bias if such alterations are implemented differently for different randomised groups. This may happen when one group of patients have more frequent contact with health-care professionals (e.g. through regular assessment of body weight, blood pressure or blood tests to assess liver function). This is a specific case of the wider class of performance bias. 29 Both experimental and control groups can be exposed to inadvertent intervention effects in this way, making it possible that the direction of the bias could go either way.
Effects on other forms of bias such as attrition or information bias
Reactions to measurement can take many forms. They can also be implicated in other well-known forms of bias in addition to those discussed above. For example, from the participant’s perspective, too much measurement can increase the burden of trial participation so that they decide to drop out. Measurement content lacking in salience can also produce such reactions. 30 In principle, there should be no bias when such effects are equivalent between randomised groups. The potential for them to interact with randomisation status becomes clearer in situations in which there are already differences in participant burden between randomised arms due to intervention exposure. When interventions are somewhat onerous and the burden quite different for control group participants, MR may be more likely to produce differential attrition.
Measurement reactivity may also be implicated in information bias, particularly when trial outcomes are self-reported and measurement leads participants, for whatever reason, to inaccurately report data about themselves. This may be a problem particularly for socially undesirable behaviours. 31 Again, for bias to be introduced to intervention effect estimates in trials, the effects of biased reporting need to be differential between randomised arms. So the question becomes ‘How likely is it that intervention content has any impact on the likelihood of biased reporting?’. When the intervention concerns the socially undesirable behaviour, this seems very likely.
Summary
In this chapter we have made a series of subtle distinctions between different forms of bias and how they may be induced by MR. In scenarios 1 and 2, it is the main effects of MR that can lead to bias, whereas in the other scenarios (i.e. scenarios 3–6) the mechanisms are more complex and involve interactions with other aspects of the study design. In scenarios 1 and 3, the effects of MR are different between randomised arms. The implication here is that MR has undermined equivalence between randomised groups. In scenarios 2 and 4, MR may lead to bias by thwarting the intended experimental contrast. In the last two scenarios (i.e. scenarios 5 and 6), MR may also generate bias through established forms of bias (e.g. performance bias) that are not usually thought of in the context of MR. Specific recommendations about how to detect MR, investigate further for its presence and deal with MR when it appears to be an important source of risk of bias is covered in the next chapter.
Chapter 3 Research informing the development of recommendations
The present research used a variety of methods to produce recommendations to minimise risk of bias from MR in trials of interventions to improve health. Specifically, we conducted (1) a series of systematic and rapid reviews, (2) a Delphi study and (3) an expert workshop to develop recommendations on how to minimise bias in trials due to MR. The study protocol has been published. 2 The present chapter describes the methods employed in each of these elements, which are summarised in Figure 1.
FIGURE 1.
Overview of research activities in the MERIT study that informed the development of the recommendations.
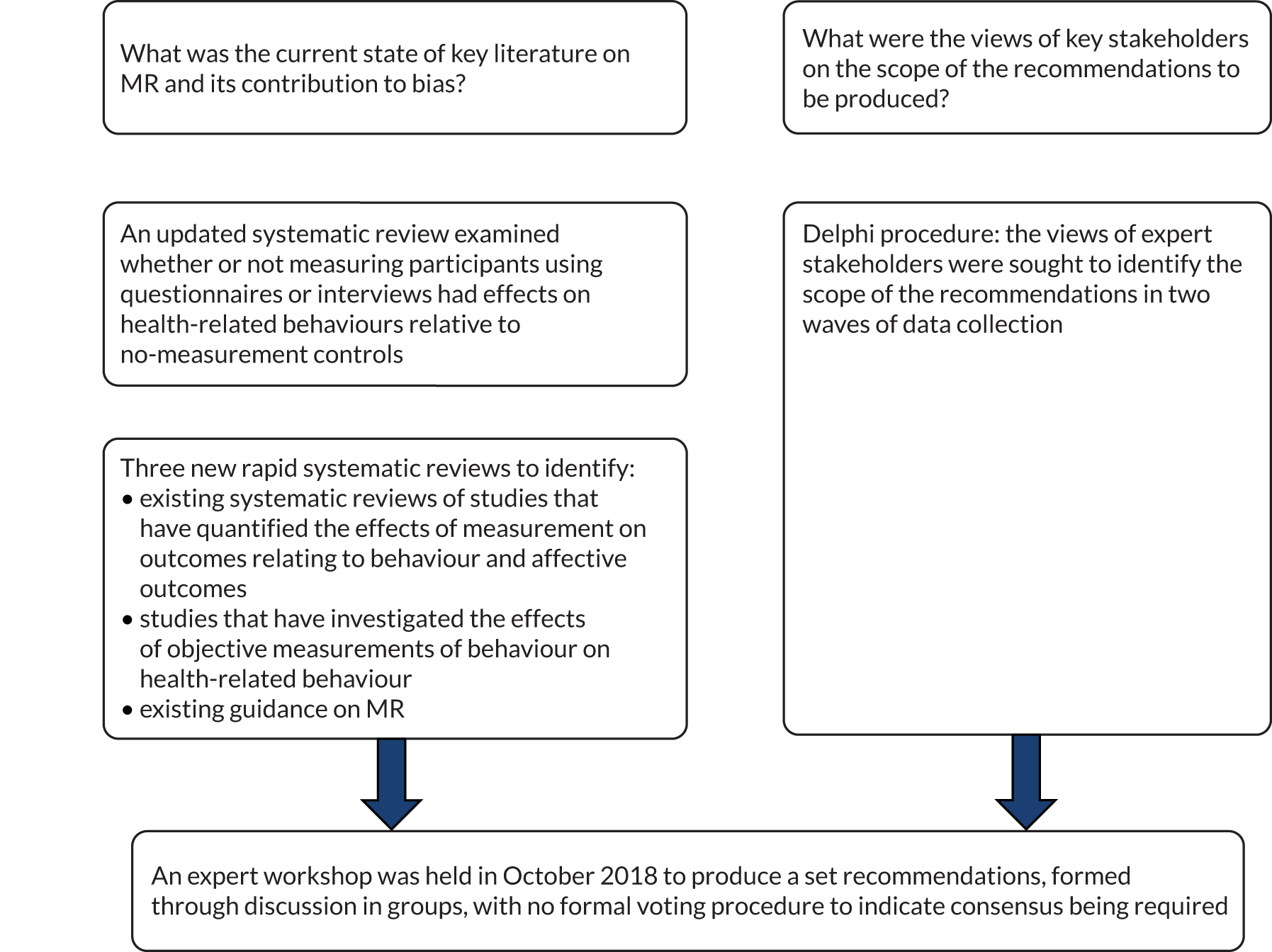
The team conducting the MERIT project was led by Professor David French (University of Manchester) and consisted of Dr Lisa Miles (University of Manchester), Professor Diana Elbourne (London School of Hygiene and Tropical Medicine), Professor Andrew Farmer (University of Oxford), Professor Martin Gulliford (King’s College London), Professor Louise Locock (University of Aberdeen), Professor Jim McCambridge (University of York) and Professor Stephen Sutton (University of Cambridge). The team was formed with the intention of providing a wide variety of expertise relevant to the formation of recommendations on this topic.
We planned to involve the public, including patients and service users, as one of the key stakeholders in the present research in the Delphi process (described in Delphi procedure to inform the scope of the recommendations). However, despite approaching a number of people who have fulfilled these roles in other research that the team have been involved in, we were not successful in recruiting anyone in the time available. It may be that the present research, which involves conducting research on the research process, is less appealing to non-specialists, even those with considerable experience in patient and public involvement.
Asking questions changes health-related behaviour: an updated systematic review and meta-analysis of randomised controlled trials
Objective
An existing systematic review on the QBE on health-related behaviours9 found that asking people questions can result in changes in behaviour. However, the overall effect was small, with many of the included studies at high risk of bias, and publication bias was also detected. A lack of pre-registration of these studies was a particular issue because many of these studies included consideration of the QBE as part of other studies, leading to a concern that, if no QBE was found, then the findings in relation to the QBE were not published. Subsequent to the search for this systematic review, which was conducted in 2012, larger studies with pre-registered protocols have been published,32,33 with generally null findings. For this reason, it seemed timely to update this systematic review to inform the MERIT study. As with the original review, this review included RCTs investigating the QBE.
Study design and methods
A systematic search for newly published trials covered January 2012 to July 2018. Eligible trials randomly allocated participants to measurement conditions, to non-measurement control conditions or to different forms of measurement conditions. Studies that reported health-related behaviour as outcomes were included and meta-analysis was performed. Subgroup analyses were conducted to assess the impact of potential prespecified moderators of the QBE and sensitivity analyses were conducted to assess whether or not there were differences in QBE on the basis of risk of bias or presence of a pre-registered protocol.
Results
Forty-three studies (33 studies from the original systematic review and 10 new studies) compared measurement with no measurement. An overall small effect was found using a random-effects model [standardised mean difference 0.06, 95% confidence interval (CI) 0.02 to 0.09; n = 104,388]. Statistical heterogeneity was substantial (I2 = 54%). In an analysis restricted to studies with a low risk of bias, the QBE remained small but significant. Sensitivity analyses indicate that there was still substantial unexplained variance, probably due to large variation in studies with respect to content of measurement, types of health-related outcomes, length of follow-up and characteristics of participants. Subgroup analyses suggested that the QBE was present for some health-related behaviours more than others. There was positive evidence of publication bias.
Conclusion
This update shows a small but significant QBE in trials with health-related outcomes, but with considerable unexplained heterogeneity. Future trials with lower risk of bias, pre-registered protocols and greater attention to blinding are needed.
Note on publication
The systematic review update on the QBE has been published. 34
Further evidence reviews to inform development of the recommendations
Three new rapid reviews were conducted to identify (1) systematic reviews of studies that have quantified the effects of measurement on outcomes relating to behaviour and affective outcomes in health and non-health contexts, (2) studies that have investigated the effects of objective measurements of behaviour on concurrent or subsequent behaviour itself and (3) existing guidance on MR.
Rapid ‘review of reviews’ of studies of measurement reactivity
This review aimed to identify existing systematic reviews of studies that have quantified the effects of measurement on outcomes relating to behaviour and affective outcomes in both health and non-health contexts to identify relevant background literature for the MERIT study.
Reviews that provide a quantitative estimate of a measurement effect are briefly described in terms of their aims, scope, methods, quality, findings and conclusions. A detailed critique of each review is not provided, but important limitations that affect the validity of the conclusions are mentioned.
Methods
The following databases were searched, limited to English-language articles published in peer-reviewed journals between 2008 and 2018 (inclusive): PsycINFO, MEDLINE, PubMed and the Cochrane Database of Systematic Reviews. Search terms were developed and tested to check that they identified reviews that were already known to the research team. The final search strategy is given in Appendix 3. Two searches were run for each database: (1) a general search to identify relevant systematic reviews and meta-analyses and (2) a more specific search for reviews and meta-analyses of the QBE or mere measurement effect. The searches were limited to the titles and abstracts of the papers in the above databases. The reference lists of identified reviews were searched manually for additional relevant reviews.
Titles and abstracts of identified records were screened by one reviewer. Full-text versions of relevant articles were obtained and screened by the same reviewer. No data extraction form was used. Relevant study characteristics and data from the final set of reviews were extracted directly to tables and text in this report. Included reviews were rated for quality by the same reviewer, using A MeaSurement Tool to Assess systematic Reviews version 2 (AMSTAR 2). 35
A search of PROSPERO using the search terms in Appendix 3 identified two reviews9,11 that had already been published, but no ongoing reviews.
Results
The searches of PsycINFO, PubMed and the Cochrane Database of Systematic Reviews yielded 1728 records. One additional record was identified from manually searching the reference lists of identified reviews. Twenty-one full-text articles were screened. Sixteen of these articles were excluded, in most cases because they did not directly address the topic of MR or they were narrative reviews or discussion papers on reactivity. 1,36–43 Several of these articles addressed reactivity of assessment of alcohol use (e.g. in the context of alcohol brief interventions). 6,36–40,42,44–52 One article37 discussed assessment reactivity in studies of interventions for intimate partner violence. However, none of these reviews reported a quantitative estimate of MR and these were therefore excluded from the present review.
A flow diagram showing the search and screening process is shown in Figure 2.
FIGURE 2.
Flow diagram of search and screening process. a, It was more efficient to screen the records for each database separately without identifying duplicates across databases.
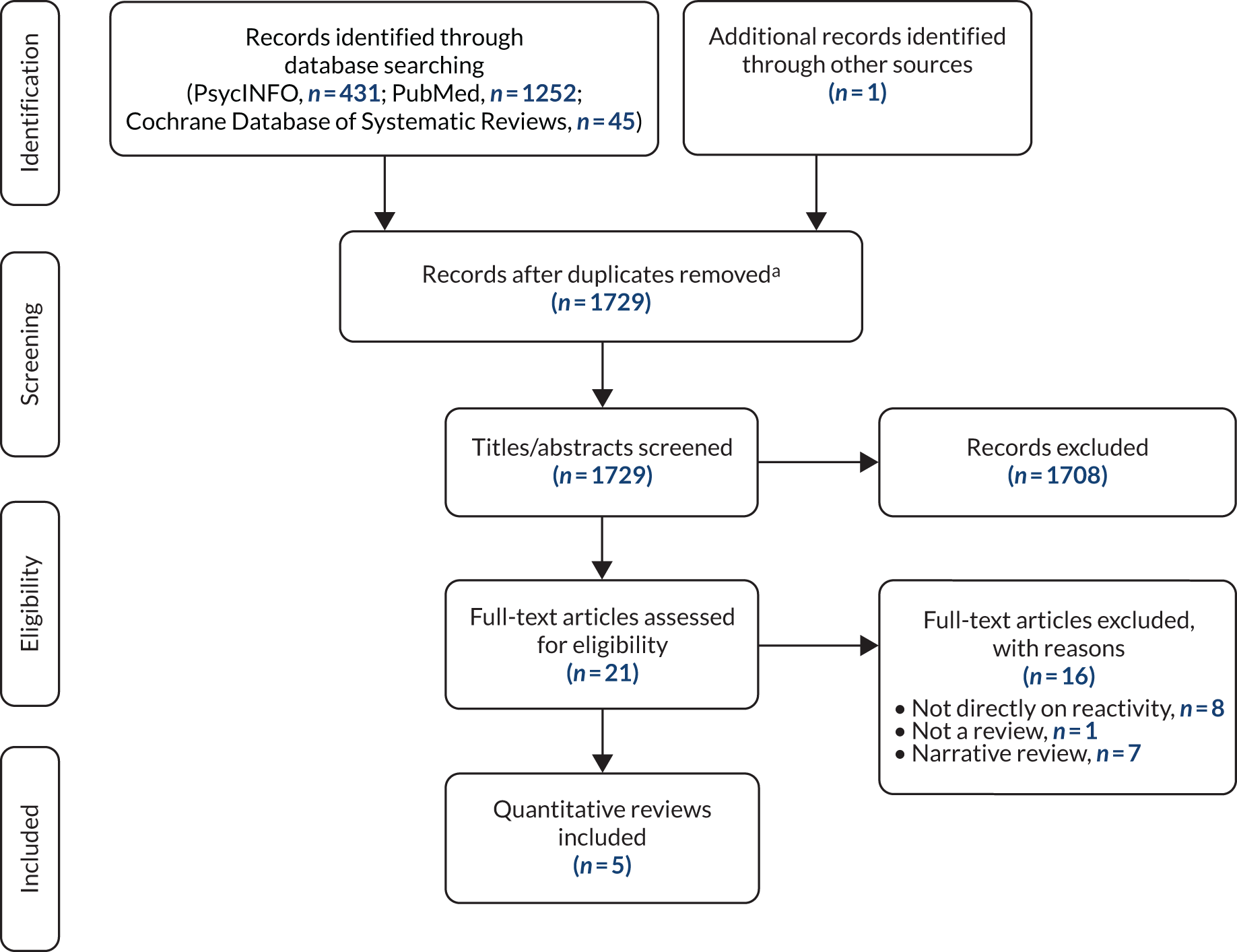
The searches failed to identify the review by McCambridge et al. 23 of evidence from Solomon four-group studies. An additional search was therefore run for reviews of studies using the Solomon design, but none was identified. The paper by McCambridge and Kypri8 was also not identified in the searches; however, the paper is clearly relevant because it includes an effect size estimate. It is therefore included in this review for the sake of completeness, although it differs in focus from the other included reviews.
The five quantitative reviews3,9–11,53 that were identified in the searches all focused on the QBE. These are described in turn. The first four reviews analysed studies that included a relevant comparison or control group (Rodrigues et al. ,9 Spangenberg et al. ,3 Wood et al. 10 and Wilding et al. 11) and the fifth (Mankarious and Kothe53) analysed prospective studies of the theory of planned behaviour (TPB). 54
The four reviews3,9–11 of the QBE that included studies with a relevant comparison or control group used broadly similar systematic review and meta-analytic methods but differed in terms of scope (type of behaviour), research designs included (RCT only vs. RCT plus non-RCT designs), type of questioning and potential moderators investigated. The headline effect size estimates are given in Table 1. The overlap between the four reviews3,9–11 in terms of included studies is shown in the Venn diagram in Appendix 4 (see Figure 4). A total of 94 studies were included in the reviews (see Appendix 4). Only nine of these studies were included in all four reviews3,9–11 and significant numbers of studies were included in only one review (e.g. 17 studies in Wilding et al. 11 and 16 studies in Spangenberg et al. 3) (see Figure 4).
Review | Number of studies | Cohen’s d | 95% CI |
---|---|---|---|
Rodrigues et al.9 | 33 | 0.09 | 0.04 to 0.13 |
Spangenberg et al.3 | 104 | 0.28 | 0.24 to 0.32 |
Wood et al.10 | 116 | 0.24 | 0.18 to 0.30 |
Wilding et al.11 | 94 | 0.15 | 0.11 to 0.19 |
Rodrigues et al.
Rodrigues et al. 9 meta-analysed data from 41 RCTs of the QBE in the domain of health-related behaviours. The authors found a small overall QBE (Cohen’s d = 0.09, 95% CI 0.04 to 0.13). Studies showed variable risk of bias and evidence of publication bias (studies with smaller or no effects were less likely to be published). No significant moderators of the effect were identified. There were no significant differences in QBE by type of behaviour, but QBEs for three behaviours (i.e. dental flossing, physical activity and screening attendance) were significantly different from zero. The authors conclude that the observed small effect size may be an overestimate of the true effect and note that in some studies participants received intervention techniques in addition to questionnaires (e.g. thank you letters). They recommend that future studies should be pre-registered.
Spangenberg et al.
Spangenberg et al. 3 synthesised findings from 104 QBE studies from 51 published and unpublished studies; all were randomised studies with a control condition in which participants responded to a neutral control question or no question. There was no restriction on the types of behaviour included. The overall weighted mean effect size (product–moment correlation) was r = 0.137 (95% CI 0.115 to 0.158; equivalent to Cohen’s d = 0.28, 95% CI 0.24 to 0.32). The authors conclude that ‘Our results clearly support that questioning people about a target behaviour is a relatively simple yet robust influence technique producing consistent, significant changes in behaviour across a wide set of behavioural domains’. 3 However, the main aim of the study was to examine a number of prespecified potential moderating variables relating to four different theoretical mechanisms (i.e. attitudes, consistency, fluency and motivations) proposed to underlie the QBE. The authors found some support for each of the four mechanisms and suggest that there may be multiple mediating processes. Significant moderator analyses showed larger effects for computer surveys (compared with paper and pencil, telephone, individual mailers and face-to-face interviews), prediction questions (compared with intentions or expectations), not specifying a time frame in the question when the question required a dichotomous response (compared with continuous or multinomial responses), behaviours related to participants’ personal welfare (compared with behaviours related to social welfare, consumption or other types of behaviours), behaviours measured by self-report, novelty of behaviour, and psychological and social risks associated with not performing the target behaviour.
Wood et al.
Wood et al. 10 meta-analysed 55 studies of the QBE. There was no restriction on the type of behaviour. Studies had to include an appropriate comparison control condition, but it is not clear if only randomised studies were included. The overall effect size from 116 tests of the QBE was a Cohen’s d of 0.24 (95% CI 0.18 to 0.30).
Like Spangenberg et al. ,3 this meta-analysis focused on potential moderators that may inform possible mediating mechanisms. Univariate moderator analyses showed larger QBEs for greater attitude accessibility; lower ease of representation; asking prediction or expectation questions (compared with mixed items or intention items only); not asking anticipated regret questions; health, consumer or other behaviours (compared with prosocial and risky or undesirable behaviours); more socially desirable behaviours; less difficult behaviours; smaller time intervals between questioning and behaviour measurement; laboratory-based studies (compared with field studies); providing an incentive to respond; and student samples (compared with mixed, unreported or non-student samples). The authors interpret these results as showing little support for any of the proposed explanations of the QBE.
Wilding et al.
In the most recent meta-analysis of the QBE, Wilding et al. 11 included 65 papers reporting 94 tests. The authors note that this is between 12 and 30 papers more than previous meta-analyses. The authors included non-RCT designs as well as RCTs. Overall, the meta-analysis yielded a small but significant effect size (Cohen’s d = 0.15, 95% CI 0.11 to 0.19).
Moderator analyses showed larger effects for student samples; laboratory settings; question type that was self-prediction or intention; specific behaviours (especially flossing, health assessment and risky driving); desirable health behaviours; behaviours not measured at baseline; studies in which baseline questioning was carried out face to face; studies that included a per-protocol analysis (compared with an intention-to-treat analysis); when the research design was a non-RCT; shorter follow-up periods; and studies at high or unclear risk of bias (compared with low).
Mankarious and Kothe
Mankarious and Kothe53 conducted a meta-analysis of 66 TPB studies that measured health behaviours at two or more time points. These were prospective observational studies and not RCTs, as in the other quantitative reviews of the QBE. They calculated Cohen’s d to estimate the standardised mean difference for behaviour from baseline to the first follow-up measurement for each study. The average change in behaviour from baseline to follow-up across all studies was small and negative (Cohen’s d = –0.03, 95% CI –0.04 to 0.11). Length of follow-up was a significant moderator in that the change in behaviour from baseline to follow-up increased as the length of follow-up increased. Behaviour type was also a significant moderator in that socially desirable behaviours showed a small increase from baseline to follow-up, whereas socially undesirable behaviours showed a small but significant decrease. Subgroup analyses showed significant decreases in binge drinking, risky driving, sugar snack consumption and sun-protective behaviour.
The authors conclude that ‘Measurement of intention at baseline resulted in significant decreases in undesirable behaviours. Changes in undesirable behaviours reported in other studies may be the result of the mere measurement effect’. 53 However, there are several problems with this interpretation. The included studies measured all the TPB constructs at baseline and so it is difficult to attribute any mere measurement effect to the measurement of intention specifically. Although the authors argue that by selecting prospective studies research participant effects other than mere measurement can be ruled out, it is not clear that this is the case. For example, observed changes in behaviour could result from social desirability effects. It is also not clear whether the observed changes represent real changes in behaviour or simply changes in reporting.
The reviews varied in quality. AMSTAR 2 total scores (calculated by assigning 1 point for ‘yes’ and 0.5 points for ‘partial yes’, with a maximum of 16 points) ranged from moderate (i.e. 8.5 points for Rodrigues et al. 9 and 9 points for Wilding et al. 11) to low (i.e. 3 points for Spangenberg et al. 3 and 3.5 points for Wood et al. 10). Although the checklist is not designed to generate a total score, the score nevertheless gives an overall indication of quality.
McCambridge and Kypri
McCambridge and Kypri8 included eight trials of the effect of answering questions on alcohol drinking behaviour. Between-group differences were 13.7 (95% CI –0.17 to 27.6) grams of alcohol per week and 1 (95% CI 0.1 to 1.9) point on the Alcohol Use Disorders Identification Test score. Therefore, answering questions on drinking in brief intervention trials appears to alter subsequent self-reported behaviour.
Discussion
The four recent reviews3,9–11 of the QBE that included studies with a comparison or control condition yielded similar small, positive effect size estimates, ranging from 0.09 to 0.28 (Cohen’s d). These should not be considered to be completely independent estimates because of the overlap in included studies. However, the overlap was less than might be expected. The reviews come to different conclusions about the practical significance of these findings. For example, Wood et al. 10 state that ‘Within the health domain, a large number of studies have demonstrated that the QBE can be harnessed as an effective intervention . . .’. 10 By contrast, Rodrigues et al. 9 suggested that the observed effect size could overestimate the true effect size and that future studies should compare the QBE with simply sending reminders to perform the behaviour (see also the commentary by Rodrigues et al. 14).
The existing findings for moderators could be used to identify conditions under which a small or zero QBE could be expected, which would enable investigators to minimise the QBE. However, the findings for moderating variables are relatively weak. They are based on correlational evidence (i.e. study characteristics that are associated with larger or smaller effect sizes for the QBE) and the moderators are frequently correlated with each other. In some cases, the moderators were assessed only indirectly. For example, Wood et al. 10 assessed the potential moderator ‘attitude accessibility’ for each included study by multiplying an independent rating (by the review team) of attitude for the target behaviour and sample by the response rate to the questionnaire. The findings are often based on a small subset of studies and statistical significance is often close to 0.05. In many cases the results need to be replicated.
Rapid review of studies of reactivity to objective measurement of behaviour
In research on health behaviours, self-report measures of behaviour are ubiquitous. Such measures have well-known limitations (e.g. social desirability bias), and it is common to see recommendations for researchers to use so-called objective measures of behaviour that are assumed to have fewer limitations. However, objective measures may have their own limitations. For example, objective measures may have reactive effects on behaviour (e.g. measuring behaviour objectively may lead to increases in that behaviour).
To identify relevant background literature for the MERIT study this review aimed to identify studies that have examined the possible reactive effects of objective measurement of behaviour. The review included the following health-related behaviours: physical activity, diet/food choice, smoking, alcohol and drug use, dental behaviours and medication adherence.
The following databases were searched, limited to English-language articles published in peer-reviewed journals between 2008 and 2018 (inclusive): PsycINFO, MEDLINE and PubMed. Searches were limited to the titles and abstracts of the papers. The reference lists of identified papers were searched manually for additional relevant papers. Titles and abstracts were screened by one reviewer. Full-text versions of relevant articles were obtained and screened by the same reviewer.
Fourteen articles13,55–67 on physical activity and two papers68,69 on medication adherence were included in the review. No studies of smoking, alcohol, drugs, diet or dental behaviours were included.
Evidence of reactivity was found in some physical activity studies but not in others. Based on studies that used experimental research designs, the following broad conclusion for practice can be made:
-
If the aim is to measure physical activity, rather than to increase it, do not ask participants to use an unsealed pedometer (i.e. a pedometer that discloses step counts to the participant) and to record their steps. Instead, use either an accelerometer or a sealed pedometer (i.e. a pedometer that does not disclose step counts to the participant) and exclude data from the first few days of use.
The two studies68,69 of reactivity to objective measurement of medication adherence using electronic containers suggest that objective measurement may increase adherence but that the effect is temporary and/or relatively small and so can be ignored, particularly if a run-in period is used (i.e. a period of monitoring from which adherence data are discarded).
This review shows clearly that more work is needed on the possible reactive effects of objective measurement. Future work should consider using experimental designs rather than simply longitudinal studies of objective measurement, the findings from which are difficult to interpret. Experimental designs can be used to test whether or not there is a ‘main effect’ of objective measurement and to estimate the size of this effect. They can also be used to isolate key components of measurement that may account for the reactive effect (e.g. feedback of behavioural information to the participant via a display of steps on a pedometer as opposed to simply wearing a pedometer with no feedback).
With developments in technology and increasing awareness of the limitations of self-report measures of behaviour there is likely to be increasing use of objective measurement of behaviours in health behaviour research.
Existing guidance on measurement reactivity
The aim of the third rapid review was to identify existing guidance statements or recommendations on how to reduce bias from MR in trials.
A search was conducted of all CONSORT statements/papers and Medical Research Council (MRC) framework/guidance on complex interventions (all versions as well as of MRC guidance on process evaluation in trials. Each document was reviewed for any relevant content related to guidance on reducing the risk of bias from MR in trials. Furthermore, the full texts of the studies included in the two rapid reviews discussed in Rapid ‘review of reviews’ of studies of measurement reactivity and Rapid review of studies of reactivity to objective measurement of behaviour were checked for any reference to existing guidance on MR. Members of the MERIT study team were also consulted to find out if they were aware of any existing guidance or recommendations related to MR.
We were not able to identify any existing guidance statements or recommendations on how to reduce bias from MR in trials from any of these sources. To the best of our knowledge, the present document is the first to present recommendations on how to reduce bias from MR in trials.
Delphi procedure to inform the scope of the recommendations
The MERIT study included a Delphi procedure70 to explore and, as far as possible, combine the views of experts to reach agreement on the precise issues that the recommendations will cover (i.e. the scope of the recommendations). The objectives of the Delphi procedure were to:
-
seek expert opinion from stakeholders on the specific topics where recommendations on MR are needed and likely to produce the largest benefits
-
identify key background literature and expertise on MR.
Methods
Delphi participants were purposively recruited and identified by examining authorship of relevant studies as well as using knowledge within the multidisciplinary research team of people with relevant expertise. The aim was to identify individuals with wide-ranging expertise relating to MR, trial design, conduct and analysis as well as to identify individuals who are likely to be key users of the final recommendations, including those involved in research synthesis and funding (see Acknowledgements).
Participants were asked to complete two rounds of an online questionnaire over a period of approximately 12 weeks from May 2018. The first round of the Delphi procedure involved 15 open-ended questions, allowing participants to share their views on what sorts of bias can arise from MR, the mechanisms by which measurement produces changes in people, and the characteristics of study design, interventions, measurement and context that can lead to such biases. Suggestions were also sought on key literature on MR.
Responses from round 1 of the Delphi were developed into themes that were then used to inform the round 2 questions. Participants from round 1 of the Delphi were asked to complete round 2. The second round of the Delphi presented participants with a list of specific topics that recommendations might consider. Participants were asked to rate their agreement with these suggested topics as well as to provide open-ended comments if they thought that any key issues were missing.
Results
A total of 40 participants took part in round 1 of the Delphi procedure (119 invitations were sent in total), covering a wide range of expertise, as shown in Table 2. Among these, 31 (78%) participants took part in the second round. The findings of the Delphi procedure were then provided to delegates at an expert workshop (see Expert workshop to develop content of the recommendations).
Expertise | Number of Delphi round 1 respondents |
---|---|
Evidence synthesis | 17 |
Trial conduct | 16 |
Health psychology/behaviour change | 12 |
Public health/epidemiology | 11 |
Qualitative/mixed methods | 11 |
Trial statistics | 7 |
Sociology | 7 |
Measurement methods | 7 |
MR | 6 |
eHealth | 6 |
Research funding | 5 |
Ecological momentary assessment | 3 |
Unknown | 2 |
Health economics | 1 |
Lay/patient | 1 |
The results of round 2 of the Delphi process are shown in Table 3. Each specific topic for inclusion was categorised into a subgroup topic (see Table 3, last column). These subgroup topics formed major components of the agenda at the expert workshop held in October 2018. Participants were provided with the ratings in Table 3 to help inform discussion.
Topic | Response, % (n) [points] | Response total | Points | Average | Subgroup | ||||
---|---|---|---|---|---|---|---|---|---|
Not at all important (1 point) | Somewhat important (2 points) | Moderately important (3 points) | Very important (4 points) | Extremely important (5 points) | |||||
Strategies for carefully designing trials to reduce the risk of bias due to MR | 0 (0) [0] | 3.23 (1) [2] | 3.23 (1) [3] | 38.71 (12) (48] | 54.84 (17) [85] | 31 | 138 | 4.45 | Study design |
How to predict when MR could lead to bias in a trial | 0 (0) [0] | 0 (0) [0] | 9.68 (3) [9] | 38.71 (12) [48] | 51.61 (16) [80] | 31 | 137 | 4.42 | Bias/appraisal |
Approaches to ensure measurement/assessments are not confounded with the intervention | 0 (0) [0] | 3.33 (1) [2] | 13.33 (4) [12] | 40 (12) [48] | 43.33 (13) [65] | 30 | 127 | 4.23 | Study design |
How to anticipate when MR is likely to be present in a trial | 0 (0) [0] | 0 (0) [0] | 12.9 (4) [12] | 58.06 (18) [72] | 29.03 (9) [45] | 31 | 129 | 4.16 | Study design/appraisal |
How to identify risk of bias due to MR in existing trials | 0 (0) [0] | 3.23 (1) [2] | 6.45 (2) [6] | 61.29 (19) [76] | 29.03 (9) [45] | 31 | 129 | 4.16 | Appraisal |
How to interpret trials that are at risk of bias due to MR | 0 (0) [0] | 0 (0) [0] | 16.13 (5) [15] | 51.61 (16) [64] | 32.26 (10) [50] | 31 | 129 | 4.16 | Appraisal |
How to identify the types of bias arising from MR | 0 (0) [0] | 0 (0) [0] | 23.33 (7) [21] | 50 (15) [60] | 26.67 (8) [40] | 30 | 121 | 4.03 | Bias |
Considerations in selecting measurement tools (e.g. objective vs. subjective) to reduce the risk of bias due to MR | 0 (0) [0] | 6.45 (2) [4] | 22.58 (7) [21] | 38.71 (12) [48] | 32.26 (10) [50] | 31 | 123 | 3.97 | Measures |
Recommendations on how unobtrusive methods of data collection could be used to remove or reduce the risk of bias due to MR | 0 (0) [0] | 9.68 (3) [6] | 16.13 (5) [15] | 41.94 (13) [52] | 32.26 (10) [50] | 31 | 123 | 3.97 | Measures |
Considerations in planning the timing and number of repeated measurements to reduce the risk of MR | 0 (0) [0] | 3.23 (1) [2] | 25.81 (8) [24] | 45.16 (14) [56] | 25.81 (8) [40] | 31 | 122 | 3.94 | Study design |
Provision of hypothetical/existing study examples to illustrate principles behind guidelines | 0 (0) [0] | 3.23 (1) [2] | 29.03 (9) [27] | 41.94 (13) [52] | 25.81 (8) [40] | 31 | 121 | 3.9 | All |
Strategies for statistical analyses of trial outcome data that aim to estimate, and adjust for, the risk of bias due to MR | 0 (0) [0] | 6.67 (2) [4] | 30 (9) [27] | 33.33 (10) [40] | 30 (9) [45] | 30 | 116 | 3.87 | Analysis |
Strategies to improve use of self-report measures to reduce the risk of MR | 0 (0) [0] | 6.45 (2) [4] | 29.03 (9) [27] | 35.48 (11) [44] | 29.03 (9) [45] | 31 | 120 | 3.87 | Measures/trial conduct |
Considerations in undertaking pilot studies to identify potential measurement reactions and how they may be addressed | 0 (0) [0] | 9.68 (3) [6] | 22.58 (7) [21] | 48.39 (15) [60] | 19.35 (6) [30] | 31 | 117 | 3.77 | Study design |
How to conceal measurements from participants or limit feedback as a way to reduce the risk of MR | 0 (0) [0] | 19.35 (6) [12] | 16.13 (5) [15] | 32.26 (10) [40] | 32.26 (10) [50] | 31 | 117 | 3.77 | Measures/trial conduct |
Recommendations on how the research team should interact with research participants to reduce the risk of MR | 0 (0) [0] | 3.33 (1) [2] | 30 (9) [27] | 53.33 (16) [64] | 13.33 (4) [20] | 30 | 113 | 3.77 | Trial conduct |
Strategies for handling risk of bias when a study’s aims require different measurement procedures across arms of a trial | 0 (0) [0] | 6.45 (2) [4] | 35.48 (11) [33] | 35.48 (11) [44] | 22.58 (7) [35] | 31 | 116 | 3.74 | Analysis |
The circumstances in which one might use non-standard trial designs (e.g. Solomon four-group designs) to assess extent of bias and/or yield unbiased estimates of effects | 0 (0) [0] | 6.45 (2) [4] | 38.71 (12) [36] | 32.26 (10) [40] | 22.58 (7) [35] | 31 | 115 | 3.71 | Study design |
Identification of gaps in knowledge on MR and how to minimise risk of bias in trials due to MR | 0 (0) [0] | 19.35 (6) [12] | 19.35 (6) [18] | 35.48 (11) [44] | 25.81 (8) [40] | 31 | 114 | 3.68 | All |
Identification of research priorities for better understanding of MR and potential for bias | 3.23 (1) [1] | 16.13 (5) [10] | 19.35 (6) [18] | 35.48 (11) [44] | 25.81 (8) [40] | 31 | 113 | 3.65 | All |
Which fields of research are most affected by bias due to MR | 0 (0) [0] | 12.9 (4) [8] | 29.03 (9) [27] | 45.16 (14) [56] | 12.9 (4) [20] | 31 | 111 | 3.58 | Bias/appraisal |
How to assess extent of MR during an internal pilot phase of a trial | 0 (0) [0] | 16.13 (5) [10] | 25.81 (8) [24] | 41.94 (13) [52] | 16.13 (5) [25] | 31 | 111 | 3.58 | Analysis/trial conduct |
Ethical implications of strategies to address MR | 6.45 (2) [2] | 16.13 (5) [10] | 25.81 (8) [24] | 32.26 (10) [40] | 19.35 (6) [30] | 31 | 106 | 3.42 | All |
How biases caused by MR might relate to existing risk-of-bias frameworks | 0 (0) [0] | 12.9 (4) [8] | 45.16 (14) [42] | 35.48 (11) [44] | 6.45 (2) [10] | 31 | 104 | 3.35 | Bias |
Theoretical explanations that may plausibly explain the effects of measurement on people who have been measured (mechanisms) | 3.23 (1) [1] | 22.58 (7) [14] | 32.26 (10) [30] | 29.03 (9) [36] | 12.9 (4) [20] | 31 | 101 | 3.26 | Mechanisms |
Recommendations on selection of research participants (recruitment strategies and inclusion criteria) to reduce the risk of MR | 6.45 (2) [2] | 32.26 (10) [20] | 32.26 (10) [30] | 16.13 (5) [20] | 12.9 (4) [20] | 31 | 92 | 2.97 | Trial conduct |
How research participants may make use of measurement for their own purposes (which may lead to bias) | 0 (0) [0] | 43.33 (13) [26] | 30 (9) [27] | 16.67 (5) [20] | 10 (3) [15] | 30 | 88 | 2.93 | Trial conduct |
Total number of respondents (for this survey round) | 31 | ||||||||
Total number of responses | 832 | ||||||||
Point average (total points all rows/responses for all rows) | 3.79 | ||||||||
Point weighted average (total points all rows/responses for all rows) | 3.79 |
Expert workshop to develop content of the recommendations
An expert workshop was held in Manchester on 4 and 5 October 2018. A total of 23 delegates attended the workshop. The delegates covered a broad range of expertise similar to that covered by the Delphi procedure (see Acknowledgements). Delegates were provided with reports of the evidence reviews (see Asking questions changes health-related behaviour: an updated systematic review and meta-analysis of randomised controlled trials, Rapid ‘review of reviews’ of studies of measurement reactivity, Rapid review of studies of reactivity to objective measurement of behaviour and Existing guidance on measurement reactivity) and the results of the Delphi procedure (see Delphi procedure to inform the scope of the recommendations) and were encouraged to refer to these as a basis for further discussions. The content of appropriate recommendations was discussed by the workshop delegates and these statements form the central part of the current recommendations. As informed by the Delphi procedure, discussions were conducted in subgroup and plenary sessions and structured around study design and bias, measurement procedures, appraisal of existing trials, trial conduct and statistical analysis.
The MERIT study team have written the current report based on notes of the workshop discussions. All workshop delegates were given opportunity to review and comment on the report and recommendations before it was finalised.
A notable limitation of the MERIT study was the shortage of high-quality evidence regarding the extent of MR (with some notable exceptions) and the circumstances that are likely to bring it about. There is a particular lack of direct evidence regarding the extent to which MR produces bias in trials. Accordingly, development of the final recommendations has relied extensively on indirect evidence processed by expert opinion, which is contingent on reasonable inference regarding the likely consequences of measurement in producing bias.
Chapter 4 Recommendations
Parts of this chapter have been reproduced with permission from French et al. 27 This is an Open Access article distributed in accordance with the terms of the Creative Commons Attribution (CC BY 4.0) license, which permits others to distribute, remix, adapt and build upon this work, for commercial use, provided the original work is properly cited. See: https://creativecommons.org/licenses/by/4.0/. The text below includes minor additions and formatting changes to the original text.
The present chapter makes a series of recommendations for people designing and conducting trials. In developing these recommendations, a limitation is the current state of knowledge. There is some evidence regarding the circumstances under which measurement will lead to reactivity, but little direct evidence about the extent to which it causes bias, let alone the effectiveness of any steps that could be taken to reduce bias. 2 Given this, many of these statements are broad recommendations about issues that may be useful to consider on the basis of indirect evidence processed by expert opinion.
The recommendations are grouped into three broad types of recommendations: (1) identify whether or not MR is likely to be a major source of bias for a new trial, (2) collect further data to inform decisions about whether or not there is risk of bias resulting from MR and (3) potential actions to minimise risk of bias from MR within a trial.
Identify whether or not measurement reactivity is likely to be a major source of bias for a new trial
It is worth noting that, in some cases, the risk of bias from MR in a particular trial may be so small that it can safely be ignored. Consideration of the ways in which MR may lead to bias, as well as other potential sources of bias, and how bias can be prevented lies at the heart of rigorous approaches to trial design and conduct. No triallist can discount selection bias or other forms of bias without properly accounting for the risk in the specific circumstances of their trial. 25 In many circumstances, although bias from MR may be present, it is likely to be of small magnitude compared with other sources of bias, such as failure of randomisation. 9 It may also be impossible to isolate bias from MR if there are other sources of bias. The features listed in Table 4 suggest circumstances in which MR bias may be more important. It is, however, worth considering that reactions to assessment can exacerbate or contribute to other sources of bias that have previously received more attention. 7 As discussed in Chapter 2, reactions to measurement can be implicated in several well-known forms of bias.
Criterion indicating risk of bias | Circumstances under which risk of bias is likely to be higher |
---|---|
Participant selection | |
Recruitment | Selection on personal motivation for participation in the trial |
Eligibility criteria | Restrictive eligibility criteria |
Education | More educated (e.g. university students) |
Measurements | |
Features of health outcome of interest | |
Participant awareness of health-related outcome of interest | Participants aware of outcome of interest (open) |
Nature of health-related outcomes | Outcomes focused on behaviour or anxiety; health-promoting behaviours (e.g. physical activity) |
Social desirability of health outcome | Outcomes with well-recognised social norms (e.g. body weight) |
Follow-up | |
Number of measurement occasions | Measurements repeated on several occasions |
Length of time to follow up | Short in relation to possible measurement effects |
Features of measurement procedures/tools | |
Equivalence of measurement procedures across trial arms | Differential across trial arms |
Similarity between measurement and BCTs | Measurement directly mimics BCTs |
Source of data | New data collected specifically for this study |
Measurements open to subjectivity | Self-report measures |
Disclosure of measured values to participants | Values disclosed to participants (immediately) |
Burden of measurement task | Onerous for participant |
Complexity of measurement task | Complex for participant |
Measurement framed in terms of goals/targets | Participants measured against specific goals/targets |
Context | Laboratory setting |
Interventions and comparators | |
Nature of the intervention | Behavioural and/or self-monitoring components included |
Blinding to arm allocation | Lack of blinding to arm allocation |
Process evaluation | |
Process measures | Measures included are assessing mechanisms of action on the primary outcome |
Timing | Conducted before/during trial outcome assessments |
Trial arms included | Conducted in only one trial arm |
Number of data collected | Extensive data collected from all participants |
Recommendation 1: consider potential for measurement reactivity causing bias at the design stage of a trial
It will be easier to prevent MR causing bias than it will be to deal with the consequences of bias through analysis after the event. Therefore, researchers should consider at the outset whether or not the trial they are planning is likely to produce this bias. It is important to consider the many measurement and assessment processes involved in a trial. This may include assessment of eligibility, baseline assessments, assessments of adherence or fidelity, process evaluations (quantitative and qualitative) and interim/final outcome assessments. Each of these measurement or assessment processes has the potential for causing MR and thereby introduces the potential for bias.
It is also important to be clear when measurement is an integral part of the intervention and, hence, should not be considered a source of bias per se, although there may be contamination issues to consider carefully. In many studies ongoing measurement may be part of an intervention (i.e. it would be part of the intervention were it to be rolled out in practice outside a trial). For instance, many weight management programmes may include regular measurement of body weight as an integral part of the intervention. 71 In this case, although regular weighing would be carried out only in the intervention group and not in the control group, any reactions to this measurement would not constitute bias (i.e. the reactions are due to an integral part of the intervention). By contrast, if such regular weighing were not part of the programme were it to be rolled out, then the assessments would be a feature of the trial design rather than the intervention design, and this imbalance between experimental arms has the potential to produce bias. For these reasons, it makes sense to have a single clear purpose for each measurement.
Recommendation 2: consider potential for measurement reactivity as a source of bias throughout the research process
As one moves from early trial planning through detailed study design to giving attention to issues arising from study conduct, it is important to consider all instances of measurement that can occur throughout the research process. For instance, when assessing eligibility of a potential participant for a trial, disclosure of health status (e.g. blood pressure or cholesterol level) to research participants at the beginning of a clinical trial could potentially lead to measurement reactions. For example, in a trial evaluating the effect of a behavioural intervention compared with usual care, disclosure of health status to participants could motivate the comparison group to seek additional support. Participants’ knowledge of their health status could also make them more or less receptive to an intervention. 72 This might be particularly problematic when subgroups of the population with a particular health risk are recruited to take part in a trial (e.g. people with obesity or people who drink alcohol heavily). 8 It is not difficult to imagine how, by simply engaging in the measurements required to assess eligibility into the trial, participants become aware of their health status and might change their thoughts, emotions or behaviour as a result. In such instances, there is a need to be careful around communications with participants regarding how measurements are used.
At the consent stage participants are often informed of the trial objectives through the patient information sheet as well as through possible informal interactions with trial personnel. Participants may then perceive specific behavioural trial outcomes to be implied norms or goals in the context of the research. This may predispose to MR, which may introduce bias subsequent to randomisation. 4 Patient information sheets should be carefully drafted so as to emphasise the concept of equipoise and the equal value attached to alternative trial interventions and outcomes. Patients may be asked to consent to masking of measurements and non-disclosure of measurement values (see Recommendation 13: consider the potential benefits of masking measures and/or withholding feedback of measured values against ethical considerations). Trial personnel should follow standard operating procedures (SOPs) that regulate informal communications with participants at the recruitment/consent stage.
In some trials, for example cluster RCTs73 and others based on routine health records,32,33 consent may not always be required at the individual participant level. Consent at general practice level, for example, enables trials to be conducted with lower levels of awareness among patients of research participation. Gaining consent at a group level in this way can help to avoid participants’ awareness of the health outcome of interest for the trial and the potential for MR to take place based on this knowledge. However, MR alone is unlikely to be a main justification for choice of a cluster randomised design.
Baseline measurements in a trial typically contribute to efficient design by enabling more precise estimation of the intervention effect. 74 However, when trial participants are exposed to baseline measurements this may contribute to MR and heighten risk of bias. This is because experiences of a previous measurement within a trial may influence responses at later measurement occasions and/or interact with the study intervention. These testing effects may differ according to subsequent trial arm allocation.
It is generally recognised that recording the delivery of face-to-face interventions results in greater adherence to intervention protocols, which can be problematic when fidelity assessments are taking place. 75 This is probably unproblematic in efficacy or ‘proof of principle’ studies, in which high levels of adherence allow easier interpretation of whether or not an intervention delivered as intended demonstrates efficacy. 76 However, in effectiveness studies, in which one is aiming to examine the effects of an intervention delivered in more ‘real-world’ settings and in which such fidelity assessments would not be enacted in routine implementation, any reactions to these assessments form an example of MR, as discussed in the present document. When the fidelity assessments are enacted in routine implementation (e.g. as part of quality assurance processes), they can be considered to be part of an intervention and, hence, any reactions to these assessments are not problematic. Assuming that adherence to the intervention protocols is not part of future practice (assuming the intervention is successful) and is likely to result in greater effects, then the use of fidelity assessments is likely to result in bias through overestimation of intervention effects (see Chapter 2).
It is good practice to draw up SOPs for the measurement procedures that encompass issues noted in this report, including consistency of measurement procedures across trial arms, masking and non-disclosure of measurement values, number of measurement occasions, etc. The SOPs should extend to regulating informal contacts/communications between trial participants and health-care providers or trial personnel either at the time measurements are taken or on other occasions.
The prospect of future measurement may also produce changes in research participants. For example, anticipatation of measurement of body weight can lead to changes in feelings of self-efficacy and self-control, as well as increased accountability for one’s own actions,77 which could potentially affect adherence to physical activity and healthy eating guidance, particularly shortly before such measurements. Similarly, electronic monitoring of medication adherence can lead to changes in adherence. 69 That is, knowledge or anticipation of measurement or disclosure of outcomes, as well as actual measurements conducted, should be considered as potential sources of reactivity.
Recommendation 3: consider specific trial features that may indicate heightened risk of bias due to measurement reactivity
Table 4 provides a series of ‘red flags’ or trial features that might indicate that MR should be considered a possible risk of bias in a study. Based on the consensus views of experts consulted for this report, it highlights features of study participants, types of interventions, features of study design and measurement issues where MR may be more likely to occur and lead to bias. Table 4 should be consulted alongside the explanatory text in Appendix 5. When direct evidence is available to support entries in the table, then this is cited in Appendix 5; otherwise, the entries are based on consensus views of experts consulted as part of the MERIT study (see Chapter 3 for a description of the process used to develop recommendations).
The entries in Table 4, or ‘red flag’ features of study design, indicate only potential for bias from MR, which may be absent on closer examination or identified as a possibility and mitigated through careful study design. The potential for measurement as a co-intervention leading to bias is implicit but not widely articulated in existing tools intended to assist in study design. 78 Table 4 provides a checklist to identify such concerns. Researchers should refer to Table 4, and associated text in Appendix 5, to determine whether or not MR and risk of bias arising from this is likely to be particularly relevant to their particular study and, if so, should further consider the following recommendations about reducing the potential for bias. Some key issues contained in Table 4 are illustrated in worked examples contained in Boxes 3 and 4.
We describe below a hypothetical trial in which there is a high likelihood of bias due to MR to illustrate the issues highlighted in Table 4. Please note that this example includes several features of poor trial design. This is deliberate to illustrate issues that are explicitly related to ‘red flags’ in Table 4.
A trial is designed to assess the effectiveness of an intervention to promote maintenance of weight loss in people with type 2 diabetes who have lost 5 kg, relative to usual care. The intervention is designed to be delivered entirely online and involves self-monitoring of body weight, physical activity and dietary behaviours as well as encouragement to seek social support for maintenance of weight loss. To assess the intervention’s mechanism of effect, 25% of participants in the intervention group are required to engage in a process evaluation. This involves patients attending their general practices to be weighed. These participants are also asked to complete questionnaires about their dietary and physical activity behaviours online. A different subsample is asked to participate in focus groups to discuss how useful they found the online intervention.
This trial has numerous features that suggest it is likely to produce a biased outcome due to MR. First, the intervention is a fairly minimal contact digital intervention and so any effect of this intervention on body weight is likely to be small. Therefore, the effects of MR do not have to be large to have a relatively substantial effect. Second, all participants are likely to be motivated to achieve the outcome, given that they have already lost 5 kg in body weight, and so they are likely to be very interested in the results of measurements made. Third, there is unbalanced measurement, with those in the intervention group receiving substantially more process measurement (i.e. attendance at general practices involves a quite burdensome form of measurement). Fourth, the process evaluation mimics some of the intended effects of the intervention because it involves (1) regular weighing, (2) regular reflection on behaviour through completion of questionnaires and (3) contact with other people who could provide social support. Fifth, because the study is not blinded, the staff at the general practices will be aware that the intervention participants are trying to maintain weight loss and may offer encouragement or other advice. The mere fact that body weight will be monitored by others may promote attempts to maintain weight loss. Last, the outcomes of measurement are likely to be available to intervention participants and so they may be receiving more information than participants in the other experimental condition.
This box considers what actions could reasonably be taken to address the high likelihood of risk of bias of the hypothetical trial described in Box 3. Please note that, even in the absence of the onerous process evaluation, the evaluation of this particular intervention in a trial invokes the risk of measurement reactions introducing bias because measurement is intrinsic to both intervention and trial design. The selection criteria create risks to be considered because they seek participants who have already demonstrated that they are motivated volunteers and they may have varying strengths of preferences for allocation. Communications with trial participants from the information sheet onwards need to be carefully constructed throughout the study to provide assurance about the equal value attached to both trial arms.
The process evaluation makes measurement differential between arms and so a strong justification is required, which makes the risks of bias worth considering in relation to other considerations. To inform this process of decision-making it would be helpful to elaborate a programme theory of how this intervention seeks to produce the weight loss maintenance outcome. This could be useful because it will clarify thinking about how measurement and other features of study design may operate in relation to the possible mechanisms of effect.
Some amendments to the study design or conduct may appear warranted when using the programme theory. For example, this may identify that the hypothesised mechanism of action for the intervention is similar to the unwanted effects of the process evaluation. This might lead the researchers to consider reducing the amount of measurement in the intervention arm, using similar measures with the control group or, perhaps better still, minimising the effects of the process evaluation. For example, the timing of focus groups should be delayed until after the primary outcome is assessed. The researchers may also decide to train administrative, clinical and research personnel in how best to interact with trial participants (e.g. regarding communication of weight measurements or the parameters of informal chat). There could be a trade-off in making the measurement experience as positive as possible to aid trial retention, with the risks that it may orientate participants to weight loss issues in ways that affect key behaviours. In many situations it will be acceptable to tolerate measurement reactions if retention is optimised, although in such circumstances capturing the extent of measurement reactions to assess their equivalence will often be worthwhile.
In this trial the participants are very likely to have been engaged with the weight management issues that are being measured for many years. Appreciation of how the participants regard the trial eligibility and subsequent measurement procedures is likely to be valuable. For example, some participants might be expected to use the annual measurement points as providing deadlines or targets in ongoing struggles with their own body weight. Indeed, in this study it is clear to all which outcomes are being studied. Therefore, designing a study to try to avoid participants using measurements for their own purposes may not be appropriate. Instead, it may be more appropriate to seek to study this phenomenon. If participants in both arms use the measurements in similar ways then bias is unlikely. For these reasons, the research team might undertake one of a range of feasibility studies before the trial. The team could interview participants after administering the planned baseline and/or process measurement procedures with a view to identifying content that is particularly salient to ongoing weight management. If this is done for both control and intervention arms that differ in terms of extent of measurement, it will provide data on possible differences between arms due to unbalanced measurement. These findings should then inform decisions about the content, timing and procedures for the main trial.
If the feasibility work suggests important reasons to be concerned about reactive effects of measurement, the research team should consider SWATs. Again, there is a wide range of possibilities to consider. These could include randomised comparisons of the volume and contents of measurement, with the former testing the effect of reducing measurement overall. Similarly, they could be directed towards study organisational issues or research staff conduct (e.g. comparing the effects of training vs. no training on participant interactions). Such studies would need to be designed without prejudice to evaluation of the main trial outcome and the statistical power to detect any possible effects considered. The same is true of the process evaluation. The team might decide to randomise a lower proportion of participants to the evaluation and make an a priori decision for main trial analysis to take account of the effect of process evaluation. SWATs do not need to be randomised. Qualitative studies of research staff accounts of their interactions with participants could be undertaken for both trial arms and contribute data to the statistical analyses. Such data could also be useful in integrating the trial outcomes with findings of the process evaluation, as is recommended in existing MRC guidance. 79
SWAT, study within a trial.
Recommendation 4: theorise potential measurement reactions as part of a logic model of how an intervention is intended to work
It is now recognised that developing a programme theory for how an intervention might have an intended effect on a primary outcome or constructing a logic model that specifies the pathways by which an intervention results in the intended outcomes is good practice and can help in selecting appropriate measures and making theory explicit in a trial. 79–81 It has also been proposed that it is useful to develop models of ‘dark logic’ by which interventions may produce harmful effects that are not intended to better understand such phenomena. 82 In line with this, it may be helpful to consider the pathways by which measurement at any stage of the trial may produce bias.
Researchers may want to consider how participants may react to the processes of measurement and to what extent the scenarios presented in Chapter 2 may be applicable to their trial.
Researchers may also find it helpful to consider how the measurement process might interact with the intervention (e.g. as a source of information or by prompting participants’ greater reflection than they would otherwise engage in). 83 It may be particularly useful to consider how changes brought about by measurement may interact with responses to the intended intervention. To construct such logic models it may be useful to involve members of the public or relevant patient groups, as well as to draw on the knowledge of the research team of the phenomenon under investigation.
The existing literature summarised in Chapter 3 is helpful to consider with regard to the potential for MR, but it is by no means exhaustive. In considering whether or not bias from MR is likely to be of concern for a specific trial it may be helpful to refer to this evidence base and other relevant published literature to ascertain if there is evidence that the measurement tools and procedures you plan to use are likely to change research participants’ thoughts, emotions or behaviour. Recommendations on decision-making on conducting further empirical work to explore MR are presented later (see Recommendation 7: consider whether or not measurement reactivity concerns for your trial warrant further empirical examination).
Recommendation 5: consider the burden of measurement procedures and potential impact on participants in comparison with the intensity and duration of the studied intervention
Regular contact with research personnel for measurements might help sustain participants’ engagement and therefore continuation in a trial, thus providing one rationale for the use of interim measurements. Alternatively, some trials have measurement points only at baseline and at a single end point, perhaps because researchers recognise that measurement may produce effects that are large compared with a comparatively minimal intervention. 84 It may be preferable to use non-measurement-related activities (e.g. newsletters) to support participants’ engagement in a trial. It is particularly concerning when the amount of contact or interaction with researchers or clinicians for baseline and follow-up measures is greater than the amount of contact or interaction with the intervention (e.g. in very brief interventions). 85 Feedback from researchers conducting measurements with participants may suggest that MR could be an issue in such circumstances (see Recommendation 8: examine feedback from research personnel regarding research participants’ reports of changes in their behaviour/thoughts/emotions as a result of measurement).
Recommendation 6: consider how participants may use measurement in trials to meet their own aims
It may be helpful to take a participant-centred approach to theorising around research participants’ potential for measurement reactions. 7 Much research examining the effects of measurement conceptualises the process of measurement as affecting passive participants. By contrast, it may be helpful to consider participants as actively pursuing their personal goals when considering the possible effects of MR in producing bias. 86 This means paying careful attention to how participants or prospective participants engage with the features of trial design. For instance, people may wish to take part in a trial to promote physical activity to gain access to outcome measurements (e.g. blood pressure or weight) or to receive regular feedback on their activity levels from an accelerometer. These outcome measurements may be intrinsically motivating in their own right and can produce changes in physical activity irrespective of the intended effects of the intervention.
Other possible goals of participants may produce patterns of responding to measurements. For instance, participants who are being assessed by health-care professionals with whom they have regular contact may wish to respond in such a way as to create a particular impression of need (i.e. to elicit services) or competence (i.e. if they wish to create a productive relationship). Therefore, regular measurement may produce changes in self-reported outcomes. In the absence of blinding, if participants believe that the treatment should be more widely available, they may exaggerate the personal benefits of a treatment to provide evidence of a positive effect of the intervention.
Arguably, the more extensive or meaningful the measurement is to a participant in a trial, the more it becomes possible that the participant will react in ways that have not been foreseen by the researchers. For example, the effects of participants using measurements in trials to meet their own aims are likely to be larger when the measurements are particularly important (e.g. when involving additional checks for people with diabetes or regular monitoring for relapse in people who have had cancer). Similarly, when highly valued concerns (e.g. the adequacy of one’s own parenting) are asked about, it may be that this process leads participants to reflect on their behaviour in ways that may lead to change. Likewise, if painful experiences are explored, it would be unsurprising if this was not to have an impact in some way, which may have implications for data collected subsequently in the study.
Collect further data to inform decisions about whether or not there is risk of bias resulting from measurement reactivity
Given the current state of knowledge, researchers will sometimes have reason to be concerned that MR will be a problem for their trial. However, they may find there to be insufficient knowledge about the extent to which it is likely to be present or if it could lead to risk of bias. This situation creates dilemmas. In these cases it may be sensible to collect further quantitative or qualitative information to inform decisions about potential modifications to trial design that aim to reduce the risk of bias from MR (see Potential actions to minimise risk of bias from measurement reactivity within a trial).
Recommendation 7: consider whether or not measurement reactivity concerns for your trial warrant further empirical examination
Having gone through processes indicated in recommendations 1–6, a judgement is required about the likelihood of risk of bias resulting from MR for a particular trial and whether or not any additional action is then needed. Options vary from taking no further action and proceeding with the trial as planned (when likelihood of risk of bias is very low) to conducting the trial using a Solomon four-group design87 (described below) when likelihood of risk of bias is very high. These two options represent the ends of a spectrum of possible decisions. Figure 3 shows a flow chart to support decision-making, with options including:
-
no further action
-
modifying study design using recommendations in Potential actions to minimise risk of bias from measurement reactivity within a trial
-
investigating risk of MR in pilot or feasibility work to inform a main trial
-
investigating risk of MR in a study within a trial (SWAT)88
-
modifying study design to a Solomon four-group design.
FIGURE 3.
Flow chart to support decision-making for recommendation 7. Boxes in purple indicate potential actions to take following decision-making. Boxes in green indicate issues to consider in decision-making. Arrows in pink indicate hypothetical interim actions to reach decisions about actions to be taken. Reproduced with permission from French et al. 27 This is an Open Access article distributed in accordance with the terms of the Creative Commons Attribution (CC BY 4.0) license, which permits others to distribute, remix, adapt and build upon this work, for commercial use, provided the original work is properly cited. See: https://creativecommons.org/licenses/by/4.0/. The figure includes minor additions and formatting changes to the original figure.
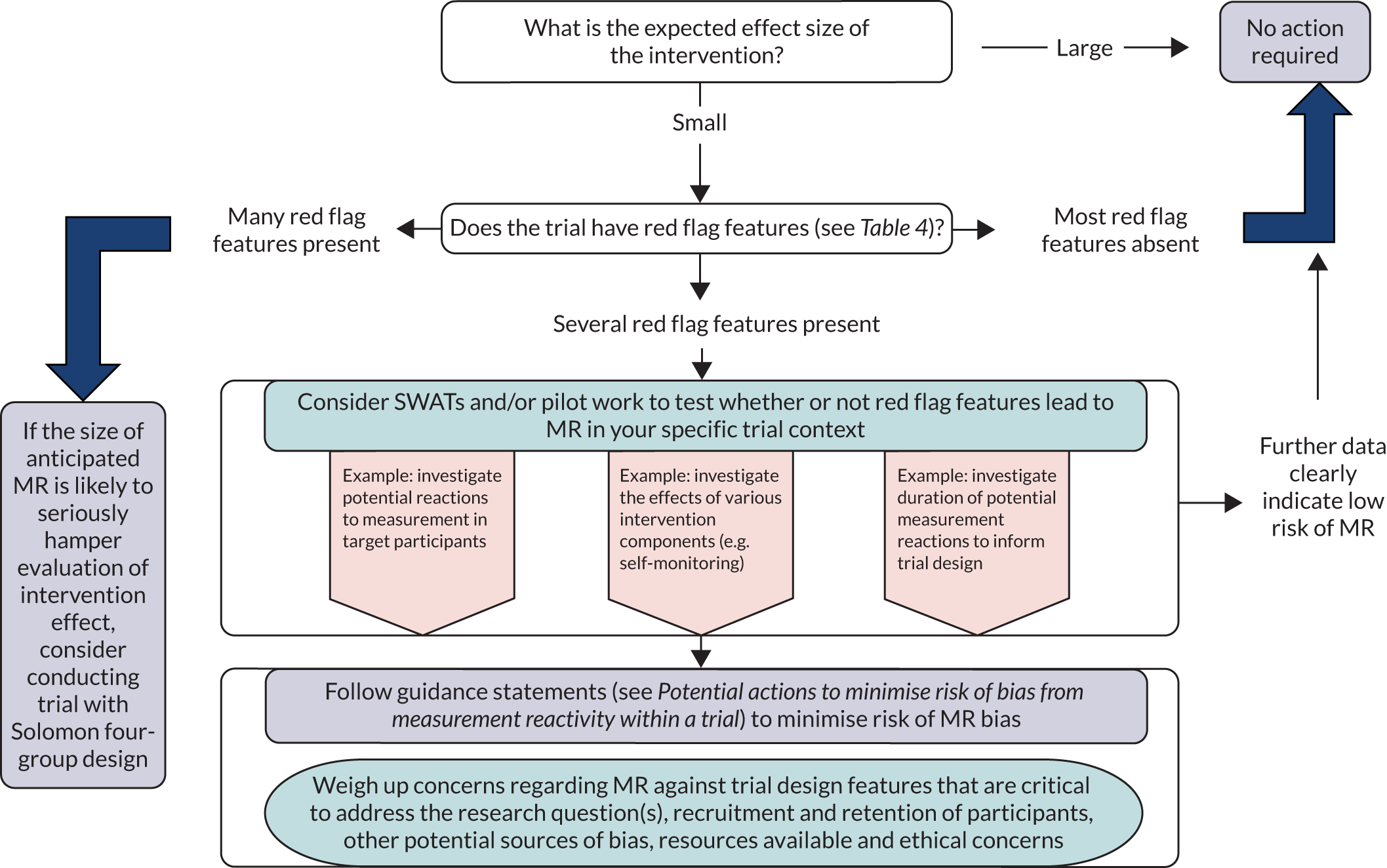
In making these decisions one will have to consider several issues, including the research question(s) for the study, recruitment and retention of trial participants, other potential sources of bias, resources available and ethical considerations. The extent of action to minimise bias from MR needs to be proportionate and weighed against these other concerns. In some cases, relatively simple changes to the study design might be achievable without unduly negative consequences. For example, pragmatic solutions could be sought to ensure that measurement procedures are identical across both arms of a trial (e.g. measurements for both arms conducted by research nurses in a clinic) or references to behavioural goals could be removed from a questionnaire (e.g. removal of references to ‘five a day’ from a question about fruit and vegetable intake). However, it is recognised that in some cases other priorities and concerns might outweigh concerns about potential for bias from MR.
In some situations, for example when theorising about MR suggests that bias could be a risk and when no empirical evidence on reactivity to a particular measurement tool or procedure is available, it might be appropriate to investigate likelihood of MR in pilot/feasibility studies. Such work would offer the potential to test reactions to measurement and inform researchers about the extent to which MR could bias trial findings. Feasibility studies could also be used to determine the presence of potential measurement reactions and quantify their duration to evaluate whether measurement reactions are short or long in relation to length of trial follow-up. It is likely that qualitative studies would be informative in terms of understanding how people could potentially react to measurement and the way in which measurement procedures might influence decisions to take part in research.
A further possibility is the incorporation of nested methodological studies (SWATs) to estimate the magnitude of bias from MR in a subset of participants. The use of subsamples comprising participants who differ in the amount of measurement they receive has the potential to increase understanding of the effects of measurement. The use of SWATs can contribute to estimations of the likely effect size of various amounts of measurement when such measurement is randomly counterbalanced between experimental conditions. Studies that are designed to allow the amount or nature of measurement to differ can often be inexpensive to carry out and would be scientifically valuable given the current state of knowledge regarding MR. The size of a SWAT is necessarily constrained by the size of the host trial and imprecise results may be obtained. A SWAT can nevertheless contribute to the overall body of evidence through updated meta-analyses.
There are obvious precedents for the use of subsamples undergoing different research procedures. It is often the case that subsets of participants undergo interviews as part of a process analysis, have their intervention sessions recorded as part of fidelity assessments or complete additional (often objective) measures to validate other (often self-report) measures. 79 These situations all present potential for bias due to MR as well as for investigating the effects of measurement on participants through SWATs. In such situations, there are ways of statistically controlling for these subsamples in analyses, should this be necessary, as well as making the comparison of these subsamples a focus of investigation in SWATs in their own right.
The Solomon four-group design aims to provide a method for specifically assessing one aspect of MR: the impact of baseline (pre-test) measurements on trial outcomes. 87 The Solomon four-group design is a factorial design in which participants are randomised to intervention and control trial arms as well as to baseline assessment or no baseline assessment. Analysis of this design includes estimation of the effect of baseline assessment and whether or not this effect differs by trial arm status. Challenges that limit use of this design include the logistical difficulty of including four trial arms, the likely increased costs associated with greater sample size requirements and the greater complexity of analytical approach. 23 With these difficulties in mind, it is likely that a Solomon four-group design is warranted only in trials in which several indicators suggest that MR is a major concern and likely to bias effect estimation. An alternative approach that may be worth considering is to undertake a large simple trial that eschews baseline measurement altogether, thereby relying on randomisation of large numbers to generate equivalence between arms to safeguard the experimental design. 89 This may be feasible in some circumstances.
Recommendation 8: examine feedback from research personnel regarding research participants’ reports of changes in their behaviour/thoughts/emotions as a result of measurement
During the course of a trial, research personnel often have several points of contact with research participants that involve informal conversations. It is possible that research participants might voluntarily offer information about changes in their behaviour, thoughts or attitudes that have arisen because of their participation in the trial or even specifically due to measurement procedures. For example, participants in a control arm of an alcohol treatment trial might describe how they have tried to cut down on their alcohol consumption because they did not realise that they were consuming more than recommended levels until they completed the study questionnaire.
It is important to allow the reporting of such feedback from research participants volunteering it (and explicitly asking for such feedback can often be useful) so that common themes can be identified and acted on if necessary. Dedicated provision for the gathering of such material in data collection plans may be needed. If research personnel consistently provide feedback consistent with MR, then such feedback may be able to inform further process evaluations, statistical analysis strategies and/or interpretation of study findings.
Potential actions to minimise risk of bias from measurement reactivity within a trial
A number of options are available when consideration of the issues suggests that MR is likely to cause bias within a trial. These are described below, along with issues that may promote or reduce enthusiasm for their adoption.
Recommendation 9: consider possible measurement reactivity when determining the overall burden of measurement in a trial
There is a wealth of evidence that many patient-reported outcomes are collected during research but often not analysed or reported. 90 This has many downsides, including being an unethical use of participant time (especially when they are in poor health), respondent fatigue and lower response rates leading to poorer-quality data. For these reasons, generally speaking, less measurement in trials would be better. In addition, when there are potential problems with measurement having reactive effects, then having less measurement may reduce the likelihood of bias due to these reactive effects.
There are also compelling reasons for having study measurements. Having baseline measures of primary outcomes reduces the required sample size through increased power if there is high correlation between the outcome at baseline and end point, and it allows for statistical adjustments to be made (e.g. when randomisation has not resulted in similar experimental groups). 91,92 Similarly, a primary outcome measure is required to detect the effects of the intervention at the main follow-up point. It is often desirable for this primary outcome to be completed on several occasions to allow it to be determined if there were initial changes in the primary outcome that were not maintained, or if the effects continued to increase because of changes producing synergistic effects in the longer term.
There are also good arguments for including measures of process to understand how and when effects are produced. Researchers may wish to consider when it is appropriate for all participants to complete all measures and how measurement design can be as economical as possible. For example, an intervention may aim to increase medication adherence by persuading patients that their medication is necessary for their good health. In this case, one would expect a larger effect on beliefs about the medication being necessary than on medication adherence because adherence is further down the causal chain of how the intervention is expected to work. Asking all research participants to complete these process measures (of beliefs about medication necessity) will almost certainly be unnecessary if the hypothesised mechanism of action is correct, as the effect size will be considerably larger than will be the case for the main outcome measure. It should instead be reasonable to ask only a subset of participants to complete these process measures. Following the same reasoning, it may also be efficient to investigate two or more hypothesised causal pathways with randomly drawn, or targeted, subsamples of participants in a single trial with the same primary outcome measure.
The use of process or interim measures within a trial may be particularly problematic for MR. First, they may act as prompts to enacting the intervention or the behaviour under investigation, particularly when the intervention is minimal and the measurements are effortful (e.g. wearing a pedometer or necessitating a visit to see a health-care professional). Second, process measures typically assess hypothesised determinants of behaviours, such as attitudes or intentions. There is good evidence that asking people to complete these kinds of measures can affect behaviour11 and also that asking people to complete measures regarding their beliefs about behaviour can stimulate the creation of new beliefs. 93 For all these reasons, it may be particularly helpful to avoid process or interim measures, or to design them optimally to reduce MR effects.
Recommendation 10: embed measurement procedures into routine clinical practice when possible
The use of unobtrusive measures has long been recommended to avoid problems of measurement affecting participants in research studies. 94 Similarly, in the present context, it will often be desirable to use measurements that are not collected primarily for research purposes, for example data in routine health records or existing data collected for other purposes (e.g. national surveys). Because measurement in trials is a potential source of bias due to MR, this threat is minimised when information from routinely collected data is used instead. However, the use of routinely collected data does require scrutiny regarding potential measurement error and/or how well the measures have been defined.
Recommendation 11: use identical measurement protocols in all arms of a trial
In line with established good practice for trial design, it is desirable to ensure that all aspects of measurement procedures are identical across all arms of a trial. This involves ensuring that all measurements are completed in the same setting at the same frequency and time points and, when relevant, by the same types of people (e.g. research nurses or general practitioners). Format and methodology should also be identical (e.g. online or pencil and paper questionnaire, semistructured interviews). As described in Chapter 2, unbalanced measurement protocols are an a priori cause indicator of a risk of bias from MR. Again, it is important to clearly distinguish between measurements that are a component of the intervention and those that are for only evaluative purposes (see Recommendation 1: consider potential for measurement reactivity causing bias at the design stage of a trial).
Sometimes differential measurement procedures across arms of a trial are employed to help address the research question (e.g. to monitor physiological effects in only the intervention group). 95,96 Researchers in these types of studies should consider whether or not this difference in measurement procedures is a problem and whether or not the control group might be asked to also complete the same measures. This may have the additional benefit of blinding trial participants to the experimental condition to which they have been randomised.
In some cases it would be unwise to give the control group the same measures. For example, if the measurement procedures are a component of the intervention, participants in the control group will necessarily receive part of the intervention. It is also the case that giving any participants measures that are expected to have an effect on the trial outcome is generally undesirable because it may produce bias towards null findings. In general, however, balanced measurement across conditions introduces fewer problems than unbalanced measurement, although minimising any measurement is usually the least problematic option.
Recommendation 12: avoid overlap between measurement and intervention
Some measurement techniques are similar, if not identical, to techniques that are designed to change health-related behaviour (see Box 5 for a detailed discussion). For example, as noted earlier, the use of pedometers to measure behaviour also appears to change behaviour. Pedometers are an efficient method of allowing people to self-monitor their behaviour when the users are not blinded to outcome. When such measurement techniques are used, there is the potential for bias as, in effect, both experimental groups are receiving behaviour change interventions by virtue of the measurement techniques employed. This raises the possibility of an underestimate of effect, especially when the intervention includes the behaviour change technique (BCT) that the measurement technique mimics.
A standardised taxonomy of BCTs16 identifies 93 distinct techniques for changing behaviour, and several appear analogous to measurement procedures. For example, the following are a selection of BCTs and definitions that the taxonomy includes, and which some measurement techniques directly mimic:
-
monitoring of behaviour by others without feedback – observe or record behaviour with the person’s knowledge as part of a behaviour change strategy
-
biofeedback – provide feedback about the body (e.g. physiological or biochemical state) using an external monitoring device as part of a behaviour change strategy
-
monitoring of emotional consequences – prompt assessment of feelings after attempts at performing the behaviour.
In addition, some other measurement approaches have many similarities to BCTs even if they do not directly mimic them. The following are again a selection:
-
information about health consequences – provide information (e.g. written, verbal, visual) about health consequences of performing the behaviour
-
focus on past success – advice to think about or list previous successes in performing the behaviour (or parts of it)
-
prompts/cues – introduce or define environmental or social stimulus with the purpose of prompting or cueing the behaviour.
The first of these BCTs (i.e. information about health consequences) could be mimicked by measurement approaches that ask participants to respond about how likely they believe are various consequences of a behaviour (e.g. smoking). Although these questions do not directly provide information, it is clear that people infer information from questions they are asked because they expect questions to be relevant to the issue being discussed. Questions asking about outcome expectancies have this property and these are common in many social cognition models.
The second BCT (i.e. focus on past successes) could be mimicked by asking participants for their judgements of whether or nor they are capable of performing a behaviour. Although such questions do not directly ask for people to focus on past successes, people use information about past successes and failures to evaluate their future likelihood of success. Questions asking about self-efficacy have this property and, again, this construct is common in many models of behaviour change.
The third BCT (i.e. prompts/cues) could be mimicked by many repeated assessments, using ecological momentary assessment designs. In these designs, questions about behaviour and its hypothesised precursors are asked on a regular basis (often daily) over a period of weeks or months. Similarly, asking someone to wear a piece of equipment, such as an accelerometer or ambulatory blood pressure monitor, could function as a prompt to remind them that they have signed up for an intervention to change behaviours (i.e. physical activity or medication adherence) that an intervention is trying to promote, or which may have an effect on one or more outcomes of the trial.
BCT, behaviour change technique.
It is a clear threat to the validity of a trial if measurement techniques are used that closely resemble the BCT that the trial is designed to evaluate. As part of the development of the logic model for the intervention and MR effects, researchers may find it helpful to consider how the measurements may constitute active interventions. It may also be worth considering that measurement techniques that mimic BCTs may also interact with other BCTs. For instance, the effects of risk communications are much greater in the presence of interventions to increase self-efficacy. 97 When the trial includes measures of self-efficacy, if such measurement acts as an intervention to increase self-efficacy, it is likely that this will synergistically interact with a risk communication intervention.
Recommendation 13: consider the potential benefits of masking measures and/or withholding feedback of measured values against ethical considerations
Withholding information about which health-related outcomes are being measured in a study could help reduce the risk of MR and potential bias in research studies. For example, research participants might supply blood samples for a study but not be informed which biological variables are being measured in their blood. This could protect from risks associated with research participants changing their behaviour (thoughts or emotions) as a result of their awareness of what is being measured (e.g. diabetes patients who are not informed that their blood glucose is being measured may be less likely to change their medication adherence, diet or physical activity than if they are aware that their blood glucose is being measured).
Another approach taken by some researchers to mask the measures of primary interest is to embed them among other ‘filler’ questions in a questionnaire to frame the data collection task in such a way that the research participant is not informed about the measures of most interest to the research team. 98 However, careful consideration of potential negative consequences of requiring participants to complete additional questions is required. Therefore, seeking a trade-off of the pros and cons to this approach is likely to be needed (see Recommendation 9: consider possible measurement reactivity when determining the overall burden of measurement in a trial).
Withholding feedback to research participants about measured values (e.g. 6 mmol/l of blood glucose) could also help reduce the risk of MR and potential bias in research studies. Using the same example, diabetes patients who are informed of their baseline fasting blood glucose values at the beginning of a trial may modify their medication adherence, diet or physical activity on the basis of that knowledge. These changes could interact with their response (or lack of it) to an intervention and consequently be a source of bias. When such changes in behaviour are a risk, withholding disclosure of measured values could reduce the risk of MR and bias.
In certain circumstances the aims of the research may be compromised by giving full information prior to data collection. This is particularly pertinent when there is evidence of, or potential for, MR. For example, there is evidence that covert sealed pedometers (described as ‘posture monitors’ to participants) do not lead to MR (increased physical activity) in contrast to the use of an unsealed pedometer. 13 It is recognised, however, that masking of study measures has the potential to not only protect against MR but also induce it. In the absence of information about a particular measurement, participants could come to their own conclusions about the role of the measure in the study and change their behaviour, emotion or cognition as a result.
Nevertheless, any participant information sheet given to a potential research participant needs to include a clear statement of all aspects of a trial that are relevant to a participant’s decision to take part. It is therefore imperative that ethical considerations are taken into account before any decisions on masking of measurements (and potentially feedback of measured values) are made. 99 Guidance on when is appropriate to provide potentially inaccurate information in research studies has been provided by a range of bodies, including the British Psychological Society. 100 In brief, this British Psychological Society report100 suggests that the amount of information withheld and the delay in disclosing the withheld information should be kept to the absolute minimum necessary. When an essential element of the research design would be compromised by full disclosure to participants, then the withholding of information should be specified and appropriately justified in the project protocol, which should be subjected to ethical review. In addition, explicit procedures should be stated to obviate any potential harm arising from such withholding. According to the British Psychological Society, information should be withheld or covert collection of data take place only if this is essential to achieve the research results required. This could be interpreted as including a situation when research results are very likely to be biased as a result of MR. It is imperative that the research objective has strong scientific merit and that an appropriate risk management and harm alleviation strategy is in place. Similar guidance is given by the Economic and Social Research Council. 101 In line with existing guidance, it is important to provide an appropriate debriefing for participants that later reveals the intention of the research, once data collection is completed.
Although masking of measures could be a potential technique for alleviating issues of MR, the issues to consider are not limited to ethics. Participants decide to take part in research for a variety of reasons. Some reasons are altruistic, but others may support participants’ own aims. 102 For example, a participant may want to be measured in some way to assess their own health status or to motivate them to achieve a health-related target. As a result, decisions to take part in research may be dependent on the feedback that participants receive. Masking measures and/or preventing feedback may therefore have a negative impact on study recruitment and retention. Motivating factors for taking part in the research and the role of measurements (and feedback) in the decision-making process are important topics to explore in patient and public involvement initiatives in the early design stages of a trial. 102,103
Recommendation 14: if measurement reactivity is likely to be present, investigations for measurement reactivity should be included a priori in the statistical analysis plan
Ideally, evidence about the expected magnitude of MR is needed to inform analysis plans (see Chapter 5). However, it may be difficult to distinguish bias due to MR from bias from other potential sources in a trial at the statistical analysis stage. For instance, a statistician can explore how dilution bias may manifest, but there are many sources of this type of bias, not just MR.
For many reasons, it is highly recommended that a statistical analysis plan be developed prior to the analysis being carried out. 104 Having considered the likelihood of MR in a particular trial based on logic, external evidence and the recommendations provided in this document, if there is some reasonable likelihood of MR being present, quantitative investigations of MR should be included a priori in the trial protocol, including a statistical analysis plan. These investigations could include sensitivity analyses based on, for example, a subgroup of trial participants measured more intensively in a qualitative substudy in both trial arms. The sensitivity analysis could explore implications of adjusting for or excluding those participants from the main quantitative analysis.
Statistical analyses should also be informed by feasibility and pilot work (see Recommendation 7: consider whether or not measurement reactivity concerns for your trial warrant further empirical examination). For example, for some measurement procedures (e.g. blood pressure or step count using pedometers) the first one or two measurements are particularly reactive. Therefore, some researchers collect multiple baseline measurements but do not use all of them. When researchers suspect that another measurement procedure that they are using could be similarly reactive, then data from a feasibility trial could be explored to look for MR in, for example, the first 1 or 2 days of measurement. If such MR appears to be present, then data from these first 1 or 2 days could be removed from the main study analysis. However, careful consideration of the potential negative consequences of requiring participants to complete additional measurements is required.
When comparing multiple trials on a single intervention/topic in a systematic review, the reviewers could consider MR as a source of heterogeneity, for example considering subgroups of trials based on whether or not they had baseline measurement.
Chapter 5 Future research
A major limitation of the evidence base used to develop the recommendations is the shortage of good-quality studies regarding the likely extent and magnitude of MR in many settings. There is a particular lack of direct evidence regarding the extent to which MR produces bias in trials, despite a good deal of speculation on this topic, including post hoc justifications for null trial effects. 105 Examples of prospectively designed studies that quantify bias are rare. 106 The present section of this report identifies the areas of research that should be prioritised to address this lack of evidence.
Recommendation 1: more primary research to quantify extent of measurement reactivity
Some aspects of MR, such as the QBE, have received a considerable amount of research attention. 9,10 This has produced estimates of the QBE as being of small magnitude, with considerable heterogeneity. There is a need for further primary studies investigating the issue of MR more broadly than in relation to the QBE, especially where there is a dearth of studies and where measures may be particularly reactive. For example, there is a particular absence of evidence for dietary assessments, which can be time-consuming and produce measures of healthy eating that are informative to research participants and, hence, may promote more reflection regarding that behaviour. If such intensive measurements do not produce compelling evidence of reactivity, then they are unlikely to lead to bias.
New primary research studies of MR should aim to have lower risk of bias than much research that has been done to date. 14 For example, many primary studies of the QBE have included confounders such as thank you letters being sent in addition to questionnaires, which makes it unclear whether any differences between experimental groups are because of a MR effect or because of the effects of reminders or prompts. 14 More recent studies of the QBE have been of higher quality (e.g. through pre-registration of study protocols).
There is currently a dearth of research on potential for MR and implications for bias in clinical trials that do not have a behavioural outcome, and future research in this area is particularly warranted. Many trials of medical interventions involve closer, more intensive follow-up than would apply in routine practice, which may be differential across trial arms. This may be justified for monitoring of the safety of untested interventions or for measuring adherence; however, it could potentially alter the results, making the present recommendations highly relevant.
There is also a shortage of studies specifically reporting the extent to which qualitative process interviews or fidelity assessments are reactive. Although many trials have included these interviews and assessments, the size of effect from such studies has not been reported. Furthermore, a priori research protocols investigating the effects of interviews and assessments have generally not been published.
Given the cost of trials, it may be most feasible for future studies that aim to quantify the extent of MR to be SWATs. 88 Such studies are nested within larger trials in which participants could be randomised to receive different measurement procedures. Such procedures could involve the extent of measurement (e.g. interim process measures or shorter questionnaires), the timing of measurement (e.g. closer to vs. further away from intervention elements), the nature of outcome measurements (e.g. objective vs. self-report) and the type of measurement procedures examined (e.g. questionnaire vs. interview). Such SWATs should be pre-registered, with full reporting of all findings (including null findings) on a range of primary and secondary trial outcomes. A particular problem in the MR literature is that it is not clear whether the absence of evidence regarding reactivity is because of a lack of research activity or non-reporting of null findings. 1 Such SWATs could also examine interacting effects of measurement and the planned intervention, given the dearth of such research reported to date. 23
A key consideration for any SWAT is that it should not detract from the key objectives of the main trial within which the SWAT is nested. For example, when the impact of including quantitative measures of hypothesised mediators is assessed, the study should still be powered to detect the hypothesised mediation effect. Any variation in measurement could be statistically controlled for in the analyses of the primary study. At the present time, it would generally be expected that measurement would have only a small effect on outcomes1 and so it should not inflate sample sizes required for the main trial purpose. It should be noted that future studies may produce evidence of larger MR effects than the limited amount of previous research indicates. At this point, it may be useful to focus on the psychological mechanisms underpinning how reactivity produces bias in trials, but this focus is currently premature.
It may be sensible to employ adaptive designs107 whereby a SWAT is conducted to consider a specific methodological issue (e.g. the effect of a set of measures on an outcome measure or primary outcome response rate) until the evidence suggests that there is no effect of this experimental manipulation and the trial can be continued without this experimental manipulation.
Recommendation 2: research priorities for studies within a trial
In general, the greatest need for evidence is where (1) there is a dearth of studies, (2) measures may be particularly reactive and (3) the outcomes are particularly important. Other than this, three specific areas identified as possible priorities for SWATs to further understanding of MR are as follows:
-
Table 4 shows the study features in which measurement may be more likely to be reactive and risk bias. It would be useful for further empirical studies to provide more compelling evidence on these study features.
-
The comparison of traditional obtrusive research methods with unobtrusive research methods. It was proposed some time ago that being observed affects the behaviour of people being observed or taking part in research studies. This is often known as the Hawthorne effect. 6 There is still little evidence on this subject relating to MR6 and there are ethical issues associated with masking and other approaches that seek to be unobtrusive that need to be explored.
-
Effects on both objective and subjective outcomes. It is still not clear how far, and in which circumstances, reactivity produces genuine changes in behaviour or just a mental recalibration of how people think about behaviour. 108 Although both a real change in behaviour and a recalibration are of interest, the mechanisms and implications would be different and so clarity is needed on this point.
Recommendation 3: more systematic reviewing to quantify extent and variability of measurement reactivity
In addition to the conduct of further primary research it would also be helpful to summarise more robustly the state of several current literatures. For example, the rapid systematic reviews conducted for the present study indicate that there are several studies that have examined the impact of objective measurement instruments (whether blinded or not) on physical activity. Other possible topics include more formal reviews of the elevation of anxiety scores on the first occasion of measurement. Future reviews may determine that there are sufficient studies of the reactive effects of nested interview studies or fidelity assessments in trials. It may also be useful to meta-synthesise qualitative studies of the experience of completing measures in trials and views about such measures.
One of the barriers to systematic reviews of the impact of measurement on outcomes concerns the poor reporting of the nature and extent of measurement. Evidence syntheses of existing research studies may require additional data on measurement within those studies. It is common for secondary trial outcomes not to be reported. 90 A corollary of this is that the measures completed by participants are thereby not reported. It is usual for journal publications to mention only those measures for which results are reported, rather than those which are completed. Future primary studies should fully report all measures that participants were asked to complete (e.g. in line with the Template for Intervention Description and Replication reporting of intended interventions in trials). 109
If sufficient SWATs are undertaken, then the results should be collated in living systematic reviews to inform researchers about where there is sufficient evidence to no longer investigate the reactive effects of particular forms of measurement and where there is a need for further primary research.
Recommendation 4: the need to better theorise when and why measurement reactivity is likely to occur
Several explanations for the presence of the QBE have been empirically examined,3,10,11 with some receiving limited empirical support. Despite this, much of this research has relied on moderator analyses within systematic reviews, and it is likely that many of the moderators anticipated will turn out to be artefacts due to confounding of numerous features. This makes it difficult to identify the precise factors that are responsible for the presence of larger MR effects. 14 Relatedly, the amount of variance explained by these moderators in health settings is typically very small,9 making them of little practical use in predicting when MR is likely to occur.
To make better progress in understanding this phenomenon there is a need for better theorising of why reactivity may be occurring, including when, where and how. Qualitative studies that are nested within trials will be useful in producing insights into how people experience the process of trial participation and the experience of measurement itself. 86 Although qualitative studies may not be able to detect automatic psychological processes among participants, they can make useful contributions to knowledge even if they do not provide the full picture of mechanisms underlying measurement reactions. Such studies may nonetheless provide the basis for important conceptual advances.
Such insights will be useful in generating logic models (see Chapter 4, Recommendation 4: theorise potential measurement reactions as part of a logic model of how an intervention is intended to work) that specify how measurement may produce changes in those people being measured. Qualitative studies nested within trials are likely to generate hypotheses regarding the circumstances when MR is likely to occur in health settings, which subsequent research could then test via experimental manipulations. Such studies could include trial or health-care staff to investigate the extent to which any reactivity is due to these staff behaving differently in the light of information from measurements completed, rather than the research participants reacting to such measurements. As with quantitative SWATs, qualitative studies with research participants or staff should be comparatively inexpensive to conduct.
Qualitative studies may also help identify some subgroups of participants that are more likely to react to measurement, and purposive sampling could help to further study these groups. Qualitative research to understand the reasons why MR occurs also has the advantage that it could help establish contextual influences on reactivity and deepen appreciation of the ethical issues involved in procedures, such as masking, that seek to minimise MR. Much existing research on MR has focused on understanding the intraindividual processes that may be responsible for reactivity. 3,11 Although useful, such research may be less useful in identifying the features of specific trials that would be more likely to produce MR. Furthermore, research on contextual influences on MR may more easily translate into what steps should be taken to reduce the likelihood of MR occurring and producing bias.
Acknowledgements
The MERIT study collaborators
The writing group would like to thank the MERIT Collaborative Group (see Appendix 1) for developing the recommendations in the October 2018 workshop.
Delphi participants
The writing group would also like to acknowledge the expert input into the scope of these recommendations from participants in an international Delphi procedure. The following people took part in the Delphi procedure and participants had a range of views (note that participation in the Delphi procedure does not necessarily mean agreement with the recommendations): Professor Chris Bonell, London School of Hygiene and Tropical Medicine; Professor Peter Bower, University of Manchester; Sir Iain Chalmers, James Lind Initiative; Dr Stacy Clemes, University of Loughborough; Dr Ruxandra Comanaru, NatCen Social Research; Professor Mark Conner, University of Leeds; Professor Cindy Cooper, University of Sheffield; Professor Paul Crane, University of Washington; Professor Diane Dixon, University of Aberdeen; Ms Ruth Dundas, University of Glasgow; Professor Diana Elbourne, London School of Hygiene and Tropical Medicine;* Professor Andrew Farmer, University of Oxford;* Professor David French, University of Manchester;* Professor Martin Gulliford, King’s College London (round 2 only);* Professor Julian Higgins, University of Bristol; Dr Lisa Hinton, THIS Institute, University of Cambridge; Professor Kate Hunt, University of Stirling; Professor Susan Jebb, University of Oxford; Professor Marie Johnston, University of Aberdeen; Professor Gerjo Kok, Maastricht University; Professor Sean Lane, Purdue University; Professor Louise Locock, University of Aberdeen;* Dr Rebecca Lynch, King’s College London; Professor Graeme MacLennan, University of Aberdeen; Professor Jim McCambridge, University of York;* Professor Elizabeth Murray, UCL; Professor John Norrie, University of Edinburgh; Professor Ronan O’Carroll, University of Stirling; Professor Rafael Perera, University of Oxford; Ms Beth Shaw, Oregon Health and Science University; Professor Paschal Sheeran, University of North Carolina at Chapel Hill; Professor Falko F Sniehotta, Newcastle University; Professor Mirjam Sprangers, Amsterdam University Medical Centers; Professor Stephen Sutton, University of Cambridge;* Professor Matthew Sydes, UCL; Professor David Torgerson, University of York; Professor Shaun Treweek, University of Aberdeen; Dr Anne van Dongen, University of York; Professor Esther van Sluijs, University of Cambridge; Professor Ian R White, MRC Clinical Trials Unit, UCL; and Professor Teun Zuiderent-Jerak, Vrije Universiteit Amsterdam.
*Member of writing group.
The writing group would also like to thank the following for their helpful comments on earlier drafts of these recommendations: Professor Ronan O’Carroll, University of Stirling, and Professor David Torgerson, University of York.
Contributions of authors
David P French (https://orcid.org/0000-0002-7663-7804) (Professor of Health Psychology, health psychology) had the initial idea for the project, developed a proposal to the MRC–National Institute for Health Research (NIHR) Methodology Research programme and wrote the grant application to the MRC/NIHR; chaired the MERIT study workshop discussions; supervised the QBE systematic review update; drafted sections of the final report and revised it critically for important intellectual content; and provided scientific oversight for the study as a whole.
Lisa M Miles (https://orcid.org/0000-0002-8971-125X) (Research Associate, public health) prepared the ethics application for the study; project managed the study as a whole; conducted and analysed the results of the Delphi procedure (with input from other authors) and conducted the QBE systematic review update with input from David P French, both of which were used to inform the recommendations; and drafted sections of the final report.
Diana Elbourne (https://orcid.org/0000-0003-3044-4545) (Professor of Healthcare Evaluation, trial statistics) provided input into the design of the study and the grant application to the MRC/NIHR; provided scientific input throughout the conduct of the project; and revised the report critically for important intellectual content.
Andrew Farmer (https://orcid.org/0000-0002-6170-4402) (Professor of General Practice, trials) provided input into the design of the study and the grant application to the MRC/NIHR; provided scientific input throughout the conduct of the project; and revised the report critically for important intellectual content.
Martin Gulliford (https://orcid.org/0000-0003-1898-9075) (Professor of Public Health, public health epidemiology and health services research) provided input into the design of the study and the grant application to MRC/NIHR; provided scientific input throughout the conduct of the project; and revised the report critically for important intellectual content.
Louise Locock (https://orcid.org/0000-0002-8109-1930) (Professor of Health Services Research, qualitative research) provided input into the design of the study and the grant application to the MRC/NIHR; provided scientific input throughout the conduct of the project; and revised the report critically for important intellectual content.
Stephen Sutton (https://orcid.org/0000-0003-1610-0404) (Professor of Behavioural Science, behaviour change) provided input into the design of the MERIT study and the grant application to the MRC/NIHR; led the three rapid reviews conducted to inform the report (with input from David P French); provided scientific input throughout the conduct of the project; and revised the report critically for important intellectual content.
Jim McCambridge (https://orcid.org/0000-0002-5461-7001) (Professor of Addictive Behaviours and Public Health, public health) developed the study proposal to the MRC–NIHR Methodology Research programme; provided input into the design of the study and the grant application to the MRC/NIHR; provided scientific input throughout the conduct of the project; drafted sections of the final report; and revised the report critically for important intellectual content.
Publications
Miles LM, Elbourne D, Farmer A, Gulliford M, Locock L, McCambridge J, et al. Bias due to MEasurement Reactions In Trials to improve health (MERIT): protocol for research to develop MRC guidance. Trials 2018;19:653.
French DP, Miles LM, Elbourne D, Farmer A, Gulliford M, Locock L, et al. Reducing bias in trials due to reactions to measurement: experts produced recommendations informed by evidence. J Clin Epidemiol 2021;139:130–9.
Data-sharing statement
All data requests should be submitted to the corresponding author for consideration. Access to anonymised data may be granted following review.
Disclaimers
This report presents independent research funded under a MRC–NIHR partnership. The views and opinions expressed by authors in this publication are those of the authors and do not necessarily reflect those of the NHS, the NIHR, the MRC, NETSCC, the HTA programme or the Department of Health and Social Care. If there are verbatim quotations included in this publication the views and opinions expressed by the interviewees are those of the interviewees and do not necessarily reflect those of the authors, those of the NHS, the NIHR, the MRC, NETSCC, the HTA programme or the Department of Health and Social Care.
References
- French DP, Sutton S. Reactivity of measurement in health psychology: how much of a problem is it? What can be done about it?. Br J Health Psychol 2010;15:453-68. https://doi.org/10.1348/135910710x492341.
- Miles LM, Elbourne D, Farmer A, Gulliford M, Locock L, McCambridge J, et al. Bias due to MEasurement Reactions In Trials to improve health (MERIT): protocol for research to develop MRC guidance. Trials 2018;19. https://doi.org/10.1186/s13063-018-3017-5.
- Spangenberg ER, Kareklas I, Devezer B, Sprott DE. A meta-analytic synthesis of the question–behavior effect. J Consum Psychol 2016;26:441-58. https://doi.org/10.1016/j.jcps.2015.12.004.
- McCambridge J, Kypri K, Elbourne D. In randomization we trust? There are overlooked problems in experimenting with people in behavioral intervention trials. J Clin Epidemiol 2014;67:247-53. https://doi.org/10.1016/j.jclinepi.2013.09.004.
- French JRP, Festinger L, Gravetter F. Research Methods in the Behavioral Sciences. New York, NY: Holt, Rinehart & Winston; 1953.
- McCambridge J, Witton J, Elbourne DR. Systematic review of the Hawthorne effect: new concepts are needed to study research participation effects. J Clin Epidemiol 2014;67:267-77. https://doi.org/10.1016/j.jclinepi.2013.08.015.
- McCambridge J, Kypri K, Elbourne D. Research participation effects: a skeleton in the methodological cupboard. J Clin Epidemiol 2014;67:845-9. https://doi.org/10.1016/j.jclinepi.2014.03.002.
- McCambridge J, Kypri K. Can simply answering research questions change behaviour? Systematic review and meta analyses of brief alcohol intervention trials. PLOS ONE 2011;6. https://doi.org/10.1371/journal.pone.0023748.
- Rodrigues AM, O’Brien N, French DP, Glidewell L, Sniehotta FF. The question–behavior effect: genuine effect or spurious phenomenon? A systematic review of randomized controlled trials with meta-analyses. Health Psychol 2015;34:61-78. https://doi.org/10.1037/hea0000104.
- Wood C, Conner M, Miles E, Sandberg T, Taylor N, Godin G, et al. The impact of asking intention or self-prediction questions on subsequent behavior: a meta-analysis. Pers Soc Psychol Rev 2016;20:245-68. https://doi.org/10.1177/1088868315592334.
- Wilding S, Conner M, Sandberg T, Prestwich A, Lawton R, Wood C, et al. The question–behaviour effect: a theoretical and methodological review and meta-analysis. Eur Rev Soc Psychol 2016;27:196-230. https://doi.org/10.1080/10463283.2016.1245940.
- Bravata DM, Smith-Spangler C, Sundaram V, Gienger AL, Lin N, Lewis R, et al. Using pedometers to increase physical activity and improve health: a systematic review. JAMA 2007;298:2296-304. https://doi.org/10.1001/jama.298.19.2296.
- Clemes SA, Parker RA. Increasing our understanding of reactivity to pedometers in adults. Med Sci Sports Exerc 2009;41:674-80. https://doi.org/10.1249/MSS.0b013e31818cae32.
- Rodrigues AM, French DP, Sniehotta FF. Commentary: the impact of asking intention or self-prediction questions on subsequent behavior: a meta-analysis. Front Psychol 2016;7. https://doi.org/10.3389/fpsyg.2016.00879.
- Harris T, Kerry SM, Limb ES, Furness C, Wahlich C, Victor CR, et al. Physical activity levels in adults and older adults 3-4 years after pedometer-based walking interventions: long-term follow-up of participants from two randomised controlled trials in UK primary care. PLOS Med 2018;15. https://doi.org/10.1371/journal.pmed.1002526.
- Michie S, Wood CE, Johnston M, Abraham C, Francis JJ, Hardeman W. Behaviour change techniques: the development and evaluation of a taxonomic method for reporting and describing behaviour change interventions (a suite of five studies involving consensus methods, randomised controlled trials and analysis of qualitative data). Health Technol Assess 2015;19. https://doi.org/10.3310/hta19990.
- Michie S, Abraham C, Whittington C, McAteer J, Gupta S. Effective techniques in healthy eating and physical activity interventions: a meta-regression. Health Psychol 2009;28:690-701. https://doi.org/10.1037/a0016136.
- Johnston M. Mood in chronic disease: questioning the answers. Curr Psychol 1999;18:71-87. https://doi.org/10.1007/s12144-999-1017-z.
- Lister AM, Rode S, Farmer A, Salkovskis PM. Does thinking about personal health risk increase anxiety?. J Health Psychol 2002;7:410-14. https://doi.org/10.1177/1359105302007004329.
- Shrout PE, Stadler G, Lane SP, McClure MJ, Jackson GL, Clavél FD, et al. Initial elevation bias in subjective reports. Proc Natl Acad Sci USA 2018;115:E15-E23. https://doi.org/10.1073/pnas.1712277115.
- Sharpe JP, Gilbert D. Effects of repeated administration of the Beck Depression Inventory and other measures of negative mood states. Pers Indiv Dif 1998;24:457-63. https://doi.org/10.1016/S0191-8869(97)00193-1.
- Madigan CD, Daley AJ, Lewis AL, Aveyard P, Jolly K. Is self-weighing an effective tool for weight loss: a systematic literature review and meta-analysis. Int J Behav Nutr Phys Act 2015;12. https://doi.org/10.1186/s12966-015-0267-4.
- McCambridge J, Butor-Bhavsar K, Witton J, Elbourne D. Can research assessments themselves cause bias in behaviour change trials? A systematic review of evidence from solomon 4-group studies. PLOS ONE 2011;6. https://doi.org/10.1371/journal.pone.0025223.
- Schulz KF, Altman DG, Moher D. CONSORT Group . CONSORT 2010 statement: updated guidelines for reporting parallel group randomised trials. BMJ 2010;340. https://doi.org/10.1136/bmj.c332.
- Higgins J, Green S. Cochrane Handbook for Systematic Reviews of Healthcare Interventions. Chichester: Wiley-Blackwell; 2008.
- Guyatt G, Oxman AD, Akl EA, Kunz R, Vist G, Brozek J, et al. GRADE guidelines: 1. Introduction – GRADE evidence profiles and summary of findings tables. J Clin Epidemiol 2011;64:383-94. https://doi.org/10.1016/j.jclinepi.2010.04.026.
- French DP, Miles LM, Elbourne D, Farmer A, Gulliford M, Locock L, et al. Reducing bias in trials due to reactions to measurement: experts produced recommendations informed by evidence. J Clin Epidemiol 2021;139:130-9. https://doi.org/10.1016/j.jclinepi.2021.06.028.
- Last JM. A Dictionary of Epidemiology. Oxford: Oxford University Press; 2001.
- McCambridge J, Sorhaindo A, Quirk A, Nanchahal K. Patient preferences and performance bias in a weight loss trial with a usual care arm. Patient Educ Couns 2014;95:243-7. https://doi.org/10.1016/j.pec.2014.01.003.
- McCambridge J, Kalaitzaki E, White IR, Khadjesari Z, Murray E, Linke S, et al. Impact of length or relevance of questionnaires on attrition in online trials: randomized controlled trial. J Med Internet Res 2011;13. https://doi.org/10.2196/jmir.1733.
- Turner CF, Ku L, Rogers SM, Lindberg LD, Pleck JH, Sonenstein FL. Adolescent sexual behavior, drug use, and violence: increased reporting with computer survey technology. Science 1998;280:867-73. https://doi.org/10.1126/science.280.5365.867.
- O’Carroll RE, Chambers JA, Brownlee L, Libby G, Steele RJ. Anticipated regret to increase uptake of colorectal cancer screening (ARTICS): a randomised controlled trial. Soc Sci Med 2015;142:118-27. https://doi.org/10.1016/j.socscimed.2015.07.026.
- McDermott L, Cornelius V, Wright AJ, Burgess C, Forster AS, Ashworth M, et al. Enhanced invitations using the question–behavior effect and financial incentives to promote health check uptake in primary care. Ann Behav Med 2018;52:594-605. https://doi.org/10.1093/abm/kax048.
- Miles LM, Rodrigues AM, Sniehotta FF, French DP. Asking questions changes health-related behavior: an updated systematic review and meta-analysis. J Clin Epidemiol 2020;123:59-68. https://doi.org/10.1016/j.jclinepi.2020.03.014.
- AMSTAR . A Measurement Tool to Assess Systematic Reviews n.d. https://amstar.ca (accessed 29 June 2020).
- Bernstein JA, Bernstein E, Heeren TC. Mechanisms of change in control group drinking in clinical trials of brief alcohol intervention: implications for bias toward the null. Drug Alcohol Rev 2010;29:498-507. https://doi.org/10.1111/j.1465-3362.2010.00174.x.
- Choo EK, Gottlieb AS, DeLuca M, Tape C, Colwell L, Zlotnick C. Systematic review of ED-based intimate partner violence intervention research. West J Emerg Med 2015;16:1037-42. https://doi.org/10.5811/westjem.2015.10.27586.
- Clifford PR, Davis CM. Alcohol treatment research assessment exposure: a critical review of the literature. Psychol Addict Behav 2012;26:773-81. https://doi.org/10.1037/a0029747.
- Heather N. Interpreting null findings from trials of alcohol brief interventions. Front Psychiatry 2014;5. https://doi.org/10.3389/fpsyt.2014.00085.
- Jenkins RJ, McAlaney J, McCambridge J. Change over time in alcohol consumption in control groups in brief intervention studies: systematic review and meta-regression study. Drug Alcohol Depend 2009;100:107-14. https://doi.org/10.1016/j.drugalcdep.2008.09.016.
- Jenkins R, McAlaney J, McCambridge J. Corrigendum to ‘Change over time in alcohol consumption in control groups in brief intervention studies: systematic review and meta-regression study’ [Drug Alcohol Depend. 100 (2009) 107–114] (https://doi.org/10.1016/j.drugalcdep.2008.09.016). Drug Alcohol Depend 2010;108. https://doi.org/10.1016/j.drugalcdep.2009.11.007.
- Schrimsher GW, Filtz K. Assessment reactivity: can assessment of alcohol use during research be an active treatment?. Alcohol Treat Q 2011;29:108-15. https://doi.org/10.1080/07347324.2011.557983.
- Wray TB, Merrill JE, Monti PM. Using Ecological Momentary Assessment (EMA) to assess situation-level predictors of alcohol use and alcohol-related consequences. Alcohol Res 2014;36:19-27.
- Clemes SA, Biddle SJ. The use of pedometers for monitoring physical activity in children and adolescents: measurement considerations. J Phys Act Health 2013;10:249-62. https://doi.org/10.1123/jpah.10.2.249.
- Fitzsimons GJ, Moore SG. Should we ask our children about sex, drugs, and rock & roll? Potentially harmful effects of asking questions about risky behaviors. J Consumer Psychology 2008;18:82-95. https://doi.org/10.1016/j.jcps.2008.01.002.
- Fraser MW, Wu S. Measures of consumer satisfaction in social welfare and behavioral health: a systematic review. Res Social Work Pract 2015;26:762-76. https://doi.org/10.1177/1049731514564990.
- French DP, Sutton S. Reactivity of measurement in health psychology: how much of a problem is it? What can be done about it?. Br J Health Psychol 2010;15:453-68. https://doi.org/10.1348/135910710X492341.
- Jenkins RJ, McAlaney J, McCambridge J. Change over time in alcohol consumption in control groups in brief intervention studies: systematic review and meta-regression study. Drug Alcohol Depend 2009;100:107-14.
- Mdege ND, Watson J. Predictors of study setting (primary care vs. hospital setting) among studies of the effectiveness of brief interventions among heavy alcohol users: a systematic review. Drug Alcohol Rev 2013;32:369-80. https://doi.org/10.1111/dar.12036.
- Stalgaitis C, Glick SN. The use of web-based diaries in sexual risk behavior research: a systematic review. Sex Transm Infect 2014;90:374-81. https://doi.org/10.1136/sextrans-2013-051472.
- Tobias R, Inauen J. Gathering time-series data for evaluating behavior-change campaigns in developing countries: reactivity of diaries and interviews. Eval Rev 2010;34:367-90. https://doi.org/10.1177/0193841X10383940.
- Wray TB, Merrill JE, Monti PM. Using ecological momentary assessment (EMA) to assess situation-level predictors of alcohol use and alcohol-related consequences. Alcohol Res 2014;36:19-27.
- Mankarious E, Kothe E. A meta-analysis of the effects of measuring theory of planned behaviour constructs on behaviour within prospective studies. Health Psychol Rev 2015;9:190-204. https://doi.org/10.1080/17437199.2014.927722.
- Ajzen I, Kuhl J, Beckmann J. Action Control: From Cognitions to Behaviors. New York, NY: Springer; 1985.
- Clemes SA, Matchett N, Wane SL. Reactivity: an issue for short-term pedometer studies?. Br J Sports Med 2008;42:68-70. https://doi.org/10.1136/bjsm.2007.038521.
- Clemes SA, Deans NK. Presence and duration of reactivity to pedometers in adults. Med Sci Sports Exerc 2012;44:1097-101. https://doi.org/10.1249/MSS.0b013e318242a377.
- Craig CL, Tudor-Locke C, Cragg S, Cameron C. Process and treatment of pedometer data collection for youth: the Canadian Physical Activity Levels among Youth study. Med Sci Sports Exerc 2010;42:430-5. https://doi.org/10.1249/MSS.0b013e3181b67544.
- Ling FC, Masters RS, McManus AM. Rehearsal and pedometer reactivity in children. J Clin Psychol 2011;67:261-6. https://doi.org/10.1002/jclp.20745.
- Ling J, King KM. Measuring physical activity of elementary school children with unsealed pedometers: compliance, reliability, and reactivity. J Nurs Meas 2015;23:271-86. https://doi.org/10.1891/1061-3749.23.2.271.
- Motl RW, Dlugonski D. Increasing physical activity in multiple sclerosis using a behavioral intervention. Behav Med 2011;37:125-31. https://doi.org/10.1080/08964289.2011.636769.
- Hilgenkamp T, Van Wijck R, Evenhuis H. Measuring physical activity with pedometers in older adults with intellectual disability: reactivity and number of days. Intellect Dev Disabil 2012;50:343-51. https://doi.org/10.1352/1934-9556-50.4.343.
- Prewitt SL, Hannon JC, Brusseau TA. Children and pedometers: a study in reactivity and knowledge. Int J Exerc Sci 2013;6:230-5.
- Albright CL, Steffen AD, Wilkens LR, White KK, Novotny R, Nigg CR, et al. Effectiveness of a 12-month randomized clinical trial to increase physical activity in multiethnic postpartum women: results from Hawaii’s Nā Mikimiki Project. Prev Med 2014;69:214-23. https://doi.org/10.1016/j.ypmed.2014.09.019.
- Dössegger A, Ruch N, Jimmy G, Braun-Fahrländer C, Mäder U, Hänggi J, et al. Reactivity to accelerometer measurement of children and adolescents. Med Sci Sports Exerc 2014;46:1140-6. https://doi.org/10.1249/MSS.0000000000000215.
- Scott JJ, Morgan PJ, Plotnikoff RC, Trost SG, Lubans DR. Adolescent pedometer protocols: examining reactivity, tampering and participants’ perceptions. J Sports Sci 2014;32:183-90. https://doi.org/10.1080/02640414.2013.815361.
- Davis RE, Loprinzi PD. Examination of accelerometer reactivity among a population sample of children, adolescents, and adults. J Phys Act Health 2016;13:1325-32. https://doi.org/10.1123/jpah.2015-0703.
- Jones KK, Zenk SN, McDonald A, Corte C. Experiences of African-American women with smartphone-based ecological momentary assessment. Public Health Nurs 2016;33:371-80. https://doi.org/10.1111/phn.12239.
- Cook P, Schmiege S, McClean M, Aagaard L, Kahook M. Practical and analytic issues in the electronic assessment of adherence. West J Nurs Res 2012;34:598-620. https://doi.org/10.1177/0193945911427153.
- Sutton S, Kinmonth AL, Hardeman W, Hughes D, Boase S, Prevost AT, et al. Does electronic monitoring influence adherence to medication? Randomized controlled trial of measurement reactivity. Ann Behav Med 2014;48:293-9. https://doi.org/10.1007/s12160-014-9595-x.
- Pill J. The Delphi method: substance, context, a critique and an annotated bibliography. Socioecon Plann Sci 1971;5:57-71. https://doi.org/10.1016/0038-0121(71)90041-3.
- Shieh C, Knisely MR, Clark D, Carpenter JS. Self-weighing in weight management interventions: a systematic review of literature. Obes Res Clin Pract 2016;10:493-519. https://doi.org/10.1016/j.orcp.2016.01.004.
- French DP, Cameron E, Benton JS, Deaton C, Harvie M. Can communicating personalised disease risk promote healthy behaviour change? A systematic review of systematic reviews. Ann Behav Med 2017;51:718-29. https://doi.org/10.1007/s12160-017-9895-z.
- Weijer C, Grimshaw JM, Eccles MP, McRae AD, White A, Brehaut JC, et al. Ottawa Ethics of Cluster Randomized Trials Consensus Group . The Ottawa statement on the ethical design and conduct of cluster randomized trials. PLOS Med 2012;9. https://doi.org/10.1371/journal.pmed.1001346.
- Vickers AJ, Altman DG. Statistics notes: analysing controlled trials with baseline and follow up measurements. BMJ 2001;323:1123-4. https://doi.org/10.1136/bmj.323.7321.1123.
- Bellg AJ, Borrelli B, Resnick B, Hecht J, Minicucci DS, Ory M, et al. Enhancing treatment fidelity in health behavior change studies: best practices and recommendations from the NIH Behavior Change Consortium. Health Psychol 2004;23:443-51. https://doi.org/10.1037/0278-6133.23.5.443.
- Medical Research Council (MRC) . Guidance on the Development and Evaluation of Complex Interventions 2019.
- Hartmann-Boyce J, Boylan AM, Jebb SA, Fletcher B, Aveyard P. Cognitive and behavioural strategies for self-directed weight loss: systematic review of qualitative studies. Obes Rev 2017;18:335-49. https://doi.org/10.1111/obr.12500.
- Chan AW, Tetzlaff JM, Altman DG, Laupacis A, Gøtzsche PC, Krleža-Jerić K, et al. SPIRIT 2013 statement: defining standard protocol items for clinical trials. Ann Intern Med 2013;158:200-7. https://doi.org/10.7326/0003-4819-158-3-201302050-00583.
- Moore GF, Audrey S, Barker M, Bond L, Bonell C, Hardeman W, et al. Process evaluation of complex interventions: Medical Research Council guidance. BMJ 2015;350. https://doi.org/10.1136/bmj.h1258.
- Van Koperen TM, Jebb SA, Summerbell CD, Visscher TL, Romon M, Borys JM, et al. Characterizing the EPODE logic model: unravelling the past and informing the future. Obes Rev 2013;14:162-70. https://doi.org/10.1111/j.1467-789X.2012.01057.x.
- Public Health England . Guidance: Introduction to Logic Models. 2018.
- Bonell C, Jamal F, Melendez-Torres GJ, Cummins S. ‘Dark logic’: theorising the harmful consequences of public health interventions. J Epidemiol Community Health 2015;69:95-8. https://doi.org/10.1136/jech-2014-204671.
- Miller W, Rollnick S. Motivational Interviewing: Helping People Change. New York, NY: Guilford Press; 2012.
- Bobrow K, Farmer AJ, Springer D, Shanyinde M, Yu LM, Brennan T, et al. Mobile phone text messages to support treatment adherence in adults with high blood pressure (SMS – text adherence support [StAR]): a single-blind, randomized trial. Circulation 2016;133:592-600. https://doi.org/10.1161/CIRCULATIONAHA.115.017530.
- Pears S, Morton K, Bijker M, Sutton S, Hardeman W. VBI Programme Team . Development and feasibility study of very brief interventions for physical activity in primary care. BMC Public Health 2015;15. https://doi.org/10.1186/s12889-015-1703-8.
- Locock L, Smith L. Personal experiences of taking part in clinical trials – a qualitative study. Patient Educ Couns 2011;84:303-9. https://doi.org/10.1016/j.pec.2011.06.002.
- Solomon RL. An extension of control group design. Psychol Bull 1949;46:137-50. https://doi.org/10.1037/h0062958.
- Treweek S, Bevan S, Bower P, Campbell M, Christie J, Clarke M, et al. Trial forge guidance 1: what is a study within a trial (SWAT)?. Trials 2018;19. https://doi.org/10.1186/s13063-018-2535-5.
- Yusuf S, Collins R, Peto R. Why do we need some large, simple randomized trials?. Stat Med 1984;3:409-22. https://doi.org/10.1002/sim.4780030421.
- Efficace F, Fayers P, Pusic A, Cemal Y, Yanagawa J, Jacobs M, et al. Quality of patient-reported outcome reporting across cancer randomized controlled trials according to the CONSORT patient-reported outcome extension: a pooled analysis of 557 trials. Cancer 2015;121:3335-42. https://doi.org/10.1002/cncr.29489.
- Frison L, Pocock SJ. Repeated measures in clinical trials: analysis using mean summary statistics and its implications for design. Stat Med 1992;11:1685-704. https://doi.org/10.1002/sim.4780111304.
- European Medicines Agency . Adjustment for Baseline Covariates in Clinical Trials 2015. www.ema.europa.eu/en/adjustment-baseline-covariates-clinical-trials (accessed 6 July 2020).
- Darker CD, French DP. What sense do people make of a theory of planned behaviour questionnaire?: a think-aloud study. J Health Psychol 2009;14:861-71. https://doi.org/10.1177/1359105309340983.
- Webb EJ. Unobtrusive Measures: Nonreactive Research in the Social Sciences. Chicago, IL: Rand McNally; 1966.
- Farmer A, Williams V, Velardo C, Shah SA, Yu LM, Rutter H, et al. Self-management support using a digital health system compared with usual care for chronic obstructive pulmonary disease: randomized controlled trial. J Med Internet Res 2017;19. https://doi.org/10.2196/jmir.7116.
- Mackillop L, Hirst JE, Bartlett KJ, Birks JS, Clifton L, Farmer AJ, et al. Comparing the efficacy of a mobile phone-based blood glucose management system with standard clinic care in women with gestational diabetes: randomized controlled trial. JMIR Mhealth Uhealth 2018;6. https://doi.org/10.2196/mhealth.9512.
- Sheeran P, Harris PR, Epton T. Does heightening risk appraisals change people’s intentions and behavior? A meta-analysis of experimental studies. Psychol Bull 2014;140:511-43. https://doi.org/10.1037/a0033065.
- Kypri K, Wilson A, Attia J, Sheeran P, Miller P, McCambridge J. Social desirability bias in the reporting of alcohol consumption: a randomized trial. J Stud Alcohol Drugs 2016;77:526-31. https://doi.org/10.15288/jsad.2016.77.526.
- McCambridge J, Kypri K, Bendtsen P, Porter J. The use of deception in public health behavioral intervention trials: a case study of three online alcohol trials. Am J Bioeth 2013;13:39-47. https://doi.org/10.1080/15265161.2013.839751.
- British Psychological Society . Code of Human Research Ethics 2014.
- Economic and Social Research Council . Research Ethics n.d. https://esrc.ukri.org/funding/guidance-for-applicants/research-ethic/ (accessed 24 September 2020).
- Locock L, Smith L. Personal benefit, or benefiting others? Deciding whether to take part in clinical trials. Clin Trials 2011;8:85-93. https://doi.org/10.1177/1740774510392257.
- McCann SK, Campbell MK, Entwistle VA. Reasons for participating in randomised controlled trials: conditional altruism and considerations for self. Trials 2010;11. https://doi.org/10.1186/1745-6215-11-31.
- Gamble C, Krishan A, Stocken D, Lewis S, Juszczak E, Doré C, et al. Guidelines for the content of statistical analysis plans in clinical trials. JAMA 2017;318:2337-43. https://doi.org/10.1001/jama.2017.18556.
- Kinmonth AL, Wareham NJ, Hardeman W, Sutton S, Prevost AT, Fanshawe T, et al. Efficacy of a theory-based behavioural intervention to increase physical activity in an at-risk group in primary care (ProActive UK): a randomised trial. Lancet 2008;371:41-8. https://doi.org/10.1016/S0140-6736(08)60070-7.
- McCambridge J, Bendtsen M, Karlsson N, White IR, Nilsen P, Bendtsen P. Alcohol assessment and feedback by email for university students: main findings from a randomised controlled trial. Br J Psychiatry 2013;203:334-40. https://doi.org/10.1192/bjp.bp.113.128660.
- Kairalla JA, Coffey CS, Thomann MA, Muller KE. Adaptive trial designs: a review of barriers and opportunities. Trials 2012;13. https://doi.org/10.1186/1745-6215-13-145.
- Golembiewski TR, Billingsley K, Yeager S. Measuring change and persistence in human affairs: types of change GENERATED by OD designs. J Appl Behav Sci 1976;12:133-57. https://doi.org/10.1177/002188637601200201.
- Hoffmann TC, Glasziou PP, Boutron I, Milne R, Perera R, Moher D, et al. Better reporting of interventions: template for intervention description and replication (TIDieR) checklist and guide. BMJ 2014;348. https://doi.org/10.1136/bmj.g1687.
- Kvalem IL, Sundet JM, Rivø KI, Eilertsen DA, Bakketeig LS. The effect of sex education on adolescents’ use of condoms: applying the Solomon four-group design. Health Educ Q 1996;23:34-47. https://doi.org/10.1177/109019819602300103.
- Farmer A, Wade A, Goyder E, Yudkin P, French D, Craven A, et al. Impact of self monitoring of blood glucose in the management of patients with non-insulin treated diabetes: open parallel group randomised trial. BMJ 2007;335. https://doi.org/10.1136/bmj.39247.447431.BE.
- Day SJ, Altman DG. Statistics notes: blinding in clinical trials and other studies. BMJ 2000;321. https://doi.org/10.1136/bmj.321.7259.504.
Appendix 1 The MERIT Collaborative Group
Peter Bower, University of Manchester.
Stacy Clemes, University of Loughborough.
Mark Conner, University of Leeds.
Ruth Dundas, University of Glasgow.
Diana Elbourne, London School of Hygiene and Tropical Medicine.
Sandra Eldridge, Queen Mary University of London.
Andrew Farmer, University of Oxford.
David French, University of Manchester.
Carrol Gamble, University of Liverpool.
Martin Gulliford, King’s College London.
Frank Kee, Queen’s University Belfast.
Alastair Leyland, University of Glasgow.
Louise Locock, University of Aberdeen.
Rebecca Lynch, King’s College London.
Graeme MacLennan, University of Aberdeen.
Jim McCambridge, University of York.
Lisa Miles, University of Manchester.
Samuel CS Rowley, Medical Research Council.
Linda Sharples, London School of Hygiene and Tropical Medicine.
Falko F Sniehotta, Newcastle University.
Claire Snowdon, London School of Hygiene and Tropical Medicine.
Mirjam Sprangers, Amsterdam University Medical Centers.
Stephen Sutton, University of Cambridge.
Appendix 2 Example of an interaction between baseline measurement and an intervention in a Solomon four-group design
The Solomon four-group design study allows identification of an interaction between a study intervention and baseline assessment. This type of study was used to test the effectiveness of a sexual health intervention on condom use in young people. 23,110 Participants were upper secondary school students in Norway (n = 2088) who were aged between 16 and 20 years. The intervention was developed based on cognitive social learning theory and social influence theory, implemented in classrooms and delivered by teachers in collaboration with peer educators. The four arms of the trial received the following:
-
sexual health intervention and pre-test questionnaire
-
sexual health intervention and no pre-test questionnaire
-
no intervention (control) and pre-test questionnaire
-
no intervention (control) and no pre-test questionnaire.
The pre-test 80-item questionnaires were given out in class and completed at home in the month (or two) preceding implementation of the intervention. The majority of the questions concerned sexual behaviour. Follow-up measures of condom use were collected at 6 and 12 months. Study results included reports of an interaction effect in a subgroup of 403 participants who had their first intercourse prior to the study and who provided follow-up data after 6 months (Table 5). The study is limited in that it does not clearly specify whether or not this subgroup analysis was pre-planned. Nevertheless, all three other conditions were found to be distinct from the reference group of those who were pre-tested and received the intervention [odds ratios for condom use at most recent intercourse were 0.31, 0.42 and 0.41 (p = 0.005) or less for each comparison].
Intervention | Pre assessment, n/N (%) | |
---|---|---|
Yes | No | |
Yes | 51/73 (70) | 21/49 (43) |
No | 76/148 (51) | 69/133 (52) |
The study authors postulated several possible explanations for the appearance of this interaction effect. Participants who received the pre-test questionnaire and the intervention were most likely to use condoms. As suggested by the study authors,110 completion of the pre-test questionnaire in the month (or two) preceding the intervention may have made students more prepared for, or familiar with, the content of the intervention than students who started the intervention unprepared. The authors suggest that it is possible that answering all the questions in the pre-test made the students reflect more on their own sexual behaviour and therefore made them more aware of the problems and more receptive to the solutions discussed during the intervention, making the topic of the intervention more relevant to them personally.
Otherwise, the results of this study indicate that the intervention itself did not have any impact on use of condoms. In such circumstances it is clear that testing the effectiveness of this intervention in a standard RCT, assuming baseline assessments on sexual health behaviour take place, could result in an overestimation of the effectiveness of the intervention as a result of biases attributed to MR. The implications of such biases have implications for health-care decision-making based on trial evidence. It is conceivable that a sexual health intervention is rolled out on the basis of evidence of persuasive effect sizes reported in a trial yet the intervention, at a policy level, proves to be ineffective because the effectiveness of the intervention is dependent on an interaction with baseline assessments that does not take place when the intervention is implemented in practice.
Appendix 3 Search strategy for rapid review of systematic reviews
PsycINFO
General search strategy
(review OR meta-analy*) AND (measure* OR assess*) AND reactiv* NOT c-reactive.
Specific search strategy
(review OR meta-analy*) AND (“question-behaviour” OR “question-behavior” OR “mere measurement”).
PubMed
General search strategy
(review[Title/Abstract] OR meta-analy*[Title/Abstract]) AND (measure*[Title/Abstract] OR assess*[Title/Abstract]) AND reactiv*[Title/Abstract] NOT c-reactive[Title/Abstract].
Specific search strategy
(review[Title/Abstract] OR meta-analy*[Title/Abstract]) AND (“question-behaviour”[Title/Abstract] OR “question-behavior”[Title/Abstract] OR “mere measurement”[Title/Abstract]).
Cochrane Database of Systematic Reviews
General search strategy
As for PsycINFO.
(Note that, in the general search for PsycINFO and PubMed, the term reactiv* led to the retrieval of many irrelevant articles that referred to C-reactive protein. Therefore, the term NOT c-reactive was added to exclude these articles.)
Appendix 4 Overlap between four recent reviews of the question–behaviour effect
FIGURE 4.
Venn diagram to show overlap between four recent reviews of the QBE.
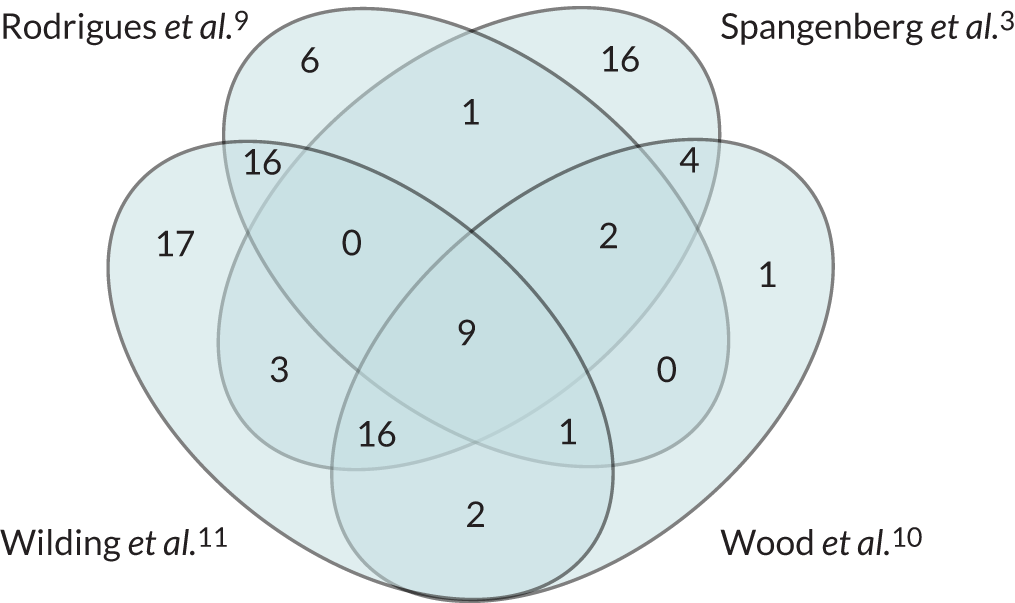
Details of the papers included in four recent reviews of the question–behaviour effect
For each paper, the review(s) in which the paper was included are indicated by numbers in square brackets as follows: 1 = Rodrigues et al. ;9 2 = Spangenberg et al. ;3 3 = Wood et al. ;10 and 4 = Wilding et al. 11
Ayres K, Conner M, Prestwich A, Hurling R, Cobain M, Lawton R, O’Connor DB. Exploring the question-behaviour effect: randomized controlled trial of motivational and question–behaviour interventions. Br J Health Psychol 2013;18:31–44. https://doi.org/10.1111/j.2044-8287.2012.02075.x [1, 3, 4]
Bendtsen P, McCambridge J, Bendtsen M, Karlsson N, Nilsen P. Effectiveness of a proactive mail-based alcohol Internet intervention for university students: dismantling the assessment and feedback components in a randomized controlled trial. J Med Internet Res 2012;14:e142. https://doi.org/10.2196/jmir.2062 [4]
Bernstein E, Edwards E, Dorfman D, Heeren T, Bliss C, Bernstein J. Screening and brief intervention to reduce marijuana use among youth and young adults in a pediatric emergency department. Acad Emerg Med 2009;16:1174–85. https://doi.org/10.1111/j.1553-2712.2009.00490.x [4]
Bernstein J, Heeren T, Edward E, Dorfman D, Bliss C, Winter M, Bernstein E. A brief motivational interview in a pediatric emergency department, plus 10-day telephone follow-up, increases attempts to quit drinking among youth and young adults who screen positive for problematic drinking. Acad Emerg Med 2010;17:890–902. https://doi.org/10.1111/j.1553-2712.2010.00818.x [1, 4]
Berry TR, Carson V. Ease of imagination, message framing, and physical activity messages. Br J Health Psychol 2010;15:197–211. [1]
Borle S, Dholakia UM, Singh SS, Westbrook RA. The impact of survey participation on subsequent customer behavior: an empirical investigation. Mark Sci 2007;26:711–26. [2]
Carey KB, Carey MP, Maisto SA, Henson JM. Brief motivational interventions for heavy college drinkers: a randomized controlled trial. J Consult Clin Psychol 2006;74:943–54. [1, 4]
Chandon P, Morwitz VG, Reinartz WJ. The short- and long-term effects of measuring intent to repurchase. J Consum Res 2004;31:566–72. [2, 3, 4]
Chandon P, Smith RJ, Morwitz VG, Spangenberg ER, Sprott DE. When does the past repeat itself? The interplay of behaviour prediction and personal norms. J Consum Res 2011;38:420–30. [2, 3]
Chapman KJ. Questionnaire Effects on Behavior: Mere Measurement Effects and the Accessibility of Attitudes and Intentions. Unpublished PhD thesis. Boulder, CO: University of Colorado Boulder; 1996. [2]
Chapman K J. Measuring intent: there’s nothing ‘mere’ about mere measurement effects. Psychol Mark 2001;18:811–41. [2, 3, 4]
Cherpitel CJ, Korcha RA, Moskalewicz J, Swiatkiewicz G, Ye Y, Bond J. Screening, brief intervention, and referral to treatment (SBIRT): 12-month outcomes of a randomized controlled clinical trial in a Polish emergency department. Alcohol Clin Exp Res 2010;34:1922–8. https://doi.org/10.1111/j.1530-0277.2010.01281.x [1, 4]
Cioffi D, Garner R. The effect of response options on decisions and subsequent behavior: sometimes inaction is better. Pers Soc Psychol Bull 1998;24:463–72. [1, 2, 3, 4]
Clifford PR, Maisto SA, Davis CM. Alcohol treatment research assessment exposure subject reactivity effects: part I. Alcohol use and related consequences. J Stud Alcohol Drugs 2007;68:519–28. https://doi.org/10.15288/jsad.2007.68.519 [1]
Conner M, Godin G, Norman P, Sheeran P. Using the question–behavior effect to promote disease prevention behaviors: two randomized controlled trials. Health Psychol 2011;30:300–9. https://doi.org/10.1037/a0023036 [1, 2, 3, 4]
Conner M, Sandberg T, Norman P. Using action planning to promote exercise behavior. Ann Behav Med 2010;40:65–76. https://doi.org/10.1007/s12160-010-9190-8 [2]
Cox AD, Cox D, Cyrier R, Graham-Dotson Y, Zimet GD. Can self-prediction overcome barriers to hepatitis B vaccination? A randomized controlled trial. Health Psychol 2012;31:97–105. https://doi.org/10.1037/a0025298 [3, 4]
Daeppen JB, Gaume J, Bady P, Yersin B, Calmes JM, Givel JC, Gmel G. Brief alcohol intervention and alcohol assessment do not influence alcohol use in injured patients treated in the emergency department: a randomized controlled clinical trial. Addiction 2007;102:1224–33. [1, 4]
Dholakia UM, Singh SS, Westbrook RA. Understanding the effects of post-service experience surveys on delay and acceleration of customer purchasing behavior: Evidence from the automotive services industry. J Serv Res 2010;13:362–78. [4]
Dholakia UM, Morwitz VG. The scope and persistence of mere-measurement effects: evidence from a field study of customer satisfaction measurement. J Consum Res 2002;29:159–67. [2, 4]
Dholakia UM, Morwitz VG, Westbrook RA. Firm-sponsored satisfaction surveys: positivity effects on customer purchase behavior? MSI Reports. Work Pap Ser 2004;4:95–112. [2]
Dignan M, Michielutte R, Blinson K, Wells HB, Case LD, Sharp P, et al. Effectiveness of health education to increase screening for cervical cancer among eastern-band Cherokee Indian women in North Carolina. J Natl Cancer Inst 1996;88:1670–6. https://doi.org/10.1093/jnci/88.22.1670 [1, 4]
Dignan MB, Michielutte R, Wells HB, Sharp P, Blinson K, Case LD, et al. Health education to increase screening for cervical cancer among Lumbee Indian women in North Carolina. Health Educ Res 1998;13:545–56. https://doi.org/10.1093/her/13.4.545 [1, 4]
Falk B. Do drivers become less risk-prone after answering a questionnaire on risky driving behaviour? Accid Anal Prev 2010;42:235–44. [4]
Fitzsimons GJ, Shiv B. Nonconscious and contaminative effects of hypothetical questions on subsequent decision making. J Consum Res 2001;28:224–38. [2]
Fitzsimons GJ, Williams P. Asking questions can change choice behavior: does it do so automatically or effortfully? J Exp Psychol Appl 2000;6:195–206. [2, 3]
Fitzsimons G, Block LG, Williams P. Asking questions about vices really does increase vice behavior. Soc Influ 2007;2:237–43. [2]
Fitzsimons GJ, Nunes JC, Williams P. License to sin: the liberating role of reporting expectations. J Consum Res 2007;34:22–31. https://doi.org/10.1086/513043 [2, 3, 4]
Fitzsimons GJ, Moore SG. Should we ask our children about sex, drugs and rock & roll? Potentially harmful effects of asking questions about risky behaviors. J Consum Psychol 2008;18:82–95. https://doi.org/10.1016/j.jcps.2008.01.002 [4]
Gerber AS, Green DP. Correction to Gerber and Green (2000), replication of disputed findings, and reply to Imai (2005). Am Polit Sci Rev 2005;99:301–13. [2]
Gerber AS, Green DP. Do phone calls increase voter turnout? An update. An Am Acad Pol Soc Sci 2005;601:142–54. [2]
Godin G, Bélanger-Gravel A, Amireault S, Vohl MC, Pérusse L. The effect of mere-measurement of cognitions on physical activity behavior: a randomized controlled trial among overweight and obese individuals. Int J Behav Nutr Phys Act 2011;8:2. https://doi.org/10.1186/1479-5868-8-2 [1, 2, 3, 4]
Godin G, Sheeran P, Conner M, Delage G, Germain M, Bélanger-Gravel A, Naccache H. Which survey questions change behavior? Randomized controlled trial of mere measurement interventions. Health Psychol 2010;29:636–44. https://doi.org/10.1037/a0021131 [1, 2, 3]
Godin G, Sheeran P, Conner M, Germain M. Asking questions changes behavior: mere measurement effects on frequency of blood donation. Health Psychol 2008;27:179–84. https://doi.org/10.1037/0278-6133.27.2.179 [1, 2, 3, 4]
Godin G, Amireault S, Vézina-Im LA, Sheeran P, Conner M, Germain M, Delage G. Implementation intentions intervention among temporarily deferred novice blood donors. Transfusion 2013;53:1653–60. https://doi.org/10.1111/j.1537-2995.2012.03939.x [4]
Goldstein DG, Imai K, Göritz AS, Gollwitzer PM. Nudging Turnout: Mere Measurement and Implementation Planning of Intentions to Vote. Unpublished manuscript. London: London Business School; 2008. [4]
Greenwald AG, Carnot CG, Beach R, Young B. Increasing voting behavior by asking people if they expect to vote. J App Psychol 1987;72:315–18. [2, 3, 4]
Greenwald AG, Klinger MR, Vande Kamp ME, Kerr KL. The Self-Prophecy Effect: Increasing Voter Turnout by Vanity-Assisted Conscious Raising. Unpublished manuscript. 1988. [2, 4]
Janiszewski C, Chandon, E. Transfer-appropriate processing response fluency, and the mere measurement effect. J Mark Res 2007;44:309–23. [2, 3, 4]
Krauss BJ, Goldsamt L, Bula E, Godfrey C, Yee DS, Palij M. Pretest assessment as a component of safer sex intervention: a pilot study of brief one-session interventions for women partners of male injection drug users in New York City. J Urban Health 2000;77:383–95. [1]
Kraut RE, McConahay JB. How being interviewed affects voting: an experiment. Public Opin Quart 1973;37:398–406. [2]
Kvalem IL, Sundet JM, Rivø KI, Eilertsen DA, Bakketeig LS. The effect of sex education on adolescents’ use of condoms: applying the Solomon four-group design. Health Educ Q 1996;23:34–47. https://doi.org/10.1177/109019819602300103 [1, 4]
Kypri K, Langley JD, Saunders JB, Cashell-Smith ML. Assessment may conceal therapeutic benefit: findings from a randomized controlled trial for hazardous drinking. Addiction 2007;102:62–70. [1, 4]
Kypri K, McAnally HM. Randomized controlled trial of a web-based primary care intervention for multiple health risk behaviors. Prev Med 2005;41:761–6. [1, 4]
Lawrence C, Ferguson E. The role of context stability and behavioural stability in the mere measurement effect: an examination across six behaviours. J Health Psychol 2012;17:1041–52. https://doi.org/10.1177/1359105311433346 [3, 4]
Levav J, Fitzsimons GJ. When questions change behavior: the role of ease of representation. Psychol Sci 2006;17:207–13. [1, 2, 3, 4]
Liu W, Aaker J. The happiness of giving: the time–ask effect. J Consum Res 2008;35:543–57. [2]
Manstead AS, Proffitt C, Smart JL. Predicting and understanding mothers’ infant-feeding intentions and behavior: testing the theory of reasoned action. J Pers Soc Psychol 1983;44:657–71. https://doi.org/10.1037//0022-3514.44.4.657 [3]
McCambridge J, Day M. Randomized controlled trial of the effects of completing the Alcohol Use Disorders Identification Test questionnaire on self-reported hazardous drinking. Addiction 2008;103:241–8. https://doi.org/10.1111/j.1360-0443.2007.02080.x [1, 4]
McCambridge J, Bendtsen M, Karlsson N, White IR, Nilsen P, Bendtsen P. Alcohol assessment and feedback by email for university students: main findings from a randomised controlled trial. Br J Psychiatry 2013;203:334–40. https://doi.org/10.1192/bjp.bp.113.128660 [4]
Milton AC, Mullan BA. An application of the theory of planned behavior – a randomized controlled food safety pilot intervention for young adults. Health Psychol 2012;31:250–9. https://doi.org/10.1037/a0025852 [4]
Moreira MT, Oskrochi R, Foxcroft DR. Personalised normative feedback for preventing alcohol misuse in university students: Solomon three-group randomised controlled trial. PLOS ONE 2012;7:e44120. https://doi.org/10.1371/journal.pone.0044120 [1, 4]
Morwitz VG, Fitzsimons GJ. The mere-measurement effect: why does measuring intentions change actual behavior? J Consum Psychol 2004;14:64–73. [2, 3, 4]
Morwitz VG, Johnson E, Schmittlein D. Does measuring intent change behavior? J Consum Res 1993;20:46–61. [2, 3, 4]
Murray M, Swan AV, Kiryluk S, Clarke GC. The Hawthorne effect in the measurement of adolescent smoking. J Epidemiol Community Health 1988;42:304–6. [4]
Nickerson DW, Rogers T. Do you have a voting plan? Implementation intentions, voter turnout, and organic plan making. Psychol Sci 2010;21:194–9. https://doi.org/10.1177/0956797609359326 [4]
Obermiller C, Spangenberg E. Improving telephone fundraising by use of self-prophecy. Int J Nonprofit Volunt Sect Market 2000;5:365–72. [2, 3, 4]
Obermiller C, Spangenberg ER, Atwood A. Getting people to give more: a telephone funds-soliciting strategy based on the self-erasing nature of errors of prediction. Mark Theory Appl 1992;3:339–45. [2, 3]
Ofir C, Simonson I. The effect of stating expectations on customer satisfaction and shopping experience. J Market Res 2007;44:164–74. [2]
O’Sullivan I, Orbell S, Rakow T, Parker R. Prospective research in health service settings: health psychology, science and the ‘Hawthorne’ effect. J Health Psychol 2004;9:355–9. https://doi.org/10.1177/1359105304042345 [1, 2]
Perkins A, Smith RJ, Sprott DE, Spangenberg ER, Knuff DC. Understanding the self-prophecy phenomenon. Eur Adv Consum Res 2008;8:462–7. [4]
Peter J, Valkenburg PM. Do questions about watching Internet pornography make people watch Internet pornography? A comparison between adolescents and adults. Int J Public Opin Res 2012;24;400–10. [4]
Richmond R, Heather N, Wodak A, Kehoe L, Webster I. Controlled evaluation of a general practice-based brief intervention for excessive drinking. Addiction 1995;90:119–32. https://doi.org/10.1046/j.1360-0443.1995.90111915.x [4]
Rimer B, Levy MH, Keintz MK, Fox L, Engstrom PF, MacElwee N. Enhancing cancer pain control regimens through patient education. Patient Educ Couns 1987;10:267–77. [1]
Sandberg T, Conner M. A mere measurement effect for anticipated regret: Impacts on cervical screening attendance. Br J Soc Psychol 2009;48:221–36. [1, 2, 3, 4]
Sandberg T, Conner M. Using self-generated validity to promote exercise behaviour. Br J Soc Psychol 2011;50:769–83. https://doi.org/10.1111/j.2044-8309.2010.02004.x [1]
Sherman SJ. On the self-erasing nature of errors of prediction. J Pers Soc Psychol 1980;39:211–21. [2, 3, 4]
Smith JK, Gerber AS, Orlich A. Self-prophecy effects and voter turnout: an experimental replication. Polit Psychol 2003;24:593–604. [2, 3, 4]
Spangenberg E. Increasing health club attendance through selfprophecy. Mark Lett 1997;8:23–31. [1, 2, 3]
Spangenberg ER, Greenwald AG. Social influence by requesting self-prophecy. J Consum Psychol 1999;8:61–89. [2, 3, 4]
Spangenberg E, Obermiller C. To cheat or not to cheat: reducing cheating by requesting self-prophecy. Market Educ Rev 1996;6:95–103. [2, 3, 4]
Spangenberg ER, Sprott DE. Self-monitoring and susceptibility to the influence of self-prophecy. J Consum Res 2006;32:550–6. [2, 3, 4]
Spangenberg ER, Sprott DE, Grohmann B, Smith RJ. Mass-communicated prediction requests: practical application and a cognitive dissonance explanation for self-prophecy. J Mark 2003;67:47–62. [2]
Spangenberg ER, Sprott DE, Obermiller C, Greenwald AG. Dissonance and the Question–Behavior Effect: Theoretical Evidence for Self-Prophecy. Unpublished paper. 2012. [2]
Spangenberg ER, Sprott DE, Knuff DC, Smith RJ, Obermiller C, Greenwald AG. Process evidence for the question–behaviour effect: influencing socially normative behaviors. Soc Influ 2012;7:211–28. [2]
Spence JC, Burgess J, Rodgers W, Murray T. Effect of pretesting on intentions and behaviour: a pedometer and walking intervention. Psychol Health 2009;24:777–89. https://doi.org/10.1080/08870440801989938 [1, 2, 3, 4]
Sprott DE, Smith RJ, Spangenberg ER, Freson TS. Specificity of prediction requests: evidence for the differential effects of self-prophecy on commitment to a health assessment. J Appl Soc Psychol 2004;34:1176–90. [1, 2, 3, 4]
Sprott DE, Spangenberg ER, Fisher R. The importance of normative beliefs to the self-prophecy effect. J Appl Psychol 2003;88:423–31. https://doi.org/10.1037/0021-9010.88.3.423 [1, 2, 3, 4]
Sprott DE, Spangenberg ER, Devezer B, Zidansek M. Gender and the Question–Behavior Effect: Evidence of Moderation From Two Experiments. Unpublished paper. 2012. [2]
Sprott DE, Spangenberg ER, Perkins AW. Two More Self-Prophecy Experiments. Paper presented at Advances in Consumer Research, Provo, UT, 1999. [2, 3]
Todd J, Mullan B. Using the theory of planned behaviour and prototype willingness model to target binge drinking in female undergraduate university students. Addict Behav 2011;36:980–6. https://doi.org/10.1016/j.addbeh.2011.05.010 [1, 4]
Træen B. Effect of an intervention to prevent unwanted pregnancy in adolescents. A randomized, prospective study from Nordland County, Norway, 1999–2001. J Community Appl Soc Psychol 2003;13:207–23. [4]
van Dongen A, Abraham C, Ruiter RA, Veldhuizen IJ. Does questionnaire distribution promote blood donation? An investigation of question–behavior effects. Ann Behav Med 2013;45:163–72. https://doi.org/10.1007/s12160-012-9449-3 [1, 4]
van Kerckhove A, Geuens M, Vermeir I. A motivational account of the question–behavior effect. J Consum Res 2012;39:111–27. [2, 3, 4]
van Kerckhove A, Geuens M, Vermeir I. Intention superiority perspectives on preference–decision consistency. J Bus Res 2012;65:692–700. [2, 3, 4]
van Sluijs EM, van Poppel MN, Twisk JW, van Mechelen W. Physical activity measurements affected participants’ behavior in a randomized controlled trial. J Clin Epidemiol 2006;59:404–11. [1, 4]
van Valkengoed IGM, Morré SA, Meijer CJLM, van den Brule AJC, Boeke AJP. Do questions on sexual behaviour and the method of sample collection affect participation in a screening programme for asymptomatic Chlamydia trachomatis infections in primary care? Int J STD AIDS 2002;13:36–38. [1, 4]
Walters ST, Vader AM, Harris TR, Jouriles EN. Reactivity to alcohol assessment measures: an experimental test. Addiction 2009;104:1305–10. https://doi.org/10.1111/j.1360-0443.2009.02632.x [1, 4]
Williams P, Fitzsimons GJ, Block LG. When consumers do not recognize ‘benign’ intention questions as persuasion attempts. J Consum Res 2004;31:540–51. [2, 3, 4]
Williams P, Block LG, Fitzsimons GJ. Simply asking questions about health behaviors increases both healthy and unhealthy behaviors. Soc Influ 2006;1:117–27. [2, 3, 4]
Wood C, Conner M, Sandberg T, Godin G, Sheeran P. Why does asking questions change health behaviours? The mediating role of attitude accessibility. Psychol Health 2014;29:390–404. https://doi.org/10.1080/08870446.2013.858343. [4]
Yalch RF. Pre-election interview effects on voter turnout. Public Opin Q 1976;40:331–6. [2]
Yardley L, Miller S, Schlotz W, Little P. Evaluation of a Web-based intervention to promote hand hygiene: exploratory randomized controlled trial. J Med Internet Res 2011;13:e107. https://doi.org/10.2196/jmir.1963 [1]
Young SD, Adelstein BD, Ellis SR. Demand characteristics in assessing motion sickness in a virtual environment: or does taking a motion sickness questionnaire make you sick? IEEE Trans Vis Comput Graph 2007;13:422–8. [4]
Appendix 5 Explanatory guide to support Table 4
Participant selection
The target population for a trial may not be homogeneous in terms of propensity to exhibit reactivity to measurement. Consequently, recruitment processes might influence the risk of bias from MR. In general, studies with less restrictive eligibility criteria and studies that employ population- or registry-based recruitment are likely to be at lower risk of bias from MR than studies that include volunteer participants who may have particular motivations to engage with the trial, including the interventions and trial measures. For example, participants with greater health awareness and knowledge (e.g. health ‘enthusiasts’ who are able to understand or interpret the meaning of study measures) might be more likely to react to measurement.
Systematic reviews that have investigated the QBE have included some moderator analyses that lend support to the idea that some population groups might be more prone to MR than others. 9–11 Specifically, one systematic review11 reported significantly larger effect sizes for the QBE in studies using student samples than in studies using health-care patients, school pupils, employees or other samples. In addition, two further systematic reviews9,10 reported greater effect sizes for the QBE in student participants than in non-student study participants.
Measurements
Features of health outcome of interest
Participants’ knowledge of the study research question, and therefore the health-related outcome of interest, can predispose them to MR. For example, a question about extent of physical activity may alert a study participant to the purpose of an intervention (i.e. to increase physical activity) and, even in a non-intervention comparator group, lead to efforts to become more active. In some cases, masking of measurements may be used to disguise the study aims from research participants and therefore reduce the risk of MR. This was shown in a study of pedometer use55 in which study participants were informed that they were wearing ‘posture monitors’ as a method of masking the purpose of the device. The devices were then unmasked and participants were told to continue to wear the pedometer knowing what it was. Mean daily step counts reported in the unmasked condition (at 1 week) were significantly higher than those recorded in the masked condition (at 1 week). This implied that participants wearing unmasked pedometers were more likely to change their behaviour in response to measurement.
Evidence to support MR is heavily reliant on the literature on the QBE. Several systematic reviews have been published that suggest a small but important QBE. 3,9–11 These have all focused on health-related behavioural outcomes. Accordingly, current knowledge suggests that trials with health-related behaviours as outcomes of interest are most at risk of bias from MR. Although not investigated to the same extent, there is also evidence that completing health-focused questionnaires increases anxiety,19 and so study outcomes related to anxiety are also likely to be at risk of bias from MR.
It is plausible that health-related outcomes with well-recognised social norms (e.g. body weight) might be at greater risk of MR. 77 Moderator analyses in a systematic review10 lend support to this (i.e. social desirability influenced the magnitude of the QBE). More socially desirable behaviours were associated with a larger effect size for the QBE.
Whether a trial is focusing on improving health-promoting behaviours (such as physical activity, screening attendance) or reducing risky health-related behaviours is also likely to influence the potential for MR. One systematic review10 reported that effect sizes for studies targeting risky behaviours were significantly smaller than for studies targeting health-promoting behaviours. Similarly, another systematic review9 reported the largest QBEs for dental flossing, physical activity and screening attendance, which are all health-promoting behaviours. Findings from a systematic review of brief alcohol interventions8 also support the idea that risky health behaviours are less likely to be affected by MR. There was no statistical difference in daily or weekly alcohol consumption between groups receiving or not receiving questions on drinking behaviour in brief alcohol intervention trials.
Follow-up
Studies in which a variable is measured at interim time points, even when the measurement is balanced across groups, are at risk of MR bias, either directly if the results of measurements are disclosed or indirectly through the process of measurement. For example, there is good evidence of effects on behaviour when questionnaires assessing hypothesised social cognitive determinants of behaviour are administered. 9,11
The relationship between length of time between baseline and follow-up measurements and the duration of potential reactivity to baseline measurements is also an important consideration. It is possible that reactive effects of measurement are short term,56 that is people change their behaviour in response to measurement when the measurement procedure or tool has ‘novelty value’, after which behaviour gradually returns to normal. Similarly, there is evidence that subjective reports of thoughts, feelings and behaviours are subject to an initial elevation bias20 whereby reports of outcomes decline over time.
Systematic reviews of the QBE support the view that a longer time interval between baseline measurement (questionnaires) and assessment of outcome is associated with a smaller QBE. 10,11 Therefore, trials with a shorter follow-up period are at greater risk of bias from MR than those with a longer follow-up period. Consequently, short-term MR is likely to be of little concern for studies with a long follow-up of several months or years, even if present. However, there is likely to be grounds for concern in a situation where measurement reactions go on for several days and follow-up measurements are taken 1–2 weeks after baseline measurements.
Features of measurement procedures/tools
Equivalence of measurement procedures across trial arms
Concerns about MR are most acute when the process or content of measurement is not balanced across the arms of a randomised trial. Any study in which measurement is unbalanced across trial arms is potentially at risk of measurement bias. Unbalanced measurement often arises from a desire to measure the impact of an intervention (e.g. use of a digital device intended to improve care) that is evaluated in the context of an unblinded clinical trial.
An example of an unbalanced design at risk of MR would be a study investigating blood glucose self-management that compares an intervention using a blood glucose measuring device with usual care (i.e. no device). Such a study could include a self-completion measure intended to understand how use of the device might lead to changes in care. The use of such a questionnaire in only the intervention arm could draw attention to aspects of the intervention (how to make better use of the device), which could affect subsequent behaviour and thus might lead to changes in outcomes arising from the process of measurement.
Similarity between measurement and behaviour change techniques
Study measures that mimic other BCTs might enhance (or diminish) response to an intervention. Some measurement techniques are similar, if not identical, to techniques that are designed to change health-related behaviour. For example, the use of pedometers to measure behaviour also appears to change behaviour because when unsealed, they allow people to self-monitor their behaviour. 12,13 A standardised taxonomy of BCTs16 identifies 93 distinct techniques for changing behaviour and several, including self-monitoring, appear analogous to measurement procedures. When such measurement techniques are used, there is the potential for bias because, in effect, both experimental groups are receiving behaviour change interventions by virtue of the measurement techniques employed (see Box 5).
Source of data
The collection of new data specifically for the purposes of conducting the study under consideration is at greater risk of MR bias than use of existing data that were not collected primarily for research purposes. Examples include data in routine health records or existing data collected for other purposes (e.g. national health surveys, consumer purchasing data, transport usage data such as cycle counter statistics). The use of unobtrusive measures has long been recommended to avoid problems of measurement affecting participants in research studies. Similarly, the threat of bias due to MR is minimised when information from routinely collected data is used instead of new data collected specifically for the purposes of the trial.
Measurements open to subjectivity
Use of self-report measurements in a trial is more likely to lead to risk of MR. The act of completing a self-report measure by a research participant provides information to the participant about the study’s health-related variable(s) of interest (e.g. alcohol intake, smoking status). Furthermore, the measured values are self-disclosed by the participant (e.g. 12 alcohol drinks per week, four cigarettes smoked per day), and so the participant becomes aware of their own health status and may change their thoughts, emotions or behaviour as a result. Systematic reviews support the idea that measuring hypothesised determinants of behaviour can produces changes in that behaviour,9,11 and there is also evidence that asking people questions about beliefs can prompt the formation of beliefs. 93
This does not mean that all objective measures are without risk of bias from MR. This depends on the nature of the objective measure and whether or not research participants are aware of, and understand, the health-related variables of interest and the measured values. Although it is an objective measure of physical activity, using an unsealed pedometer as a measure of physical activity also provides the participant with information about the health-related behaviour of interest (i.e. physical activity) and the measured values are disclosed because the number of steps taken per day is visible on the device. The use of unsealed pedometers is therefore subject to a high risk of MR. In other situations, objective measures of health-related variables could be at low risk of MR. For example, blood samples can be taken to measure blood cholesterol level. It is possible that how the blood sample is used to assess health is concealed from the research participant (i.e. they know that blood is taken, but not that blood cholesterol is going to be measured) and it is possible that measured values are not disclosed to the participant. In such circumstances the risk of MR is likely to be low.
Studies that involve unobtrusive measurement of data, or use data collected without participant awareness (possibly collected through electronic media) or using masked measurement techniques, will generally be associated with lower risk of MR bias.
Disclosure of measured values to participants
The results of measurements conducted on research participants may or may not be disclosed (via feedback) to those research participants. Receiving feedback about clinical measurements from a study is important for some, if not many, participants. For example, people with diabetes welcome the results of blood tests for blood glucose levels taken at intervals. 111 Furthermore, there is also evidence that study participants wearing sealed pedometers show lower daily step counts than participants with unsealed pedometers. 13 This implies that participants wearing unsealed pedometers are more likely to change their behaviour in response to measurement.
Potential for MR is greater when measured values are disclosed to research participants either at the time of measurement or shortly afterwards. In the case of self-reported measures, these values are obviously immediately available to the research participants as they produce them. Some objective measures also have the potential for MR if disclosed, for example if a research participant receives feedback on their body weight or their steps per day (from an unsealed pedometer). In some cases, feedback can be deliberately withheld to reduce the risk of reactivity (e.g. use of a sealed pedometer). 13 In considering the potential effects of disclosure of research measurements to participants it is important to think about all aspects of the research process and participants’ understanding and familiarity with the measured values. As well as values disclosed during the trial, measured values disclosed during assessment of participants’ eligibility for taking part in a trial can have an impact (e.g. when study participants are selected based on health status).
Burden of measurement task
The burden of a measurement task for a research participant is an important factor when considering potential for MR and bias. In general, the more onerous a measurement task is for a research participant,the more likely it is that they will change their emotions, thoughts or behaviour as a result. The time commitment required of a research participant is likely to have an impact. For example, measurements that require a person to travel to a health-care setting for an appointment may allow time for contemplation about the measurement. Conversely, completion of a question on a mobile phone might barely interrupt a person’s day and so there is little opportunity to think about the measurement. Similarly, measurement tasks that require activities to be recorded (e.g. food diaries for recording daily body weight) can be burdensome for research participants and may be more likely to lead to measurement reactions than tasks that require little time and effort from participants.
Complexity of measurement task
In general, the more complex a measurement task is for a research participant, the more likely it is to result in the participant changing their emotions, thoughts or behaviour. The amount of interaction with research personnel can be thought of as a component of complexity of a measurement task. Physiological measures taken in a clinic, for example, involve much more interaction with research personnel than completion of a questionnaire at home. The physical requirements of a measurement task can also influence potential for reactivity. For example, providing a urine sample is simpler than taking part in a cardiorespiratory fitness test.
Measurement framed in terms of goals/targets
The wording of items in self-report questionnaires has the potential to inform participants about reference ranges. For example, participants might be asked about their alcohol intake in terms of consuming > 14 units or ≤ 14 units of alcohol per week. Such wording implicitly informs participants that 14 units per week is an upper limit for recommended alcohol intake. The participant then, simply by completing the question, has an impression of whether they are drinking above or below recommended levels and might change their thoughts, emotions or behaviour as a result. Similarly, another factor potentially leading to inadvertent setting of goals for study participants is through feedback of measured values. If, for example, a participant receives feedback that their total blood cholesterol is 3.5 mmol/l and this falls within the normal range, then the inclusion of the reference range provides information about health risk to study participants. This has potential consequences for the participants’ health-related thoughts, emotions or behaviour.
Context
The context and the type of setting (e.g. clinic, community, online, at home) in which measurement takes place are important variables for consideration. The setting often influences the amount and intensity of participant interaction with research personnel during measurements. When possible, embedding study measurement procedures onto routine clinical procedures is advantageous in terms of reducing the risk of measurement reactions (see Chapter 4, Recommendation 10: embed measurement procedures into routine clinical practice when possible). Systematic review evidence suggests that measurements taken in laboratory settings are more likely to lead to reactivity. One systematic review11 reported that laboratory-based QBE studies produced the largest overall effect on cognitions or behaviour and that the effect size was significantly greater than those observed in medical, community and online settings. Similarly, a further systematic review10 reported the QBE to be significantly stronger in laboratory settings than in field settings. It is important not only to consider the potential impact of context or setting of measurements in all aspects of the trial but also, as previously discussed, to maintain consistency in methods employed across trial arms.
Interventions and comparators
Nature of the intervention
Self-management and self-monitoring interventions are intended to change trial participant behaviour. However, it is important that baseline or interim measurements collected during the trial do not replicate the intervention, inadvertently delivering it to the control or comparison group. For example, if a questionnaire repeatedly asks about consumption of ‘five a day’ fruit and vegetable intake then this can convey a behavioural goal. Similarly, if participants are then repeatedly asked about their fruit and vegetable intake, this can encourage self-monitoring of a behaviour that many participants may previously have not devoted much attention to.
The dual use of measurement to track outcomes and to guide delivery of components of an intervention is a circumstance in which bias from MR is likely to be a risk. For instance, the use of tracking or self-monitoring data as an outcome and also a behavioural goal is likely to be problematic, as is using self-weighing as an intervention component and also as a measurement procedure. It is important to make a clear distinction between measurement procedures that are part of the intervention and those used for evaluative purposes.
Blinding to arm allocation
Blinding is normally employed in trials to conceal trial arm allocation to reduce differential treatment and assessment of participants. This enables assessment of patient outcomes to be completed without knowledge of the treatment received. Blinding is especially important when subjective outcome measures are employed. 112
Most studies of behavioural interventions are open studies in which trial arm allocation cannot be concealed. Lack of blinding may contribute to conditions in which MR can develop because of interactions between measurements and interventions. For example, those participants in the intervention arm of a trial may receive measurements of blood glucose or hypertension and receive feedback on these measurements, potentially alongside a discussion with a nurse or other health-care professional, which may increase motivation to take prescribed medicine or modify other health-related behaviours.
Process evaluation
Process evaluation in a trial focuses on evaluating the delivery of trial interventions. 79 A process evaluation may ask how interventions are delivered, what mechanisms account for intervention effects and how external factors influence the delivery and impact of interventions. Process evaluations typically employ mixed methods that combine qualitative and quantitative elements. These elements may include quantitative measures of mechanism, qualitative interviews with trial participants or fidelity assessments with those providing the intervention. Such assessments are often unbalanced across trial arms and may contribute to bias by heightening participants’ awareness of research participation and the objectives of a trial, with potential impact on trial outcome assessment. If process evaluation measures are conducted in only one arm of the trial, then MR is more likely to cause bias, because measurement procedures are no longer equivalent across trial arms.
It is fairly common for a process evaluation to aim to assess mechanisms that account for intervention effects (i.e. when specific measures are included to directly assess mechanisms of action of the primary outcome, this creates a greater risk of bias from MR). 9,11 Qualitative investigations can occur before the collection of the primary outcome. Such investigations may be particularly likely to produce bias because they are likely to be quite intensive and possibly more memorable to many research participants than the intervention itself, especially for trials with minimal interventions. The overall amount of measurement conducted as part of the process evaluation is also an important consideration. In line with recommendation 9 (see Chapter 4, Recommendation 9: consider possible measurement reactivity when determining the overall burden of measurement in a trial), from a perspective of MR bias, less measurement in trials would be preferable. Therefore, process evaluations that involve extensive measurement of data in all study participants are particularly prone to bias from MR.
Glossary
- Behaviour change technique
- A systematic procedure included as an active component of an intervention designed to change behaviour.
- Bias
- Systematic deviation of results or inferences, leading to results or conclusions that are systematically (as opposed to randomly) different.
- Logic model
- A graphic that represents the theory of how an intervention produces its outcomes. It represents, in a simplified way, a hypothesis or ‘theory of change’ about how an intervention works.
- Measurement reactivity
- The response of a study participant to the act of being measured. It can take the form of a change in behaviour, emotions or self-provided data.
- Question–behaviour effect
- When the act of asking questions about behaviour produces changes in the behaviour being asked about.
- Study within a trial
- A self-contained research study that has been embedded within a host trial with the aim of evaluating or exploring alternative ways of delivering or organising a particular trial process.
List of abbreviations
- AMSTAR 2
- A MeaSurement Tool to Assess systematic Reviews version 2
- BCT
- behaviour change technique
- CI
- confidence interval
- CONSORT
- Consolidated Standards of Reporting Trials
- MERIT
- MEasurement Reactions In Trials
- MR
- measurement reactivity
- MRC
- Medical Research Council
- NIHR
- National Institute for Health Research
- QBE
- question–behaviour effect
- RCT
- randomised controlled trial
- SOP
- standard operating procedure
- SWAT
- study within a trial
- TPB
- theory of planned behaviour