Notes
Article history
The research reported in this issue of the journal was commissioned and funded by the Evidence Synthesis Programme on behalf of NICE as project number NIHR127852. The contractual start date was in November 2018. The draft report began editorial review in March 2020 and was accepted for publication in May 2021. The authors have been wholly responsible for all data collection, analysis and interpretation, and for writing up their work. The HTA editors and publisher have tried to ensure the accuracy of the authors’ report and would like to thank the reviewers for their constructive comments on the draft document. However, they do not accept liability for damages or losses arising from material published in this report.
Permissions
Copyright statement
Copyright © 2021 Murphy et al. This work was produced by Murphy et al. under the terms of a commissioning contract issued by the Secretary of State for Health and Social Care. This is an Open Access publication distributed under the terms of the Creative Commons Attribution CC BY 4.0 licence, which permits unrestricted use, distribution, reproduction and adaption in any medium and for any purpose provided that it is properly attributed. See: https://creativecommons.org/licenses/by/4.0/. For attribution the title, original author(s), the publication source – NIHR Journals Library, and the DOI of the publication must be cited.
2021 Murphy et al.
Chapter 1 Background
In May 2017, the US Food and Drug Administration (FDA) granted accelerated approval to pembrolizumab (Keytruda, Merck Group, Darmstadt, Germany) for the treatment of solid tumours with the microsatellite instability high (MSI-H) or the deficient mismatch repair (dMMR) biomarker. 1 This was the first time that a cancer treatment was approved based on a common biomarker rather than the location in the body where the tumour originated (i.e. a histology-independent approval). It represented an important paradigm shift, meaning that oncological diseases can now be classified by either tumour biomarker status or tumour histogenesis. The first histology-independent marketing authorisation was also granted by the European Medicines Agency (EMA) in 2019. 2
A histology-independent marketing authorisation would probably include a large number of tumour sites. For example, the main larotrectinib (Vitrakvi, Loxo Oncology Inc., Stamford, CT, USA, and Bayer, Leverkusen, Germany) study enrolled patients across 12 tumour types. 3 Given that it is unlikely to be feasible or desirable to conduct a separate appraisal for each tumour site contained within a histology-independent indication, the National Institute for Health and Care Excellence (NICE) will need to consider how to develop a process/approach that will allow a single, biomarker-driven appraisal for histology-independent cancer drugs.
This research aims to inform future NICE policy on how to appraise cancer drugs with histology-independent indications.
Aims and objectives
The main aim of the project was to explore the implications for the NICE technology appraisal (TA) process of assessing histology-independent products. The specific objectives were to:
-
determine the types of evidence and analyses required to support NICE appraisals of histology-independent products
-
identify the nature of the evidence likely to be available at the point of marketing authorisation
-
identify and implement a case study to highlight methods and evidence challenges and to explore alternative ways of addressing these
-
develop a conceptual framework to establish the evidence and analyses required to inform cost-effectiveness analyses and to guide NICE decision-making and potential Cancer Drugs Fund (CDF) data collection requirements
-
suggest any specific changes to the current NICE methods guide4 for TAs or additional requirements relating to histology-independent drugs
-
make recommendations for further methodological research.
Objectives 1 and 2 are addressed in Chapters 2–5. We undertook a series of targeted reviews to determine the type of evidence that is likely to be available at the point of marketing authorisation and to consider the evidence and analyses likely to be required to support a NICE appraisal.
These reviews included:
-
a review of FDA and EMA websites to identify relevant documents relating to regulatory issues and benefit–risk approaches relevant for histology-independent indications (see Chapter 2)
-
an overview of key statistical literature addressing the design and analysis of histology-independent trials (see Chapter 3)
-
a systematic review to identify published meta-analyses evaluating the use of overall response rate (ORR) and duration of response (DoR) as surrogate end points for progression-free survival (PFS) and overall survival (OS) (see Chapter 4)
-
a targeted review of published NICE TAs where marketing authorisation was based on single-arm studies using ORR as a primary outcome (see Chapter 5).
Objectives 3 and 4 are addressed in Chapters 6 and 7. Chapter 6 outlines a series of challenges for histology-independent appraisals and presents alternative approaches that might be used to investigate and account for different sources of uncertainty and heterogeneity. Chapter 7 presents an exemplar economic model to illustrate the nature of the assessments that could be used to assess the cost-effectiveness of a new histology-independent treatment and to inform NICE decision-making. A framework to inform approval and research policies for histology-independent technologies is proposed to help determine the appropriateness of different policy recommendations and to identify key uncertainties that might be used to inform and prioritise the value of further data collection.
Objectives 5 and 6 are addressed in Chapter 8. Based on the findings of the research, a series of recommendations are provided concerning whether or not changes in the current NICE methods guide are required for the appraisal of histology-independent products. Finally, a series of recommendations are provided concerning priorities for further methodological research.
Chapter 2 A review of Food and Drug Administration and European Medicines Agency documents relating to regulatory issues and benefit–risk approaches relevant for histology-independent indications
A targeted search of the FDA and EMA websites was conducted to identify relevant documents outlining regulatory approaches to the evaluation of histology-independent indications. The FDA and EMA websites were searched using the following key terms: ‘histology-independent’, ‘site-agnostic’ and ‘tissue-agnostic’. A narrative review of relevant documents was undertaken to summarise the regulatory requirements and guidance, including arrangements for post-licensing data collection. The objective was to provide insights into the current regulatory context for the benefit–risk evaluations performed by the FDA and EMA and to consider their relevance for economic modelling.
It is likely that histology-independent approvals will be granted via accelerated or conditional approval processes from the FDA and EMA. Hence, the narrative review was supplemented with relevant regulatory documents related to these processes. The list of identified regulatory sources considered is reported in Appendix 1.
The targeted searches were also used to identify any completed FDA/Oncologic Drugs Advisory Committee and EMA/Committee for Medicinal Products for Human Use (CHMP) reviews of existing histology-independent products to provide further insights into the nature of the evidence available at the time of approval, the key issues and uncertainties raised by FDA and EMA in assessing benefits and risks, and the nature of any mandated post-licensing data collection requirements.
Food and Drug Administration guidance for histology-independent products
The website searches yielded two preliminary guidance documents issued by the FDA in 2018, which addressed issues specific to histology-independent products: Developing Targeted Therapies in Low-frequency Molecular Subsets of a Disease5 and Master Protocols: Efficient Clinical Trial Design Strategies to Expedite Development of Oncology Drugs and Biologics. 6
The FDA defines a therapy as ‘targeted’ if it is intended for subsets of patients within a clinically defined disease based on either a common molecular alteration or a grouping of different underlying molecular alterations that share a common functional effect. The FDA guidance on developing targeted therapies in low-frequency molecular subsets focuses on two main issues that appear relevant to histology-independent products: (1) recommendations on how to group patients with different molecular alterations for eligibility in clinical trials and (2) general approaches to evaluating the benefits and risks of targeted therapies, where some molecular alterations may occur at low frequencies.
The FDA guidance recognises that certain targeted therapies may be effective in multiple groups of patients who have different underlying molecular alterations because of similarities in the functional effect observed across different molecular alterations. Hence, the guidance allows grouping of patients with different molecular alterations where ‘it is reasonable to expect that the grouped patients will have similar pharmacological responses based on a strong scientific rationale’. 5 Although this guidance is directed towards the grouping of molecular alterations, the same considerations might also apply to grouping different histologies based on a common molecular alteration. The FDA guidance notes that evidence to support a grouping strategy can come from computational, experimental or clinical sources, with clinical sources being considered the strongest form of evidence, but that any submitted evidence must always support a strong scientific rationale.
The FDA guidance stipulates that evidence supporting the efficacy of the drug for each molecular subset should be transparently reported, including information on the number of patients with specific molecular alterations included in the trial and the outcomes of these patients. However, the guidance also acknowledges that, although targeted therapies may be effective in multiple molecular subsets, certain subsets may contain only a small number of patients (or even none) despite eligibility criteria that permit their inclusion. The FDA guidance document notes that the small numbers of patients in this situation would preclude meaningful empirical inferences about treatment benefits or risks in patients with those particular molecular alterations. However, the FDA posits that the grouping guidance should also permit the generalisation of evidence from other, better-populated patient subgroups within the same clinical trial. Consequently, provided that the company was able to support its case for molecular grouping, the FDA appears to be likely to approve the therapy for all patients who meet the inclusion criteria for the trial, irrespective of their actual enrolment. Although this issue is specifically directed towards different molecular subsets, it appears equally relevant to histology-independent products, for which specific histologies may include small patient numbers and some histologies may not be represented at all.
Importantly, the FDA guidance also highlights that the indication may need to be further refined after the initial approval. If substantive data emerge indicating a lack of efficacy in certain molecular subgroups for which the drug was initially indicated, the FDA will consider narrowing the intended population as appropriate. In addition, the FDA notes that additional post-marketing studies may be required to provide additional information regarding the risks and benefits of the drug in subsets of patients with limited or no enrolment in clinical trials. Such evidence may be requested based on real-world evidence, traditional controlled trials or data from other sources, including ongoing trials.
The FDA guidance also recognises the importance of using analytically validated assays when enrolling patients into clinical trials. The assay should be able to identify all possible molecular alterations typical of the patient groups that are expected to respond to the developed therapy. The FDA also recommends that, if a test is necessary for the safe and effective use of the drug, an approved assay should be already commercially available at the time of drug approval. An exception to this case might be granted for conditions with high unmet need (e.g. life-threatening diseases with no suitable treatment alternatives).
An additional characteristic of histology-independent drugs is the use of novel and more efficient trial designs using master protocols. Master protocols are used to evaluate multiple drugs and/or multiple cancer subpopulations in parallel, using a single protocol. The FDA guidance document notes that a range of different terms are used to refer to the specific design of trials within a master protocol (e.g. umbrella, basket or platform; see Chapter 3 for further details on these designs).
The FDA guidance acknowledges the potential advantages of master protocols in terms of their flexibility and efficiency for drug development, but also raises concerns regarding difficulties in attributing efficacy and assessing safety, including overinterpretation of findings. In the context of histology-independent products, the most relevant aspects of the guidance relate to the use of basket trials to evaluate a single investigational drug or drug combination in different populations (defined by disease stage, histology or treatment history) and statistical considerations for non-randomised, activity-estimating designs. The guidance document highlights that basket trials undertaken using a master protocol are usually designed as single-arm activity-estimating trials with ORR as the primary end point. The guidance document notes that a strong response signal seen in a substudy may allow for subsequent expansion to generate data that could potentially support a marketing approval. The guidance document also emphasises the need for each substudy to include specific objectives, the scientific rationale for the inclusion of each population and a detailed statistical analysis plan (SAP) that includes justification for sample size and stopping rules for futility (i.e. the inability of the study to achieve statistically significant results).
The statistical guidance also makes recommendations for studies using non-randomised protocols, for which the primary end point is ORR, outlining that the planned sample size should be sufficient to rule out a clinically unimportant response rate based on the lower bound of the 95% confidence interval (CI) around the observed response rate. The guidance also recommends using designs, such as Simon’s7 two-stage design, that limit the exposure to an ineffective drug (see Chapter 3). Specific recommendations concerning the SAP include prespecification of the timing of the final analysis; ensuring adequate data collection and follow-up of all patients for efficacy and safety; and providing a description of the plan for independent review of confirmed ORR in solid tumours for each substudy.
Although the current guidance suggests that marketing approval requires subsequent expansion of a substudy or substudies, the guidance on statistical considerations also notes that if preliminary results suggest a major advance over available therapy, then the sponsor is encouraged to meet the FDA review division to discuss modifications to the protocol. Hence, it appears feasible for the results from master protocols using basket trials to be used to support marketing approval in specific circumstances and where the clinical protocol and SAP ensure that the data are of adequate quality.
Food and Drug Administration special approval processes
The initial histology-independent cancer drugs approved by the FDA represent novel products tackling severely limiting conditions with no alternative curative options. For this reason, they have not been considered within the standard FDA review process, but rather have been considered under processes that make provision for special approval to facilitate and expedite development and appraisal of new drugs treating serious or life-threatening conditions. 8 This has been the case for the three histology-independent approvals by the FDA for larotrectinib, pembrolizumab and entrectinib (Rozlytrek, Genentech Inc., South San Francisco, CA, USA). 1,9,10
The accelerated approval pathway is intended for those drugs that provide evidence of an effect on a surrogate end point reasonably likely to predict benefit in terms of a meaningful advantage over existing therapies. Surrogate end points are defined as substitutes for clinical outcomes that directly measure the effectiveness of a drug on length and quality of life, feelings or functioning. In cases for which measuring direct clinical outcomes, such as OS, would be impractical or unethical, surrogate end points can be accepted. Importantly, the surrogate outcome is not a direct measurement of clinical benefit but must predict, and at a minimum correlate, with the clinical benefit of interest. The strength of the evidence supporting the surrogate relationship is, therefore, essential to justify the use of a specific surrogate outcome and to establish whether or not this can support a traditional approval route or accelerated approval.
To date, ORR has been the most commonly used surrogate end point supporting accelerated approvals by the FDA. 11 One important reason for this is that ORR can be directly attributable to drug effect and, hence, single-arm studies conducted in patients with refractory tumours for whom no available therapy exists are considered to provide an appropriate assessment of ORR. However, the FDA also acknowledges that the clinical benefits of interest may not always be predicted by, or correlate with, ORR. Hence, the use of measures, such as ORR, to support an accelerated approval or traditional approval end point ultimately depends on the disease context and the magnitude of the effect, among other factors.
Food and Drug Administration review of histology-independent products
Food and Drug Administration review of pembrolizumab
The FDA approved pembrolizumab on 23 May 2017 for the treatment of adult and paediatric patients with unresectable or metastatic MSI-H or dMMR solid tumours. The approval is for patients who have progressed following prior treatment and who have no satisfactory alternative treatment options, and for the treatment of unresectable or metastatic MSI-H or dMMR colorectal cancer (CRC) that has progressed following treatment with a fluoropyrimidine, oxaliplatin (Eloxatin, Sanofi-Aventis, Paris, France) and irinotecan (Campto, Pfizer, New York City, NY, USA). 1
The efficacy of pembrolizumab in patients with MSI-H or dMMR solid tumours was derived from five uncontrolled, open-label, multicohort, multicentre, single-arm studies. Patients received either 200 mg of pembrolizumab every 3 weeks or 10 mg/kg of pembrolizumab every 2 weeks. Treatment continued until unacceptable toxicity or disease progression (up to a maximum of 24 months of treatment).
A total of 149 patients with MSI-H or dMMR cancers were included across the five clinical trials. The median age of patients was 55 years; 98% of patients had metastatic disease and 2% of patients had locally advanced, unresectable disease. In total, 90 (60%) out of the 149 patients had CRC, with the remainder diagnosed with other tumour types. The median number of prior therapies for metastatic or unresectable disease was two.
The identification of MSI-H or dMMR tumour status was prospectively established for the majority of patients (n = 135/149) using local laboratory-developed polymerase chain reaction (PCR) tests for MSI-H status or immunohistochemistry (IHC) tests for dMMR. Tumours from the remaining 14 patients were retrospectively identified as MSI-H using a central laboratory-developed PCR test.
The primary end point used for the FDA review was ORR, as assessed by blinded independent central radiologists (BICRs) using the Response Evaluation Criteria in Solid Tumours (RECIST) guidelines (version 1.1). The ORR was 39.6% (95% CI 31.7% to 47.9%). DoR was considered as a key secondary end point. Although the median DoR was not reached, 78% of responding patients had a DoR of ≥ 6 months. Overall, the safety profile of pembrolizumab was considered acceptable relative to durable responses observed in patients with advanced MSI-H/dMMR cancers.
A total of 16 tumour types were included in the combined data set. Consistent responses were reported between subjects with gastrointestinal (GI) cancer (i.e. CRC, small bowel, gastro-oesophageal junction and pancreas) and subjects with non-GI MSI-H cancer, with ORRs of 36.8% (MSI-H GI, n = 125) and 41.7% (MSI-H non-GI, n = 24). However, the FDA noted that some of the tumours (e.g. breast, prostate, sarcoma and renal cell) were represented by only one or two patients and that there was uncertainty as to whether or not the results apply to all disease types with MSI-H/dMMR status.
The key question considered by the FDA within their review was whether or not the presence of MSI-H/dMMR represents a unique biomarker that predicts a consistent response to pembrolizumab and similar clinical benefit across different primary tumours. In addressing this question, the FDA highlighted specific features associated with MSI-H/dMMR that are common across primary cancers, including increased lymphocytic infiltration and an increased mutational tumour burden with non-synonymous mutations. These features were noted to have been previously identified as correlating with an increased response to checkpoint inhibitors, including pembrolizumab, in tumours that had not been assessed for MSI-H or dMMR. Based on these common histological features, the FDA concluded that there was a strong biologic rationale that MSI-H/dMMR cancer represents a specific subpopulation of patients with cancer who are likely to derive clinical benefit from pembrolizumab.
Pembrolizumab was approved by the FDA for this indication under accelerated approval based on ORR and DoR. Despite a common biology among MSI-H/dMMR tumours, the FDA review also highlighted other differences among patients with different types of cancer that could influence the response to therapy with pembrolizumab (e.g. the degree of immunosuppression related to previous cytotoxic chemotherapy). Given the uncertainties that remain concerning the generalisability of the results to all disease types with MSI-H/dMMR status, a condition of the approval requires the sponsor to submit results of further studies to better characterise the response rate and its duration. These studies are required to include 124 patients with CRC and at least 300 patients with non-CRC, including a sufficient number of patients with prostate cancer, thyroid cancer, small cell lung cancer (SCLC) and ovarian cancer, as well as 25 children.
The FDA review noted that further randomised trials will be challenging to conduct in the histology-independent setting given concerns over equipoise. The FDA also questioned whether or not it would be scientifically appropriate to ‘lump’ all tumour types together into a single randomised trial given the different natural histories. The FDA also noted that, although response may not be entirely predictive of effects on clinical benefit, checkpoint inhibitor therapy, including pembrolizumab, has demonstrated beneficial effects on OS with similar response rates in other tumour types.
In the absence of a companion diagnostic test for the identification of MSI-H or dMMR tumour status, the FDA review noted the uncertainties regarding the use of laboratory-developed tests. These uncertainties concerned the rate of false positives in IHC tests for dMMR and false negatives in PCR tests for MSI-H, and whether or not performance characteristics may differ by the site of the primary tumour. Given these uncertainties, additional post-marketing studies were requested to assess and establish the performance characteristics of MSI-H and dMMR tests.
Food and Drug Administration review of larotrectinib
On 26 November 2018, the FDA granted accelerated approval to larotrectinib for adult and paediatric patients with solid tumours who have a neurotrophic tyrosine receptor kinase (NTRK) gene fusion without a known acquired resistance mutation; who are metastatic or for whom surgical resection is likely to result in severe morbidity; who have no satisfactory alternative treatments; or whose cancer has progressed following treatment. 9
As agreed with the FDA, the submission was supported by pooled safety and efficacy data from the first 55 patients who were enrolled in three multicentre, open-label single-arm studies. These studies enrolled subjects with solid tumours harbouring a NTRK fusion if they met the following criteria:
-
documented NTRK fusion, as determined by local testing
-
non-central nervous system (CNS) primary tumour with one or more measurable lesions at baseline, as assessed by RECIST 1.1
-
received one or more doses of larotrectinib.
The ORR, which was determined by an Independent Review Committee (IRC), was used as the primary end point for efficacy. DoR was a secondary end point, which was defined as the number of months from the start date of partial response (PR) or complete response (CR) to the date of disease progression or death, whichever occurred earlier.
Assuming that the observed ORR was ≥ 50%, a sample size of 55 patients was selected to provide 80% power to achieve a lower boundary of the two-sided 95% exact binomial CI about the estimated ORR exceeding 30%. Ruling out a lower limit of 30% for ORR was considered clinically meaningful. All patients were required either to have progressed following previous systemic therapy for their disease or to have required surgery with significant morbidity for locally advanced disease. The data cut-off time point for the primary analysis was July 2017, approximately 6 months after enrolment of the 55th patient.
The pooled sample included 12 tumour sites, of which the most frequent were salivary gland tumours (22% of patients), soft tissue sarcoma (20%) and infantile fibrosarcoma (IFS) (13%). More common tumours, such as lung or colon cancer, were represented less (n = 4 patients; 7% each) because they tend to rarely express a NTRK fusion. The sample was also heterogeneous in terms of prior cancer therapy, with patients having undergone different types of therapy (i.e. surgery, radiotherapy, systemic therapy) and different numbers of previous lines of therapy (45% having undergone one to two lines, and 35% having undergone three or more lines).
At the time of data cut-off, the estimated ORR was 75% (95% CI 61% to 85%), including 22% of patients with a CR and 53% of patients with a PR. Although the median DoR had not been reached, 30 out of 41 (73%) responders had a DoR of at least 6 months and 16 out of 41 (39%) responders had a DoR of at least 12 months.
The clinical and statistical review included an exploratory subgroup analysis that was performed by study, demographics and tumour type. Based on these analyses, the effectiveness of larotrectinib was reported to be reasonably similar irrespective of age, sex and race; however, no definitive conclusions were made given the limited sample size. A numerical difference in ORR was reported among patients with different tumour types, NTRK gene fusions or status of radiotherapy. Across different tumour types, three tumour types had at least seven patients: salivary gland (n = 12), soft tissue (n = 11) and IFS (n = 7). The ORR in these tumour types was reported to be higher than 75%. Conversely, it was reported that the ORR in colon cancer appeared to be lower (one out of four patients). No response was reported in the two patients with primary CNS lymphoma.
The FDA review concluded that, although the results showed that treatment with larotrectinib results in durable overall responses in patients with a variety of tumour types, there was insufficient clinical experience to conclude that the response rates achieved with larotrectinib were consistent across all NTRK fusion cancers.
A key issue addressed in the review was the potential risk that larotrectinib could be ineffective in some tumour types, even in the presence of a NTRK fusion. The FDA concluded that the risk of ineffectiveness was low owing to the strong rationale presented by the company, which was supported by clinical and non-clinical data. The strength of the evidence was assessed against the following criteria: the ability of the biomarker to identify a population with common features, the similarity of response across tumour types and the ability to reliably identify the biomarker at the screening phase.
The FDA considered the totality of evidence presented by the sponsor to be sufficiently strong to consider pooling the results across trials and patients, supporting a histology-independent indication. The FDA also concluded that, although there was a risk that larotrectinib may be ineffective in some tumours, the level of risk was deemed to be low and was considered acceptable given that the product is approved only for the treatment of patients who have no satisfactory alternative treatment options or whose cancer has progressed following treatment. As a result, the FDA did not consider that patients would be forgoing effective therapies when treated with larotrectinib.
The primary risks of larotrectinib were identified as hepatotoxicity and neurotoxicity. However, these adverse reactions were considered largely manageable and reversible with dose modification or discontinuation. Overall, the toxicity profile of larotrectinib was considered acceptable when considered against the durable effects across different cancer types in patients with limited or no effective treatment options.
The ORR was considered to be a surrogate end point that was reasonably likely to predict benefit, in accordance with the requirements of the accelerated approval process. The clinical effect was deemed to be sufficiently large and the effect was durable, which provided a meaningful advantage over the available therapy for patients with NTRK fusion solid tumours. The population was also considered to have a high unmet medical need given the serious, life-threatening and rare nature of their cancers. However, the FDA specified that the ORR evidence was not sufficiently strong to support a regular approval, given the large number of histological subtypes and the small sample size. This led to a degree of uncertainty regarding the magnitude of the treatment effect of larotrectinib in any single histological subtype.
A key post-marketing requirement is that the company conduct further studies that provide additional data to verify and confirm the clinical benefit of larotrectinib through more precise estimation of ORR and DoR in several specific tumour types [CRC, non-small cell lung cancer (NSCLC), CNS tumours and melanoma]. These tumour types were not well represented in the existing efficacy population. A minimum of 40 patients with cancers other than CRC, NSCLC, CNS tumours, melanoma, soft tissue sarcoma, thyroid cancer, IFS and salivary cancers [e.g. breast cancer, gastrointestinal stromal tumours (GISTs), cholangiocarcinoma and biliary tract cancers] are also required to be studied. ORR and DoR are required as end points and all responding patients are required to be followed for at least 12 months from the onset of response. In addition, a final report is requested from the first 55 patients enrolled with NTRK fusion solid tumours to further characterise the DoR, including follow-up of at least 2 years from the onset of response for responding patients.
Importantly, the FDA concluded that it would not be feasible or appropriate to conduct a randomised trial to demonstrate that larotrectinib improves OS in patients with NTRK fusion. The reasons included the extreme rarity of NTRK fusion cancers, the lack of equipoise in settings without available therapies and the expectations for patient crossover. Consistent with their review of pembrolizumab, the FDA again queried whether or not it would even be scientifically appropriate to ‘lump’ these tumour types together into a single randomised trial, given differences in natural history between different tumour sites.
The identification of positive NTRK gene fusion status was determined in the clinical efficacy analysis set using next-generation sequencing (NGS) for 91% of patients and fluorescence in situ hybridisation (FISH) for the remaining 9% of patients. The company did not submit an application for an in vitro companion diagnostic device. Despite this, the clinical review team was supportive of approval, citing the availability of a reliable non-companion device and the efficacy of larotrectinib. However, the development and validation of a companion diagnostic test by the sponsor was agreed as part of a series of post-marketing commitments.
Food and Drug Administration review of entrectinib
On 15 August 2019, the FDA granted accelerated approval to entrectinib for adults and paediatric patients aged ≥ 12 years with solid tumours who have a NTRK gene fusion without a known acquired resistance mutation, are metastatic or for whom surgical resection is likely to result in severe morbidity, and have progressed following treatment or have no satisfactory standard therapy. 10
This indication was approved by the FDA under accelerated approval based on ORR and DoR. The submission was supported by pooled efficacy and safety results from the first 54 adult patients with unresectable or metastatic solid tumours harbouring a NTRK fusion enrolled across three single-arm studies. All patients were required to have cancer that progressed following effective systemic therapy for their disease, if available, or would have required surgery with significant morbidity for locally advanced disease.
The median age of the patients was 55 years. The most common tumours (≥ 5%) were lung cancer (56%), sarcoma (8%) and colon cancer (5%). In total, 96% of patients had metastatic disease and 4% had locally advanced, unresectable disease. All patients had received prior treatment for their cancer, including surgery, radiotherapy or systemic antineoplastic therapy.
The ORR and DoR, as assessed by BICR using RECIST v1.1, were the primary end points. PFS, as assessed by BICR and OS, was included as a secondary end point. The effectiveness of entrectinib in paediatric patients aged ≥ 12 years was established based on extrapolation of data in adult patients with solid tumours harbouring a NTRK gene fusion and pharmacokinetic data in adolescents enrolled in the STARTRK-NG study. 12
In the first 54 patients, the ORR was 57% (95% CI 43% to 71%). This was clinically meaningful because the results excluded a lower bound of the 95% CI for ORR of 30%. At the data cut-off time point (i.e. 31 May 2018), the median DoR was not reached. Among the 31 responding patients, 55% had a DoR of ≥ 6 months and 39% had a DoR of ≥ 12 months.
Exploratory ORR results for subgroups defined by tumour type and by NTRK gene fusion partner were presented. Although there was no formal discussion of these results, a general disclaimer was provided that noted that the subgroup results should be treated with caution owing to the small sample sizes and the single-arm design.
Only limited details were reported for secondary end points. The estimated median PFS was reported to be 11.2 months (95% CI 8.0 to 14.9 months). Less than 30% of deaths were observed by the clinical cut-off date (31 May 2018), which was considered to be too immature to be considered in the clinical review.
The most serious adverse events reported with entrectinib were congestive heart failure (CHF), CNS adverse reactions, skeletal fractures, hyperuricemia, hepatotoxicity, QT prolongation and vision disorders. Although serious in nature, these events were also reported to be manageable and reversible with dose modification or discontinuation of entrectinib.
The FDA drew similar conclusions for entrectinib to their earlier review of larotrectinib (see Food and Drug Administration review of larotrectinib). Although acknowledging that there was uncertainty regarding the magnitude and durability of the treatment effect of entrectinib in any specific histological subtype of solid tumours, they concluded that the risk of treatment was low, using a similar rationale to that previously described for larotrectinib.
Similar post-marketing requirements were reported for entrectinib to those for larotrectinib. This requires the company to conduct additional single-arm studies to obtain data to verify and further characterise the clinical benefit of entrectinib in an adequate number of patients with common histological tumour types, including colon cancer and melanoma. Additional post-marketing requirements also include the conduct of additional studies to further characterise the risks of CHF and skeletal fractures with entrectinib.
European Medicines Agency guidance for histology-independent products
To date, the EMA has not published any guidance specific to the evaluation of histology-independent products. However, the proceedings of two workshops were identified in the searches: one specifically addressing histology-independent indications13 and a second discussing the use of single-arm studies in oncology. 14
A revision to the current Guideline on the Evaluation of Anticancer Medicinal Products in Man15 is currently under consultation. 16 The concept paper underlying the revision explicitly states the need to address the use of biomarkers in oncology, which was not covered by the previous guideline. This development recognises the increasingly important role that biomarkers have in both defining disease and developing treatment strategies. Biomarker-based treatments also have the possibility to span across tumour sites and are likely to be assessed using innovative study designs, such as basket and umbrella trials. These study designs were not considered in the current guideline;15 therefore, an update was recommended by the Oncology Working Party. The update will focus on better identifying the role of biomarkers in the development pathway, developing evidence standards in the context of rare cancers and outlining the main aspects and principles of innovative study design, including the use of basket trials.
European Medicines Agency special approval processes
Similar to the FDA, the EMA provides alternative marketing authorisation pathways to cover situations in which the nature or quality of the evidence would not be sufficient to support traditional approval. Conditional approval from the EMA is a form of conditional marketing authorisation for those medicines that target unmet medical needs for serious conditions with a positive benefit–risk balance, but that do not have comprehensive data available. To grant conditional approval, agreement is required on additional post-marketing studies to confirm the initial assessment of the benefit–risk balance. This marketing authorisation is valid for 1 year and can be renewed annually following a rolling review, provided that the benefit–risk assessment is still considered to be positive.
European Medicines Agency review of approved histology-independent indications
To date, only one histology-independent product has received marketing authorisation in the EU. Larotrectinib received conditional marketing authorisation on 19 September 2019. 2 The authorisation recommends larotrectinib as monotherapy for the treatment of adult and paediatric patients with solid tumours who display a NTRK gene fusion; who have a disease that is locally advanced, metastatic or where surgical resection is likely to result in severe morbidity; and who have no satisfactory treatment options.
The EMA review was supported using several different analysis sets. The primary analysis set (PAS) was based on the same 55 patients who were considered in the earlier FDA review of larotrectinib. The analysis of the PAS was based on a pooled analysis of patients consecutively enrolled from three single-arm studies.
The EMA review identified several concerns regarding the PAS. First, the restriction to the first 55 patients was considered to have been arbitrarily chosen. Second, the exclusion of CNS tumours was considered to introduce a bias in the efficacy estimates. Finally, restricting the analysis to patients who received one or more doses was not considered to accord with the intention-to-treat (ITT) principle.
Following requests from the CHMP, further analysis sets [extended patient analysis set (ePAS) and ePAS2] were submitted, which included additional data from an extended follow-up and a larger pooled analysis population. The ePAS (n = 73) included all patients who met all PAS eligibility criteria, as of 19 February 2018, and had a central review of tumour response by the IRC. ePAS included an additional 18 patients compared with the PAS (n = 55). The ePAS2 (n = 93) included all patients who met all PAS eligibility criteria and had either discontinued the study or ≥ 6 months’ follow-up by 30 July 2018. ePAS2 included an additional 38 patients compared with the PAS (n = 55). The ePAS2 was the main efficacy analysis set considered in the EMA review.
A further cohort that included paediatric and adult patients with primary CNS tumours (n = 9) was reported separately. This cohort represented a prespecified exclusion criterion from the original analysis of the PAS. This cohort was considered to have a potentially lower likelihood of response than the other cohorts given the results from earlier animal studies, which indicated low penetration of larotrectinib into CNS tissues. However, the review also acknowledged that CNS penetration in cancer patients taking larotrectinib may be more substantial than that suggested by prior evidence.
The primary end point considered was ORR by IRC assessment, which was defined as the proportion of patients with the best overall response of CR or PR. Secondary end points included time to response (TTR), DoR, PFS (including PFS rate at 6 and 12 months) and OS (including survival rate at 12 months).
In the ePAS2 analysis, the ORR by the IRC was 72% (n = 67/93) (95% CI 62% to 81%). The ORR results were considered by the EMA review to be outstanding. The median TTR was 1.8 months by the IRC [interquartile range (IQR) 1.71–1.94 months]. The median DoR was not estimable (NE). However, 72% of responding patients were reported to have had a DoR of ≥ 6 months and 42% had a DoR of ≥ 12 months. The review also noted that the percentage of patients with durable responses appeared to be larger in previously submitted data with shorter follow-up. Concerns were expressed that the difference in results between alternative follow-up times indicated that limited early data might overestimate the true treatment effect.
The EMA review noted that there was substantial heterogeneity across the three separate studies and that the primary end point was based on a crude proportion of responses. The review also highlighted that sensitivity analyses provided by the sponsor that utilised tumour type as a random factor provided slightly lower estimates than the crude proportions. Further re-analysis by the EMA involved investigating alternative selections of cohorts from the three studies. These analyses indicated that the crude ORR appeared in the upper end (the 90th percentile) of the distribution of possible estimates, suggesting a possible selection bias. However, the review also noted that a large majority of all possible ORR estimates were above 50%, indicating a true effect of a relevant magnitude.
The median PFS was 27.4 months [95% CI 13.8 to NE months] by the IRC. The PFS rate at 6 months was 77% and the PFS rate at 12 months was 64% (95% CI 51% to 76%). The median OS was not reached in the ePAS2 owing to the low event rate of 15% (n = 14/93 dead) at a median follow-up time of 16.7 months. The OS rate at 12 months was 88% (95% CI 81% to 95%). All nine patients in the CNS group were noted to still be alive at the final data cut-off time point.
The EMA review highlighted the immaturity of the OS and PFS data. In addition, although the PFS and OS data were considered important for contextualising the ORR and DoR results, the pooling of many different types of primary malignancies with inherently different prognoses led to a conclusion that the data should be interpreted with caution.
The subgroup analysis reported in the EMA review included an analysis of ORR by tumour type. The ORR was reported to be highly variable across the studied tumour types, ranging from 0% in individual patients with breast cancer, cholangiocarcinoma and pancreatic cancer to 100% in four patients with GIST. The review indicated that tumour types for which NTRK gene fusions are characteristic (or even considered pathognomonic) of the disease, such as IFS (n = 13), salivary gland/mammary analogue secretory carcinoma (MASC) (n = 10) and congenital mesoblastic nephroma (n = 1), tended to have higher ORRs (92%, 80% and 100%, respectively). However, the review also concluded that the tumour-specific estimates were not robust owing to the small sample sizes of the individual subgroups. Of the nine patients with primary CNS tumours, one had an objective response (PR) and the remaining eight had stable disease as the best response. Six patients were reported to be progression-free at last follow-up. The CHMP considered that there was no scientific rationale to exclude previously treated CNS patients with no satisfactory treatment options available and that the indication should cover these patients also.
A key question that was considered in the EMA review was whether or not the available data supported the assumption that NTRK gene mutations are oncogenic driver mutations and that the mechanism of action is independent of tumour histology. This assumption was considered necessary to conclude that larotrectinib would result in clinically relevant activity in tumours expressing NTRK fusion proteins, regardless of the tissue of tumour origin. Additional advice was sought to address this question from the Scientific Advisory Group (SAG) in Oncology and the EMA Biostatistics Working Party.
The consensus view of the SAG was that the available data did not support the hypothesis that NTRK gene fusions are universally oncogenic drivers, independent of tumour type/histology and other disease characteristics. The SAG also concluded that the mechanism of action may differ according to histology and other characteristics, and that the existing data were insufficient to establish activity regardless of tumour type and other characteristics. However, the SAG also recognised that preclinical and clinical data supported NTRK as an oncogenic driver in some paediatric malignancies. In addition, fusion genes affecting NTRK 1/2/3 were reported to be highly recurrent in certain rare malignancies. ETV6–NTRK3 was noted to be present in > 95% of secretory carcinomas of the breast, MASC of the salivary glands, congenital fibrosarcoma and cellular mesoblastic nephromas. As reported in the EMA review, this led one expert to suggest the possibility of having a histology-independent approval for cancers with proven NTRK fusions as oncogenic ‘drivers’, provided that NGS could exclude other alterations being significant drivers for tumour progression. However, it was also noted that data do not currently exist to establish the efficacy of such a strategy.
The SAG acknowledged the strong rationale and the available clinical data for several specific tumour types (IFS, salivary gland/MASC and congenital mesoblastic nephroma) for which NTRK fusions have been established as oncogenic drivers independent of other characteristics. The SAG also noted that larotrectinib has shown important activity in GIST with NTRK after resistance/relapse with imatinib (Gleevec, Novartis, Basel, Switzerland) (ORR n = 5/5), reflecting a probable similar role for NTRK fusions. For these selected conditions, given the strong rationale and the available clinical data, the SAG concluded that efficacy has been established in the absence of available treatments of proven efficacy in terms of convincing clinical efficacy end points. However, for other conditions the review concluded that the role of NTRK fusions had not been properly studied and could not be appropriately established with existing data, given the lack of comprehensive sequencing of tumour tissue prior to treatment initiation. Concerns were also expressed from the SAG regarding the small sample sizes in different tumour types, the significant heterogeneity observed in terms of response rates and the very low ORR observed in different tumour types (ORR 0% to 33%). The low ORRs were also noted to be reported in common tumour types for which occurrence of NTRK gene fusion is rare (e.g. lung, colon and breast).
The SAG concluded that neither the available evidence nor the reasonable extrapolations supported the proposed indication to include all solid tumours independently of tumour type. The SAG considered that clinical decisions to use larotrectinib were justified for the rare conditions for which existing evidence more clearly supported the role of NTRK fusions as oncogenic drivers. For other conditions, the acceptable safety profile supported use in situations for which established alternatives are lacking or for which available alternatives are associated with high morbidity and mortality.
Further to the SAG comments, the CHMP highlighted that a certain degree of heterogeneity in response is unavoidable in the same way because there will be important effect modifiers within any indication. Thus, the critical issue considered by the CHMP was whether or not the studies were likely to be representative of the treated population once the product is authorised and whether or not the uncertainties are acceptable given the available data and the intended use as a last-line treatment in patients without satisfactory treatment options.
The clinical review concluded that, although the efficacy results were outstanding for a late-stage disease setting, significant uncertainties remained concerning the robustness and generalisability of these estimates. The review also acknowledged that the results may change in a negative direction as further evidence is generated. However, the magnitude of the current effect estimates was considered to be of sufficient size to support a probably large treatment benefit observed in practice. The review also noted that the interactions between treatment and tumour type required further exploration.
The available data were not considered comprehensive and a conditional approval was concluded to be appropriate by the EMA. The conditional approval was granted based on a positive benefit–risk balance and the requirement that the company provide additional comprehensive data. As part of this requirement, the company is required to submit a prospective cohort of 75 patients as part of the NAVIGATE study (LOXO-TRK-15002),3 for which at least 1 year of follow-up is available, and to perform an overall pooled analysis including the ePAS2/CNS cohort to give increased precision for the estimates of ORR and DoR. In addition, the company plans to enrol 200 additional patients in NAVIGATE (LOXO-TRK-15002)3 and as part of the SCOUT study (LOXO-TRK-15003)17 within a 36-month period post approval. It is planned for 80 patients to be recruited for four common tumour types (lung cancer, CRC, melanoma and non-secretory breast cancer) and 120 patients in other tumour types. At least nine (and up to 20) patients will be recruited in each of the four common tumour types, permitting a more precise estimate of efficacy in common cancers for which NTRK fusions are rare.
Overview of registered or completed trials for histology-independent products in development
Research from NICE suggests that there are approximately 20 technologies currently in development for histology-independent indications. We undertook searches of the clinicaltrials.gov website using the list of histology-independent products provided by NICE. Information was extracted for those trials that are more likely to be vehicles for regulatory approval, that is combined Phase Ib/II, Phase II and Phase III trials. The aim of this review was to clarify whether or not the level of evidence available during the FDA/EMA appraisals of the initial histology-independent products is likely to be representative of that of future products in other indications.
Appendix 2 provides a summary of the registered or completed Phase Ib/II, Phase II and Phase III trials identified using searches of the clinical trials.gov website. Of the 20 products considered, three products (pembrolizumab, larotrectinib and entrectinib) were excluded because more detailed evaluations of the regulatory submissions have been summarised in Food and Drug Administration review of histology-independent products and European Medicines Agency review of approved histology-independent indications. Of the remaining 17 products, only 13 products had registered trials that were considered potentially suitable for regulatory purposes. A total of 36 relevant trials were identified for these 13 products. In total, 13 of the trials were for one drug [olaparib (Lynparza, AstraZeneca, Cambridge, UK)]. The products that were identified included drugs already approved for specific indications (e.g. olaparib), for which there was an aim to expand their existing marketing authorisation, and novel products, for which initial approval in a histology-independent context may be sought (e.g. LOXO-295).
Over 90% (n = 33) of the 36 registered trials were single-arm studies. ORR was the most common primary end point (n = 27), although PFS was reported as a primary end point in four studies. DoR (n = 18), PFS (n = 28) and OS (n = 24) were commonly included as secondary end points.
Of the 36 trials, only three trials were formally referred to as basket trials. A total of 19 of the remaining 33 studies (58%) included separate treatment or population cohorts, suggesting that the analyses may explore differences between the separate cohorts. The remaining studies reported no details on specific cohorts or subgroups that might be considered.
Summary and implications
The study design and evidence considered by the FDA and EMA for the initial approvals of histology-independent products appear consistent with the type of evidence that may be expected for future approvals (e.g. single-arm studies with ORR as the primary end point). Although the FDA has now issued specific guidance concerning the conduct and reporting of basket trials to evaluate a single investigational drug or drug combination in different populations, the design of many ongoing or recently completed studies clearly pre-date this guidance. Only a small number of the trials were formally referred to as a basket trial and there was a lack of clarity in the design of many studies concerning whether or not separate cohorts would be formally considered. As a result, it appears to be likely that the current case-by-case approach employed by the regulators in determining the appropriateness and quality of the underpinning evidence to support a histology-independent approval will continue for the foreseeable future.
The central question considered by both the FDA and the EMA concerns the biologic rationale and strength of existing clinical evidence to support the assumption that a biomarker-defined population (e.g. MSI-H/dMMR or NTRK) is sufficient to establish clinically relevant activity independent of tumour histology. Neither the FDA nor the EMA considered that the current evidence base for any of the three products was sufficiently robust to establish this. Indeed, both agencies raised important uncertainties regarding the generalisability of the results across all individual histology sites. However, the magnitude of the effect in the overall population was considered clinically important and the risk associated with approving the treatment in specific tumours was considered to be low owing to the strong biologic rationale and the intended approval as a last-line treatment in patients without satisfactory treatment options.
It is evident from the FDA and the EMA reviews for larotrectinib that the evidence base is rapidly developing over time, such that the later EMA review included an additional 38 patients (n = 93) compared with the FDA review (n = 55). It is also notable that the advice of the SAG to the EMA, based on this larger data set, appeared to differentiate the strength of the biological rationale and the available clinical evidence for several specific tumour types. For a few specific tumour types (IFS, salivary gland/MASC and congenital mesoblastic nephroma), the SAG concluded that NTRK fusions had been established as oncogenic drivers, independent of other characteristics. The SAG also concluded that evidence for GIST was sufficiently strong to support a similar role of NTRK fusions as an oncogenic driver. For these specific tumour types, the SAG concluded that efficacy has been established in the absence of available treatments of proven efficacy in terms of convincing clinical efficacy end points and that clinical decisions to use larotrectinib were justified. For other conditions, the acceptable safety profile supported use in situations for which established alternatives are lacking or for which available alternatives are associated with high morbidity and mortality.
Both the FDA and the EMA reviews ultimately concluded that the evidence for these existing products was not sufficient to support a routine approval for a histology-independent label. The further evidential requirements focus on three specific aspects: (1) increasing the precision for the estimates of ORR and DoR and extending the length of follow-up in the overall population; (2) the generation of new evidence to increase the precision of efficacy in more common cancers for which NTRK fusions are rare (e.g. lung, colorectal, melanoma and non-secretory) and for which current evidence is sparse; and (3) the development and validation of a companion diagnostic test. As a result, important new evidence will emerge over time to address some of the key uncertainties identified by the EMA and FDA.
The reviews also highlighted two important challenges that need further consideration. First, the design and conduct of trials to support histology-independent products are likely to differ from those of more conventional products. The use of novel and efficient basket trial designs using master protocols will present additional challenges to NICE in terms of Health Technology Assessment (HTA) assessment. Hence, the rationale and statistical basis for the design of these studies warrants further consideration. Second, the initial evidence supporting the basket trials is likely to be focused on surrogate end points, such as ORR and DoR. Our reviews show that, although data on more policy-relevant outcomes, such as PFS and OS, are being collected, there is likely to be a number of potential challenges regarding their interpretation in the absence of a comparator arm, possible bias owing to confounding (e.g. receipt of subsequent therapies) and the likely immaturity of these end points at the time of initial marketing authorisation.
It is notable that neither the FDA nor the EMA reviews considered that the evidence on PFS or OS was sufficiently robust to draw any meaningful conclusions in relation to these end points. Instead, both agencies relied on the magnitude of the ORR and DoR as providing evidence to support a potentially meaningful difference in more policy-relevant intermediate (e.g. PFS) and final clinical outcomes (e.g. OS), drawing on existing surrogate relationships. Hence, the surrogate relationships between response-based outcomes (ORR and DoR) are likely to be central to HTA and economic modelling in helping to inform and/or validate longer-term extrapolations of PFS and OS owing to the probable immaturity of these end points.
The following chapters attempt to address these challenges by considering in more detail the nature and design of the trials (see Chapter 3) and the existing evidence evaluating the use of response-based outcomes as surrogate end points for PFS and OS (see Chapter 4).
Chapter 3 An overview of key statistical literature addressing the design and analysis of histology-independent trials
The literature on adaptive designs and complex innovative trial designs was reviewed, focusing on trial design and analysis methods proposed for oncology studies and, in particular, ‘master protocol’ designs proposed to assess histology-independent drugs. The review was based on known articles in the area (both methodological and applied) and following up of relevant reference lists.
Adaptive Phase II studies
The first step in evaluating a novel treatment is to conduct a Phase II study to determine whether or not the drug has a sufficient level of disease activity to warrant further investigation. To minimise the exposure of patients to ineffective drugs, adaptive two-stage designs have been proposed, in which the second stage of the study is not activated if the first stage shows that the treatment is not effective. The first such design was proposed by Gehan18 in 1961, where the first stage enrols 14 patients and if no responses are observed the trial is terminated. If at least one response is observed in stage 1, the second stage of accrual is activated to obtain an estimate of the response probability with a prespecified standard error. Patients from both stages are used for the estimation of the response rate and an implicit 20% threshold for response rates is considered promising for further study. Fleming19 also studied multistage designs, with acceptance (i.e. proceed with study) or rejection (i.e. stop the study) possible at each stage based on prespecified probabilities: p0, the largest response probability that, if true, would imply that the drug is not sufficiently effective to warrant further investigation; and p1, the smallest probability that would imply that the treatment has a therapeutic effect worthy of further investigation. The acceptable probabilities of making incorrect decisions (type I and type II errors) are also required. In Fleming’s19 design, early rejection occurs only when interim results are quite extreme, which permits the final analysis to be unaffected by interim monitoring; however, this is not always desirable for Phase II trials of agents that are likely to be inactive. Although these designs were popular for many years, they did not optimise sample size or allow for early termination when the drug has low tumour activity – a key ethics concern. This led to the development of Simon’s two-stage design,7 which minimises the expected sample size when the true response is less than some predetermined level. Similar to Fleming’s approach,19 investigators prespecify p0, p1 and the acceptable type I and type II error bounds. This is currently one of the most commonly used adaptive designs and, although it can be extended to multiple stages, in practice only two stages are usually used. Extensions of Simon’s two-stage design7 have been proposed to address the uncertainty in the expected response for p1: if this is too optimistic, Simon’s design7 would reject a potentially promising treatment, whereas if it was too pessimistic, it would require more patients to be recruited than necessary. 20
Bayesian approaches to adaptive Phase II trials have also been proposed. 21–24 These approaches terminate the trial early if the predictive probability that the treatment is not sufficiently effective at the maximum sample size is below a prespecified level; provide a posterior distribution for the true response probability; and allow the calculation that the true probability of response is above a certain value, or the calculation of an interval that has a 95% probability of containing the true response proportion (note that this is not provided by CIs obtained using a frequentist approach).
Master protocol designs
Typically, adaptive Phase II oncology studies are conducted separately for each patient subgroup, based on histology or biomarker activity. However, concerns have been raised about the ability of traditional clinical trial designs to facilitate timely access to innovative technologies owing to the increasingly small populations being targeted in oncology trials. A traditional Phase III study would never be expected to recruit enough individuals to achieve statistical significance on the primary outcome. The use of complex innovative trial designs with ‘master protocols’ and basket trials has been proposed to accelerate the access to innovative targeted technologies and precision medicine. A consensus statement on their design, conduct and interpretation has recently been published. 25 Master protocol trials use a centralised screening platform to identify eligible patients and a common protocol for different substudies, which may each focus on patients with specific markers or histologies. The main advantages of master protocols are enhanced patient participation, given that more patients are eligible to enter the trial, and a simplification of the trial process, given that a single protocol is approved for use on multiple substudies. Basket trials typically include patients with diverse conditions who share a particular feature or biomarker that can be treated with a single therapy. The key underlying assumption of a basket trial is that the condition depends on the target pathway and that the proposed therapy inhibits this target. 26
In oncology, basket trials use a master protocol to define patient eligibility by the presence of a particular biomarker or molecular alteration, regardless of histology. The substudies, or baskets, are then defined by a particular histology or other disease-specific characteristics, for example mutation type. Because individual patients are recruited independently of tumour location or subtype, they are more likely to be eligible for enrolment. 26–28 However, a critical consideration is the heterogeneity in prognosis across the different histologies; therefore, standardised response rates, reflecting tumour shrinkage, are typically used instead of survival outcomes, such as PFS or OS. 29 In addition, given that the majority of basket trials do not have a control arm, stable disease or survival outcomes would be difficult to interpret unless they were clearly better than what is expected under standard therapy for all tumours. 30 Therefore, a further crucial assumption in these designs is that response is a sufficient measure of clinical benefit.
Although designed to improve recruitment, basket trials can still fail to recruit sufficient patients to some or all baskets. For example, the CUSTOM trial31 failed to recruit enough patients for some baskets covering rare mutations. In addition, because basket trials rely on the assumption that molecular profiling is a good predictor of response, they may fail in situations in which histological tumour type predicts response better than the biomarkers or mutations defining the baskets. 27,29,32
Although advocated as ideal, randomisation to a control arm is rare in basket trials33 owing to the differences in standard of care (SoC) across the different tumour types defining the baskets. 25,27,30 Adaptive designs for confirmatory basket trials with concurrent (non-randomised) control groups have been proposed, and their challenges and limitations discussed. 34 However, the lack of a concurrent, randomised, control arm remains a key limitation of these trial designs and, in particular, for the interpretation of such trials in HTA processes. 25
Non-randomised basket trials are typically exploratory and use similar two-stage designs to traditional Phase II clinical trials, with each substudy (basket) analysed separately. Tumour types that are expected to have a sufficient frequency of the targeted genomic alteration are enrolled into their own basket, while others are enrolled into a combined basket. Typically, these studies are designed so that each basket will recruit a certain number of patients and if a certain prespecified proportion of these patients respond, the basket is considered ‘promising’ or successful, and either accrual is expanded or a separate confirmatory study is planned. If insufficient responses are observed, the basket is ‘pruned’ owing to low promise of efficacy and recruitment to that basket is stopped. Different designs can be used, with varying thresholds for response rates selected depending on the indication and prior expectations of efficacy, and with suitable corrections for false-positive rates. 29,35
Heterogeneity of effect in basket trials
Heterogeneity of effect across different baskets is a key concern. One way to account for this is to analyse each basket separately as if it was an independent study. For example, a basket study of vemurafenib (Zelboraf, Roche, Basel, Switzerland) in multiple non-melanoma cancers with BRAF V600 mutations used an adaptive Simon two-stage design20 with stopping rules defined independently for each basket, and considered a response rate at week 8 of 15% to be low, a response rate of 45% to be high and a response rate of 35% to be low but still indicative of efficacy. 32 They found that not all tumour types responded homogeneously to treatment, with some tumour types not meeting the prespecified criteria for response. Similarly, the CUSTOM trial31 used Simon’s optimal two-stage design, defining p0 = 0.3 and p1 = 0.6 based on previous literature. The trial aimed to identify targets for molecular biomarkers in NSCLC, SCLC and thymic malignancies and to simultaneously evaluate five different targeted therapies in each of the three histologies, which resulted in a total of 15 study arms. A high response rate to erlotinib (Tarceva, Roche, Basel, Switzerland) was identified from only 15 NSCLC patients with an epidermal growth factor receptor (EGFR) mutation, but another therapy, selumetinib (Koselugo, AstraZeneca, Cambridge, UK), failed to achieve a promising response in patients with Kirsten rat sarcoma (KRAS) viral oncogene homologue mutations. 31
However, a separate analysis of each basket does not allow for the possibility that some subgroups may react similarly to the drug, particularly if they share a common biomarker that the novel therapy is targeting. By analysing each basket separately, efficiency may be lost by not allowing information gathered from one basket to inform the next, thus increasing the required sample sizes in each basket. In practice, many standard Phase II designs will ignore potential heterogeneity and pool all patients for analysis, which, in effect, ignores the specific basket-defining tumour characteristics (e.g. histology) and assumes equal efficacy across all baskets. 35 If this approach is taken, trial planning and analysis are similar to a standard Phase II trial and, for example, Simon’s two-stage design can be used. Although allowing analysis with a much smaller number of included patients, pooling all patients ignores the potential for heterogeneity across baskets and effectively assumes that it is zero, which can miss treatments that are active in only some baskets36 and can lead to large biases in overall estimated effects. In addition, if the drug is truly active or inactive in all baskets, this will be an inefficient design. 37,38
Frequentist adaptive designs for basket studies that try to acknowledge this potential for heterogeneity across baskets have been proposed. In the context of Phase II studies with heterogeneous populations, a design that tests global response across the whole population, while allowing a different response for each subgroup, was proposed by London and Chang. 39 Simon’s two-stage design was extended to use a more flexible strategy that both tests each subgroup and tests the combined population, which allows the trial to stop if either a subgroup or the combined population show futility, that is the inability of the study to achieve statistically significant results, at prespecified thresholds (that are not necessarily the same). 40 Negative results in one subgroup would lead to stopping recruitment in that basket alone, unless the combined response for the whole population was below the acceptable threshold. This design leads to smaller sample sizes than separate analyses of each basket when the drug is inactive across all subgroups and to more power when there is activity in all subgroups. It also retains the individual tests for each subgroup, which allows the identification of promising baskets. This design requires prespecification of the expected response rates and prevalence in each subgroup to specify the expected response rate in the overall population. Although the average prevalence in the clinical population may be known, owing to the often small samples recruited, the observed prevalence as the trial enrols patients may be quite different. A design that allows the rejection values to be adjusted depending on the observed prevalence in the trial was proposed by Jung et al. 41
Cunanan et al. 42 later proposed an efficient study design for the specific scenario of the typical basket trial in oncology, which assesses the homogeneity of the baskets’ response rates at an interim analysis, aggregating the baskets in the second stage (i.e. full borrowing of information) if results suggest effectiveness in all or most baskets, or treating each basket separately (i.e. no borrowing) otherwise. Their basic premise is that the design can be made more efficient by aggregating information from separate baskets in which it can be assumed that the drug has similar efficacy, based on an interim analysis. Thus, the second stage of the design could have a much smaller sample size for the same power to demonstrate clinical efficacy. The first stage of the design is based on the parallel, independent two-stage Simon’s design. When each basket has recruited a small number of patients, the heterogeneity in response across baskets is evaluated. If the results support the assumption that the drug’s effects are similar across baskets, either the trial is terminated for futility (if response is low) or a decision is made to continue to the second stage, at which all baskets will be pooled for analysis. If there is evidence of heterogeneity across the baskets, the trial will continue only for those baskets showing a promising level of response and these will be analysed separately at the end of the trial. This type of design answers the overall question of efficacy in the whole population more efficiently when there is evidence of homogeneity at an interim stage, while also shortening trial duration. 43 However, this is at the expense of loss of accuracy at assessing efficacy within each separate basket. 42 A different approach to testing has also been proposed, which replaces the question of whether or not there is response to therapy with the question of whether or not there are differences by tumour type (i.e. across baskets). 44
Although acknowledging the potential for heterogeneity, once a decision has been made on whether or not heterogeneity is present, the analysis proceeds either as separate independent studies for each basket or as a single aggregate study combining all of the baskets. Thus, either complete homogeneity or completely unrelated effects are assumed. A less restrictive assumption is that efficacy is similar (rather than equal or completely different) across baskets, with the different histologies not determining a particular ordering of effectiveness a priori (i.e. the baskets are exchangeable). Bayesian hierarchical models (BHMs)45,46 are particularly suited for this situation because they estimate the heterogeneity and allow information to be borrowed on the effects of the treatment across baskets, increasing precision of estimates compared with analysing all baskets separately, while reducing the chances of obtaining extreme estimates in baskets with few patients. Thall et al. 46 proposed a BHM that produces estimates of efficacy (e.g. probability of response) for each basket that are shrunken towards the mean efficacy (e.g. pooled probability of response) across all baskets. The model is an extension of a Bayesian Phase II design in which the trial is stopped if the posterior probability that the response rate is at least π* falls below a prespecified cut-off point, and can be applied to both binary and time-to-event (TTE) data. 47 Each basket is assumed to have a different treatment effect (event probability or event rate), θj, and these are assumed exchangeable (i.e. similar) and correlated a priori. Specifically, it is assumed that the θj follows a BHM, while allowing a separate stopping rule for each basket. Thus, the model will identify subgroups in which results are not promising, which can be dropped at a subsequent stage. Because the effects are assumed to be correlated across baskets, data from each individual basket will provide information on the effects in all of the other baskets, so that, for example, a longer survival time for a patient in a given basket will increase the posterior distributions of all θj, on average. In other words, information is borrowed across baskets, which shrinks the observed effects towards the pooled mean effect. Outputs from the resulting analysis include the posterior distributions for the effect (e.g. response or event rate) in each basket, the posterior distributions for the pooled effect across all baskets and the posterior distribution for the heterogeneity across baskets. In addition, a predictive distribution for the effect in a new study sampling baskets from the same overall population can be calculated to reflect the full degree of uncertainty owing to both the sample size and the observed heterogeneity in effects across the observed baskets. A Phase II trial of imatinib in 10 histological subtypes of sarcoma used this design: accrual within a sarcoma subtype would stop if it was unlikely that its response rate was at least 30%. 36,46,48
The BHM was shown to be a better design for a single-arm, non-randomised trial with a tumour response end point when there is a possibility of different effects in different subgroups of patients than Simon’s optimal two-stage design and the Bayesian adaptive design with no borrowing. 49 However, the hierarchical borrowing can make it more difficult to find a single basket in which the treatment is promising, although it is more likely than the other designs to correctly conclude futility or efficacy.
Any borrowing and precision gains from a BHM are advantageous only if the exchangeability assumption is reasonable. An approach for assessing homogeneity at an interim analysis and proceeding with a BHM in the second stage only if efficacy is deemed reasonably homogeneous has been proposed. 50 This approach avoids problems caused by implementing a complete pooling model at the second stage42 or proceeding with a fully exchangeable BHM when there is evidence of outlying baskets.
Hierarchical designs have been criticised when there is insufficient information in the outcome data to determine whether or not borrowing across subgroups is appropriate. 36,51 In addition, unknown between-subgroup heterogeneity, which drives the amount of borrowing, poses a major problem when the number of baskets is small (less than 10, as a rule of thumb)36 because it cannot be well inferred from the data and the results will be sensitive to model specification, in particular to the specification of the prior distribution for the borrowing parameter. 36,52 Alternatives to complete pooling or borrowing across all baskets have been proposed, which extend the BHM to allow borrowing of information across similar baskets while avoiding too optimistic borrowing for extreme baskets. 51,53–57
A model that allows non-exchangeable prior distributions to be specified was proposed for the scenario in which it is not expected a priori that all subgroups will be exchangeable. For example, some tumour types may be associated with a better or worse prognosis and their response to treatment is expected to differ. Different models can be used to implement this assumption: we can accept that a particular tumour characteristic (e.g. prognosis) defines exchangeability so that different categories are formed and exchangeability is allowed only between tumours in the same category (e.g. poor, intermediate and good prognosis), or we can treat the appropriate grouping as a random quantity to be estimated from the data, indexed by a categorical covariate of interest (e.g. prognosis). 53 Thus, the estimation of the treatment effect for a particular subgroup borrows more strength from other subgroups that, according to the prior beliefs, are more likely to be exchangeable, but the models allow the data to correct any prior beliefs that are not supported by the available data. When there is no a priori information on which subgroups might be exchangeable or not, an exchangeable–non-exchangeable model51 allows for selected special exchangeability patterns specified in the model to be determined by the treatment response data. This model extends the BHM to allow θj to be either exchangeable with some of the other subgroups or non-exchangeable with any of them, in which case the effect will be estimated independently of all other subgroups. Prior weights for the exchangeable probability of each subgroup are specified to reflect an a priori belief that a subgroup behaves systematically differently to the others. Essentially, the model determines whether some borrowing or no borrowing of information should be carried out across subgroups. Outputs include a global heterogeneity parameter across subgroups and mixture weights that describe the similarity of subgroups in the exchangeable component of the model, while also identifying subgroups that behave differently (i.e. show a low probability of being exchangeable). Although a pooled mean effect for the exchangeable component of the model can be obtained, the focus is on the effects for each individual subgroup, which incorporate different levels of borrowing according to the model. The prior distributions specified for the heterogeneity parameter and for the exchangeability weights can influence the results and need to be specified carefully. The use of this model for trial design requires careful consideration of the specification of the prior distributions and mixture weights, but has been found to perform well in various scenarios. 51 Extensions of these ideas to incorporate more information and, thus, improve performance of the trial design or simplify computation have been proposed. For example, the Bayesian latent subgroup trial design54 defines different latent subgroups within which more borrowing is allowed by jointly modelling biomarker measurements and treatment responses. This allows grouping of different cancers according to biomarker measurements routinely collected during a trial, effectively using internal trial information to inform the adaptive borrowing, which determines the decision to proceed to the next stage. Fujikawa et al. 56 proposed a Bayesian basket design that borrows information across the subgroups that have the most similar posterior distributions based on a prespecified threshold of similarity, which is simple to compute. Decisions can be made at the interim stage to stop or continue with the trial and this design can also determine which subgroups show efficacy in the final analysis, based on predefined criteria. Unlike the fully exchangeable BHM, in these models obtaining and interpreting predictive distributions of effects is not meaningful given that we can no longer reasonably assume that a new tumour type (subgroup) would have been sampled from the same distributions as the observed subgroups (i.e. we cannot assume that all subgroups are exchangeable).
Owing to the increased number of parameters being estimated, the hierarchical approach may increase uncertainty unnecessarily if response to treatment is indeed homogeneous across all subgroups. Therefore, when there is a strong rationale for expecting a uniform level of response it may be preferable to use a simple pooling of information across subgroups. 36 However, a priori assumptions of homogeneity in trial design or analysis need to be carefully justified because, in most cases, basket trials include patients with very clinically heterogeneous tumour types. In addition, the available empirical evidence does not generally support the assumption of homogeneity of activity of drugs across different histologies.
Previous basket trials have shown heterogeneity in the effectiveness of agents across tumour types, which lends support to the a priori assumption that effects may be heterogeneous. A recent trial32 of vemurafenib in 122 patients with BRAF V600–mutated cancers across multiple tumour types (including CRC, NSCLC, Erdheim–Chester disease and Langerhans’-cell histiocytosis, primary brain tumours, cholangiocarcinoma and anaplastic thyroid cancer) found evidence of response in some tumour types, including NSCLC and Erdheim–Chester disease and Langerhans cell histiocytosis, but not in CRC. 32 This heterogeneity in response was also observed in previous separate independent studies, which showed a positive response to vemurafenib in patients with BRAF-positive metastatic melanoma,58 but not in BRAF-positive colon cancer patients. 59 A trial of imatinib,60 a tyrosine kinase inhibitor (TKI), that included 196 patients across 40 different subtypes, found evidence of activity of imatinib in only five malignancies. Another basket trial of imatinib in 10 histological subtypes of advanced sarcoma concluded that, although rare dramatic responses were seen, imatinib was not an active agent in these subtypes, although it had previously shown effectiveness in another subtype of soft tissue sarcoma. 48 Similarly, trastuzumab (Herceptin, Roche, Basel, Switzerland), which is known to be effective in the treatment of women with HER2 (human epidermal growth factor receptor 2)-positive breast cancer,61 was not shown to be effective in HER2-positive recurrent endometrial cancer62 or HER2-positive NSCLC. 63 This evidence suggests that the treatment effects in different cancer types may not be exchangeable. Therefore, the design of basket trials should allow for the possibility of heterogeneity in treatment effects across tumour types, opting only for a design that assumes homogeneity in very special cases or where data from previous stages clearly support it.
Summary and implications
Complex innovative study designs are being used to address multiple clinical questions in an attempt to speed up regulatory approval and the access of drugs with new mechanisms of action to patients. Adaptive basket trials are particularly suited to assess efficacy of histology-independent drugs, although their reliance on surrogate outcomes, small sample sizes and mostly uncontrolled designs pose challenges for HTA.
A recent consensus statement has provided recommendations for the planning, design and statistical analysis of complex study designs, including considerations on ensuring their relevance for HTA. 25 These include encouraging comparative randomised studies; ensuring that the primary outcome, typically a surrogate of the clinical outcome of interest in HTA, is likely to adequately predict the clinical outcomes of interest; and using analysis methods that allow borrowing of information across baskets. 25
Although it is challenging to determine the correct level of borrowing of information (exchangeability) across baskets,25 the approaches described in Heterogeneity of effect in basket trials allow the treatment effect in any basket to be informed by the effects in all other baskets, therefore maximising the information available. Their interpretation and potential use in NICE TAs is described in Chapters 6 and 7.
Chapter 4 A systematic review to identify published meta-analyses evaluating the use of response rates and duration of response as surrogate end points for progression-free and overall survival
Parts of this chapter have been reproduced from Cooper et al. 64 This is an Open Access article distributed in accordance with the terms of the Creative Commons Attribution (CC BY 4.0) license, which permits others to distribute, remix, adapt and build upon this work, for commercial use, provided the original work is properly cited. See: https://creativecommons.org/licenses/by/4.0/. The text below includes minor additions and formatting changes to the original text.
Introduction
It is generally accepted that decisions about the use of new and existing health technologies should ideally be informed by estimates of treatment effects derived from high-quality randomised controlled trials (RCTs) that measure patient-relevant end points over a clinically appropriate time frame. Such ‘final’ end points typically involve the measurement of health benefits and adverse events that reflect aspects of the disease and its treatment that are important to patients (and potentially also their carers) and that relate to ‘how the patient feels, functions or survives’. 65 In the context of evaluating treatments for advanced/metastatic cancer, the key matter of concern is often whether or not the use of a given heath technology leads to improvements in OS (a final end point) compared with existing standard treatments. However, the estimation of treatment effects on OS may be subject to numerous problems, including potential confounding resulting from the use of post-progression treatments, insufficient study follow-up resulting in data immaturity or simply that data on OS have not been collected. In such instances, determining the impact of health technologies becomes more challenging and may rely on the use of other surrogate or intermediate end points to estimate treatment effects on final end points. These surrogate end points are intended to substitute for and predict a final patient-relevant clinical outcome. 66 In terms of advanced/metastatic cancer, potentially relevant surrogate end points may vary according to the tumour type and site, but commonly include PFS, time to progression (TTP) and response-based outcomes [such as ORR, CR, PR, very good partial response (VGPR) and DoR]. These surrogate end points are often considered attractive because they typically require smaller sample sizes, occur faster and are less expensive to collect in clinical trials than final outcomes, thereby reducing the costs associated with data collection and expediting the time required for bringing new technologies to market.
It has long been recognised that the reliance on surrogates may lead to invalid conclusions regarding the net health effects of technologies, which, in turn, have the potential to lead to patient harm. 67 Much of the published literature around the use of surrogate end points has focused on the development and application of frameworks for their validation. 68,69 In his seminal paper, Prentice68 put forward stringent criteria for the validation of surrogate end points in Phase III trials. In general terms, these criteria require that the surrogate end point must be a correlate of the net effect of treatment on the final clinical outcome; in other words, there must be a single pathway from the treatment to the true end point that is mediated exclusively by the surrogate end point. 70 Applied surrogate validation studies commonly adopt a meta-analytic (meta-regression) approach based on multiple studies to assess whether or not the apparent relationship between the surrogate and the final end point remains constant in the presence of various sources of heterogeneity, such as differences in patient population, study design and treatments received. 69
Based on the National Institutes of Health Biomarkers Definition Working Group’s preferred terms and definitions71 and the 1999 JAMA Users’ Guide,72 Elston and Taylor73 proposed a hierarchy of levels of surrogate validation. Level 3 of the hierarchy relates to biological plausibility; this is the weakest form of validation and is typically based on pathophysiological studies and/or an understanding of the disease process. Level 2 requires the presence of a consistent association between the surrogate outcome and the final end point; this may be assessed using observational studies or arm-based analyses of trials that have measured both the surrogate and the final outcome. This level of validation requires an assessment of the individual-level (absolute) association between end points and is usually undertaken using correlation analysis. Level 1 of the hierarchy represents the strongest level of surrogate validation; to achieve this level of validation, the treatment effects on the surrogate outcome must correspond to a commensurate treatment effect on the final outcome. Demonstrating this level of validity requires an analysis of correlation in terms of treatment effects between arms based on data from RCTs (sometimes referred to as trial-level association). Other validation frameworks have been proposed to assess the strength of association between surrogate and final end points. These include the criteria proposed by the German Institute for Quality and Efficiency in Health Care74 (IQWiG) (based on the treatment effect association only) and the Biomarker-Surrogate Evaluation Schema (BSES2) criteria75 (based on both absolute and treatment effect associations). These frameworks differ in terms of the types of analyses and the strength of the relationship required to determine the reliability of the surrogate.
The means by which health economic models use information on relationships between surrogate and final end points differ between appraisals, but may be broadly categorised into two general situations. First, data are available on both the surrogate and the final end points from one or more studies relating to the technology under consideration, and the relationship between the surrogate and the final end points is not informed by external data (and in some instances may not be quantified at all). Second, data are available on the impact of the technology on the surrogate end point, but information relating to the final end point from the same study is not available or is not used to inform the model. In this case, external data (e.g. meta-regressions and/or other forms of predictive model) may be required to quantify the relationship between the surrogate and the final end point. This review is more relevant to the second situation, whereby the degree of confidence that can be placed in the results of the model may be influenced by judgements about whether or not the surrogate can be considered valid.
In the context of histology-independent treatments, data on OS and potentially other TTE outcomes, such as PFS, are likely to be immature. Consequently, there may be a need to rely on surrogate outcomes, such as response rate, using data from external sources to estimate other more clinically meaningful final outcomes. This section presents a systematic review of response-based outcomes as surrogates for PFS, TTP and OS in advanced or metastatic cancer, across any tumour site. The review focuses on meta-analyses and meta-regressions. Analyses are presented both for absolute associations and for treatment-effect associations between response-based outcomes and PFS, TTP and/or OS. In addition, the IQWiG and BSES2 criteria are used to assess the strength of association between surrogate and final end points. Where data permit, the review also explores the surrogate threshold effect (STE) associated with response-based outcomes: this corresponds to the smallest treatment effect on the surrogate that predicts a non-zero treatment effect on the true end point. 76
Where available, the results of published regression models are also reported; if the ORR was deemed to be valid in one or more tumour types, one option would be to use the coefficients from models to quantify the relationship between ORR and PFS/OS. Other approaches for incorporating surrogate outcomes in health economic models are discussed at the end of the chapter.
Methods
Review question
This systematic review sought to address the following research question: ‘What is the strength of the association between response outcomes and PFS, TTP or OS across different types of cancer (primarily advanced or metastatic), based on meta-analyses or meta-regression studies assessing the statistical relationship between these outcomes?’.
Inclusion and exclusion criteria
The inclusion and exclusion criteria for the review are shown in Table 1. Inclusion was restricted to articles that reported meta-analyses and meta-regressions across multiple studies and that reported the strength of association between response outcomes (ORR, CR, PR, VGPR or DoR) and PFS, TTP or OS. The included meta-regressions could themselves include RCTs and/or single-arm studies. However, individual reports analysing single trials or single cohorts were excluded. Included meta-analyses could report absolute associations and/or treatment effect associations. These associations had to be reported as a correlation coefficient (e.g. Pearson r or Spearman’s rs) and/or a coefficient of determination (R2) between relevant outcomes.
Field | Inclusion | Exclusion |
---|---|---|
Disease area |
|
|
Surrogate end points | Response end points:
|
|
Final end points |
|
|
Study and data type |
|
|
Type of analysis reported |
|
|
Language |
|
|
Studies of any cancer and any treatment were included. The review focused mainly on studies of advanced or metastatic cancers (and/or treatment with palliative intent) because these studies were more likely to report PFS and OS. However, studies reporting relevant outcomes were included even where the stage was not specifically restricted to advanced/metastatic disease for all patients or where this was unclear (this applied particularly to haematological cancers). Studies were excluded if they explicitly referred to adjuvant or neo-adjuvant treatment or treatments that are given with curative intent.
Search strategy
Five databases [MEDLINE, EMBASE™ (Elsevier, Amsterdam, the Netherlands), Web of Science™ (Clarivate Analytics, Philadelphia, PA, USA), the Cochrane Database of Systematic Reviews and Cumulative Index to Nursing and Allied Health Literature (CINAHL)] were searched from inception to March 2019. Search terms included cancer terms AND response terms AND terms for PFS, TTP and/or OS AND terms for regression, correlation, prediction, association or relationship AND terms for end point and/or surrogate. Search results were limited to the English language and to studies undertaken in humans. The MEDLINE search strategy is provided in Appendix 3.
In addition, a citation search was undertaken based on two existing meta-reviews77,78 of surrogate relationships; this identified studies that have cited any of the 48 articles included in the review by Fischer et al. 77 and/or any of the 19 articles included in the review by Davis et al. 78 In addition, relevant existing meta-reviews, including Fischer et al. ,77 Davis et al. ,78 Savina et al. 79 and Haslam et al. ,80 and any further reviews identified during searching, were checked for relevant studies.
Study selection process
The titles and abstracts of the articles retrieved by the search were examined by one reviewer and a subset were checked by a second reviewer early in the process, followed by a discussion to ensure that there was consistency in the selection decisions. Full texts were examined by one reviewer and a subset were checked by a second reviewer, with any discrepancies resolved through discussion.
Data extraction
Data were extracted by one reviewer and all data were checked by a second reviewer. The following data were extracted:
-
author and date
-
cancer type and stage, number of patients, number of included studies and the design of included studies (RCT or single arm and publication dates)
-
treatment type, treatment line and other subgroups, as reported
-
data type [aggregate-level data or individual patient data (IPD)]
-
surrogate and final end points analysed (e.g. ORR to OS)
-
response criteria used, if reported (e.g. RECIST)
-
measures of outcomes [e.g. hazard ratio (HR), odds ratio (OR), relative risk (RR) or difference between medians]
-
statistical methods for correlation and regression, whether weighted, whether adjusted, coefficient reported [e.g. Pearson or Spearman correlation coefficient (r or rs), regression coefficient of determination (R2)]
-
absolute association results (i.e. between absolute values of the surrogate and final end points based on data from individual arms of RCTs or single-arm studies) – correlation coefficient, regression R2 and regression equation
-
treatment effect association results (i.e. between treatment effects for surrogate and treatment effects for final end points, based on between-group differences from RCTs) – correlation coefficient, regression R2 and regression equation
-
data, as above, for subgroups
-
STE,76 that is the smallest treatment effect on the surrogate that predicts a non-zero treatment effect on the true end point.
Data synthesis
Data were tabulated and described in a narrative synthesis. Plots were constructed to illustrate the reported associations. Some of the included meta-regression studies reported multiple subgroup analyses with differing results. Therefore, for associations between absolute values of end points, the plots show the range of correlation coefficients per study, across all subgroup analyses. Where an included meta-regression study reported on more than one cancer type, these are shown separately on the plots. All types of correlation coefficient were included, for example Pearson’s r and Spearman’s rs. If no correlation coefficient was reported, Pearson’s r was calculated as the square root of R2, if available.
For associations between treatment effects, the plots show the range of regression coefficients of determination (R2) per study, across all subgroup analyses. The plots include both adjusted and unadjusted R2 values, as well as values from weighted and unweighted regressions. For studies in which R2 was not reported, this was calculated as the square of the Pearson’s (r) correlation coefficient, if available. R2 was not calculated from other correlation coefficients, such as Spearman’s r, or where the method of correlation was unclear.
Scoring the strength of association
Two separate sets of criteria have been developed to assess the strength of association between end points. These include the criteria proposed by IQWiG74 (based on the treatment effect association) and the BSES2 criteria75 (based on both absolute and treatment effect associations). In this review, both the IQWiG and the BSES2 criteria were used to assess the strength of association between the surrogate and the final end points.
The IQWiG criteria74 (Table 2) are based on the correlation coefficient (r) for the treatment effect association. Where r was not reported it was calculated as the square root of R2, if available. Some slight modifications were made to the IQWiG scoring criteria because the medium score bracket was not clearly defined (see Table 2); these modifications were based on the approach used in the previous review by Savina et al. 79 The IQWiG score was generated based on the magnitude of r, irrespective of its sign (i.e. a negative correlation could generate a high score).
IQWiG score | Criteria (based on r for treatment–effect association)a |
---|---|
High | The lower CI of r is ≥ 0.85 |
Medium +b | r ≥ 0.85 with no reported CI or r ≥ 0.85 with wide CIs (lower limit < 0.85) |
Medium | 0.85 > r ≥ 0.7 and the upper CI of r is ≥ 0.7 and the lower CI of r is < 0.85, or 0.85 > r ≥ 0.7 with no reported CI |
Low | The upper CI of r is < 0.7 or r < 0.7 with no reported CI |
The BSES2 criteria75 (Table 3) require R2 values for both the individual and the treatment effect associations. Where R2 was not reported, it was calculated as the square of r, if available. BSES2 criteria were used as an adaptation from the original BSES criteria, as described in Savina et al. 79 The original BSES criteria require R2 for both individual-level and treatment effect associations and a value for the STE. Given that so few articles report STE, this review used BSES2, which does not require the STE.
BSES2 score | Criteria (based on R2 for both treatment effect and individual-level associations)a |
---|---|
Excellent | R2 (treatment effect) ≥ 0.6 and R2 (absolute) ≥ 0.6 |
Good | R2 (treatment effect) ≥ 0.4 and R2 (absolute) ≥ 0.4 |
Fair | R2 (treatment effect) ≥ 0.2 and R2 (absolute) ≥ 0.2 |
Poor | R2 (treatment effect) < 0.2 and/or R2 (absolute) < 0.2 |
Results
Number of included studies
The literature search generated 2829 citations (Figure 1), of which 2630 were excluded during the review of titles and abstracts. In total, 64 references to 63 studies were included in the review. 81–144 The study characteristics for the 63 included studies are shown in Appendix 4. The detailed results of the included studies are shown in Appendices 5 and 6. Studies excluded at the full-text stage, with reasons for exclusion, are listed in Appendix 7.
FIGURE 1.
The PRISMA flow diagram for study inclusion. Reproduced with permission from Cooper et al. 64 This is an Open Access article distributed in accordance with the terms of the Creative Commons Attribution (CC BY 4.0) license, which permits others to distribute, remix, adapt and build upon this work, for commercial use, provided the original work is properly cited. See: http://creativecommons.org/licenses/by/4.0/. The figure includes minor additions and formatting changes to the original figure.
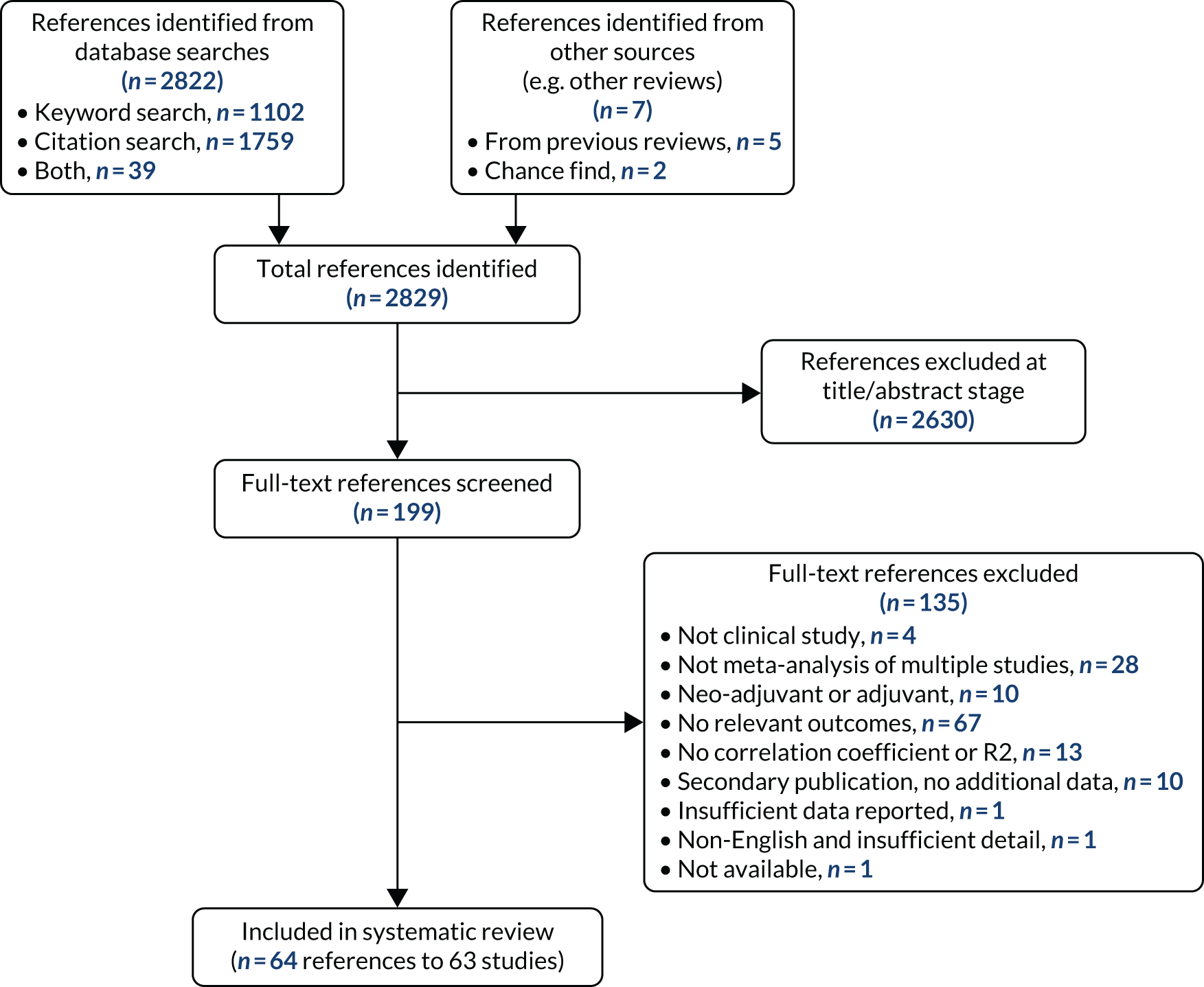
Characteristics of the included studies
Full details of the study characteristics for the 63 included studies are shown in Appendix 4 (note that eight references81,109,111,128,130,140,141,144 appear on more than one row because they report on more than one cancer type).
Surrogate relationships, cancer types and treatments
A summary of the surrogate relationships, cancer types and treatments is provided in Table 4. The most commonly reported surrogate relationships were ORR to OS (n = 57 studies), ORR to PFS (n = 22 studies), CR to OS (n = 8 studies) and CR to PFS (n = 7 studies). Other response outcomes (DoR, PR and VGPR/CR) were reported in only one to two studies each.
Surrogate relationship | Cancer type | Disease stage | Line of treatment | Treatment type |
---|---|---|---|---|
|
|
|
|
|
Twenty different cancer types were analysed (see Table 4), the most common being NSCLC (n = 16 studies), CRC (n = 10 studies), various solid tumours (n = 8 studies) and breast cancer (n = 5 studies). The disease stage was advanced/metastatic in 43 studies and unclear in nine studies (see Table 4), while the remainder (n = 11 studies) gave other descriptions, mostly indicating advanced, extensive or recurrent disease. The treatment was first line in 23 studies, later lines or combinations of lines in 32 studies, and not reported in eight studies. The treatment type was chemotherapy in 21 studies, immune checkpoint inhibitors in nine studies, targeted therapy in eight studies and various other treatment combinations in the remainder of the studies.
Data types reported
Table 5 summarises the data types reported in the included meta-regressions. The various meta-regressions included between four and 191 primary studies and between 407 and 44,125 patients each. The majority of meta-regressions (n = 44) included only RCTs, while 17 included both RCTs and single-arm studies and two included single-arm studies only. Most of the meta-regressions (n = 58) analysed aggregate data (e.g. medians or another summary measure per study arm), while five analysed IPD. Across all meta-regressions, 32 reported absolute (individual-level) associations, 38 reported treatment effect (trial-level) associations and only four reported the STE.
Number of primary studies per meta-regression | Number of patients per meta-regression | Included study types per meta-regression | Data types | Absolute association reported | Treatment effect association reported | STE reported |
---|---|---|---|---|---|---|
n = 4–191 | n = 407–44,125 |
|
|
n = 32 | n = 38 | n = 4 |
Results of the included studies
Absolute (individual-level) correlation and regression
The range of the absolute (individual-level) correlation coefficients reported in each meta-regression is summarised in Table 6 and illustrated in Figures 2 (for the association between ORR and PFS) and 3 (for the association between ORR and OS). Each horizontal row in the plots illustrates the range of correlation coefficients across all subgroup analyses within a single meta-regression study. Where an included meta-regression reported on more than one cancer type, these are shown separately on the plots. It is worth noting that the meta-regressions varied both in terms of the number of included primary studies (shown as N on the plots) and in terms of the treatment type, line of treatment and precise clinical population; all of these details are provided in Appendix 5, together with correlation coefficients for all individual subgroup analyses.
Surrogate relationship | Number of studies | Cancer types and references | Range of r or rs across studies and subgroup analyses | Further details |
---|---|---|---|---|
ORR to PFS | 12 | NSCLC,108,128,141 ovarian,129,135 RCC,126 NHL,117 SCLC,122 MM,118 CRC,115 CUP,125 NET107 and various141 | –0.72 to 0.96 | See Appendix 5 and Figure 2 |
ORR to TTP | 1 | Gastric105 | 0.41 to 0.56 | See Appendix 5 |
ORR to OS | 27 | NSCLC,108,112,113,128,131,134,141 CRC,98,115,138 ovarian,129,135 breast,114,127 gastric,105,133 various,123,128,141 pancreatic,100 RCC,81,126 gastro-oesophageal,124 urothelial,81,82 AML,83 SCLC,122 glioblastoma,101 CUP125 and NET106 | –0.40 to 1.00 | See Appendix 5 and Figure 3 |
CR to PFS | 2 | SCLC122 and NHL144 | 0.22 to 0.83 | See Appendix 5 |
CR to OS | 3 | NSCLC,112 SCLC122 and gastro-oesophageal124 | –0.04 to 0.62 | See Appendix 5 |
PR to PFS | 1 | SCLC122 | 0.35 to 0.70 | See Appendix 5 |
PR to OS | 1 | SCLC122 | 0.29 to 0.66 | See Appendix 5 |
VGPR/CR to PFS | 0 | – | a | See Appendix 5 |
DoR to PFS | 0 | – | – | – |
DoR to OS | 0 | – | – | – |
FIGURE 2.
Correlation (r or rs) between the absolute (individual-level) value of ORR and the absolute (individual-level) value of PFS. For each study, the plot illustrates the range of correlation coefficients across all subgroup analyses. N represents the number of studies included in each meta-regression. CUP, cancer of unknown primary. Reproduced with permission from Cooper et al. 64 This is an Open Access article distributed in accordance with the terms of the Creative Commons Attribution (CC BY 4.0) license, which permits others to distribute, remix, adapt and build upon this work, for commercial use, provided the original work is properly cited. See: http://creativecommons.org/licenses/by/4.0/. The figure includes minor additions and formatting changes to the original figure.
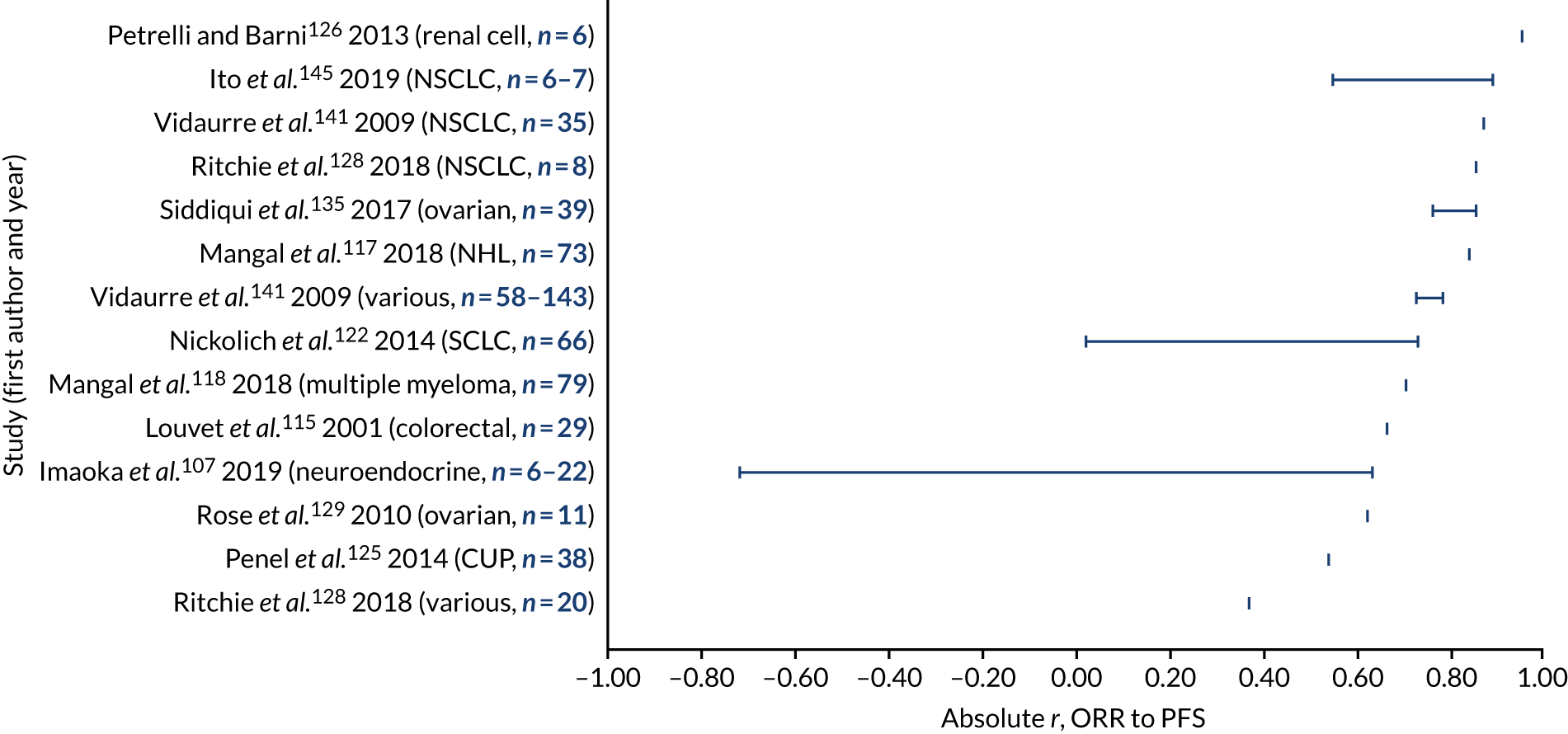
FIGURE 3.
Correlation (r or rs) between the absolute (individual-level) value of ORR and the absolute (individual-level) value of OS. For each study, the plot illustrates the range of correlation coefficients across all subgroup analyses. N represents the number of studies included in each meta-regression. AML, acute myeloid leukaemia; CUP, cancer of unknown primary. Reproduced with permission from Cooper et al. 64 This is an Open Access article distributed in accordance with the terms of the Creative Commons Attribution (CC BY 4.0) license, which permits others to distribute, remix, adapt and build upon this work, for commercial use, provided the original work is properly cited. See: http://creativecommons.org/licenses/by/4.0/. The figure includes minor additions and formatting changes to the original figure.
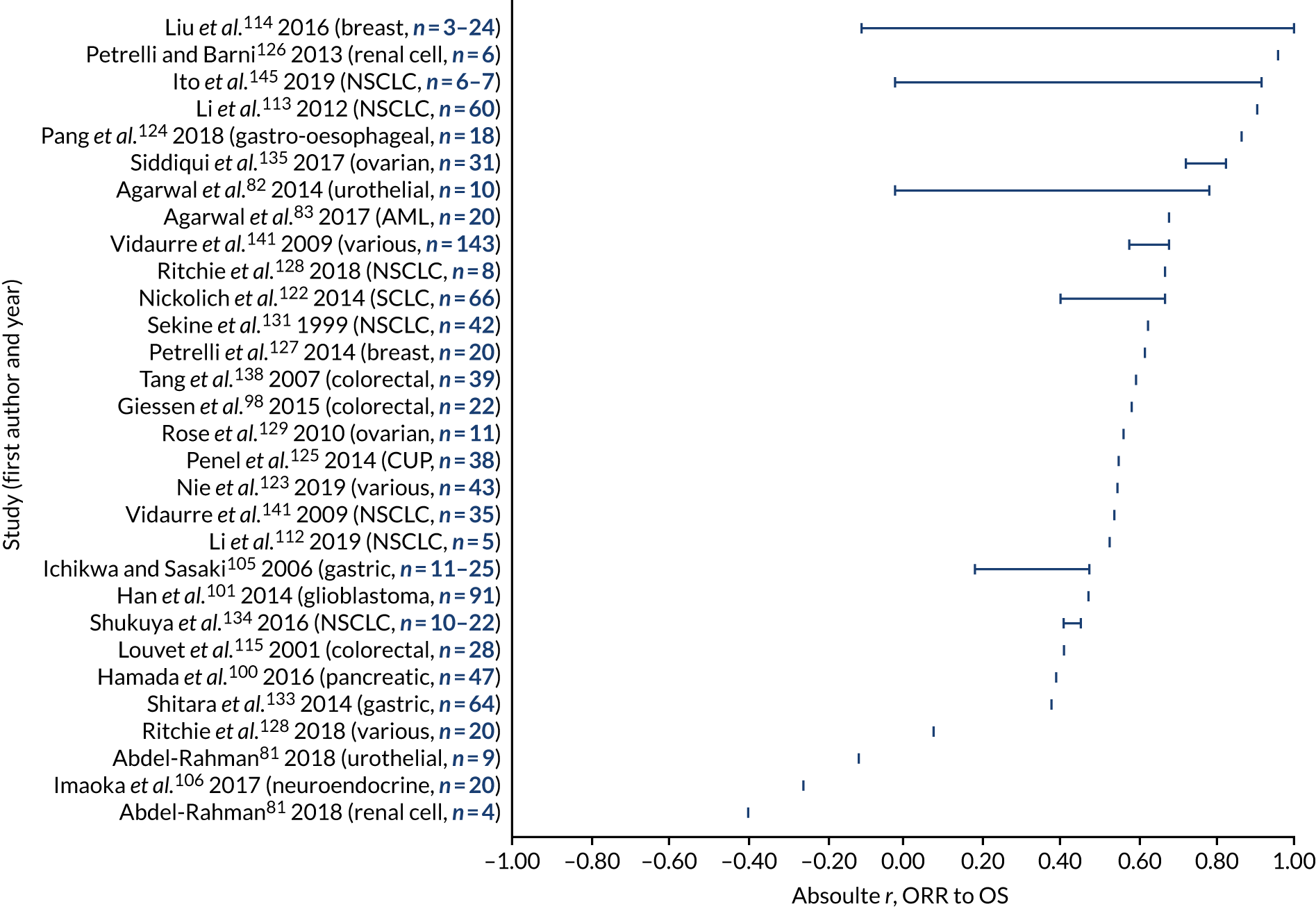
Overall response rate and progression-free survival (or time to progression)
The reported correlation coefficients (Pearson’s r or Spearman’s rs) between absolute ORR and PFS ranged from –0.72 to 0.96, based on multiple analyses within 12 studies across 10 cancer types (see Figure 2 and Table 6; full details in Appendix 5). 107,108,115,117,118,122,125,126,128,129,135,141 Across those studies that report only a single analysis, the correlation coefficient was generally above 0.60; however, some estimates were lower. Confidence intervals around the correlation coefficients were rarely reported (not shown in Figure 2; see Appendix 5). Few separate meta-regressions reported on the same tumour site; therefore, it is difficult to assess whether or not the ORR may be a more reliable surrogate in certain cancer types than others. One study reported on the ORR and TTP (gastric cancer, correlation rs = 0.41 to 0.56 across subgroup analyses, not shown on the plot). 105
Overall response rate and overall survival
The reported correlation coefficients between absolute ORR and OS ranged from –0.40 to 1.00, based on 27 studies across 15 cancer types (see Figure 3 and Table 6; full details in Appendix 5). 81–83,98,100,101,105,106,108,112–115,122–129,131,133–135,138,141 The CIs around the correlation coefficients, where reported, were generally fairly wide (not shown in Figure 3). The majority of correlation coefficients were above 0.40; however, several estimates were lower. The correlation coefficients reported from multiple analyses within the same study, and those reported across separate studies, did not suggest a clear pattern by cancer type.
Complete response and progression-free survival or overall survival
The correlation coefficients between absolute CR and PFS in two studies of SCLC122 and non-Hodgkin’s lymphoma (NHL)144 ranged from 0.22 to 0.83, while the correlation coefficients between absolute CR and OS ranged from –0.04 to 0.62, based on three studies of NSCLC,112 SCLC122 and gastro-oesophageal cancer124 (see Table 6; full details in Appendix 5).
Partial response and progression-free survival or overall survival
The correlation coefficient between absolute PR and PFS ranged from 0.35 to 0.70 across subgroup analyses within one study of SCLC,122 while the highest correlation coefficient between absolute PR and OS ranged from 0.29 to 0.66 in the same study122 (see Table 6; full details in Appendix 5).
Duration of response and progression-free survival or overall survival
No studies reported on the absolute association between DoR and PFS or OS.
Treatment effect (trial-level) correlation and regression
The range of treatment effect (trial-level) R2 values reported in each meta-regression is summarised in Table 7 and illustrated in Figures 4 (for the association between ORR and PFS) and 5 (for the association between ORR and OS). Each horizontal row in the plots illustrates the range of R2 values across all subgroup analyses within a single meta-regression study. Where an included meta-regression reported on more than one cancer type, these are shown separately on the plots. It is worth noting that the meta-regressions varied both in terms of the number of included primary studies (shown as n on the plots) and in terms of the treatment type, line of treatment and precise clinical population; all of these details are provided in Appendix 6, together with R2 values for all individual subgroup analyses.
Surrogate relationship | Number of studies | Cancer types and references | Range of R2 across studies and subgroup analyses | Further details |
---|---|---|---|---|
ORR to PFS | 9 | NSCLC,84,85,108,140 ovarian,90,135 various130,142 and CRC89 | 0.18 to 0.94 | See Appendix 6 and Figure 4 |
ORR to TTP | 0 | – | – | |
ORR to OS | 30 | NSCLC,84,85,103,108,109,121,140 CRC,88,89,92,94,136 various,110,120,123,130,142 pancreatic,91,100,116 SCLC,97,104 RCC,95,126 breast,86,99 ovarian,90 prostate,93 BTC119 and soft tissue sarcoma137 | –0.08 to 0.84 | See Appendix 6 and Figure 5 |
CR to PFS | 1 | NHL132 | 0.45 to 0.93 | See Appendix 6 |
CR to OS | 2 | Breast99 and SCLC97 | 0.05 to 0.48 | See Appendix 6 |
PR to PFS | 0 | – | – | |
PR to OS | 0 | – | – | |
DoR to PFS | 0 | – | See Appendix 6 | |
DoR to OS | 0 | a | See Appendix 6 |
FIGURE 4.
Regression R2 between treatment effects (trial level) for ORR and PFS. For each study, the plot illustrates the range of correlation coefficients across all subgroup analyses. N represents the number of studies included in each meta-regression. Reproduced with permission from Cooper et al. 64 This is an Open Access article distributed in accordance with the terms of the Creative Commons Attribution (CC BY 4.0) license, which permits others to distribute, remix, adapt and build upon this work, for commercial use, provided the original work is properly cited. See: http://creativecommons.org/licenses/by/4.0/. The figure includes minor additions and formatting changes to the original figure.
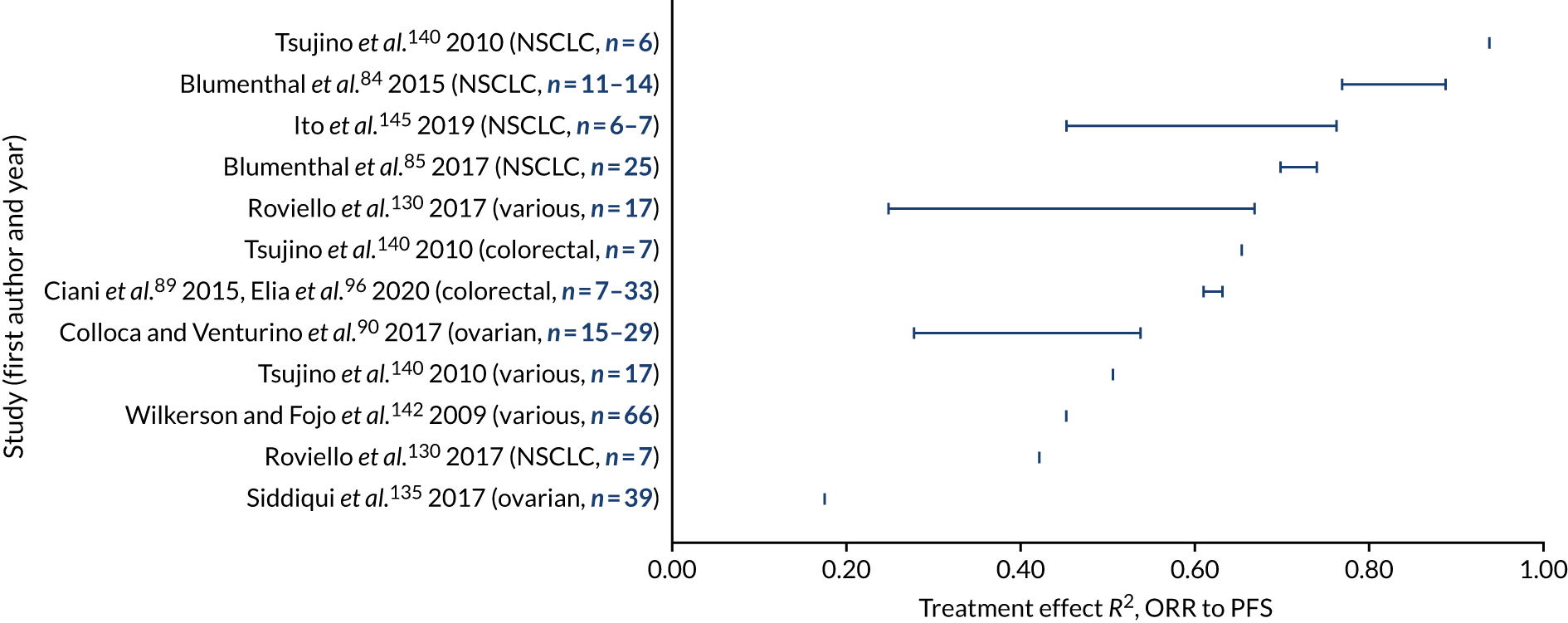
FIGURE 5.
Regression R2 between treatment effects (trial level) for ORR and OS. For each study, the plot illustrates the range of correlation coefficients across all subgroup analyses. N represents the number of studies included in each meta-regression. Reproduced with permission from Cooper et al. 64 This is an Open Access article distributed in accordance with the terms of the Creative Commons Attribution (CC BY 4.0) license, which permits others to distribute, remix, adapt and build upon this work, for commercial use, provided the original work is properly cited. See: http://creativecommons.org/licenses/by/4.0/. The figure includes minor additions and formatting changes to the original figure.
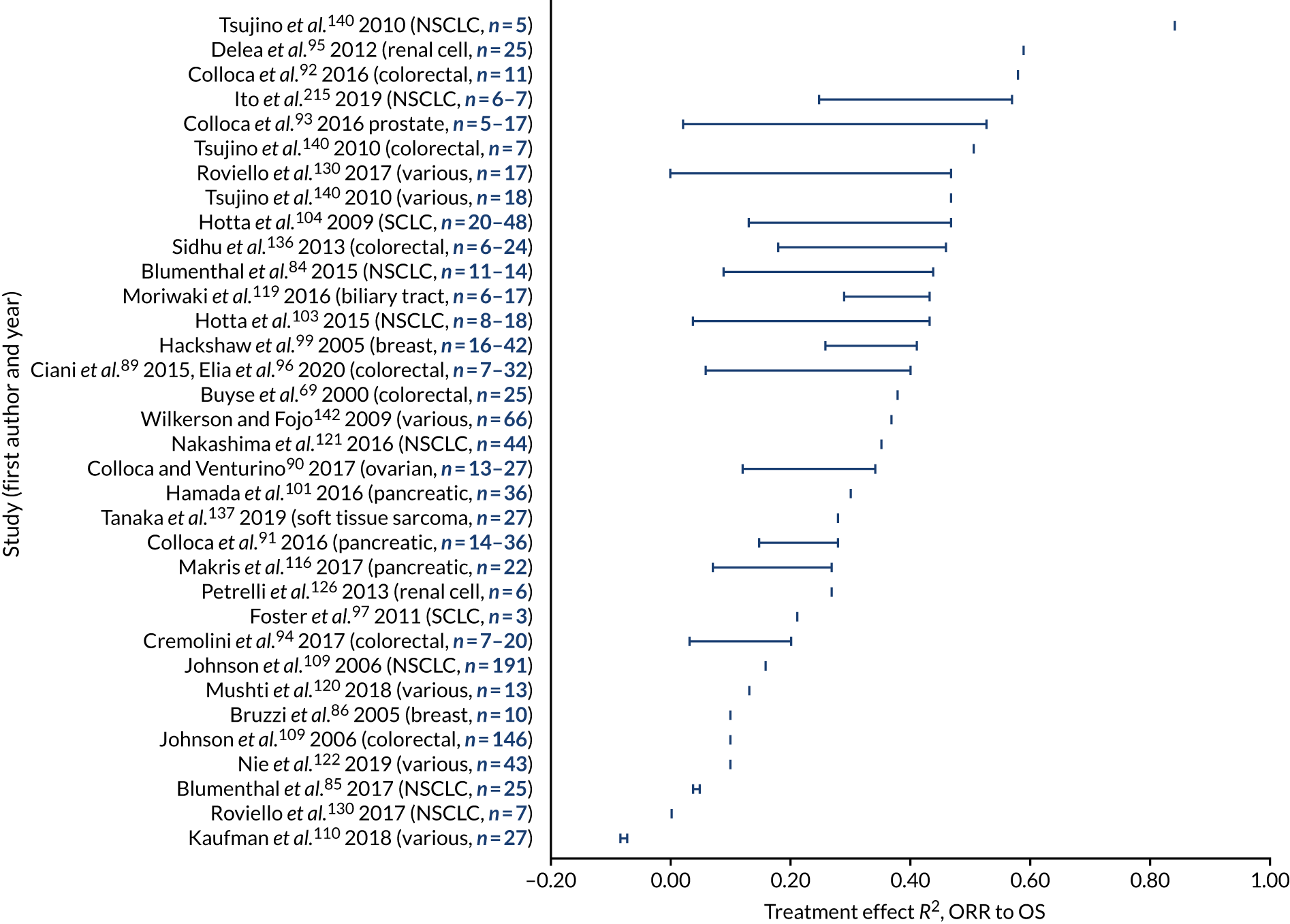
Overall response rate and progression-free survival
The regression R2 values for the treatment effect association between ORR and PFS ranged from 0.18 to 0.94, based on nine studies across four cancer types: NSCLC,84,85,108,140 ovarian cancer,90,135 CRC89 and various solid tumours130,142 (see Figure 4 and Table 7; full details in Appendix 6). The majority of R2 values were above 0.40. The R2 values that were reported from multiple analyses within the same study and those that were reported across separate studies did not suggest a clear pattern by cancer type. Confidence intervals around the R2 values, where reported, were generally fairly wide (not shown in Figure 4; see Appendix 6).
Overall response rate and overall survival
The regression R2 values for the treatment effect association between ORR and OS ranged from –0.08 to 0.84, based on 30 studies across 11 cancer types (see Figure 5 and Table 7; full details in Appendix 6). 84–86,88–95,97,99,100,103,104,108–110,116,119–121,123,126,130,136,137,140,142 With the exception of one analysis, all R2 values were below 0.60. The R2 values that were reported from multiple analyses within the same study and those that were reported across separate studies did not suggest a clear pattern by cancer type. Confidence intervals around the R2 values, where reported, were generally wide (not shown in Figure 5).
Complete response and progression-free survival or overall survival
The regression R2 for the treatment effect association between CR and PFS ranged from 0.45 to 0.93 in one study of NHL,132 while the regression R2 for the treatment effect association between CR and OS within two studies of breast cancer99 and SCLC97 ranged from 0.05 to 0.48 (see Table 7; full details in Appendix 6).
Partial response and progression-free survival or overall survival
No studies reported the treatment effect association between PR and PFS or OS.
Duration of response and progression-free survival or overall survival
No studies reported R2 between DoR and OS or PFS. Two studies in CRC92 and pancreatic cancer91 reported Spearman’s correlation coefficients between DoR and OS, ranging from 0.40 to 0.76 (see Table 7; full details in Appendix 6).
Regression equations
Regression equations for absolute (individual-level) relationships
Regression equations for absolute (individual-level) associations were reported in six studies105,115,117,135,139,144 and are summarised in Table 8.
Surrogate relationship | Cancer types and references | Surrogate | Final | Intercept | Slope |
---|---|---|---|---|---|
ORR to PFS | Colorectal115 | ORR | Median PFS | 3.20 | 0.10 |
Lung (NSCLC)139 | ORR | Median PFS | NR | 0.07 | |
Ovarian135 | ORR | Median PFS | 2.59 | 0.12 | |
NHL117 | Log-odds ORR | Log-median PFS | 1.97 | 0.41 | |
ORR to TTP | Gastric105 | ORR | Median TTP | 1.73 | 0.09 |
ORR to OS | Colorectal115 | ORR | Median OS | 10.45 | 0.09 |
Lung (NSCLC)139 | ORR | Median OS | NR | 0.26 | |
Ovarian135 | ORR | Median OS | 9.48 | 0.28 | |
Gastric105 | ORR | Median OS | 5.89 | 0.08 | |
CR to PFS | NHL144 | CR | Median PFS | 0.83 | 0.46 |
NHL117 | Log-odds CR | Log-median PFS | 2.38 | 0.34 |
For the relationship between the ORR and the median PFS/TTP, five studies across five cancer types105,115,117,135,139 reported regression equations (one study used log-odds ORR),117 with intercepts ranging from 1.73 to 3.20 and slopes ranging from 0.07 to 0.41.
Regression equations for treatment effect (trial-level) relationships
The regression equations for treatment effect (trial-level) associations were reported in 13 studies87,89,94–96,99,104,109,119,121,130,132,140 and are summarised in Table 9. These are presented separately for regressions based on the difference in response and regressions based on the RR or OR for response. There was substantial variation in effect measures for both the surrogate and the final outcomes (e.g. difference in medians, HR and OR).
Surrogate relationship | Cancer types and references | Subgroup | Based on difference in response | Based on RR or OR for response | ||||||
---|---|---|---|---|---|---|---|---|---|---|
Surrogate | Final | Intercept | Slope | Surrogate | Final | Intercept | Slope | |||
ORR to PFS | Lung (NSCLC)140 | Difference in ORR | HR PFS | NR | –0.02 | |||||
Colorectal140 | Difference in ORR | HR PFS | NR | –0.04 | ||||||
Various140 | Difference in ORR | HR PFS | NR | –0.02 | ||||||
Colorectal89,96 | log-OR ORR | log-HR PFS | –0.05 | –0.32 | ||||||
Breast87 | log-OR ORR | log-HR PFS | 0.10 | 0.50 | ||||||
Various (immuno)130 | log-OR ORR | log-HR PFS | –0.13 | –0.24 | ||||||
ORR to OS | Colorectal94 | All | Difference in ORR | Difference in median OS | NR | 0.07 | ||||
Anti-angiogenic | 0.13 | |||||||||
Non-anti-angiogenic | 0.14 | |||||||||
Colorectal109 | Difference in ORR | Difference in median OS | 0.34 | 0.10 | ||||||
Lung (NSCLC)109 | Difference in ORR | Difference in median OS | –0.05 | 0.09 | ||||||
Colorectal140 | Difference in ORR | HR OS | NR | –0.03 | ||||||
Lung (NSCLC)140 | Difference in ORR | HR OS | NR | –0.01 | ||||||
Various140 | Difference in ORR | HR OS | NR | –0.02 | ||||||
Colorectal89,96 | All | log-OR ORR | log-HR OS | –0.03 | –0.05 | |||||
No crossover | –0.04 | –0.10 | ||||||||
Breast99 | All | log-OR ORR | log-HR OS | –0.01 | 0.28 | |||||
Recruited pre-1990 | NR | 0.28 | ||||||||
Recruited 1990 or after | NR | 0.24 | ||||||||
Lung (NSCLC)121 | ln-OR ORR | ln-HR OS | –0.02 | –0.13 | ||||||
Various (immuno)130 | log-OR ORR | log-HR OS | –0.13 | –0.26 | ||||||
Colorectal94 | All | RR of ORR | HR OS | NR | –0.03 | |||||
Anti-angiogenic | –0.11 | |||||||||
Non-anti-angiogenic | –0.06 | |||||||||
Renal cell95 | ln-RR ORR | -ln-HR OS | –0.11 | 0.30 | ||||||
Biliary tract119 | Chemotherapy | Ratio of ORR | Log-ratio of median OS | 0.01 | 0.28 | |||||
Gemcitabine | 0.02 | 0.27 | ||||||||
Targeted therapy | 0.12 | 0.16 | ||||||||
Lung (SCLC)104 | All | RR of ORR | Difference in median OS | 0.00 | 0.06 | |||||
Published 1990–96 | 0.00 | 0.04 | ||||||||
Published 1997–2008 | 0.00 | 0.09 | ||||||||
CR to PFS | NHL132 | log-OR CR at 30 months | log-HR PFS | –0.09 | –0.64 | |||||
NHL132 | log-OR CR at 24 months | log-HR PFS | 0.04 | –0.73 | ||||||
CR to OS | Breast99 | All | log-OR CR | log-HR OS | –0.01 | 0.13 | ||||
Recruited pre-1990 | NR | 0.09 | ||||||||
Recruited 1990 or after | NR | 0.16 |
For the relationship between ORR and PFS, one study of three cancer types140 reported regression equations for the difference in ORR compared with the HR for PFS, with slopes ranging from –0.02 to –0.04 (intercepts were not reported). Three studies across three cancer types87,89,96,130 reported regression equations for the log-OR for ORR compared with the log-HR for PFS, with intercepts ranging from –0.13 to 0.10 and slopes ranging from –0.32 to 0.50.
For the relationship between ORR and OS, two studies in colorectal cancer94,109 and NSCLC109 reported regression equations for the difference in ORR compared with the difference in median OS, with intercepts ranging from –0.05 to 0.34 and slopes ranging from 0.07 to 0.14. One study of three cancer types140 reported regression equations for the difference in ORR compared with the HR for OS, with slopes ranging from –0.01 to –0.03 (intercepts were not reported). Seven studies across six cancer types89,94–96,99,119,121,130 reported regression equations for the ratio measures of ORR (OR or RR) compared with the ratio measures of OS (generally HR), with intercepts ranging from –0.13 to 0.12 and slopes ranging from –0.26 to 0.30. One study in SCLC104 reported a regression equation for the RR of ORR compared with the difference in median OS, with an intercept of 0 and slopes ranging from 0.04 to 0.09.
For the relationship between CR and PFS, one study in NHL132 reported regression equations for log-OR CR compared with log-HR PFS, with intercepts ranging from –0.09 to 0.04 and slopes ranging from –0.73 to –0.64.
For the relationship between CR and OS, one study in breast cancer99 reported regression equations for log-OR CR compared with log-HR PFS, with an intercept of –0.01 (where reported) and slopes ranging from 0.09 to 0.16.
Surrogate threshold effect
The STE (the smallest treatment effect on the surrogate that predicts a non-zero treatment effect on the true end point)76 was reported in only four studies (Table 10). 89,102,132,140 For the relationship between ORR and PFS, one study of various solid tumours140 reported that a difference in ORR of 15% would be required to predict a non-zero treatment effect on the HR for PFS. For the relationship between ORR and OS, two studies in various solid tumours140 and NSCLC102 reported that a difference in ORR of 21% and 55%, respectively, would be required to predict a non-zero treatment effect on the HR for OS. In addition, one study102 reported that a difference in ORR of 41% would be required to predict a non-zero treatment effect on the difference in median OS, and a further study in CRC89 reported that an OR of 0.28 for ORR would be required to predict a non-zero treatment effect on the OR for OS. Finally, for the relationship between CR and PFS, one study in NHL132 reported that an OR of 1.56 for CR (at 30 months) would be required to predict a non-zero treatment effect on the HR for PFS.
Surrogate relationship | Cancer types and references | Based on difference in response | Based on OR for response | ||||
---|---|---|---|---|---|---|---|
Surrogate | Final | STE (%) | Surrogate | Final | STE | ||
ORR to PFS | Various140 | Difference in ORR | HR PFS | 15 | |||
ORR to OS | Colorectal89 | OR ORR | OR OS | 0.28 | |||
NSCLC102 | Difference in ORR | HR OS | 55 | ||||
Difference in ORR | Difference in median OS | 41 | |||||
Various140 | Difference in ORR | HR OS | 21 | ||||
CR to PFS | NHL132 | OR CR at 30 months | HR PFS | 1.56 |
Institute for Quality and Efficiency in Health Care and Biomarker-Surrogate Evaluation Schema-2 scores for the strength of association
This section reports the results from the IQWiG and BSES2 scoring for the strength of association between surrogate and final end points. As described in Scoring the strength of association, IQWiG scoring requires a correlation coefficient (r) for the treatment effect association, while BSES2 scoring requires R2 values for both the individual-level and the treatment effect associations. IQWiG and BSES2 scores were calculated for all subgroup analyses with sufficient data; therefore, studies reporting more subgroups were more strongly represented in this analysis.
For the IQWiG scores (Figure 6), of 202 analyses (across 63 studies), zero (0%) scored high, 15 (7%) scored medium +, 26 (13%) scored medium, 76 (38%) scored low and 85 (42%) were not evaluable.
FIGURE 6.
Summary of the IQWiG scores across all 202 analyses included in the review. Reproduced with permission from Cooper et al. 64 This is an Open Access article distributed in accordance with the terms of the Creative Commons Attribution (CC BY 4.0) license, which permits others to distribute, remix, adapt and build upon this work, for commercial use, provided the original work is properly cited. See: http://creativecommons.org/licenses/by/4.0/. The figure includes minor additions and formatting changes to the original figure.
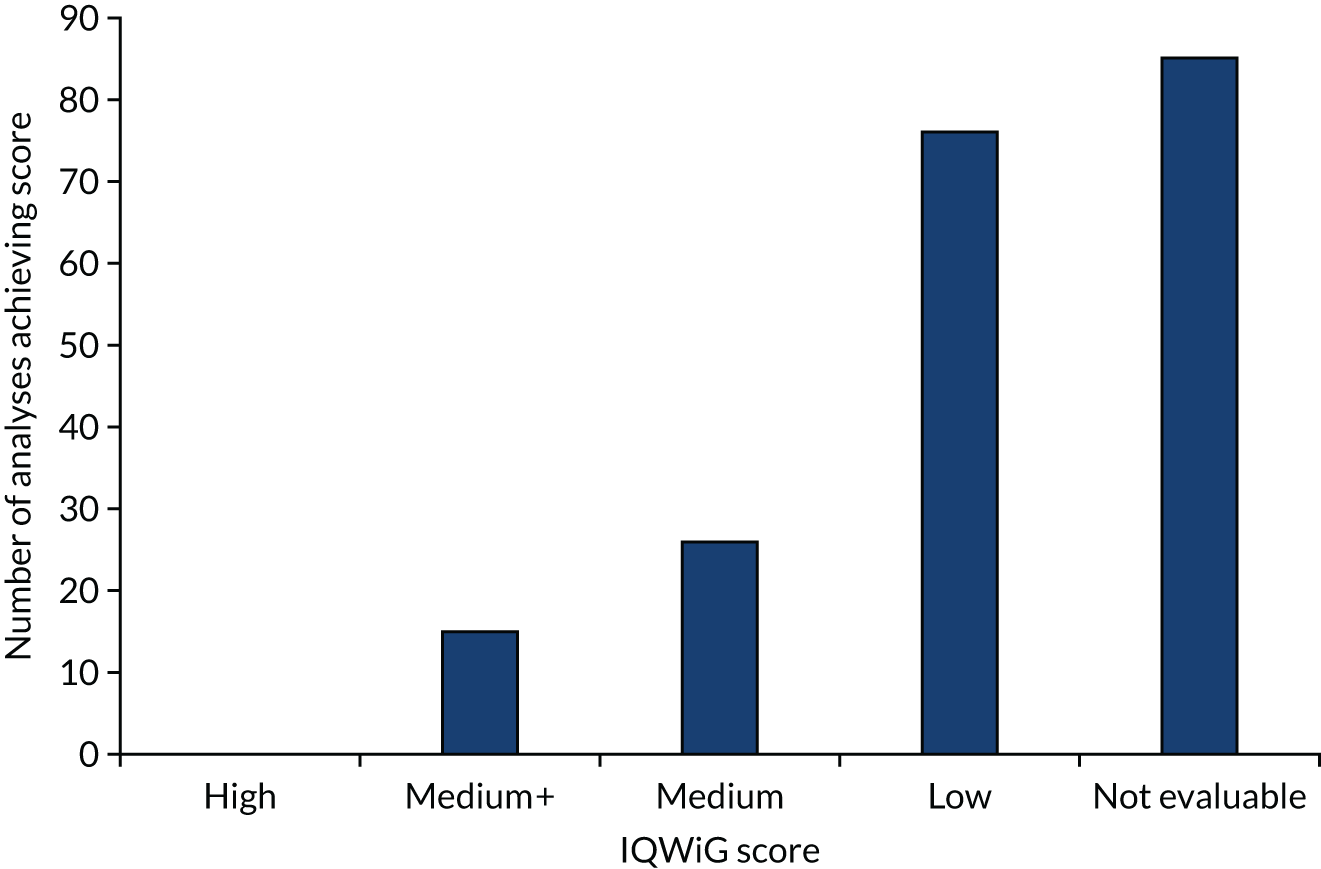
For the BSES2 scores (Figure 7), of 202 analyses (across 63 studies), zero (0%) scored excellent, three (1%) scored good, three (1%) scored fair, seven (3%) scored poor and 189 (94%) were not evaluable.
FIGURE 7.
Summary of the BSES2 scores across all 202 analyses included in the review.
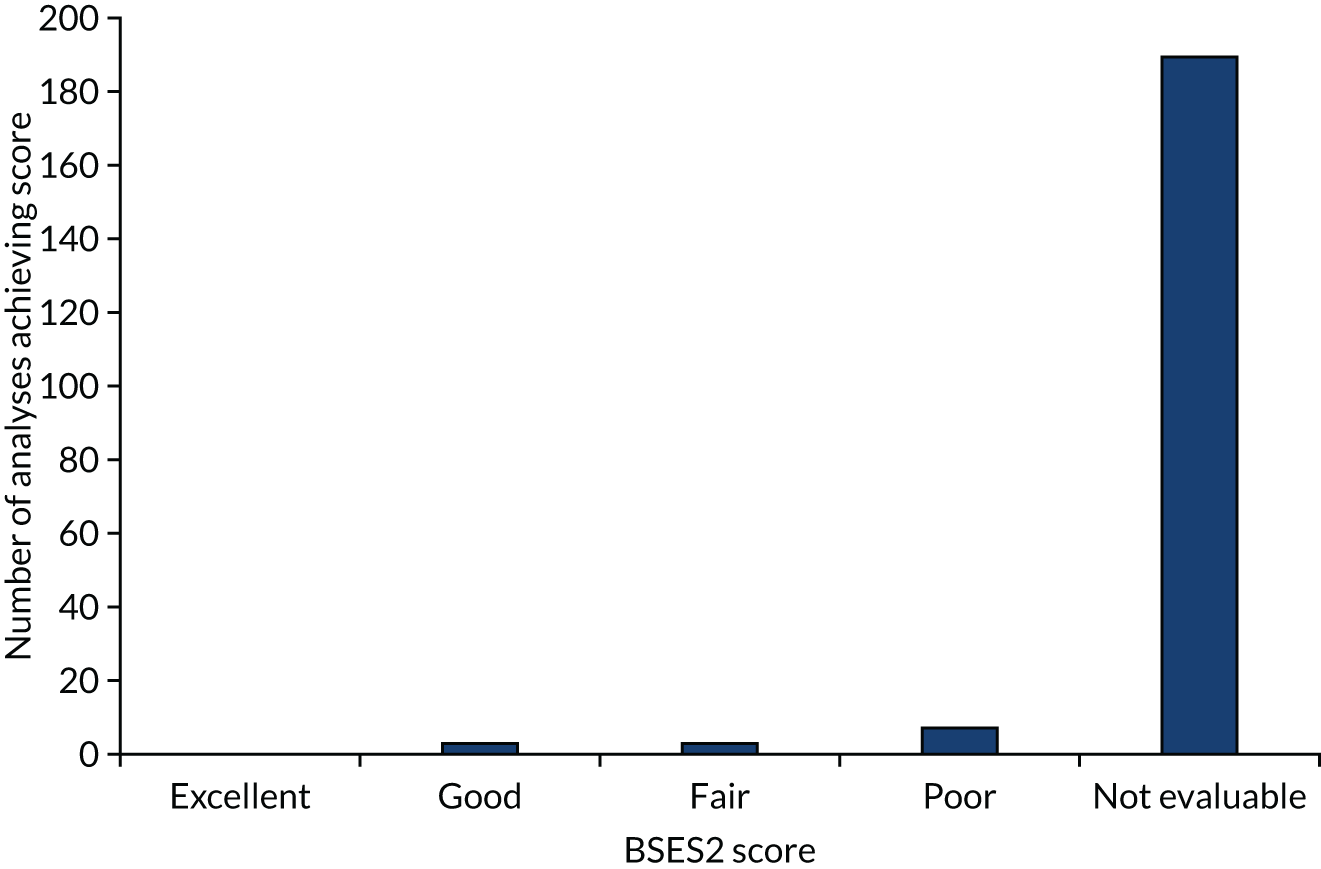
Discussion
Summary of the main findings
Types of analysis identified
This systematic review summarises correlation and regression analyses for the strength of the association between response outcomes and PFS, TTP or OS across different types of cancer (primarily advanced or metastatic), based on included meta-analyses and meta-regression studies. In total, the review included 63 studies across 20 cancer types, most commonly NSCLC, CRC and breast cancer and analyses of various solid tumours. The most commonly analysed relationships were between ORR and either PFS or OS, with other response outcomes (such as CR, DoR and PR) reported in fewer analyses. The majority of studies (n = 44) included only RCTs, while the remainder also included single-arm studies.
Absolute (individual-level) associations
For the absolute (individual-level) association, the reported correlation coefficients between ORR and PFS ranged from –0.72 to 0.96, based on multiple analyses within 12 studies across 10 cancer types, while correlations between ORR and OS ranged from –0.40 to 1.00, based on 27 studies across 15 cancer types. Confidence intervals were generally fairly wide and were often not reported. The correlation coefficients that were reported from multiple analyses within the same study, and those reported across separate studies, did not suggest a clear pattern by cancer type. For analyses of CR, the correlation coefficients between CR and PFS in two studies ranged from 0.22 to 0.83, while those between CR and OS ranged from –0.04 to 0.62, based on three studies.
Treatment effect (trial-level) associations
For the treatment effect (trial-level) association, the regression R2 between ORR and PFS ranged from 0.18 to 0.94, based on nine studies across four cancer types, while the R2 values between ORR and OS ranged from –0.08 to 0.84, based on 30 studies across 11 cancer types. Again, there was no clear pattern between cancer types. For analyses of CR, the highest R2 between CR and PFS ranged from 0.45 to 0.93 in one study, while that between CR and OS ranged from 0.05 to 0.48 within two studies.
Regression equations and surrogate threshold effect
Regression equations were reported in 14 studies for the relationship between ORR and OS, and in eight studies for the relationship between ORR and PFS. There was substantial variation in effect measures for both the surrogate and the final outcomes (e.g. difference in medians, HR and OR). The STE, the smallest treatment effect on the surrogate that predicts a non-zero treatment effect on the true end point,76 was reported in only four studies.
Strength of association between response and survival outcomes (Institute for Quality and Efficiency in Health Care and Biomarker-Surrogate Evaluation Schema-2 scoring)
The strength of association across all studies and all subgroup analyses was assessed using the IQWiG and BSES2 scoring systems. In general, scores were relatively low, which indicates poor association between response and survival outcomes overall. Of 202 analyses that used IQWiG scoring, 42% were not evaluable and 38% scored low, with 13% scoring medium, 7% medium + and 0% high. When using BSES2 scores, the majority of analyses (94%) were not evaluable because they did not report R2 for both individual-level and treatment effect associations, with 3% scoring poor, 1% fair, 1% good and 0% excellent.
Strengths and limitations
In this review, a comprehensive search was undertaken to identify relevant studies. The reported data were highly heterogeneous in terms of the effect measure and method of analysis. Therefore, some simplifying assumptions had to be made to allow the data to be summarised. Correlation coefficients were summarised regardless of method (Pearson’s, Spearman’s or other). R2 values were summarised irrespective of whether or not the regression was weighted and whether or not the R2 was adjusted. For treatment effect associations, R2 values were summarised regardless of effect measure (e.g. HR, OR and difference in medians).
Summary of findings
Based on this review, the association between response outcomes and PFS/TTP/OS varies widely between studies and generally scores low to medium on IQWiG and BSES2 scoring systems; however, a large number of analyses were not evaluable. There is no clear pattern for the strength of association by cancer type. Previous reviews assessing multiple surrogate end points have also concluded that response-based end points were poor surrogates for OS. 79,80
Implications for the economic analysis of histology-independent therapies based on overall response rate as a surrogate for progression-free survival or overall survival
The review presented in this chapter provides information that could be used to inform judgements about whether or not response-based outcomes might be considered as a valid surrogate for PFS and OS. If the surrogate end point is considered valid, or potentially even if it is not, one may consider using that surrogate as the basis for estimating health gains within a health economic model. There are four main options relating to the use of response-based outcomes as a surrogate for OS or PFS within the economic analysis of histology-independent therapies.
1. Use meta-analyses to predict the relationship between the surrogate and the final outcome
As shown in Tables 8 and 9, 14 studies report regression equations for ORR to OS and eight studies report equations for ORR to PFS. These equations could be used together with the observed ORR in the studies of histology-independent therapies to estimate the absolute PFS/OS or the incremental gains in PFS/OS. However, the patient populations included in these studies may not correspond to the populations in the studies of histology-independent therapies in terms of tumour sites or types, and none specifically relate to patients with NTRK fusion-positive cancers (or other relevant biomarkers). From a practical point of view, a number of decisions would be required to apply these analyses within a model: (1) which regression equation to use in instances whereby multiple analyses exist for an individual histology site; (2) the form of regression analysis used to estimate the relationship (i.e. ‘absolute’ regressions that estimate final outcomes for an individual treatment group or ‘trial-level’ equations that predict the treatment effects between groups); and (3) how to model the surrogate relationship where no studies exist for an individual histology site. In addition, concerns regarding the strength of the relationship between ORR and PFS/OS within the tumour sites under consideration should be borne in mind.
It has been suggested that the stringent application of criteria for surrogate validation based on correlations may not be important and that predictions may still be made even where the association is weak, provided that they reflect all uncertainty surrounding the treatment effects. 146 In addition, NICE technical support document (TSD) 20146 notes that the meta-regression approaches included in this review are limited because they ignore the uncertainty associated with the treatment effect on the surrogate end point (which is treated as a fixed covariate in the analysis), the consequence being that predictions based on these regression analyses will fail to fully reflect that uncertainty. Recently developed methods, such as the bivariate random-effects meta-analysis (BRMA) model and its extensions,146,147 provide an approach for both the validation and the prediction of surrogate end points within a Bayesian framework. In principle, this approach could be used to generate predictions of treatment effects on final outcomes in a way that allows for borrowing of information across studies and that fully accounts for all uncertainty surrounding the surrogate relationship. In instances whereby the surrogate association is weak, this would manifest as a wider interval around the prediction and increased uncertainty surrounding modelled outcomes and costs. This approach is intuitively appealing; it would, however, render the published meta-regressions redundant because it would require re-analyses of the input data and the implementation of new meta-analyses for each histology site.
2. Land-marking analysis
This review included only meta-analytic studies and, by design, excluded individual studies that did not include multiple cohorts of patients. Some of the studies that were excluded from the review during the sifting stage adopted a land-marking approach (see Chapter 7, Discussion, for more details of this approach) within individual patient cohorts to explore the impact of response-based outcomes on OS, with differences between responders and non-responders reported in terms of a HR. Given an underlying baseline model of OS for non-responders, it may be possible to estimate the incremental impact on OS by combining the ORRs observed in the histology-independent studies with the HR derived from the land-marking analyses. However, the published land-marking studies generally related to a single tumour type and the study populations do not specifically relate to patients with NTRK fusion-positive tumours (or other relevant biomarkers).
3. Risk prediction models
During sifting, the review authors identified a small number of risk prediction studies. These studies reported multivariable statistical models to estimate the final outcome (OS/PFS) as a function of some response-based variable (e.g. ORR) together with other clinical parameters (e.g. age, sex and clinical characteristics). These studies may also provide a source of HRs for the impact of response on OS/PFS, but, again, these typically relate to a single tumour type and do not specifically relate to patients with NTRK fusion-positive tumours (or other relevant biomarkers).
4. Do not use response as a surrogate for progression-free survival/overall survival
The systematic review suggests that, taken generally, ORR may not be a reliable surrogate for PFS or OS based on current frameworks for surrogate validation. The review did not indicate any particular pattern whereby ORR performs better or worse according to tumour type or site. Even where a means of predicting PFS/OS on the basis of ORR for a given tumour site exists (e.g. using conventional meta-regressions or BRMA), in the absence of a strong relationship between the surrogate and the final end points the resulting estimates may be highly uncertain and difficult to interpret. It should be noted, however, that the alternative may involve extrapolating highly immature PFS and OS data, which are also subject to substantial uncertainty; hence, this may not represent a sufficiently robust solution either.
Conclusions
This systematic review suggests that response end points, such as ORR and CR, may not be reliable surrogates for PFS or OS. The strength of association varied widely between studies and subgroups and, in general, there was no clear pattern by cancer type.
Despite the potentially weak validity of response as a surrogate for PFS and OS, it may still be considered preferable to adopt a surrogate-based modelling approach informed by predictions from meta-analyses that capture all relevant uncertainty than to ignore potential surrogate relationships and extrapolate heavily censored PFS and OS data. The recently developed BRMA approach outlined in the Decision Support Unit (DSU) TSD 20146 may serve an important role in ensuring that all uncertainty around the surrogate relationship is reflected in the predictions used in the model. Ultimately, the most appropriate modelling approach will depend on the characteristics of the evidence available from the histology-independent study.
Chapter 5 A targeted review of published National Institute for Health and Care Excellence technology appraisals for which initial marketing authorisation was based on response outcomes from single-arm studies
We undertook a targeted review of 10 published NICE TAs for which marketing authorisation was based on response rates from single-arm studies. The aim of the review was to highlight alternative analytic and structural approaches that have been proposed in previous appraisals to inform the extrapolation of surrogate end points based on ORR and DoR, and/or to handle uncertainties owing to immaturity in PFS and OS data. The case studies also served to identify a broader range of issues that are likely to be relevant for the appraisal of histology-independent products.
A thematic-based review is used to summarise key issues and uncertainties raised by the Evidence Review Groups (ERGs) and NICE committees. The review is presented in Appendix 8.
Summary and implications
The challenges of using a partitioned survival approach and relying on independent extrapolations of PFS and OS based on immature data are particularly evident in those appraisals for which median OS was not reached. In these specific appraisals, a range of alternative approaches were used, including conventional parametric extrapolation approaches, the use of expert judgement and the use of evidence from a proxy population with more mature evidence. In each of these appraisals, the committee highlighted significant concerns regarding the uncertainty and robustness of the incremental cost-effectiveness ratio (ICER) estimates, leading to recommendations within the CDF rather than routine NHS commissioning.
One important finding was that none of the 10 TAs explored the use of surrogate relationships to help to inform the PFS and OS extrapolations. This could be considered surprising, given that the primary end point in the underpinning studies is a surrogate end point for clinical benefit and given the concerns noted by EMA and FDA regarding the challenges of interpretation and potential bias in assessing TTE end points based on single arm studies using ORR as the primary end point. However, it might also reflect the concerns regarding the reliability of ORR and CR as surrogates for PFS or OS (see Chapter 4).
Owing to the nature of basket trials, significant heterogeneity may be present in the study populations enrolled in the trials (see Chapter 3). The potential importance of accounting for heterogeneity and exploring the cost-effectiveness in subgroups of the target population is acknowledged in the current NICE methods guide. 4 Differences in the cost-effectiveness and decision uncertainty across these separate subgroups may lead to an optimised recommendation that is more restrictive than the marketing authorisation.
The review also demonstrated that the heterogeneity within an overall target population is often a critical aspect of the appraisal. The committee acknowledged the importance of accounting for heterogeneity in a variety of sources in addition to relative effectiveness, including prognosis, health-related quality of life (HRQoL) and the cost of comparator therapies, which were likely to differ, impacting the cost-effectiveness estimates. The majority of TAs included only a small number of subgroups, most commonly based on alternative positions of a new treatment in an existing pathway. It is notable that in most of these appraisals, either separate studies were available for different subgroups or it was more feasible to undertake subgroup analyses than in histology-independent appraisals, given the larger sample sizes. Although examples were identified that appeared more relevant to histology-independent appraisals, these were also limited to relatively small numbers of subgroups informed by separate studies or with sufficient numbers to present stratified results. However, it was evident from the appraisals of interventions with a broad marketing authorisation that the committee preferred to be explicit about the different sources of heterogeneity, leading to specific recommendations for subgroups within the broader population.
Committees have routinely considered the diagnostic accuracy of the available testing and the appropriateness of the proposed testing strategies. The feasibility of introducing new testing pathways was also the subject of committee discussions. The predictive validity of the target genetic mutations was well established, with company submissions providing an overview of the clinical basis for the predictive validity of the target mutation. The prognostic validity of target mutations was, in contrast, poorly understood in all three appraisals reviewed, which meant that only limited conclusions could be drawn regarding the prognosis of patients when receiving standard care.
Although the review of TAs identified several important themes that are likely to be relevant to histology-independent appraisals, there are also important differences owing to the nature of the study designs and the greater levels of heterogeneity within the target population. Chapter 6 provides a more detailed consideration of some of the potential challenges that are envisaged and considers a range of alternative analytic approaches that might be required.
Chapter 6 Issues and challenges for exploring heterogeneity for histology-independent appraisals
Parts of this chapter have been reproduced from Murphy et al. 148 This is an Open Access article distributed in accordance with the terms of the Creative Commons Attribution (CC BY-NC 4.0) license, which permits others to distribute, remix, adapt and build upon this work, for non-commercial use, provided the original work is properly cited. See: https://creativecommons.org/licenses/by-nc/4.0/. The text below includes minor additions and formatting changes to the original text.
The clinical effectiveness and cost-effectiveness of a treatment will often depend on the characteristics of patients and the circumstances under which they receive treatment. The fact that different patient groups have different characteristics and, therefore, will derive different benefit from treatments is called ‘heterogeneity’. 149–151
Heterogeneity matters for two main reasons. First, if benefits differ by patient characteristics, estimates of the treatment benefit must match the patient population that is expected to receive the treatment (the target population) in routine clinical practice. Second, there can be health benefits from making tailored decisions for particular groups of patients. This gain from recognising differences between subgroups of patients and potentially ‘optimising’ recommendations within a product’s licence is called the value of heterogeneity (VoH). 150–152
The exploration of sources of heterogeneity and the use of subgroup analysis is recommended within the NICE reference case analysis. 4 Ignoring these differences could mean that a treatment that is not cost-effective for the total population (combining all subgroups) may be cost-effective in specific subgroups. Making a ‘one size fits all’ recommendation would then result in a potentially cost-effective treatment being withheld from a subset of patients for whom the treatment would represent an appropriate use of NHS resources. Conversely, a treatment that appears cost-effective for the total population may not be cost-effective in particular subgroups. In this case a ‘one size fits all’ approach could result in the treatment being recommended in identifiable subgroups in which the value of providing the new treatment is lower than the opportunity cost. That is, the health gain for these specific subgroups is not sufficient to offset the potential health lost from a reduction in the provision of services elsewhere in the NHS that is necessary to fund the new treatment.
In the case of histology-independent treatments, heterogeneity is particularly important to consider. This is because an important source of heterogeneity is differences in tumour histology. Although a treatment may be clinically effective across a range of tumour sites, there are theoretical and empirical reasons to expect that cost and health consequences could vary significantly across tumour sites. This is in addition to the usual sources of heterogeneity (e.g. age and sex), which are present in conventional treatments.
There are a number of sources of heterogeneity that are relevant to histology-independent decision-making. The main focus of this chapter is heterogeneity between subgroups, as defined by histology. However, it must be stressed that heterogeneity owing to other characteristics is also relevant.
The following sections identify a number of particular challenges for histology-independent appraisals and present alternative approaches that might be used to investigate and account for different sources of heterogeneity. A formal framework is presented in Chapter 7.
Treatment effectiveness
The available evidence is likely to consist of response and immature PFS and OS data for patients with different tumours included in one or more single-arm studies with a basket design. Methods to test for heterogeneity in response by tumour type or other relevant characteristics (typically related to the target mutation) can be used during trial conduct and can inform stopping rules in an adaptive trial design framework (see Chapter 3). If heterogeneity is explored within the trial and it is concluded that a pooled analysis is justified (i.e. the treatment effect is sufficiently homogeneous across the tumour types), these results can be re-evaluated during the appraisal process and a decision can be made on whether or not it is appropriate to accept the company’s proposal of a homogeneous treatment effect across the tumours. A decision made within the trial to discontinue recruitment to one or more baskets owing to an unsuitable response should caution against a completely histology-independent recommendation. In such cases, there will need to be a case made for which tumour types can be considered to have sufficient evidence of effect for a recommendation and which should be excluded, given the trial evidence of insufficient response.
Regardless of how the trial was originally designed and analysed, if outcomes are available for each tumour type, some of the frameworks described in Chapter 3 can be useful to explore the potential for heterogeneity in effects across tumours. The adaptive phase can be ignored and the methods can be used to estimate mean outcomes for each histology, with appropriate uncertainty, as well as pooled posterior and predictive mean outcomes that account for the potential lack of uniformity of effect across tumours. The BHM46 is simple to implement and is particularly suited to this framework given that it starts from the assumption that treatment effects are exchangeable (rather than identical) across tumours, a more reasonable assumption in the absence of evidence to the contrary, and produces estimates of the level of heterogeneity across tumours and of the pooled treatment effects for each tumour, which can be used to judge whether or not the assumption of homogeneity is reasonable. In addition, this model allows the prediction of the effect in unrepresented tumour types as long as they can also be assumed to have exchangeable effects (i.e. drawn from the same distribution of effects) as the included tumour types.
The BHM works by assuming that for each tumour type j, the measures of effect θj are exchangeable and follow a normal distribution:
where σ is the standard deviation quantifying the between-tumour heterogeneity and µ is the pooled mean effect across all tumour types. Prior distributions must be selected for µ and σ, and are likely to have some influence on the posterior estimates,46,52 particularly when a small number of tumour types and patients per tumour are included. A Uniform(0,5) prior distribution was found to be robust in a simulation study. 52 The sensitivity of results to the prior distributions should be assessed. When the outcome is binary, for example response, θj represents the log-odds of response in tumour site, j, and the probability of response in each site, pj, is recovered as:
The probabilities that the response rates for each tumour type are at least of a certain magnitude can also be calculated, and heterogeneity in these probabilities can guide conclusions on the plausibility of a homogeneous response. Typically, a value of 30% is used to define a meaningful response, but any other value can be used, depending on context.
In the case in which the tumour types included in the trial are not reflective of the entire licensed indication, the predictive distribution of effect (e.g. the probability response) in a new histology, θNEW, can be obtained as:
This will reflect the full degree of uncertainty owing to both the sample size and the observed heterogeneity in effects across the observed tumour sites. The resulting distribution represents the predictive probability of response in a ‘new’, that is, unrepresented tumour type.
Although in theory the BHM can be applied to dichotomous (e.g. tumour response) or TTE outcomes (e.g. PFS and OS),46 the assumption of exchangeability of the effects of treatment on survival outcomes across tumour outcomes is harder to justify than the equivalent assumption made for the effects of treatment on response. As noted in Chapter 3, a critical consideration in designing basket trials is the heterogeneity in survival prognosis across the different histologies. This is the motivation for evaluating measures, such as standardised response rates, which reflect tumour shrinkage, rather than survival outcomes. 29,30 In addition, the nature of the survival data available, which tends to be immature and based on only a few patients per tumour type, will make estimation of a hierarchical model challenging, unless informative prior distributions are used on key parameters.
The model proposed by Leon-Novelo et al. 53 can be used in the scenario for which it is not expected that all subgroups will be a priori exchangeable (see Chapter 3) and there is a particular tumour characteristic (e.g. prognosis or type of NTRK fusion) that defines exchangeability, so that different categories can be predefined (e.g. poor, intermediate and good prognosis). If these a priori exchangeable categories can be predefined, the approach is similar to the BHM and a prediction for unrepresented tumour types in each category can be made. However, this would no longer generate a truly histology-independent recommendation because results might differ across tumours in different categories.
Hybrid exchangeable/non-exchangeable models,51,53 in which exchangeability is determined by the data, are less relevant as exploratory models for HTA because their results would be harder to interpret. This is because exchangeability cannot be assumed to apply across all tumour types and predictive distributions of effects can no longer be assumed to represent the expected effect in unrepresented tumour types, given that these are not necessarily fully exchangeable with the included tumour types. In addition, in an exchangeable/non-exchangeable scenario, a histology-independent recommendation would be hard to justify because the assumption would be that effects differ across tumour types, which could affect clinical effectiveness and cost-effectiveness estimates.
Exploring heterogeneity in response: case study
To demonstrate the impact of allowing for heterogeneity in response and to explore the potential heterogeneity in effects across tumours, response data were analysed using a BHM framework. 46
For the purpose of this analysis, the response data used were the published efficacy evidence available for the tyrosine kinase (TRK) inhibitor, larotrectinib. 3,153 The results, presented as a post hoc pooling of 55 patients covering 12 tumour types from three non-randomised single-arm Phase I/II basket studies, including the number of patients and responses by tumour type, are shown in Table 11.
Tumour ID | Tumour type | Patients (n) | ORR | |
---|---|---|---|---|
Responders (n) | Observed response (%) | |||
1 | Soft tissue sarcoma | 11 | 10 | 91 |
2 | Salivary gland | 12 | 10 | 83 |
3 | IFS | 7 | 7 | 100 |
4 | Thyroid | 5 | 5 | 100 |
5 | Lung | 4 | 3 | 75 |
6 | Melanoma | 4 | 2 | 50 |
7 | Colon | 4 | 1 | 25 |
8 | GIST | 3 | 3 | 100 |
9 | Cholangiocarcinoma | 2 | 0 | 0 |
10 | Appendix | 1 | 0 | 0 |
11 | Breast | 1 | 0 | 0 |
12 | Pancreas | 1 | 0 | 0 |
Total | 55 | 41 | 74.5 |
We can consider each of the tumour types as a ‘basket’ or group and analyse the response data using a BHM framework to explore the potential heterogeneity in effects across tumours.
Methods
For the response outcome, data available for each of the tumour types in the published literature are the number of responders, xj, out of the total number of patients, nj for tumour type, j, which are assumed to follow a binomial likelihood:
where pj is the probability of response for tumour type, j, with j = 1, . . . ,G, and G is the total number of tumour types. The log-odds of response in tumour type, j, θj, was modelled on the log-odds scale: logit(θj) = pj. The BHM assumes that for each of the G tumour types, the log-odds of response, θj, are exchangeable and follow a Normal distribution (see Equation 1).
We used a relatively conservative normal prior distribution for µ, centred around a probability of response of 0.3 (a log-odds of –0.8473), which is often considered as a promising response rate, with a variance of 10 across all tumour types. The sensitivity of the results to a more favourable prior distribution, for which the prior probability of response across all tumour types is centred around a mean of 0.5 (a log-odds of 0) with the same variance, was assessed.
The prior for the between-tumour heterogeneity standard deviation is specified as Uniform(0,5), which was found to be robust in a simulation study. 46,52 An inverse-gamma (2, 20) prior distribution for the between-tumour variance had previously been proposed,46 meaning that the between-tumour precision has prior mean of 0.10 and variance of 0.005. Inverse-gamma prior distributions were found to lead to posterior distributions, which are highly sensitive to the chosen parameters and are, therefore, not recommended in most cases. 52 The sensitivity of the results to the inverse-gamma prior distribution, to the between-tumour heterogeneity variance and to using different half-normal prior distributions for the between-study standard deviation was assessed. 52 Half-normal prior distributions with precision from 0.01 to 0.1 and 1 were also assessed.
Given that the tumour types included in the analysis population are not reflective of the full licensed indication (i.e. a truly histology-independent marketing authorisation will encompass all tumour types, not just those represented in the trial), the predictive distribution for the response rate in a new tumour type is calculated to reflect the full degree of uncertainty owing to both the sample size and the observed heterogeneity in effects across the observed tumours. The resulting distribution is the probability of response in a ‘new’, that is, unrepresented, tumour type.
The model was adapted from Thall et al. 46 and was estimated using Markov chain Monte Carlo in OpenBUGS (OpenBUGS Foundation, MRC Biostatistics Unit, Cambridge, UK),154 implemented in R (version 3.6.0) (The R Foundation for Statistical Computing, Vienna, Austria) using R2OpenBUGS155 (version 3.2.3.2). The BUGS code used is presented in Appendix 9.
The model fit was assessed by plotting individual tumour contributions to the residual deviance (in a well-fitting model these are expected to be close to 1) and by comparing the total residual deviance with the number of tumour types, G. Convergence was assessed by visual inspection of the Brooks–Gelman–Rubin plots and assessment of the R^ statistic. 156,157
Results
For all analyses, 55,000 iterations were run on two parallel chains and the first 5000 iterations were discarded as ‘burn-in’. Model fit statistics are presented in Appendix 10.
The prior distributions used for the base-case analysis are:
The BHM estimates substantial between-group heterogeneity (posterior median of 2.86 on the log-odds scale), although there is considerable uncertainty [95% credible interval (CrI) of 0.92 to 4.83] (Figure 8). This suggests that there is considerable variability across tumour types.
FIGURE 8.
Prior and posterior distributions for the between-group heterogeneity standard deviations.
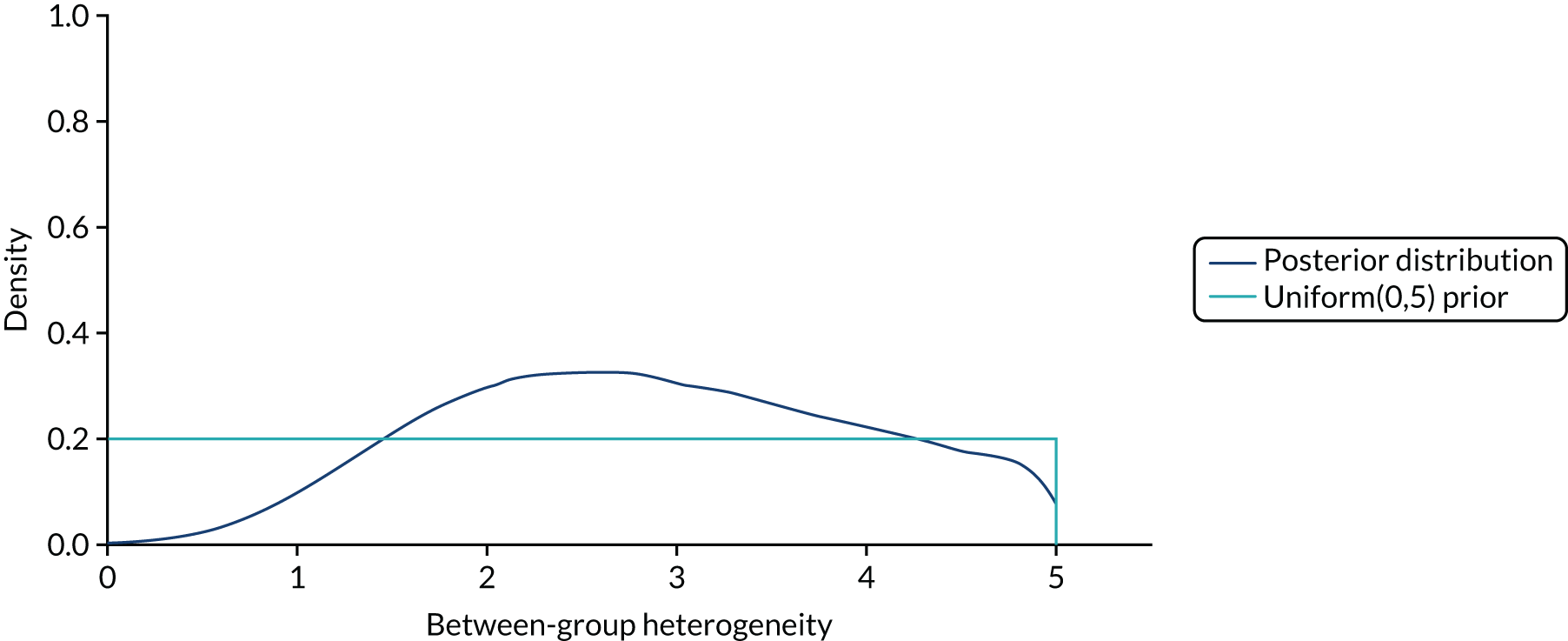
The estimated mean response rate across all tumour types is 0.609 (95% CrI 0.160 to 0.918). This is lower than the mean response rate of 0.745 observed in the efficacy evaluable data set. The response probability predicted for an unrepresented tumour type is 0.569; however, the 95% CrI is wide, meaning that this probability could be as low as 0.2% or as high as 99.9% (Table 12 and Figure 9).
Probability | Mean | Median | 95% CrI |
---|---|---|---|
Posterior | 0.609 | 0.641 | 0.160 to 0.918 |
Predictive | 0.569 | 0.649 | 0.002 to 0.999 |
FIGURE 9.
Posterior and predictive distributions of response probability.
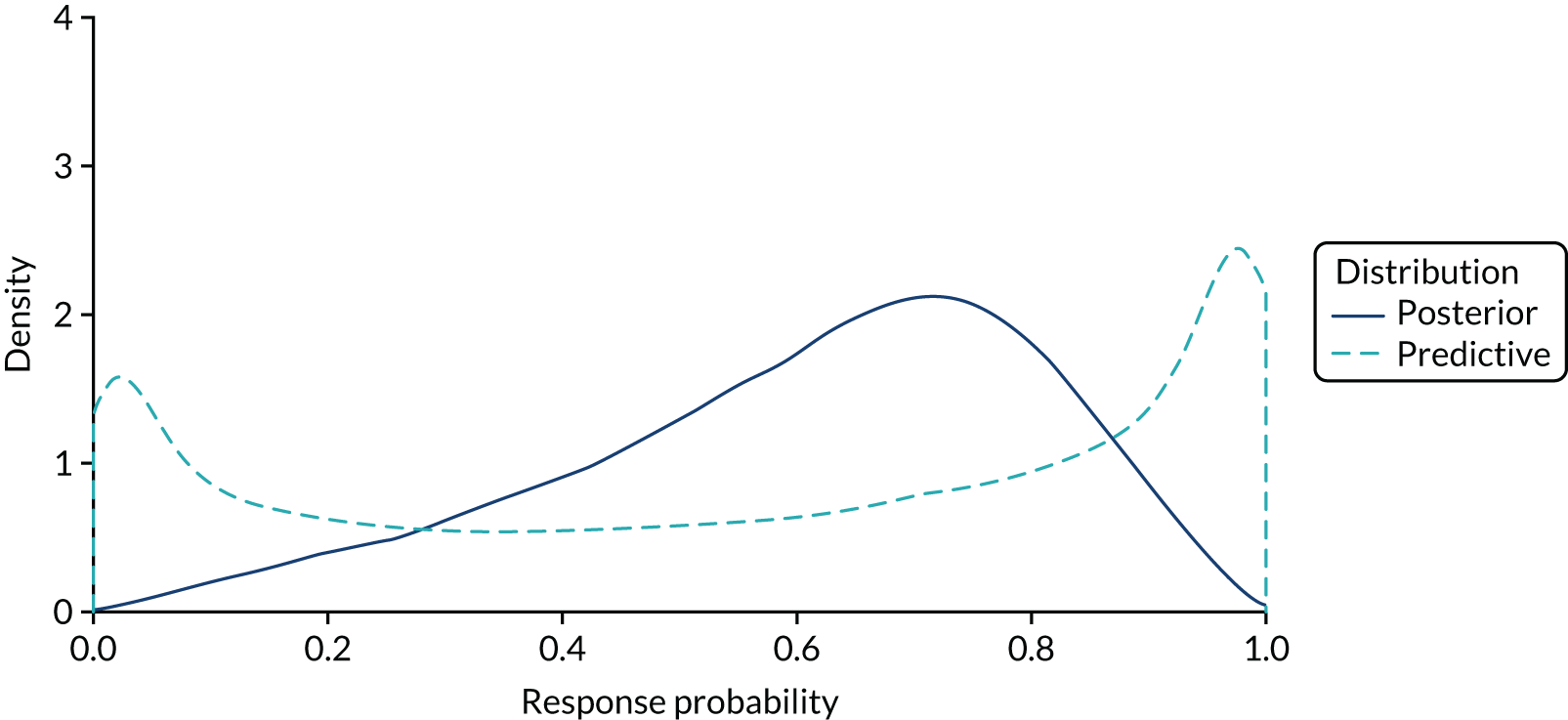
The estimated probabilities of response for each tumour type are shown in Table 13. The effect of allowing information to be borrowed across the tumour types is to shrink the observed response probabilities towards the pooled mean response probability. Tumour types with a smaller number of patients borrow more information than tumour types with a larger number of patients and, therefore, have values closer to the pooled mean.
Tumour type | Observed response (%) | Estimated mean response based on BHM (%) | 95% CrI (%) | Probability of response rate of ≥ 30% | Probability of response rate of ≥ 10% |
---|---|---|---|---|---|
Sarcoma | 91 | 88 | 66 to 99 | 1.000 | 1.000 |
Salivary | 83 | 82 | 58 to 97 | 1.000 | 1.000 |
IFS | 100 | 93 | 70 to 100 | 1.000 | 1.000 |
Thyroid | 100 | 92 | 63 to 100 | 1.000 | 1.000 |
Lung | 75 | 73 | 30 to 98 | 0.976 | 0.999 |
Melanoma | 50 | 52 | 12 to 89 | 0.835 | 0.984 |
Colon | 25 | 32 | 3 to 75 | 0.484 | 0.854 |
GIST | 100 | 88 | 49 to 100 | 0.996 | 1.000 |
Cholangiocarcinoma | 0 | 21 | 0 to 76 | 0.281 | 0.555 |
Appendix | 0 | 30 | 0 to 90 | 0.416 | 0.650 |
Breast | 0 | 30 | 0 to 90 | 0.415 | 0.653 |
Pancreas | 0 | 30 | 0 to 90 | 0.413 | 0.648 |
Figure 10 shows the posterior distributions of the probabilities of response for each of the 12 tumour types included in the efficacy evaluable data set. Although the observed response suggested that cholangiocarcinoma, appendix, breast and pancreas tumours did not respond to larotrectinib, the posterior distributions of these tumour types are wide and their 95% CrIs suggest that response rates of 76% are plausible.
FIGURE 10.
Posterior distribution for the probabilities of response by tumours type. (a) Sarcoma, salivary, IFS and thyroid; (b) lung, melanoma, colon and GIST; (c) cholangiocarcinoma, appendix, breast and pancreas.
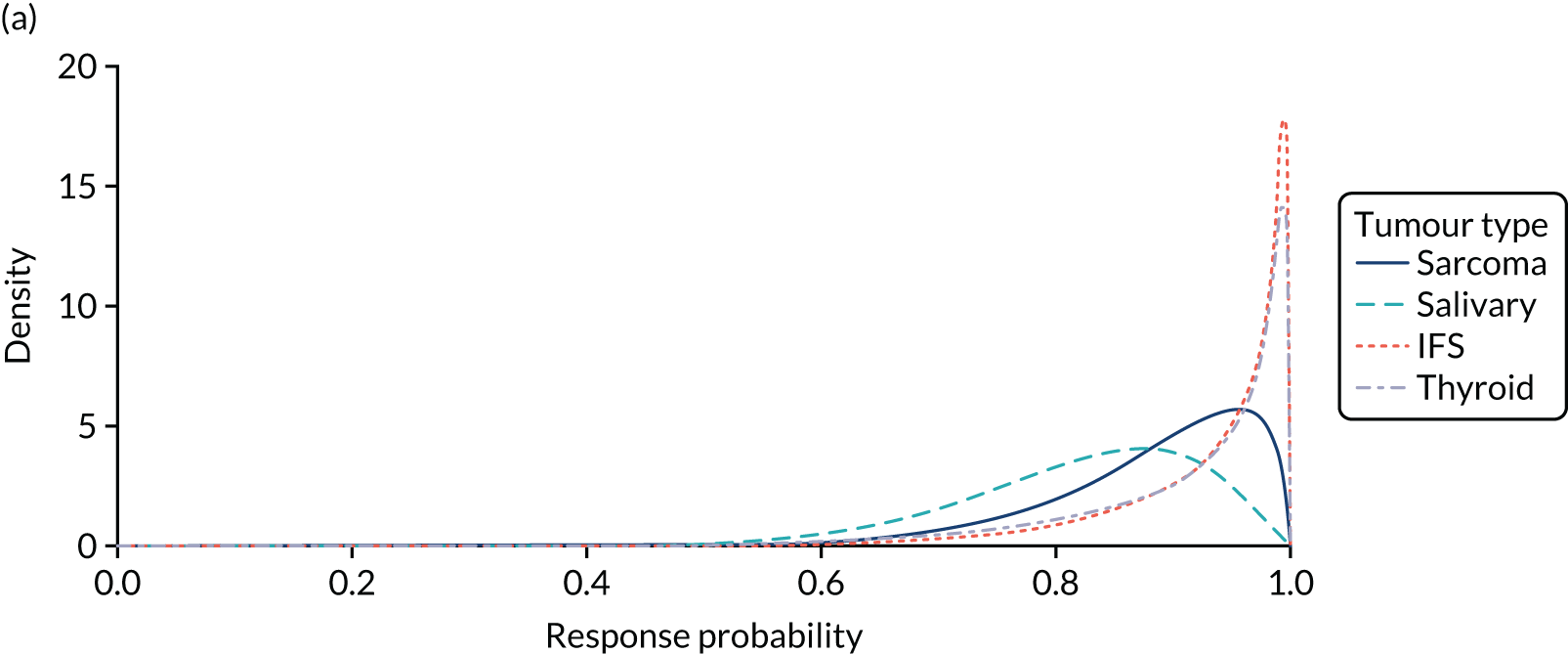
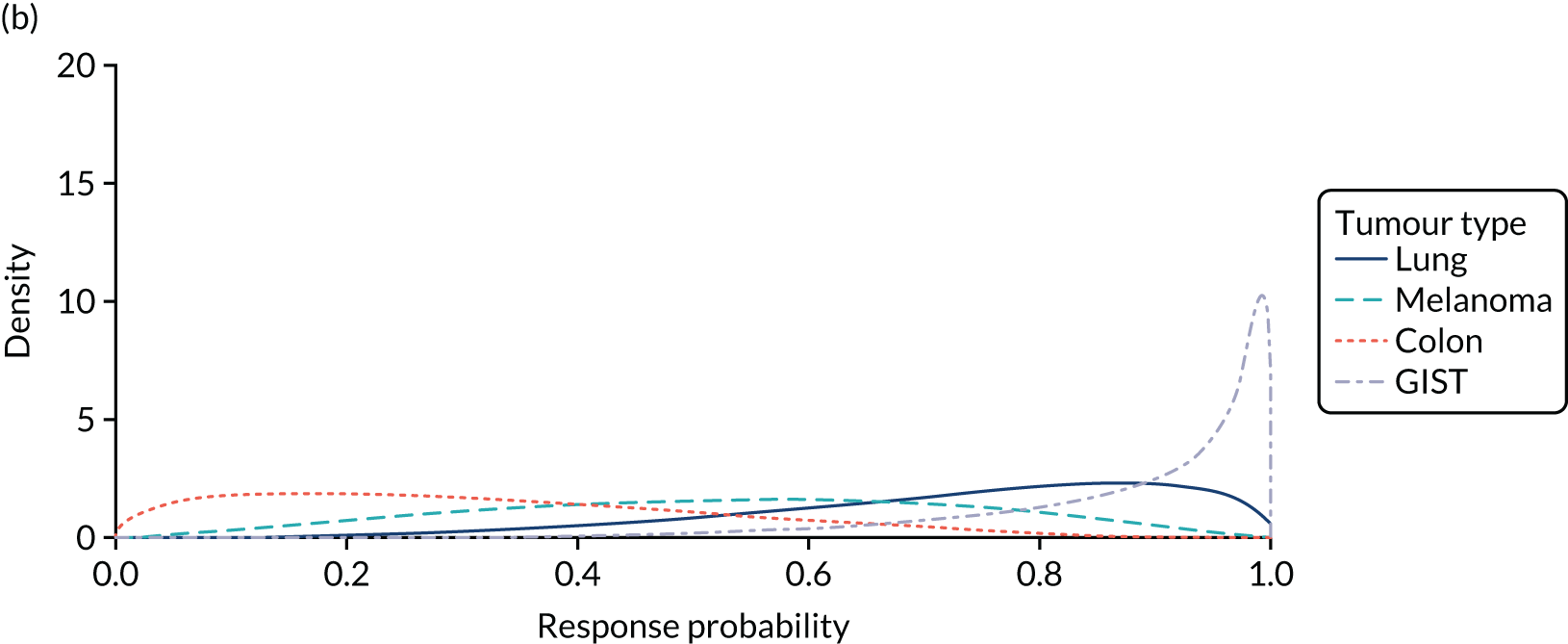
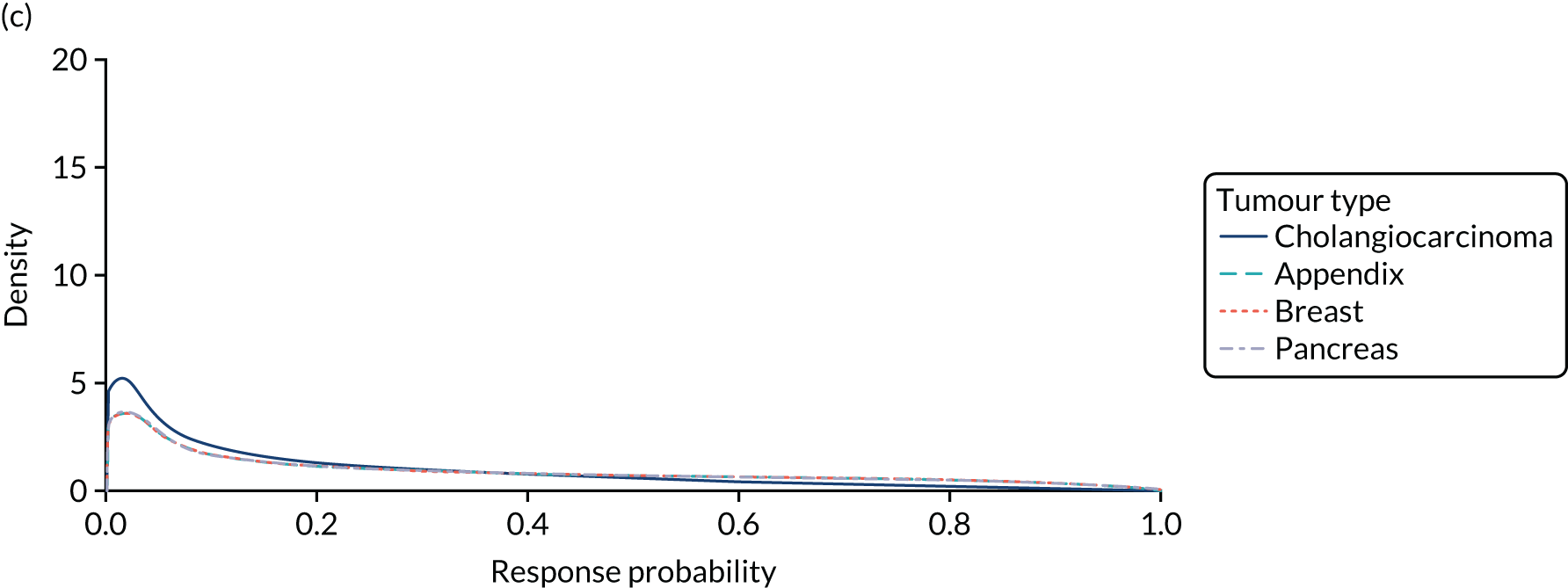
The results were insensitive to the use of the inverse-gamma prior, the half-normal prior and the uniform prior centred on a log-odds of response of 0.5. The results were also insensitive to the use of a more favourable precision of the between-tumour heterogeneity standard deviation of 0.1 and 0.5. For full results of the sensitivity analysis, see Appendix 11.
Implications for the appraisal of histology-independent technologies
Heterogeneity in the treatment effects is likely to be an important issue in the appraisal of histology-independent technologies. As can be seen from the results of the worked example (see Exploring heterogeneity in response: case study), the BHM suggests that there is substantial heterogeneity in response across tumour types. This can be seen in the estimate of the between-group heterogeneity, with the BHM estimating a posterior median standard deviation for the heterogeneity of 2.86 on the log-odds scale, which is considered large. Heterogeneity can also be seen in the predictive distribution of response, appearing in Figure 9 as a bimodal distribution with density concentrated around a probability of response of 0 and 1. This can be explained by the individual tumour response rates shown in Figure 10, which suggest that, even under the assumption of exchangeability of response, there are tumour types in which the data suggest that response is likely and tumour types in which it is not likely.
The results of this analysis challenge the strong assumption of homogeneity in response across such a variety of tumour types when treated with larotrectinib. The assumption of homogeneity may mask important information about empirical evidence of tumour response and the BHM provides a vehicle through which to account for the potential heterogeneity.
Counterfactual
The feasibility of conducting RCTs in histology-independent populations is likely to be challenging and it is expected that histology-independent technologies will often seek (and receive) EMA/FDA approval with limited or no data from randomised experiments (see Food and Drug Administration review of larotrectinib and Food and Drug Administration review of entrectinib. The lack of control data means that the evaluation of the cost-effectiveness of histology-independent technologies will require the generation of a comparator arm, for example by generating a control based on a historical control.
The interpretation of relative effect estimates from single-arm studies compared with historical controls is potentially subject to bias owing to differences between patients selected as historical controls and patients recruited to the single-arm studies. Differences between the patient populations can arise for a variety of reasons, including differences among accrual sites or differences in patient characteristics (e.g. age, performance status or other prognostic factors). For example, more recently diagnosed patients may have milder manifestations of a condition owing to improved (and, therefore, commonly increased) diagnostic sensitivity. Treatment effect differences may also be attributable to secular trends in clinical care (e.g. changes in diagnostic methods, classification criteria or outcome ascertainment) or other unknown confounders.
The challenges of generating an appropriate historical control are present in many appraisals wherever such comparisons are made. These challenges may be particularly acute when considering a histology-independent technology. In the context of a histology-independent appraisal, the generation of an appropriate historical control data set is complicated by the need to cover multiple tumour types/histologies, which not only creates challenges for generating an appropriate comparator data set, but also potentially exacerbates the potential for confounding bias.
The need to cover multiple tumour types/histologies means that it is unlikely that any single data set will provide sufficient coverage to represent the whole target population. It is, therefore, likely that multiple data sources will need to be identified, as was implemented in the ongoing NICE appraisal of larotrectinib. 158 The identification of historical control data would ideally be undertaken through an appropriate systematic review; however, this creates practical challenges because the resource required to implement this across multiple tumour types/histologies is extensive. In the larotrectinib appraisal, the focus of company searches for historical control data was limited to previous NICE TAs covering the tumour types included in the company’s single-arm study. Although this can be considered a reasonable pragmatic step, there is the potential for alternative, and plausibly more relevant, sources of historical data to be missed.
Other challenges that result from the need to generate a data set that covers such a broad variety of tumour types include the possibility that no relevant data exist for some relevant tumour types. For example, NTRK fusions are present in a number of rare tumour types that have not been subject to NICE guidance, and in the larotrectinib appraisal158 the company submission was forced to make arbitrary assumptions regarding the outcomes of patients for whom relevant comparator data could not be identified.
The identification of appropriate historical data will also need to address uncertainties regarding the positioning of therapy and any discrepancy between the licensed indication and the trials. The line of therapy may be an important prognostic factor because patients in later lines of therapy will tend to have fewer treatment options and may have accrued chemotherapy-related toxicity, limiting their tolerability to further treatment. Attempting to match control patients’ characteristics to the observed line of therapy in the intervention arm, however, creates challenges in relation to ensuring internal and external validity – namely, whether lines of therapy in the historical comparator data set should match those of the intervention arm or whether the historical control should attempt to reflect the eligible population and, therefore, maintain external validity, in which case the relative effect estimates may be biased. Indeed, this tension between internal and external validity may extend to other patient characteristics, particularly where the pool of patients in the intervention arm for a particular tumour is small, as recruited patients may not be fully representative of the eligible population. This tension, therefore, may typify a general issue of whether or not to match patient characteristics in the control arm to those in the intervention arm.
A further issue with using historical controls is that the target mutation may be prognostic in some or all tumours and it may be difficult to obtain relevant historical data limited to patients who harbour the target mutation, particularly where this mutation is rare. There is also the possibility that the prognostic value of the mutation may differ across tumour sites, which further complicates any attempt to adjust for the prognostic value of a mutation. In addition, in the context of a new target mutation, the prognostic value in different tumour types may not have been investigated sufficiently and is likely to be unknown for most, if not all, tumour types. For example, there is evidence to suggest an association between the presence of a NTRK fusion and unfavourable disease presentation159,160 and better prognosis in patients with congenital mesoblastic nephroma who harbour a NTRK fusion than in those without the genetic abnormality. 161 The evidence across tumour types is limited but the prognosis of patients with NTRK fusions may vary between cancer types and between NTRK fusion types. 161 From the evidence available, it is also unclear if NTRK fusions are in themselves prognostic or if it is their association with other specific prognostic factors, such as age and Eastern Cooperative Oncology Group (ECOG) performance status, that drives the observed differences in prognosis.
Adjustment for confounding bias
A key factor in the reliability of estimates of effectiveness based on observational data is the statistical analysis used; a large number of studies have sought to develop and evaluate methods for adjusting and eliminating bias resulting from confounding. These include methods such as regression analysis, propensity scoring and population-adjusted indirect comparisons [matching-adjusted indirect comparison (MAIC) and Simulated Treatment Comparison (STC)]. 162 These and other methods are frequently used in the literature and have been previously applied and accepted by NICE appraisal committees where no randomised evidence exists. In theory, these methods could be applied in the context of a histology-independent appraisal. Implementing such approaches could, however, be challenging because of the large number of source data sets involved, which means that population characteristics may not be reported across all comparator data sources and would necessarily require strong assumptions about the prognostic value of population characteristics across tumour types. Furthermore, even if a suitable adjusted comparison could be generated, the small sample sizes typically seen in the Phase II trials would be able to account for only a small number of observed characteristics. This limits the potential for these methods to fully account for confounding biases and increases the likelihood of residual confounding bias. Despite these limitations, such methods would generally be considered to be preferable to a naive comparison, which takes no account of differences across groups.
Gaps in the reporting of baseline characteristics, variability in the prognostic value of characteristics across tumour types/histologies and difficulties of matching comparator data to the likely limited available Phase II trial for histology-independent technologies will also create additional challenges of interpretation and validation of comparisons with historical data, as it will be challenging to assess the comparability of patients in the historical control with those in the available single-arm trial data.
Alternative approaches to developing a comparator
Because of these significant concerns of confounding bias and the challenges of generating a truly comparable comparator data set, other approaches to generating a comparator data set should be considered and their limitations explored. For example, two alternative methods outlined in Hatswell et al. 163,164 could be used, in which patients in the single-arm trial are used to generate a control arm.
The first approach proposed by Hatswell et al. 163 uses effectiveness data on non-responders as a proxy for patients not receiving an active treatment. Comparator effectiveness estimates of PFS and OS under this approach would, therefore, be based on observed PFS and OS among non-responders in the integrated efficacy analysis. The advantage of this approach is that all patients in the non-responder subgroup met the same trial inclusion/exclusion criteria and received the same line of treatment. The rationale behind this approach is that patients in whom no response is observed represent those with a lack of treatment effect (because they have no response to treatment) and, therefore, are representative of a counterfactual for whom no effective therapy exists. The patient population is, therefore, likely to be better matched with the intervention arm because they are drawn from the same population.
This approach, however, also requires strong assumptions, namely that there are no differences other than response status between responders and non-responders that explain the survival outcomes and that non-responders derive equivalent benefit to that received on current SoC. The reasonableness of these assumptions is likely to be specific to a particular appraisal; however, as discussed in Chapter 4, the reliability of response as a surrogate is likely to be variable across tumour types. The assumption of no treatment benefit or harm may also not hold because some patients may receive some benefit from treatment, even if they do not have a PR or CR.
When considering the appropriateness of this approach, the relative advantages and disadvantages will need to be considered and it may be that this approach is considered reasonable only where there is substantive evidence of heterogeneity in treatment effects justifying the need to appropriately account for this heterogeneity in the economic analysis.
The second approach164 uses data taken from the trial patients’ previous line of treatment to derive OS and PFS curves. In this approach, the inverse of the ratio between the average TTP on their previous therapy and the mean extrapolated PFS with the active therapy [also called the growth modulation index (GMI) multiplier] is applied to all health outcomes (PFS and OS) for the active therapy. This crude adjustment assumes that the active therapy is more effective in terms of both PFS and OS than the comparator, by the same proportion as the GMI multiplier. Therefore, the resulting GMI-adjusted total mean life-years gained (LYG) and quality-adjusted life-years (QALYs) are assumed to correspond to comparator outcomes and are applied in the calculation of the ICER (based on LYG and QALYs). The main advantage is that effect estimates are drawn from the same population as the intervention arm and, therefore, are better matched; however, there are also disadvantages. First, this can be implemented only for patients who have received a previous line of therapy. Second, it also assumes that the ratio of TTP across lines of therapy is indicative of the treatment effect and it is uncertain to what degree this is likely to hold true. Finally, because this method can estimate PFS only, it requires that assumptions are made about the impact of TTP gains on OS [namely that either OS increases proportionally with TTP or post-progression survival (PPS) is the same across therapies], which, similarly, may not hold true. Further research considering the reasonableness of these assumptions may be helpful. Consideration could also be given to the potential role of expert elicitation to inform these judgements.
Implications for the appraisal of histology-independent technologies
The broad marketing authorisation, heterogeneous populations and uncertainties regarding the position of histology-independent technologies creates a number of significant challenges to creating appropriate historical control data. The confidence in estimates of effect may increase by utilising methods of population adjustment, but the scope of such methods may be more limited in the context of histology-independent appraisals. The assessment of the scope for residual confounding bias is also likely to be made more complicated, further reducing the confidence in comparisons. It is unclear whether or not the use of non-randomised evidence and, in particular, single-arm studies will ever be considered adequate. Alternative methods of developing a comparator may, therefore, be of value to decision-makers and should be considered as alternatives.
Generalisability
The extent to which evidence is generalisable to the population of interest is a key consideration in the appraisal of histology-independent cancer technologies. There may be a number of uncertainties concerning the generalisability of the available evidence, including the different types and distribution of histologies in the clinical studies (and the extent to which these represent the specific types and distribution of histologies that would be expected in routine clinical practice); the potential impact of unrepresented histologies not represented in existing clinical studies; and the position in the treatment pathway. Each of these issues is discussed in turn in the following sections.
Distribution of tumour types
As outlined in Chapter 2 (see Food and Drug Administration review of histology-independent products and European Medicines Agency review of approved histology-independent indications), the evidence likely to be available for decision-making will include a number of histologies or tumour types, with limited data on each. When integrating these clinical data into a cost-effectiveness analysis, one important issue is to consider the distribution of patients across the different tumour types.
One approach would be to utilise the distribution of tumour types present in the clinical evidence to generate an average cost-effectiveness estimate. Underlying this approach, however, is the assumption that the cost-effectiveness of a histology-independent technology does not vary across tumour types or that the proportions of histologies are representative of the proportions eligible to receive the intervention in the full licensed population. The former assumption is very unlikely to hold owing to the potential for differences in effectiveness across tumour types, prognosis, comparators, costs and HRQoL. Furthermore, as shown in the comparison of the distributions in Table 14, there is a mismatch between the distribution of certain histologies in the trial populations. For instance, breast cancer patients represent 11% of the entrectinib trial but only 1.8% of the larotrectinib trial. If we imagine that larotrectinib and entrectinib produce identical clinical results, the resulting average cost-effectiveness estimates of the two distributions will be different given that different tumour types have different testing costs (see Genomic testing for histology-independent drugs), different SoC costs and outcomes, and potentially different prognoses.
Histology | Trial proportion (%) |
---|---|
Larotrectinib efficacy evaluable data set | |
Soft tissue sarcoma | 20.00 |
Salivary gland | 21.80 |
Thyroid | 9.10 |
Lung | 7.30 |
Colon | 7.30 |
Cholangiocarcinoma | 3.60 |
Breast | 1.80 |
Pancreas | 1.80 |
IFS | 12.70 |
Melanoma | 7.30 |
GIST | 5.50 |
Appendix | 1.80 |
Entrectinib efficacy evaluable data set | |
Sarcoma | 24.00 |
Salivary gland (MASC) | 13.00 |
Thyroid | 9.00 |
NSCLC | 18.00 |
Colorectal | 7.00 |
Cholangiocarcinoma | 2.00 |
Breast | 11.00 |
Pancreatic | 6.00 |
Neuroendocrine | 6.00 |
Gynaecological | 4.00 |
The significance of these differences for the overall assessment of cost-effectiveness will depend on the degree of heterogeneity across separate inputs relevant to economic modelling. If the trial distribution is not considered to represent the distribution expected to be seen in the population under a histology-independent license, any decision based on a single ICER estimate for the trial population will be subject to potential bias. The magnitude and direction of this bias will be difficult to determine without a more explicit assessment of heterogeneity in different sources relevant to the economic model.
Where differences between the trial population and the licensed population are considered significant, approaches should be explored that allow for the re-weighting of the clinical population so that the model population better reflects the treated population.
Unrepresented tumour types
A further issue to consider is whether or not the trial evidence encompasses all of the histologies covered by marketing authorisation. If histologies exist that are not represented in the trials but are covered under the marketing authorisation, decision-makers will have evidence on effectiveness from only the subset of the total population that is potentially eligible for the intervention.
For example, within the clinical evidence available for entrectinib (see Table 11), 12 histologies were included. However, it is known that upwards of a further 17 histologies have been shown to harbour NTRK fusions and will be covered by the anticipated marketing authorisation; hence, this total could be even larger. 165 Any decisions made on the evidence alone would, therefore, be implicitly assuming that the 12 included types are representative of the full population.
The impact of the unrepresented population is potentially significant and its importance will depend on the number of unrepresented histologies and the proportion of the eligible population in unrepresented histologies relative to the observed histologies. It is also important to consider unrepresented tumours for which there is significant uncertainty regarding the homogeneity of clinical benefits or significant heterogeneity in costs across tumour types. For example, where there is limited support for the assumption of homogeneous efficacy across histologies, it may be important to characterise the uncertainty in the efficacy within the unrepresented population. Equally, there is significant evidence of variability of testing costs across tumour types and, therefore, ignoring unrepresented tumour types may impact significantly on average testing costs.
Position in the treatment pathway
A further potential limitation regarding the generalisability of the available clinical evidence relates to the position in the pathway at which patients are treated. This is complicated in part because the position in the pathway may vary substantively across tumour types according to the availability of alternative treatments, but also because of the potential for a mismatch between the trial population and the eligible population, as dictated by the marketing authorisation. The latter may be a significant issue because the recruitment of patients to a histology-independent trial is necessarily more complicated and there are potential significant challenges to identifying patients owing to the relative rarity of target genetic mutations. Thus, as observed in the entrectinib and larotrectinib clinical data, patients were recruited across multiple lines of therapy, even within the same tumour type.
This heterogeneity generates a number of issues, not least with respect to the external validity of the trial. Line of therapy may be a significant prognostic factor and failure to adjust for this may impact significantly on estimates of relative effectiveness, particularly if the comparator population is not matched to the position that patients were treated in the treatment arm. This issue may also impact in other ways, including on the final distribution of patients eligible for treatment, because fewer patients will be eligible for treatment in second and subsequent lines of therapy. Furthermore, it may have implications for testing, affecting either the total costs of testing or the population that will be eligible for testing.
Example
The example considers the TRK inhibitor, larotrectinib, which is used for the treatment of solid tumours harbouring a NTRK gene fusion, and the proportion of tumour types presented in the clinical evidence, as outlined in Exploring heterogeneity in response: case study.
First, to quantify the size of the population that will benefit from TRK inhibitors, the total number of patients eligible each year was calculated. This was estimated using the tumour-specific NTRK fusion prevalence, cancer incidence and proportion of patients with advanced or metastatic disease:
The full method for calculating the annual eligible population is described in Appendix 12.
Table 15 presents the calculations of the annual eligible population for TRK inhibitors based on the tumour types represented in the larotrectinib trial. The prevalence of NTRK fusion varies across tumour types, ranging from 92.2% (MASC) to 0.07% (breast cancer). Paediatric patients with infantile fibrosarcoma, a tumour type with a high NTRK fusion prevalence, make up the largest proportion of the eligible population (n = 27). Despite the low prevalence of NTRK fusion, patients with CRC contribute a substantial proportion of the eligible population (n = 23).
Tumour type | Prevalence of NTRK fusion (%) | Cancer incidence (England) (%) | Per cent with stage III/IV cancer at diagnosis | Annual TRK-inhibitor eligible population (n) |
---|---|---|---|---|
Soft tissue sarcoma | 0.56 | 2740 | 32 | 5 |
Appendix | 4.00 | 540 | 74 | 16 |
Breast | 0.07 | 46,102 | 15 | 5 |
Cholangiocarcinoma | 0.10 | 556 | 60 | 0 |
Colorectal | 0.12 | 34,825 | 55 | 23 |
IFS | 90.90 | 59 | 51 | 27 |
MASC | 92.90 | 11 | 22 | 2 |
Melanoma | 0.21 | 13,740 | 9 | 3 |
NSCLC | 0.09 | 32,576 | 57 | 17 |
Pancreatic | 0.26 | 8388 | 78 | 17 |
Thyroid | 0.92 | 2195 | 31 | 6 |
GIST | 1.28 | 734 | 40 | 4 |
Total | 125 |
The resulting distribution of the eligible population (Table 16) shows that the proportions of tumour types in the eligible population differ substantially to the proportions in the larotrectinib trial (see Table 16). For example, soft tissue sarcoma represents 20% of the population in the larotrectinib trial yet represents only 4% of the population eligible to receive larotrectinib.
Tumour Type | Trial population (n) | Distribution of tumour types in the trial (%) | Eligible population (n) | Distribution of tumour types in the eligible population (%) | Distribution of tumour types in the eligible population, including the unrepresented tumour types (%) | Assumed proportion treated based on line of therapy (%) | Treated population based on line of therapy | Eligible population including line of therapy (%) |
---|---|---|---|---|---|---|---|---|
Represented | ||||||||
Soft tissue sarcoma | 11 | 20 | 5 | 4.00 | 1.80 | 90 | 4.5 | 4.20 |
Appendix | 1 | 2 | 16 | 12.80 | 5.80 | 30 | 4.8 | 4.40 |
Breast | 1 | 2 | 5 | 4.00 | 1.80 | 30 | 1.5 | 1.40 |
Cholangiocarcinoma | 2 | 4 | 0 | 0.00 | 0.00 | 30 | 0 | 0.00 |
Colorectal | 4 | 7 | 23 | 18.40 | 8.30 | 30 | 6.9 | 6.40 |
GIST | 3 | 5 | 4 | 3.20 | 1.40 | 30 | 1.2 | 1.10 |
IFS | 7 | 13 | 27 | 21.60 | 9.80 | 90 | 24.3 | 22.40 |
MASC | 12 | 22 | 2 | 1.60 | 0.70 | 60 | 1.2 | 1.10 |
Melanoma | 4 | 7 | 3 | 2.40 | 1.10 | 30 | 0.9 | 0.80 |
Lung | 4 | 7 | 17 | 13.60 | 6.20 | 60 | 10.2 | 9.40 |
Pancreatic | 1 | 2 | 17 | 13.60 | 6.20 | 30 | 5.1 | 4.70 |
Thyroid | 5 | 9 | 6 | 4.80 | 2.20 | 30 | 1.8 | 1.70 |
Unrepresented | ||||||||
Congenital mesoblastic nephroma | – | – | 0 | – | 0.10 | 30 | 0.07 | 0.10 |
Cervix | – | – | 2 | – | 0.70 | 30 | 0.62 | 0.60 |
Gastro–oesophageal junction | – | – | 4 | – | 1.40 | 30 | 1.16 | 1.10 |
HNSCC | – | – | 24 | – | 8.60 | 30 | 7.14 | 6.60 |
Neuroendocrine | – | – | 7 | – | 2.50 | 30 | 2.08 | 1.90 |
Ovarian | – | – | 4 | – | 1.40 | 30 | 1.13 | 1.00 |
Papillary thyroid tumour | – | – | 44 | – | 15.80 | 30 | 13.07 | 12.10 |
Paediatric high-grade glioma | – | – | 4 | – | 1.30 | 30 | 1.06 | 1.00 |
Paediatric melanoma | – | – | 2 | – | 0.80 | 30 | 0.62 | 0.60 |
Prostate | – | – | 44 | – | 16.00 | 30 | 13.29 | 12.30 |
Renal cell carcinoma | – | – | 8 | – | 3.00 | 30 | 2.45 | 2.30 |
Salivary gland | – | – | 6 | – | 2.00 | 30 | 1.68 | 1.60 |
Secretory breast carcinoma | – | – | 1 | – | 0.20 | 60 | 0.35 | 0.30 |
Sinonasal adenocarcinoma | – | – | 0 | – | 0.00 | 30 | 0 | 0.00 |
Uterine | – | – | 1 | – | 0.50 | 30 | 0.43 | 0.40 |
High-grade glioma | – | – | 1 | – | 0.50 | 60 | 0.8 | 0.70 |
Total | 55 | 100 | 276 | 100 | 100 | – | 108 | 100 |
Unrepresented tumour types
In addition, NTRK fusions have been found in numerous tumour types that were not included in the larotrectinib trial. Following a histology-independent approval decision, patients with these tumour types will be eligible for treatment. In addition to the 12 tumour sites included in the larotrectinib trial, there is evidence of NTRK fusions in an additional 17 tumour types or anatomical sites. 165 The annual eligible population making up the unrepresented tumour types for larotrectinib was again estimated using Equation 4. The size of the unrepresented population was calculated to be 152 patients, 55% of the annual eligible population. The calculation of the size of the unrepresented population can be seen Appendix 11.
The eligible population, including the unrepresented population, is shown in Table 16. As can be seen from the comparison of the proportion of tumour types in the eligible population with and without the inclusion of the unrepresented tumour types, the proportions differ. Individuals with soft tissue sarcoma represented 4% of the tumours in the eligible population and 1.8% when the unrepresented population was included.
Position in the treatment pathway
If we assume that the TRK inhibitors will be given as a first-line therapy and that 100% of patients will receive it for every tumour type, the distribution of tumour types will be the real-world distribution. However, testing and position in the treatment pathway can impact this distribution.
The position at which genomic testing is offered to identify NTRK fusions will alter the annual population eligible for larotrectinib and other TRK inhibitors. If testing was offered at the position in the treatment pathway that larotrectinib would be given, the annual eligible population will be smaller than the population identified by upfront screening because some individuals who were NTRK fusion positive could have responded to alternative therapies, not been fit enough or have died before becoming eligible for TRK inhibitor treatment (see Genomic testing for histology-independent drugs).
Given that the position of larotrectinib is likely to differ between tumours, owing to the availability of other ‘satisfactory’ therapies, the overall distribution of individuals across tumour types in the eligible population is likely to change relative to the distribution assuming that 100% of eligible patients receive the TRK inhibitor as a first-line therapy (see Table 16).
To demonstrate the impact that the position in the treatment pathway will have on the distribution of patients eligible for treatment with larotrectinib, an estimate of the likely position was obtained from the FDA review for larotrectinib. 9 For the tumour types for which there was no indication of where larotrectinib would be positioned, it was assumed that the drug would be offered as a third-line therapy. Based on clinical advice, it was assumed that, for the tumours for which larotrectinib was offered as first-line therapy, 90% of eligible patients would be fit enough for treatment. It was assumed that, in those who were offered larotrectinib as a second-line and third-line therapy, 60% and 30% of the eligible population would be treated with larotrectinib, respectively. As can be seen in Table 16, when the position in the treatment pathway is considered, the distribution of tumour types changes. For example, IFS increased from 9.8% to 22.4% of the population when accounting for the position in the treatment pathway.
By comparing the trial distribution in Table 16 with the eligible population, including the unrepresented tumour types and allowing for the position in the treatment in the pathway, we can see the considerable difference in the proportions of tumour types. If the cost-effectiveness of a histology-independent technology is based on single estimates of costs and outcomes based on the average of tumour types present in the clinical evidence, the resulting uncertainty will be significant given that incremental costs and outcomes are likely to differ substantially across tumour types.
Implications for the appraisal of histology-independent technologies
In summary, the trial population may include only a subset of the total population potentially eligible for the intervention. Indeed, it is feasible that the majority of histologies potentially harbouring the biomarker will not be represented in the evidence. Furthermore, matching the line of therapy in the trial population to the eligible population is important given the likely prognostic effect of line of therapy. However, matching can be difficult if there is ambiguity in the treatment position specified within the relevant marketing authorisation.
Genomic testing for histology-independent drugs
Genomic testing is likely to be integral to identifying patients who are eligible for histology-independent therapy. The NICE approval of numerous targeted therapies has been coupled with significant investment in genomic testing services in the NHS. 153 Although genomic services are currently set up to identify oncogenic mutations in over 60 tumour types,166 the provision of histology-independent testing poses new challenges that need to be considered before appraising the value of histology-independent technologies.
Overview of molecular testing in the UK
Substantial investment and changes to genomic testing services have been undertaken in the last 5 years after a demand to improve the access to genomic services in the NHS to inform the most effective treatment pathway for a patient with cancer. 158 In 2018, the NHS launched the Genomic Medicine Service and a National Genomic Testing Strategy, which was based in seven genomic laboratory hubs across England. 153 Although this provides positive steps to improve the availability of genomic testing across the UK, the services are still being implemented, leading to limited capacity in some genomic laboratory hubs.
In March 2019, the genomic test directory listed 968 genomic tests available for 64 adult and paediatric tumour types. 166 Although this may seem an exhaustive number of tests for a large proportion of tumour types, it is far from inclusive. Patients with some common tumours, including prostate cancer (a population that contributes 15% of the annual incidence of solid tumours in England), are not eligible for any form of genomic testing because there are currently no effective targeted therapies licensed on the NHS. Although the absence of genomic testing until now may be because of limited evidence of known somatic or hereditary mutation that will be of prognostic or diagnostic value, the provision of a targeted histology-independent therapy would require screening of all cancers, regardless of current availability.
Types of genomic test
Tumourigenesis, the process of cancer growth and development, is driven by genetic alterations that result in sustained cell proliferation or the inhibition of cell division and death. 167 In fact, by the time that a cancer is diagnosed, there are likely to be millions of genetic mutations within a single malignancy. 168
Many of these alterations occur during tumour development but do not contribute to tumour growth, commonly known as ‘passenger mutations’; therefore, these play no functional role in cancer development. These mutations may occur in non-coding sequences of DNA that are removed during the transcription of DNA to RNA as part of gene expression. By contrast, the ‘driver’ mutations are involved in the neoplastic growth of the tumour, which directly result in the prolific growth of the cancer cells. Driver mutations can be differentiated further with respect to whether they solely influence the initial cancer development or whether oncogenic growth and proliferation are dependent on the mutation, regardless of its position in the disease pathway. 169
Therefore, the role of genetic testing is two-fold: first, to detect the presence or absence of a specific mutation and, second, to determine whether the mutation is acting as an oncogenic driver or whether it is merely a passenger mutation in tumourigenesis.
There are a variety of tests that are available to identify the presence of a mutation in individuals. These include DNA- and RNA-based panel tests, whole-genome sequencing (WGS), IHC, FISH and reverse transcription polymerase chain reaction (RT-PCR). Each of these tests determines the presence or absence of a genetic mutation in different ways, from identifying a known driver mutation using targeted tests in DNA and RNA to sequencing the entire genome, or determining the level of expression of a particular protein. The suitability of the alternative types of test will probably depend on the target mutation and the test’s diagnostic accuracy to correctly detect the respective alteration, the prevalence of the genetic mutation within each tumour type and the current testing provision. Table 17 summarises the key characteristics of each test type, noting key advantages and limitations.
Test | Methodology | Advantages | Disadvantages |
---|---|---|---|
DNA-based NGS | Analyses genomic DNA from a tumour sample and can be used to identify mutations in multiple genes concurrently. Targeted panels can be used to identify particular DNA rearrangement, known to have an oncogenic effect |
|
|
WGS | Sequences the entire genome of DNA against a comparator to identify specific genetic alterations known to play a role in tumourigenesis | ||
RNA-based NGS | Analyses the transcriptome (the collection of all RNA sequences in a cell). RNA sequencing provides a more accurate test for determining whether or not genetic mutations are expressed as proteins |
|
|
IHC | IHC detects the expression of a protein through the use of antibodies, which bind to a specific receptor (or antigen) on the protein of interest. A tag attached to the antibody will react if bound and produce a stain, which signals the expression of the protein |
|
|
FISH | Uses a probe on a sequence of DNA that complements a particular genetic alteration.173 Each probe is labelled with a fluorescent marker that, when illuminated, will indicate the presence of the mutation |
|
|
RT-PCR | Uses a probe on a sequence of RNA that complements a particular genetic alteration.173 Each probe is labelled with a fluorescent marker that, when illuminated, will indicate the presence of the mutation |
|
Tests may be combined as part of a testing strategy, where confirmatory testing is implemented to verify that a mutation is being expressed. This allows for diagnostic accuracy to be maintained, while reducing the use of more expensive and resource-intensive test types. For example, IHC may be used as a screening tool to detect protein expression, with a further confirmatory test implemented to verify that the protein expression is caused by the mutation of interest. The relevance of strategies based around IHC may, however, become more limited as panel testing using NGS is expanded within the NHS.
Because of the variable provision of testing in the NHS across tumour types, the most appropriate testing strategy will probably depend on the tumour type. For example, all paediatric patients with advanced and metastatic cancer in the NHS will receive WGS at diagnosis by 2020158 and, therefore, any testing strategy is likely to be built on this provision. The appropriateness of each testing strategy will also depend on the prevalence of the genetic alternations across tumour types. Diagnostic accuracy will vary depending on the prevalence of the genetic alteration within each tumour type even when the sensitivity and specificity are held constant (see Appendix 12).
Implications of testing for appraisal of histology-independent technologies
The need for companion diagnostic testing to implement histology-independent technologies has several consequences for cost-effectiveness. These considerations include resource implications associated with implementing testing and the impact of alternative testing strategies on the modelled population, as well as broader implications regarding the feasibility of expanding testing services. These issues are briefly discussed in the following sections, followed by a worked example considering the implementation of testing for NTRK fusions.
Costs
The costs associated with identifying patients will be driven by a range of factors, including the testing strategy adopted and current provision of testing in the NHS. Because these may vary across tumour types, incremental testing costs may also vary across tumour types. The variability in testing costs across tumour types will also be determined by variability in the frequency of a genetic mutation across specific tumour types, with increased rarity increasing the costs of identifying an eligible patient. As is illustrated in the worked example of NTRK fusions below, the variability in the frequency of target genetic alterations can be significant, ranging from < 0.2% to > 90%. This has a significant impact on the number of patients who need to be screened [number needed to screen (NNS)] and, consequently, the variability in the tumour type-specific costs of identifying patients is similarly wide. Testing costs are a significant source of heterogeneity and, if all testing costs are attributable to a single histology-independent drug, are likely to render a technology cost-ineffective for some tumour types. In the context of NTRK fusions, which, on average, occur in < 0.5% of all advanced cancer patients, the average costs of testing are high and are likely to represent a significant proportion of the total incremental costs associated with the implementation of TRK inhibitors.
Attributing testing costs
The current NICE methods guide outlines that the costs of testing should be included if they are specifically associated with the provision of the technology being appraised. 4 The implementation of wide-scale genomic testing is, however, likely to represent a public good that may allow for the identification of other relevant genetic alterations (e.g. where wide-spread panel testing is implemented). This may be of particular relevance where there are multiple targeted therapies available or likely to become available in the near future. Accounting for such positive externalities may be important because testing costs may not justify the implementation of a specific single technology but may be justifiable when shared across multiple technologies. The estimation of the magnitude of any positive externalities resulting from testing is, however, non-trivial and methods of how to attribute testing costs across multiple technologies have not been established. How costs should be attributed across technologies is currently unclear; for example, costs could be split equally or by the size of the eligible population and would necessitate a co-ordinating role for either NHS England or NICE to potentially set a tariff on which attributable testing costs could be based. 9,10
Feasibility
Although there is currently provision for genomic testing for several cancers within the NHS, there are significant uncertainties surrounding the practical feasibility of providing wide-scale histology-independent testing.
The feasibility of testing is also dependent on whether testing is offered at the point of diagnosis or at the position in the treatment pathway at which the drug would be given. Where testing is implemented on eligibility for treatment, the NNS will be indicative of the number of patients who will go on to receive treatment because it is expected that all patients who test positive receive the therapy. Given that entrectinib and larotrectinib are offered when there is no ‘acceptable’ alternative therapy,9,10 there may be significant disparity between the NNS and the final number of patients who go on to receive therapy. This is because there is significant attrition in the number of patients who go on to receive second or later lines of therapy, as a result of patients either dying or becoming unfit for treatment.
Given the potential variety of histology-independent drugs that could be available across a range of positions in treatment pathways in each tumour type, genomic testing at diagnosis of advanced or metastatic cancer is the most plausible, despite that the initial investment would be significant. Based on the annual incidence of cancer in the UK and the average proportion of individuals with advanced or metastatic cancer, 94,595 individuals would require genomic testing each year. This figure represents a significant increase in the number of molecular and genomic tests and, given the variability in the UK’s capacity to implement wide-scale NGS testing, it is expected that it will take some time for the appropriate infrastructure to be put in place. A phased introduction of NGS panel testing is likely over the next few years, with NHS England anticipating that full implementation of pan-cancer testing will be in place by the end of 2022. 158
Identifying patients eligible for TRK inhibitors based on the presence of a neurotrophic tyrosine receptor kinase fusion: a worked example
This section considers how testing for NTRK fusions might be implemented in the NHS and provides an illustrative example of how both NNS and testing costs might vary across tumour types.
A variety of testing strategies have been proposed for identifying patients with a NTRK fusion, pending the approval of two histology-independent TRK inhibitors. 172,174 The European Society for Medical Oncology (ESMO) proposes that the standard testing pathway should differ depending on the frequency of NTRK fusions in each tumour type and whether or not genomic sequencing is currently provided by the NHS. 166 In the tumour types for which there is a lower frequency of NTRK fusions and for which there is no genomic testing available, it is suggested that IHC is used for initial screening; NTRK gene rearrangements are then confirmed using RNA-based NGS. IHC is high throughput and inexpensive, making it a practical screening tool to use in a large population. Following this with more expensive and highly accurate RNA-based NGS is a plausible testing strategy for identifying tumours. Diagnostic accuracy is further taken into account by stratifying the tumour types into different testing strategies, depending on their NTRK fusion prevalence.
Conversely, it has also been suggested that front-line NGS should be offered to all individuals to detect a NTRK fusion. 174 Although this would require substantial investment, this testing strategy would ‘future-proof’ histology-independent testing because additional mutations could be added to pre-existing panels. This would mean that a single tumour sample could be screened to identify a number of genetic alterations. However, in the short term this testing strategy will require significant resources to implement nationally.
The most exhaustive approach to identify NTRK fusions utilises DNA-based NGS and RNA sequencing. Given that DNA-based NGS is currently available for some tumours, there will be reduced incremental investment in providing RNA sequencing, which would be used to confirm protein expression in the positive cases.
Although there is the potential for NTRK fusions to be observed in any tumour type, current evidence documents the occurrence of NTRK fusions in only around 30 different tumour types. 175–178 However, this list cannot be considered complete. It is plausible that NTRK fusions occur in common tumour types, but with such rarity that they are yet to be detected. There are also likely to be a number of rarer tumour types that express NTRK fusions but are not included in any current database. To align with the available evidence on the prevalence of NTRK fusion, we distinguish between tumour types within a single anatomical site only when there is supporting evidence on the prevalence of NTRK fusions to do so.
Methods
For each tumour type for which there is evidence to support the prevalence of NTRK fusions, we estimated the following:
-
the NNS, or number of individuals who would require genomic testing each year to identify one individual with a NTRK fusion
-
the average cost of testing associated with identifying one NTRK fusion patient
-
an illustration of the cost-effectiveness of NTRK testing for each tumour type.
This was implemented for three testing strategies that could be adopted to identify patients with NTRK fusions.
The first testing strategy was based on recent recommendations for the identification of NTRK fusions published by the ESMO. 174 IHC followed by confirmatory RNA-based NGS would be recommended for the tumour types in which NTRK fusions are rare. In the tumours where NTRK fusions are highly prevalent, first-line FISH should be utilised. For the tumour types for which WGS is currently available and reimbursed by the NHS, RNA-based NGS would be required to confirm the presence of an oncogenic NTRK fusion.
To complement the substantial investment in genomic testing services in the NHS, the second strategy was assumed to be based on using RNA-based NGS as a first-line test for all patients. For tumour types for which WGS is currently available, it was assumed that RNA-based NGS would be used to confirm the presence of an oncogenic NTRK fusion.
Finally, an alternative testing strategy was considered based on an exhaustive approach outlined by ESMO, which seeks to maximise current testing availability of DNA-based NGS in each tumour type. Under this approach, DNA-based NGS is used as a first-line screening tool, followed by confirmatory RNA-based NGS. This was suggested by ESMO to be the most exhaustive approach to identify NTRK fusions. 174
Number needed to screen
The NNS to identify one patient eligible for TRK inhibitors is based on NTRK fusion prevalence and the diagnostic accuracy of the respective tests (see Appendix 11 for details of the calculations). To our knowledge, there is no literature concerning the diagnostic accuracy of WGS in detecting NTRK fusions. As a result, the diagnostic accuracy of WGS for detecting NTRK fusions was based on sensitivity and specificity estimates of DNA-based NGS. Table 18 presents the diagnostic accuracy for each test.
Test | Sensitivity (%) | Specificity (%) |
---|---|---|
RNA sequencing179 | 100 | 100 |
WGS and DNA-based NGS171 | 81.10 | 99.86 |
Immunohistochemistry180 | 87.90 | 81.10 |
FISH (ETV6–NTRK3)181 | 80.00 | 100 |
The NNS with a first-line (FL) test was estimated using the tumour type-specific prevalence of NTRK fusions and the corresponding first-line test sensitivity (Sn) using the following equation:
Confirmatory RNA testing is required for patients who require first-line IHC, WGS or DNA-based NGS. The NNS with a confirmatory (C) test was estimated using the sensitivity and specificity of the respective test and the tumour-specific NTRK fusion prevalence:
The cost of testing to identify one eligible patient for TRK inhibitors
The incremental cost of testing to identify one eligible patient was estimated for each tumour. Genomic testing, in the form of DNA-based NGS, WGS and FISH, is currently reimbursed by the NHS for some tumours. The price for each test was acquired from a UK Genomic Centre: IHC and FISH were costed at £150, and DNA- and RNA-based NGS were priced at £250 and £350, respectively. 182 The cost of WGS was assumed to be £800. 182
The incremental cost to identify one eligible patient in each tumour type was calculated by:
An average of the tumour-specific testing costs was used to calculate the cost of identifying one individual with a NTRK fusion for each testing strategy. The average cost was weighted in accordance with the annual eligible population for TRK inhibitors for each tumour.
Value of implementing neurotrophic tyrosine receptor kinase testing
To illustrate how cost-effectiveness may vary across tumour types because of the variation in testing costs, a hypothetical scenario was considered in which ICERs were calculated for each tumour type. This analysis was based on the incremental costs of testing only and excludes other costs associated with the treatment (e.g. drug costs). The ICERs estimated the difference in testing costs between testing for NTRK and current testing provision relative to the benefits (quantified by QALYs). Incremental benefits were based on the Canadian Agency for Drugs and Technologies in Health183 assessment of the cost-effectiveness of larotrectinib, which estimated that larotrectinib produced an additional 0.833 QALYs per patient compared with standard care.
Results
Tables 19–21 present the number of individuals who would need to be tested to identify one eligible patient for each testing strategy. The incremental cost associated with testing and hypothetical estimates of cost-effectiveness of testing are also presented.
Tumour type | NNS | Incremental cost to identify one patient (£) | ICER (NTRK fusion testing vs. current testing provision) (£) | |
---|---|---|---|---|
First line | Confirmatory | |||
Tumours in the trial | ||||
Appendix | 30.83 | 6.16 | 6780 | 8139 |
Breast | 1625.22 | 307.95 | 351,567 | 422,049 |
Cholangiocarcinoma | 1137.66 | 215.80 | 246,179 | 295,533 |
Colorectal | 948.05 | 179.97 | 205,195 | 246,333 |
GIST | 88.88 | 17.58 | 19,486 | 23,393 |
IFS | 1.36 | 1.00 | 350 | 420 |
MASC | 1.35 | 0.00 | 0 | 0 |
Melanoma | 541.74 | 103.17 | 117,372 | 140,903 |
NSCLC | 1264.06 | 239.69 | 273,502 | 328,334 |
Pancreatic | 437.56 | 83.48 | 94,853 | 113,870 |
Soft tissue sarcoma | 220.19 | 1.31 | 457 | 549 |
Thyroid | 123.66 | 24.16 | 27,003 | 32,417 |
Tumours not represented in the trial | ||||
Cervix | 344.74 | 65.94 | 74,791 | 89,785 |
Congenital Mesoblastic Nephroma | 2.03 | 1.00 | 350 | 421 |
Gastro–oesophageal junction | 1137.66 | 215.80 | 246,179 | 295,533 |
HNSCC | 299.38 | 57.37 | 64,986 | 78,015 |
High-grade glioma | 2275.31 | 430.82 | 492,084 | 590,737 |
Neuroendocrine | 379.22 | 72.46 | 82,243 | 98,731 |
Ovarian | 455.06 | 86.79 | 98,637 | 118,411 |
Papillary thyroid tumour | 8.55 | 2.40 | 2124 | 2549 |
Paediatric high-grade glioma | 23.27 | 1.03 | 361 | 433 |
Paediatric melanoma | 11.10 | 1.01 | 355 | 426 |
Prostate | 455.06 | 86.79 | 98,637 | 118,411 |
Renal cell carcinoma | 455.06 | 86.79 | 98,637 | 118,411 |
Salivary gland | 66.14 | 13.29 | 14,572 | 17,493 |
Secretory breast carcinoma | 1.36 | 0.00 | 0 | 0 |
Sinonasal adenocarcinoma | 455.06 | 86.79 | 98,637 | 118,411 |
Uterine | 1137.66 | 215.80 | 246,179 | 295,533 |
Tumour type | NNS | Incremental cost to identify one patient (£) | ICER (£) | |
---|---|---|---|---|
First line | Confirmatory | |||
Tumours in the trial | ||||
Appendix | 30.83 | 0.00 | 10,789 | 12,952 |
Breast | 1428.57 | 0.00 | 500,000 | 600,240 |
Cholangiocarcinoma | 1000.00 | 0.00 | 350,000 | 420,168 |
Colorectal | 833.33 | 0.00 | 291,667 | 350,140 |
GIST | 78.13 | 0.00 | 27,344 | 32,826 |
IFS | 1.36 | 1.00 | 350 | 420 |
MASC | 1.08 | 1.00 | 215 | 258 |
Melanoma | 476.19 | 0.00 | 166,667 | 200,080 |
NSCLC | 1111.11 | 0.00 | 388,889 | 466,853 |
Pancreatic | 384.62 | 0.00 | 134,615 | 161,603 |
Thyroid | 220.19 | 1.31 | 457 | 549 |
Soft tissue sarcoma | 108.70 | 0.00 | 38,043 | 45,670 |
Tumours not represented in the trial | ||||
Cervix | 303.03 | 0.00 | 106,061 | 127,324 |
Congenital mesoblastic nephroma | 2.03 | 1.00 | 350 | 421 |
Gastro–oesophageal junction | 1000.00 | 0.00 | 350,000 | 420,168 |
HNSCC | 263.16 | 0.00 | 92,105 | 110,571 |
High-grade glioma | 2000.00 | 0.00 | 700,000 | 840,336 |
Neuroendocrine | 333.33 | 0.00 | 116,667 | 140,056 |
Ovarian | 400.00 | 0.00 | 140,000 | 168,067 |
Papillary thyroid tumour | 7.52 | 0.00 | 2632 | 3159 |
Paediatric high-grade glioma | 23.27 | 1.03 | 361 | 433 |
Paediatric melanoma | 11.10 | 1.01 | 355 | 426 |
Prostate | 400.00 | 0.00 | 140,000 | 168,067 |
Renal cell carcinoma | 400.00 | 0.00 | 140,000 | 168,067 |
Salivary gland | 58.14 | 0.00 | 20,349 | 24,428 |
Secretory breast carcinoma | 1.09 | 1.00 | 218 | 262 |
Sinonasal adenocarcinoma | 400.00 | 0.00 | 140,000 | 168,067 |
Uterine | 1000.00 | 0.00 | 350,000 | 420,168 |
Tumour type | NNS | Incremental cost to identify one patient (£) | ICER (£) | |
---|---|---|---|---|
First line | Confirmatory | |||
Tumours in the trial | ||||
Appendix | 30.83 | 1.04 | 8071 | 9689 |
Breast | 1761.49 | 3.46 | 1213 | 1456 |
Cholangiocarcinoma | 1233.05 | 2.72 | 309,215 | 371,206 |
Colorectal | 1027.54 | 2.44 | 853 | 1024 |
GIST | 96.33 | 1.13 | 24,480 | 29,387 |
IFS | 1.36 | 1.00 | 350 | 420 |
MASC | 1.33 | 1.00 | 483 | 580 |
Melanoma | 587.16 | 1.82 | 637 | 765 |
NSCLC | 1370.05 | 2.92 | 1021 | 1225 |
Pancreatic | 474.25 | 1.66 | 119,144 | 143,030 |
Thyroid | 220.19 | 1.31 | 457 | 549 |
Soft tissue sarcoma | 134.03 | 1.19 | 415 | 498 |
Tumours not represented in the trial | ||||
Cervix | 373.65 | 1.52 | 93,945 | 112,779 |
Congenital mesoblastic nephroma | 2.03 | 1.00 | 350 | 421 |
Gastro–oesophageal junction | 1233.05 | 2.72 | 309,215 | 371,206 |
HNSCC | 324.49 | 1.45 | 618,081 | 741,994 |
High-grade glioma | 2466.09 | 4.45 | 81,630 | 97,995 |
Neuroendocrine | 411.02 | 1.57 | 103,305 | 124,015 |
Ovarian | 493.22 | 1.69 | 591 | 710 |
Papillary thyroid tumour | 9.27 | 1.01 | 361 | 433 |
Paediatric high-grade glioma | 23.27 | 1.03 | 355 | 426 |
Paediatric melanoma | 11.10 | 1.01 | 354 | 425 |
Prostate | 493.22 | 1.69 | 123,896 | 148,734 |
Renal cell carcinoma | 493.22 | 1.69 | 123,896 | 148,734 |
Salivary gland | 71.69 | 1.10 | 18,307 | 21,977 |
Secretory breast carcinoma | 1.34 | 1.00 | 485 | 582 |
Sinonasal adenocarcinoma | 493.22 | 1.69 | 123,896 | 148,734 |
Uterine | 1233.05 | 2.72 | 309,215 | 371,206 |
Hierarchical approach: treating at diagnosis
For the tumour types represented in the trial, the tumour-specific costs to identify one eligible patient ranged from £0 (MASC) to £351,567 (breast cancer). Assuming that the eligible population is distributed in line with the trial distribution, the average incremental cost of testing is £64,198. Re-weighting the tumour-specific costs so that the tumour types included in the trial align with the expected prevalence of these tumours in the real world increases this to £107,030. However, this does not account for the testing costs associated with tumours that are not represented in the larotrectinib trial. When these are included and appropriately weighted in line with the prevalence of these tumour types, the average incremental cost to identify one individual eligible for treatment is £85,502. Based on this cost estimate and assuming that larotrectinib generates an additional 0.833 incremental QALYs, the ICER is estimated to be £102,644 per QALY gained, with tumour-specific ICERs ranging from less than £500 per QALY gained to over £500,000 per QALY gained.
First-line ribonucleic acid-based next-generation sequencing: treating at diagnosis
The incremental cost of testing to identify one individual eligible for TRK inhibitors using first-line RNA-based NGS is higher across tumour types than the cost of the hierarchical approach (with the exception of the tumours for which WGS is available). For the tumours included in the larotrectinib trial, the incremental testing costs range from £215 (MASC) to £500,000 (breast cancer). Based on the trial distribution, the average incremental cost to identify one eligible patient is £91,213. Using the real-world distribution, this increases substantially to £151,967 per patient identified. When including the unrepresented tumour types, the average incremental costs fall slightly to £121,321 per patient identified. If larotrectinib were to provide an incremental clinical benefit of 0.833 QALYs, and assuming zero treatment costs, these testing costs would imply an ICER of £145,643 per QALY gained.
Exhaustive approach: treating at diagnosis
Under the most exhaustive approach, in which DNA-based NGS is used as a first-line test followed by confirmatory NGS, the incremental costs of testing are lower than that for the other two strategies because a significant proportion of the costs for some tumour types are currently reimbursed. The incremental cost associated with identifying an individual who is eligible for a TRK inhibitor is lowest in IFS (£350). Given that there is no genomic testing currently available for patients with cholangiocarcinoma, identifying one patient eligible for a TRK inhibitor is the most costly (£309,215) within the trial population. Based on the distribution of tumour types within the trial, the average incremental testing cost associated with identifying one individual eligible for treatment is £15,252. Under the real-world distribution, this increases to £19,245 per patient identified. The average incremental cost to identify one individual eligible for treatment, including the tumour types unrepresented in the trial, is £53,480. The associated ICER was £64,202 per QALY gained.
Exhaustive approach: treating at line of therapy
Table 22 summarises the number of individuals who would need to be tested to identify one eligible patient using an exhaustive testing strategy carried out at diagnosis of advanced or metastatic cancer and treatment with larotrectinib provided in the appropriate position in the pathway. The incremental cost associated with testing and hypothetical estimates of cost-effectiveness of testing are also presented. The results show that the average incremental testing cost to identify one individual eligible for treatment is higher than when treatment is offered as first-line therapy. The annual population eligible for treatment is lower (n = 109); thus, the average incremental cost of testing to identify one individual eligible for treatment is estimated to be £113,424.
Tumour type | Proportion eligible based on treating at line of therapy (%) | Incremental cost to identify NTRK fusion patient (£) | ICER |
---|---|---|---|
Tumours in the trial | |||
Appendix | 30 | 26,903 | 32,297 |
Breast | 30 | 4042 | 4852 |
Cholangiocarcinoma | 30 | 1,030,717 | 1,237,355 |
Colorectal | 30 | 2843 | 3413 |
GIST | 30 | 81,598 | 97,957 |
IFS | 90 | 389 | 467 |
MASC | 60 | 805 | 966 |
Melanoma | 30 | 2124 | 2549 |
NSCLC | 60 | 1701 | 2042 |
Pancreatic | 30 | 397,146 | 476,766 |
Soft tissue sarcoma | 90 | 508 | 610 |
Thyroid | 30 | 1384 | 1661 |
Tumours not represented in the trial | |||
Cervix | 30 | 313,150 | 375,930 |
Congenital mesoblastic nephroma | 30 | 1168 | 1402 |
Gastro–oesophageal junction | 30 | 1,030,717 | 1,237,355 |
HNSCC | 30 | 2,060,269 | 2,473,312 |
High-grade glioma | 60 | 136,050 | 163,325 |
Neuroendocrine | 30 | 344,349 | 413,384 |
Ovarian | 30 | 1970 | 2365 |
Papillary thyroid tumour | 30 | 1203 | 1444 |
Paediatric high-grade glioma | 30 | 1183 | 1420 |
Paediatric melanoma | 30 | 1180 | 1416 |
Prostate | 30 | 412,985 | 495,781 |
Renal cell carcinoma | 30 | 412,985 | 495,781 |
Salivary gland | 30 | 61,022 | 73,256 |
Secretory breast carcinoma | 60 | 808 | 969 |
Sinonasal adenocarcinoma | 30 | 412,985 | 495,781 |
Uterine | 30 | 1,030,717 | 1,237,355 |
Implications for the cost-effectiveness of TRK inhibitors
The costs associated with additional testing for targets are likely to be substantial and will have a significant bearing on the cost-effectiveness of histology-independent technologies. Tumour-specific costs of identifying relevant fusions are also likely to represent a significant source of heterogeneity owing to the variable frequency of targets across tumour types. This heterogeneity in testing costs is likely to mean that for some tumour types for which NTRK fusions are rare, NTRK testing will not be cost-effective. Opportunities to share testing costs across multiple health-care technologies may reduce the cost burden of molecular testing on a specific health-care technology, potentially increasing the financial viability of testing. However, it is currently unclear what mechanism would be used to share testing costs across multiple technologies.
Model structure and extrapolation
Partitioned survival modelling (PSM) is the most common modelling approach used for NICE appraisals of interventions for advanced or metastatic cancers. 184 This approach uses survival analysis of observed TTE end points to derive state membership estimates. Given that estimates of mean survival times are required for cost-effectiveness analysis, parametric models are fitted to the observed TTE end points to extrapolate the observed survival data over an appropriate time horizon. The choice of appropriate parametric models to extrapolate the observed data is usually based on a series of assessments, including visual inspection of the Kaplan–Meier curves and log-cumulative hazard plots; visual fit of extrapolated models to observed data; statistical fit based on goodness-of-fit statistics; and clinical plausibility of the extrapolation. 185 In a PSM, PFS and OS are usually extrapolated independently and directly inform the state membership for the ‘Progression-free’ and ‘Death’ states over time, respectively. The difference between PFS and OS allows the proportion of patients in the progressed health state to be estimated.
The use of PSM presents several challenges for the assessment of histology-independent products. First, the more heterogeneous overall population may make it more challenging to fit a single conventional parametric curve. Second, the immaturity of the PFS and OS data will result in considerable uncertainty surrounding the extrapolated curves. This may lead to wide variation in the resulting predictions for survival models that have similar goodness of fit to the observed data. Each of these challenges is now considered in more detail.
Standard parametric models can include proportional hazard-based models (exponential, Weibull and Gompertz) and the accelerated failure time models (log-normal, log-logistic and generalised gamma). However, the additional heterogeneity arising from the inclusion of different tumour sites is likely to result in more complex hazard functions, which may not be appropriately captured using standard parametric distributions. Consequently, the use of flexible parametric models, mixture models or response-based models may be required. 186
Flexible parametric approaches directly model the effect of time on the hazard function using splines. The splines are used to form a series of polynomial distributions joined by ‘knots’. Changes in the modelled hazard function at specific time points can be accommodated by using different polynomial distributions between each knot. The number of knots determines the number of parameters required to model the hazard function. In a simple case with zero knots, these models are the same as conventional parametric distributions. These approaches provide greater flexibility than conventional parametric modelling. However, they also present potential challenges for histology-independent appraisals. First, the approach captures heterogeneity via the effect of time on the hazard function. This may not be appropriate for achieving accurate projections of PFS and OS where the main source of heterogeneity is the difference in the natural history between different tumour sites. Second, the flexible parametric approach extrapolates beyond the data using only the final segment of the curve. The small number of patients and the immaturity of the PFS and OS data may mean that survival projections are particularly unreliable in the final segment.
Although the impact of the inclusion of tumour sites that may have different natural histories on the hazard function might be accommodated by flexible parametric approaches, the inclusion of multiple subsets of patients may provide evidence of different survival distributions within the observed TTE data. Parametric mixture models can be used to capture heterogeneity within a population by using two (or more) distinct distributions. Although the use of a mixture model may provide a more appropriate approach to capture between-tumour heterogeneity, there may remain challenges in determining how many mixes are appropriate and whether or not the predicted long-term hazards are plausible from the resulting mixture. There also remain issues regarding the application of different HRQoL or cost estimates for individual tumour sites, as the different mixture distributions are not explicitly assigned to any individual tumour site or grouping. Hence, although mixture models provide an approach to account for heterogeneity within the TTE end points, they do not provide a basis for accounting for heterogeneity in other inputs, which may affect cost-effectiveness estimates.
Another approach that has been proposed to account for heterogeneity in TTE end points is the use of response-based landmark models. This approach models survival conditional on response status, identified at a predefined response evaluation landmark time based on a clinical definition of response. Survival is modelled from the landmark point to avoid the problem of ‘immortal time’ bias arising from the fact that responders have to survive to the point at which response is assessed. Separate survival curves are then fitted to the different response categories. Intuitively, this approach appears to be particularly aligned to the appraisal of histology-independent technologies where response measures are used as the primary end point. This approach allows for a distinction to be made between the HRQoL of responders and HRQoL of non-responders (and between individual tumour sites), as well as allowing for potential differences in the costs of care. However, there may be challenges in determining whether or not a single landmark time point is appropriate and how uncertainty around this should be dealt with. Although different response time points can be accommodated within the survival analysis using a time-varying covariate, inevitably this will increase the complexity of the economic model and may require individual patient simulation approaches. There also remain issues concerning the potentially small number of patients recruited in the underpinning studies and the immaturity of the PFS and OS data. Further subdividing patients into responder categories may result in more uncertain survival predictions. In addition, although separate survival curves may better account for heterogeneity within the survival data, the approach does not resolve the fundamental problem of immaturity in these end points.
Although several approaches exist to account for heterogeneity in survival end points, they all have several important limitations in the context of histology-independent appraisals. First, the use of single ‘full population’ ICERs, across multiple tumour sites with potentially different treatment effectiveness, comparators, costs and HRQoL, will be difficult to interpret. A single ICER may conceal significant variation in the tumour-specific ICERs, driven by a combination of factors, including the observable variability in the relative effectiveness between tumour types. Ignoring these differences could mean that a treatment that is not cost-effective for the total population (combining all subgroups) may be cost-effective in specific subgroups. Conversely, a treatment that appears cost-effective for the total population may not be cost-effective for particular subgroups. Given the amount of heterogeneity associated with a histology-independent appraisal, estimating the average cost-effectiveness for the full patient population covered in the scope may not provide enough information to decision-makers about whether or not the drug is potentially cost-effective across all subgroups.
Second, the approaches rely on extrapolations of the observed survival data, which will potentially be immature at the time of initial appraisal such that the resulting predictions will be highly uncertain. Different survival models that appear to fit the observed data equally well may lead to significant variation in the longer-term survival predictions. Consequently, it is unlikely that a single survival distribution (or a single specification of a more flexible parametric, mixture model or response-based approach) will adequately characterise uncertainties over the longer-term extrapolation period. To more formally account for the uncertainty surrounding choice of survival distribution, a model averaging approach may be required. 187,188 This approach involves the parameterisation of uncertainty surrounding the choice of distribution, incorporating all plausible distributions as part of a weighted distribution. Uncertainty in the probabilistic analysis will then reflect both the parametric uncertainty associated within each distribution and the uncertainty surrounding the choice of preferred method. However, such an approach presents additional challenges in the context of histology-independent appraisals, for which the external validation of survival projections from a heterogeneous population including multiple tumour types will be difficult and expert elicitation may be required to determine the weights to be applied as part of any weighted distribution. Furthermore, this heterogeneity will also result in the ‘at-risk’ population changing over time. That is, tumour types with poorer prognosis will experience events earlier than patients with a more favourable prognosis. Hence, the composition of the population will probably change significantly over the extrapolation period. This limits the appropriateness of applying a single ‘average’ utility or cost to the population within the model.
The greater immaturity in PFS/OS for trials that are powered on response end points may present challenges to fitting reliable survival distributions. In these circumstances, surrogate relationships may be required to link response-based outcomes (e.g. ORR and DoR) to longer-term estimates of PFS and/or OS. Although Chapter 4 highlighted a range of alternative approaches that could be used, the lack of any clear pattern by cancer type inevitably presents challenges for using a surrogate-based modelling approach to a model that includes a heterogeneous mix of patients.
Given the importance of exploring the impact of heterogeneity more explicitly for decision-making, approaches are required that can accommodate different sources of heterogeneity within the overall population, more appropriately estimate the average cost-effectiveness for the full patient population covered in the scope and facilitate assessment of whether or not the drug is potentially cost-effective across all subgroups. The BHM framework provides an important approach that can more fully explore the potential heterogeneity in effects across tumours. The BHM allows assessments to be made for each tumour type, as well as a pooled assessment across all tumour types, accounting for the potential lack of uniformity of effect across tumours. An additional advantage of this framework is the ability to predict the response probability that would be expected in a ‘new’ tumour type (i.e. a tumour that is not represented in the trial data), which will give a measure of the uncertainty in the response rates in tumour types in the target population but for which no data are available (see Treatment effectiveness).
Heterogeneity in TTE outcomes (PFS and OS) can be explored using the BHM in a similar way to that presented for response outcomes. 46 The model assumes a common parametric distribution for each tumour type, but with a different location parameter. Information on this parameter can be borrowed across the different tumours, according to an estimated heterogeneity parameter. The results from this model would be different distributions of PFS or OS for each tumour type, which could be incorporated in the economic model to further explore how heterogeneity in outcomes by tumour type influences the expected ICERs. Although the BHM can borrow information across tumour types and is designed to allow inferences with few events per tumour type, it is unclear whether or not this type of model would provide useful results given the immaturity of the survival data, the small number of patients for most tumour types, the expected lack of exchangeability of the survival outcomes and the potential for requiring informative prior distribution.
To address concerns regarding the maturity of the TTE end points, BHM could be applied to specific landmark survival time points (e.g. 6 or 12 months) for which more robust data exist, with surrogate relationships employed to predict longer-term survival conditional on survival up to these specific time points. Alternatively, BHM could be applied to the response data, for which fewer observations are required on response outcomes to draw meaningful conclusions about differences between tumour types. These response assessments could then be applied to conditional PFS and OS distributions from the overall population or be linked to external surrogate relationships. However, as reported in Chapter 4, the use of external surrogate relationships would require the use of surrogate multivariable statistical models to estimate the final outcome (OS/PFS), which may not specifically relate to the different tumours or a specific biomarker population.
Although such an approach is less desirable than having robust TTE data for the overall population and each specific subgroup of interest, it may provide a basis for the initial explorations of the potential impact and importance of heterogeneity. This would appear more appropriate than ignoring this heterogeneity within initial assessments. Importantly, such assessments may also help to guide further data collection and prioritise specific subgroups for which existing evidence may be scarce and/or for which these exploratory analyses indicate potentially important impacts on the likely cost-effectiveness of a new treatment within the full population.
Summary and implications
The previous sections identified a number of particular challenges for histology-independent appraisals and have explored alternative approaches that might be used to investigate and account for different sources of heterogeneity and uncertainty. Although not comprehensive, we have focused on areas of evidence and analysis that are anticipated to be the most challenging for the appraisal of histology-independent products. Given the nature of these challenges, it is likely that a range of alternative approaches will be required to address different sources of heterogeneity. The implications for the assessments of cost-effectiveness and uncertainty will also need to be made explicit. Equally important is the need to ensure that these assessments present the results in a manner that can help to inform NICE decisions, both in determining the appropriateness of different recommendations and in identifying key uncertainties that might be used to inform and prioritise the value of further data collection.
Chapter 7 presents a potential framework that could be used to inform approval and research policies for histology-independent products, including NICE decision-making and CDF data collection arrangements.
Chapter 7 A decision framework to inform approval and research policies for histology-independent technologies
An exemplar case study was developed to illustrate the nature of the assessments that could be used to evaluate the cost-effectiveness of a new histology-independent treatment. Based on these assessments, a framework is proposed to help to inform approval and research policies for histology-independent technologies. A brief summary of the case study is presented in the following section. Further details are reported separately in Appendix 12.
Exemplar case study
The case study considers a hypothetical TRK inhibitor (‘Drug X’) compared with the current SoC for the treatment of solid tumours that harbour a NTRK gene fusion. Although the case study draws on clinical evidence from an existing TRK inhibitor, specifically the response outcomes and the BHM reported in Chapter 6, Treatment effectiveness, for larotrectinib, all other inputs are based on stylised assumptions. Importantly, the purpose of the case study is not to make any recommendations concerning the likely cost-effectiveness of any existing or new histology-independent treatment. Instead, the aim is to illustrate the nature and sequence of assessments that could potentially be used to help to inform NICE approval decisions and CDF data collection arrangements.
The economic model uses a landmark response-based structure (see Chapter 6, Model structure and extrapolation) that incorporates separate PFS and OS distributions, conditioned on response status in the overall study population. That is, the same conditional PFS and OS distributions that are assumed for responders and non-responders are applied to each individual histology. The use of conditional PFS and OS data, therefore, assumes a perfect surrogate relationship between response outcomes and PFS and OS end points, which is the same across all tumour types. Hence, heterogeneity in PFS and OS across individual histologies is assumed in the case study to be entirely mediated through different response rates.
The use of a response-based modelling approach necessitates additional assumptions compared with a situation in which robust TTE data are available for the overall population and each specific subgroup of interest. Equally, there may be a range of alternative modelling approaches that could be developed based on landmark survival times and/or alternative surrogate relationships. The purpose of the case study is not to make specific recommendations regarding the model structure and associated parameter assumptions, but to present a more general framework to demonstrate how heterogeneity within the overall population could potentially be explored within a cost-effectiveness analysis and how the results could be presented to inform alternative policy decisions more appropriately. However, it should be acknowledged that assessments of heterogeneity in survival outcomes at the point of initial marketing authorisation may be challenging unless these are linked to a surrogate outcome (e.g. response and DoR), for which more robust assessments of heterogeneity are likely to be feasible.
The model structure consists of three mutually exclusive health states: (1) progression-free disease, (2) progressed disease and (3) death. State occupancy in the model is derived using a dual-partitioned survival approach that uses PFS curves to partition OS into those patients who are progression-free and those who have progressed disease, based on response status at a specific landmark time point.
Survival for Drug X is calculated as a weighted average of the responder and non-responder survival curves based on the ORR assumed in the analysis. Survival in the SoC arm was modelled assuming a 0% response. The case study, therefore, also makes a strong assumption that effectiveness for SoC management is the same across all tumour types and is equal to the conditional PFS and OS estimates derived from non-responders to Drug X.
In line with the NICE reference case, the model considers a NHS and Personal Social Services perspective in terms of capturing costs and QALYs, and discounts both using a 3.5% discount rate. Results are presented over a lifetime (30-year) time horizon.
The response rates used in the analysis were based on the BHM analysis of the larotrectinib FDA data (see Table 13). By linking the BHM estimates for response rates to the conditional OS and PFS estimates, the case study model explores the implications for cost-effectiveness of heterogeneity in the overall population by considering individual histology-specific estimates of cost-effectiveness alongside estimates for the overall population.
Stylised input parameters were used for all other economic model parameters and are summarised in Table 23. The acquisition cost for Drug X in the case study was assumed to be priced at a level such that the ICER in the overall population would be close to the upper limit of NICE’s end-of-life threshold range (circa £50,000 per QALY gained). Given that a number of separate scenarios are presented in the case study, the estimate of the acquisition cost was derived from the scenario that was considered to best represent a base-case scenario. This scenario included testing costs and estimates of the effectiveness in tumour sites that were not represented in the clinical evidence base. For a more detailed description of the underlying assumptions and justification for the parameters, see Appendix 13.
Parameter | Value (95% CI) |
---|---|
Effectiveness (months)a | |
Median PFS | |
Responders | 24 (21.6 to 26.4) |
Non-responders | 6 (5.4 to 6.6) |
Median OS | |
Responders | 36 (32.4 to 39.6) |
Non-responders | 12 (10.8 to 13.2) |
Utilities | |
Progression-free | |
Drug X | 0.79 (0.71 to 0.87) |
SoC | 0.72 (0.65 to 0.79) |
Post progression | |
Drug X | 0.64 (0.57 to 0.71) |
SoC | 0.64 (0.57 to 0.71) |
Costs (£) (per month) | |
Drug acquisition costs | |
Drug X | 1250 (–) |
SoC | 20 (–) |
Health state costsb | |
Progression free | 350 (315 to 385) |
Post progression | 500 (450 to 550) |
Terminal care cost | 6878 (one-off cost) (–) |
The model results are based on a probabilistic sensitivity analysis (PSA), which was implemented using 10,000 samples.
Histology-specific incremental cost-effective ratios and overall cost-effectiveness
The case study starts with an assessment of cost-effectiveness based on the trial population and excludes testing costs. Issues around the generalisability of the trial population and the impact of including testing costs are then explored.
Table 24 presents the mean total costs, QALYs and ICERs associated with the histology-independent technology (Drug X) and SoC for each histology included in the trial. Mean survival with SoC is < 2 years for all individual histologies and Drug X is expected to increase life expectancy by > 3 months. This suggests that the end-of-life criteria has been met and, therefore, the ICER for all histologies should be compared with a maximum threshold of £50,000 per additional QALY. 4
Subgroup | Per-patient level | ICER (£) | |
---|---|---|---|
Cost (£) | QALYs | ||
Sarcoma | |||
Drug X | 61,314 | 2.70 | 27,520 |
SoC | 14,471 | 0.99 | – |
Salivary | |||
Drug X | 58,697 | 2.58 | 27,969 |
SoC | 14,471 | 0.99 | – |
IFS | |||
Drug X | 63,332 | 2.79 | 27,213 |
SoC | 14,471 | 0.99 | – |
Thyroid | |||
Drug X | 62,615 | 2.76 | 27,318 |
SoC | 14,471 | 0.99 | – |
Lung | |||
Drug X | 55,032 | 2.41 | 28,721 |
SoC | 14,471 | 0.99 | – |
Melanoma | |||
Drug X | 46,963 | 2.03 | 31,267 |
SoC | 14,471 | 0.99 | – |
Colon | |||
Drug X | 38,667 | 1.65 | 36,857 |
SoC | 14,471 | 0.99 | – |
GIST | |||
Drug X | 61,234 | 2.69 | 27,535 |
SoC | 14,471 | 0.99 | – |
Cholangiocarcinoma | |||
Drug X | 34,261 | 1.45 | 43,658 |
SoC | 14,471 | 0.99 | – |
Appendix | |||
Drug X | 37,773 | 1.61 | 37,859 |
SoC | 14,471 | 0.99 | – |
Breast | |||
Drug X | 37,768 | 1.61 | 37,863 |
SoC | 14,471 | 0.99 | – |
Pancreas | |||
Drug X | 37,751 | 1.61 | 37,930 |
SoC | 14,471 | 0.99 | – |
The ICERs estimated for the individual histologies range from £27,213 to £37,930 per QALY gained. The large differences in response rates, ranging between 29.9% and 93.3%, appear to have only a moderate effect on the ICER estimates reported across individual histology sites. The reason for this is that the overall cost of Drug X is assumed to be closely related to the expected survival outcomes of treatment, specifically the duration of PFS. As the response rate increases (or decreases), the duration of treatment also increases (or decreases), such that the total cost of the treatment is closely related to the expected survival outcomes. For treatment regimens that are given for a fixed duration, as opposed to a treat-until-disease-progression (or unacceptable toxicity) strategy, the impact of heterogeneity in the response data would be expected to have a greater impact on the ICER estimates across individual histologies. Similarly, in situations in which heterogeneity in the surrogate relationship is also evident across tumour sites, a greater impact on the ICER estimates across individual histologies would be expected.
A ‘histology-independent’ recommendation is defined here as the approval of Drug X for use in any histology that exhibits the specific biomarker (e.g. NTRK). If a histology-independent approval is sought, it is necessary to consider the ‘average’ or ‘pooled’ ICER across all histologies. Table 25 illustrates how a pooled ICER is calculated with the frequency of each histology based on the relative histology frequency observed in the trial (see Table 14).
Subgroup | Observed outcomes | Weighted consequences | |||
---|---|---|---|---|---|
ΔCost (£) | ΔQALYs | Frequency (%) | ΔCost (£) | ΔQALYs | |
Sarcoma | 46,844 | 1.70 | 20.00 | 9369 | 0.34 |
Salivary gland | 44,227 | 1.58 | 21.82 | 9649 | 0.35 |
IFS | 48,861 | 1.80 | 12.73 | 6219 | 0.23 |
Thyroid | 48,144 | 1.76 | 9.09 | 4377 | 0.16 |
Lung | 40,561 | 1.41 | 7.27 | 2950 | 0.10 |
Melanoma | 32,492 | 1.04 | 7.27 | 2363 | 0.08 |
Colon | 24,197 | 0.66 | 7.27 | 1760 | 0.05 |
GIST | 46,763 | 1.70 | 5.45 | 2551 | 0.09 |
Cholangiocarcinoma | 19,791 | 0.45 | 3.64 | 720 | 0.02 |
Appendix | 23,302 | 0.62 | 1.82 | 424 | 0.01 |
Breast | 23,297 | 0.62 | 1.82 | 424 | 0.01 |
Pancreas | 23,280 | 0.61 | 1.82 | 423 | 0.01 |
Total | 41,227 | 1.44 | |||
Pooled ICER | £28,573 |
This analysis illustrates that the pooled cost-effectiveness of Drug X depends on the frequency and distribution of the individual histologies. The frequency of histologies in the target population will ultimately be determined by the testing strategy implemented in clinical practice. Depending on the testing strategy and the expected distribution of histologies in the target population, the pooled ICER may alter. For example, evidence on the expected prevalence of NTRK fusions in specific histologies suggests that the distribution of histologies expected in clinical practice may differ significantly from that observed in the trial.
Table 26 shows the relative frequency of histologies expected in clinical practice, assuming routine screening of all histologies. The table also illustrates how the pooled ICER can change based on differences in the expected distribution of histologies in the target population compared with those observed within the trial.
Subgroup | Observed outcomes | Weighted consequences | |||
---|---|---|---|---|---|
ΔCost (£) | ΔQALYs | Frequency (%) | ΔCost (£) | ΔQALYs | |
Sarcoma | 46,844 | 1.70 | 3.93 | 1840 | 0.07 |
Salivary gland | 44,227 | 1.58 | 1.80 | 796 | 0.03 |
IFS | 48,861 | 1.80 | 21.89 | 10,693 | 0.39 |
Thyroid | 48,144 | 1.76 | 5.01 | 2412 | 0.09 |
Lung | 40,561 | 1.41 | 13.37 | 5424 | 0.19 |
Melanoma | 32,492 | 1.04 | 2.08 | 675 | 0.02 |
Colon | 24,197 | 0.66 | 18.39 | 4450 | 0.12 |
GIST | 46,763 | 1.70 | 3.01 | 1407 | 0.05 |
Cholangiocarcinoma | 19,791 | 0.45 | 0.27 | 53 | 0.00 |
Appendix | 23,302 | 0.62 | 12.78 | 2978 | 0.08 |
Breast | 23,297 | 0.62 | 3.87 | 902 | 0.02 |
Pancreas | 23,280 | 0.61 | 13.61 | 3169 | 0.08 |
Total | 34,798 | 1.15 | |||
ICER | £30,364 |
The pooled ICER based on the distribution of histologies expected in clinical practice is £30,364 per QALY. This is marginally higher than the estimate based on the distribution of tumour sites reported in the trial data. The evidence indicates that more common tumour sites, such as colon and pancreas, that have low frequency of NTRK fusions may be under-represented in the trial population and, conversely, certain rarer tumour sites with high frequency of NTRK fusions are potentially over-represented (e.g. sarcoma and salivary gland).
This example illustrates the importance of understanding the frequency of histologies expected in the target population and the necessity of modelling histology-specific cost and health consequences. When the expected distribution of histologies is expected to differ between the trial and the target population, failure to account for this could result in a biased estimate of the pooled ICER. The magnitude of any bias will depend on the extent of heterogeneity in relevant model inputs between tumour sites.
Screening to identify eligible patients
The previous analyses did not include the costs of identifying the population of patients with the biomarker of interest. However, a variety of tests and testing strategies may be required to identify eligible patients. As a result, the cost of patient identification may vary significantly across histologies. Indeed, even if homogeneity in all other model inputs is assumed, the cost-effectiveness estimates will inevitably vary based on differences in the costs of identifying patients with the specific biomarker. Consequently, when evaluating the overall cost-effectiveness of a technology, it is necessary to consider the joint costs and benefits of the testing/treatment strategy. 189,190
Table 27 updates the previous results by including an arbitrary per-patient testing cost of £50. The results clearly demonstrate that even a small per-patient testing cost can result in significant variation in the ICER estimates across the individual histologies.
Subgroup | Per-patient level | Frequency of mutation (%) | NNS (n) | Cost of testing (£50 per test) (£) | Cost (including testing) (%) | ICER (£) | |
---|---|---|---|---|---|---|---|
Cost (excluding testing) (£) | QALYs | ||||||
Sarcoma | |||||||
Drug X | 61,314 | 2.70 | 0.56 | 178.57 | 8929 | 70,243 | 32,765 |
SoC | 14,471 | 0.99 | – | – | 14,471 | – | |
Salivary | |||||||
Drug X | 58,697 | 2.58 | 92.90 | 1.08 | 54 | 58,751 | 28,003 |
SoC | 14,471 | 0.99 | – | – | 14,471 | – | |
IFS | |||||||
Drug X | 63,332 | 2.79 | 90.90 | 1.10 | 55 | 63,387 | 27,244 |
SoC | 14,471 | 0.99 | – | – | 14,471 | – | |
Thyroid | |||||||
Drug X | 62,615 | 2.76 | 0.92 | 108.70 | 5435 | 68,049 | 30,402 |
SoC | 14,471 | 0.99 | – | – | 14,471 | – | |
Lung | |||||||
Drug X | 55,032 | 2.41 | 0.09 | 1111.11 | 55,556 | 110,588 | 68,060 |
SoC | 14,471 | 0.99 | – | – | 14,471 | – | |
Melanoma | |||||||
Drug X | 46,963 | 2.03 | 0.21 | 476.19 | 23,810 | 70,773 | 54,178 |
SoC | 14,471 | 0.99 | – | – | 14,471 | – | |
Colon | |||||||
Drug X | 38,667 | 1.65 | 0.12 | 833.33 | 41,667 | 80,334 | 100,326 |
SoC | 14,471 | 0.99 | – | – | 14,471 | – | |
GIST | |||||||
Drug X | 61,234 | 2.69 | 1.28 | 78.13 | 3906 | 65,140 | 29,836 |
SoC | 14,471 | 0.99 | – | – | 14,471 | – | |
Cholangiocarcinoma | |||||||
Drug X | 34,261 | 1.45 | 0.10 | 1000.00 | 50,000 | 84,261 | 153,956 |
SoC | 14,471 | 0.99 | – | – | 14,471 | – | |
Appendix | |||||||
Drug X | 37,773 | 1.61 | 4.00 | 25.00 | 1250 | 39,023 | 39,889 |
SoC | 14,471 | 0.99 | – | – | 14,471 | – | |
Breast | |||||||
Drug X | 37,768 | 1.61 | 0.07 | 1428.57 | 71,429 | 109,196 | 153,952 |
SoC | 14,471 | 0.99 | – | – | 14,471 | – | |
Pancreas | |||||||
Drug X | 37,751 | 1.61 | 0.07 | 1428.57 | 71,429 | 109,180 | 154,304 |
SoC | 14,471 | 0.99 | – | – | 14,471 | – |
Tumour-specific costs of identifying biomarker-positive patients are also likely to represent a significant source of heterogeneity owing to the variable frequency of targets across tumour types. This is evident in the individual ICER estimates, which now show much greater variation across different tumour sites than the previous analysis, which excluded per-patient testing costs.
The key variable driving the testing costs and the ICER estimates for the test/treat strategy is the NNS. For now, we assume that the test is perfect: it correctly classifies all individuals as having or not having the mutation (i.e. there are no false positives or false negatives). In this situation, the NNS is 1 divided by the expected frequency of the mutation in each histology. For histologies in which the mutation is very common (‘high frequency histologies’, for example salivary), there is a very small NNS because almost every person (92.9%) screened has the mutation. The opposite is the case for ‘low frequency histologies’ in which the mutation is rare. In pancreatic cancer, 1429 people (i.e. 1/0.07%) need to be screened to identify one individual with the mutation. A testing cost of £50 per test increases the overall costs of Drug X by £71,429, from £37,751 to £109,180 in pancreatic cancer. This increases the ICER from £37,930 to £154,304 in pancreatic cancer. The ICER for each histology has increased, but in the histologies with moderate to high frequency of mutation the increase in the ICER is more modest.
The above analysis assumes that the test (or testing strategy) is perfect; however, if this is not the case, this will result in patients being misclassified. Patients who do not have the mutation will be classified as having the mutation (false positives) and patients who have the mutation will be missed (false negatives). Such misclassifications may have important implications for costs and health. 149,190 The number of false positives and negatives will depend on the testing strategy, test characteristics (sensitively/specificity) and frequency of the mutation in each histology. 165,172,191 The possibility of misclassification presents two tasks: (1) calculating the correct ICER given a specific test or testing strategy, and (2) choosing the optimal test or testing strategy. Both of these tasks require estimates of the costs and QALYs associated with false positives and false negatives, which will probably differ by histology. For costly new treatments and those with significant side effects, false positives may have substantial consequences. The scale of consequences associated with false negatives will depend on the additional benefits of treatment. This means that the consequences of missing a potential patient (false negative) will be larger in those histologies in which the treatment results in larger QALY benefits.
The value of heterogeneity and population health
The preceding sections show how heterogeneity in treatment effectiveness and testing costs can be explored using pooled ICERs and individual histology ICERs. However, ICERs have an important limitation: they do not give an indication of the scale of consequences for population health. Understanding the benefits and costs of treatment at a population level will help to understand the consequences of decision-making in the presence of heterogeneity and uncertainty.
To understand the implications of heterogeneity for population health requires that benefits and costs are expressed in health or monetary equivalents, using net health benefits (NHBs) or net monetary benefits (NMBs). The same information used to provide ICER estimates can also be expressed as the per-patient NHB (or NMBs), which includes benefits, harms and NHS/Personal Social Services costs. 192–194 The NHB is the difference between any health gained with the intervention and the health forgone elsewhere in the health-care system (i.e. owing to the need to displace existing treatments and services to fund a new and more costly treatment), all expressed in QALY terms. NMB is equivalent, but everything is expressed in monetary terms.
Table 28 illustrates how NHB and NMB are calculated given an assumed threshold of £50,000 per QALY. Testing costs are now included in this analysis.
Subgroup | Per-patient level | £50,000 per QALY threshold | |||
---|---|---|---|---|---|
ΔCost (£) | ΔQALYs | Health forgone (ΔCost/£50,000) | NHB (ΔQALY – health forgone) | NMB (ΔQALYs × £50,000 – ΔCost) (£) | |
Sarcoma | 55,772 | 1.70 | 1.12 | 0.59 | 29,337 |
Salivary gland | 44,281 | 1.58 | 0.89 | 0.70 | 34,784 |
IFS | 48,916 | 1.80 | 0.98 | 0.82 | 40,859 |
Thyroid | 53,579 | 1.76 | 1.07 | 0.69 | 34,539 |
Lung | 96,117 | 1.41 | 1.92 | –0.51 | –25,505 |
Melanoma | 56,302 | 1.04 | 1.13 | –0.09 | –4342 |
Colon | 65,863 | 0.66 | 1.32 | –0.66 | –33,039 |
GIST | 50,670 | 1.70 | 1.01 | 0.68 | 34,245 |
Cholangiocarcinoma | 69,791 | 0.45 | 1.40 | –0.94 | –47,125 |
Appendix | 24,552 | 0.62 | 0.49 | 0.12 | 6223 |
Breast | 94,725 | 0.62 | 1.89 | –1.28 | –63,961 |
Pancreas | 94,709 | 0.61 | 1.89 | –1.28 | –64,020 |
For sarcoma, the additional per-patient cost of £55,772 can be represented as 1.12 QALYs (in NMB terms ≈ £55,572/£50,000) in health forgone elsewhere in the health system, based on a NICE threshold of £50,000 per QALY. This can then be compared with the additional benefits of 1.7 QALYs, resulting in an overall positive NHB of approximately 0.59 QALYs (≈ 1.7–1.12 QALYs) per person treated in this histology. Hence, for each sarcoma patient treated with Drug X, the overall gain to the health system is expected to be 0.59 QALYs per annum. However, for certain other histologies (e.g. colon), the additional health gained with Drug X is more than offset by health forgone elsewhere. This means that, for every colon cancer patient who receives Drug X, it is expected that 0.66 QALYs will be lost per annum elsewhere in the health system.
The advantage of NHBs and NMBs is that they can be used to help to understand the population-level consequences of alternative policy decisions. Understanding the scale of population consequences requires information on the number of patients who are expected to be treated by histology. This will depend on the incidence (number of new cases per year) and prevalence (number of current cases) of the mutation for each histology. It will also depend on the screening strategy used to identify cases and where in the treatment pathway Drug X is used. To simplify the case study, we assume only incident cases and a perfect screening strategy, which means that all patients who can potentially benefit are correctly identified. The expected population health consequences of approving Drug X are shown in Table 29.
Subgroup | Per patient level £50,000 per QALY threshold | Population level | ||
---|---|---|---|---|
Health forgone (ΔCost/£50,000) | NHB (ΔQALY – health forgone) | Incidence | NHB, QALYs (NMB, £) | |
Sarcoma | 1.12 | 0.59 | 5 | 2.88 (144,046) |
Salivary gland | 0.89 | 0.70 | 2 | 1.56 (78,201) |
IFS | 0.98 | 0.82 | 27 | 22.35 (1,117,570) |
Thyroid | 1.07 | 0.69 | 6 | 4.32 (216,219) |
Lung | 1.92 | –0.51 | 17 | –8.52 (–426,230) |
Melanoma | 1.13 | –0.09 | 3 | –0.23 (–11,276) |
Colon | 1.32 | –0.66 | 23 | –15.19 (–759,374) |
GIST | 1.01 | 0.68 | 4 | 2.57 (128,728) |
Cholangiocarcinoma | 1.40 | –0.94 | 0.3 | –0.31 (–15,727) |
Appendix | 0.49 | 0.12 | 16 | 1.99 (99,383) |
Breast | 1.89 | –1.28 | 5 | –6.19 (–309,616) |
Pancreas | 1.89 | –1.28 | 17 | –21.78 (–1,089,034) |
Total | 125 | –17 (–827,110) |
The number of patients with sarcoma who express the biomarker is approximately five per year. This means that treating identified sarcoma patients with Drug X is expected to result in a gain of 2.88 QALYs per year to the health system when compared with SoC. This contrasts with treating biomarker-positive patients who have colon or pancreatic cancer. Using Drug X in these populations is expected to result in a loss of 15.19 and 21.78 QALYs, respectively, per year.
By totalling the yearly NHB across all histologies, Table 29 shows that Drug X is expected to result in an overall loss of approximately 17 QALYs per year. This implies that a histology-independent approval for Drug X is not expected to be cost-effective. Although Drug X appears cost-effective in some individual histologies (e.g. sarcoma and IFS), the overall consequences of approving for all histologies would result in an overall annual loss of health to the health system. The analyses illustrate the importance of information on the relative frequency of histologies expected in the target population.
Histology-dependent recommendations and the value of heterogeneity
The assessments presented in Table 29 can also be used to compare the population consequences of making different policy recommendations. Decision-makers, such as NICE, have the option of different approval policies:
-
no stratification – histology-independent approval
-
partial stratification – approval in a clinically defined set of histologies
-
full stratification – approval only in histologies in which cost-effective is demonstrated.
These policies will determine the type of recommendations that are feasible and the relevant health consequences that need to be considered. The following section deals only with approval policies based on expected values, without addressing the impact of uncertainty in decision-making. Uncertainty, the need for further evidence and alternative mechanisms to reduce the risk of decision-making are considered in subsequent sections.
No stratification: histology-independent approval
This represents an ‘all or nothing’ approval policy in which the intervention is approved for all histologies or for none. There is no stratification of decision-making by histology. In this case, the relevant metric is the pooled ICER (or pooled NHB/NMB equivalent) across all histologies. Based on the results shown in Table 29, Drug X would not be approved for use in any histology because the pooled NHB is negative (correspondingly, the pooled ICER would be higher than the £50,000 per QALY threshold). The health system is expected to lose approximately 17 QALYs per year if Drug X was granted a histology-independent approval.
However, a further consideration when making histology-independent decisions is that some histologies, which may harbour the mutation of interest, may not be directly observed in the evidence base at the time of decision-making, despite the inclusion/exclusion criteria of the trial permitting their inclusion. Given that these patients may be treated in clinical practice, consideration should be given to the potential impact of considering histologies that are not represented in the trial data. The larger the incidence of unrepresented NTRK fusion-positive histologies, the greater the influence this can have on decision-making. In this case study, it is estimated that there are 151 NTRK fusion-positive cases in the set of unrepresented histologies each year (see Table 16). This is a larger number than the 125 cases in the observed set of histologies represented in the trial and should be explicitly considered if a histology-independent approval is sought.
If, as in this case study, the economic model is developed around the probability or degree of response in each histology and a BHM has been used to analyse response, the predictive distribution could be used to estimate response in the unrepresented histologies. This assumes that the effects for the unrepresented histologies are exchangeable with the observed histologies. For Drug X, the predictive distribution for response in unrepresented histologies has a mean response probability of 57% and is highly uncertain, with a 95% CrI ranging from 1% to 100%. If estimates for the remaining parameters (e.g. quality of life and testing costs) can be sourced from the literature or generalised from the observed histologies, ICER and NHB estimates can also be estimated for unrepresented histologies.
The results shown in Table 30 include the impact of including unrepresented histologies. To simplify the case study, we collapse all unpresented histologies into one ‘unrepresented’ histology category. The response probability comes from the predictive distribution from the BHM; costs and quality of life are assumed to be the same across all observed and unobserved histologies. The average testing costs for unrepresented histologies were estimated to be £14,322 per patient tested. This estimate was based on observational data on NTRK fusion prevalence (see Appendix 12).
Subgroup | Per-patient level, £50,000 per QALY threshold | Population level | ||
---|---|---|---|---|
Health forgone (ΔCost/£50,000) | NHB (ΔQALY – health forgone) | Incidence | NHB, QALYs (NMB, £) | |
Sarcoma | 1.12 | 0.59 | 5 | 2.88 (144,046) |
Salivary gland | 0.89 | 0.70 | 2 | 1.56 (78,201) |
IFS | 0.98 | 0.82 | 27 | 22.35 (1,117,570) |
Thyroid | 1.07 | 0.69 | 6 | 4.32 (216,219) |
Lung | 1.92 | –0.51 | 17 | –8.52 (–426,230) |
Melanoma | 1.13 | –0.09 | 3 | –0.23 (–11,276) |
Colon | 1.32 | –0.66 | 23 | –15.19 (–759,374) |
GIST | 1.01 | 0.68 | 4 | 2.57 (128,728) |
Cholangiocarcinoma | 1.40 | –0.94 | 0.3 | –0.31 (–15,727) |
Appendix | 0.49 | 0.12 | 16 | 1.99 (99,383) |
Breast | 1.89 | –1.28 | 5 | –6.19 (–309,616) |
Pancreas | 1.89 | –1.28 | 17 | –21.78 (–1,089,034) |
Unrepresented | 0.97 | 0.15 | 151 | 22.33 (1,116,748) |
Total | 276 | 5.79 (289,638) |
After taking account of the unrepresented histologies, Drug X is now estimated to be cost-effective in the overall population with positive NHBs. In this example, a histology-independent approval, including an assessment of the potential impact of unrepresented tumour sites, would result in an expected overall gain to the health system of approximately 5.79 QALYs (NMB ≈ £290,000) per year. Treating individuals with histologies that are unrepresented in the trial data is expected to result in positive NHB, given the assumptions made here. This is because of the relatively high mean response rate (57%) predicted by the BHM.
Although it may be challenging to identify data to inform benefits in unrepresented histologies, consideration to the magnitude and potential impact of these histologies should be explicitly considered.
Partial stratification: approval in a defined set of histologies
This is similar to the previous approval policy; however, in this case, the intervention is approved only for a clinically defined set of histologies, that is there is partial stratification of decision-making by histology. The relevant metric here is the pooled ICER (or pooled NHB equivalent) for the defined subset of histologies. The basis for selecting a subset of histologies can be based on theoretical and/or empirical grounds. For example, Chapter 2, European Medicines Agency review of approved histology-independent indications, highlighted comments from the SAG to the EMA about larotrectinib that appeared to differentiate the strength of the biological rationale and the available clinical evidence for several specific tumour types (e.g. IFS, salivary gland/MASC, congenital mesoblastic nephroma and GIST). For these specific tumour types, the SAG concluded that efficacy has been established in the absence of available treatments of proven efficacy in terms of convincing clinical efficacy end points and that clinical decisions to use larotrectinib were justified.
To illustrate the implications of a policy decision based on partial stratification, we assume that there is sufficient ground to consider restricting an approval decision for Drug X to only those patients with IFS, salivary gland and GISTs. Evidence for patients with congenital mesoblastic nephroma was not available at the time of the FDA assessment; therefore, these patients are not included in the data used to inform the BHM.
As shown in Table 31, a decision to approve Drug X in only these three individual histologies is expected to result in an overall annual gain to the health system of 26.49 QALYs. Although partial stratification results in fewer patients receiving Drug X than in a full histology-independent approval (i.e. 33 patients annually vs. 276 patients), there would be an overall gain to the health system from a policy decision based on partial stratification. This gain is equivalent to approximately 20.7 QALYs per annum. In other words, a policy to fully approve a histology-independent product could result in an annual loss of 20.7 QALYs to the health system compared with an optimised approval decision based on a partial stratification approach.
Subgroup | Per-patient level £50,000 per QALY threshold | Population level | ||
---|---|---|---|---|
Health forgone (ΔCost/£50,000) | NHB, QALYs (NMB, £) | Incidence | NHB, QALYs (NMB, £) | |
Salivary gland | 0.89 | 0.70 (34,800) | 2 | 1.56 (78,200) |
IFS | 0.98 | 0.82 (40,850) | 27 | 22.35 (1,117,600) |
GIST | 1.01 | 0.68 (34,250) | 4 | 2.57 (128,700) |
Total | 33 | 26.49 (1,324,500) |
The majority of the gains from partial stratification are achieved by avoiding the approval of Drug X in histologies with high testing costs and relatively high incidence (e.g. lung, colon, breast and pancreatic cancer), for which Drug X does not appear to be cost-effective based on current evidence. A further advantage of partial stratification over no stratification is that assumptions about unrepresented histologies can be avoided in decision-making. However, a disadvantage of partial stratification is a potential increase in monitoring costs required to prevent the use of Drug X outside its subset of approved histologies. 151
Full stratification: approval only in histologies in which cost-effectiveness is demonstrated
This is a fully histology-dependent approval policy in which the technology is restricted for use only in those histologies in which it has been shown to be potentially cost-effective based on expected ICER/NHB estimates. Given the ICER/NHB estimates presented in Table 29, Drug X appears to be potentially cost-effective in the following histologies: sarcoma, salivary gland, IFS, thyroid, GIST and appendix. These are the histologies in which NHBs are greater than zero. Equivalently, they each have ICERs below £50,000 per QALY gained. Taking the sum of the NHB across each of these histologies results in an overall annual gain of 35.68 QALYs to the health system from a fully stratified approval decision for Drug X. The expected number of patients treated annually based on full stratification is estimated to be 60.
The additional value of distinguishing between different types of patients represents the VoH. 150–152 In this example, the VoH represents the difference between the NHB of a fully stratified recommendation and a histology-independent recommendation with no stratification. This difference is equivalent to 29.89 QALYs per year.
Exploring the VoH may help to inform NICE committees of the consequences of alternative policy options, in terms of both the expected number of patients who would be eligible to receive a specific new treatment and their overall consequences to the health system. Although a histology-independent approval might be considered appropriate on the basis that this results in an overall positive annual NHB compared with rejecting the technology, it is also important to consider the potential consequences of such an approval policy compared with a more restrictive or optimised recommendation. In this case study, there appear to be significant gains to the health system that could be achieved by an optimised recommendation. Importantly, an approval decision based on partial stratification using only three individual histologies appears to confer approximately 74% of the gains that are potentially achieved based on a full stratification policy.
Uncertainty and decision-making
Decisions about the approval of technologies were discussed in Histology-specific incremental cost-effective ratios and overall cost-effectiveness. However, decision-makers, such as NICE, also need to consider the risk associated with decision-making under uncertainty. Given the limitations in study design and sample size, there will always be uncertainties about the cost and health consequences associated with different treatment options. All ICERs and NHBs discussed previously will be associated with uncertainty. This means that, although the central estimate of the ICER/NHB indicates that a treatment is cost-effective, there is also a risk that the treatment is not cost-effective. For example, a treatment that meets the end-of-life criteria may have a central ICER estimate of £45,000 per QALY and, therefore, is expected to be cost-effective. However, owing to uncertainty, there may be a 40% chance that the true ICER is above £50,000 per QALY. The health losses associated with this eventuality are the risk of decision-making under uncertainty.
Uncertainties can be divided into two categories: those that arise from assumptions inherent in constructing models (structural uncertainties) and those that are a result of imprecision in parameter estimates owing to limited sample size (parameter uncertainties). Previous research has shown how uncertainties associated with imprecision can be addressed through further data collection or pricing schemes. 194,195 In this section, we will show how these approaches can be used to reduce risk in decision-making for histology-independent technologies. In addition, we show how stratified decision-making represents an additional approach to managing risk associated with uncertainty.
The consequences of uncertainty
This section introduces value of information (VOI) as a framework to quantify the health effects of uncertainty. VOI analyses can provide decision-makers with metrics to help to understand the drivers of decision uncertainty and assess alternative strategies that could be used to manage this risk. The uncertainty associated with a histology-independent decision (‘no stratification’) is illustrated below. The implications for partial and full stratification will be addressed in subsequent sections.
The NHB results previously reported in Table 30 are illustrated graphically in Figure 11. In addition, uncertainty around the expected (mean) estimates of NHB are represented using a 95% CI. This is computed from the mean and 95% percentiles of the PSA for each histology. Figure 11 also plots the patient-level pooled NHB. This is analogous to the pooled ICER reported in Tables 25 and 26. The pooled NHB is a weighted average of the NHB associated with different histologies. Weights come from the incidence of NTRK-positive histologies reported in Table 30, with a perfect screening strategy assumed.
FIGURE 11.
Net health benefits per person across histologies with uncertainty. Positive numbers favour the intervention (drug) compared with usual care alone.
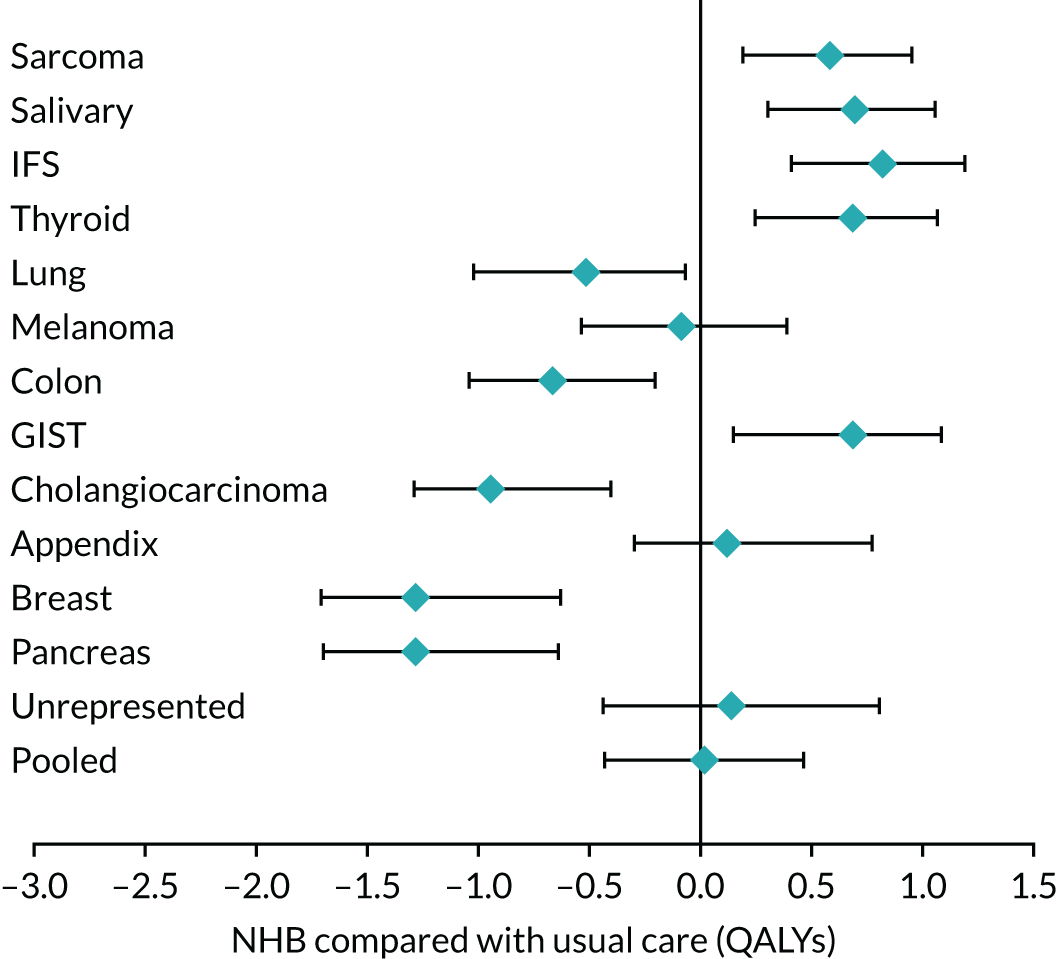
Figure 11 shows that Drug X is expected to result in additional NHB in sarcoma, salivary, IFS, thyroid and GISTs, with the 95% CI not crossing the line of equivalence with SoC. It is also expected to be cost-effective in the appendix and in those histologies that are unrepresented in the trial, but this is uncertain. This uncertainty can be expressed in terms of the likelihood or probability that Drug X is not cost-effective compared with SoC (i.e. 47% and 39% in appendix and unrepresented cancers, respectively). For lung, melanoma, colon, cholangiocarcinoma, breast and pancreatic cancer, the model estimates that there is approximately 0% chance that Drug X is cost-effective.
As in the previous analysis, the pooled population represents the expected consequences of a histology-independent recommendation. Drug X is expected to result in 0.02 additional QALYs per person treated and there is a 52% chance that it is cost-effective compared with SoC. The pooled estimate relies on the relative incidence of histologies in the target population. Although uncertainty in histology incidence is not addressed quantitatively in this case study, this can be propagated through the PSA in the same manner as other uncertainties.
The health system consequences of decision-making can be better informed with reference to population health. Figure 12 shows the population NHB for each histology and the pooled NHB. This is calculated by multiplying the per-person NHB for each group illustrated in Figure 11 by the incidence for each group (see Table 30).
FIGURE 12.
Population net health effects across histologies with uncertainty. Positive numbers favour the intervention (drug) compared with usual care alone.
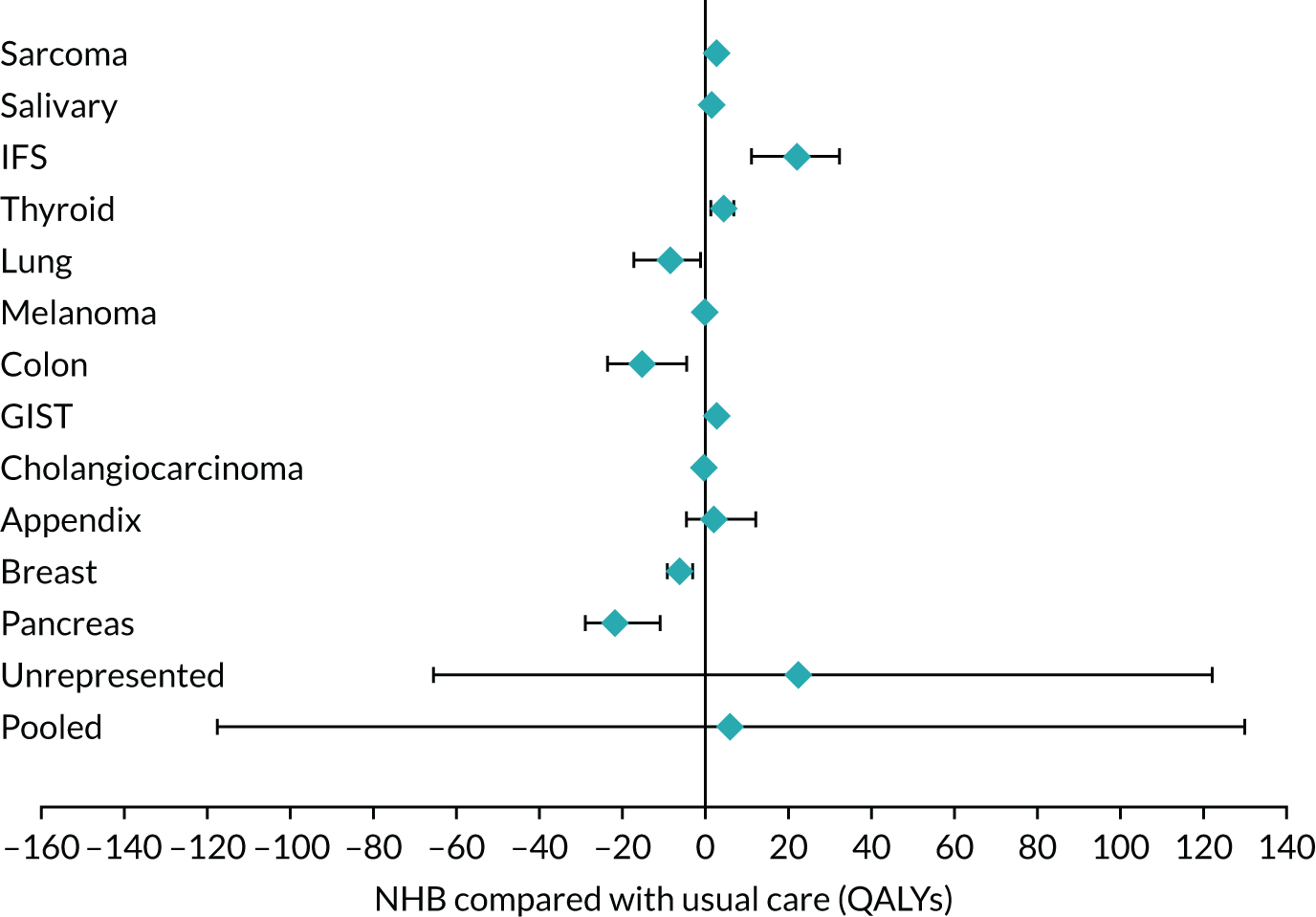
Figure 12 shows that, although uncertainty in per-person NHB may be similar across histologies, the consequences of approval and uncertainty vary substantially when the size of populations are taken into account. The figure shows that for many histologies (e.g. sarcoma, salivary, melanoma and breast), the health consequences of approval and/or uncertainty are limited owing to their small population. By contrast, the health consequences associated with the unrepresented histologies are relatively large, as decisions in this group affect 151 individuals each year.
The pooled category represents the health consequences of the ‘no stratification’ approval policy (i.e. a histology-independent approval). A histology-independent approval is expected to result in a gain of 5.79 QALYs per year, on average (consistent with Table 30). However, the 95% CI indicates that this is highly uncertain, with approval potentially resulting in losses of up to 120 QALYs per year (illustrated in Figure 12 by the lower CI, which extends to –120). The following section will describe how VOI methods can be used to quantify the health consequences of this uncertainty to help inform decision-making and approval policies.
Quantifying the health consequences of uncertainty
Histology-independent decision-making (no stratification) is concerned with making approval decisions based on pooled cost-effectiveness estimates. From Figure 12, Drug X is expected to provide an expected benefit of (0.02 × 276 ≈) 5.79 QALYs per year at the pooled population level. However, there is uncertainty about this benefit. VOI methods can be used to quantify the health consequences of uncertainty, that is the risk associated with decision-making with current information. 193,196,197 Uncertainty matters because it means that there is a chance of making the wrong decision. Quantifying the expected health consequences of uncertainty is achieved by multiplying the chance of making a wrong decision by the health consequences of making the wrong decision. This is illustrated in Figure 13.
FIGURE 13.
Estimating the health consequences of uncertainty.
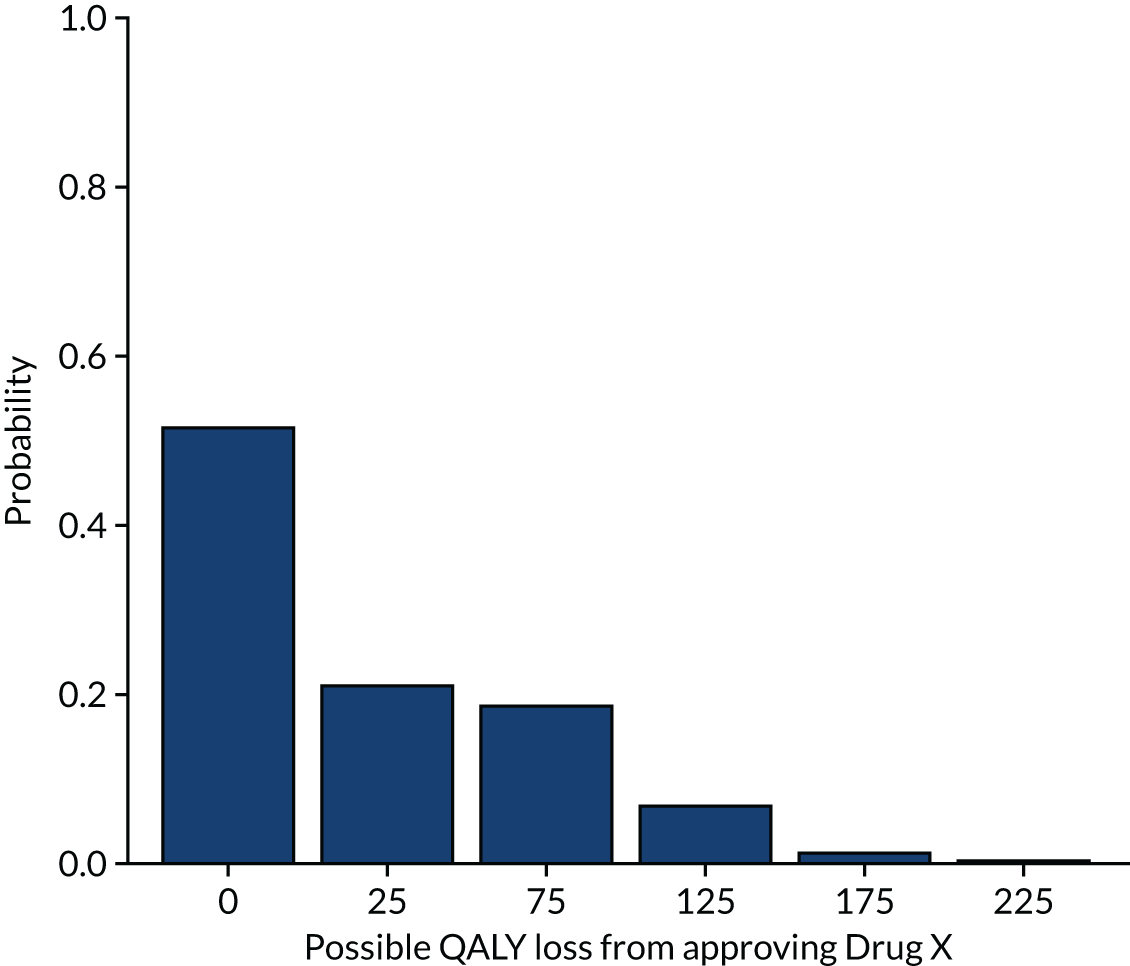
If Drug X is more cost-effective than SoC in the pooled population, there are zero health consequences of uncertainty. The tall left-hand bar in Figure 13 shows that there is estimated to be a 52% chance that Drug X is cost-effective in the pooled population. This corresponds to a 52% chance of zero consequences of uncertainty.
Making an incorrect decision (e.g. approving Drug X when it is not cost-effective) will have health consequences. For the pooled population, there is a 48% chance that the decision to approve Drug X is incorrect. As shown in Figure 13, these health consequences are not uniform. There is a greater chance of more limited consequences than a smaller chance of greater consequences. Figure 13 shows that there is a 21% chance of Drug X resulting in a loss of 25 QALYs per year (second bar from the left). There is a 19% chance of a loss of 75 QALYs per year (third bar from the left), and so on. The weighted average over this range of outcomes provides an estimate of the health consequences of uncertainty. This is estimated to be 29.52 QALYs per year, equivalent to approximately 0.108 QALYs per person.
This quantitative approach to the risks associated with uncertainty can be used to assess policy options that address this risk. In the following sections, we will illustrate three approaches to managing this risk: further data collection, pricing agreements and stratified decision-making.
Managing risk through further data collection
Further data collection is one approach to reduce risk associated with uncertainty. The imprecision in parameter estimates owing to limited sample size (e.g. OS) can be reduced by collecting data on these parameters. 198
Decisions about further data collection are important because under current policy arrangements when NICE is unable to approve a technology for routine use owing to parameter uncertainties it may recommend it for inclusion into the CDF if it is eligible. 199 Topics that are eligible for the CDF are reimbursed for a time-limited duration following the development of a managed access agreement (MAA). The MAA consists of (1) a data collection agreement (DCA), which specifies the data that must be collected that could sufficiently resolve the parameter uncertainties identified by the appraisal committee, and (2) a commercial access agreement, which ensures that the technology is reimbursed at a cost-effective price during the period of the MAA. A technology remains in the CDF until the data collection agreed in the DCA is complete; it then proceeds to reappraisal and exits the fund.
The MAA covers the entire eligible population determined by the NICE guidance, which means that entry to the CDF is equivalent to an ‘approval with research’ decision, which is reassessed after the data collection period (usually 2 years). 199,200 The assessments required to inform the suitability of an ‘approval with research’ decision over ‘only in research’, approve and reject are covered in detail elsewhere. 197 Explicit consideration of these assessments could aid the transparency of CDF entry requirements. However, it is beyond the scope of this report to suggest reforms to CDF processes or to determine the appropriate size of the CDF budget. These issues have been commented on elsewhere and require further research. 200–202
The aim here is to provide a framework to understand how the CDF, in its current form, can help to address the risk associated with histology-independent technologies. The intention is to demonstrate how a unified decision framework could enable CDF data collection arrangements to be considered alongside other risk reduction strategies (e.g. pricing schemes and stratified decision-making).
Decision uncertainty resolved by the Cancer Drugs Fund
Previously, the value of resolving all uncertainty was estimated to be 29.52 QALYs per year (NMB of ≈ £1.48M); therefore, this is an upper bound for the risk, which can be resolved through further research each year. However, there are many sources of uncertainty in any model, for example uncertainties in baseline risks, health-state costs and HRQoL. Different types of research will potentially be required to inform different model parameters. For example, observational survey research may be sufficient to address uncertainties about HRQoL in specific disease states, whereas randomised research may be required to resolve uncertainties in the relative effects of interventions. 198 Research on particular parameters will resolve more or less uncertainty depending on how central these parameters are to the decision between the treatment alternatives. This means that research on some parameters is more valuable than research on others.
The upper bound for the value of additional research on specific parameters (or set of parameters) can be calculated using an extension of VOI methods. These are called expected value of partial perfect information (EVPPI) methods. 197,203 To estimate the value of resolving uncertainty in a specific parameter, the EVPPI method estimates the payoff (in QALYs or GBP) from the clinical decision if the parameter of interest was known with certainty compared with the payoff if that parameter remained uncertain. The difference between these two scenarios is the EVPPI. This decomposes the overall upper bound for the value of research into the value of resolving uncertainty in specific parameters (or sets of parameters).
To illustrate the EVPPI analysis using the case study, consider the case in which only information on OS (for responders and non-responders) could be collected through CDF arrangements. This may be because of organisational or time constraints. Estimating EVPPI using the Gaussian process method suggested by Strong et al. ,203 the upper bound for the value of research on OS is 12.16 QALYs (NMB of ≈ £0.6M) per year. This can be compared with 29.52 QALYs per year (NMB of ≈ £1.48M), which is the value of resolving the uncertainty associated with all parameters in the model. EVPPI methods provide a more accurate assessment of the risk that can be resolved with particular data collection strategies. This same approach can be applied to any uncertainties that are parameterised in a decision model. As shown in Tables 24 and 25, the distribution of histologies in practice can influence the cost-effectiveness of Drug X when making histology-independent recommendations. If uncertainty about the distribution of histologies can be parameterised, EVPPI methods can be used to understand the value of research to resolve these uncertainties.
These methods can be used to help to prioritise data collection. Although the CDF financial resource constraint is softened by the expenditure control mechanism, the real resources required to co-ordinate and quality control data collection are limited. 199 In the case where high-quality data on certain parameters are challenging to collect through the CDF, EVPPI methods can be used to understand the risk that can be resolved by collecting data on these parameters. This can be used to determine (1) whether or not there is any value in collecting data on a specific parameter; (2) whether or not the benefits of the additional information are sufficient to justify the additional costs of collecting the data; and (3) whether or not other approaches, such as pricing schemes or stratification, would be more appropriate to resolve the decision risks.
The EVPPI methods are an important extension to VOI analysis in decision-making. However, EVPPI estimates are still upper bounds for the value of additional research on individual parameters. This is because EVPPI assumes that uncertainty in the parameter of interest is completely resolved, that is it is the value of research if an infinite sample size was collected. Expected value of sample information methods relax this assumption by assessing the value of commissioning research with finite sample sizes. 198,204,205
Managing risk through pricing schemes
The NICE process allows for consideration of a variety of pricing schemes, including patient access schemes, commercial access agreements and flexible pricing. 206 These schemes can facilitate pricing arrangements, such as simple discounts or more complex ‘pay-for-performance’ arrangements. In this section, we illustrate the effect of a simple discount and a pay-for-performance scheme on uncertainty and the expected value of a technology.
Simple discounts have been identified in previous research as an effective approach for payers to reduce the risk of approving technologies that are not cost-effective. 194,195,200 Reducing the price of a technology that is expected to be cost-effective has two implications: (1) the value of implementing the technology will increase owing to the resources saved and (2) the risk of the technology not being cost-effective will decrease. Under the current price (£1250 per month), a histology-independent recommendation for Drug X is expected to result in 5.79 additional QALYs per year. However, owing to uncertainty in parameters, there is also a 48% chance that Drug X is not cost-effective. As shown previously, the expected health consequences of this uncertainty have been estimated to be 29.52 QALYs per year.
With a 10% simple discount (£1125 per month), the expected value of Drug X is estimated to increase to 21.4 QALYs per year. Furthermore, the risk of Drug X not being cost-effective is reduced to 42%. The potentially negative health consequences associated with the uncertainty are reduced to 23.91 QALYs per year (a reduction in risk of 5.61 QALYs). A 20% simple discount (£1000 per month) increases the expected value of Drug X to 30.04 QALYs per year and reduces the consequences of uncertainty to 21.3 QALYs per year (a reduction in risk of 8.22 QALYs).
To illustrate the use of more complex pricing schemes, we also implemented a pay-for-performance scheme. In this scenario, the undiscounted cost of Drug X (£1250 per month) is incurred only if a patient responds to treatment. This has two impacts on risk and cost-effectiveness. First, this acts in a similar manner to a price discount because the average response is expected to be approximately 60% according to the BHM (i.e. reducing the effective price by 40%). Second, the risk associated with Drug X not resulting in the expected outcomes is now shifted from the payer to the company. 194 These two impacts reduce the health consequences of uncertainty to the health system. With this pricing scheme, the expected value of Drug X increases to 51.95 QALYs per year and reduces the consequences of uncertainty (i.e. the expected risk) to 9.35 QALYs per year (a reduction in risk of 20.17 QALYs).
The examples here illustrate how alternative pricing approaches can be used to increase the expected value of a technology, as well as impacting the risk and consequences associated with uncertainty.
Managing risk through stratified decision-making
The previous discussion described how uncertainty can be addressed when making histology-independent decisions in which the technology is expected to be used across all histologies (no stratification). In this section, we discuss how to apply these same principles under partial and full stratification.
Partial stratification
The previous sections described how uncertainty and associated risk could be managed through further data collection and pricing schemes. Both assumed that the health technology would be approved for use in all histologies or none (i.e. histology-independent approval decisions). In this section, we discuss stratification as an additional approach to reducing risk in approval decisions for products with a histology-independent marketing authorisation.
In the case of partial stratification, the intervention is approved only for a clinically defined set of histologies. The relevant metric for decision-making is the pooled NHB for the subset of histologies of interest. Therefore, it is the uncertainty in this pooled NHB that is of relevance to decision-making. Figure 14 illustrates the uncertainty in pooled NHB for the approval of Drug X in IFS, salivary gland and GISTs only.
FIGURE 14.
Population net health effects with uncertainty for partially stratified decision-making. Positive numbers favour the intervention (drug) compared with usual care alone.
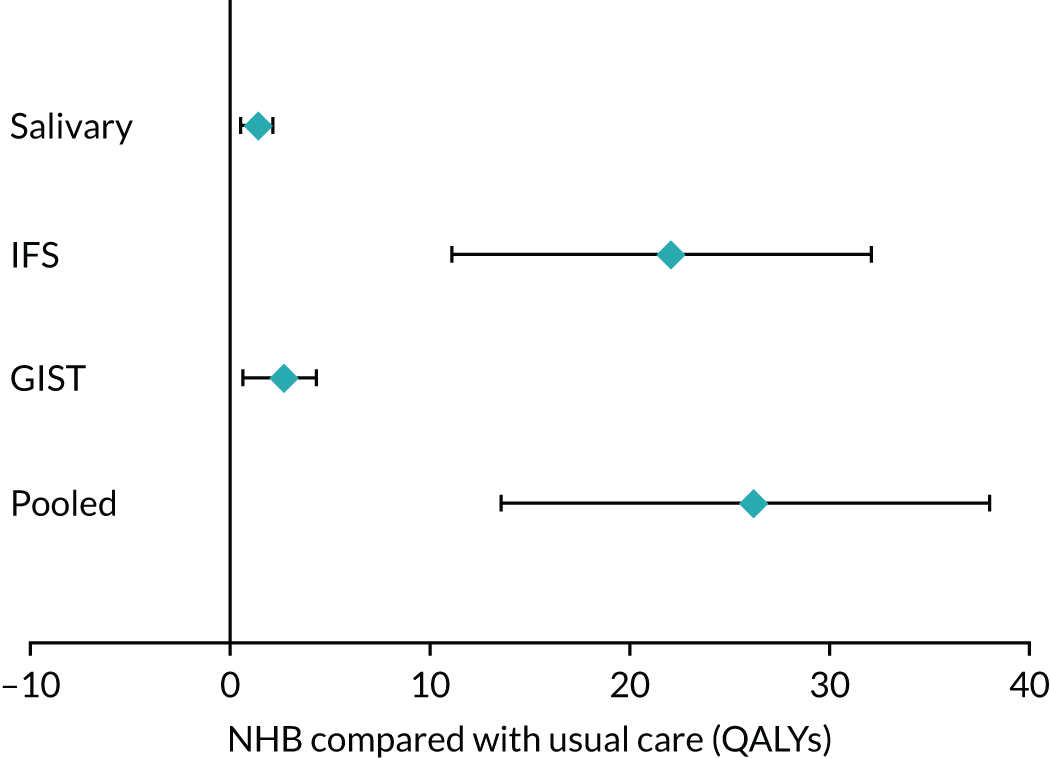
Figure 14 shows that Drug X is expected to provide positive NHB in each of IFS, salivary gland and GISTs individually. Implementing Drug X in this subset is expected to result in approximately 26.5 additional QALYs per year over SoC (this corresponds to Table 31). Figure 14 graphically represents the uncertainty in this estimate. The 95% CI for the pooled effect is far from the line of equivalence between Drug X and SoC, indicating that there is not much uncertainty in cost-effectiveness in this subset of histologies. Given the model assumptions, it is estimated that there is now a 0% risk that Drug X is not cost-effective. This means that the risk in approving Drug X has been eliminated, without the need to carry out additional research or wait for research to report. For this reason, a routine commissioning decision may be considered appropriate for this specific subset of tumour sites.
Full stratification
Under full stratification, there is the option to make different decisions for different histologies. This is a fully histology-dependent approval policy in which the technology is restricted for use only in those histologies that it has been shown to be cost-effective. Figure 15 illustrates the population-level uncertainties in making fully stratified recommendations.
FIGURE 15.
Population net health effects with uncertainty for fully stratified decision-making. Positive numbers favour the intervention (drug) compared with usual care alone.
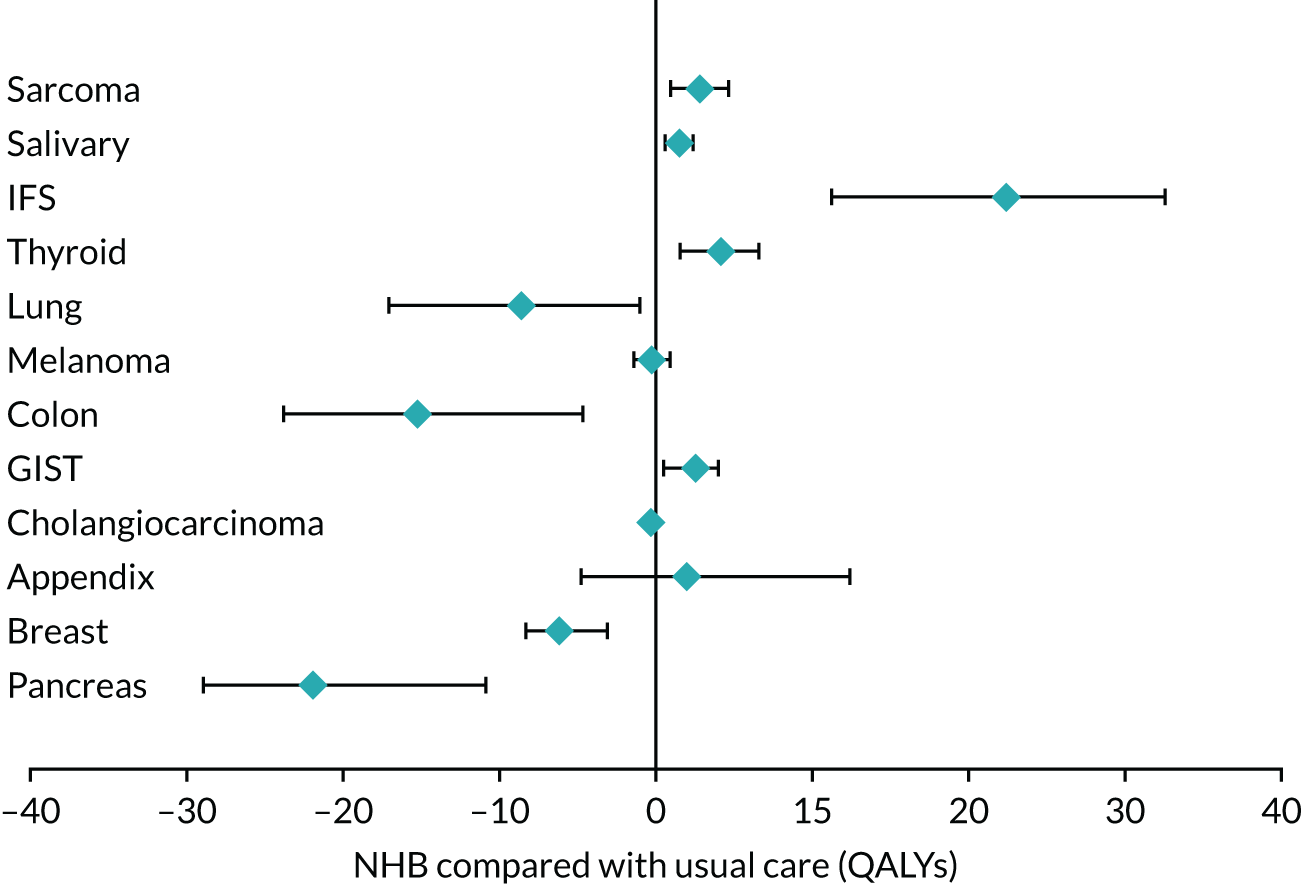
Approval in sarcoma, salivary gland, IFS, thyroid, GIST and appendix cancers is expected to provide a positive NHB for all. Approval of Drug X in the remaining histologies is expected to result in a loss of population health. Figure 15 also shows that uncertainty about health benefits (or losses) differs across histologies. The 95% uncertainty bounds cross the line of equivalence for melanoma and appendix cancers only.
Because separate approval decisions can be made for each histology, the risk associated with decision-making should be estimated for each histology separately. The risk associated with uncertainty in each histology is reported in Table 32, along with the expected annual value of approving the treatment for each histology.
Subgroup | Population-level £50,000 per QALY threshold | ||
---|---|---|---|
Incidence | Health impact of uncertainty per year, QALYs (NMB, £) | Health impact of approval per year, QALYs (NMB, £) | |
Sarcoma | 5 | 0.02 (1216) | 2.88 (144,046) |
Salivary gland | 2 | 0 (113) | 1.56 (78,201) |
IFS | 27 | 0.01 (577) | 22.35 (1,117,570) |
Thyroid | 6 | 0.01 (665) | 4.32 (216,219) |
Lung | 17 | 0.03 (1398) | –8.52 (–426,230) |
Melanoma | 3 | 0.23 (9950) | –0.23 (–11,276) |
Colon | 23 | 0.02 (1045) | –15.19 (–759,374) |
GIST | 4 | 0.01 (407) | 2.57 (128,728) |
Cholangiocarcinoma | 0 | 0 (2) | –0.31 (–15,727) |
Appendix | 16 | 1.1 (55,078) | 1.99 (99,383) |
Breast | 5 | 0 (0) | –6.19 (–309,616) |
Pancreas | 17 | 0 (0) | –21.78 (1,089,034) |
Total | 125 | 1.41 (70,452) |
Table 32 shows that, of the histologies included, the largest risks are associated with decisions about appendix and melanoma histologies. This is because these two histologies have uncertainty bounds in Figure 15 that cross the line of equivalence. Given that further research appears most valuable in these histologies, this may help to prioritise further data collection. EVPPI assessments can be applied to these histologies to understand the parameters that are driving uncertainty. For other histologies, it is clear that Drug X is cost-effective (e.g. IFS) or not cost-effective (e.g. pancreas) based on current evidence; therefore, there appears to be limited risk of making the wrong decision and little value in further research.
Table 32 also illustrates that the total risk associated with decision-making has reduced for stratified decision-making compared with no stratification. The expected health consequence of uncertainty was 29.52 QALYs per year for no stratification. This was zero for partial stratification and approximately 1.41 QALYs per year for full stratification (implying a reduction of 28.11 QALYs).
The change in uncertainty (as measured by VOI) from less stratification to more stratification has been called the ‘dynamic value of heterogeneity’ in the literature. 151 It should be noted that increasing stratification may increase or decrease the uncertainty in decision-making. When the characteristic that the treatment is being stratified by is important in explaining heterogeneity (such as histology in the case study), stratification will increase the value of implementing the treatment while reducing the risk of making an incorrect decision. This is because variability in outcomes is translated into heterogeneity. However, if a stratification characteristic contains little information to distinguish outcomes, uncertainty may increase with stratification owing to sample splitting. 151
Comparing approaches to risk management in histology-independent technologies
When making a histology-independent approval decision, with current evidence and without any discount, Drug X appeared cost-effective based on expected values but the health consequences of uncertainty were estimated to be 29.52 QALYs per year. Three approaches to risk management were explored: further data collection, pricing schemes and stratified decision-making.
The upper bound for the value of further data collection on OS was expected to be 12.16 QALYs; this is compared with a reduction in risk of 5.61 QALYs from a 10% price discount, 8.22 QALYs from a 20% discount, 20.17 QALYs from a pay-for-performance scheme and a reduction of 28.11 QALYs from stratification.
The magnitude of uncertainty resolved through data collection will depend on which parameters can be informed by feasible research. Owing to institutional or ethics constraints, data collection may not be possible for some parameters and this places limits on this approach to risk management.
As discussed previously, the degree of uncertainty resolved through stratifying by histology will depend on the importance of histologies in explaining heterogeneity in cost-effectiveness. In cases in which cost-effectiveness does not vary significantly across histologies, the risk reduction from stratification will be lower. There may also be additional costs associated with (partially or fully) stratified recommendations, for example the costs of monitoring clinician behaviour to ensure that treatments are not being used in histologies for which they do not have approval. 150,151 In principle, this cost can be incorporated into the analysis of alternative policy options; however, reliable data to predict these costs may be difficult to find. 207
When considering the impact of pricing schemes, the magnitude of risk reduction will depend on the pricing arrangement. 194 For simple discounts, the risk reduction will increase with the scale of the price reduction. Neither a 10% nor a 20% simple discount reduced the risk of approval as much as either further data collection or stratified decision-making in the case study. A pay-for-performance scheme reduced the risk more than further data collection, but not as much as stratified decision-making. It should be noted that, when comparing price reductions (or stratification) with data collection, it is important to take account of the fact that data collection takes time to report whereas the other risk management policies can theoretically begin immediately.
Pricing schemes and stratified decision-making can also increase the value of approving technologies in addition to addressing risk. A 10% and 20% discount increased the value of Drug X from 5.79 to 21.4 and 30.04 additional QALYs per year, respectively. Partial and full stratification increased this to 26.49 and 35.68 QALYs per year, respectively. The gain in value from stratification is a result of making more optimised decisions. This has been called the ‘static value of heterogeneity’ in the literature. 151 The pay-for-performance scheme increased the potential value of approval by the greatest extent. It resulted in an additional 51.95 QALYs per year from the approval of Drug X. This gain is mostly because of the substantial discount implied by the pay-for-performance scheme. Because these approaches to risk management (further data collection, pricing schemes and stratified decision-making) are not mutually exclusive, each one can be used in combination to address the risk of approving a technology that is not cost-effective. 195
Discussion
We have illustrated a framework for decision-making that takes account of uncertainty and heterogeneity associated with histology-independent technologies. The aim was to outline assessments that can help to support NICE and CDF decision-making, both in making approval decisions and in managing risks associated with uncertainties.
It is evident that heterogeneity in the cost-effectiveness of histology-independent technologies can arise from a number of sources and that these should be explicitly considered when making decisions. Even if clinical outcomes were identical across individual histologies, differences in the costs of identification can lead to important cost-effectiveness differences between individual histologies. In situations in which the target population is expected to differ from the trial population (i.e. in terms of the distribution of histology types), explicit modelling of heterogeneity will be required to support NICE decision-making. If any histologies exist that are unrepresented in the trial population, consideration will be required to the potential costs and health consequences in unrepresented histologies along with their frequency in the target population to support a histology-independent approval.
The framework explored the health consequences associated with three different approval policies: no stratification (histology-independent approval), partial stratification and full stratification. This demonstrated the potential health gains from making stratified decisions. As discussed above, modelling the costs and health consequences associated with heterogeneity will often be required to make histology-independent decisions. This means that the assessments and assumptions required for stratified decision-making will often be the same as those required for histology-independent decision-making. Furthermore, because partially and fully stratified decision-making allows for approval only in the subset of histologies for which there are observed data, these stratified approaches can be less dependent on strong assumptions. This is because they avoid the requirements to estimate ICERs/NHBs for unrepresented histologies.
The role of stratified decision-making was also illustrated as an approach to reducing the risk associated with uncertainty. This was compared with two other approaches to risk management: further data collection and pricing schemes. 195,196,204 This analysis showed that each approach can reduce the risk associated with uncertainty. Stratified decision-making was shown to be the most effective policy for risk reduction in the case study. The factors that determine the magnitude of uncertainty resolved by each approach were discussed and it was highlighted that these factors will differ across histology-independent technologies. The policy or combination of policies chosen in a specific scenario will depend on procedural feasibility and the characteristics of a given proposal.
Limitations of the analysis and directions for future research
A limitation of the analysis in this section is that ‘unrepresented histologies’ are included as a homogeneous group. In reality, there may be significant heterogeneity between different unrepresented histologies. The sections on stratified decision-making assumed that Drug X could be approved only in represented treatments. Theoretically, this need not be the case. If unrepresented histologies were not treated as a homogeneous group but were considered individually, it is likely that for some histologies Drug X would be expected to be cost-effective and for others it would not. The uncertainty surrounding each would also differ. If approval for individual unrepresented histologies was feasible, the decision uncertainty remaining after full stratification would be larger than reported in Managing risk through stratified decision-making. This is because the uncertainty reported for fully stratified decision-making (1.41 QALYs per year) considers uncertainty only in the represented histologies. Including uncertainty in unrepresented histologies would necessarily increase this.
For the sake of clearly illustrating the core principles of decision-making under uncertainty, other simplifying assumptions were made. Namely, one-off infrastructure costs, population prevalence and test uncertainty were not explicitly modelled; these assumptions should be relaxed in future research. 197
One-off infrastructure costs are relevant to calculate per-person testing costs. In the case study, we have assumed a one-off testing cost of £50 per individual tested. However, testing approaches based on NGS may require large up-front investments in infrastructure. A recommended approach to incorporate capital costs, such as testing infrastructure, is to divide the one-off expenditure by the total population of patients who are expected to use the infrastructure. 197,208 For histology-independent technologies, this includes individuals across a range of histologies and over the expected lifetime for the infrastructure. This has several potential important implications for decision-making.
The first is that any stratification of approval by histology will necessarily mean that testing costs will be spread over a smaller number of patients. This will have the effect of increasing per-person testing costs when treatments are approved for subsets of histologies, reducing the expected health gains associated with stratification. Second, if reimbursement decisions are changed before the end of the assumed lifetime of the one-off infrastructure investment and some proportion of these costs are not recoverable, this has important implications for decision-making under uncertainty. The presence of significant irrecoverable costs increases the costs associated with initially implementing then subsequently removing a technology from general use. Taking account of these costs will tend to favour more conservative approaches to decision-making, which demand less uncertainty before a treatment is approved for widespread use. 197 This has implications for the CDF because MAAs stipulate approval of technologies for the entire eligible population as determined by the NICE guidance alongside research. 199 Explicit consideration of significant irrecoverable costs in this context will make the costs of inclusion into the CDF more transparent.
A third implication of investment costs is that testing infrastructure, such as NGS, may provide a basis for the use of other health technologies that use the same infrastructure. This means that the population of patients who are expected to use the infrastructure extends across all treatments and indications expected to use the infrastructure.
A further simplification of the case study was that it was assumed that the test that was used to identify eligible patients was perfect, that is it results in zero false positives and zero false negatives. The reality of testing will differ in two ways: (1) the accuracy of a test may not be perfect and will, therefore, misclassify a certain proportion of patients and (2) the false-positive and false-negative rate will be estimated with uncertainty, meaning that the rate of misclassification may not be known with certainty. For point (1), the consequences of misclassification and the analytical approaches to deal with this have been discussed in Screening to identify eligible patients. For point (2), if uncertainty in the false-positive and false-negative rate can be parameterised, the health consequences of this uncertainty can be managed using the same EVPPI methods as illustrated in the case study.
The case study was also built on a simplified surrogate relationship between response and survival. Survival was assumed to be determined by response, and, conditional on response or non-response, it was assumed to be homogeneous across histologies. The aim of this model was to link heterogeneity in response to heterogeneity in costs and health outcomes. However, as discussed in Chapter 4, the relationship between response and survival is highly uncertain, variable and may be very weak. Further research is required to better inform how surrogate outcomes, such as response, can be linked to costs and health outcomes.
Comparing approaches to risk management in histology-independent technologies compared data collection, pricing schemes and stratified decision-making as alternative approaches to manage risk and increase the health impact of decision-making. Considering the full range of options has important implications for price negotiations in histology-independent technologies. The health impacts of stratified decision-making could be used as a benchmark in negotiating discounts required for histology-independent approval. For example, to obtain a histology-independent approval, the reimbursement decision-maker could require a pricing scheme sufficient to reduce risk to the level that would exist under stratified decision-making. Any approval policy will create a specific set of incentives for research and pricing strategy. 209,210 Further research is required to understand the incentives provided by current arrangements and the potential benefits of changes to policy.
Finally, the case study focused on histology as the main source of heterogeneity. However, heterogeneity could be explored using a range of alternative characteristics and subgroups. To move from histology as the main source of heterogeneity to considering a wider range of characteristics requires an understanding of how different characteristics can be utilised and combined in different ways in decision-making. 151 How best to decide on which characteristics to utilise in decision-making and how they should interact is a complex question that requires further research. It is also important to note that the case study has focused on observable sources of heterogeneity. Inevitably, there will be unobservable sources of heterogeneity (e.g. unobserved differences between patients and/or studies) that cannot be explicitly addressed but will need to be taken into account by decision-makers when interpreting these findings.
Chapter 8 Recommendations for practice and further research
Drawing on the research findings, recommendations are provided relating to three distinct areas:
-
the types of analysis and evidence required to inform decisions regarding histology-independent drugs by NICE
-
potential changes to the NICE methods guide for TAs or additional requirements relating to histology-independent drugs
-
priorities for methodological research.
Types of analyses and evidence required to inform decisions regarding histology-independent products
Treatment effectiveness
Complex innovative study designs are increasingly used to improve the efficiency of the drug development process and to speed up regulatory approval and the access of drugs with new mechanisms of action. Adaptive basket trials are particularly suited to assess the efficacy of histology-independent drugs, although their reliance on surrogate outcomes, small sample sizes and mostly uncontrolled designs pose challenges for HTA. Adequately designed and analysed basket studies that assess the homogeneity of outcomes and allow borrowing of information across baskets, where appropriate, are recommended. In particular, the use of comparative and randomised designs and primary outcomes that can adequately predict the clinical outcomes of interest is recommended where feasible.
The potential for heterogeneity in treatment effects, either across tumour types or across other characteristics, is likely to be an important issue in the appraisal of histology-independent technologies. Careful consideration should be given to the appropriateness of the assumptions of homogeneity of treatment effects and NICE committees should expect to see an exploration of this assumption in company submissions. Bayesian hierarchical methods, which are frequently used in the analysis of basket trials, may provide a useful vehicle with which to explore any heterogeneity. Where there is evidence of heterogeneity in treatment effects and estimates of cost-effectiveness, consideration should be given to optimised recommendations.
Counterfactual
Generating a counterfactual is likely to be challenging in the context of histology-independent technologies and, in the absence of randomised evidence, it is likely that no single approach will be able to provide robust estimates of relative effectiveness. Companies developing histology-independent technologies, therefore, should be encouraged to consider several alternatives. Consideration should be given to the relative strengths and weaknesses of these alternatives when evaluating the most appropriate comparison. Evidence on the prognostic and predicative performance of the biomarkers should also be considered where possible, although it is recognised that such data may be limited at the time of submission.
Generalisability
The trial evidence available to support the approval of histology-independent technologies may differ substantially from the patients eligible for treatment in practice. Significant differences may, for example, be seen in the distribution of tumour types, positioning of the technology and subsequent treatments received. The potential for heterogeneity in treatment effects means that differences between the trial population and the eligible population may have an important impact on estimates of cost-effectiveness. Where possible, it is important that such differences are properly accounted for. Consideration of the differences between the trial population and the eligible population should also be borne in mind when considering an appropriate counterfactual data set.
Trial evidence supporting histology-independent technologies may not offer complete coverage of the eligible population. For this reason, there may be no effectiveness evidence supporting a proportion of the eligible population. Appropriate consideration should be given to these unrepresented tumour types in the appraisal of histology-independent technologies. BHM may be able to provide an estimate of the distribution of treatment effects in this population. Data collection plans, where considered appropriate, should consider the potential for collecting evidence in unpresented tumours to better inform estimates of effect. Consideration should also be given to the fact that unrepresented tumours are not a single tumour type and may be heterogeneous. For this reason, blanket approval or collection of data in unrepresented tumours may not be appropriate.
Genomic testing
Genomic testing is likely to be integral to identify patients eligible for histology-independent therapy. Genomic testing costs may vary substantially across tumour types and, therefore, represent an important potential source of heterogeneity that should be appropriately considered. It is possible that some tumour types will not be cost-effective on the basis of genomic testing costs alone. Current NICE guidance provides that testing should be included where necessary to support a new health-care technology. Investment in universal provision of genomic testing, however, generates challenges to this model because some testing strategies may be used to identify multiple potential targets. In principle, it may, therefore, be appropriate to apportion testing costs over several technologies. It is currently unclear how this should be undertaken or who should make such judgments.
Model structure
Alternative sources of heterogeneity that may impact cost-effectiveness estimates (e.g. baseline risk, treatment effect, costs and HRQoL) should be explicitly acknowledged and appropriately reflected in any economic model. Where an economic analysis is developed using a partitioned survival analysis approach based on the direct extrapolation of TTE end points, appropriate exploration of the validity of pooling PFS and OS across prespecified subgroups and histologies (where data permit) should be undertaken (e.g. separate presentation of Kaplan–Meier curves and landmark PFS and OS rates). The process of internal and external validation should be clearly described.
The BHM approaches may be useful to support the validity of pooling PFS and OS data. Where there is substantive evidence of heterogeneity in treatment effects, consideration should be given to alternative model structures that are better able to reflect this heterogeneity, including the use of landmark response approaches. If such a model is used, evidence supporting the proposed surrogate final relationship should be presented and uncertainty surrounding the surrogate relationships included in the model should be fully characterised. Although concerns remain regarding the validity of response as a surrogate for PFS and OS, a surrogate-based modelling approach informed by predictions from meta-analyses that capture all relevant uncertainty may be preferable to the extrapolation of heavily censored and potentially confounded PFS and OS data. The BRMA approach outlined in DSU TSD 20146 is recommended to ensure that all uncertainty around the surrogate relationship is reflected in the predictions used in the model.
Consideration of uncertainty
Uncertainty is inherent to all decisions made by NICE and other reimbursement agencies, but may be particularly acute when considering histology-independent technologies owing to the limitations of the underlying evidence base. When considering the implications of uncertainty, due consideration should be given to the scale and consequence of decisions because often populations may be small with limited consequences to the overall health system. Quantification of the consequences of uncertainty using NHB may provide an important framework to add to the assessments already routinely specified with the existing TA methods guide4 to quantify decision uncertainty. Routine presentation of such metrics should be considered.
Potential changes to the National Institute for Health and Care Excellence methods guide for technology appraisals and/or additional requirements relating to histology-independent drugs
In practice, there may be barriers to NICE making partially or fully stratified decisions. This is because the NICE TA process has been developed primarily to make approval decisions for a technology in a defined population. However, stratified decision-making can be considered as a subgroup analysis in which histology is the relevant source of heterogeneity. The NICE methods guide recognises that costs and the capacity to benefit may differ across patients with differing characteristics and recommends that this should be explored as part of the reference case. 4 The assessments outlined in our report are consistent with and should be supplemented by the existing NICE guidance on subgroup analysis.
The quantity of subgroups that result from fully stratified decision-making could present a challenge to implementing this approach in practice. Partial stratification of approval decisions is one approach to address this. Partial stratification would reduce the number of approval decisions that must be made compared with full stratification. A transparent and accountable process for deciding which histologies should be grouped together would be required under this approach. The process for deciding which histologies should be grouped together could be usefully informed by the criteria for defining subgroups in the NICE methods guide. According to the current process, subgroups should be based on the expectation of ‘differential clinical or cost-effectiveness, biologically plausible mechanisms, social characteristics or other clearly justified factors’ (© NICE 2013. Guide to the Methods of Technology Appraisal. Available at: www.nice.org.uk/process/pmg9/chapter/foreword. All rights reserved. Subject to Notice of rights. NICE guidance is prepared for the National Health Service in England. All NICE guidance is subject to regular review and may be updated or withdrawn. NICE accepts no responsibility for the use of its content in this product/publication). 4 The relevant subgroups should be defined at the scoping stage but with the possibility of subgroup identification later in the process. This same process may be appropriate to define which histologies should be grouped together to make partially stratified approval decisions.
A further issue for NICE methods and processes concerns the approval of a histology-independent treatment in histologies that are not included in the main clinical studies. This could be considered as a specific case of a more general problem concerning the approval of treatments in populations for which there is limited or no direct evidence. This problem is faced in different forms, two of which are outlined here to provide additional context for approval decisions covering unrepresented histologies. The first scenario pertains to making decisions about treatments using unrepresentative data. For example, approval is commonly granted for populations that are only imperfectly represented in trial data. This is the problem of external validity and is common with randomised trial data because clinical trials tend to be conducted populations that differ from the population of interest. 211 The second scenario is using a technology in new indications for which there are no data. For example, pembrolizumab has been submitted for approval in a range of indications, including squamous NSCLC, urothelial cancer, and head and neck cancer, among others. 212–214 In this case, approval may not be granted for a new indication unless there is direct evidence in the population of interest. The approval in unrepresented histologies for histology-independent technologies represents a space between these two scenarios. Approval decisions for treatments for use in unrepresented histologies will depend on context; in some cases it will be more similar to approving in a slightly different population and in others it will be more analogous to approving in a completely new indication.
Assessments of heterogeneity in survival outcomes at the point of initial marketing authorisation may be challenging owing to data immaturity and potential confounding, unless these are more explicitly linked to a surrogate outcome (e.g. response and DoR) for which more robust assessments of heterogeneity may be feasible. Although BHM approaches could in theory be explored in the context of TTE end points (PFS, OS), the small numbers, potential for greater heterogeneity, high censoring and potential confounding remain important obstacles. However, many of the challenges associated with immaturity in TTE end points and the potential confounding in uncontrolled Phase II studies are not restricted to histology-independent appraisals. Our review of NICE TAs for products approved with ORR as the primary end point identified a potential disconnect between the regulators’ acceptance of surrogate end points and the limited use of surrogate relationships in the corresponding NICE appraisals. Although this disconnect may reflect legitimate concerns regarding the reliability of ORR and CR as surrogates for PFS or OS, we recommend that exploration of the surrogate relationships between response-based outcomes (ORR and DoR) should be more routinely considered in economic modelling to help to inform and/or validate longer-term extrapolations of PFS and OS owing to the likely immaturity of these end points. NICE will need to consider whether or not their existing methods guide needs to be more explicit about the challenges of uncontrolled Phase II studies and whether or not more specific guidance is required concerning the role and use of surrogate end points in these circumstances.
The presentation of the scale of the consequences of heterogeneity and decision uncertainty using population NHB may provide an important additional approach to the assessments already routinely specified with the existing TA methods guide. Similar arguments have been made in the context of regenerative medicines and cell therapies. 194 As part of their ongoing methods review, NICE could consider whether or not the types of metrics presented in this report should be routinely requested within company submissions.
Priorities for future methodological research
Methods were suggested that allowed for potential sources of heterogeneity of effect across tumour type or other patient characteristics to be accounted for, while still allowing some degree of borrowing of strength when estimating treatment effectiveness. However, the estimation of the level of heterogeneity can be poor when evidence is sparse. Approaches for considering external evidence and expert opinion to construct an informative prior distribution for the heterogeneity parameter may be an area for further research.
Even if the heterogeneity parameter can be well estimated, it is unclear what degree of borrowing should be allowed when there is evidence of a high or very high level of heterogeneity. In particular, the implications of borrowing strength across treatment effects in the presence of very high heterogeneity and consequences for uncertainty in decision-making should be researched.
So far, methods, such as the BHM, that allow borrowing of information have mainly been applied to response end points. Their extension to TTE end points and potential for adjustment for known prognostic factors and other confounders would be an interesting area for further research. In addition, further research should also consider the application of BHM approaches to surrogate relationships to determine the validity of borrowing across different subgroups and drug classes.
Given the increasing use of uncontrolled Phase II studies to support initial regulatory approval based on surrogate end points, further methodological research is required to determine the basis for selecting between alternative surrogate end points for HTA assessments and, specifically, the appropriate basis for selecting specific landmark response and survival time points.
Given the importance of testing costs as a source of heterogeneity and the lack of a clear consensus on the appropriate basis for apportioning costs between current and future targets, further methodological research should more fully establish how these costs should be appropriately included in future NICE appraisals.
Acknowledgements
We acknowledge and thank Jacoline Bouvy (Senior Scientific Adviser, NICE) and Sophie Cooper (Scientific Adviser, NICE) for their advice throughout the project and for their comments on the report.
Patient and public involvement
No patient and public involvement was undertaken.
Contribution of authors
Peter Murphy (https://orcid.org/0000-0001-8864-1416) [Research Fellow (Health Economist)] contributed to the review of issues for cost-effectiveness, the application of the Bayesian hierarchical approach and the exemplar case study, and wrote sections of the report.
David Glynn (https://orcid.org/0000-0002-0989-1984) [Research Fellow (Health Economist)] developed the decision framework, undertook the analyses using the case study and wrote sections of the report.
Sofia Dias (https://orcid.org/0000-0002-2172-0221) [Professor of HTA (Statistician)] had overall responsibility for the clinical effectiveness sections of the report. She contributed to the protocol, the review of statistical literature addressing the design and analysis of histology-independent trials, and writing the report.
Robert Hodgson (https://orcid.org/0000-0001-6962-2893) [Research Fellow (Health Economist)] contributed to the protocol, the review of issues for cost-effectiveness, the diagnostic testing issues, the development of the exemplar case study and writing the report.
Lindsay Claxton (https://orcid.org/0000-0002-1795-7568) [Research Fellow (Health Economist)] contributed to the review of issues for cost-effectiveness and writing the report.
Lucy Beresford (https://orcid.org/0000-0001-6803-5566) [NIHR Research Training Fellow (Systematic Reviewer)] contributed to the review of diagnostic testing issues and writing the report.
Katy Cooper (https://orcid.org/0000-0002-7702-8103) [Senior Research Fellow (Systematic Reviewer)] contributed to the systematic review of surrogate end points and writing the report.
Paul Tappenden (https://orcid.org/0000-0001-6612-2332) (Professor of Health Economic Modelling) contributed to the protocol, the systematic review of surrogate end points and writing the report.
Kate Ennis (https://orcid.org/0000-0003-4284-217X) [Research Associate (Health Economist)] contributed to the systematic review of surrogate end points and writing the report.
Alessandro Grosso (https://orcid.org/0000-0001-7211-438X) [Research Fellow (Health Economist)] contributed to the review of regulatory guidance and the review of previous NICE appraisals.
Kath Wright (https://orcid.org/0000-0002-9020-1572) (Information Specialist) undertook the search for studies, managed references and wrote sections of the report.
Anna Cantrell (https://orcid.org/0000-0003-0040-9853) (Information Specialist) undertook the search for studies, managed references for the review of surrogates and wrote sections of the report.
Matt Stevenson (https://orcid.org/0000-0002-3099-9877) (Professor of HTA) contributed to the protocol and commented on all sections of the report.
Stephen Palmer (https://orcid.org/0000-0002-7268-2560) (Professor of Health Economics) had overall responsibility for the project. He contributed to the protocol and to all aspects of the work, including writing the report.
Publications
Cooper K, Tappenden P, Cantrell A, Ennis K. A systematic review of meta-analyses assessing the validity of tumour response endpoints as surrogates for progression-free or overall survival in cancer. Br J Cancer 2020;123:1686–96.
Murphy P, Claxton L, Hodgson R, Glynn D, Beresford L, Walton M, et al. Exploring heterogeneity in histology-independent technologies and the implications for cost-effectiveness. Med Decis Making 2021;41:165–78.
Data-sharing statement
The report is based on an assessment of a hypothetical case study and, therefore, the data generated are not suitable for sharing beyond that contained within the report. Further information can be obtained from the corresponding author.
Disclaimers
This report presents independent research funded by the National Institute for Health Research (NIHR). The views and opinions expressed by authors in this publication are those of the authors and do not necessarily reflect those of the NHS, the NIHR, NETSCC, the HTA programme or the Department of Health and Social Care. If there are verbatim quotations included in this publication the views and opinions expressed by the interviewees are those of the interviewees and do not necessarily reflect those of the authors, those of the NHS, the NIHR, NETSCC, the HTA programme or the Department of Health and Social Care.
References
- US Food and Drug Administration (FDA), Center for Drug Evaluation and Research . Approval Package for Application Number 125514Orig1s014. Trade Name: Keytruda. Generic or Proper Name: Pembrolizumab 2017.
- European Medicines Agency (EMA) . Assessment Report: VITRAKVI. International Non-Proprietary Name: Larotrectinib 2019.
- Drilon A, Laetsch TW, Kummar S, DuBois SG, Lassen UN, Demetri GD, et al. Efficacy of Larotrectinib in TRK fusion-positive cancers in adults and children. N Engl J Med 2018;378:731-9. https://doi.org/10.1056/NEJMoa1714448.
- National Institute for Health and Care Excellence (NICE) . Guide to the Methods of Technology Appraisal 2013.
- U.S. Food and Drug Administration (FDA), Center for Drug Evaluation and Research, Center for Biologics Evaluation and Research . Developing Targeted Therapies in Low-Frequency Molecular Subsets of a Disease. Guidance for Industry 2018.
- U.S. Food and Drug Administration (FDA), Center for Drug Evaluation and Research, Center for Biologics Evaluation and Research, Oncology Center of Excellence . Master Protocols: Efficient Clinical Trial Design Strategies to Expedite Development of Oncology Drugs and Biologics. Guidance for Industry 2018.
- Simon R. Optimal two-stage designs for phase II clinical trials. Control Clin Trials 1989;10:1-10. https://doi.org/10.1016/0197-2456(89)90015-9.
- U.S. Food and Drug Administration (FDA), Center for Drug Evaluation and Research, Center for Biologics Evaluation and Research . Guidance for Industry Expedited Programs for Serious Conditions – Drugs and Biologics 2014.
- U.S. Food and Drug Administration (FDA) . Drug Approval Package: Vitrakvi (Larotrectinib) 2018. www.accessdata.fda.gov/drugsatfda_docs/nda/2018/210861Orig1s000_21171Orig1s000TOC.cfm (accessed 17 January 2020).
- U.S. Food and Drug Administration (FDA), Center for Drug Evaluation and Research . Approval Package for Application Number 212725Orig1s000, 212726Orig1s000. Trade Name: ROZLYTREK® Capsules, 100 Mg and 200 Mg (Entrectinib) 2019.
- Kim C, Prasad V. Strength of validation for surrogate end points used in the US Food and Drug Administration’s approval of oncology drugs. Mayo Clin Proc 2016;91:713-25. https://doi.org/10.1016/j.mayocp.2016.02.012.
- Doebele RC, Drilon A, Paz-Ares L, Siena S, Shaw AT, Farago AF, et al. Entrectinib in patients with advanced or metastatic NTRK fusion-positive solid tumours: integrated analysis of three phase 1-2 trials. Lancet Oncol 2020;21:271-82. https://doi.org/10.1016/S1470-2045(19)30691-6.
- European Medicines Agency (EMA) . Workshop on Site and Histology - Independent Indications in Oncology 2017. www.ema.europa.eu/en/events/workshop-site-histology-independent-indications-oncology (accessed 17 January 2020).
- European Medicines Agency (EMA) . Workshop on Single-Arm Trials in Oncology 2016. www.ema.europa.eu/en/events/workshop-single-arm-trials-oncology (accessed 17 January 2020).
- European Medicines Agency (EMA) . Guideline on the Evaluation of Anticancer Medicinal Products in Man 2017.
- European Medicines Agency (EMA) . Concept Paper on the Revision of the Guideline on the Evaluation of Anticancer Medicinal Products in Man 2018.
- Laetsch TW, DuBois SG, Mascarenhas L, Turpin B, Federman N, Albert CM, et al. Larotrectinib for paediatric solid tumours harbouring NTRK fusions: phase 1 results from a multicentre, open-label, phase 1/2 study. Lancet Oncol 2018;19:705-14. https://doi.org/10.1016/S1470-2045(18)30119-0.
- Gehan EA. The determination of the number of patients required in a preliminary and a follow-up trial of a new chemotherapeutic agent. J Chronic Dis 1961;13:346-53. https://doi.org/10.1016/0021-9681(61)90060-1.
- Fleming TR. One-sample multiple testing procedure for phase II clinical trial. Biometrics 1982;38:143-51. https://doi.org/10.2307/2530297.
- Lin Y, Shih WJ. Adaptive two-stage designs for single-arm phase IIA cancer clinical trials. Biometrics 2004;60:482-90. https://doi.org/10.1111/j.0006-341X.2004.00193.x.
- Herson J. Predictive probability early termination plans for phase II clinical trials. Biometrics 1979;35:775-83. https://doi.org/10.2307/2530109.
- Thall PF, Simon R. Practical Bayesian guidelines for phase IIB clinical trials. Biometrics 1994;50:337-49. https://doi.org/10.2307/2533377.
- Heitjan DF. Bayesian interim analysis of phase II cancer clinical trials. Stat Med 1997;16:1791-802. https://doi.org/10.1002/(SICI)1097-0258(19970830)16:16<1791::AID-SIM609>3.0.CO;2-E.
- Tan SB, Machin D. Bayesian two-stage designs for phase II clinical trials. Stat Med 2002;21:1991-2012. https://doi.org/10.1002/sim.1176.
- Blagden SP, Billingham L, Brown LC, Buckland SW, Cooper AM, Ellis S, et al. Effective delivery of Complex Innovative Design (CID) cancer trials – a consensus statement. Br J Cancer 2020;122:473-82. https://doi.org/10.1038/s41416-019-0653-9.
- Redig AJ, Jänne PA. Basket trials and the evolution of clinical trial design in an era of genomic medicine. J Clin Oncol 2015;33:975-7. https://doi.org/10.1200/JCO.2014.59.8433.
- Renfro LA, Sargent DJ. Statistical controversies in clinical research: basket trials, umbrella trials, and other master protocols: a review and examples. Ann Oncol 2017;28:34-43. https://doi.org/10.1093/annonc/mdw413.
- Hyman DM, Taylor BS, Baselga J. Implementing genome-driven oncology. Cell 2017;168:584-99. https://doi.org/10.1016/j.cell.2016.12.015.
- Renfro LA, Mandrekar SJ. Definitions and statistical properties of master protocols for personalized medicine in oncology. J Biopharm Stat 2018;28:217-28. https://doi.org/10.1080/10543406.2017.1372778.
- Simon R. New designs for basket clinical trials in oncology. J Biopharm Stat 2018;28:245-55. https://doi.org/10.1080/10543406.2017.1372779.
- Lopez-Chavez A, Thomas A, Rajan A, Raffeld M, Morrow B, Kelly R, et al. Molecular profiling and targeted therapy for advanced thoracic malignancies: a biomarker-derived, multiarm, multihistology phase II basket trial. J Clin Oncol 2015;33:1000-7. https://doi.org/10.1200/JCO.2014.58.2007.
- Hyman DM, Puzanov I, Subbiah V, Faris JE, Chau I, Blay JY, et al. Vemurafenib in multiple nonmelanoma cancers with BRAF V600 mutations. N Engl J Med 2015;373:726-36. https://doi.org/10.1056/NEJMoa1502309.
- Le Tourneau C, Delord JP, Gonçalves A, Gavoille C, Dubot C, Isambert N, et al. Molecularly targeted therapy based on tumour molecular profiling versus conventional therapy for advanced cancer (SHIVA): a multicentre, open-label, proof-of-concept, randomised, controlled phase 2 trial. Lancet Oncol 2015;16:1324-34. https://doi.org/10.1016/S1470-2045(15)00188-6.
- Beckman RA, Antonijevic Z, Kalamegham R, Chen C. Adaptive design for a confirmatory basket trial in multiple tumor types based on a putative predictive biomarker. Clin Pharmacol Ther 2016;100:617-25. https://doi.org/10.1002/cpt.446.
- Antonijevic Z, Beckman R. Platform Trial Designs in Drug Development: Umbrella Trials and Basket Trials. Boca Raton, FL: CRD Press; 2019.
- Freidlin B, Korn EL. Borrowing information across subgroups in phase II trials: is it useful?. Clin Cancer Res 2013;19:1326-34. https://doi.org/10.1158/1078-0432.CCR-12-1223.
- Simon R. Critical review of umbrella, basket, and platform designs for oncology clinical trials. Clin Pharmacol Ther 2017;102:934-41. https://doi.org/10.1002/cpt.814.
- Simon RM, Antonijevic Z, Beckman R. Platform Trials in Drug Development: Umbrella Trials and Basket Trials. Boca Raton, FL: CRC Press; 2019.
- London WB, Chang MN. One- and two-stage designs for stratified phase II clinical trials. Stat Med 2005;24:2597-611. https://doi.org/10.1002/sim.2139.
- Leblanc M, Rankin C, Crowley J. Multiple histology phase II trials. Clin Cancer Res 2009;15:4256-62. https://doi.org/10.1158/1078-0432.CCR-08-2069.
- Jung SH, Chang MN, Kang SJ. Phase II cancer clinical trials with heterogeneous patient populations. J Biopharm Stat 2012;22:312-28. https://doi.org/10.1080/10543406.2010.536873.
- Cunanan KM, Iasonos A, Shen R, Begg CB, Gönen M. An efficient basket trial design. Stat Med 2017;36:1568-79. https://doi.org/10.1002/sim.7227.
- Simon R, Geyer S, Subramanian J, Roychowdhury S. The Bayesian basket design for genomic variant-driven phase II trials. Semin Oncol 2016;43:13-8. https://doi.org/10.1053/j.seminoncol.2016.01.002.
- Palmer AC, Plana D, Sorger PK. Comparing the efficacy of cancer therapies between subgroups in basket trials. Cell Syst 2020;11:449-60.E2. https://doi.org/10.1101/401620.
- Spiegelhalter DJ, Abrams KR, Myles J. Bayesian Approaches to Clinical Trials and Health-Care Evaluation. New York, NY: Wiley; 2004.
- Thall PF, Wathen JK, Bekele BN, Champlin RE, Baker LH, Benjamin RS. Hierarchical Bayesian approaches to phase II trials in diseases with multiple subtypes. Stat Med 2003;22:763-80. https://doi.org/10.1002/sim.1399.
- Thall PF, Sung HG. Some extensions and applications of a Bayesian strategy for monitoring multiple outcomes in clinical trials. Stat Med 1998;17:1563-80. https://doi.org/10.1002/(SICI)1097-0258(19980730)17:14%3C1563::AID-SIM873%3E3.0.CO;2-L.
- Chugh R, Wathen JK, Maki RG, Benjamin RS, Patel SR, Meyers PA, et al. Phase II multicenter trial of imatinib in 10 histologic subtypes of sarcoma using a Bayesian hierarchical statistical model. J Clin Oncol 2009;27:3148-53. https://doi.org/10.1200/JCO.2008.20.5054.
- Berry SM, Broglio KR, Groshen S, Berry DA. Bayesian hierarchical modeling of patient subpopulations: efficient designs of Phase II oncology clinical trials. Clin Trials 2013;10:720-34. https://doi.org/10.1177/1740774513497539.
- Liu R, Liu Z, Ghadessi M, Vonk R. Increasing the efficiency of oncology basket trials using a Bayesian approach. Contemp Clin Trials 2017;63:67-72. https://doi.org/10.1016/j.cct.2017.06.009.
- Neuenschwander B, Wandel S, Roychoudhury S, Bailey S. Robust exchangeability designs for early phase clinical trials with multiple strata. Pharm Stat 2016;15:123-34. https://doi.org/10.1002/pst.1730.
- Cunanan KM, Iasonos A, Shen R, Gönen M. Variance prior specification for a basket trial design using Bayesian hierarchical modeling. Clin Trials 2019;16:142-53. https://doi.org/10.1177/1740774518812779.
- Leon-Novelo LG, Bekele BN, Müller P, Quintana F, Wathen K. Borrowing strength with nonexchangeable priors over subpopulations. Biometrics 2012;68:550-8. https://doi.org/10.1111/j.1541-0420.2011.01693.x.
- Chu Y, Yuan Y. BLAST: Bayesian latent subgroup design for basket trials accounting for patient heterogeneity. J R Stat Soc Ser C Appl Stat 2018;67:723-40. https://doi.org/10.1111/rssc.12255.
- Chu Y, Yuan Y. A Bayesian basket trial design using a calibrated Bayesian hierarchical model. Clin Trials 2018;15:149-58. https://doi.org/10.1177/1740774518755122.
- Fujikawa K, Teramukai S, Yokota I, Daimon T. A Bayesian basket trial design that borrows information across strata based on the similarity between the posterior distributions of the response probability. Biom J 2020;62:8-330. https://doi.org/10.1002/bimj.201800404.
- Hobbs BP, Landin R. Bayesian basket trial design with exchangeability monitoring. Stat Med 2018;37:3557-72. https://doi.org/10.1002/sim.7893.
- Flaherty KT, Puzanov I, Kim KB, Ribas A, McArthur GA, Sosman JA, et al. Inhibition of mutated, activated BRAF in metastatic melanoma. N Engl J Med 2010;363:809-19. https://doi.org/10.1056/NEJMoa1002011.
- Prahallad A, Sun C, Huang S, Di Nicolantonio F, Salazar R, Zecchin D, et al. Unresponsiveness of colon cancer to BRAF(V600E) inhibition through feedback activation of EGFR. Nature 2012;483:100-3. https://doi.org/10.1038/nature10868.
- Heinrich MC, Joensuu H, Demetri GD, Corless CL, Apperley J, Fletcher JA, et al. Phase II, open-label study evaluating the activity of imatinib in treating life-threatening malignancies known to be associated with imatinib-sensitive tyrosine kinases. Clin Cancer Res 2008;14:2717-25. https://doi.org/10.1158/1078-0432.CCR-07-4575.
- Hudis CA. Trastuzumab – mechanism of action and use in clinical practice. N Engl J Med 2007;357:39-51. https://doi.org/10.1056/NEJMra043186.
- Fleming GF, Sill MW, Darcy KM, McMeekin DS, Thigpen JT, Adler LM, et al. Phase II trial of trastuzumab in women with advanced or recurrent, HER2-positive endometrial carcinoma: a Gynecologic Oncology Group study. Gynecol Oncol 2010;116:15-20. https://doi.org/10.1016/j.ygyno.2009.09.025.
- Gatzemeier U, Groth G, Butts C, van Zandwijk N, Shepherd F, Ardizzoni A, et al. Randomized phase II trial of gemcitabine-cisplatin with or without trastuzumab in HER2-positive non-small-cell lung cancer. Ann Oncol 2004;15:19-27. https://doi.org/10.1093/annonc/mdh031.
- Cooper K, Tappenden P, Cantrell A, Ennis K. A systematic review of meta-analyses assessing the validity of tumour response endpoints as surrogates for progression-free or overall survival in cancer. Br J Cancer 2020;123:1686-96. https://doi.org/10.1038/s41416-020-01050-w.
- Fleming TR, Powers JH. Biomarkers and surrogate endpoints in clinical trials. Stat Med 2012;31:2973-84. https://doi.org/10.1002/sim.5403.
- Taylor RS, Elston J. The use of surrogate outcomes in model-based cost-effectiveness analyses: a survey of UK Health Technology Assessment reports. Health Technol Assess 2009;13. https://doi.org/10.3310/hta13080.
- Fleming TR, DeMets DL. Surrogate end points in clinical trials: are we being misled?. Ann Intern Med 1996;125:605-13. https://doi.org/10.7326/0003-4819-125-7-199610010-00011.
- Prentice RL. Surrogate endpoints in clinical trials: definition and operational criteria. Stat Med 1989;8:431-40. https://doi.org/10.1002/sim.4780080407.
- Buyse M, Molenberghs G, Burzykowski T, Renard D, Geys H. The validation of surrogate endpoints in meta-analyses of randomized experiments. Biostatistics 2000;1:49-67. https://doi.org/10.1093/biostatistics/1.1.49.
- Heller G. Statistical controversies in clinical research: an initial evaluation of a surrogate end point using a single randomized clinical trial and the Prentice criteria. Ann Oncol 2015;26:2012-16. https://doi.org/10.1093/annonc/mdv333.
- Biomarkers Definitions Working Group . Biomarkers and surrogate endpoints: preferred definitions and conceptual framework. Clin Pharmacol Ther 2001;69:89-95. https://doi.org/10.1067/mcp.2001.113989.
- Bucher HC, Guyatt GH, Cook DJ, Holbrook A, McAlister FA. Users’ guides to the medical literature: XIX. Applying clinical trial results. A. How to use an article measuring the effect of an intervention on surrogate end points. JAMA 1999;282:771-8. https://doi.org/10.1001/jama.282.8.771.
- Elston J, Taylor RS. Use of surrogate outcomes in cost-effectiveness models: a review of United Kingdom health technology assessment reports. Int J Technol Assess Health Care 2009;25:6-13. https://doi.org/10.1017/S0266462309090023.
- German Institute of Quality and Efficiency in Health Care (IQWiG) . Validity of Surrogate Endpoints in Oncology 2011.
- Lassere MN, Johnson KR, Schiff M, Rees D. Is blood pressure reduction a valid surrogate endpoint for stroke prevention? An analysis incorporating a systematic review of randomised controlled trials, a by-trial weighted errors-in-variables regression, the surrogate threshold effect (STE) and the Biomarker-Surrogacy (BioSurrogate) Evaluation Schema (BSES). BMC Med Res Methodol 2012;12. https://doi.org/10.1186/1471-2288-12-27.
- Buyse M, Molenberghs G, Paoletti X, Oba K, Alonso A, van der Elst W, et al. Statistical evaluation of surrogate endpoints with examples from cancer clinical trials. Biom J 2016;58:104-32. https://doi.org/10.1002/bimj.201400049.
- Fischer A, Hernandez-Villafuerte K, Latimer N, Henshall C. Extrapolation from Progression-Free Survival to Overall Survival in Oncology. London: Office of Health Economics; 2016.
- Davis S, Tappenden P, Cantrell A. A Review of Studies Examining the Relationship Between Progression-free Survival and Overall Survival in Advanced or Metastatic Cancer. Sheffield: University of Sheffield Report for NICE Decision Support Unit; 2012.
- Savina M, Gourgou S, Italiano A, Dinart D, Rondeau V, Penel N, et al. Meta-analyses evaluating surrogate endpoints for overall survival in cancer randomized trials: a critical review. Crit Rev Oncol Hematol 2018;123:21-4. https://doi.org/10.1016/j.critrevonc.2017.11.014.
- Haslam A, Hey SP, Gill J, Prasad V. A systematic review of trial-level meta-analyses measuring the strength of association between surrogate end-points and overall survival in oncology. Eur J Cancer 2019;106:196-211. https://doi.org/10.1016/j.ejca.2018.11.012.
- Abdel-Rahman O. Surrogate end points for overall survival in trials of PD-(L)1 inhibitors for urinary cancers: a systematic review. Immunotherapy 2018;10:139-48. https://doi.org/10.2217/imt-2017-0115.
- Agarwal N, Bellmunt J, Maughan BL, Boucher KM, Choueiri TK, Qu AQ, et al. Six-month progression-free survival as the primary endpoint to evaluate the activity of new agents as second-line therapy for advanced urothelial carcinoma. Clin Genitourin Cancer 2014;12:130-7. https://doi.org/10.1016/j.clgc.2013.09.002.
- Agarwal SK, Mangal N, Menon RM, Freise KJ, Salem AH. Response rates as predictors of overall survival: a meta-analysis of acute myeloid leukemia trials. J Cancer 2017;8:1562-7. https://doi.org/10.7150/jca.18686.
- Blumenthal GM, Karuri SW, Zhang H, Zhang L, Khozin S, Kazandjian D, et al. Overall response rate, progression-free survival, and overall survival with targeted and standard therapies in advanced non-small-cell lung cancer: US Food and Drug Administration trial-level and patient-level analyses. J Clin Oncol 2015;33:1008-14. https://doi.org/10.1200/JCO.2014.59.0489.
- Blumenthal GM, Zhang L, Zhang H, Kazandjian D, Khozin S, Tang S, et al. Milestone analyses of immune checkpoint inhibitors, targeted therapy, and conventional therapy in metastatic non-small cell lung cancer trials: a meta-analysis. JAMA Oncol 2017;3. https://doi.org/10.1001/jamaoncol.2017.1029.
- Bruzzi P, Del Mastro L, Sormani MP, Bastholt L, Danova M, Focan C, et al. Objective response to chemotherapy as a potential surrogate end point of survival in metastatic breast cancer patients. J Clin Oncol 2005;23:5117-25. https://doi.org/10.1200/JCO.2005.02.106.
- Burzykowski T, Buyse M, Piccart-Gebhart MJ, Sledge G, Carmichael J, Lück HJ, et al. Evaluation of tumor response, disease control, progression-free survival, and time to progression as potential surrogate end points in metastatic breast cancer. J Clin Oncol 2008;26:1987-92. https://doi.org/10.1200/JCO.2007.10.8407.
- Buyse M, Thirion P, Carlson RW, Burzykowski T, Molenberghs G, Piedbois P. Relation between tumour response to first-line chemotherapy and survival in advanced colorectal cancer: a meta-analysis. Meta-Analysis Group in Cancer. Lancet 2000;356:373-8. https://doi.org/10.1016/S0140-6736(00)02528-9.
- Ciani O, Buyse M, Garside R, Peters J, Saad ED, Stein K, et al. Meta-analyses of randomized controlled trials show suboptimal validity of surrogate outcomes for overall survival in advanced colorectal cancer. J Clin Epidemiol 2015;68:833-42. https://doi.org/10.1016/j.jclinepi.2015.02.016.
- Colloca G, Venturino A. Trial-level analysis of progression-free survival and response rate as end points of trials of first-line chemotherapy in advanced ovarian cancer. Med Oncol 2017;34. https://doi.org/10.1007/s12032-017-0939-9.
- Colloca G, Venturino A, Guarneri D. Analysis of response-related and time-to-event endpoints in randomized trials of gemcitabine-based treatment versus gemcitabine alone as first-line treatment of patients with advanced pancreatic cancer. Clin Colorectal Cancer 2016;15:264-76. https://doi.org/10.1016/j.clcc.2015.11.006.
- Colloca G, Venturino A, Guarneri D. Analysis of clinical end points of randomised trials including bevacizumab and chemotherapy versus chemotherapy as first-line treatment of metastatic colorectal cancer. Clin Oncol 2016;28:e155-64. https://doi.org/10.1016/j.clon.2016.05.001.
- Colloca G, Vitucci P, Venturino A. Trial level analysis of prostate-specific antigen-related versus unrelated endpoints in phase III trials of first-line and second-line medical treatments of patients with metastatic castration-resistant prostate cancer. Clin Genitourin Cancer 2016;14:389-97. https://doi.org/10.1016/j.clgc.2016.03.022.
- Cremolini C, Antoniotti C, Pietrantonio F, Berenato R, Tampellini M, Baratelli C, et al. Surrogate endpoints in second-line trials of targeted agents in metastatic colorectal cancer: a literature-based systematic review and meta-analysis. Cancer Res Treat 2017;49:834-45. https://doi.org/10.4143/crt.2016.249.
- Delea TE, Khuu A, Heng DY, Haas T, Soulières D. Association between treatment effects on disease progression end points and overall survival in clinical studies of patients with metastatic renal cell carcinoma. Br J Cancer 2012;107:1059-68. https://doi.org/10.1038/bjc.2012.367.
- Elia EG, Städler N, Ciani O, Taylor RS, Bujkiewicz S. Combining tumour response and progression free survival as surrogate endpoints for overall survival in advanced colorectal cancer. Cancer Epidemiol 2020;64. https://doi.org/10.1016/j.canep.2019.101665.
- Foster NR, Qi Y, Shi Q, Krook JE, Kugler JW, Jett JR, et al. Tumor response and progression-free survival as potential surrogate endpoints for overall survival in extensive stage small-cell lung cancer: findings on the basis of North Central Cancer Treatment Group trials. Cancer 2011;117:1262-71. https://doi.org/10.1002/cncr.25526.
- Giessen C, Laubender RP, Ankerst DP, Stintzing S, Modest DP, Schulz C, et al. Surrogate endpoints in second-line treatment for mCRC: a systematic literature-based analysis from 23 randomised trials. Acta Oncol 2015;54:187-93. https://doi.org/10.3109/0284186X.2014.938830.
- Hackshaw A, Knight A, Barrett-Lee P, Leonard R. Surrogate markers and survival in women receiving first-line combination anthracycline chemotherapy for advanced breast cancer. Br J Cancer 2005;93:1215-21. https://doi.org/10.1038/sj.bjc.6602858.
- Hamada T, Nakai Y, Isayama H, Yasunaga H, Matsui H, Takahara N, et al. Progression-free survival as a surrogate for overall survival in first-line chemotherapy for advanced pancreatic cancer. Eur J Cancer 2016;65:11-20. https://doi.org/10.1016/j.ejca.2016.05.016.
- Han K, Ren M, Wick W, Abrey L, Das A, Jin J, et al. Progression-free survival as a surrogate endpoint for overall survival in glioblastoma: a literature-based meta-analysis from 91 trials. Neuro Oncol 2014;16:696-70. https://doi.org/10.1093/neuonc/not236.
- Hashim M, Pfeiffer BM, Bartsch R, Postma M, Heeg B. Do surrogate endpoints better correlate with overall survival in studies that did not allow for crossover or reported balanced postprogression treatments? An application in advanced non-small cell lung cancer. Value Health 2018;21:9-17. https://doi.org/10.1016/j.jval.2017.07.011.
- Hotta K, Kato Y, Leighl N, Takigawa N, Gaafar RM, Kayatani H, et al. Magnitude of the benefit of progression-free survival as a potential surrogate marker in phase 3 trials assessing targeted agents in molecularly selected patients with advanced non-small cell lung cancer: systematic review. PLOS ONE 2015;10. https://doi.org/10.1371/journal.pone.0121211.
- Hotta K, Kiura K, Fujiwara Y, Takigawa N, Oze I, Ochi N, et al. Association between incremental gains in the objective response rate and survival improvement in phase III trials of first-line chemotherapy for extensive disease small-cell lung cancer. Ann Oncol 2009;20:829-34. https://doi.org/10.1093/annonc/mdp020.
- Ichikawa W, Sasaki Y. Correlation between tumor response to first-line chemotherapy and prognosis in advanced gastric cancer patients. Ann Oncol 2006;17:1665-72. https://doi.org/10.1093/annonc/mdl174.
- Imaoka H, Sasaki M, Takahashi H, Hashimoto Y, Ohno I, Mitsunaga S, et al. Progression-free survival as a surrogate endpoint in advanced neuroendocrine neoplasms. Endocr Relat Cancer 2017;24:475-83. https://doi.org/10.1530/ERC-17-0197.
- Imaoka H, Sasaki M, Takahashi H, Hashimoto Y, Ohno I, Mitsunaga S, et al. Alternate endpoints for phase II trials in advanced neuroendocrine tumors. Oncologist 2019;24:47-53. https://doi.org/10.1634/theoncologist.2017-0651.
- Iezzi R, Pompili M, Rinninella E, Annicchiarico E, Garcovich M, Cerrito L, et al. TACE with degradable starch microspheres (DSM-TACE) as second-line treatment in HCC patients dismissing or ineligible for sorafenib. Eur Radiol 2019;29:1285-92. https://doi.org/10.1007/s00330-018-5692-8.
- Johnson KR, Ringland C, Stokes BJ, Anthony DM, Freemantle N, Irs A, et al. Response rate or time to progression as predictors of survival in trials of metastatic colorectal cancer or non-small-cell lung cancer: a meta-analysis. Lancet Oncol 2006;7:741-6. https://doi.org/10.1016/S1470-2045(06)70800-2.
- Kaufman HL, Schwartz LH, William WN, Sznol M, Fahrbach K, Xu Y, et al. Evaluation of classical clinical endpoints as surrogates for overall survival in patients treated with immune checkpoint blockers: a systematic review and meta-analysis. J Cancer Res Clin Oncol 2018;144:2245-61. https://doi.org/10.1007/s00432-018-2738-x.
- Lee L, Wang L, Crump M. Identification of potential surrogate end points in randomized clinical trials of aggressive and indolent non-Hodgkin’s lymphoma: correlation of complete response, time-to-event and overall survival end points. Ann Oncol 2011;22:1392-403. https://doi.org/10.1093/annonc/mdq615.
- Li J, He Q, Yu X, Khan K, Weng X, Guan M. Complete response associated with immune checkpoint inhibitors in advanced non-small-cell lung cancer: a meta-analysis of nine randomized controlled trials. Cancer Manag Res 2019;11:1623-9. https://doi.org/10.2147/CMAR.S188551.
- Li X, Liu S, Gu H, Wang D. Surrogate end points for survival in the target treatment of advanced non-small-cell lung cancer with gefitinib or erlotinib. J Cancer Res Clin Oncol 2012;138:1963-9. https://doi.org/10.1007/s00432-012-1278-z.
- Liu L, Chen F, Zhao J, Yu H. Correlation between overall survival and other endpoints in metastatic breast cancer with second- or third-line chemotherapy: literature-based analysis of 24 randomized trials. Bull Cancer 2016;103:336-44. https://doi.org/10.1016/j.bulcan.2016.01.002.
- Louvet C, de Gramont A, Tournigand C, Artru P, Maindrault-Goebel F, Krulik M. Correlation between progression free survival and response rate in patients with metastatic colorectal carcinoma. Cancer 2001;91:2033-8. https://doi.org/10.1002/1097-0142(20010601)91:11<2033::AID-CNCR1229>3.0.CO;2-J.
- Makris EA, MacBarb R, Harvey DJ, Poultsides GA. Surrogate end points for overall survival in metastatic, locally advanced, or unresectable pancreatic cancer: a systematic review and meta-analysis of 24 randomized controlled trials. Ann Surg Oncol 2017;24:2371-8. https://doi.org/10.1245/s10434-017-5826-2.
- Mangal N, Salem AH, Li M, Menon R, Freise KJ. Relationship between response rates and median progression-free survival in non-Hodgkin’s lymphoma: a meta-analysis of published clinical trials. Hematol Oncol 2018;36:37-43. https://doi.org/10.1002/hon.2463.
- Mangal N, Salem AH, Menon RM, Freise KJ. Use of depth of response to predict progression-free survival in relapsed or refractory multiple myeloma: evaluation of results from 102 clinical trials. Hematol Oncol 2018;36:547-53. https://doi.org/10.1002/hon.2514.
- Moriwaki T, Yamamoto Y, Gosho M, Kobayashi M, Sugaya A, Yamada T, et al. Correlations of survival with progression-free survival, response rate, and disease control rate in advanced biliary tract cancer: a meta-analysis of randomised trials of first-line chemotherapy. Br J Cancer 2016;114:881-8. https://doi.org/10.1038/bjc.2016.83.
- Mushti SL, Mulkey F, Sridhara R. Evaluation of overall response rate and progression-free survival as potential surrogate endpoints for overall survival in immunotherapy trials. Clin Cancer Res 2018;24:2268-75. https://doi.org/10.1158/1078-0432.CCR-17-1902.
- Nakashima K, Horita N, Nagai K, Manabe S, Murakami S, Ota E, et al. Progression-free survival, response rate, and disease control rate as predictors of overall survival in phase III randomized controlled trials evaluating the first-line chemotherapy for advanced, locally advanced, and recurrent non-small cell lung carcinoma. J Thorac Oncol 2016;11:1574-85. https://doi.org/10.1016/j.jtho.2016.04.025.
- Nickolich M, Babakoohi S, Fu P, Dowlati A. Clinical trial design in small cell lung cancer: surrogate end points and statistical evolution. Clin Lung Cancer 2014;15:207-12. https://doi.org/10.1016/j.cllc.2013.12.001.
- Nie RC, Chen FP, Yuan SQ, Luo YS, Chen S, Chen YM, et al. Evaluation of objective response, disease control and progression-free survival as surrogate end-points for overall survival in anti-programmed death-1 and anti-programmed death ligand 1 trials. Eur J Cancer 2019;106:1-11. https://doi.org/10.1016/j.ejca.2018.10.011.
- Pang Y, Shen Z, Sun J, Wang W. Does the use of targeted agents in advanced gastroesophageal cancer increase complete response? A meta-analysis of 18 randomized controlled trials. Cancer Manag Res 2018;10:5505-14. https://doi.org/10.2147/CMAR.S174063.
- Penel N, Ryckewaert T, Kramar A. What is an active regimen in carcinoma of unknown primary sites? Analysis of correlation between activity endpoints reported in phase II trials. Correlation of activity endpoints in phase II trials. Bull Cancer 2014;101:E19-24. https://doi.org/10.1684/bdc.2014.1934.
- Petrelli F, Barni S. Surrogate end points and postprogression survival in renal cell carcinoma: an analysis of first-line trials with targeted therapies. Clin Genitourin Cancer 2013;11:385-9. https://doi.org/10.1016/j.clgc.2013.07.012.
- Petrelli F, Barni S. Surrogate endpoints in metastatic breast cancer treated with targeted therapies: an analysis of the first-line phase III trials. Med Oncol 2014;31. https://doi.org/10.1007/s12032-013-0776-4.
- Ritchie G, Gasper H, Man J, Lord S, Marschner I, Friedlander M, et al. Defining the most appropriate primary end point in phase 2 trials of immune checkpoint inhibitors for advanced solid cancers: a systematic review and meta-analysis. JAMA Oncol 2018;4:522-8. https://doi.org/10.1001/jamaoncol.2017.5236.
- Rose PG, Tian C, Bookman MA. Assessment of tumor response as a surrogate endpoint of survival in recurrent/platinum-resistant ovarian carcinoma: a Gynecologic Oncology Group study. Gynecol Oncol 2010;117:324-9. https://doi.org/10.1016/j.ygyno.2010.01.040.
- Roviello G, Andre F, Venturini S, Pistilli B, Curigliano G, Cristofanilli M, et al. Response rate as a potential surrogate for survival and efficacy in patients treated with novel immune checkpoint inhibitors: a meta-regression of randomised prospective studies. Eur J Cancer 2017;86:257-65. https://doi.org/10.1016/j.ejca.2017.09.018.
- Sekine I, Tamura T, Kunitoh H, Kubota K, Shinkai T, Kamiya Y, et al. Progressive disease rate as a surrogate endpoint of phase II trials for non-small-cell lung cancer. Ann Oncol 1999;10:731-3. https://doi.org/10.1023/a:1008303921033.
- Shi Q, Flowers CR, Hiddemann W, Marcus R, Herold M, Hagenbeek A, et al. Thirty-month complete response as a surrogate end point in first-line follicular lymphoma therapy: an individual patient-level analysis of multiple randomized trials. J Clin Oncol 2017;35:552-60. https://doi.org/10.1200/JCO.2016.70.8651.
- Shitara K, Matsuo K, Muro K, Doi T, Ohtsu A. Correlation between overall survival and other endpoints in clinical trials of second-line chemotherapy for patients with advanced gastric cancer. Gastric Cancer 2014;17:362-70. https://doi.org/10.1007/s10120-013-0274-6.
- Shukuya T, Mori K, Amann JM, Bertino EM, Otterson GA, Shields PG, et al. Relationship between overall survival and response or progression-free survival in advanced non-small cell lung cancer patients treated with anti-PD-1/PD-L1 antibodies. J Thorac Oncol 2016;11:1927-39. https://doi.org/10.1016/j.jtho.2016.07.017.
- Siddiqui MK, Tyczynski J, Pahwa A, Fernandes AW. Objective response rate is a possible surrogate endpoint for survival in patients with advanced, recurrent ovarian cancer. Gynecol Oncol 2017;146:44-51. https://doi.org/10.1016/j.ygyno.2017.03.515.
- Sidhu R, Rong A, Dahlberg S. Evaluation of progression-free survival as a surrogate endpoint for survival in chemotherapy and targeted agent metastatic colorectal cancer trials. Clin Cancer Res 2013;19:969-76. https://doi.org/10.1158/1078-0432.CCR-12-2502.
- Tanaka K, Kawano M, Iwasaki T, Itonaga I, Tsumura H. Surrogacy of intermediate endpoints for overall survival in randomized controlled trials of first-line treatment for advanced soft tissue sarcoma in the pre- and post-pazopanib era: a meta-analytic evaluation. BMC Cancer 2019;19. https://doi.org/10.1186/s12885-019-5268-2.
- Tang PA, Bentzen SM, Chen EX, Siu LL. Surrogate end points for median overall survival in metastatic colorectal cancer: literature-based analysis from 39 randomized controlled trials of first-line chemotherapy. J Clin Oncol 2007;25:4562-8. https://doi.org/10.1200/JCO.2006.08.1935.
- Tsujino K, Kawaguchi T, Kubo A, Aono N, Nakao K, Koh Y, et al. Response rate is associated with prolonged survival in patients with advanced non-small cell lung cancer treated with gefitinib or erlotinib. J Thorac Oncol 2009;4:994-1001. https://doi.org/10.1097/JTO.0b013e3181a94a2f.
- Tsujino K, Shiraishi J, Tsuji T, Kurata T, Kawaguchi T, Kubo A, et al. Is response rate increment obtained by molecular targeted agents related to survival benefit in the phase III trials of advanced cancer?. Ann Oncol 2010;21:1668-74.
- Vidaurre T, Wilkerson J, Simon R, Bates SE, Fojo T. Stable disease is not preferentially observed with targeted therapies and as currently defined has limited value in drug development. Cancer J 2009;15:366-73. https://doi.org/10.1097/PPO.0b013e3181b9d37b.
- Wilkerson J, Fojo T. Progression-free survival is simply a measure of a drug’s effect while administered and is not a surrogate for overall survival. Cancer J 2009;15:379-85. https://doi.org/10.1097/PPO.0b013e3181bef8cd.
- Zer A, Prince RM, Amir E, Abdul Razak A. Evolution of randomized trials in advanced/metastatic soft tissue sarcoma: end point selection, surrogacy, and quality of reporting. J Clin Oncol 2016;34:1469-75. https://doi.org/10.1200/JCO.2015.64.3437.
- Zhu R, Lu D, Chu YW, Chai A, Green M, Zhang N, et al. Assessment of correlation between early and late efficacy endpoints to identify potential surrogacy relationships in non-Hodgkin lymphoma: a literature-based meta-analysis of 108 phase II and phase III studies. AAPS J 2017;19:669-81. https://doi.org/10.1208/s12248-017-0056-x.
- Ito K, Miura S, Sakaguchi T, Murotani K, Horita N, Akamatsu H, et al. The impact of high PD-L1 expression on the surrogate endpoints and clinical outcomes of anti-PD-1/PD-L1 antibodies in non-small cell lung cancer. Lung Cancer 2019;128:113-19. https://doi.org/10.1016/j.lungcan.2018.12.023.
- Bujkiewicz S, Achana F, Papanikos T, Riley RD, Abrams KR. NICE DSU Technical Support Document 20: Multivariate Meta-Analysis of Summary Data for Combining Treatment Effects on Correlated Outcomes and Evaluating Surrogate Endpoints. Report by The Decision Support Unit 2019.
- Bujkiewicz S, Jackson D, Thompson JR, Turner RM, Städler N, Abrams KR, et al. Bivariate network meta-analysis for surrogate endpoint evaluation. Stat Med 2019;38:3322-41. https://doi.org/10.1002/sim.8187.
- Murphy P, Claxton L, Hodgson R, Glynn D, Beresford L, Walton M, et al. Exploring heterogeneity in histology-independent technologies and the implications for cost-effectiveness. Med Decis Making 2021;41:165-78. https://doi.org/10.1177/0272989X20980327.
- Phelps CE, Mushlin AI. Focusing technology assessment using medical decision theory. Med Decis Making 1988;8:279-89. https://doi.org/10.1177/0272989X8800800409.
- Coyle D, Buxton MJ, O’Brien BJ. Stratified cost-effectiveness analysis: a framework for establishing efficient limited use criteria. Health Econ 2003;12:421-7. https://doi.org/10.1002/hec.788.
- Espinoza MA, Manca A, Claxton K, Sculpher MJ. The value of heterogeneity for cost-effectiveness subgroup analysis: conceptual framework and application. Med Decis Making 2014;34:951-64. https://doi.org/10.1177/0272989X14538705.
- Basu A, Meltzer D. Value of information on preference heterogeneity and individualized care. Med Decis Making 2007;27:112-27. https://doi.org/10.1177/0272989X06297393.
- Cancer Research UK. Cancer Research UK Policy Statement: Patient Access to Molecular Diagnostics and Targeted Medicines in England. London: Cancer Research UK; 2018.
- Lunn D, Jackson C, Best N, Thomas A, Spiegelhalter D. The BUGS Book. Boca Raton, FL: CRC Press; 2013.
- Sturtz S, Ligges U, Gelman A. R2WinBUGS: a package for running WinBUGS from R. J Stat Softw 2005;12:1-16. www.jstatsoft.org/v2/i03.
- Brooks SP, Gelman A. General methods for monitoring convergence of iterative simulations. J Comput Graph Stat 1998;7:434-55. https://doi.org/10.1080/10618600.1998.10474787.
- Gelman A, Rubin DB. Inferences from iterative simulation using multiple sequences. Stat Sci 1992;7:457-72. https://doi.org/10.1214/ss/1177011136.
- National Institute for Health and Care Excellence (NICE) . Larotrectinib for Treating NTRK Fusion-Positive Advanced Solid Tumours 2019.
- Bongarzone I, Vigneri P, Mariani L, Collini P, Pilotti S, Pierotti MA. RET/NTRK1 rearrangements in thyroid gland tumors of the papillary carcinoma family: correlation with clinicopathological features. Clin Cancer Res 1998;4:223-8.
- Prasad ML, Vyas M, Horne MJ, Virk RK, Morotti R, Liu Z, et al. NTRK fusion oncogenes in pediatric papillary thyroid carcinoma in northeast United States. Cancer 2016;122:1097-107. https://doi.org/10.1002/cncr.29887.
- Vokuhl C, Nourkami-Tutdibi N, Furtwängler R, Gessler M, Graf N, Leuschner I. ETV6–NTRK3 in congenital mesoblastic nephroma: a report of the SIOP/GPOH nephroblastoma study. Pediatr Blood Cancer 2018;65. https://doi.org/10.1002/pbc.26925.
- Phillippo DM, Ades AE, Dias S, Palmer S, Abrams KR, Welton NJ. NICE DSU Technical Support Document 18: Methods for Population-adjusted Indirect Comparisons in Submissions to NICE. Sheffield: ScHARR, University of Sheffield; 2016.
- Hatswell AJ, Thompson GJ, Maroudas PA, Sofrygin O, Delea TE. Estimating outcomes and cost effectiveness using a single-arm clinical trial: ofatumumab for double-refractory chronic lymphocytic leukemia. Cost Eff Resour Alloc 2017;15. https://doi.org/10.1186/s12962-017-0071-x.
- Hatswell AJ, Sullivan WG. Creating historical controls using data from a previous line of treatment – two non-standard approaches. Stat Methods Med Res 2020;29:1563-72. https://doi.org/10.1177/0962280219826609.
- Hsiao SJ, Zehir A, Sireci AN, Aisner DL. Detection of tumor NTRK gene fusions to identify patients who may benefit from tyrosine kinase (TRK) inhibitor therapy. J Mol Diagn 2019;21:553-71. https://doi.org/10.1016/j.jmoldx.2019.03.008.
- NHS England . National Genomic Test Directory 2019. www.england.nhs.uk/publication/national-genomic-test-directories/ (accessed 15 May 2019).
- Vogelstein B, Kinzler KW. Cancer genes and the pathways they control. Nat Med 2004;10:789-99. https://doi.org/10.1038/nm1087.
- Morganti S, Tarantino P, Ferraro E, D’Amico P, Viale G, Trapani D, et al. Complexity of genome sequencing and reporting: next generation sequencing (NGS) technologies and implementation of precision medicine in real life. Crit Rev Oncol Hematol 2019;133:171-82. https://doi.org/10.1016/j.critrevonc.2018.11.008.
- Eifert C, Powers RS. From cancer genomes to oncogenic drivers, tumour dependencies and therapeutic targets. Nat Rev Cancer 2012;12:572-8. https://doi.org/10.1038/nrc3299.
- Sheikine Y, Kuo FC, Lindeman NI. Clinical and technical aspects of genomic diagnostics for precision oncology. J Clin Oncol 2017;35:929-33. https://doi.org/10.1200/JCO.2016.70.7539.
- Solomon JP, Hechtman JF. Detection of NTRK fusions: merits and limitations of current diagnostic platforms. Cancer Res 2019;79:3163-8. https://doi.org/10.1158/0008-5472.CAN-19-0372.
- Penault-Llorca F, Rudzinski ER, Sepulveda AR. Testing algorithm for identification of patients with TRK fusion cancer. J Clin Pathol 2019;72:460-7. https://doi.org/10.1136/jclinpath-2018-205679.
- Wright C, Gray S. Epigenetic Cancer Therapy. London: Academic Press; 2015.
- Marchiò C, Scaltriti M, Ladanyi M, Iafrate AJ, Bibeau F, Dietel M, et al. ESMO recommendations on the standard methods to detect NTRK fusions in daily practice and clinical research. Ann Oncol 2019;30:1417-27. https://doi.org/10.1093/annonc/mdz204.
- Sigal DS, Bhangoo MS, Hermel JA, Pavlick DC, Frampton G, Miller VA, et al. Comprehensive genomic profiling identifies novel NTRK fusions in neuroendocrine tumors. Oncotarget 2018;9:35809-12. https://doi.org/10.18632/oncotarget.26260.
- Okamura R, Boichard A, Kato S, Sicklick JK, Bazhenova L, Kurzrock R. Analysis of NTRK alterations in pan-cancer adult and pediatric malignancies: implications for NTRK-targeted therapeutics. JCO Precis Oncol 2018;2018. https://doi.org/10.1200/PO.18.00183.
- U.S. Food and Drug Administration (FDA), Center for Drug Evaluation and Research . NDA Multidisciplinary Review and Evaluation NDA 210861 and NDA 211710 VITRAKVI (Larotrectinib) 2016.
- Amatu A, Sartore-Bianchi A, Siena S. NTRK gene fusions as novel targets of cancer therapy across multiple tumour types. ESMO Open 2016;1. https://doi.org/10.1136/esmoopen-2015-000023.
- Williams HL, Walsh K, Diamond A, Oniscu A, Deans ZC. Validation of the Oncomine™ focus panel for next-generation sequencing of clinical tumour samples. Virchows Arch 2018;473:489-503. https://doi.org/10.1007/s00428-018-2411-4.
- Solomon JP, Linkov I, Rosado A, Mullaney K, Rosen EY, Frosina D, et al. NTRK fusion detection across multiple assays and 33,997 cases: diagnostic implications and pitfalls. Mod Pathol 2020;33:38-46. https://doi.org/10.1038/s41379-019-0324-7.
- Makretsov N, He M, Hayes M, Chia S, Horsman DE, Sorensen PH, et al. A fluorescence in situ hybridization study of ETV6–NTRK3 fusion gene in secretory breast carcinoma. Genes Chromosomes Cancer 2004;40:152-7. https://doi.org/10.1002/gcc.20028.
- Scottish Science Advisory Council (SSAC) . Informing the Future of Genomic Medicine in Scotland 2019.
- Canadian Agency for Drugs and Techonologies in Health (CADTH) . Larotrectinib (Vitrakvi) for Neurotrophic Tyrosine Receptor Kinase (NTRK) Positive Solid Tumours: Pan-Canadian Oncology Drug Review. Final Economic Guidance Report 2019.
- Woods B, Sideris E, Palmer S, Latimer N, Soares M. Partitioned Survival Analysis for Decision Modelling in Health Care: A Critical Review. Sheffield: Decision Support Unit, ScHARR, University of Sheffield; 2017.
- Latimer NJ. NICE DSU Technical Support Document 14: Survival Analysis for Economic Evaluations alongside Clinical Trials - Extrapolation with Patient-level Data. Sheffield: Decision Support Unit, ScHARR, University of Sheffield; 2011.
- Ouwens MJNM, Mukhopadhyay P, Zhang Y, Huang M, Latimer N, Briggs A. Estimating lifetime benefits associated with immuno-oncology therapies: challenges and approaches for overall survival extrapolations. PharmacoEconomics 2019;37:1129-38. https://doi.org/10.1007/s40273-019-00806-4.
- Price MJ, Welton NJ, Briggs AH, Ades AE. Model averaging in the presence of structural uncertainty about treatment effects: influence on treatment decision and expected value of information. Value Health 2011;14:205-18. https://doi.org/10.1016/j.jval.2010.08.001.
- Jackson CH, Bojke L, Thompson SG, Claxton K, Sharples LD. A framework for addressing structural uncertainty in decision models. Med Decis Making 2011;31:662-74. https://doi.org/10.1177/0272989X11406986.
- Australian Government Department of Health . Co-Dependent and Hybrid Technologies 2017. www1.health.gov.au/internet/hta/publishing.nsf/Content/co-1 (accessed 17 January 2020).
- Soares MO, Walker S, Palmer SJ, Sculpher MJ. Establishing the value of diagnostic and prognostic tests in health technology assessment. Med Decis Making 2018;38:495-508. https://doi.org/10.1177/0272989X17749829.
- Albert CM, Davis JL, Federman N, Casanova M, Laetsch TW. TRK fusion cancers in children: a clinical review and recommendations for screening. J Clin Oncol 2019;37:513-24. https://doi.org/10.1200/JCO.18.00573.
- Stinnett AA, Mullahy J. Net health benefits: a new framework for the analysis of uncertainty in cost-effectiveness analysis. Med Decis Making 1998;18:68-80. https://doi.org/10.1177/0272989X98018002S09.
- Briggs A, Claxton K, Sculpher MJ. Decision Modelling for Health Economic Evaluation. Oxford: Oxford University Press; 2006.
- Hettle R, Corbett M, Hinde S, Hodgson R, Jones-Diette J, Woolacott N, et al. The assessment and appraisal of regenerative medicines and cell therapy products: an exploration of methods for review, economic evaluation and appraisal. Health Technol Assess 2017;21. https://doi.org/10.3310/hta21070.
- Grimm S, Strong M, Brennan A, Wailoo A. Framework for Analysing Risk in Health Technology Assessments and Its Application to Managed Entry Agreements. Report by the Decision Support Unit 2016.
- Claxton K. The irrelevance of inference: a decision-making approach to the stochastic evaluation of health care technologies. J Health Econ 1999;18:341-64. https://doi.org/10.1016/S0167-6296(98)00039-3.
- Claxton K, Palmer S, Longworth L, Bojke L, Griffin S, Soares M, et al. A comprehensive algorithm for approval of health technologies with, without, or only in research: the key principles for informing coverage decisions. Value Health 2016;19:885-91. https://doi.org/10.1016/j.jval.2016.03.2003.
- Ades AE, Lu G, Claxton K. Expected value of sample information calculations in medical decision modeling. Med Decis Making 2004;24:207-27. https://doi.org/10.1177/0272989X04263162.
- NHS England Cancer Drugs Fund Team . Appraisal and Funding of Cancer Drugs from July 2016 (Including the New Cancer Drugs Fund): A New Deal for Patients, Taxpayers and Industry 2016.
- Claxton K. Pharmaceutical Pricing: Early Access, The Cancer Drugs Fund and the Role of NICE. York: Centre for Health Economics, University of York; 2016.
- Grieve R, Abrams K, Claxton K, Goldacre B, James N, Nicholl J, et al. Cancer Drugs Fund requires further reform. BMJ 2016;354. https://doi.org/10.1136/bmj.i5090.
- Morrell L, Wordsworth S, Schuh A, Middleton MR, Rees S, Barker RW. Will the reformed Cancer Drugs Fund address the most common types of uncertainty? An analysis of NICE cancer drug appraisals. BMC Health Serv Res 2018;18. https://doi.org/10.1186/s12913-018-3162-2.
- Strong M, Oakley JE, Brennan A. Estimating multiparameter partial expected value of perfect information from a probabilistic sensitivity analysis sample: a nonparametric regression approach. Med Decis Making 2014;34:311-26. https://doi.org/10.1177/0272989X13505910.
- Strong M, Oakley JE, Brennan A, Breeze P. Estimating the expected value of sample information using the probabilistic sensitivity analysis sample: a fast, nonparametric regression-based method. Med Decis Making 2015;35:570-83. https://doi.org/10.1177/0272989X15575286.
- Willan AR, Pinto EM. The value of information and optimal clinical trial design. Stat Med 2005;24:1791-806. https://doi.org/10.1002/sim.2069.
- National Institute for Health and Care Excellence (NICE) . Guide to the Processes of Technology Appraisal 2018.
- Kim DD, Basu A. New metrics for economic evaluation in the presence of heterogeneity: focusing on evaluating policy alternatives rather than treatment alternatives. Med Decis Making 2017;37:930-41. https://doi.org/10.1177/0272989X17702379.
- Drummond MF, Sculpher MJ, Claxton K, Stoddart GL, Torrance GW. Methods for the Economic Evaluation of Health Care Programmes. Oxford: Oxford University Press; 2015.
- Claxton K. OFT, VBP: QED?. Health Econ 2007;16:545-58.
- Rothery C, Claxton K, Palmer S, Epstein D, Tarricone R, Sculpher M. Characterising uncertainty in the assessment of medical devices and determining future research needs. Health Econ 2017;26:109-23. https://doi.org/10.1002/hec.3467.
- Kennedy-Martin T, Curtis S, Faries D, Robinson S, Johnston J. A literature review on the representativeness of randomized controlled trial samples and implications for the external validity of trial results. Trials 2015;16. https://doi.org/10.1186/s13063-015-1023-4.
- National Institute for Health and Care Excellence (NICE) . Pembrolizumab With Carboplatin and Paclitaxel for Untreated Metastatic Squamous Non-Small-Cell Lung Cancer 2019.
- National Institute for Health and Care Excellence (NICE) . Pembrolizumab for Untreated PD-L1-Positive Locally Advanced or Metastatic Urothelial Cancer When Cisplatin Is Unsuitable 2018.
- National Institute for Health and Care Excellence (NICE) . Pembrolizumab for Untreated Metastatic or Unresectable Recurrent Squamous Cell Head and Neck Cancer 2020. www.nice.org.uk/guidance/TA661 (accessed 20 February 2020).
- European Medicines Agency . Workshop on Site and Histology – Independent Indications of Oncology 2018. www.ema.europa.eu/en/events/workshop-site-histology-independent-indications-oncology (accessed October 2021).
- European Medicines Agency . Workshop on Single-Arm Studies in Oncology 2016. www.ema.europa.eu/en/events/workshop-single-arm-trials-oncology (accessed October 2021).
- US Food and Drug Administration . Developing Targeted Therapies in Low-Frequency Molecular Subsets of a Disease 2018. www.fda.gov/downloads/Drugs/GuidanceComplianceRegulatoryInformation/Guidances/UCM588884.pdf (accessed October 2021).
- US Food and Drug Administration . Master Protocols: Efficient Clinical Trial Design Strategies to Expedite Development of Oncology Drugs and Biologics 2018. www.fda.gov/downloads/Drugs/GuidanceComplianceRegulatoryInformation/Guidances/UCM621817.pdf (accessed October 2021).
- US Department of Health and Human Services, U.S. Food and Drug Administration, Center for Drug Evaluation and Research, Center for Biologics Evaluation and Research . Guidance for Industry: Expedited Programs for Serious Conditions – Drugs and Biologics 2014. www.fda.gov/downloads/Drugs/Guidances/UCM358301.pdf (accessed October 2021).
- Food and Drug Administration . Table of Surrogate Endpoints That Were the Basis of Drug Approval or Licensure n.d. www.fda.gov/drugs/development-resources/table-surrogate-endpoints-were-basis-drug-approval-or-licensure (accessed 17 January 2020).
- Food and Drug Administration (FDA) . Clinical Trial Endpoints for the Approval of Cancer Drugs and Biologics Guidance for Industry 2018. www.fda.gov/regulatory-information/search-fda-guidance-documents/clinical-trial-endpoints-approval-cancer-drugs-and-biologics (accessed 17 January 2020).
- US Food and Drug Administration . Tissue Agnostic Therapies in Oncology: Regulatory Considerations for Orphan Drug Designation n.d. www.fda.gov/downloads/NewsEvents/MeetingsConferencesWorkshops/UCM598186.pdf (accessed October 2021).
- European Medicines Agency . Essential Considerations for Successful Qualification of Novel Methodologies 2017. www.ema.europa.eu/documents/other/essential-considerations-successful-qualification-novel-methodologies_en.pdf (accessed October 2021).
- European Medicines Agency . Biostatistics 2021. www.ema.europa.eu/en/human-regulatory/research-development/scientific-guidelines/clinical-efficacy-safety/biostatistics (accessed October 2021).
- European Medicines Agency . Predictive Biomarker-Based Assay Development in the Context of Drug Development and Lifecycle 2017. www.ema.europa.eu/en/predictive-biomarker-based-assay-development-context-drug-development-lifecycle (accessed October 2021).
- European Medicines Agency . Guideline on the Evaluation of Anticancer Medicinal Products in Man Rev. 5 2017. www.ema.europa.eu/documents/scientific-guideline/guideline-evaluation-anticancer-medicinal-products-man-revision-5_en.pdf (accessed October 2021).
- European Medicines Agency . Appendix 4 to the Guideline on the Evaluation of Anticancer Medicinal Products in Man 2015. www.ema.europa.eu/documents/scientific-guideline/evaluation-anticancer-medicinal-products-man-appendix-4-condition-specific-guidance-rev2_en.pdf (accessed October 2021).
- National Institute for Health and Care Excellence (NICE) . Ceritinib for Previously Treated Anaplastic Lymphoma Kinase Positive Non-Small-Cell Lung Cancer. Technology Appraisal Guidance [TA395] 2016.
- Kim DW, Mehra R, Tan DS, Felip E, Chow LQ, Camidge DR, et al. Activity and safety in ceritinib in patients with ALK-rearranged non-small-cell lung cancer (ASCEND-1): updated results from the multicentre, open-label, phase 1 trial. Lancet Oncol 2016;17:452-63. https://doi.org/10.1016/S1470-2045(15)00614-2.
- Crinò L, Ahn MJ, De Marinis F, Groen HJ, Wakelee H, Hida T, et al. Multicenter phase II study of whole-body and intracranial activity with ceritinib in patients with ALK-rearranged non-small-cell lung cancer previously treated with chemotherapy and crizotinib: results from ASCEND-2. J Clin Oncol 2016;34:2866-78. https://doi.org/10.1200/JCO.2015.65.5936.
- National Institute for Health and Care Excellence (NICE) . Osimertinib for Treating Locally Advanced or Metastatic EGFR T790M Mutation-Positive Non-Small-Cell Lung Cancer 2016.
- Yang JC, Ahn MJ, Kim DW, Ramalingam SS, Sequist LV, Su WC, et al. Osimertinib in pretreated T790M-positive advanced non-small-cell lung cancer: AURA study phase II extension component. J Clin Oncol 2017;35:1288-96. https://doi.org/10.1200/JCO.2016.70.3223.
- Goss G, Tsai CM, Shepherd FA, Bazhenova L, Lee JS, Chang GC, et al. Osimertinib for pretreated EGFR Thr790Met-positive advanced non-small-cell lung cancer (AURA2): a multicentre, open-label, single-arm, phase 2 study. Lancet Oncol 2016;17:1643-52. https://doi.org/10.1016/S1470-2045(16)30508-3.
- Ahn MJ, Tsai CM, Shepherd FA, Bazhenova L, Sequist LV, Hida T, et al. Osimertinib in patients with T790M mutation-positive, advanced non–small cell lung cancer: long-term follow-up from a pooled analysis of 2 phase 2 studies. Cancer 2019;125:892-901. https://doi.org/10.1002/cncr.31891.
- National Institute for Health and Care Excellence (NICE) . Nivolumab for Treating Relapsed or Refractory Classical Hodgkin Lymphoma 2017.
- Younes A, Santoro A, Shipp M, Zinzani PL, Timmerman JM, Ansell S, et al. Nivolumab for classical Hodgkin's lymphoma after failure of both autologous stem-cell transplantation and brentuximab vedotin: a multicentre, multicohort, single-arm phase 2 trial. Lancet Oncol 2016;17:1283-94. https://doi.org/10.1016/S1470-2045(16)30167-X.
- Lesokhin AM, Ansell SM, Arman P, Scott EC, Halwani A, Gutierrez M, et al. Nivolumab in patients with relapsed or refractory hematologic malignancy: preliminary results of a phase 1b study. J Clin Oncol 2016;34:2698-704. https://doi.org/10.1200/JCO.2015.65.9789.
- National Institute for Health and Care Excellence (NICE) . Venetoclax for Treating Chronic Lymphocytic Leukaemia 2017.
- Davids MS, Roberts AW, Seymour JK, Pagel JM, Kahl BS, Wierda WG, et al. Phase I first-in-human study of venetoclax in patients with relapsed or refractory non-Hodgkin lymphoma. J Clin Oncol 2017;35:826-33. https://doi.org/10.1200/JCO.2016.70.4320.
- Stilgenbauer S, Eichhorst B, Schetelig J, Coutre S, Seymour JF, Munir T, et al. Venetoclax in relapsed or refractory chronic lymphocytic leukaemia with 17p deletion: a multicentre, open-label, phase 2 study. Lancet Oncol 2016;17:768-78. https://doi.org/10.1016/S1470-2045(16)30019-5.
- Stilgenbauer S, Eichhorst B, Schetelig J, Hillmen P, Seymour JF, Coutre S, et al. Venetoclax for patients with chronic lymphocytic leukemia with 17p deletion: results from the full population of a phase II pivotal trial. J Clin Oncol 2018;36:1973-80. https://doi.org/10.1200/JCO.2017.76.6840.
- Coutre S, Choi M, Furman RR, Eradat H, Heffner L, Jones JA, et al. Venetoclax for patients with chronic lymphocytic leukemia who progressed during or after idelalisib therapy. Blood 2018;131:1704-11. https://doi.org/10.1182/blood-2017-06-788133.
- Jones JA, Mato AR, Wierda WG, Davids MS, Choi M, Cheson BD, et al. Venetoclax for chronic lymphocytic leukaemia progressing after ibrutinib: an interim analysis of a multicentre, open-label, phase 2 trial. Lancet Oncol 2018;19:65-7. https://doi.org/10.1016/S1470-2045(17)30909-9.
- National Institute for Health and Care Excellence (NICE) . Atezolizumab for Untreated PD-L1-Positive Locally Advanced or Metastatic Urothelial Cancer When Cisplatin Is Unsuitable 2018.
- Balar AV, Galsky MD, Rosenberg JE, Powles T, Petrylak DP, Bellmunt J, et al. Atezolizumab as first-line treatment in cisplatin-ineligible patients with locally advanced and metastatic urothelial carcinoma: a single-arm, multicentre, phase 2 trial. Lancet Oncol 2017;389:67-76. https://doi.org/10.1016/S0140-6736(16)32455-2.
- Necchi A, Joseph RW, Loriot Y, Hoffman-Censits J, Perez-Gracia JL, Petrylak DP, et al. Atezolizumab in platinum-treated locally advanced or metastatic urothelial carcinoma: post-progression outcomes from the phase II IMvigor210 study. Ann Oncol 2017;28:3044-50. https://doi.org/10.1093/annonc/mdx518.
- Rosenberg JE, Hoffman-Censits J, Powles T, van der Heijden MS, Balar AV, Necchi A, et al. Atezolizumab in patients with locally advanced and metastatic urothelial carcinoma who have progressed following treatment with platinum-based chemotherapy: a single-arm, multicentre, phase 2 trial. Lancet 2016;387:1909-20. https://doi.org/10.1016/S0140-6736(16)00561-4.
- National Institute for Health and Care Excellence (NICE) . Daratumumab Monotherapy for Treating Relapsed and Refractory Multiple Myeloma 2018.
- Lonial S, Weiss BM, Usmani SZ, Singhai S, Chari A, Bahlis NJ, et al. Daratumumab monotherapy in patients with treatment-refractory multiple myeloma (SIRIUS): an open-label, randomised, phase 2 trial. Lancet 2016;387:1551-60. https://doi.org/10.1016/S0140-6736(15)01120-4.
- Lokhorst HM, Plesner T, Laubach JP, Nahi H, Gimsing P, Hansson M, et al. Targeting CD38 with daratumumab monotherapy in multiple myeloma. New Engl J Med 2015;373:1207-19. https://doi.org/10.1056/NEJMoa1506348.
- Usmani SZ, Weiss BM, Plesner T, Bahlis NJ, Belch A, Lonial S, et al. Clinical efficacy of daratumumab monotherapy in patients with heavily pretreated relapsed or refractory multiple myeloma. Blood 2016;128:37-44. https://doi.org/10.1182/blood-2016-03-705210.
- National Institute for Health and Care Excellence (NICE) . Avelumab for Treating Metastatic Merkel Cell Carcinoma 2017.
- Kaufman HL, Russell J, Hamid O, Bhatia S, Terheyden P, D’Angelo SP, et al. Avelumab in patients with chemotherapy-refractory metastatic Merkel cell carcinoma: a multicentre, single-group, open-label, phase 2 trial. Lancet Oncol 2016;17:1374-85. https://doi.org/10.1016/S1470-2045(16)30364-3.
- D’Angelo SP, Russell J, Lebbé C, Chmielowski B, Gambichler T, Grob JJ, et al. Efficacy and safety of first-line avelumab treatment in patients with stage IV metastatic Merkel cell carcinoma: a preplanned interim analysis of a clinical trial. JAMA Oncol 2018;4. https://doi.org/10.1001/jamaoncol.2018.0077.
- National Institute for Health and Care Excellence (NICE) . Crizotinib for Treating ROS1-Positive Advanced Non-Small-Cell Lung Cancer 2018.
- Shaw AT, Ou SHI, Bang YJ, Camidge DR, Solomon BJ, Salgia R, et al. Crizotinib in ROS1-rearranged non–small-cell lung cancer. New Engl J Med 2014;371:1963-71. https://doi.org/10.1056/NEJMoa1406766.
- Shaw AT, Riely GJ, Bang YJ, Kim DW, Camidge DR, Solomon BJ, et al. Crizotinib in ROS1-rearranged advanced non-small-cell lung cancer (NSCLC): updated results, including overall survival, from PROFILE 1001. Ann Oncol 2019;30:1121-6. https://doi.org/10.1093/annonc/mdz131.
- National Institute for Health and Care Excellence (NICE) . Pembrolizumab for Treating Relapsed or Refractory Classical Hodgkin Lymphoma 2018.
- Chen R, Zinzani PL, Fanale MA, Armand P, Johnson NA, Brice P, et al. Phase II study of the efficacy and safety of pembrolizumab for relapsed/refractory classic Hodgkin lymphoma. J Clin Oncol 2017;35:2125-32. https://doi.org/10.1200/JCO.2016.72.1316.
- National Institute for Health and Care Excellence (NICE) . Brigatinib for Treating ALK-Positive Non-Small-Cell Lung Cancer After Crizotinib 2019.
- Kim DW, Tiseo M, Ahn MJ, Reckamp KL, Hansen KH, Kim SW, et al. Brigatinib in patients with crizotinib-refractory anaplastic lymphoma kinase-positive non-small-cell lung cancer: a randomized, multicenter phase II trial. J Clin Oncol 2017;35:2490-8. https://doi.org/10.1200/JCO.2016.71.5904.
- Gettinger SN, Bazhenova LA, Langer CJ, Salgia R, Gold KA, Rosell R, et al. Activity and safety of brigatinib in ALK-rearranged non-small-cell lung cancer and other malignancies: a single-arm, open-label, phase 1/2 trial. Lancet Oncol 2016;17:1683-96. https://doi.org/10.1016/S1470-2045(16)30392-8.
- Hatswell AJ, Freemantle N, Baio G. Economic evaluations of pharmaceuticals granted a marketing authorisation without the results of randomised trials: a systematic review and taxonomy. PharmacoEconomics 2017;35:163-76. https://doi.org/10.1007/s40273-016-0460-6.
- Faria R, Hernandez Alava M, Manca A, Wailoo AJ. The Use of Observational Data to Inform Estimates of Treatment Effectiveness for Technology Appraisal: Methods for Comparative Individual Patient Data. Report by the Decision Support Unit 2015.
- Bell H, Wailoo A, Hernandez M, Grieve R, Faria R, Gibson L, et al. The Use of Real World Data for the Estimation of Treatment Effects in NICE Decision Making. Report by the Decision Support Unit 2016.
- Wright SJ, Newman WG, Payne K. Accounting for capacity constraints in economic evaluations of precision medicine: a systematic review. PharmacoEconomics 2019;37:1011-27. https://doi.org/10.1007/s40273-019-00801-9.
- Marmor S, Portschy PR, Tuttle TM, Virnig BA. The rise in appendiceal cancer incidence: 2000–2009. J Gastrointest Surg 2015;19:743-50. https://doi.org/10.1007/s11605-014-2726-7.
- World Population Review . Western European Countries 2020 2019. http://worldpopulationreview.com/countries/western-european-countries/ (accessed 12 December 2019).
- Office for National Statistics . Cancer Registration Statistics, England: 2017 2019. www.ons.gov.uk/releases/cancerregistrationstatisticsengland2017 (accessed June 2019).
- Cancer Research UK. Breast Cancer Incidence by Stage at Diagnosis. London: Cancer Research UK; 2014.
- Coombes N, Elliss-Brookes L, Johnson S, Lyons J, Mackay C, Morement H, et al. National Cancer Intelligence Network. Rare and Less Common Cancers: Incidence and Mortality in England, 2010 to 2013. London: Public Health England; 2015.
- Tsuchiya N, Sawada Y, Endo I, Saito K, Uemura Y, Nakatsura T. Biomarkers for the early diagnosis of hepatocellular carcinoma. World J Gastroenterol 2015;21:10573-83. https://doi.org/10.3748/wjg.v21.i37.10573.
- Thrumurthy SG, Thrumurthy SS, Gilbert CE, Ross P, Haji A. Colorectal adenocarcinoma: risks, prevention and diagnosis. BMJ 2016;354. https://doi.org/10.1136/bmj.i3590.
- Cancer Research UK. Bowel Cancer Incidence by Stage at Diagnosis. London: Cancer Research UK; 2016.
- Starczewska Amelio JM, Cid Ruzafa J, Desai K, Tzivelekis S, Muston D, Khalid JM, et al. Prevalence of gastrointestinal stromal tumour (GIST) in the United Kingdom at different therapeutic lines: an epidemiologic model. BMC Cancer 2014;14. https://doi.org/10.1186/1471-2407-14-364.
- PDQ Adult Treatment Editorial Board . Gastrointestinal Stromal Tumors Treatment (Adult) (PDQ®): Patient Version 2020. https://www.ncbi.nlm.nih.gov/books/NBK65929/ (accessed November 2021).
- Orbach D, Rey A, Cecchetto G, Oberlin O, Casanova M, Thebaud E, et al. Infantile fibrosarcoma: management based on the European experience. J Clin Oncol 2010;28:318-23. https://doi.org/10.1200/JCO.2009.21.9972.
- Skálová A, Vanecek T, Sima R, Laco J, Weinreb I, Perez-Ordonez B, et al. Mammary analogue secretory carcinoma of salivary glands, containing the ETV6–NTRK3 fusion gene: a hitherto undescribed salivary gland tumor entity. Am J Surg Pathol 2010;34:599-608. https://doi.org/10.1097/PAS.0b013e3181d9efcc.
- Luk PP, Selinger CI, Eviston TJ, Lum T, Yu B, O’Toole SA, et al. Mammary analogue secretory carcinoma: an evaluation of its clinicopathological and genetic characteristics. Pathology 2015;47:659-66. https://doi.org/10.1097/PAT.0000000000000322.
- Sethi R, Kozin E, Remenschneider A, Meier J, VanderLaan P, Faquin W, et al. Mammary analogue secretory carcinoma: update on a new diagnosis of salivary gland malignancy. Laryngoscope 2014;124:188-95. https://doi.org/10.1002/lary.24254.
- Cancer Research UK. Melanoma Skin Cancer Incidence by Stage at Diagnosis. London: Cancer Research UK; 2016.
- Care Quality Improvement Department, Royal College of Physicians . National Lung Cancer Audit Annual Report 2018 (for the Audit Period 2017) 2019.
- Pancreatic Cancer UK. Types of Pancreatic Cancer. London: Pancreatic Cancer UK; 2018.
- Cancer Research UK. Pancreatic Cancer Incidence by Stage at Diagnosis. London: Cancer Research UK; 2016.
- Cancer Research UK. Soft Tissue Sarcoma Incidence Statistics 2010. www.cancerresearchuk.org/health-professional/cancer-statistics/statistics-by-cancer-type/soft-tissue-sarcoma/incidence#heading-Four (accessed 27 February 2019).
- American Cancer Society . Cancer Facts and Figures 2017. www.cancer.org/research/cancer-facts-statistics/all-cancer-facts-figures/cancer-facts-figures-2017.html (accessed 24 January 2020).
- Deen MH, Burke KM, Janitz A, Campbell J. Cancers of the thyroid: overview and statistics in the United States and Oklahoma. J Okla State Med Assoc 2016;109:333-8.
- Cancer Research UK. Cervical Cancer Incidence by Stage at Diagnosis. London: Cancer Research UK; 2016.
- Gooskens SL, Houwing ME, Vujanic GM, Dome JS, Diertens T, Coulomb-l’Herminé A, et al. Congenital mesoblastic nephroma 50 years after its recognition: a narrative review. Pediatr Blood Cancer 2017;64. https://doi.org/10.1002/pbc.26437.
- National Institute for Health and Care Excellence (NICE) . Pembrolizumab for Previously Treated Oesophageal or Gastrooesophageal Junction Cancer Draft Scope 2018.
- Cancer Research UK. Oesophageal Cancer Incidence by Stage at Diagnosis. London: Cancer Research UK; 2016.
- Cancer Research UK. Head and Neck Cancers Statistics 2016. www.cancerresearchuk.org/health-professional/cancer-statistics/statistics-by-cancer-type/head-and-neck-cancers (accessed June 2019).
- Public Health England . Glioblastoma in England 2007-2011 2016.
- Wang Y, Jiang T. Understanding high grade glioma: molecular mechanism, therapy and comprehensive management. Cancer Lett 2013;331:139-46. https://doi.org/10.1016/j.canlet.2012.12.024.
- UK and Ireland Neuroendcrine Tumour Society (UKI NETS) . Incidence and Prevalence of Neuroendocrine Tumours in England 2017.
- Cancer Research UK . Ovarian Cancer Incidence by Stage at Diagnosis 2016. www.cancerresearchuk.org/health-professional/cancer-statistics/statistics-by-cancer-type/ovarian-cancer/incidence (accessed June 2019).
- Farrimond J. Analysis of the Cancer Registry Combined Database for Use with the Brain and CNS Registry. London: National Cancer Intelligence Network, Eastern Cancer Registration and Information Centre; 2010.
- Cancer Research UK. Children’s Cancer Incidence Statistics. London: Cancer Research UK; 2014.
- Austin MT, Xing Y, Hayes-Jordan AA, Lally KP, Cormier JN. Melanoma incidence rises for children and adolescents: an epidemiologic review of pediatric melanoma in the United States. J Pediatr Surg 2013;48:2207-13. https://doi.org/10.1016/j.jpedsurg.2013.06.002.
- Brzeziańska E, Karbownik M, Migdalska-Sek M, Pastuszak-Lewandoska D, Włoch J, Lewiński A. Molecular analysis of the RET and NTRK1 gene rearrangements in papillary thyroid carcinoma in the Polish population. Mutat Res 2006;599:26-35. https://doi.org/10.1016/j.mrfmmm.2005.12.013.
- Cancer Research UK . Prostate Cancer Incidence by Stage at Diagnosis 2016. www.cancerresearchuk.org/health-professional/cancer-statistics/statistics-by-cancer-type/prostate-cancer/incidence#heading-Three (accessed June 2019).
- Cancer Research UK. Kidney Cancer: Stages, Types and Grades. London: Cancer Research UK; 2016.
- Horowitz DP, Sharma CS, Connolly E, Gidea-Addeo D, Deutsch I. Secretory carcinoma of the breast: results from the survival, epidemiology and end results database. Breast 2012;21:350-3. https://doi.org/10.1016/j.breast.2012.02.013.
- Jacob JD, Hodge C, Franko J, Pezzi CM, Goldman CD, Klimberg VS. Rare breast cancer: 246 invasive secretory carcinomas from the National Cancer Data Base. J Surg Oncol 2016;113:721-5. https://doi.org/10.1002/jso.24241.
- Rushton L, Bagga S, Bevan R, Brown T, Cherrie J, Holmes P, et al. The Burden of Occupational Cancer in Great Britain. London: Health & Safety Executive; 2010.
- Cancer Research UK . Uterine Cancer Incidence By Stage At Diagnosis 2016. www.cancerresearchuk.org/health-professional/cancer-statistics/statistics-by-cancer-type/uterine-cancer/incidence#heading-Three (accessed June 2019).
- Lee SJ, Kim NKD, Lee S-H, Kim ST, Park SH, Park JO, et al. NTRK gene amplification in patients with metastatic cancer. Precision Future Med 2017;1:129-37. https://doi.org/10.23838/pfm.2017.00142.
- Lange AM, Lo HW. Inhibiting TRK proteins in clinical cancer therapy. Cancers 2018;10.
- National Cancer Institute . Gastrointestinal Stromal Tumors Treatment (Adult) (PDQ®)–Health Professional Version 2018. www.cancer.gov/types/soft-tissue-sarcoma/hp/gist-treatment-pdq (accessed June 2019).
- Demetri GD, Paz-Ares L, Farago AF, Liu SV, Chawla SP, Tosi D, et al. Efficacy and safety of entrectinib in patients with NTRK fusion-positive tumours: pooled analysis of STARTRK-2, STARTRK-1, and ALKA-372-001. Ann Oncol 2018;29. https://doi.org/10.1093/annonc/mdy483.003.
- Hyman D, van Tilburg C, Albert C, Tan D, Geoerger B, Farago A, et al. 445PD Durability of response with larotrectinib in adult and pediatric patients with TRK fusion cancer. Ann Oncol 2019;30:v162-3. https://doi.org/10.1093/annonc/mdz244.007.
- Georghiou T, Bardsley M. Exploring the Cost of End of Life Care. London: Nuffield Trust; 2014.
Appendix 1 List of regulatory sources
-
Workshop on site and histology-independent indications in oncology. 215
-
Workshop on single-arm studies in oncology. 216
-
Developing targeted therapies in low-frequency molecular subsets of a disease guidance for industry. 217
-
Master protocols: efficient clinical trial design strategies to expedite development of oncology drugs and biologics guidance for industry. 218
-
Guidance for industry-expedited programmes for serious conditions – drugs and biologics. 219
-
Table of surrogate end points that were the basis of drug approval or licensure. 220
-
Guidance for Industry Clinical Trial Endpoints for the Approval of Cancer Drugs and Biologics. 221
-
Tissue agnostic therapies in oncology. Regulatory considerations for orphan drug designation. 222
-
Essential considerations for successful qualification of novel methodologies. 223
-
Scientific guidelines on biostatistics (e.g. investigation of subgroups in clinical trials, multiplicity issues in clinical trials, extrapolation of efficacy and safety in medicine development, methodological issues in confirmatory clinical trials planned with an adaptive design). 224
-
Predictive biomarker-based assay development in the context of drug development and lifecycle. 225
-
Guideline on the evaluation of anticancer medicinal products in man. 226
-
Appendix 4 to the guideline on the evaluation of anticancer medicinal products in man. 227
Appendix 2 Summary of trials
Product | Indications | Clinical evidence | |||
---|---|---|---|---|---|
Study | Clinical outcomes | Study design | Patient population | ||
Merestinib (Eli Lilly, Indianapolis, IN, USA) | Solid tumours | NCT02920996 | Primary: 1. ORR (up to 2 years) (MET cohort) Secondary:1. OS rate (up to 2 years) (MET cohort) 2. PFS rate (2 years) (MET cohort) 3. DoR (up to 2 years) (MET cohort) 4. Safety (2 years) (all participants) |
|
NSCLC with MET exon 14 mutation or solid tumours with a NTRK rearrangement |
Avelumab (Bavencio, Merck Group, Darmstadt, Germany and Pfizer, New York City, NY, USA) plus talazoparib (Talzenna, Pfizer, New York City, NY, USA) | Locally advanced (primary or recurrent) or metastatic solid tumours | NCT03330405 | Primary: 1. Safety (28 days) 2. Overall response (24 months) Secondary:1. Pharmacokinetics (15 days) 2. Immunogenicity (15 days) 3. Overall response (24 months) 4. PSA or CA-125 tumour marker (24 months) 5. PD-L1 levels (24 months) 6. Time to tumour response (24 months) 7. DoR (24 months) 8. PFS (24 months) 9. OS (24 months) 10. PSA response (24 months) |
|
Patients with locally advanced (primary or recurrent) or metastatic solid tumours, including NSCLC, triple negative breast cancer, hormone receptor positive (HR+) breast cancer, recurrent platinum sensitive ovarian cancer, urothelial cancer and castration resistant prostate cancer |
Locally advanced or metastatic RAS-mutant solid tumours | NCT03637491 | Primary: 1. Safety (28 days) 2. Confirmed objective response (24 months) Secondary:1. Pharmacokinetics (12 months) 2. Objective response (24 months) 3. Time to tumour response (24 months) 4. DoR (24 months) 5. OS (24 months) 6. PFS (24 months) 7. Pharmacokinetics (3 months) 8. Biomarker levels (PD-L1, tumour mutational burden and DNA damage repair) |
|
Patients with locally advanced or metastatic KRAS- or NRAS-mutant NSCLC, pancreatic ductal adenocarcinoma or other KRAS- or NRAS-mutant solid tumours | |
Solid tumours with a BRCA or ATM defect | NCT03565991 | Primary: 1. Confirmed objective response (24 months) Secondary:1. Confirmed objective response by the investigator (24 months) 2. Time to tumour response (24 months) 3. DoR (24 months) 4. PFS (24 months) 5. OS (24 months) 6. PSA and CA-125 response (24 months) 7. Circulating tumour cell level 8. Pharmacokinetics (up to 24 months) 9. Immunogenicity (up to 24 months) |
|
Patients with locally advanced or metastatic solid tumours with a BRCA or ATM defect | |
LOXO-195 (Bayer, Leverkusen, Germany) | Solid tumours | NCT03215511 | Primary: 1. MTD 2. Best overall response (up to 2 years) Secondary:1. Safety (up to 24 months) 2. Overall response (24 months) 3. Pharmacokinetics (5 months) 4. DoR (up to 24 months) 5. PFS (up to 24 months) 6. OS (up to 24 months) 7. Clinical benefit rate (up to 24 months) |
|
Patients with unresectable or metastatic solid tumours and progressed or intolerant to prior TRK inhibitor |
TPX-0005 (TP Therapeutics, Inc., San Diego, CA, USA) | Advanced solid tumours harbouring ALK, ROS1, or NTRK1–3 rearrangements | NCT03093116 | Primary: 1. Maximum tolerated dose (28 days of first dose) 2. Recommended Phase 2 dose (28 days of first dose) 3. ORR (2–3 months after treatment start) Secondary:1. Effect of food on AUC (2–3 months after treatment start) 2. TTR (3 years) 3. DoR (3 years) 4. CBR (3 years) 5. PFS (3 years) 6. OS (3 years) 7. Intracranial ORR (3 years) 8. CNS PFS (3 years) |
|
Patients aged ≥ 18 years with histologically or cytologically confirmed locally advanced or metastatic solid tumour (including NHL) harbouring ALK, ROS1, NTRK1–3 gene rearrangement |
Sunitinib (Sutent, Pfizer, New York City, NY, USA) | Refractory solid tumours | NCT02691793 | Primary: 1. PFS (24 months) Secondary:1. ORR (24 months) 2. TTP (24 months) 3. OS (24 months) 4. Number of subjects with AE (24 months) |
|
Patients aged ≥ 19 years with RET fusion positive or FGFR2 fusion/other FGFR mutation refractory solid tumour and/or specific sensitivity to Sunitinib by Avatar scan that has progressed following standard therapy or that has not responded to standard therapy or for which there is no standard therapy |
Advanced rare tumours | NCT01396408 | Primary: 1. OR (every 4 weeks) Secondary:1. DoR/TTP/PFS/OS (48 months) 2. Translational research (48 months) 3. Safety (daily up to 4 weeks after treatment) |
|
Patients aged ≥ 16 years with histologically or cytologically confirmed advanced rare tumours:
|
|
Olaparib | Advanced (unresectable and/or metastatic) cancers | NCT03742895 | Primary: 1. ORR (up to 53 months) Secondary:1. DoR (up to 53 months) 2. OS (up to 53 months) 3. PFS (up to 53 months) 4. AEs (up to 53 months) 5. Time to earliest progression by cancer antigen-125 (up to 53 months) |
|
Patients aged ≥ 18 years with multiple types of advanced cancer (unresectable and/or metastatic) that (1) have progressed or been intolerant to SoC therapy, and (2) are positive for homologous recombination repair mutation or homologous recombination deficiency |
Advanced solid tumours, NHL or histiocytic disorders with defects in DNA damage repair genes | NCT03233204 | Primary: 1. ORR (up to 4 years) Secondary:1. PFS (up to 4 years) 2. Toxicity (up to 4 years) 3. PK (up to 4 years) Other:1. Change in tumour genomic profile (up to 4 years) |
|
Patients aged 1–21 years with solid tumours, NHL or histiocytic disorders with defects in DNA damage repair genes that have spread to other places in the body and have come back or do not respond to treatment | |
Glioma, cholangiocarcinoma or solid tumours with IDH1 or IDH2 mutations | NCT03212274 | Primary: 1. ORR (up to completion of course eight) Secondary:1. PFS (up to 1 year) 2. AE (up to 1 year) |
|
Patients aged ≥ 18 years diagnosed with a glioma, cholangiocarcinoma or other solid malignant tumour that has progressed despite standard therapy, or for which no effective standard therapy exists, with biopsy-confirmed evidence of an IDH1 or IDH2 mutation associated with neomorphic activity of the encoded proteins. Only specific mutations that lead to a neomorphic phenotype will be eligible for enrolment and include IDH1 (R132V, R132G, R132S, R132L, R132C and R132H) and IDH2 (R140W, R140L, R140Q, R172W, R172G, R172S, R172M and R172K) | |
Advanced cancer with a confirmed BRCA1 and/or BRCA2 mutation | NCT01078662 | Primary: 1. Tumour response rate (maximum up to 29 months) Secondary:1. ORR (up to 29 months) 2. PFS (up to 29 months) 3. OS (up to 29 months) 4. OS (12 months) 5. DoR (up to 29 months) 6. Disease control rate at week 16 |
|
Patients aged ≥ 18 years with malignant solid tumours for which no standard treatment exists and with confirmed documented deleterious or suspected deleterious BRCA mutation (ovarian, breast, prostate, pancreatic, advanced tumours) | |
Patients with tumours harbouring damaging mutations in homologous DNA repair genes or mutations, such as ATM, CHK2, MRN (MRE11/NBS1/RAD50), CDKN2A/B and APOBEC | NCT02576444 | Primary: 1. ORR (change from baseline to 16 weeks) |
|
Patients aged ≥ 18 years with histologically documented metastatic cancer (not hematologic malignancies) | |
Relapsed or refractory tumour | NCT02813135 | Primary: 1. ORR (56 days) 2. TTP (56 days) |
|
Patients aged < 18 years with haematological or solid tumour malignancy that has progressed despite standard therapy, or for which no effective standard therapy exists | |
Advanced cancer with a tumour that harbours a genomic variant known to be a drug target or to predict sensitivity to a drug | NCT02693535 | Primary: 1. ORR (at 16 weeks of treatment) Secondary:1. OS (up to 3 years) |
|
Patients aged 12 years with histologically proven locally advanced or metastatic solid tumour, multiple myeloma or B-cell NHL who are no longer benefiting from standard anticancer treatment or for whom, in the opinion of the treating physician, no such treatment is available or indicated | |
Relapsed or refractory advanced solid tumours, NHLs, or histiocytic disorders | NCT03155620 | Primary: 1. ORR (up to 4 years) Secondary:1. Safety (up to 4 years) 2. PFS (up to 4 years) 3. PK (up to 4 years) Other:1. Genomics (up to 4 years) |
Design: Phase II (separate cohorts specified)
|
Paediatric patients with solid tumours, NHLs or histiocytic disorders that have progressed following at least one line of standard systemic therapy and/or for which no standard treatment exists that has been shown to prolong survival | |
Cancers of unknown primary site | NCT03498521 | Primary: 1. PFS (up to 48 months) Secondary:1. OS (up to 48 months) 2. ORR 3. Duration of benefit (up to 48 months) 4. AE (up to 48 months) |
|
Patients aged ≥ 18 years with histologically confirmed cancer of unknown primary site (non-specific subset) in accordance with criteria from ESMO, version 1, who have achieved disease control after three cycles of first-line platinum doublet induction chemotherapy | |
NHL, multiple myeloma and advanced solid tumours | NCT03297606 | Primary: 1. ORR (4 years) Secondary:1. AE (up to 4 years) 2. PFS (up to 4 years) |
|
Patients aged ≥ 18 years with a histologically proven incurable metastatic solid tumour (excluding primary brain tumours), multiple myeloma or B-cell NHL (excluding CLL, SLL and HCL), for whom there is no standard treatment known to prolong life or who have refused such treatment | |
Refractory solid tumours | NCT03239015 | Primary: 1. ORR (2 months) Secondary:1. PFS (2 months) 2. OS (1 month) 3. AE (1 month) |
|
Patients aged 18–75 years with malignant solid tumours diagnosed histologically. Common solid tumour patients that have no standard choice after multiple lines of therapy; rare solid tumour patients that did not have any standard recommended treatment | |
Advanced solid tumours | NCT02029001 | Primary: 1. Induction progression-free rate 2. PFS (up to 36 months) Secondary:1. ORR (over induction period) 2. OS 3. QoL (QLQ-C30) 4. Safety Other:1. DoR 2. Cost-effectiveness |
|
Patients aged ≥ 18 years with histologically or cytologically confirmed diagnosis of metastatic or locally advanced and unresectable solid tumour of any type, not amenable to curative treatment. Concerning primitive tumours of the CNS, all histological types of malignant tumours (including parenchymal and meningeal tumours) are eligible | |
Advanced solid tumour, multiple myeloma or NHL | NCT02925234 | Primary: 1. Per cent of patients treated based on molecular profile (6 months after treatment initiation) 2. Objective tumour response (6 months) 3. Stable disease (6 months) 4. AE ≥ G3 (6 months) Secondary:1. PFS (up to 1 year) 2. OS (up to 1 year) 3. Duration of treatment (6 months) |
|
Patients aged ≥ 18 years with a histologically proven locally advanced or metastatic solid tumour, multiple myeloma or B-cell NHL who are no longer benefiting from standard anticancer treatment or for whom no such treatment is available or indicated | |
LOXO-292 (Bayer, Leverkusen, Germany) | Advanced solid tumours, RET fusion-positive solid tumours and medullary thyroid cancer | NCT03157128 | Primary: 1. Dosage 2. ORR (up to 2 years) Secondary:1. AE (2 years) 2. ORR (2 years) 3. ORR/DoR/CBR/PFS/OS (2 years) Other:1. Genomics 2. HRQoL (QLQ-C30) |
|
Patients aged ≥ 12 years with advanced solid tumours, including RET fusion-positive solid tumours, medullary thyroid cancer and other tumours with RET activation |
Epacadostat (Merck Group, Darmstadt, Germany and Incyte, Wilmington, DE, USA) with pembrolizumab | Advanced or metastatic solid tumours | NCT03085914 | Primary: 1. Phase 1 – Safety and tolerability (up to 27 months) 2. Phase 2 – ORR (up to 24 months) Secondary:1. Phase 1 – ORR (up to 24 months) 2. Phase 2 – safety and tolerability (up to 27 months) 3. DoR (up to 24 months) 4. PFS (up to 24 months) |
|
Patients aged ≥ 18 years with histologically or cytologically confirmed diagnosis of selected advanced or metastatic solid tumours |
Advanced or metastatic malignancies | NCT03277352 | Primary: 1. Phase 1 – AE (up to 18 months) 2. Phase 2 – ORR/CRR (up to 18 months) Secondary:1. Disease control rate (18 months) 2. DoR (18 months) 3. Duration of disease control (18 months) 4. PFS (18 months) 5. OS (at 1 and 2 years) |
|
Patients aged ≥ 18 years with locally advanced or metastatic disease; locally advanced disease must not be amenable to resection with curative intent | |
Advanced solid tumours | NCT02959437 | Primary: 1. Phase I – AE (up to 18 months) 2. Phase II – ORR (up to 18 months) Secondary:1. Phase I ORR 2. Phase II AE 3. PFS (up to 18 months) 4. DoR (up to 18 months) |
|
Patients aged ≥ 18 years with histologically or cytologically confirmed advanced or metastatic solid tumours who have failed prior standard therapy (disease progression, subject refusal or intolerance is also allowable). Part 1 is a dose-escalation assessment to evaluate the safety and tolerability of the combination therapies. Once the recommended doses have been determined, subjects with previously treated NSCLC, microsatellite-stable CRC, head and neck squamous cell carcinoma, urothelial carcinoma, and melanoma will be enrolled into expansion cohorts in part 2 | |
Durvalumab (Infinzi, AstraZeneca, Cambridge, UK) with tremelimumab | Advanced solid and haematological cancers | NCT03837899 | Primary: 1. Recommended Phase II dose in patients receiving chemotherapy (15 months) 2. Safety and tolerability (up to 4 years) 3. ORR (up to 4 years) Secondary:1. PK (15 months) |
|
Paediatric patients (up to 17 years) with solid tumours, which must have progressed or be refractory to standard therapies |
Advanced rare solid tumours | NCT02938793 | Primary: 1. Antitumour activity (24 months) 2. AEs (24 months) Secondary:1. Expression of PD-1 |
|
Adult patients with a diagnosis of a rare advanced solid malignancy meeting EORTC criteria. Subjects must have failed or been ineligible for standard treatment options, if available | |
Advanced malignancies | NCT02978482 | Primary: 1. Plasma concentration 2. AEs 3. ORR (12 months after last patient is dosed or withdrawn, or study is discontinued) Secondary:1. Anti-drug antibody 2. CR/PR/stable disease/progressive disease (6 months after last patient is dosed) 3. OS (12 months after last evaluable patient is first dosed) |
|
Chinese adult patients with histologically or cytologically confirmed advanced and/or metastatic solid tumours other than HCC, refractory or intolerable to existing standard of treatment | |
Advanced solid malignancies | NCT03084471 | Primary: 1. Safety: AEs Secondary:1. Safety: treatment-related adverse events and treatment discontinued/interrupted 2. OS (up to 5 years following date of first patient initiation) |
|
Adult patients with a life expectancy of ≥ 12 weeks and no prior exposure to anti-PD-1 or anti-PD-L-1 | |
Somatically hyper-mutated recurrent solid tumours | NCT03911557 | Primary: 1. TTP ratio (2 years) Secondary:1. PFS (2 years) |
|
Adult patients with relapsed/refractory solid tumour patients (not previously treated with anti-PD-1/PD-L1 or anti-CTLA-4 immunotherapy), whose tumours expressed a high or moderate tumour mutational burden | |
Advanced rare tumours | NCT02879162 | Primary: 1. ORR (48 months) Secondary:1. AEs 2. TTP (48 months) 3. PFS (38 months) 4. Response duration (48 months) |
|
Patients aged ≥ 16 years with histologically and/or cytologically confirmed cancer that is advanced/metastatic/recurrent or unresectable and for which no curative therapy exists. The list includes salivary carcinoma; carcinoma of unknown primary site with tumour infiltrating lymphocytes and/or expressing PD-L1; mucosal melanoma; acral melanoma; osteosarcoma; undifferentiated pleomorphic sarcoma; clear cell carcinoma of the ovary; and squamous cell carcinoma of the anal canal | |
BLU-667 (Roche, Basel, Switzerland) | Solid tumours | NCT03037385 (EudraCT 2016–004390–41) | Primary: 1. Tolerability (12 months) 2. Safety (24 months) 3. ORR (up to 2 years) Secondary:1. DoR, PFS and OS (up to 2 years) 2. RET gene status and correlation between RET gene status and ORR, DoR and DCR (up to 2 years) 3. Pharmacokinetics (4 months) 4. Pharmacodynamics (12 months) |
|
Enrolling patients with medullary thyroid cancer, RET-altered NSCLC and other RET-altered solid tumours |
Atezolizumab (Tecentriq, Roche, Basel, Switzerland) | Solid tumours | NCT02458638 | Primary: 1. NPR (18 weeks) Secondary:1. NPR (24 weeks) 2. ORR (24 weeks) 3. BOR (24 weeks) 4. DoR (24 weeks) 5. PFS (24 weeks) 6. TTP (24 weeks) 7. OS (24 weeks) 8. Safety (24 weeks) |
|
Enrolling adults patients with advanced solid tumours who have received at least one line of prior systemic therapy or for whom no alternative therapy to prolong survival exists |
Cobimetinib (Cotellic, Roche, Basel, Switzerland) | Solid tumours | NCT02639546 | Primary: 1. Safety (1 month) 2. Dosing (1 month) 3. Pharmacokinetics (12 months) 4. Percentage of patients with OR (6.75 years) 5. PFS (6.75 years) Secondary:1. DoR (up to 6.75 years) 2. OS (up to 6.75 years) |
|
Enrolling paediatric and young adult participants with solid tumours with known or potential kinase pathway activation (RAS/RAF/MEK/ERK pathway involvement) for whom standard therapy has proven to be ineffective or intolerable or for whom no curative standard-of-care treatment options exist |
Crizotinib (Xalkori, Pfizer, New York City, NY, USA) | Solid and liquid tumours | NCT01524926 (CREATE) | Primary: 1. Antitumour activity Secondary:1. Safety 2. PFS 3. DCR 4. OS 5. DoR |
|
Enrolling patients with advanced tumours induced by causal alterations of ALK and/or MET |
Solid tumours | NCT02034981 | Primary: 1. ORR (8 weeks) Secondary:1. Safety (up to 2.5 years) 2. DCR (4 months) 3. DoR 4. PFS 5. OS |
|
Enrolling patients harbouring an alteration on ALK, MET or ROS1 |
Appendix 3 The MEDLINE search strategy
Date range searched: inception to March 2019.
Date searched: March 2019.
Search strategy
-
*Neoplasms/
-
(cancer$ or neoplasm$ or tumour$ or tumour$ or malignan$ or oncology or lymphoma$ or sarcoma$ or melanoma$ or myeloma$ or carcinoma$).tw.
-
1 or 2
-
tumour response$.tw.
-
tumour response$.tw.
-
objective response$.tw.
-
ORR.tw.
-
“duration of response$”.tw.
-
dor.tw.
-
response rate$.tw.
-
complete response$.tw
-
overall response$.tw
-
4 or 5 or 6 or 7 or 8 or 9 or 10 or 11 or 12
-
3 and 13
-
Regression analysis/
-
regression.tw.
-
relationship.tw.
-
correlation.tw.
-
prediction.tw.
-
association.tw.
-
15 or 16 or 17 or 18 or 19 or 20
-
14 and 21
-
endpoint$.tw.
-
end point$.tw.
-
(surrogate or surrogacy).tw.
-
23 or 24 or 25
-
22 and 26
-
progression-free survival/
-
“progression free survival”.tw.
-
“overall survival”.tw.
-
(pfs or os).tw.
-
“time to progression”.tw.
-
ttp.tw.
-
28 or 29 or 30 or 31 or 32 or 33
-
27 and 34
-
limit 35 to (english language and humans)
Appendix 4 Table of study characteristics (ordered by cancer type, then author)
Study (first author and year) | Cancer | Surrogate outcome | Final outcome | Stage | Line | Treatment | Studies (n) | Patients (n) | Study types | Publication/search years | Data type | Response criteria | Absolute association | Treatment effect association | STE reported |
---|---|---|---|---|---|---|---|---|---|---|---|---|---|---|---|
Agarwal 201783 | Acute myeloid leukaemia | ORR and CR | OS | Various | First | Systemic | 20a | NR | RCT and SA | 2004–16 | AD | NR | Yes | ||
Moriwaki 2016119 | Biliary tract | ORR | OS | Advanced | First | Chemotherapy | 17a | 2040 | RCT | Up to 2015 | AD | NR | Yes | ||
Bruzzi 200586 | Breast | ORR | OS | Metastatic | All | Chemotherapy | 10 | 2126 | RCT | 1991–2001 | IPD | WHO (n = 8), ECOG (n = 1), NR (n = 1) | Yes | ||
Burzykowski 200887 | Breast | ORR | PFS and OS | Metastatic | First | Chemotherapy | 11 | 3953 | RCT | 1999–2008 | IPD | WHO | Yes | ||
Hackshaw 200599 | Breast | ORR and CR | OS | Metastatic | First | Chemotherapy | 42b | 9163 | RCT | 1966–2005 | AD | NR | Yes | ||
Liu 2016114 | Breast | ORRb | OS | Metastatic | Second and third | Chemotherapy | 24 | 8617 | RCT | 1999–2014 | AD | NR | Yes | ||
Petrelli 2014127 | Breast | ORR | OS | Metastatic or advanced | First | Targeted therapy and chemotherapy | 20a | 10,138a | RCT | 2000–12 | AD | NR | Yes | ||
Buyse 200088 | Colorectal | ORR | OS | Advanced | First | Chemotherapy | 25 | 3791 | RCT | Collected 1990–6 | IPD | WHO | Yes | ||
Ciani 201589 Elia 202096 | Colorectal | ORR | PFS and OS | Advanced or metastatic | All | Systemic | 33 | NR | RCT | 2003–13 | AD | RECIST or WHO | Yes | Yes | |
Colloca 201692 | Colorectal | ORR and DoR | OS | Metastatic | First | Bevacizumab and chemotherapy | 11 | NR | RCT | 2000–14 | AD | RECIST | Yes | ||
Giessen 201598 | Colorectal | ORR | OS | Metastatic | Second | Chemotherapy | 22 | 10,509 | RCT | 2000–13 | AD | RECIST (n = 17), WHO (n = 5) | Yes | ||
Cremolini 201794 | Colorectal | ORR | OS | Metastatic | Second | Targeted therapy | 20b | 7571 | RCT | Up to 2015 | AD | NR | Yes | ||
Johnson 2006109 | Colorectal | ORR | OS | Metastatic | First | Chemotherapy | 146a | 35,337a | RCT | Up to 2005 | AD | NR (very few RECIST) | Yes | ||
Louvet 2001115 | Colorectal | ORR | PFS and OS | Metastatic | First | Various | 29 | 13,498 | RCT | 1990–2000 | AD | NR | Yes | ||
Sidhu 2013136 | Colorectal | ORR | OS | Metastatic | First (most) | Chemotherapy ± targeted therapy | 24a | 20,438a | RCT | 2000–11 | AD | NR | Yes | ||
Tang 2007138 | Colorectal | ORR | OS | Metastatic | First | Chemotherapy | 39 | 18,668 | RCT | 1990–2005 | AD | NR | Yes | Yes | |
Tsujino 2010140 | Colorectal | ORR | PFS and OS | Advanced | NR | Targeted therapy | 7 | NR | RCT | Up to 2009 | AD | NR | Yes | ||
Ichikawa 2006105 | Gastric | ORR | TTP and OS | Advanced | First | Chemotherapy | 25 | 4593 | RCT | NR | AD | WHO, SWOG, RECIST, Japan | Yes | ||
Shitara 2014133 | Gastric | ORRb | OS | Advanced | Second and third | Chemotherapy | 64 | 4286 | RCT and SA | 2002 to 2012–13 | AD | NR | Yes | ||
Pang 2018124 | Gastro-oesophageal | ORRb and CR | OS | Advanced | First and second | Targeted therapy | 18 | 7892 | RCT | Up to 2018 | AD | RECIST | Yes | ||
Han 2014101 | Glioblastoma | ORR | OS | Unclear | Various | Various | 91a | 7125a | RCT and SA | 1991–2012 | AD | NR (‘standard criteria’) | Yes | ||
Blumenthal 201785 | Lung (NSCLC) | ORR | PFS and OS | Metastatic | Various | Chemotherapy, immune checkpoint inhibitors or targeted therapy | 25 | 20,013a | RCT | 2003–16 | AD | RECIST or WHO | Yes | ||
Blumenthal 201584 | Lung (NSCLC) | ORR | PFS and OS | Metastatic | Various | Chemotherapy or targeted therapy | 14 | 12,567a | RCT | 2003–13 | AD | RECIST (n = 11) or WHO (n = 3) | Yes | ||
Hashim 2018102 | Lung (NSCLC) | ORR | OS | Advanced | Second and subsequent | Various | 140 | 41,725 | RCT | Up to 2016 | AD | NR | Yes | Yes | |
Hotta 2015103 | Lung (NSCLC) | ORR | OS | Advanced | Various | Targeted therapy | 18 | 7633a | RCT | 2003–14 | AD | NR | Yes | ||
Ito 2019145 | Lung (NSCLC) | ORR | PFS and OS | Advanced | Various | Immune checkpoint inhibitors [PD-(L)1] | 7 | 3752a | RCT | NR | AD | NR | Yes | Yes | |
Johnson 2006109 | Lung (NSCLC) | ORR | OS | Advanced | First | Chemotherapy | 191a | 44,125a | RCT | Up to 2005 | AD | NR (very few RECIST) | Yes | ||
Li 2019112 | Lung (NSCLC) | ORRb and CR | OS | Advanced | First and second | Immune checkpoint inhibitors | 5a | 4803a | RCT | Up to 2018 | AD | RECIST | Yes | ||
Li 2012113 | Lung (NSCLC) | ORR | OS | Advanced | First and second | Targeted therapy | 60 | 9903 | RCT and SA | Up to 2011 | AD | RECIST (n = 52), WHO (n = 10) | Yes | ||
Nakashima 2016121 | Lung (NSCLC) | ORR | OS | Advanced, locally advanced and recurrent | First | Chemotherapy | 44 | 22,709 | RCT | 2005–15 | AD | RECIST | Yes | ||
Ritchie 2018128 | Lung (NSCLC) | ORRb | PFS and OS | Advanced | All | Immune checkpoint inhibitors [PD-(L)1 or CTLA4] | 8 | NR | RCT | 2000–17 | AD | NR | Yes | Yes | |
Roviello 2017130 | Lung (NSCLC) | ORR | PFS and OS | Unclear | Various | Immune checkpoint inhibitors | 7b | 3369b | RCT | Up to 2017 | AD | RECIST or mWHO | Yes | ||
Sekine 1999131 | Lung (NSCLC) | ORR | OS | Unclear | Various | Chemotherapy | 42 | 1935 | SA and one RCT | 1988–97 | AD | WHO | Yes | ||
Shukuya 2016134 | Lung (NSCLC) | ORR | OS | Advanced | All | (a) Immune checkpoint inhibitors (PD-(L)1) | (a) 10a | NR | RCT and SA | 2012–16 | AD | RECIST (most) | Yes | ||
(b) Chemotherapy [docetaxel (Taxotere, Sanofi-Aventis, Paris, France)] | (b) 22a | ||||||||||||||
Tsujino 2010140 | Lung (NSCLC) | ORR | PFS and OS | Advanced | NR | Targeted therapy | 6 | NR | RCT | Up to 2009 | AD | NR | Yes | ||
Tsujino 2009139 | Lung (NSCLC) | ORR | PFS and OS | Advanced | NR | Targeted therapy | 28 | 6171 | RCT and SA | Up to 2007 | AD | RECIST (n = 21), WHO (n = 9) | Yes | ||
Vidaurre 2009141 | Lung (NSCLC) | ORRb | PFS and OS | Advanced, locally advanced, unresectable or metastatic | NR | Chemotherapy or targeted therapy | 35 | NR | RCT and SA | 2006–8 | AD | NR | Yes | ||
Foster 201197 | Lung (SCLC) | ORR and CR | OS | Extensive stage | First | Chemotherapy | Three RCTs (32 centres) | 596a | RCT | Trials initiated 1987–99 | AD | NR (CR = disappearance; PR ≥ 50% reduction) | Yes | ||
Hotta 2009104 | Lung (SCLC) | ORR | OS | Extensive disease | First | Chemotherapy | 48 | 8779 | RCT | 1990–2008 | AD | WHO (n = 23), ECOG (n = 2), RECIST (n = 1), Japan (n = 1) or NR | Yes | ||
Nickolich 2014122 | Lung (SCLC) | ORR, CR and PR | PFS and OS | Limited or extensive disease | First and second and maintenance | Various | 66a | 8471a | RCT and SA | 1983–2010 | AD | NR | Yes | ||
Mangal 2018118 | Multiple myeloma | ORR,b CR, VGPR or CR | PFS | Relapsed/refractory | Second and subsequent | Various | 79a | 13,322a | RCT and SA | 1999–2016 | AD | IMWG | Yes | ||
Imaoka 2019107 | Neuroendocrine | ORR | PFS | Advanced | Various | Systemic | 22 | 1310 | RCT and SA | 1996–2016 | AD | RECIST (n = 20) and WHO (n = 2) | Yes | ||
Imaoka 2017106 | Neuroendocrine | ORR | OS | Advanced | Various | Systemic | 20 | 2530 | RCT and SA | 1996–2016 | AD | NR | Yes | ||
Lee 2011111 | NHL (aggressive) | CR | PFS and OS | Unclear | First | Chemotherapy | 36a | 16,103a | RCT | 1990–2009 | AD | NR | Yes | ||
Lee 2011111 | NHL (indolent) | CR | PFS and OS | Unclear | First | Chemotherapy | 15a | 5128a | RCT | 1990–2009 | AD | NR | Yes | ||
Mangal 2018117 | NHL | ORRb and CR | PFS | Stage III/IV > 75% in most cohorts | Various | Various | 73 | 6071 | RCT and SA | 1996–2015 | AD | NR | Yes | ||
Shi 2017132 | NHL (indolent; follicular) | CR 30 months and CR 24 months | PFS | Unclear | First | Chemotherapy or immunotherapy (induction or maintenance) | 13 | 3837 | RCT | 1990–2011 | IPD | NR (CR = disappearance) | Yes | Yes | |
Zhu 2017144 | NHL (indolent; follicular) | CR | PFS | Unclear | NR | Chemotherapy, immunotherapy or targeted therapy | 13 | NR | RCT and SA | 1993–2013 | AD | NR | Yes | ||
Zhu 2017144 | NHL (mantle cell) | CR | PFS | Unclear | NR | Chemotherapy, immunotherapy or targeted therapy | NR | NR | RCT and SA | 1993–2013 | AD | NR | Yes | ||
Colloca 201790 | Ovarian | ORR and CR | PFS and OS | Advanced | First | Chemotherapy | 29 | NR | RCT | 1990–2016 | AD | WHO (n = 24), RECIST (n = 8) | Yes | ||
Rose 2010129 | Ovarian | ORRb | PFS and OS | Recurrent/platinum-resistant | Second | Various | 11 | 407 | SA | 1994–2004 | IPD | WHO (n = 10) and RECIST (n = 1) | Yes | ||
Siddiqui 2017135 | Ovarian | ORRb | PFS and OS | Advanced, recurrent | Second and subsequent | Chemotherapy | 39a | 9223a | RCT | 2000–15 | AD | NR | Yes | Yes | |
Colloca 201691 | Pancreatic | ORR and DoR | PFS and OS | Advanced or metastatic | First | Gemcitabine and chemotherapy or targeted therapy | 36b | NR | RCT | 1997–2014 | AD | RECIST | Yes | ||
Hamada 2016100 | Pancreatic | ORR | OS | Advanced | First | Chemotherapy | 47 | 15,906a | RCT | 1995–2015 | AD | NR | Yes | Yes | |
Makris 2017116 | Pancreatic (adenocarcinoma) | ORR | OS | Locally advanced, unresectable or metastatic | First | Chemotherapy (gemcitabine) | 22b | 10,379b | RCT | 2000–15 | AD | NR (RR = shrinkage or disappearance) | Yes | ||
Colloca 201693 | Prostate | ORR | OS | Metastatic (castration resistant) | First and second | Chemotherapy, hormonal and targeted therapy | 17 | NR | RCT | 1995–2014 | AD | NR (CR = disappearance; PR = ≥ 30% reduction) | Yes | ||
Abdel-Rahman 201881 | Renal cell | ORR | OS | Advanced | Various | Immune checkpoint inhibitors [PD-(L)1] | 4 | 1093 | RCT and SA | Up to 2017 | AD | RECIST | Yes | ||
Delea 201295 | Renal cell | ORR | OS | Metastatic | NR | Cytokine or targeted | 25b | 10,943a | RCT | 1997–2010 | AD | NR | Yes | ||
Petrelli 2013126 | Renal cell | ORR | PFS and OS | Metastatic | First | Targeted | 6a | 3188a | RCT | Up to 2011 | AD | NR | Yes | Yes | |
Tanaka 2019137 | Soft tissue sarcoma | ORR | OS | Advanced | First | Chemotherapy | 27a | 6156a | RCT | 1974–2017 | AD | NR | Yes | ||
Zer 2016143 | Soft tissue sarcoma | ORR | OS | Advanced or metastatic | All | Systemic | 52a | 9762a | RCT | 1974–2014 | AD | NR | Yes | ||
Penel 2014125 | Unknown primary | ORRb | PFS and OS | Unclear | NR | NR | 38a | NR | SA | 1997–2011 | AD | RECIST or WHO | Yes | ||
Abdel-Rahman 201881 | Urothelial | ORR | OS | Advanced | Various | Immune checkpoint inhibitors [PD-(L)1] | 9 | 1699 | RCT and SA | Up to 2017 | AD | RECIST | Yes | ||
Agarwal 201482 | Urothelial | ORR | OS | Advanced (operable or metastatic) | Second | Chemotherapy or biologic | 10 | 560 | RCT and SA | NR | AD | RECIST | Yes | ||
Kaufman 2018110 | Various solid tumours | ORR | OS | Unclear | Various | Immune checkpoint inhibitors ± chemotherapy | 27a | 10,300a | RCT | 2005–17 | AD | RECIST or mWHO | Yes | ||
Mushti 2018120 | Various solid tumours | ORRb | OS | Unclear | NR | Immune checkpoint inhibitors [PD-(L)1] | 13 | 6722 | RCT | 2014–16 | AD | RECIST | Yes | ||
Nie 2019123 | Various solid tumours | ORRb | OS | Advanced or recurrent | Various | Immune checkpoint inhibitors [PD-(L)1] | 43a | 15,088a | RCT and SA | Up to 2018 | AD | RECIST | Yes | Yes | |
Ritchie 2018128 | Various solid tumours | ORRb | PFS and OS | Advanced | All | Immune checkpoint inhibitors [PD-(L)1 or CTLA4] | 20a | 10,828a | RCT | 2000–17 | AD | NR | Yes | Yes | |
Roviello 2017130 | Various solid tumours | ORR | PFS and OS | Unclear | Various | Immune checkpoint inhibitors | 17a | 8994a | RCT | Up to 2017 | AD | RECIST or mWHO | Yes | ||
Tsujino 2010140 | Various solid tumours | ORR | PFS and OS | Advanced | NR | Targeted | 18 | NR | RCT | Up to 2009 | AD | NR | Yes | Yes | |
Vidaurre 2009141 | Various | ORRb | PFS and OS | Advanced, locally advanced, unresectable or metastatic | NR | Chemotherapy or targeted | 143a | 6974a | RCT and SA | 2006–08 | AD | NR | Yes | ||
Wilkerson 2009142 | Various solid tumours | ORR | PFS and OS | Metastatic | NR | NR | 66a | NR | RCT | NR | AD | NR | Yes |
Appendix 5 Absolute correlation and regression results (ordered by outcome type, then cancer type, then author)
Study (first author and year) | Surrogate outcome | Final outcome | Cancer | Line subgroups | Treatment | Studies (n) | Patients (n) | Absolute correlation methods | Correlation coefficient | Absolute regression methods | Regression R2 (95% CI); p-value | Linear regression equation |
---|---|---|---|---|---|---|---|---|---|---|---|---|
ORR vs. PFS (or TTP) | ||||||||||||
Louvet 2001115 | ORR | PFS | Colorectal | First | Various | 29 | 13,498 | Spearman (ORR vs. median PFS) | rs = 0.66; p < 0.0001 | LR (ORR vs. median PFS) | PFS = 3.2 + 0.1 × ORR | |
Ichikawa 2006105 | ORR | TTP | Gastric | First | Chemotherapy (any) | 12a | 2144 | Spearman weighted (ORR vs. median TTP) | rs = 0.49; p < 0.0001 | WLR (ORR vs. median TTP) | TTP = 1.73 + 0.09 × ORR | |
Ichikawa 2006105 | ORR | TTP | Gastric | First | Chemotherapy (novel) | 8a | 1077 | Spearman weighted (ORR vs. median TTP) | rs = 0.41; p = 0.018 | |||
Ichikawa 2006105 | ORR | TTP | Gastric | First | Chemotherapy (non-novel) | 7a | 1067 | Spearman weighted (ORR vs. median TTP) | rs = 0.56; p = 0.0053 | |||
Ito 2019145 | ORR | PFS | Lung (NSCLC) | Various | Immune checkpoint inhibitors [PD-(L)1] | 6 | 3752b | (a) Pearson weighted | (a) r = 0.55; p < 0.0001 | WLR R2 (ORR vs. median PFS) | R2 = 0.30; p = 0.206 | |
(b) Spearman weighted (ORR vs. median PFS) | (b) rs = 0.33; p < 0.0001 | |||||||||||
Ito 2019145 | ORR | PFS | Lung (NSCLC) | Various: high PD-L1 expression | Immune checkpoint inhibitors [PD-(L)1] | 7 | 1381 | (a) Pearson weighted | (a) r = 0.90; p < 0.0001 | WLR R2 (ORR vs. median PFS) | R2 = 0.81; p = 0.006 | |
(b) Spearman weighted (ORR vs. median PFS) | (b) rs = 0.48; p < 0.0001 | |||||||||||
Ritchie 2018128 | ORR | PFS | Lung (NSCLC) | All | Immune checkpoint inhibitors [PD-(L)1 or CTLA4] | 8 | NR | Correlation (NR) (ORR vs. 6-month PFS) | r = 0.85 (95% CI 0.63 to 1.06); p = NR | |||
Tsujino 2009139 | ORR | PFS | Lung (NSCLC) | NR | Targeted therapy | 18a | 3790a | LR (ORR vs. median PFS) | R2 = NR; p = 0.001 | Slope: 0.072 | ||
Vidaurre 2009141 | ORR | PFS | Lung (NSCLC) | NR | Chemotherapy or targeted therapy | 35 | NR | Regression (NR) (ORR vs. median PFS) | R2 = 0.75; p < 0.0001 | |||
Nickolich 2014122 | ORR | PFS | Lung (SCLC) | First and second and maintenance: limited or extensive | Various | 66b | 8471b | Pearson (ORR vs. median PFS) | r = 0.73; p < 0.0001 | |||
Nickolich 2014122 | ORR | PFS | Lung (SCLC) | First and second and maintenance: limited disease | Various | 66b | 8471b | Pearson (ORR vs. median PFS) | r = 0.02; p = 0.978 | |||
Nickolich 2014122 | ORR | PFS | Lung (SCLC) | First and second and maintenance: extensive disease | Various | 66b | 8471b | Pearson (ORR vs. median PFS) | r = 0.51; p = 0.013 | |||
Mangal 2018118 | ORR | PFS | Multiple myeloma | Second and subsequent | Various | 79b | 13,322b | WLR adjusted R2 (logit ORR vs. log-median PFS) | Adjusted R2 = 0.50; p = NR | |||
Imaoka 2019107 | ORR | PFS | Neuroendocrine | Various | Systemic | 22 | 1310 | Pearson (ORR vs. median PFS) | r = 0.37 (95% CI –0.05 to 0.80); p = 0.085 | |||
Imaoka 2019107 | ORR | PFS | Neuroendocrine | Various: published 1996–2010 | Systemic | 6a | NR | Pearson (ORR vs. median PFS) | r = –0.08 (95% CI –0.76 to 0.60); p = 0.824 | |||
Imaoka 2019107 | ORR | PFS | Neuroendocrine | Various: published 2011–16 | Systemic | 16a | NR | Pearson (ORR vs. median PFS) | r = 0.43 (95% CI –0.07 to 0.93); p = 0.095 | |||
Imaoka 2019107 | ORR | PFS | Neuroendocrine | Various | Cytotoxic | Nine arms | NR | Pearson (ORR vs. median PFS) | r = 0.63 (95% CI 0.03 to 1.22); p = 0.041 | |||
Imaoka 2019107 | ORR | PFS | Neuroendocrine | Various | Non-cytotoxic | 18 arms | NR | Pearson (ORR vs. median PFS) | r = 0.18 (95% CI –0.27 to 0.62); p = 0.432 | |||
Imaoka 2019107 | ORR | PFS | Neuroendocrine | Various | Targeted | 19 arms | NR | Pearson (ORR vs. median PFS) | r = 0.42 (95% CI –0.06 to 0.90); p = 0.086 | |||
Imaoka 2019107 | ORR | PFS | Neuroendocrine | Various | Non-targeted | Eight arms | NR | Pearson (ORR vs. median PFS) | r = –0.72 (95% CI –1.09 to –0.35); p < 0.001 | |||
Mangal 2018117 | ORR | PFS | NHL | Various | Various | 73 | 6071 | LR adjusted R2 (logit ORR vs. log-median PFS) | Adjusted R2 = 0.70; p = NR | log-(median PFS) = 1.97 + 0.414 × logit (ORR) | ||
Rose 2010129 | ORR | PFS | Ovarian | Second | Various | 11 | 407 | (a) Pearson | (a) r = 0.62; p = 0.044 | |||
(b) Kendall Tau-b (ORR vs. median PFS) | (b) r = 0.48; p = 0.042 | |||||||||||
Siddiqui 2017135 | ORR | PFS | Ovarian | Second and subsequent | Chemotherapy | 39b | 9223b | (a) Pearson weighted (ORR vs. median PFS) | (a) r = 0.85; p < 0.001 | (a) WLR R2 (ORR vs. median PFS): unadjusted; | (a) R2 = 0.72; p = NR | Median PFS = 2.59 + 0.12 × ORR |
(b) Pearson unweighted (ORR vs. median PFS) | (b) r = 0.76; p < 0.001 | (b) WLR R2 (ORR vs. median PFS): adjusted | (b) Adjusted R2 = 0.72; p = NR | |||||||||
Petrelli 2013126 | ORR | PFS | Renal cell | First | Targeted | 6b | 3188b | Spearman weighted (ORR vs. median PFS) | rs = 0.96; p < 0.0001 | |||
Penel 2014125 | ORR | PFS | Unknown primary | NR | NR | 38b | NR | Pearson via WLR (ORR vs. median PFS) | r = 0.54; p < 0.0001 | |||
Ritchie 2018128 | ORR | PFS | Various solid tumours | All | Immune checkpoint inhibitors [PD-(L)1 or CTLA4] | 20b | 10,828b | Correlation (NR) (ORR vs. 6-month PFS) | r = 0.37 (95% CI 0.06 to 0.95); p = NR | |||
Vidaurre 2009141 | ORR | PFS | Various | NR | Chemotherapy | 85 | 3982a | Regression (NR) (ORR vs. median PFS) | R2 = 0.53; p < 0.0001 | |||
Vidaurre 2009141 | ORR | PFS | Various | NR | Targeted | 58 | 2992a | Regression (NR) (ORR vs. median PFS) | R2 = 0.61; p< 0.0001 | |||
Vidaurre 2009141 | ORR | PFS | Various | NR | Chemotherapy or targeted therapy | 143b | 6974b | Regression (NR) (ORR vs. median PFS) | R2 = 0.56; p < 0.0001 | |||
ORR vs. OS | ||||||||||||
Agarwal 201783 | ORR | OS | Acute myeloid leukaemia | First | Systemic | 20b | NR | WLR adjusted R2 (logit ORR vs. log-median OS) | Adjusted R2 = 0.45; p = NR | |||
Liu 2016114 | ORR | OS | Breast | Second and third | Chemotherapy | 24 | 8617 | Spearman (ORR vs. median OS) | rs = 0.54 (95% CI 0.29 to 0.72); p < 0.0001 | |||
Liu 2016114 | ORR | OS | Breast | Second and third: previous anthracycline/taxanes | Chemotherapy | 15a | NR | Spearman (ORR vs. median OS) | rs = 0.62 (95% CI 0.32 to 0.84); p = NR | |||
Liu 2016114 | ORR | OS | Breast | Second and third: previous trastuzumab/bevacizumab | Chemotherapy | 5a | NR | Spearman (ORR vs. median OS) | rs = 0.78 (95% CI 0.19 to 1.0); p = NR | |||
Liu 2016114 | ORR | OS | Breast | Second and third | Chemotherapy (taxanes) | 21a | NR | Spearman (ORR vs. median OS) | rs = 0.49 (95% CI –0.19 to 0.92); p = NR | |||
Liu 2016114 | ORR | OS | Breast | Second and third | Chemotherapy (antimetabolites) | 22a | NR | Spearman (ORR vs. med OS) | rs = –0.10; p = NR | |||
Liu 2016114 | ORR | OS | Breast | Second and third: HER2 positive | Chemotherapy | 5a | NR | Spearman (ORR vs. median OS) | rs = 0.96 (95% CI 0.80 to 1.00); p = NR | |||
Liu 2016114 | ORR | OS | Breast | Second and third: HER2 negative | Chemotherapy | 3a | NR | Spearman (ORR vs. median OS) | rs = 1.00; p = NR | |||
Petrelli 2014127 | ORR | OS | Breast | First | Targeted therapy and chemotherapy | 20b | 10,138b | Spearman weighted (ORR vs. median OS) | rs = 0.61 (95% CI 0.59 to 0.63); p = NR | |||
Giessen 201598 | ORR | OS | Colorectal | Second | Chemotherapy | 22 | 10,509 | Pearson weighted (log-odds ORR vs. log-median OS) | r = 0.58 (95% CI 0.38 to 0.72); p = 0.003 | |||
Louvet 2001115 | ORR | OS | Colorectal | First | Various | 28a | 13,284a | Spearman (ORR vs. median OS) | rs = 0.41; p = 0.0009 | LR (ORR vs. median OS) | OS = 10.45 + 0.088 × ORR | |
Tang 2007138 | ORR | OS | Colorectal | First | Chemotherapy | 39 | 18,668 | Spearman (ORR vs. median OS) | rs = 0.59 (95% CI 0.42 to 0.72); p < 0.000001 | |||
Ichikawa 2006105 | ORR | OS | Gastric | First | Chemotherapy (any) | 25 | 4593 | Spearman weighted (ORR vs. median OS) | rs = 0.45; p < 0.0001 | WLR (ORR vs. median OS) | OS = 5.89 + 0.08 × ORR | |
Ichikawa 2006105 | ORR | OS | Gastric | First | Chemotherapy (novel) | 11a | 1170 | Spearman weighted (ORR vs. median OS) | rs = 0.18; p = 0.12 | |||
Ichikawa 2006105 | ORR | OS | Gastric | First | Chemotherapy (non-novel) | 20a | 3423 | Spearman weighted (ORR vs. median OS) | rs = 0.47; p < 0.0001 | |||
Shitara 2014133 | ORR | OS | Gastric | Second and third | Chemotherapy | 64 | 4286 | Spearman (ORR vs. median OS) | rs = 0.38 (95% CI 0.16 to 0.6); p = NR | |||
Pang 2018124 | ORR | OS | Gastro-oesophageal | First and second | Targeted | 18 | 7892 | Correlation (NR) (ORR vs. median OS) | r = 0.86; p < 0.0001 | |||
Han 2014101 | ORR | OS | Glioblastoma | Various | Various | 91b | 7125b | WLR R2 (ORR vs. median OS) | R2 = 0.22 (95% CI 0.04 to 0.42); p = NR | |||
Ito 2019145 | ORR | OS | Lung (NSCLC) | Various | Immune checkpoint inhibitors (PD-(L)1) | 6 | 3752b | (a) Pearson weighted | (a) r = –0.02; p = 0.4564 | |||
(b) Spearman weighted (ORR vs. median OS) | (b) rs = –0.14; p < 0.0001 | |||||||||||
Ito 2019145 | ORR | OS | Lung (NSCLC) | Various: high PD-L1 expression | Immune checkpoint inhibitors [PD-(L)1] | 7 | 1381 | (a) Pearson weighted | (a) r = 0.92; p < 0.0001; | WLR R2 (ORR vs. median OS) | R2 = 0.84; p = 0.004 | |
(b) Spearman weighted (ORR vs. median OS) | (b) rs = 0.77; p < 0.0001 | |||||||||||
Li 2019112 | ORR | OS | Lung (NSCLC) | First and second | Immune checkpoint inhibitors | 5b | 4803b | Pearson (ORR vs. median OS) | r = 0.52; p = 0.28 | LR (ORR vs. median OS) | R2 = 0.27; p = NR | |
Li 2012113 | ORR | OS | Lung (NSCLC) | First and second | Targeted therapy | 60 | 9903 | WLSR R2 (ORR vs. median OS) | R2 = 0.83; p < 0.000001 | |||
Ritchie 2018128 | ORR | OS | Lung (NSCLC) | All | Immune checkpoint inhibitors [PD-(L)1 or CTLA4] | 8 | NR | Correlation (NR) (ORR vs. 12-month OS) | r = 0.66 (95% CI 0.17 to 1.08); p = NR | |||
Sekine 1999131 | ORR | OS | Lung (NSCLC) | Various | Chemotherapy | 42 | 1935 | Pearson (ORR vs. median OS) | r = 0.62; p < 0.001 | |||
Shukuya 2016134 | ORR | OS | Lung (NSCLC) | All | Immune checkpoint inhibitors [PD-(L)1] | 10b | NR | Spearman weighted (ORR vs. median OS) | rs = 0.45; p = 0.141 | |||
Shukuya 2016134 | ORR | OS | Lung (NSCLC) | All | Chemotherapy (docetaxel) | 22b | NR | Spearman weighted (ORR vs. median OS) | rs = 0.41; p = 0.053 | |||
Tsujino 2009139 | ORR | OS | Lung (NSCLC) | NR | Targeted therapy | 28 | 6171 | LR (ORR vs. median OS) | R2 = NR; p < 0.0001 | Slope: 0.258 | ||
Vidaurre 2009141 | ORR | OS | Lung (NSCLC) | NR | Chemotherapy or targeted therapy | 35 | NR | Regression (NR) (ORR vs. median OS) | R2 = 0.28; p = 0.0024 | |||
Nickolich 2014122 | ORR | OS | Lung (SCLC) | First and second and maintenance: limited or extensive | Various | 66b | 8471b | Pearson (ORR vs. median OS) | r = 0.66; p < 0.0001 | |||
Nickolich 2014122 | ORR | OS | Lung (SCLC) | First and second and maintenance: limited disease | Various | 66b | 8471b | Pearson (ORR vs. median OS) | r = 0.40; p = 0.193 | |||
Nickolich 2014122 | ORR | OS | Lung (SCLC) | First and second and maintenance: extensive disease | Various | 66b | 8471b | Pearson (ORR vs. median OS) | r = 0.44; p = 0.012 | |||
Imaoka 2017106 | ORR | OS | Neuroendocrine | Various | Systemic | 20 | 2530 | Spearman (ORR vs. median OS) | rs = –0.26 (95% CI –0.64 to 0.11); p = 0.164 | |||
Rose 2010129 | ORR | OS | Ovarian | Second | Various | 11 | 407 | (a) Pearson | (a) r = 0.56; p = 0.071 | |||
(b) Kendall Tau-b (ORR vs. median OS) | (b) r = 0.40; p = 0.086 | |||||||||||
Siddiqui 2017135 | ORR | OS | Ovarian | Second and subsequent | Chemotherapy | 31b | 9223b | (a) Pearson weighted (ORR vs. median OS) | (a) r = 0.82; p < 0.001 | (a) WLR R2 (ORR vs. median OS): unadjusted | (a) R2 = 0.67; p = NR | Median OS = 9.48 + 0.28 × ORR |
(b) Pearson unweighted (ORR vs. median OS) | (b) 0.71; p < 0.001 | (b) WLR R2 (ORR vs. median OS):adjusted | (b) Adjusted R2 = 0.66; p = NR | |||||||||
Hamada 2016100 | ORR | OS | Pancreatic | First | Chemotherapy | 47 | 15,906b | Spearman (ORR vs. median OS) | rs = 0.39 (95% CI 0.20 to 0.55); p < 0.001 | |||
Abdel-Rahman 201881 | ORR | OS | Renal cell | Various | Immune checkpoint inhibitors [PD-(L)1] | 4 | 1093 | Pearson (ORR vs. median OS) | r = –0.40; p = 0.436 | |||
Petrelli 2013126 | ORR | OS | Renal cell | First | Targeted | 6b | 3188b | Spearman weighted (ORR vs. median OS) | rs = 0.96; p < 0.0001 | |||
Penel 2014125 | ORR | OS | Unknown primary | NR | NR | 38b | NR | Pearson via WLR (ORR vs. median OS) | r = 0.54; p < 0.0001 | |||
Abdel-Rahman 201881 | ORR | OS | Urothelial | Various | Immune checkpoint inhibitors [PD-(L)1] | 9 | 1699 | Pearson (ORR vs. median OS) | r = –0.12; p = 0.758 | |||
Agarwal 201482 | ORR | OS | Urothelial | Second | Chemotherapy or biologic | 10 | 560 | Pearson (ORR vs. 12-month OS) | r = 0.37; p = 0.30 | (a) WLR R2 (ORR vs. 12-month OS): unadjusted | (a) R2 = 0.26; p = NR | |
(b) WLR R2 (ORR vs. 12-month OS): adjusted (RE) | (b) Adjusted R2 = 0.16; p = 0.1359 | |||||||||||
Agarwal 201482 | ORR | OS | Urothelial | Second: operable | Chemotherapy | NR | 214b | Pearson (ORR vs. 12-month OS) | r = 0.78; p = NR | WLR adjusted R2 (ORR vs. 12-month OS) | Adjusted R2 = 0.54; p = NR | |
Agarwal 201482 | ORR | OS | Urothelial | Second: metastatic | Chemotherapy | NR | 391b | Pearson (ORR vs. 12-month OS) | r = –0.018; p = NR | WLR adjusted R2 (ORR vs. 12-month OS) | Adjusted R2 = –0.13; p = NR | |
Nie 2019123 | ORR | OS | Various solid tumours | Various | Immune checkpoint inhibitors [PD-(L)1] | 43b | 15,088b | Squared Spearman (ORR vs. median OS) | R2s = 0.29; p < 0.001 | |||
Ritchie 2018128 | ORR | OS | Various solid tumours | All | Immune checkpoint inhibitors [PD-(L)1 or CTLA4] | 20b | 10,828b | Correlation (NR) (ORR vs. 12-month OS) | r = 0.08 (95% CI –0.17 to 0.70); p = NR | |||
Vidaurre 2009141 | ORR | OS | Various | NR | Chemotherapy | 85 | 3982a | Regression (NR) (ORR vs. median OS) | R2 = 0.35; p < 0.0001 | |||
Vidaurre 2009141 | ORR | OS | Various | NR | Targeted therapy | 58 | 2992a | Regression (NR) (ORR vs. median OS) | R2 = 0.45; p < 0.0001 | |||
Vidaurre 2009141 | ORR | OS | Various | NR | Chemotherapy or targeted therapy | 143b | 6794b | Regression (NR) (ORR vs. median OS) | R2 = 0.33; p < 0.0001 | |||
CR vs. PFS | ||||||||||||
Nickolich 2014122 | CR | PFS | Lung (SCLC) | First and second and maintenance: limited or extensive | Various | 66b | 8471b | Pearson (CR vs. median PFS) | r = 0.71; p < 0.0001 | |||
Nickolich 2014122 | CR | PFS | Lung (SCLC) | First and second and maintenance: limited disease | Various | 66b | 8471b | Pearson (CR vs. median PFS) | r = 0.22; p = 0.491 | |||
Nickolich 2014122 | CR | PFS | Lung (SCLC) | First and second and maintenance: extensive disease | Various | 66b | 8471b | Pearson (CR vs. median PFS) | r = 0.35; p = 0.116 | |||
Mangal 2018118 | CR | PFS | Multiple myeloma | Second and subsequent | Various | 79b | 13,322b | WLR adjusted R2 (logit CR vs. log-median PFS) | Adjusted R2 = 0.47; p = NR | |||
Mangal 2018117 | CR | PFS | NHL | Various | Various | 73 | 6071 | LR adjusted R2 (logit CR vs. log-median PFS) | Adjusted R2 = 0.57; p = NR | log-(median PFS) = 2.38 + 0.340 × logit (CR) | ||
Zhu 2017144 | CR | PFS | NHL (indolent; follicular) | NR | Chemotherapy, immunotherapy or targeted therapy | 13 | NR | (a) WLR R2: CR vs. median PFS | (a) R2 = 0.69 (95% CI 0.22 to 0.89); p = NR | Median PFS = 0.83 + 0.46 × CR | ||
(b) ) WLR R2: CR vs. 3-year PFS | (b) R2 = 0.44; p = NR | |||||||||||
Zhu 2017144 | CR | PFS | NHL (mantle cell) | NR | Chemotherapy, immunotherapy or targeted therapy | NR | NR | WLR R2 (CR vs. median PFS) | R2 = 0.39; p = NR | |||
CR vs. OS | ||||||||||||
Agarwal 201783 | CR | OS | Acute myeloid leukaemia | First | Systemic | 20b | NR | WLR adjusted R2 (logit CR vs. log-median OS) | Adjusted R2 = 0.48; p = NR | |||
Pang 2018124 | CR | OS | Gastro-oesophageal | First and second | Targeted | 18 | 7892 | Correlation (NR) (CR vs. median OS) | r = 0.43; p = 0.18 | |||
Li 2019112 | CR | OS | Lung (NSCLC) | First and second | Immune checkpoint inhibitors | 5a | 4103a | Pearson (CR vs. median OS) | r = 0.19; p = 0.75 | LR (CR vs. median OS) | R2 = 0.04; p = NR | |
Nickolich 2014122 | CR | OS | Lung (SCLC) | First and second and maintenance: limited or extensive | Various | 66b | 8471b | Pearson (CR vs. median OS) | r = 0.62; p < 0.0001 | |||
Nickolich 2014122 | CR | OS | Lung (SCLC) | First and second and maintenance: limited disease | Various | 66b | 8471b | Pearson (CR vs. median OS) | r = –0.04; p = 0.863 | |||
Nickolich 2014122 | CR | OS | Lung (SCLC) | First and second and maintenance: extensive disease | Various | 66b | 8471b | Pearson (CR vs. median OS) | r = 0.19; p = 0.295 | |||
PR (or VGPR or CR) vs. PFS | ||||||||||||
Nickolich 2014122 | PR | PFS | Lung (SCLC) | First and second and maintenance: limited or extensive | Various | 66b | 8471b | Pearson (PR vs. median PFS) | r = 0.35; p = 0.019 | |||
Nickolich 2014122 | PR | PFS | Lung (SCLC) | First and second and maintenance: limited disease | Various | 66b | 8471b | Pearson (PR vs. median PFS) | r = 0.70; p = 0.011 | |||
Nickolich 2014122 | PR | PFS | Lung (SCLC) | First and second and maintenance: extensive disease | Various | 66b | 8471b | Pearson (PR vs. median PFS) | r = 0.49; p = 0.035 | |||
Mangal 2018118 | VGPR or CR | PFS | Multiple myeloma | Second and subsequent | Various | 79b | 13,322b | WLR adjusted R2 (VGPR or CR vs. median PFS) | Adjusted R2 = 0.64; p = NR | |||
PR vs. OS | ||||||||||||
Nickolich 2014122 | PR | OS | Lung (SCLC) | First and second and maintenance: limited or extensive | Various | 66b | 8471b | Pearson (PR vs. median OS) | r = 0.29; p = 0.018 | |||
Nickolich 2014122 | PR | OS | Lung (SCLC) | First and second and maintenance: limited disease | Various | 66b | 8471b | Pearson (PR vs. median OS) | r = 0.60; p = 0.009 | |||
Nickolich 2014122 | PR | OS | Lung (SCLC) | First and second and maintenance: extensive disease | Various | 66b | 8471b | Pearson (PR vs. median OS) | r = 0.66; p = 0.0002 |
Appendix 6 Treatment effect correlation and regression results (ordered by outcome type, then cancer type, then author)
Study (first author and year) | Surrogate outcome | Final outcome | Cancer | Line subgroups | Treatment | Studies (n) | Patients (n) | Treatment effect correlation methods | Correlation coefficient | Treatment effect regression methods | Regression R2 | Linear regression equation | STE | IQWiG | BSES2 |
---|---|---|---|---|---|---|---|---|---|---|---|---|---|---|---|
ORR vs. PFS | |||||||||||||||
Burzykowski 200887 | ORR | PFS | Breast | First | Chemotherapy | 11 | 3953 | Spearman via LR with Plackett copula (log-OR ORR vs. log-HR PFS) | rs = 0.96 (95% CI 0.73 to 1.19); p = NR | LR | log-HR PFS = 0.10 + 0.50 × log-OR ORR | NR | Medium + | NE | |
Ciani 201589 Elia 202096 | ORR | PFS | Colorectal | All | Systemic | 33 | NR | LR: adjusted R2 (log-OR ORR vs. log-HR PFS) | Adjusted R2 = 0.61 (95% CI 0.27 to 0.87); p = NR | log-HR PFS = –0.05 – 0.32 × log-OR ORR | NR | Medium | NE | ||
Ciani 201589 Elia 202096 | ORR | PFS | Colorectal | All: no crossover | Systemic | 7 | NR | LR: adjusted R2 (log-OR ORR vs. log-HR PFS) | Adjusted R2 = 0.63 (95% CI 0.03 to 0.99); p = NR | log-HR PFS = –0.05 – 0.31 × log-OR ORR | NR | Medium | NE | ||
Tsujino 2010140 | ORR | PFS | Colorectal | NR | Targeted | 7 | NR | LR (unweighted) R2 (difference in ORR vs. HR PFS) | R2 = 0.65; p = 0.029 | Slope: –0.037 | NR | Medium | NE | ||
Blumenthal 201785 | ORR | PFS | Lung (NSCLC) | Various | Chemotherapy, immune checkpoint inhibitors or targeted therapy | 25 | 20,013b | (a) WLR R2: OR ORR vs. HR PFS | (a) R2 = 0.74 (95% CI 0.55 to 0.88); p = NR | NR | Medium + | NE | |||
(b) WLR R2: 6-month ratio ORR vs. HR PFS | (b) R2 = 0.70 (95% CI 0.50 to 0.84); p = NR | ||||||||||||||
Blumenthal 201584 | ORR | PFS | Lung (NSCLC) | Various | Chemotherapy or targeted therapy | 14 | 12,567b | WLR R2 (log-OR ORR vs. log-HR PFS) | R2 = 0.89 (95% CI 0.80 to 0.98); p = NR | NR | Medium + | NE | |||
Blumenthal 201584 | ORR | PFS | Lung (NSCLC) | Various | Chemotherapy | 11 | 11,701b | WLR R2 (log-OR ORR vs. log-HR PFS) | R2 = 0.77 (95% CI 0.58 to 0.96); p = NR | NR | Medium + | NE | |||
Ito 2019145 | ORR | PFS | Lung (NSCLC) | Various | Immune [PD-(L)1] | 6 | 3752b | (a) Pearson weighted | (a) r = –0.87; p < 0.0001 | WLR R2 (OR ORR vs. HR PFS) | R2 = 0.76; p = 0.011 | NR | Medium + | Fair | |
(b) Spearman weighted (OR ORR vs. HR PFS) | (b) rs = –0.97; p < 0.0001 | ||||||||||||||
Ito 2019145 | ORR | PFS | Lung (NSCLC) | Various: high PD-L1 expression | Immune checkpoint inhibitors [PD-(L)1] | 7 | 1381 | (a) Pearson weighted | (a) r = 0.67; p < 0.0001 | WLR R2 (OR ORR vs. HR PFS) | R2 = 0.45; p = 0.101 | NR | Low | Good | |
(b) Spearman weighted (OR ORR vs. HR PFS) | (b) rs = 0.56; p < 0.0001 | ||||||||||||||
Ritchie 2018128 | ORR | PFS | Lung (NSCLC) | All | Immune checkpoint inhibitors [PD-(L)1 or CTLA4] | 8 | NR | Correlation (NR), weighted (OR ORR vs. HR PFS) | r = 0.74 (95% CI 0.38 to 1.08); p = NR | NR | Medium | Good | |||
Roviello 2017130 | ORR | PFS | Lung (NSCLC) | Various | Immune checkpoint inhibitors | 7a | 3369a | WLR R2 (log-OR ORR vs. log-HR PFS) | R2 = 0.42 (95% CI 0.003 to 0.85); p = 0.06 | NR | Low | NE | |||
Tsujino 2010140 | ORR | PFS | Lung (NSCLC) | NR | Targeted | 6 | NR | LR (unweighted) R2 (difference in ORR vs. HR PFS) | R2 = 0.94; p = 0.002 | Slope: –0.015 | NR | Medium + | NE | ||
Colloca 201790 | ORR | PFS | Ovarian | First | Chemotherapy | 29 | NR | Spearman (difference in ORR vs. difference in median PFS) | rs = 0.64; p < 0.001 | LR R2 (log-RR ORR vs. log-HR PFS) | R2 = 0.28; p = 0.005 | NR | Low | NE | |
Colloca 201790 | ORR | PFS | Ovarian | First: published 1990–2002 | Chemotherapy | 15 | NR | Spearman (difference in ORR vs. difference in median PFS) | rs = 0.64; p = 0.018 | LR R2 (log-RR ORR vs. log-HR PFS) | R2 = 0.32; p = 0.046 | NR | Low | NE | |
Colloca 201790 | ORR | PFS | Ovarian | First: published 2003–16 | Chemotherapy | 16 | NR | Spearman (difference in ORR vs. difference in median PFS) | rs = 0.58; p = 0.019 | LR R2 (log-RR ORR vs. log-HR PFS) | R2 = 0.53; p = 0.003 | NR | Medium | NE | |
Siddiqui 2017135 | ORR | PFS | Ovarian | Second and subsequent | Chemotherapy | 39b | 9223b | Pearson weighted (OR ORR vs. HR PFS) | r = 0.42; p = NR | NR | Low | Poor | |||
Colloca 201691 | ORR | PFS | Pancreatic | First | Gemcitabine and chemotherapy or targeted therapy | 33a | NR | Spearman (difference in ORR vs. difference in median PFS) | rs = 0.34; p = NR | NR | Low | NE | |||
Colloca 201691 | ORR | PFS | Pancreatic | First | Gemcitabine and targeted therapy | 14a | NR | Spearman (difference in ORR vs. difference median PFS) | rs = 0.25; p = NR | NR | Low | NE | |||
Ritchie 2018128 | ORR | PFS | Various solid tumours | All | Immune checkpoint inhibitors [PD-(L)1 or CTLA4] | 20b | 10,828b | Correlation (NR), weighted (OR ORR vs. HR PFS) | r = 0.63 (95% CI 0.35 to 0.89); p = NR | NR | Medium | Poor | |||
Roviello 2017130 | ORR | PFS | Various solid tumours | Various | Immune checkpoint inhibitors | 17b | 8994b | WLR R2 (log-OR ORR vs. log-HR PFS) | R2 = 0.32 (95% CI 0.02 to 0.76); p = 0.01 | log-HR PFS = –0.1281 – 0.2384 × log-OR ORR | NR | Low | NE | ||
Roviello 2017130 | ORR | PFS | Various solid tumours | Various | Immune checkpoint inhibitors (CTLA-4) | 17b | 8994b | WLR R2 (log-OR ORR vs. log-HR PFS) | R2 = 0.67 (95% CI 0.02 to 1.00); p = 0.05 | NR | Medium | NE | |||
Roviello 2017130 | ORR | PFS | Various solid tumours | Various | Immune checkpoint inhibitors [PD-(L)1] | 17b | 8994b | WLR R2 (log-OR ORR vs. log-HR PFS) | R2 = 0.25 (95% CI 0.02 to 1.00); p = 0.08 | NR | Low | NE | |||
Tsujino 2010140 | ORR | PFS | Various solid tumours | NR | Targeted | 17 | NR | LR (unweighted) R2 (difference in ORR vs. HR PFS) | R2 = 0.50; p = 0.001 | Slope: –0.022 | 15% | Medium | NE | ||
Wilkerson 2009142 | ORR | PFS | Various solid tumours | NR | NR | 66b | NR | (a) LR (unweighted R2): difference in ORR vs. HR PFS | (a) R2 = 0.45; p < 0.0001 | NR | Medium | NE | |||
(b) LR (unweighted R2): difference in ORR vs. difference in median PFS | (b) R2 = 0.62; p < 0.0001 | ||||||||||||||
ORR vs. OS | |||||||||||||||
Moriwaki 2016119 | ORR | OS | Biliary tract | First | Chemotherapy | 17b | 2040 | WLR R2 (ratio ORR vs. log-ratio median OS) | R2 = 0.29 (95% CI 0.01 to 0.65); p = 0.021 | log-ratio median OS = 0.013 + 0.282 × ratio ORR | NR | Low | NE | ||
Moriwaki 2016119 | ORR | OS | Biliary tract | First | Chemotherapy (gemcitabine) | 14b | 1880 | WLR R2 (ratio ORR vs. log-ratio median OS) | R2 = 0.39 (95% CI 0.02 to 0.75); p = 0.013 | log-ratio median OS = 0.020 + 0.268 × ratio ORR | NR | Low | NE | ||
Moriwaki 2016119 | ORR | OS | Biliary tract | First | Targeted | 6b | 953 | WLR R2 (ratio ORR vs. log-ratio median OS) | R2 = 0.43 (95% CI 0.03 to 0.89); p = 0.090 | log-ratio median OS = 0.119 + 0.155 × ratio ORR | NR | Low | NE | ||
Bruzzi 200586 | ORR | OS | Breast | All | Chemotherapy | 10 | 2126 | (a) WLR R2: log-OR ORR vs. log-HR OS | (a) R2 = 0.10 (95% CI 0.00 to 0.43); p = NR | NR | Low | NE | |||
(b) WLR R2: difference in ORR vs. difference in median OS | (b) R2 = 0.20 (95% CI 0 to 0.65); p = NR | ||||||||||||||
Burzykowski 200887 | ORR | OS | Breast | First | Chemotherapy | 11 | 3953 | Spearman via LR with Plackett copula (log-OR ORR vs. log-HR OS) | rs = 0.57 (95% CI –0.31 to 1.44); p = NR | NR | Medium | NE | |||
Hackshaw 200599 | ORR | OS | Breast | First | Chemotherapy | 42a | 9163 | WLR R2 (log-OR ORR vs. log-HR OS) | R2 = 0.34; p < 0.0001 | log-HR OS = –0.0081 + 0.28 × log-OR ORRSlope: 0.28 | NR | Low | NE | ||
Hackshaw 200599 | ORR | OS | Breast | First: recruited pre-1990 | Chemotherapy | 26a | 5244a | WLR R2 (log-OR ORR vs. log-HR OS) | R2 = 0.26; p = 0.004 | Slope: 0.28 | NR | Low | NE | ||
Hackshaw 200599 | ORR | OS | Breast | First: recruited 1990 or after | Chemotherapy | 16a | 3919a | WLR R2 (log-OR ORR vs. log-HR OS) | R2 = 0.41; p = 0.005 | Slope: 0.24 | NR | Low | NE | ||
Buyse 200088 | ORR | OS | Colorectal | First | Chemotherapy | 25 | 3791 | WLR R2 (log-OR ORR vs. log-HR OS) | R2 = 0.38 (95% CI 0.09 to 0.68); p = NR | NR | Low | NE | |||
Ciani 201589 Elia 202096 | ORR | OS | Colorectal | All | Systemic | 32 | NR | Spearman (log-OR ORR vs. log-OR OS) | rs = 0.53; p < 0.01 | (a) WLSR R2 (log-OR ORR vs. log-OR OS) (time point NR) | (a) R2 = 0.06 (95% CI 0.01 to 0.29); p = NR | log-HR OS = –0.03 – 0.05 × log-OR ORR | 0.28 | Low | NE |
(b) Adjusted R2 (log-OR ORR vs. log-HR OS) | (b) Adjusted R2 = 0.33 (95% CI 0.00 to 0.91); p = NR | ||||||||||||||
Ciani 201589 Elia 202096 | ORR | OS | Colorectal | All: no crossover | Systemic | 7 | NR | LR: adjusted R2 (log-OR ORR vs. log-HR OS) | Adjusted R2 = 0.40 (95% CI 0.00 to 0.96); p = NR | log-HR OS = –0.04 – 0.10 × log-OR ORR | NR | Low | NE | ||
Colloca 201692 | ORR | OS | Colorectal | First | Bevacizumab and chemotherapy | 11 | NR | Spearman (difference in ORR vs. difference in median OS) | rs = 0.82; p < 0.001 | LR R2 (difference in ORR vs. difference in median OS) | R2 = 0.58; p = 0.002 | NR | Medium | NE | |
Cremolini 201794 | ORR | OS | Colorectal | Second | Targeted | 20a | 7571 | (a) Pearson (via WLR): rr ORR vs. HR OS | (a) r = 0.17; p = 0.476 | (a) WLR R2: rr ORR vs. HR OS | (a) R2 = 0.03; p = 0.476 | (a) Slope: 0.029 | NR | Low | NE |
(b) Pearson (via WLR): difference in ORR vs. difference in median OS | (b) r = 0.35; p = 0.092 | (b) WLR R2: difference in ORR vs. difference in median OS | (b) R2 = 0.12; p = 0.092 | (b) Slope: 0.071 | |||||||||||
Cremolini 201794 | ORR | OS | Colorectal | Second | Targeted, anti-angiogenic | 13a | NR | (a) Pearson (via WLR): rr ORR vs. HR OS | (a) r = 0.36; p = 0.249 | (a) WLR R2: rr ORR vs. HR OS | (b) R2 = 0.13; p = 0.249 | (a) Slope: –0.113 | NR | Low | NE |
(b) Pearson (via WLR): difference in ORR vs. difference in median OS | (b) r = 0.52; p = 0.038 | (b) WLR R2: difference in ORR vs. difference in median OS | (b) R2 = 0.27; p = 0.038 | (b) Slope: 0.133 | |||||||||||
Cremolini 201794 | ORR | OS | Colorectal | Second | Targeted, not anti-angiogenic | 7a | NR | (a) Pearson (via WLR): rr ORR vs. HR OS | (a) r = 0.44; p = 0.274 | (a) WLR R2: rr ORR vs. HR OS | (b) R2 = 0.20; p = 0.274 | (a) Slope: –0.064 | NR | Low | NE |
(b) Pearson (via WLR): difference in ORR vs. difference in median OS | (b) r = 0.63; p = 0.068 | (b) WLR R2: difference in ORR vs. difference in median OS | (b) R2 = 0.40; p = 0.068 | (b) Slope: 0.143 | |||||||||||
Johnson 2006109 | ORR | OS | Colorectal | First | Chemotherapy | 146b | 35,337b | WLSR R2 (difference in ORR vs. difference om median OS) | R2 = 0.10; p < 0.0001 | Difference in median OS = 0.340 + 0.096 × difference in ORR | NR | Low | NE | ||
Sidhu 2013136 | ORR | OS | Colorectal | First (most) | Chemotherapy ± targeted | 24b | 20,438b | (a) Correlation (NR): OR ORR vs. HR OS | (a) r = 0.62 (95% CI 0.37 to 0.79); p = NR | (a) LR (unweighted) R2: OR ORR vs. HR OS | (a) R2 = 0.39 (95% CI 0.13 to 0.62); p = NR | NR | Medium | NE | |
(b) Correlation (NR): difference in ORR vs. HR OS | (b) r = 0.64 (95% CI 0.39 to 0.79); p = NR | (b) LR (unweighted) R2: difference in ORR vs. HR OS | (b) R2 = 0.41 (95% CI 0.15 to 0.63); p = NR | ||||||||||||
(c) Correlation (NR): ratio ORR vs. HR OS | (c) r = 0.52 (95% CI 0.23 to 0.72); p = NR | (c) LR (unweighted) R2: ratio ORR vs. HR OS | (c) R2 = 0.27 (95% CI 0.05 to 0.52); p = NR | ||||||||||||
Sidhu 2013136 | ORR | OS | Colorectal | First (most) | Targeted and chemotherapy | 13 | 12,060a | (a) Correlation (NR): OR ORR vs. HR OS | (a) r = 0.50 (95% CI 0.05 to 0.75); p = NR | (a) LR (unweighted) R2: OR ORR vs. HR OS | (a) R2 = 0.25 (95% CI 0.00 to 0.57); p = NR | NR | Medium | NE | |
(b) Correlation (NR): difference in ORR vs. HR OS | (b) r = 0.58 (95% CI 0.19 to 0.80); p = NR | (b) LR (unweighted) R2: difference in ORR vs. HR OS | (b) R2 = 0.33 (95% CI 0.04 to 0.64); p = NR | ||||||||||||
(c) Correlation (NR): ratio ORR vs. HR OS | (c) r = 0.42 (95% CI 0.00 to 0.71); p = NR | (c) LR (unweighted) R2: ratio ORR vs. HR OS | (c) R2 = 0.18 (95% CI 0.00 to 0.51); p = NR | ||||||||||||
Sidhu 2013136 | ORR | OS | Colorectal | First (most) | Targeted (anti-EGFR) | 9 | 7792a | (a) Correlation (NR): OR ORR vs. HR OS | (a) r = 0.67 (95% CI 0.27 to 0.86); p = NR | (a) LR (unweighted) R2: OR ORR vs. HR OS | (a) R2 = 0.45 (95% CI 0.07 to 0.74), p = NR | NR | Medium | NE | |
(b) Correlation (NR): difference in ORR vs. HR OS | (b) r = 0.72 (95% CI 0.35 to 0.88); p = NR | (b) LR (unweighted) R2: difference in ORR vs. HR OS | (b) R2 = 0.52 (95% CI 0.12 to 0.78); p = NR | ||||||||||||
(c) Correlation (NR): ratio ORR vs. HR OS | (c) r = 0.52 (95% CI 0.00 to 0.79); p = NR | (c) LR (unweighted) R2: ratio ORR vs. HR OS | (c) R2 = 0.27 (95% CI 0.00 to 0.62); p = NR | ||||||||||||
Sidhu 2013136 | ORR | OS | Colorectal | First (most) | Targeted (anti-EGFR), KRAS non-mutant | 6a | 4916a | (a) Correlation (NR): OR ORR vs. HR OS | (a) r = 0.68 (95% CI 0.07 to 0.89); p = NR | (a) LR (unweighted) R2: OR ORR vs. HR OS | (a) R2 = 0.46 (95% CI 0.01 to 0.80); p = NR | NR | Medium | NE | |
(b) Correlation (NR): difference in ORR vs. HR OS | (b) r = 0.81 (95% CI 0.38 to 0.94); p = NR | (b) LR (unweighted) R2: difference in ORR vs. HR OS | (b) R2 = 0.65 (95% CI 0.15 to 0.88); p = NR | ||||||||||||
(c) Correlation (NR): ratio ORR vs. HR OS | (c) r = 0.48 (95% CI 0.00 to 0.82); p = NR | (c) LR (unweighted) R2: ratio ORR vs. HR OS | (c) R2 = 0.23 (95% CI 0.00 to 0.67); p = NR | ||||||||||||
Tang 2007138 | ORR | OS | Colorectal | First | Chemotherapy | 39 | 18,668 | Spearman (difference in ORR vs. difference in median OS) | rs = 0.39 (95% CI 0.08 to 0.63); p = 0.015 | NR | Low | Poor | |||
Tsujino 2010140 | ORR | OS | Colorectal | NR | Targeted therapy | 7 | NR | LR (unweighted) R2 (difference in ORR vs. HR OS) | R2 = 0.51; p = 0.072 | Slope: 0.029 | NR | Medium | NE | ||
Blumenthal 201785 | ORR | OS | Lung (NSCLC) | Various | Chemotherapy, immune checkpoint inhibitors or targeted therapy | 25 | 20,013b | (a) WLR R2: OR ORR vs. HR OS | (a) R2 = 0.04 (95% CI 0.0002 to 0.28); p = NR | NR | Low | NE | |||
(b) WLR R2: 6-month ratio ORR vs. HR OS | (b) R2 = 0.05 (95% CI 0.0001 to 0.31); p = NR | ||||||||||||||
Blumenthal 201584 | ORR | OS | Lung (NSCLC) | Various | Chemotherapy or targeted therapy | 14 | 12,567b | WLR R2 (log-OR ORR vs. log-HR OS) | R2 = 0.09 (95% CI 0 to 0.33); p = NR | NR | Low | NE | |||
Blumenthal 201584 | ORR | OS | Lung (NSCLC) | Various | Chemotherapy | 11 | 11,701b | WLR R2 (log-OR ORR vs. log-HR OS) | R2 = 0.44 (95% CI 0.08 to 0.80); p = NR | NR | Low | NE | |||
Hashim 2018102 | ORR | OS | Lung (NSCLC) | Second and subsequent | Various | 140 | 41,725 | (a) Correlation (NR) via WLR: difference in ORR vs. log-HR OS | (a) r = 0.17 (95% CI 0.00 to 0.38); p = NR | NA | Low | NE | |||
(b) Correlation (NR) via WLR: difference in ORR vs. difference in median OS | (b) r = 0.18 (95% CI 0.02 to 0.34); p = 0.032 | ||||||||||||||
Hashim 2018102 | ORR | OS | Lung (NSCLC) | Second and Phase III | Various | 59 | 32,348 | (a) Correlation (NR) via WLR: difference in ORR vs. log-HR OS | (a) r = 0.37 (95% CI 0.09 to 0.60); p = NR | NA | Low | NE | |||
(b) Correlation (NR) via WLR: difference in ORR vs. difference in median OS | (b) r = 0.13 (95% CI 0.00 to 0.38); p = 0.32 | ||||||||||||||
Hashim 2018102 | ORR | OS | Lung (NSCLC) | Second and Phase III, excluding per–protocol crossover | Various | 54 | 30,654 | (a) Correlation (NR) via WLR: difference in ORR vs. log-HR OS | (a) r = 0.40 (95% CI 0.10 to 0.63); p = NR | NA | Low | NE | |||
(b) Correlation (NR) via WLR: difference in ORR vs. difference in median OS | (b) r = 0.36 (95% CI 0.10 to 0.57); p = 0.0074 | ||||||||||||||
Hashim 2018102 | ORR | OS | Lung (NSCLC) | Second and Phase III, excluding per–protocol crossover | Various | 38 | 22,574 | (a) Correlation (NR) via WLR: difference in ORR vs. log-HR OS | (a) r = 0.52 (95% CI 0.18 to 0.75); p = NR | (a) 55% | Medium | NE | |||
(b) Correlation (NR) via WLR: difference in ORR vs. difference in median OS | (b) r = 0.45 (95% CI 0.15 to 0.67); p = 0.0051 | (b) NA | |||||||||||||
Hashim 2018102 | ORR | OS | Lung (NSCLC) | Second and Phase III, excluding crossover or unbalanced post-progression treatments | Various | 18 | 13,349 | (a) Correlation (NR) via WLR: difference in ORR vs. log-HR OS | (a) r = 0.16 (95% CI 0.00 to 0.60); p = NR | (a) NA | Low | NE | |||
(b) Correlation (NR) via WLR: difference in ORR vs. difference in median OS | (b) r = 0.53 (95% CI 0.08 to 0.80); p = 0.024 | (b) 41% | |||||||||||||
Hotta 2015103 | ORR | OS | Lung (NSCLC) | Various | Targeted therapy | 18 | 7633b | WLR R2 (OR ORR vs. HR OS) | R2 = 0.10; p = NR | NR | Low | NE | |||
Hotta 2015103 | ORR | OS | Lung (NSCLC) | Various and molecularly selected | Targeted therapy | 8 | NR | WLR R2 (OR ORR vs. HR OS) | R2 = 0.04; p = NR | NR | Low | NE | |||
Hotta 2015103 | ORR | OS | Lung (NSCLC) | Various: non-molecularly selected | Targeted therapy | 10 | NR | WLR R2 (OR ORR vs. HR OS) | R2 = 0.43; p = NR | NR | Low | NE | |||
Ito 2019145 | ORR | OS | Lung (NSCLC) | Various | Immune checkpoint inhibitors [PD-(L)1] | 6 | 3752b | (a) Pearson weighted | (a) r = –0.75; p < 0.0001 | WLR R2 (OR ORR vs. HR OS) | R2 = 0.57; p = 0.051 | NR | Medium | Poor | |
(b) Spearman weighted (OR ORR vs. HR OS) | (b) rs = –0.96; p < 0.0001 | ||||||||||||||
Ito 2019145 | ORR | OS | Lung (NSCLC) | Various: high PD-L1 expression | Immune checkpoint inhibitors [PD-(L)1] | 7 | 1381 | (a) Pearson weighted | (a) r = –0.50; p < 0.0001 | WLR R2 (OR ORR vs. HR OS) | R2 = 0.25; p = 0.253 | NR | Low | Fair | |
(b) Spearman weighted (OR ORR vs. HR OS) | (b) rs = –0.21; p < 0.0001 | ||||||||||||||
Johnson 2006109 | ORR | OS | Lung (NSCLC) | First | Chemotherapy | 191b | 44,125b | WLSR R2 (difference in ORR vs. difference in median OS) | R2 = 0.16; p < 0.0001 | Difference in median OS = –0.048 + 0.090 × difference in ORR | NR | Low | NE | ||
Nakashima 2016121 | ORR | OS | Lung (NSCLC) | First | Chemotherapy | 44 | 22,709 | Spearman, weighted (ln-OR ORR vs. HR OS) | rs = 0.57; p = NR | WLSR adjusted R2 (ln-OR ORR vs. ln-HR OS) | Adjusted R2 = 0.35; p = NR | ln-HR OS = –0.023 – 0.133 × ln-OR ORR | NR | Low | NE |
Ritchie 2018128 | ORR | OS | Lung (NSCLC) | All | Immune checkpoint inhibitors [PD-(L)1 or CTLA4] | 8 | NR | Correlation (NR) weighted (OR ORR vs. HR OS) | r = 0.68 (95% CI 0.08 to 1.10); p = NR | NR | Low | Good | |||
Roviello 2017130 | ORR | OS | Lung (NSCLC) | Various | Immune checkpoint inhibitors | 7a | 3369a | WLR R2 (log-OR ORR vs. log-HR OS) | R2 = 0.0007 (95% CI 0.09 to 0.91); p = 0.94 | NR | Low | NE | |||
Tsujino 2010140 | ORR | OS | Lung (NSCLC) | NR | Targeted therapy | 5 | NR | LR (unweighted) R2 (difference in ORR vs. HR OS) | R2 = 0.84; p = 0.030 | Slope: –0.011 | NR | Medium + | NE | ||
Foster 201197 | ORR | OS | Lung (SCLC) | First | Chemotherapy | 3 (32 centres) | 596b | Spearman (log-OR ORR vs. log-HR OS) | rs = 0.52; p = NR | WLSR R2 (log-OR ORR vs. log-HR OS) | R2 = 0.21; p = NR | NR | Low | NE | |
Hotta 2009104 | ORR | OS | Lung (SCLC) | First | Chemotherapy | 48 | 8779 | WLR R2 (rr ORR vs. difference in median OS) | R2 = 0.33; p = NR | Difference in median OS = 0.00 + 0.06 × rr ORR | NR | Low | NE | ||
Hotta 2009104 | ORR | OS | Lung (SCLC) | First: clear criteria | Chemotherapy | 43 comparisons | NR | WLR R2 (rr ORR vs. difference in median OS) | R2 = 0.19; p = NR | NR | Low | NE | |||
Hotta 2009104 | ORR | OS | Lung (SCLC) | First: WHO criteria | Chemotherapy | 23 comparisons | NR | WLR R2 (rr ORR vs. difference in median OS) | R2 = 0.13; p = NR | NR | Low | NE | |||
Hotta 2009104 | ORR | OS | Lung (SCLC) | First: non-WHO criteria | Chemotherapy | 20 comp | NR | WLR R2 (rr ORR vs. difference in median OS) | R2 = 0.28; p = NR | NR | Low | NE | |||
Hotta 2009104 | ORR | OS | Lung (SCLC) | First: published 1990–6 | Chemotherapy | 26 comp | NR | WLR R2 (rr ORR vs. difference in median OS) | R2 = 0.23; p = NR | Difference in median OS = 0.00 + 0.04 × rr ORR | NR | Low | NE | ||
Hotta 2009104 | ORR | OS | Lung (SCLC) | First: published 1997–2008 | Chemotherapy | 26 comp | NR | WLR R2 (rr ORR vs. difference in median OS) | R2 = 0.47; p = NR | Difference in median OS = 0.00 + 0.09 × rr ORR | NR | Low | NE | ||
Colloca 201790 | ORR | OS | Ovarian | First | Chemotherapy | 27 | NR | Spearman (difference in ORR vs. difference in median OS) | rs = 0.41; p = 0.035 | LR R2 (log-RR ORR vs. log-HR OS) | R2 = 0.12; p = 0.073 | NR | Low | NE | |
Colloca 201790 | ORR | OS | Ovarian | First: published 1990–2002 | Chemotherapy | 13 | NR | Spearman (difference in ORR vs. difference in median OS) | rs = 0.65; p = 0.016 | LR R2 (log-RR ORR vs. log-HR OS) | R2 = 0.15; p = 0.199 | NR | Low | NE | |
Colloca 201790 | ORR | OS | Ovarian | First: published 2003–16 | Chemotherapy | 14 | NR | Spearman (difference in ORR vs. difference in median OS) | rs = –0.02; p = 0.940 | LR R2 (log-RR ORR vs. log-HR OS) | R2 = 0.34; p = 0.027 | NR | Low | NE | |
Siddiqui 2017135 | ORR | OS | Ovarian | Second and subsequent | Chemotherapy | 31b | 9223b | NR | NE | NE | |||||
Colloca 201691 | ORR | OS | Pancreatic | First | Gemcitabine and chemotherapy or targeted therapy | 36a | NR | Spearman (difference in ORR vs. difference in median OS) | rs = 0.29; p = 0.067 | NR | Low | NE | |||
Colloca 201691 | ORR | OS | Pancreatic | First | Gemcitabine and chemotherapy | 22a | NR | Spearman (difference in ORR vs. difference in median OS) | rs = 0.23; p = 0.250 | LR R2 (log-RR ORR vs. log-HR OS) | R2 = 0.15; p = NR | NR | Low | NE | |
Colloca 201691 | ORR | OS | Pancreatic | First | Gemcitabine and targeted therapy | 14a | NR | Spearman (difference in ORR vs. difference in median OS) | rs = 0.55; p = 0.035 | LR R2 (log-RR ORR vs. log-HR OS) | R2 = 0.28; p = NR | NR | Low | NE | |
Hamada 2016100 | ORR | OS | Pancreatic | First | Chemotherapy | 36 | 15,906b | Spearman via WLSR (log-OR ORR vs. log-HR OS) | rs = –0.16 (95% CI –0.27 to –0.05); p = 0.007 | WLSR adjusted R2 (log-OR ORR vs. log-HR OS) | Adjusted R2 = 0.30; p = 0.007 | NR | Low | Poor | |
Makris 2017116 | ORR | OS | Pancreatic (adenocarcinoma) | First | Chemotherapy (gemcitabine) | 22a | 10,379a | (a) Pearson (log-HR OS vs. log-OR ORR): weighted by sample size | (a) r = 0.27 (95% CI –0.14 to 0.60); p = 0.20 | NR | Low | NE | |||
(b) Pearson (log-HR OS vs. log-OR ORR): fixed effect | (b) r = 0.52 (95% CI 0.16 to 0.76); p = 0.007 | ||||||||||||||
(c) Pearson (log-HR OS vs. log-OR ORR): random effects | (c) r = 0.45 (95% CI 0.07 to 0.72); p = 0.02 | ||||||||||||||
Colloca 201693 | ORR | OS | Prostate | First and second | Chemotherapy, hormonal and targeted therapy | 17 | NR | Pearson (difference in ORR vs. difference in median OS) | r = 0.38; p = 0.132 | LR R2 (log-RR ORR vs. log-HR OS) | R2 = 0.007; p = 0.789 | NR | Low | NE | |
Colloca 201693 | ORR | OS | Prostate | First and second: published 1995–2004 | Chemotherapy, hormonal and targeted therapy | 5 | NR | Pearson (difference in ORR vs. difference in median OS) | r = 0.35; p = 0.560 | LR R2 (log-RR ORR vs. log-HR OS) | R2 = 0.53; p = 0.275 | NR | Medium | NE | |
Colloca 201693 | ORR | OS | Prostate | First and second: published 2005–14 | Chemotherapy, hormonal and targeted therapy | 12 | NR | Pearson (difference in ORR vs. difference in median OS) | r = 0.41; p = 0.185 | LR R2 (log-RR ORR vs. log-HR OS) | R2 = 0.02; p = 0.690 | NR | Low | NE | |
Delea 201295 | ORR | OS | Renal cell | NR | Cytokine or targeted therapy | 25a | 10,943b | Pearson weighted (ln-rr ORR vs. –ln-HR OS) | r = 0.78; p < 0.0001 | WLSR adjusted R2 (ln-rr ORR vs. –ln-HR OS) | Adjusted R2 = 0.59; p < 0.0001 | –ln-HR OS = –0.11 + 0.30 × ln-rr ORR | NR | Medium | NE |
Petrelli 2013126 | ORR | OS | Renal cell | First | Targeted therapy | 6b | 3188b | (a) Pearson weighted | (a) r = 0.52; p < 0.0001 | LR | R2 = 0.27; p = NR | NR | Low | Fair | |
(b) Spearman weighted (difference in median OS vs. difference in ORR) | (b) rs = 0.49; p < 0.0001 | ||||||||||||||
Tanaka 2019137 | ORR | OS | Soft tissue sarcoma | First | Chemotherapy | 27b | 6156b | Kendall’s Tau (log-OR ORR vs. log-HR OS) | τ = 0.41; p = NR | Regression (NR) R2 (log-OR ORR vs. log-HR OS) | R2 = 0.28 (95% CI 0.02 to 0.54); p = NR | NR | Low | NE | |
Zer 2016143 | ORR | OS | Soft tissue sarcoma | All | Systemic | 52b | 9762b | Correlation (NR) via WLR (OR ORR vs. HR OS) | r = 0.51; p = NR | NR | Low | NE | |||
Kaufman 2018110 | ORR | OS | Various solid tumours | Various | Immune checkpoint inhibitors and chemotherapy | 27b | 10,300b | WLR adjusted R2 (OR ORR vs. HR OS) | Adjusted R2 = –0.07; p = 0.866 | NR | NE | NE | |||
Kaufman 2018110 | ORR | OS | Various solid tumours | Various | Immune checkpoint inhibitors alone | NR | NR | WLR adjusted R2 (OR ORR vs. HR OS) | Adjusted R2 = –0.08; p = 0.799 | NR | NE | NE | |||
Mushti 2018120 | ORR | OS | Various solid tumours | NR | Immune checkpoint inhibitors [PD-(L)1] | 13 | 6722 | WLR R2 (OR ORR vs. HR OS) | R2 = 0.13; p = NR | NR | Low | NE | |||
Nie 2019123 | ORR | OS | Various solid tumours | Various | Immune checkpoint inhibitors [PD-(L)1] | 43b | 15,088b | WLR R2 (ln-OR ORR vs. ln-HR OS) | R2 = 0.10; p = 0.053 | NR | Low | Poor | |||
Ritchie 2018128 | ORR | OS | Various solid tumours | All | Immune checkpoint inhibitors [PD-(L)1 or CTLA4] | 20b | 10,828b | Correlation (NR), weighted (OR ORR vs. HR OS) | r = 0.57 (95% CI 0.23 to 0.89); p = NR | NR | Low | Poor | |||
Roviello 2017130 | ORR | OS | Various solid tumours | Various | Immune checkpoint inhibitors | 17b | 8994b | WLR R2 (log-OR ORR vs. log-HR OS) | R2 = 0.47 (95% CI 0.03 to 0.77); p = 0.001 | log-HR OS = –0.1329 – 0.2575 × log-OR ORR | NR | Low | NE | ||
Roviello 2017130 | ORR | OS | Various solid tumours | Various | Immune checkpoint inhibitors (CTLA-4) | 17b | 8994b | WLR R2 (log-OR ORR vs. log-HR OS) | R2 = 0.00 (95% CI 0.00 to 0.97); p = 0.96 | NR | Low | NE | |||
Roviello 2017130 | ORR | OS | Various solid tumours | Various | Immune checkpoint inhibitors [PD-(L)1] | 17b | 8994b | WLR R2 (log-OR ORR vs. log-HR OS) | R2 = 0.18 (95% CI 0.00 to 0.97); p = 0.17 | NR | Low | NE | |||
Tsujino 2010140 | ORR | OS | Various solid tumours | NR | Targeted therapy | 18 | NR | LR (unweighted) R2 (difference in ORR vs. HR OS) | R2 = 0.47; p = 0.002 | Slope: –0.016 | 21% | Low | NE | ||
Wilkerson 2009142 | ORR | OS | Various solid tumours | NR | NR | 66b | NR | (a) LR (unweighted R2): difference in ORR vs. HR OS | (a) R2 = 0.37; p < 0.0001 | NR | Low | NE | |||
b) LR (unweighted R2): difference in ORR vs. difference in median OS | (b) R2 = 0.34; p < 0.0001 | ||||||||||||||
CR vs. PFS | |||||||||||||||
Lee 2011111 | CR | PFS | NHL (aggressive) | First | Chemotherapy | 12b | NR | Spearman (difference in CR vs. difference in 3-year PFS) | rs = 0.63 (95% CI 0.21 to 0.84); p = 0.005 | NR | Medium | NE | |||
Lee 2011111 | CR | PFS | NHL (indolent) | First | Chemotherapy | 6b | NR | Spearman (difference in CR vs. difference in 3-year PFS) | rs = 0.41 (95% CI –0.52 to 0.88); p = 0.35 | NR | Medium | NE | |||
Shi 2017132 | CR | PFS | NHL (indolent; follicular) | First | Chemotherapy or immunotherapy (induction or maintenance) | 13 | 3837 | (a) WLSR R2 | (a) R2 WLS = 0.88 (95% CI 0.77 to 0.96); p = NR | log-HR PFS = –0.093 – 0.636 × log-OR CR 30 months | 1.56 | Medium + | NE | ||
(b) Bivariate Plackett copula model (log-OR CR 30 months vs. log-HR PFS) | (b) R2 Copula = 0.86 (95% CO 0.72 to 1.00); p = NR | ||||||||||||||
Shi 2017132 | CR | PFS | NHL (indolent; follicular) | First | Rituximab-based (induction or maintenance) | 9 | 2851 | (a) WLSR R2 | (a) R2 WLS = 0.85 (95% CI 0.62 to 0.97); p = NR | NR | Medium + | NE | |||
(b) Bivariate Plackett copula model (log-OR CR 30 months vs. log-HR PFS) | (b) R2 Copula = 0.80 (95% CI 0.56 to 1.00); p = NR | ||||||||||||||
Shi 2017132 | CR | PFS | NHL (indolent; follicular) | First | Non-rituximab-based (induction or maintenance) | 4 | 986 | (a) WLSR R2 | (a) R2 WLS = 0.91 (95% CI 0.05 to 1.00); p = NR | NR | Medium + | NE | |||
(b) Bivariate Plackett copula model (log-OR CR 30 months vs. log-HR PFS) | (b) R2 Copula = 0.96 (95% CI 0.90 to 1.00); p = NR | ||||||||||||||
Shi 2017132 | CR | PFS | NHL (indolent; follicular) | First | Induction | 8 | 2207 | (a) WLSR R2 | (a) R2 WLS = 0.89 (95% CI 0.75 to 0.98); p = NR | NR | Medium + | NE | |||
(b) Bivariate Plackett copula model (log-OR CR 30 months vs. log-HR PFS) | (b) R2 Copula = 0.89 (95% CI 0.74 to 1.00); p = NR | ||||||||||||||
Shi 2017132 | CR | PFS | NHL (indolent; follicular) | First | Maintenance | 5 | 1630 | (a) WLS (reported as R2 WLS) | (a) R2 WLS = 0.93 (95% CI 0.84 to 1.00); p = NR | NR | Medium + | NE | |||
(b) Bivariate Plackett copula model (reported as R2 copula), CR 30 months vs. PFS | (b) R2 Copula = 0.89 (95% CI 0.71 to 1.00); p = NR | ||||||||||||||
Shi 2017132 | CR | PFS | NHL (indolent; follicular) | First: high FLIPI score | Chemotherapy or immunotherapy (induction or maintenance) | 9 | 1415 | (a) WLSR R2 | (a) R2 WLS = 0.87 (95% CI 0.68 to 0.98); p = NR | NR | Medium + | NE | |||
(b) Bivariate Plackett copula model (log-OR CR 30 months vs. log-HR PFS) | (b) R2 Copula = 0.73 (95% CI 0.42 to 1.00); p = NR | ||||||||||||||
Shi 2017132 | CR | PFS | NHL (indolent; follicular) | First: low to intermediate FLIPI score | Chemotherapy or immunotherapy (induction or maintenance) | 10 | 1882 | (a) WLSR R2 | (a) R2 WLS = 0.45 (95% CI 0.02 to 0.93); p = NR | NR | Low | NE | |||
(b) Bivariate Plackett copula model (log-OR CR 30 months vs. log-HR PFS) | (b) R2 Copula = 0.57 (0.17 to 0.97), p = NR | ||||||||||||||
Shi 2017132 | CR | PFS | NHL (indolent; follicular) | First | Chemotherapy or immunotherapy (induction or maintenance) | 11 | 2728 | (a) WLSR R2 | (a) R2 WLS = 0.84 (95% CI 0.63 to 0.95); p = NR | log-HR PFS = 0.043 – 0.726 × log-OR CR 24 months | NR | Medium + | NE | ||
(b) Bivariate Plackett copula model (log-OR CR 24 months vs. log-HR PFS) | (b) R2 Copula = 0.67 (95% CI 0.35 to 0.99); p = NR | ||||||||||||||
Shi 2017132 | CR | PFS | NHL (indolent; follicular) | First: stage IV | Chemotherapy or immunotherapy (induction or maintenance) | NR | 2585 | (a) WLSR R2 | (a) R2 WLS = 0.92 (95% CI 0.85 to 0.97); p = NR | NR | Medium + | NE | |||
(b) Bivariate Plackett copula model (log-OR CR 30 months vs. log-HR PFS) | (b) R2 Copula = 0.94 (95% CI 0.87 to 1.00); p = NR | ||||||||||||||
Colloca 201790 | CR | PFS | Ovarian | First | Chemotherapy | 12 | NR | Spearman (difference in RR vs. difference in median PFS) | rs = 0.19; p = 0.555 | NR | Low | NE | |||
CR vs. OS | |||||||||||||||
Hackshaw 200599 | CR | OS | Breast | First | Chemotherapy | 41a | 9163b | WLR R2 (log-OR CR vs. log-HR OS) | R2 = 0.12; p = 0.02 | log-HR OS = –0.0097 + 0.13 × log-OR CRSlope: 0.13 | NR | Low | NE | ||
Hackshaw 200599 | CR | OS | Breast | First: recruited pre-1990 | Chemotherapy | 26a | 5244b | WLR R2 (log-OR CR vs. log-HR OS) | R2 = 0.05; p = 0.24 | Slope: 0.09 | NR | Low | NE | ||
Hackshaw 200599 | CR | OS | Breast | First: recruited 1990 or after | Chemotherapy | 15a | 3919b | WLR R2 (log-OR CR vs. log-HR OS) | R2 = 0.36; p = 0.01 | Slope: 0.16 | NR | Low | NE | ||
Foster 201197 | CR | OS | Lung (SCLC) | First | Chemotherapy | Three (32 centres) | 596b | Spearman (log-OR CR vs. log-HR OS) | rs = 0.50; p = NR | WLSR R2 (log-OR CR vs. log-HR OS) | R2 = 0.48; p = NR | NR | Low | NE | |
Lee 2011111 | CR | OS | NHL (aggressive) | First | Chemotherapy | 36b | 16,103b | (a) Spearman: difference in CR vs. difference in 3-year OS | (a) rs = 0.58 (95% CI 0.29 to 0.77); p = 0.004 | NR | Medium | NE | |||
(b) Spearman: difference in CR vs. difference in 5-year OS | (b) rs = 0.50 (95% CI 0.23 to 0.74); p = 0.01 | ||||||||||||||
Lee 2011111 | CR | OS | NHL (indolent) | First | Chemotherapy | 15b | 5128b | (a) Spearman: difference in CR vs. difference in 3-year OS | (a) rs = 0.41 (95% CI –0.10 to 0.74); p = 0.098 | NR | Medium | NE | |||
(b) Spearman: difference in CR vs. difference in 5-year OS | (b) rs = 0.21 (95% CI –0.34 to 0.50); p = 0.44 | ||||||||||||||
Colloca 201790 | CR | OS | Ovarian | First | Chemotherapy | 12 | NR | Spearman (difference in pCR vs. difference in median OS) | rs = 0.42; p = 0.180 | NR | Low | NE | |||
DoR vs. OS | |||||||||||||||
Colloca 201692 | DoR | OS | Colorectal | First | Bevacizumab and chemotherapy | 5 | NR | Spearman (difference in median DoR vs. difference in median OS) | rs = 0.70; p = 0.188 | NR | Medium | NE | |||
Colloca 201691 | DoR | OS | Pancreatic | First | Gemcitabine and chemotherapy or targeted therapy | 7b | NR | Spearman (difference in median DoR vs. difference in median OS) | rs = 0.76; p = 0.049 | NR | Medium | NE | |||
Colloca 201691 | DoR | OS | Pancreatic | First | Gemcitabine and chemotherapy | 3b | NR | Spearman (difference in median DoR vs. difference in median OS) | rs = 0.50; p = 0.667 | NR | Low | NE | |||
Colloca 201691 | DoR | OS | Pancreatic | First | Gemcitabine and targeted | 4b | NR | Spearman (difference in median DoR vs. difference in median OS) | rs = 0.40; p = 0.600 | NR | Low | NE |
Appendix 7 Studies excluded at full-text screening (n = 135)
Not a clinical study (n = 4)
-
Ascierto PA, Long GV. Progression-free survival landmark analysis: a critical endpoint in melanoma clinical trials. Lancet Oncol 2016;17:1037–9.
-
Buyse M, Molenberghs G, Paoletti X, Oba K, Alonso A, Van der Elst W, Burzykowski T. Statistical evaluation of surrogate endpoints with examples from cancer clinical trials. Biom J 2016;58:104–32. https://doi.org/10.1002/bimj.201400049
-
Estey E, Othus M, Lee SJ, Appelbaum FR, Gale RP. New drug approvals in acute myeloid leukemia: what’s the best end point? Leukemia 2016;30:521–5.
-
Xia Y, Cui L, Yang B. A note on breast cancer trials with pCR-based accelerated approval. J Biopharm Stat 2014;24:1102–14. https://doi.org/10.1080/10543406.2014.931410
Not a meta-analysis of multiple studies (n = 28)
-
Choi SI, Yu A, Kim BH, Ko EJ, Park SS, Nam BH, Park JW. A model predicting survival of patients with recurrent or progressive hepatocellular carcinoma: the MORE score. J Gastroenterol Hepatol 2017;32:651–8. https://doi.org/10.1111/jgh.13532
-
Fiteni F, Bonnetain F. Surrogate end points for overall survival in breast cancer trials: a review. Breast 2016;29:44–8. https://doi.org/10.1016/j.breast.2016.06.005
-
Fiteni F, Westeel V, Bonnetain F. Surrogate endpoints for overall survival in lung cancer trials: a review. Expert Rev Anticancer Ther 2017;17:447–54. https://doi.org/10.1080/14737140.2017.1316196
-
Goorts B, van Nijnatten TJ, de Munck L, Moossdorff M, Heuts EM, de Boer M, et al. Clinical tumor stage is the most important predictor of pathological complete response rate after neoadjuvant chemotherapy in breast cancer patients. Breast Cancer Res Treat 2017;163:83–91. https://doi.org/10.1007/s10549-017-4155-2
-
Liu L, Zhao Y, Jia J, Chen H, Bai W, Yang M, et al. The prognostic value of alpha-fetoprotein response for advanced-stage hepatocellular carcinoma treated with sorafenib combined with transarterial chemoembolization. Sci Rep 2016;6:19851. https://doi.org/10.1038/srep19851
-
Long J, Zheng JS, Sun B, Lu N. Microwave ablation of hepatocellular carcinoma with portal vein tumor thrombosis after transarterial chemoembolization: a prospective study. Hepatol Int 2016;10:175–84. https://doi.org/10.1007/s12072-015-9673-6
-
Mähringer-Kunz A, Weinmann A, Schmidtmann I, Koch S, Schotten S, Pinto Dos Santos D, et al. Validation of the SNACOR clinical scoring system after transarterial chemoembolisation in patients with hepatocellular carcinoma. BMC Cancer 2018;18:489. https://doi.org/10.1186/s12885-018-4407-5
-
Matsubara Y, Sakabayashi S, Nishimura T, Ishida T, Ohuchi N, Teramukai S, Fukushima M. Surrogacy of tumor response and progression-free survival for overall survival in metastatic breast cancer resistant to both anthracyclines and taxanes. Int J Clin Oncol 2011;16:623–9. https://doi.org/10.1007/s10147-011-0231-5
-
Mauguen A, Michiels S, Rondeau V. Joint model imputation to estimate the treatment effect on long-term survival using auxiliary events. J Biopharm Stat 2017;27:1043–53. https://doi.org/10.1080/10543406.2017.1295249
-
Meyer M, Hohenberger P, Apfaltrer P, Henzler T, Dinter DJ, Schoenberg SO, Fink C. CT-based response assessment of advanced gastrointestinal stromal tumor: dual energy CT provides a more predictive imaging biomarker of clinical benefit than RECIST or Choi criteria. Eur J Radiol 2013;82:923–8. https://doi.org/10.1016/j.ejrad.2013.01.006
-
Michl M, Stintzing S, von Weikersthal LF, Decker T, Kiani A, Vehling-Kaiser U, et al. CEA response is associated with tumour response and survival in patients with KRAS exon 2 wild-type and extended RAS wild-type metastatic colorectal cancer receiving first-line FOLFIRI plus cetuximab or bevacizumab (FIRE-3 trial) (aEuro). Ann Oncol 2016;27:1565–72.
-
Mosconi C, Gramenzi A, Ascanio S, Cappelli A, Renzulli M, Pettinato C, et al. Yttrium-90 radioembolization for unresectable/recurrent intrahepatic cholangiocarcinoma: a survival, efficacy and safety study. Br J Cancer 2016;115:297–302. https://doi.org/10.1038/bjc.2016.191
-
Motzer RJ, Bukowski RM, Figlin RA, Hutson TE, Michaelson MD, Kim ST, et al. Prognostic nomogram for sunitinib in patients with metastatic renal cell carcinoma. Cancer 2008;113:1552–8. https://doi.org/10.1002/cncr.23776
-
Nault JC, Nkontchou G, Nahon P, Grando V, Bourcier V, Barge S, et al. Percutaneous treatment of localized infiltrative hepatocellular carcinoma developing on cirrhosis. Ann Surg Oncol 2016;23:1906–15. https://doi.org/10.1245/s10434-015-5064-4
-
Négrier S, Bushmakin AG, Cappelleri JC, Korytowsky B, Sandin R, Charbonneau C, et al. Assessment of progression-free survival as a surrogate end-point for overall survival in patients with metastatic renal cell carcinoma. Eur J Cancer 2014;50:1766–71.
-
Ogasawara S, Chiba T, Ooka Y, Suzuki E, Inoue M, Wakamatsu T, et al. Analysis of sorafenib outcome: focusing on the clinical course in patients with hepatocellular carcinoma. PLOS ONE 2016;11:e0161303. https://doi.org/10.1371/journal.pone.0161303
-
Park S, Kim HJ, Choi CM, Lee DH, Kim SW, Lee JS, et al. Predictive factors for a long-term response duration in non-squamous cell lung cancer patients treated with pemetrexed. BMC Cancer 2016;16:417. https://doi.org/10.1186/s12885-016-2457-0
-
Plano Sánchez AI, Velasco Roces L, Zapico García I, López EL, Hernandez M, Parejo M, Peña-Díaz J. Value of α-fetoprotein as an early biomarker for treatment response to sorafenib therapy in advanced hepatocellular carcinoma. Oncology Letters 2018;15:8863–70.
-
Prados M, Cloughesy T, Samant M, Fang L, Wen PY, Mikkelsen T, et al. Response as a predictor of survival in patients with recurrent glioblastoma treated with bevacizumab. Neuro Oncol 2011;13:143–51. https://doi.org/10.1093/neuonc/noq151
-
Qian Q, Zhan P, Yu L, Shi Y, Cheng J, Wei S, et al. Baseline levels and decrease in serum soluble intercellular adhesion molecule-1 during chemotherapy predict objective response and survival in patients who have advanced non-small-cell lung cancer. Clin Lung Cancer 2011;12:131–7. https://doi.org/10.1016/j.cllc.2011.03.009
-
Ramanathan RK, Goldstein D, Korn RL, Arena F, Moore M, Siena S, et al. Positron emission tomography response evaluation from a randomized phase III trial of weekly nab-paclitaxel plus gemcitabine versus gemcitabine alone for patients with metastatic adenocarcinoma of the pancreas. Ann Oncol 2016;27:648–53. https://doi.org/10.1093/annonc/mdw020
-
Riaz A, Gabr A, Abouchaleh N, Ali R, Al Asadi A, Mora R, et al. Radioembolization for hepatocellular carcinoma: statistical confirmation of improved survival in responders by landmark analyses. Hepatology 2018;67:873–83. https://doi.org/10.1002/hep.29480
-
Riedl CC, Pinker K, Ulaner GA, Ong LT, Baltzer P, Jochelson MS, et al. Comparison of FDG-PET/CT and contrast-enhanced CT for monitoring therapy response in patients with metastatic breast cancer. Eur J Nucl Med Mol Imaging 2017;44:1428–37. https://doi.org/10.1007/s00259-017-3703-7
-
Salvador-Coloma C, Lorente D, Palanca S, Simarro J, Mancheño N, Sandoval J, et al. Early radiological response as predictor of overall survival in non-small cell lung cancer (NSCLC) patients with epidermal growth factor receptor mutations. J Thorac Dis 2018;10:1386–93. https://doi.org/10.21037/jtd.2018.02.30
-
Schwarz JK, Siegel BA, Dehdashti F, Grigsby PW. Association of posttherapy positron emission tomography with tumor response and survival in cervical carcinoma. JAMA 2007;298:2289–95.
-
Ter-Minassian M, Zhang S, Brooks NV, Brais LK, Chan JA, Christiani DC, et al. Association between tumour progression endpoints and overall survival in patients with advanced neuroendocrine tumours. Oncologist 2017;22:165–72.
-
Yau T, Yao TJ, Chan P, Wong H, Pang R, Fan ST, Poon RT. The significance of early alpha-fetoprotein level changes in predicting clinical and survival benefits in advanced hepatocellular carcinoma patients receiving sorafenib. Oncologist 2011;16:1270–9. https://doi.org/10.1634/theoncologist.2011-0105
-
Zabor EC, Heller G, Schwartz LH, Chapman PB. Correlating surrogate endpoints with overall survival at the individual patient level in BRAFV600E-mutated metastatic melanoma patients treated with vemurafenib. Clin Cancer Res 2016;22:1341–7. https://doi.org/10.1158/1078-0432.CCR-15-1441
Neoadjuvant or adjuvant treatment (n = 10)
-
Berruti A, Amoroso V, Gallo F, Bertaglia V, Simoncini E, Pedersini R, et al. Pathologic complete response as a potential surrogate for the clinical outcome in patients with breast cancer after neoadjuvant therapy: a meta-regression of 29 randomized prospective studies. J Clin Oncol 2014;32:3883–91. https://doi.org/10.1200/JCO.2014.55.2836
-
Bonnetain F, Bosset JF, Gerard JP, Calais G, Conroy T, Mineur L, et al. What is the clinical benefit of preoperative chemoradiotherapy with 5FU/leucovorin for T3-4 rectal cancer in a pooled analysis of EORTC 22921 and FFCD 9203 trials: surrogacy in question? Eur J Cancer 2012;48:1781–90.
-
Broglio KR, Quintana M, Foster M, Olinger M, McGlothlin A, Berry SM, et al. Association of pathologic complete response to neoadjuvant therapy in HER2-positive breast cancer with long-term outcomes: a meta-analysis. JAMA Oncol 2016;2:751–60. https://doi.org/10.1001/jamaoncol.2015.6113
-
Cortazar P, Zhang L, Untch M, Mehta K, Costantino JP, Wolmark N, et al. Pathological complete response and long-term clinical benefit in breast cancer: the CTNeoBC pooled analysis. Lancet 2014;384:164–72. https://doi.org/10.1016/S0140-6736(13)62422-8
-
Korn EL, Sachs MC, McShane LM. Statistical controversies in clinical research: assessing pathologic complete response as a trial-level surrogate end point for early-stage breast cancer. Ann Oncol 2016;27:10–15. https://doi.org/10.1093/annonc/mdv507
-
Nekljudova V, Loibl S, von Minckwitz G, Schneeweiss A, Glück S, Crane R, et al. Trial-level prediction of long-term outcome based on pathologic complete response (pCR) after neoadjuvant chemotherapy for early-stage breast cancer (EBC). Contemp Clin Trials 2018;71:194–8.
-
Petrelli F, Borgonovo K, Cabiddu M, Ghilardi M, Lonati V, Barni S. Pathologic complete response and disease-free survival are not surrogate endpoints for 5-year survival in rectal cancer: an analysis of 22 randomized trials. J Gastrointest Oncol 2017;8:39–48.
-
Petrelli F, Coinu A, Cabiddu M, Ghilardi M, Vavassori I, Barni S. Correlation of pathologic complete response with survival after neoadjuvant chemotherapy in bladder cancer treated with cystectomy: a meta-analysis. Eur Urol 2014;65:350–7. https://doi.org/10.1016/j.eururo.2013.06.049
-
Petrelli F, Tomasello G, Barni S. Surrogate end-points for overall survival in 22 neoadjuvant trials of gastro-oesophageal cancers. Eur J Cancer 2017;76:8–16.
-
Petrelli F, Tomasello G, Ghidini M, Lonati V, Passalacqua R, Barni S. Disease-free survival is not a surrogate endpoint for overall survival in adjuvant trials of pancreatic cancer: a systematic review of randomized trials. HPB (Oxford) 2017;19:944–50.
No relevant outcomes (n = 67)
Aggarwal C, Borghaei H. Treatment paradigms for advanced non-small cell lung cancer at academic medical centers: involvement in clinical trial endpoint design. Oncologist 2017;22:700–8. https://doi.org/10.1634/theoncologist.2016-0345
Araujo RLC, Herman P, Riechelmann RP. Recurrence-free survival as a putative surrogate for overall survival in phase III trials of curative-intent treatment of colorectal liver metastases: systematic review. World J Clin Oncol 2017;8:266–72. https://doi.org/10.5306/wjco.v8.i3.266
Buyse M, Burzykowski T, Carroll K, Michiels S, Sargent DJ, Miller LL, et al. Progression-free survival is a surrogate for survival in advanced colorectal cancer. J Clin Oncol 2007;25:5218–24.
Cai J, Ma H, Huang F, Zhu D, Bi J, Ke Y, Zhang T. Correlation of bevacizumab-induced hypertension and outcomes of metastatic colorectal cancer patients treated with bevacizumab: a systematic review and meta-analysis. World J Surg Oncol 2013;11:306. https://doi.org/10.1186/1477-7819-11-306
Chirila C, Odom D, Devercelli G, Khan S, Sherif BN, Kaye JA, et al. Meta-analysis of the association between progression-free survival and overall survival in metastatic colorectal cancer. Int J Colorectal Dis 2012;27:623–34. https://doi.org/10.1007/s00384-011-1349-7
Colloca GA, Venturino A, Guarneri D. Early tumour shrinkage after first-line medical treatment of metastatic colorectal cancer: a meta-analysis. Int J Clin Oncol 2019;24:231–40.
Forsythe A, Chandiwana D, Barth J, Thabane M, Baeck J, Shor A, Tremblay G. Is progression-free survival a more relevant endpoint than overall survival in first-line HR+/ HER2- metastatic breast cancer? Cancer Manag Res 2018;10:1015–25.
Forsythe A, Chandiwana D, Barth J, Thabane M, Baeck J, Tremblay G. Progression-free survival/time to progression as a potential surrogate for overall survival in HR+, HER2- metastatic breast cancer. Breast Cancer 2018;10:69–78. https://doi.org/10.2147/BCTT.S162841
Foster NR, Renfro LA, Schild SE, Redman MW, Wang XF, Dahlberg SE, et al. Multitrial evaluation of progression-free survival as a surrogate end point for overall survival in first-line extensive-stage small-cell lung cancer. J Thorac Oncol 2015;10:1099–106. https://doi.org/10.1097/JTO.0000000000000548
Francini E, Petrioli R, Rossi G, Laera L, Roviello G. PSA response rate as a surrogate marker for median overall survival in docetaxel-based first-line treatments for patients with metastatic castration-resistant prostate cancer: an analysis of 22 trials. Tumour Biol 2014;35:10601–7. https://doi.org/10.1007/s13277-014-2559-8
Glynne-Jones R, Mawdsley S, Pearce T, Buyse M. Alternative clinical end points in rectal cancer--are we getting closer? Ann Oncol 2006;17:1239–48.
Grünwald V, Lin X, Kalanovic D, Simantov R. Early tumour shrinkage: a tool for the detection of early clinical activity in metastatic renal cell carcinoma. Eur Urol 2016;70:1006–15.
Grünwald V, McKay RR, Krajewski KM, Kalanovic D, Lin X, Perkins JJ, et al. Depth of remission is a prognostic factor for survival in patients with metastatic renal cell carcinoma. Eur Urol 2015;67:952–8. https://doi.org/10.1016/j.eururo.2014.12.036
Gyawali B, Hey SP, Kesselheim AS. A comparison of response patterns for progression-free survival and overall survival following treatment for cancer with PD-1 inhibitors: a meta-analysis of correlation and differences in effect sizes. JAMA Netw Open 2018;1:e180416. https://doi.org/10.1001/jamanetworkopen.2018.0416
Harshman LC, Xie W, Moreira RB, Bossé D, Ruiz Ares GJ, Sweeney CJ, Choueiri TK. Evaluation of disease-free survival as an intermediate metric of overall survival in patients with localized renal cell carcinoma: a trial-level meta-analysis. Cancer 2018;124:925–33. https://doi.org/10.1002/cncr.31154
Hasan B, Greillier L, Pallis A, Menis J, Gaafar R, Sylvester R, et al. Progression free survival rate at 9 and 18 weeks predict overall survival in patients with malignant pleural mesothelioma: an individual patient pooled analysis of 10 European Organisation for Research and Treatment of Cancer Lung Cancer Group studies and an independent study validation. Eur J Cancer 2014;50:2771–82. https://doi.org/10.1016/j.ejca.2014.07.020
Hotta K, Kiura K, Fujiwara Y, Takigawa N, Hisamoto A, Ichihara E, et al. Role of survival post-progression in phase III trials of systemic chemotherapy in advanced non-small-cell lung cancer: a systematic review. PLOS ONE 2011;6:e26646. https://doi.org/10.1371/journal.pone.0026646
Huan HB, Wu LL, Lau WY, Wen XD, Zhang L, Yang DP, et al. Surrogate endpoint for overall survival in assessment of adjuvant therapies after curative treatment for hepatocellular carcinoma: a re-analysis of meta-analyses of individual patients’ data. Oncotarget 2017;8:90291–90300. https://doi.org/10.18632/oncotarget.18853
Jiang ZC, Ding P, Geng Z. Principal causal effect identification and surrogate end point evaluation by multiple trials. J R Stat Soc Ser B Stat Methodol 2016;78:829–48.
Kasuga A, Hamamoto Y, Takeuchi A, Kawasaki K, Suzuki T, Hirata K, et al. Positive relationship between subsequent chemotherapy and overall survival in pancreatic cancer: meta-analysis of postprogression survival for first-line chemotherapy. Cancer Chemother Pharmacol 2017;79:595–602. https://doi.org/10.1007/s00280-017-3263-3
Kasuga A, Hamamoto Y, Takeuchi A, Okano N, Togasaki K, Aoki Y, et al. Post-progression survival following second-line chemotherapy in patients with advanced pancreatic cancer previously treated with gemcitabine: a meta-analysis. Invest New Drugs 2018;36:939–48. https://doi.org/10.1007/s10637-018-0589-6
Kataoka K, Nakamura K, Mizusawa J, Kato K, Eba J, Katayama H, et al. Surrogacy of progression-free survival (PFS) for overall survival (OS) in esophageal cancer trials with preoperative therapy: literature-based meta-analysis. Eur J Surg Oncol 2017;43:1956–61.
Kudo M, Izumi N, Sakamoto M, Matsuyama Y, Ichida T, Nakashima O, et al. Survival analysis over 28 years of 173,378 patients with hepatocellular carcinoma in Japan. Liver Cancer 2016;5:190–7. https://doi.org/10.1159/000367775
Kundu MG, Acharyya S. Surrogacy of progression free survival for overall survival in metastatic breast cancer studies: meta-analyses of published studies. Contemp Clin Trials 2017;53:20–8.
Kurokawa Y, Shibata T, Sasako M, Sano T, Tsuburaya A, Iwasaki Y, Fukuda H. Validity of response assessment criteria in neoadjuvant chemotherapy for gastric cancer (JCOG0507-A). Gastric Cancer 2014;17:514–21. https://doi.org/10.1007/s10120-013-0294-2
Lee DW, Jang MJ, Lee KH, Cho EJ, Lee JH, Yu SJ, et al. TTP as a surrogate endpoint in advanced hepatocellular carcinoma treated with molecular targeted therapy: meta-analysis of randomised controlled trials. Br J Cancer 2016;115:1201–5. https://doi.org/10.1038/bjc.2016.322
Li C, Xiang A, Chen X, Yin K, Lu J, Yin W. Optimizing the treatment of bevacizumab as first-line therapy for human epidermal growth factor receptor 2 (HER2)-negative advanced breast cancer: an updated meta-analysis of published randomized trials. Onco Targets Ther 2017;10:3155–68. https://doi.org/10.2147/OTT.S138600
Li D, Lv H, Hao X, Dong Y, Dai H, Song Y. Prognostic value of bone scan index as an imaging biomarker in metastatic prostate cancer: a meta-analysis. Oncotarget 2017;8:84449–58. https://doi.org/10.18632/oncotarget.19680
Li M, Dave N, Salem AH, Freise KJ. Model-based meta-analysis of progression-free survival in non-Hodgkin lymphoma patients. Medicine 2017;96:e7988. https://doi.org/10.1097/MD.0000000000007988
Lien K, Georgsdottir S, Sivanathan L, Chan K, Emmenegger U. Low-dose metronomic chemotherapy: a systematic literature analysis. Eur J Cancer 2013;49:3387–95. https://doi.org/10.1016/j.ejca.2013.06.038
Liu Y, Waterton JC. Imaging Biomarkers in Clinical Trials. In Martí-Bonmatí L, Alberich-Bayarri, editors. Imaging Biomarkers: Development and Clinical Imagination. Cham: Springer; 2017. pp. 295–306.
Loupakis F, Bria E, Vaccaro V, Cuppone F, Milella M, Carlini P, et al. Magnitude of benefit of the addition of bevacizumab to first-line chemotherapy for metastatic colorectal cancer: meta-analysis of randomized clinical trials. J Exp Clin Cancer Res 2010;29:58. https://doi.org/10.1186/1756-9966-29-58
Martens A, Wistuba-Hamprecht K, Geukes Foppen M, Yuan J, Postow MA, Wong P, et al. Baseline peripheral blood biomarkers associated with clinical outcome of advanced melanoma patients treated with ipilimumab. Clin Cancer Res 2016;22:2908–18. https://doi.org/10.1158/1078-0432.CCR-15-2412
Mauguen A, Pignon JP, Burdett S, Domerg C, Fisher D, Paulus R, et al. Surrogate endpoints for overall survival in chemotherapy and radiotherapy trials in operable and locally advanced lung cancer: a re-analysis of meta-analyses of individual patients’ data. Lancet Oncol 2013;14:619–26. https://doi.org/10.1016/S1470-2045(13)70158-X
Meeks JJ, Bellmunt J, Bochner BH, Clarke NW, Daneshmand S, Galsky MD, et al. A systematic review of neoadjuvant and adjuvant chemotherapy for muscle-invasive bladder cancer. Eur Urol 2012;62:523–33. https://doi.org/10.1016/j.eururo.2012.05.048
Mehra N, Dolling D, Sumanasuriya S, Christova R, Pope L, Carreira S, et al. Plasma cell-free DNA concentration and outcomes from taxane therapy in metastatic castration-resistant prostate cancer from two phase III trials (FIRSTANA and PROSELICA). Eur Urol 2018;74:283–91.
Michiels S, Pugliano L, Marguet S, Grun D, Barinoff J, Cameron D, et al. Progression-free survival as surrogate end point for overall survival in clinical trials of HER2-targeted agents in HER2-positive metastatic breast cancer. Ann Oncol 2016;27:1029–34.
Miura Y, Imai H, Sakurai R, Kaira K, Sunaga N, Minato K, et al. The effect of post-progression survival on overall survival among patients with sensitive relapse of small cell lung cancer. Med Oncol 2018;35:45. https://doi.org/10.1007/s12032-018-1107-6
Miyake H, Harada K, Ozono S, Fujisawa M. Prognostic significance of early tumor shrinkage under second-line targeted therapy for metastatic renal cell carcinoma: retrospective multi-institutional study in Japan. Mol Diagn Ther 2016;20:385–92. https://doi.org/10.1007/s40291-016-0206-3
Miyake H, Miyazaki A, Imai S, Harada K, Fujisawa M. Early tumor shrinkage under treatment with first-line tyrosine kinase inhibitors as a predictor of overall survival in patients with metastatic renal cell carcinoma: a retrospective multi-institutional study in Japan. Target Oncol 2016;11:175–82. https://doi.org/10.1007/s11523-015-0385-6
Mocellin S, Baretta Z, Roqué I Figuls M, Solà I, Martin-Richard M, Hallum S, Bonfill Cosp X. Second-line systemic therapy for metastatic colorectal cancer. Cochrane Database Syst Rev 2017;1:CD006875. https://doi.org/10.1002/14651858.CD006875.pub3
Montagnani F, DI Leonardo G, Pino MS, Martella F, Perboni S, Ribecco A, Fioretto L. Progression-free survival as a surrogate end-point in advanced colorectal cancer treated with antiangiogenic therapies. Anticancer Res 2016;36:4259–65.
Morris PD, Laurence JM, Yeo D, Crawford M, Strasser SI, McCaughan GW, Sandroussi C. Can response to locoregional therapy help predict longterm survival after liver transplantation for hepatocellular carcinoma? A systematic review. Liver Transpl 2017;23:375–85. https://doi.org/10.1002/lt.24689
Munshi NC, Avet-Loiseau H, Rawstron AC, Owen RG, Child JA, Thakurta A, et al. Association of minimal residual disease with superior survival outcomes in patients with multiple myeloma: a meta-analysis. JAMA Oncol 2017;3:28–35. https://doi.org/10.1001/jamaoncol.2016.3160
Othus M, van Putten W, Lowenberg B, Petersdorf SH, Nand S, Erba H, et al. Relationship between event-free survival and overall survival in acute myeloid leukemia: a report from SWOG, HOVON/SAKK, and MRC/NCRI. Haematologica 2016;101:e284–6. https://doi.org/10.3324/haematol.2015.138552
Petrelli F, Coinu A, Cabiddu M, Borgonovo K, Ghilardi M, Lonati V, Barni S. Early analysis of surrogate endpoints for metastatic melanoma in immune checkpoint inhibitor trials. Medicine 2016;95:e3997. https://doi.org/10.1097/MD.0000000000003997
Peveling-Oberhag J, Arcaini L, Bankov K, Zeuzem S, Herrmann E. The anti-lymphoma activity of antiviral therapy in HCV-associated B-cell non-Hodgkin lymphomas: a meta-analysis. J Viral Hepat 2016;23:536–44. https://doi.org/10.1111/jvh.12518
Polley MY, Lamborn KR, Chang SM, Butowski N, Clarke JL, Prados M. Six-month progression-free survival as an alternative primary efficacy endpoint to overall survival in newly diagnosed glioblastoma patients receiving temozolomide. Neuro Oncol 2010;12:274–82. https://doi.org/10.1093/neuonc/nop034
Pujol JL, Pirker R, Lynch TJ, Butts CA, Rosell R, Shepherd FA, et al. Meta-analysis of individual patient data from randomized trials of chemotherapy plus cetuximab as first-line treatment for advanced non-small cell lung cancer. Lung Cancer 2014;83:211–18. https://doi.org/10.1016/j.lungcan.2013.11.006
Rotolo F, Pignon JP, Bourhis J, Marguet S, Leclercq J, Tong Ng W, et al. Surrogate end points for overall survival in loco-regionally advanced nasopharyngeal carcinoma: an individual patient data meta-analysis. J Natl Cancer Inst 2017;109:djw239.
Shafrin J, Brookmeyer R, Peneva D, Park J, Zhang J, Figlin RA, Lakdawalla DN. The value of surrogate endpoints for predicting real-world survival across five cancer types. Curr Med Res Opin 2016;32:731–9. https://doi.org/10.1185/03007995.2016.1140027
Shi Q, Schmitz N, Ou FS, Dixon JG, Cunningham D, Pfreundschuh M, et al. Progression-free survival as a surrogate end point for overall survival in first-line diffuse large B-cell lymphoma: an individual patient-level analysis of multiple randomized trials (SEAL). J Clin Oncol 2018;36:2593–602. https://doi.org/10.1200/JCO.2018.77.9124
Shih T, Lindley C. Bevacizumab: an angiogenesis inhibitor for the treatment of solid malignancies. Clin Ther 2006;28:1779–802.
Shimokawa M, Kogawa T, Shimada T, Saito T, Kumagai H, Ohki M, Kaku T. Overall survival and post-progression survival are potent endpoint in phase III trials of second/third-line chemotherapy for advanced or recurrent epithelial ovarian cancer. J Cancer 2018;9:872–9. https://doi.org/10.7150/jca.17664
Sjoquist KM, Lord SJ, Friedlander ML, John Simes R, Marschner IC, Lee CK. Progression-free survival as a surrogate endpoint for overall survival in modern ovarian cancer trials: a meta-analysis. Ther Adv Med Oncol 2018;10:1758835918788500. https://doi.org/10.1177/1758835918788500
Song SY, Seo H, Kim G, Kim AR, Kim EY. Trends in endpoint selection in clinical trials of advanced breast cancer. J Cancer Res Clin Oncol 2016;142:2403–13. https://doi.org/10.1007/s00432-016-2221-5
Sonpavde G, Pond GR, Rosenberg JE, Bajorin DF, Regazzi AM, Choueiri TK, et al. Complete response as an intermediate end point in patients receiving salvage systemic therapy for urothelial carcinoma. Clin Genitourin Cancer 2015;13:185–92. https://doi.org/10.1016/j.clgc.2014.09.004
Suciu S, Eggermont AMM, Lorigan P, Kirkwood JM, Markovic SN, Garbe C, et al. Relapse-free survival as a surrogate for overall survival in the evaluation of stage II-III melanoma adjuvant therapy. J Natl Cancer Inst 2018;110:87–96. https://doi.org/10.1093/jnci/djx133
Tan A, Porcher R, Crequit P, Ravaud P, Dechartres A. Differences in treatment effect size between overall survival and progression-free survival in immunotherapy trials: a meta-epidemiologic study of trials with results posted at ClinicalTrials.gov. J Clin Oncol 2017;35:1686–94. https://doi.org/10.1200/JCO.2016.71.2109
Terashima T, Yamashita T, Takata N, Nakagawa H, Toyama T, Arai K, et al. Post-progression survival and progression-free survival in patients with advanced hepatocellular carcinoma treated by sorafenib. Hepatol Res 2016;46:650–6. https://doi.org/10.1111/hepr.12601
Terashima T, Yamashita T, Toyama T, Arai K, Kawaguchi K, Kitamura K, et al. Surrogacy of time to progression for overall survival in advanced hepatocellular carcinoma treated with systemic therapy: a systematic review and meta-analysis of randomized controlled trials. Liver Cancer 2019;8:130–9. https://doi.org/10.1159/000489505
Trotman J, Luminari S, Boussetta S, Versari A, Dupuis J, Tychyj C, et al. Prognostic value of PET-CT after first-line therapy in patients with follicular lymphoma: a pooled analysis of central scan review in three multicentre studies. Lancet Haematol 2014;1:e17–27. https://doi.org/10.1016/S2352-3026(14)70008-0
Valentini V, van Stiphout RG, Lammering G, Gambacorta MA, Barba MC, Bebenek M, et al. Selection of appropriate end-points (pCR vs 2yDFS) for tailoring treatments with prediction models in locally advanced rectal cancer. Radiother Oncol 2015;114:302–9. https://doi.org/10.1016/j.radonc.2015.02.001
van Mackelenbergh MT, Denkert C, Nekljudova V, Karn T, Schem C, Marmé F, et al. Outcome after neoadjuvant chemotherapy in estrogen receptor-positive and progesterone receptor-negative breast cancer patients: a pooled analysis of individual patient data from ten prospectively randomized controlled neoadjuvant trials. Breast Cancer Res Treat 2018;167:59–71. https://doi.org/10.1007/s10549-017-4480-5
Xie W, Regan MM, Buyse M, Halabi S, Kantoff PW, Sartor O, et al. Metastasis-free survival is a strong surrogate of overall survival in localized prostate cancer. J Clin Oncol 2017;35:3097–104. https://doi.org/10.1200/JCO.2017.73.9987
Xu XS, Ryan CJ, Stuyckens K, Smith MR, Saad F, Griffin TW, et al. Correlation between prostate-specific antigen kinetics and overall survival in abiraterone acetate-treated castration-resistant prostate cancer patients. Clin Cancer Res 2015;21:3170–7. https://doi.org/10.1158/1078-0432.CCR-14-1549
Zhao S, Zhang Z, Zhang Y, Hong S, Zhou T, Yang Y, et al. Progression free survival and one year milestone survival as surrogates for overall survival in previously treated advanced non small cell lung cancer. Int J Cancer 2018;144:2854–66.
No correlation coefficient or R2 (n = 13)
Lee CK, Lord S, Marschner I, Wu YL, Sequist L, Rosell R, et al. The value of early depth of response in predicting long-term outcome in EGFR-mutant lung cancer. J Thorac Oncol 2018;13:792–800.
Loupakis F, Cremolini C, Salvatore L, Schirripa M, Lonardi S, Vaccaro V, et al. Clinical impact of anti-epidermal growth factor receptor monoclonal antibodies in first-line treatment of metastatic colorectal cancer: meta-analytical estimation and implications for therapeutic strategies. Cancer 2012;118:1523–32. https://doi.org/10.1002/cncr.26460
Mandrekar SJ, Qi Y, Hillman SL, Allen Ziegler KL, Reuter NF, Rowland KM, et al. Endpoints in phase II trials for advanced non-small cell lung cancer. J Thorac Oncol 2010;5:3–9. https://doi.org/10.1097/JTO.0b013e3181c0a313
Mardis M, Davis J, Benningfield B, Elliott C, Youngstrom M, Nelson B, et al. Shift-to-shift handoff effects on patient safety and outcomes: a systematic review. Am J Med Qual 2017;32:34–42.
Meyer T, Palmer DH, Cheng AL, Hocke J, Loembé AB, Yen CJ. mRECIST to predict survival in advanced hepatocellular carcinoma: analysis of two randomised phase II trials comparing nintedanib vs sorafenib. Liver Int 2017;37:1047–55. https://doi.org/10.1111/liv.13359
An MW, Dong X, Meyers J, Han Y, Grothey A, Bogaerts J, et al. Evaluating continuous tumour measurement-based metrics as phase II endpoints for predicting overall survival. J Natl Cancer Inst 2015;107:1–7.
Okines AF, Gonzalez de Castro D, Cunningham D, Chau I, Langley RE, Thompson LC, et al. Biomarker analysis in oesophagogastric cancer: results from the REAL3 and TransMAGIC trials. Eur J Cancer 2013;49:2116–25. https://doi.org/10.1016/j.ejca.2013.02.007
Park WH, Shim JH, Han SB, Won HJ, Shin YM, Kim KM, et al. Clinical utility of des-γ-carboxyprothrombin kinetics as a complement to radiologic response in patients with hepatocellular carcinoma undergoing transarterial chemoembolization. J Vasc Interv Radiol 2012;23:927–36. https://doi.org/10.1016/j.jvir.2012.04.021
Pinato DJ, Arizumi T, Jang JW, Allara E, Suppiah PI, Smirne C, et al. Combined sequential use of HAP and ART scores to predict survival outcome and treatment failure following chemoembolization in hepatocellular carcinoma: a multi-center comparative study. Oncotarget 2016;7:44705–44718. https://doi.org/10.18632/oncotarget.9604
Schwaederle M, Zhao M, Lee JJ, Lazar V, Leyland-Jones B, Schilsky RL, et al. Association of biomarker-based treatment strategies with response rates and progression-free survival in refractory malignant neoplasms: a meta-analysis. JAMA Oncol 2016;2:1452–9. https://doi.org/10.1001/jamaoncol.2016.2129
Shao YY, Lin ZZ, Hsu C, Shen YC, Hsu CH, Cheng AL. Early alpha-fetoprotein response predicts treatment efficacy of antiangiogenic systemic therapy in patients with advanced hepatocellular carcinoma. Cancer 2010;116:4590–6. https://doi.org/10.1002/cncr.25257
Wong D, Ko AH, Hwang J, Venook AP, Bergsland EK, Tempero MA. Serum CA19-9 decline compared to radiographic response as a surrogate for clinical outcomes in patients with metastatic pancreatic cancer receiving chemotherapy. Pancreas 2008;37:269–74. https://doi.org/10.1097/MPA.0b013e31816d8185
Zhang Y, Zhang M, Chen M, Mei J, Xu L, Guo R, et al. Association of sustained response duration with survival after conventional transarterial chemoembolization in patients with hepatocellular carcinoma. JAMA Netw Open 2018;1:e183213. https://doi.org/10.1001/jamanetworkopen.2018.3213
Secondary publication, no additional data (n = 10)
Burzykowski T, Molenberghs G, Buyse M. The validation of surrogate end points by using data from randomized clinical trials: a case-study in advanced colorectal cancer. J R Stat Soc Ser A Stat Soc 2004;167:103–24.
Lee LM, Wang L, Crump M. Identification of potential surrogate endpoints in randomized clinical trials of aggressive non-hodgkin lymphoma: correlation of complete response, time-to-event and overall survival data. Blood 2009;114:1424–, Abstract 3699.
Narkhede MS, Cheson BD. Surrogate endpoints and risk adaptive strategies in previously untreated follicular lymphoma. Clin Lymphoma Myeloma Leuk 2018;18:447–51.
Rotolo F, Paoletti X, Burzykowski T, Buyse M, Michiels S. A Poisson approach to the validation of failure time surrogate endpoints in individual patient data meta-analyses. Stat Methods Med Res 2019;28:170–83. https://doi.org/10.1177/0962280217718582
Rotolo F, Paoletti X, Michiels S. surrosurv: an R package for the evaluation of failure time surrogate endpoints in individual patient data meta-analyses of randomized clinical trials. Comput Methods Programs Biomed 2018;155:189–98.
Savina M, Gourgou S, Italiano A, Dinart D, Rondeau V, Penel N, et al. Meta-analyses evaluating surrogate endpoints for overall survival in cancer randomized trials: a critical review. Crit Rev Oncol Hematol 2018;123:21–41.
Sherrill B, Amonkar M, Wu Y, Hirst C, Stein S, Walker M, Cuzick J. Relationship between effects on time-to-disease progression and overall survival in studies of metastatic breast cancer. Br J Cancer 2008;99:1572–8. https://doi.org/10.1038/sj.bjc.6604759
Sherrill B, Kaye JA, Sandin R, Cappelleri JC, Chen C. Review of meta-analyses evaluating surrogate endpoints for overall survival in oncology. Onco Targets Ther 2012;5:287–96. https://doi.org/10.2147/OTT.S36683
Van der Elst W, Molenberghs G, Alonso A. Exploring the relationship between the causal-inference and meta-analytic paradigms for the evaluation of surrogate endpoints. Stat Med 2016;35:1281–98. https://doi.org/10.1002/sim.6807
Xie W, Halabi S, Tierney JF, Sydes MR, Collette L, Dignam JJ, et al. A systematic review and recommendation for Reporting of Surrogate Endpoint Evaluation using Meta-analyses (ReSEEM). JNCI Cancer Spectr 2019;3:pkz002.
Insufficient data reported (n = 1)
Zierhut ML, Puchalski T, Rizo A, Xiu L, Tian H, Sharma A, et al. Is complete remission rate predictive of median overall survival? A model-based meta-analysis in patients with acute myeloid leukemia. J Pharmacokinet Pharmacodyn 2017;44:S59–S.
Non-English and insufficient detail (n = 1)
Paoletti X, Rotolo F, Michiels S. How a biomarker can become an acceptable substitution criteria? Bull Cancer 2016;103:S63–70.
Not available (n = 1)
Woo KM, Gönen M, Schnorr G, Silvestri GA, Bach PB. Surrogate markers and the association of low-dose CT lung cancer screening with mortality. JAMA Oncol 2018;4:1006–8. https://doi.org/10.1001/jamaoncol.2018.1263
Appendix 8 A targeted review of published National Institute for Health and Care Excellence technology appraisals for which initial marketing authorisation was based on response outcomes from single-arm studies
For each TA, a summary of the main clinical studies supporting the intervention being assessed along with the number of patients, the study location, the magnitude of the ORR and whether or not PFS and OS data were available were extracted. Full results can be seen in Table 33.
Intervention | NICE TA | Clinical study | Sample size (n) | Number of countries | ORR outcomes, response rate (%) (95% CI) | PFS outcomes, median (months) (95% CI) | OS outcomes, median (months) (95% CI) |
---|---|---|---|---|---|---|---|
Ceritinib | TA395228 | ASCEND-1229 | 163 | Multiple | 56.4 (48.5 to 64.2) | 6.9 (5.6 to 8.7) | 16.7 (14.78 to NE) |
ASCEND-2230 | 140 | Multiple | 38.6 (30.5 to 47.2) | 5.7 (5.4 to 7.6) | 14.9 (13.5 to NE) | ||
Osimertinib (Tagrisso, AstraZeneca, Cambridge, UK) | TA416231 | AURA ext232 | 201 | Multiple | 61.3 (54.2 to 68.1) | NC (8.1 to NC) | NC |
AURA2233 | 210 | Multiple | 70.9 (64.0 to 77.1) | 8.6 (8.3 to 9.7) | NC | ||
Pooled234 | 411 | Multiple | 66.1 (61.2 to 70.7) | 9.7 (8.3 to NC) | NC | ||
Nivolumab | TA462235 | CheckMate 205 cohort B236 | 80 | Multiple | 67.5 (57.2 to 77.8) | 14.78 (11.33 to NA) | NRe |
CheckMate 205 cohort C236 | 98 | Multiple | 73.0 (64.3 to 81.7) | 11.17 (8.51 to NA) | NRe | ||
CA209–039237 | 15 | Multiple | 60 (NRe) | 12.65 (5.91 to NA) | NRe | ||
Venetoclax | TA487238 | M12–175239 | 67 | Multiple | 82.1 (70.8 to 90.4) | 41.4 (17.7 to 41.5) | NA |
M13–982240,241 | 158 | Multiple | 77.2 (66.9 to 83.5) | 27.2 (21.9 to NA) | NRe | ||
M14–032 (cohort A)242,243 | 43 | Single | 67.4 (51.5 to 80.9) | NRe | NRe | ||
M14–032 (cohort B)242 | 21 | Single | 57.1 (34.0 to 78.2) | NRe | NRe | ||
Atezolizumab | TA492244 | IMvigor 210 Cohort 1245,246 | 119 | Multiple | 19.3 (12.66 to 27.58) | 2.7 (2.1 to 4.2) | 15.9 (10.4 to NE) |
IMvigor 210 Cohort 2247 | 310 | Multiple | 15.1 (11.3 to 19.6) | 2.1 (2.1 to 2.1) | 7.9 (6.7 to 9.3) | ||
Daratumumab | TA510248 | MMY2002249 | 106 | Multiple | 29.2 (20.8 to 38.9) | 3.7 (2.8 to 4.6) | 18.6 (13.7 to NRe) |
GEN501250 | 42 | Multiple | 35.7 (21.6 to 52.0) | 6.2 (4.2 to 11.6) | NRe (18.7 to NRe) | ||
Pooled251 | 148 | Multiple | 31.1 (23.7 to 39.2) | 4.0 (2.8 to 5.6) | 20.1 (16.6 to NRe) | ||
Avelumab | TA517252 | JAVELIN Part A253 | 88 | Multiple | 33.0 (23.3 to 43.8) | 2.7 (0.03 to 28.9) | 12.9 (7.5 to NE)d |
JAVELIN Part B254 | 39 | Multiple | 62.1 (42.3 to 79.3)b | 9.1 (1.9 to NRe) | NRe (9.1 to NRe)c | ||
Crizotininb | TA529255 | PROFILE 1001256,257 | 53 | Multiple | 69.8 (55.7 to 81.7) | 19.3 (14.8 to NRe) | NRe |
Pembrolizumab | TA540258 | KEYNOTE-087 cohort 1259 | 69 | Multiple | 75.4 (63.5 to 84.9) | 16.7 (11.2 to NRe) | NA |
KEYNOTE-087 cohort 2259 | 81 | Multiple | 66.7 (55.3 to 76.8) | 11.1 (7.6 to 13.7) | NA | ||
Brigatinib | TA571260 | ALTA (arm B)261 | 110 | Multiple | 56.4 (45.2 to 67.0)a | 15.6 (11.1 to 21.0) | 34.1 (27.7, NRe) |
ALTA (arm A)261,262 | 112 | Multiple | 45.5 (34.8 to 56.5)a | 9.2 (7.4 to 11.1) | 29.5 (18.2, NRe) | ||
Study 101 | 25 | Multiple | 76 (54.9 to 90.6)a | 16.3 (9.2 to NE); range 0.5–27.8) | NRe (1.4 to 24.3) |
Issue 1: overall response rate as a primary end point and possible surrogate relationship assumed for progression-free survival/overall survival
Given the different maturity in PFS and OS data evident in Table 33, the review further subdivides the TAs based on whether both median PFS and OS were reached or median PFS only was reached. This allowed the consideration of whether or not the approaches to dealing with different levels of maturity in these end points differed.
All 10 TAs used a model structure based on a PSM or area under the curve analysis comprising three mutually exclusive health states: (1) PFS (progression free), (2) progressive disease (PD; progression) and (3) death. Importantly, despite ORR being the primary end point supporting marketing authorisation, none of the TAs made use of the ORR or DoR data. Instead, the proposed approaches relied on extrapolations of the available PFS and OS data or used external evidence and/or assumptions.
Median progression-free survival and overall survival reached
The seven TAs for which the median PFS and OS had been reached are:
-
TA395228 – ceritinib (Zykadia, Novartis, Basel, Switzerland) for anaplastic lymphoma kinase (ALK) and NSCLC
-
TA492244 – atezolizumab for PD-L1 and urothelial cancer
-
TA510248 – daratumumab (Darzalex, Janssen-Cilag Ltd, Beerse, Belgium) for relapsed and refractory multiple myeloma
-
TA462235 – nivolumab (Opdivo, Bristol-Myers Squibb, New York City, NY, USA) for relapsed or refractory classical Hodgkin lymphoma (RRcHL)
-
TA540258 – pembrolizumab for RRcHL
-
TA487238 – venetoclax (Venclyxto, Abbvie, Lake Bluff, IL, USA) for chronic lymphocytic leukaemia
-
TA571260 – brigatinib (Alunbrig, Takeda, Tokyo, Japan) for ALK-positive NSCLC.
The observed survival data in each TA were extrapolated over a lifetime horizon using conventional parametric survival modelling. In accordance with the NICE DSU Technical Support Document 14,185 each company approached the data limitation by fitting various candidate distributions to the observed data, assessing statistical goodness of fit and clinical plausibility. Although median PFS and OS were reached in the clinical studies, the ERGs consistently highlighted concerns with the immaturity of the OS data relative to the long extrapolation periods applied within the economic models.
There appeared to be no obvious trend in terms of the committee’s final decision based solely on median OS having been reached. Atezolizumab and daratumumab both received recommendations for use in the CDF owing to uncertainty in their respective ICER estimates attributed to survival data immaturity. 244,248 Venetoclax also received a recommendation for use in the CDF. However, the uncertainty raised by the committee for this TA centred on the trial population and whether or not their disease severity reflected those in the NHS and not specifically uncertainty in the survival data. 238 Nivolumab and brigatinib both received recommendations for routine use in the NHS, despite immaturity of the survival data being highlighted by the committee and the ERGs. 235,260
Median progression-free survival reached and median overall survival not reached
The TAs based on studies that had not reached median OS were:
-
TA416 – osimertinib for EGFR T790M mutation-positive NSCLC231
-
TA517 – avelumab for Merkel cell carcinoma (MCC)252
-
TA529 – crizotinib for ROS1-positive NSCLC. 255
For these appraisals, there was a clearer trend in the final NICE decision, given the greater uncertainty surrounding the OS data. Recommendations for all three products were restricted to use in the CDF. There was also greater variation in the modelling approaches used to extrapolate OS data.
The TA of osimertinib231 included data from two studies, neither of which reached median OS at the time of the NICE appraisal. Despite the immaturity of the OS evidence, the extrapolation of OS was still undertaken using conventional parametric survival modelling. The committee concluded that these extrapolations were highly uncertain based on the very immature OS data, making it difficult to determine a robust cost-effectiveness estimate. Osimertinib was subsequently approved for use within the CDF despite the lack of robustness of the ICER estimates. The most critical factor appeared to be the committee’s view that there was plausible potential in the ICER estimates and that the uncertainties in OS would be addressed by an ongoing Phase III RCT.
For the TA of avelumab,252 the evidence base was derived from two cohorts: (1) treatment-experienced (second-line and further) metastatic patients (JAVELIN part A) and (2) treatment-naive (first-line) metastatic patients (JAVELIN part B). Each cohort was considered separately, reflecting a potentially different position of avelumab in the pathway. However, important differences in data maturity were evident in the second-line and further (median PFS and OS reached) and first-line (mean PFS but not OS reached) positions. Recruitment of first-line patients was also reported to be ongoing, such that more mature survival data were expected over time.
For the first-line and further populations, the company considered that the data were too immature to be extrapolated. As an alternative to extrapolating based on the immature evidence, the company proposed to estimate the relative improvement with avelumab that might be seen in treatment-naive patients compared with those in the treatment-experienced group. The company elicited a hypothetical HR for PFS and OS from clinical experts. The elicited HRs were then applied to the treatment-experienced avelumab PFS and OS curves (based on more mature evidence) to estimate equivalent estimates for treatment-naive patients receiving avelumab.
The ERG expressed significant concerns regarding the approach employed to adjust treatment effectiveness between treatment lines. Despite the immaturity in the survival data, the ERG expressed a preference to use independent survival functions fitted to the available PFS and OS data rather than using elicited HRs. However, the ERG also noted that using the observed survival data did not solve the fundamental issue of data immaturity in the treatment-naive population.
For first-line treatment of MCC, NICE recommended that avelumab was used in the CDF. This reflected the committee’s concerns regarding the immaturity of the PFS and OS data and the proposed use of clinical assumptions rather than direct evidence. The committee acknowledged that ongoing data collection in JAVELIN part B would reduce the uncertainty about the progression-free and overall survival benefit and that there was plausible potential for first-line use of avelumab to be cost-effective, if further trial data proved favourable.
Crizotinib for treating ROS1-positive advanced NSCLC was the only TA in which the evidence of clinical benefit was based on a single clinical study. The clinical-effectiveness evidence was based on a single-arm study (n = 53), with a median follow-up of 25.4 months. Median OS had not been reached at the time of the appraisal. Owing to the small study and immature survival data from this study, the company proposed the use of more mature PFS and OS data from previous RCTs of crizotinib for ALK-positive NSCLC as a proxy for ROS1-positive patients.
The committee considered the use of proxy data for ROS1-positive patients from a RCT to be more robust than using the available immature ROS1-positive PFS and OS curves from a single-arm study. However, using data from a proxy population was concluded to be far from ideal, making the assessment of clinical effectiveness and cost-effectiveness highly uncertain. Although the committee agreed to explore the proxy data in its decision-making, the committee also stated that the approach was very unusual and should not set a precedent for such an approach in future appraisals.
Given these uncertainties, crizotinib was not recommended for routine use in the NHS for patients. However, crizotinib was recommended for use in the CDF. The committee considered that further data on the use of crizotinib within the CDF would help to address uncertainties in existing survival data estimates, particularly the comparability of ROS1-positive and ALK-positive advanced NSCLC.
Issue 2: challenges of heterogeneous populations
Owing to the nature of basket trials, significant heterogeneity may be present in the study populations enrolled in the trials. The potential importance of accounting for heterogeneity and exploring the cost-effectiveness in subgroups of the target population is acknowledged in the current NICE methods guide. 4 Differences in the cost-effectiveness and decision uncertainty across these separate subgroups may lead to an optimised recommendation that is more restrictive than the marketing authorisation.
Although the review of the 10 TAs provided examples of appraisals in which heterogeneity had been accounted for within an overall target population, the evidence for the separate subgroups was commonly derived from separate studies relevant to specific subgroups or from studies in which there were relatively large numbers of patients to undertake meaningful subgroup analysis. These appraisals also typically considered only a small number of subgroups, most commonly based on alternative positions of a new treatment in an existing pathway (i.e. first or second line). Although these findings are helpful in demonstrating the potential importance of accounting for heterogeneity within a target population, important differences are also expected for histology-independent appraisals, given the potential for a much larger number of potential subsets and smaller sample sizes. The review was subsequently broadened to consider select additional TAs for which issues related to heterogeneity were considered more like those expected for histology-independent appraisals.
The NICE appraisals for neuroendocrine tumours (NETs), considered in TA449 [everolimus (Afinitor®; Novartis, Basel, Switzerland) and sunitinib] and TA539 (lutetium), appear to be particularly relevant. NETs affect different organs, namely the pancreas, GI tissue and lungs. The broad population covered by the marketing authorisation was acknowledged in the NICE scoping documents, which stated that the relevant population was people with progressed unresectable or metastatic neuroendocrine tumours according to the specific locations covered by the existing and anticipated marketing authorisations. However, heterogeneity with the licensed population was recognised and the NICE scopes also stated that the location of the tumour should be considered as a basis for identifying possible subgroups.
In both TAs, the NICE committee considered each organ separately and issued optimised recommendations based on tumour site. For example, everolimus and sunitinib were both recommended for pancreatic NET, while everolimus was recommended only for GI and lung NETs. The optimised recommendations were possible because the companies either submitted separate evidence for the different sites or provided subgroup analysis related to specific organs. In these appraisals, the committee acknowledged the importance of considering each organ separately, noting that prognosis, quality of life and cost of comparator therapies were likely to differ, which would affect the cost-effectiveness estimates.
A similar example is found in the appraisal of denosumab for the prevention of skeletal-related events in adults with bone metastases from solid tumours (TA265). The scope of this appraisal covered a broad population characterised by a wide range of histologies, given that almost any form of solid tumour can metastasise to the bone. Again, the NICE scope acknowledged that there was possible heterogeneity within the licensed population and suggested that the appraisal should also consider patient subgroups based on location or type of primary cancer.
Separate studies were available for TA265 for different tumour types, thus allowing for separate clinical effectiveness and cost-effectiveness analyses to be performed. For example, the company submitted a model assessing the cost-effectiveness of denosumab in the three different patient groups: breast, prostate and other solid tumours. Different risks, such as skeletal-related adverse events and mortality, and utility values were assigned to reflect differences between cancer types. The separate analyses led, again, to separate recommendations. Denosumab was approved for routine use for adults with bone metastases from breast cancer and other solid tumours, but not from prostate cancer.
Issue 3: challenges of developing a counterfactual
Company submissions supporting histology indications will frequently, if not always, present data collected as part of single-arm studies. The lack of a direct comparator creates challenges because estimates of comparative effectiveness are essential to perform robust cost-effectiveness assessments. A previous review comparing the results from single-arm studies with those from randomised designs led the authors to conclude that single-arm studies can be considered to provide reliable indicators of treatment benefit only when the disease natural history is very well known, the patient population is homogeneous and the control (standard care) treatment has little affect on outcomes. 194 Current guidance on the selection of a counterfactual and methods to deal with possible biases has also been reported to be limited. 263
Hatswell et al. 263 previously performed a review and developed a taxonomy of approaches used in economic modelling for drugs, which were previously licensed by the FDA or EMA without RCT data. The most commonly identified approach used a historical control, although there was variation in the sources of comparison data (i.e. single trial, meta-analysis of multiple trials, registry data or expert opinion). Importantly, the review highlighted that most submissions did not try to control for differences between trials, thus performing a ‘naive’ comparison.
Naive comparisons are prone to bias in the presence of systematic differences between patients across clinical studies. Several approaches have been proposed to control for observable (and unobservable) differences between non-randomised comparisons by balancing baseline covariates or matching patients. These methods are outlined in a series of NICE DSU TSDs162,264 and a related report. 265 TSD17264 provides practical guidance on methods used to analyse treatment effect data from non-randomised studies, including an algorithm for method selection. The methods reviewed are separated according to the assumption of selection on observables (such as regression adjustment and propensity score matching) or selection on unobservables (instrumental variable and panel data methods). Natural experiment designs are also considered, utilising difference in differences and regression discontinuity approaches. A subsequent DSU report builds on TSD17 by assessing current guidance by NICE on the use of real-world data,265 another situation in which the analyses are particularly prone to selection bias. Finally, TSD18162 (namely in its sub-sections ‘Matching-adjusted Indirect Comparisons“ and ”Simulated Treatment Comparisons’) considers the use of novel methodologies for improving indirect comparisons, while controlling for imbalances in baseline characteristics across different studies.
The approach used to estimate the counterfactual in the 10 NICE TAs included in the review was classified according to the taxonomy developed by Hatswell et al. 263 This taxonomy distinguishes between the approach taken to developing a comparison group and the source of these data (Table 34). One appraisal was excluded (TA529)255 because the submission was based on proxy data from a randomised trial.
Intervention | NICE TA | Classification | |
---|---|---|---|
Approach taken | Source of comparison data | ||
Ceritinib | TA395228 | Historical control | Clinical trial |
Osimertinib | TA416231 | Historical control | Clinical trial |
Nivolumab | TA462235 | Historical control | Case series |
Venetoclax | TA487238 | Historical control | Clinical trial |
Atezolizumab | TA492244 | Historical control | Meta-analysis |
Daratumumab | TA510248 | Historical control | Mixed sources |
Avelumab | TA517252 | Historical control | Mixed sources |
Pembrolizumab | TA540258 | Historical control | Case series |
Brigatinib | TA571260 | Historical control | Clinical trial |
All nine TAs generated a counterfactual by using a historic control. However, there was variation in the source of comparison data.
Single clinical trial
The four TAs generating a counterfactual using data from a single external trial arm were TA395 (ceritinib for ALK and NSCLC),228 TA416 (osimertinib for EGFR T790M and NSCLC),231 TA487 (venetoclax for chronic lymphocytic leukaemia)238 and TA571260 (brigatinib for ALK and NSCLC). Each of these TAs considered only a single source of external evidence or, when multiple sources were available, did not make an attempt to pool the data, and instead conducted indirect comparisons using one source at a time.
In these appraisals, the committee expressed concerns that the single-arm design of the trials made it difficult to assess the efficacy of the new treatment owing to the lack of a comparator arm. The committee also expressed concerns that these difficulties were compounded by the small numbers of patients in the trials. Although the use of a historic control from a single external trial was generally accepted as an approach to inform the counterfactual, the committees clearly closely scrutinised the source of external data and the adjustment approaches applied. This was particularly evident when only naive comparisons were presented, as was the case for the appraisals of ceritinib and venetoclax.
The evidence used in the ceritinib submission was critiqued by the committee owing to the lack of an appropriate match between patient characteristics and the limited information about the treatments received by the historical control. This led the committee to conclude that the naive approach presented by the company was inappropriate. However, in the absence of any suitable alternative estimates or approaches, the committee concluded that the results presented by the company represented the best evidence available for their decision-making even though they were highly uncertain.
Similarly, in the venetoclax appraisal the committee highlighted the lack of any attempt to match for difference in baseline characteristics and considered the approach to be biased in favour of venetoclax. Again, in the absence of any alternative approaches, the naive approach was concluded to provide an acceptable basis for decision-making, but the results were highly uncertain.
Both the osimertinib and the brigatinib appraisals used adjusted comparisons. The osimertinib appraisal used a subgroup of patients with EGFR T790M+ in the control arm of an external prospective, randomised Phase III study and undertook comparative analyses using propensity score matching. Although the committee and the ERG acknowledged the company’s approach to adjusting for possible confounding, concerns remained regarding the immaturity of OS data and the small number of patients.
In the brigatinib appraisal, data for the comparator, ceritinib, came from two separate trials that were assessed separately. The company performed both a naive comparison and an unanchored MAIC. The committee acknowledged the consistency of the results across both the naive and the adjusted analysis. Despite limitations identified relating to the assumptions of the MAIC, the consistency across the different sets of results appeared to provide reassurance to the committee, who considered that the comparator evidence was acceptable.
Case series
The TAs supporting the submission with the use of case series data were TA462 (nivolumab for classical Hodgkin’s lymphoma)235 and TA540 (pembrolizumab for classical Hodgkin lymphoma). 258 Both submissions compared their respective product with SoC data collected from a US database of patients who had been treated with brentuximab vedotin between 2007 and 2015. In both submissions, naive indirect comparisons and MAICs were carried out. The main issue raised by the committee was related to the relevance of the US database to UK practice. For both TAs, the committee acknowledged that the US database might not fully represent UK practice. However, the committee also deemed it to be the best available evidence, while acknowledging that the comparative effectiveness results were highly uncertain.
Meta-analysis
TA492 (atezolizumab for PD-L1 and urothelial cancer)244 derived comparator data from historical trial sources using a range of approaches, including a STC, MAIC and network meta-analysis.
The initial company submission presented a STC. A STC is a statistical model that describes the outcomes in terms of the covariates fitted to the IPD for the treatment of interest. This model is used to predict the outcomes that would have been observed in a population with the same characteristics as the historical comparator data source(s). The company then performed a network meta-analysis by linking the outcomes of the various STCs for separate comparators. The ERG highlighted several concerns, particularly the limited number of covariates used in the STC prediction model and the lack of justification for the covariate selection. In response to consultation, the company also provided results from a MAIC to validate the results from the STC.
The committee acknowledged the ERG’s concerns and concluded that the STC analysis was not robust. The committee agreed that the MAIC provided useful validation, but that did not alter its view that the adjustment approaches were not robust. Although the committee acknowledged that atezolizumab was likely to be clinically effective, they had concerns about the magnitude of the effect size given the lack of robust adjustment. Atezolizumab was subsequently approved for use within the CDF based on the committee’s view that there was plausible potential the treatment was cost-effective and that the key uncertainties surrounding comparative efficacy would be addressed by an ongoing RCT.
Mixed sources
Two TAs, TA510 (daratumumab for multiple myeloma)248 and TA517 (avelumab for MCC),252 considered different approaches and sources. The main submission for daratumumab was based on a MAIC between the daratumumab trials and other comparator trials. However, the ERG and committee expressed concerns about the unreliability of the estimates because of the number of variables that could be controlled for. The company subsequently performed an additional regression analysis of IPD from the pooled daratumumab cohort and the International Myeloma Foundation registry. The ERG considered that multivariate regression and MAIC were very different methods and, therefore, it was inappropriate to use the multivariate regression to validate the results of the MAIC. Accordingly, the committee concluded that it was not possible to establish the relative effectiveness of daratumumab owing to the high level of uncertainty in the relative effectiveness estimates, issues with the number of variables controlled for in the MAIC and the lack of cross-validation of the MAIC with other estimates. Despite these concerns, the committee approved daratumumab for use in the CDF. This was justified by the committee based on the plausible potential that daratumumab could be cost-effective and the view that additional data being collected within the early access programme would provide more robust evidence on the clinical effectiveness of daratumumab.
In the avelumab appraisal, the company performed a naive comparison with a retrospective observational study of patients with metastatic MCC. The company supplemented this with regression analysis, but the ERG had concerns owing to data immaturity and small numbers of patients. Again, problems were mostly around identification of subgroups and variables that might influence the final estimates and lack of suitable head-to-head data.
Issue 4: validation of a new test and biomarker
Three TAs were selected for the case studies in this section; these were of targeted technologies that were ‘first in class’ or positioned at a new point in the treatment pathway at which diagnostic testing was not presently commonplace for the relevant genomic alteration. These appraisals contained a discussion of the specific issue of identifying patients with the genomic alteration for which the technology was licensed. We explored how evidence related to the diagnostic accuracy of the test and the predictive and/or prognostic performance of the biomarker was considered.
Diagnostic accuracy
To ensure that individuals are able to access targeted treatments, diagnostic tests are required to identify eligible patients. These tests are not specifically appraised during the STA process; however, it is important to consider the diagnostic accuracy of available testing and the appropriateness of proposed testing strategies because these have implications on the population that is identified and the costs incurred. Implementation of diagnostic strategies with a low sensitivity (high rates of false negative patients) would mean that a proportion of patients are likely to be missed, while strategies with low specificity (high rates of false-positive patients) may result in additional resources allocated to unnecessary procedures.
Crizotinib for untreated anaplastic lymphoma kinase-positive non-small cell lung cancer (TA406)
To identify an ALK-positive patient, it was assumed that the implemented testing strategy would consist of patients first tested with IHC, with positive cases confirmed by FISH. Little detail was provided into the diagnostic accuracy of these types of test to identify ALK mutations; however, it was stated that studies have indicated that IHC is sensitive and specific for determining ALK status and is a viable alternative to FISH. Although the validation of a companion diagnostic test is not required in the context of a NICE submission, the company’s IHC test for detecting ALK status did have FDA approval as a companion diagnostic for crizotinib and also received CE marketing for use in Europe.
To calculate the cost per ALK-positive patient of testing for ALK status, the company submission described the expected distribution of NSCLC patients according to IHC and FISH tests using data pooled from two sources to estimate the total testing costs. These used assumptions regarding the positivity rate of the IHC test using a specific antibody for ALK testing to estimate the number of confirmatory FISH tests that would be required. The company noted that two antibodies were available for use in ALK testing; however, only the antibody that was considered to be more accurate was used in the analysis.
The ERG provided further detail on the accuracy of the IHC test compared with the FISH test. It was acknowledged that there was a possibility that some patients would be incorrectly treated with crizotinib if this testing strategy is adopted, but the exact number was unknown. The ERG considered that the proposed test strategy of IHC followed by confirmatory FISH to be reasonable in the context of their diagnostic accuracy.
Osimertinib for T790M non-small cell lung cancer (TA416)
The testing strategy in the analysis of osimertinib consisted of either tissue biopsy or circulating tumour DNA (ctDNA) followed by biopsy in those who were negative for the T790M mutation.
The clinical effectiveness of osimertinib was evaluated in the AURA clinical trial programme. Patients were screened using a tissue biopsy and were centrally assessed. The tissue biopsy was shown to have a high accuracy rate, with the large majority of patients who were screened as eligible for osimertinib being confirmed as T790M positive (three patients were later found to not have the T790M mutation and one was of unknown status with insufficient tissue to carry out the test).
The sensitivity and specificity of the tissue biopsy and ctDNA were described within the context of estimating the expected testing costs to identify a patient with the T790M mutation. The sensitivity and specificity of tissue biopsy were obtained from a single study and from unpublished results for ctDNA. The company estimated the overall positive detection rate of 60.1% (i.e. for every 1.66 patients tested, one patient is identified as T790M-mutation positive and is eligible for osimertinib treatment). Owing to limitations of data, the company made assumptions regarding the diagnostic accuracy, assuming that it would be equal in patients who would be eligible for osimertinib as a second-line and as a third-line treatment. The affect of varying the diagnostic accuracy of these tests on the cost-effectiveness of osimertinib was not explored.
Crizotinib for ROS1-positive non-small cell lung cancer (TA529)
The primary testing strategy for the identification of patients with the ROS1 oncogene was expected to consist of IHC screening followed by confirmatory FISH. However, in the pivotal clinical trial for crizotinib in ROS1-positive NSCLC, the majority of patients were identified at the screening stage through either central or local testing using FISH and a small number were identified using PCR. Retrospective testing using NGS showed that two patients in the trial were actually ROS1 negative.
The cost of identifying ROS1-positive patients in the economic analysis consisted of IHC followed by confirmatory FISH. The number of confirmatory FISH tests required was based on the expected sensitivity and specificity of IHC. The company cited an 83% specificity and 100% sensitivity for IHC, as suggested by a validation study for the use of ROS1 IHC staining in screening for ROS1 translocations in lung cancer. FISH was assumed to have a sensitivity and specificity of 100%, given that FISH was the reference test in the diagnostic accuracy study that provided the specificity of IHC in ROS1 testing.
Predictive validity
Predictive biomarkers provide an estimate of the expected response to treatment and are often targets for treatment. If a biomarker is not predictive, the targeted treatment will not work for patients without the biomarker present. It is important to understand the predictive nature of the biomarker: it will be difficult, without evidence to support this, to estimate and adjust for the degree of error in estimates of relative effectiveness.
Crizotinib for untreated anaplastic lymphoma kinase-positive non-small cell lung cancer (TA406)
Anaplastic lymphoma kinase was identified as a key oncogenic driver in a number of cancers, including NSCLC. The role of the ALK oncogene in cancer development and the clinical basis for the underlying mechanism of crizotinib in relation to the ALK oncogene is described in the company submission. Crizotinib is an inhibitor of ALK and is alleged to block the activity of the abnormal ALK protein, which slows the growth and spread of the cancer in ALK-positive NSCLC and may cause the cancer to shrink.
Given that there was no evidence presented for the impact of crizotinib in ALK-negative patients, it was not possible to compare outcomes with those who are ALK positive; therefore, for this reason, the predictive validity of the ALK oncogene cannot be commented on in this respect.
Osimertinib for T790M non-small cell lung cancer (TA416)
The submission provided a description for the clinical basis of the predictive validity of the T790M mutation. Osimertinib was positioned as a second-line option for those who did not respond to an EGFR TKI for NSCLC. EGFR mutation status had been established as a key predictive biomarker in NSCLC, correlating with sensitivity to an EGFR TKI. However, patients subsequently develop resistance to therapy, which can be because of either secondary mutations or activation of bypass signalling pathways. The T790M mutations account for 50–60% of all cases of acquired resistance, and secondary T790M mutations were believed to provide resistance to EGFR TKIs by two potential mechanisms.
As with crizotinib for ALK-positive NSCLC, no mutation-negative patients received treatment with osimertinib; therefore, it was not possible to compare outcomes with those who are mutation positive and, subsequently, evaluate empirical evidence of the predictive validity of the T790M mutation.
Crizotinib for ROS1-positive non-small cell lung cancer (TA529)
Given that there was no comparative evidence for patients in a ROS1-positive population, the company assumed equivalent efficacy of crizotinib as was observed in ALK-positive patients. The similarities between these two groups of patients were recognised by the EMA, who considered the generalisability of data from ALK-positive patients to the ROS1-positive patients to be sufficient in their approval of crizotinib. The rationale for the similarities was described in terms of both their biological basis and the similarities in observed clinical behaviour, such as response to crizotinib, patient characteristics (e.g. age and smoking status) and histology.
The appraisal committee considered that relative effectiveness remained uncertain but agreed to explore the proxy data in its decision-making. However, the committee regarded this approach as very unusual and stated that this should not set a precedent for the use of data from proxy populations in future appraisals. The lack of knowledge on the implications of the ROS1 oncogene was a factor in the decision to recommend crizotinib through the CDF to collect data about its use in ROS1-positive advanced NSCLC. The committee concluded that collecting data on disease progression in people with ROS1-positive NSCLC treated with crizotinib would help to address the uncertainties around the survival benefit and the comparability of ROS1-positive and ALK-positive NSCLC populations.
Retrospective testing using NGS showed that two patients enrolled in the pivotal trial crizotinib were actually ROS1 negative. Although the ROS1-negative patients were included in the ITT analysis of the trial data, the company presented a scenario in which these patients were excluded from the analyses. These two patients were described as having a worse response than or comparable response to most other ROS1-positive patients, which suggests a predictive impact associated with the ROS1 oncogene.
Prognostic validity
Prognostic biomarkers indicate the likelihood that a patient will have a particular disease course or natural history independent of treatment, such as the risk of disease progression or the mean survival time. Those with the biomarker present might be expected to experience a different course of disease to someone without the biomarker. The prognostic implications of the target mutation are important when considering the outcomes of patients receiving standard care. This is especially the case given that trials of new targeted therapies often do not contain a control arm. In many cases in which the relevance of the biomarker is a new discovery, evidence for the natural history of patients with the target mutation will be limited. When only the clinical outcomes of the targeted therapy are known, estimating the relative clinical effectiveness, and subsequently the cost-effectiveness, will be challenging.
Crizotinib for untreated anaplastic lymphoma kinase-positive non-small cell lung cancer (TA406)
The evidence on the prognosis of ALK-positive patients receiving standard chemotherapy was limited at the time of the appraisal, with research into ALK-positive patients having been studied only in the context of investigations of crizotinib.
The life expectancy for patients with ALK-positive NSCLC receiving standard care could not be established with any certainty. Four estimates of the median OS for chemotherapy were presented, but the applicability of these trials to the decision problem was limited. Two trials were identified that enrolled a population that was not specifically ALK positive, that is it is possible that both ALK-positive and ALK-negative patients were enrolled, and a further trial was identified of ALK-positive patients, of whom the majority were not a first-line population, having received previous treatments for advanced disease.
The prognosis of ALK-positive patients was compared to that of general NSCLC patients, but it was considered that differences in survival could be a result of differences in the patient populations. It was established that ALK-positive patients tended to be younger, with a median age in the early 50s for ALK-positive patients as opposed to mid- to late 60s for ALK-negative NSCLC, and are more likely to be non-smokers.
However, no information was presented regarding the disease burden of ALK-positive patients relative to ALK-negative NSCLC patients and, therefore, it was not possible to draw any conclusions as to the impact of ALK status to the prognosis of these patients.
Osimertinib for T790M non-small cell lung cancer (TA416)
The role of the T790M mutation in patient prognosis was not understood at the time of this appraisal, and the discussion regarding a plausible biological basis for any differences between groups of patients was not presented. However, the company presented a number of analyses comparing outcomes for T790M mutation-positive and T790M mutation-negative patients to demonstrate empirically the extent to which this biomarker may influence prognosis.
The company presented the results of a subgroup analysis by the presence of T790M status for patients receiving chemotherapy. However, it was acknowledged that the trial used in the example was not designed to explore differences between T790M mutation-positive and T790M mutation-negative patients, and the patients were identified retrospectively as having the EGFR T790M mutation; therefore, the conclusions that could be drawn from this analysis were limited.
The median TTP for patients on untargeted chemotherapy was demonstrated as being similar between T790M mutation-positive and T790M mutation-negative patients; however, there was some limited evidence to show that there may be some long-term differences, with a Kaplan–Meier plot for OS illustrating some divergence between the two groups after 12 months (the T790M mutation-positive group having marginally poorer survival).
Clinical advice given to the ERG, however, contradicted this evidence of poorer prognosis and suggested that patients with EGFR mutation-positive NSCLC have a better prognosis than patients in an unselected advanced NSCLC population. This is because they tend to be younger and have fewer co-morbidities. This difference in opinion demonstrates that the role of T790M mutations in the prognosis of NSCLC was yet to be established.
Crizotinib for ROS1-positive non-small cell lung cancer (TA529)
The ROS1 oncogene was a relatively new discovery at the time of the appraisal and ROS1-positive advanced NSCLC is an ultra-orphan indication. For this reason, little was known about the natural history, patient characteristics and clinical effectiveness of untargeted chemotherapy for tumours that are ROS1 positive.
For this reason, the majority of the discussion regarding the prognostic validity of the ROS1 mutation was limited to its biological basis, with very limited clinical evidence yet available to demonstrate any differences between ROS1-positive and ROS1-negative groups empirically.
At the time of the appraisal, differences between the characteristics of ROS1-positive patients and the characteristics of patients with unselected NSCLC had been established to only a limited degree, with ROS1 positivity showing some associations with non-smoker status and a younger age at diagnosis, both of which are established prognostic factors. NSCLC associated with an underlying ROS1 gene rearrangement is fundamentally different from unselected NSCLC, as disease progression in ROS1-positive NSCLC patients is dependent on the activated ROS1 receptor tyrosine kinase protein.
A systematic review conducted by the company found that the limited studies that reported long-term outcomes for ROS1 patients on chemotherapy were based on very small patient numbers and were not considered to provide reliable estimates of OS. As a result, the prognosis of ROS1 patients on chemotherapy was assumed to be equivalent to that of ALK-positive patients, and data from patients with ALK-positive NSCLC were used as a proxy for the life expectancy of ROS1-positive NSCLC patients treated with current SoC. The similarities between ROS1-positive and ALK-positive NSCLC allowed for the use of the better-quality data available in the latter indication. Evidence in the ALK-positive population was more established, with a large Phase III trial of previously treated patients and two previous NICE appraisals in this indication, and there was greater clinician experience. Clinical experts predicted that ROS1-positive advanced NSCLC patients will be comparable with overall ALK-positive patients owing to the similar patient characteristics and homology (see Chapter 6, Types of genomic test). Similar to ALK-positive NSCLC, ROS1-positive NSCLC was not considered to be a favourable prognostic factor.
As a result of the uncertainty regarding the comparability of the ROS1 and ALK populations, the most plausible ICERs were considered highly uncertain and crizotinib was recommended for use in the CDF for this indication. This enabled evidence to be collected on patient characteristics and natural history, to further understand the ROS1 population and similarities to the ALK-positive population.
Issue 5: implementation challenges of incorporating a new diagnostic approach/pathway
To ensure that individuals can access targeted treatments, such as those that are histology independent, the infrastructure to identify such patients is required. The introduction or alteration of such infrastructure is associated with several challenges. Capacity constraints have been identified as a key barrier to the introduction of precision medicines into the NHS. 266 An increase in service provision may result in an investment in NHS genomics services to increase staffing capacity and laboratory infrastructure and a need for education and training to ensure that clinicians are aware of where targeted medicines could fit within the treatment pathway. Not only will the requirement of diagnostic tests for patient identification result in additional costs to the NHS, the way that patients are identified could also have implications on the type of patients who receive treatment and how similar they are to the patients enrolled in the trials. There may be a variety of testing strategies that could be used in clinical practice, including the diagnostic tests that are used and in which sequence they are used. The time at which patients are identified, whether tested at diagnosis or after treatment failure, may influence the relevant comparator treatment, which differs by treatment line. In this section, we discuss the extent to which these issues are explored in a number of TAs of targeted therapies.
Crizotinib for anaplastic lymphoma kinase-positive non-small cell lung cancer (TA406)
Crizotinib for the treatment of ALK-positive NSCLC was evaluated initially as a second-line therapy (TA296), with a first-line indication evaluated subsequently in this appraisal. At the time of the appraisal, infrastructure was already in place for the service provision and management of molecular testing to confirm ALK status, with several providers set up with this testing facility. Several issues regarding the implementation of ALK testing were discussed, including the testing strategy, the timing of testing, the unit costs of testing and the impact of testing to the number of eligible patients who receive treatment.
Testing strategy
The company provided details of a two-tiered testing approach to identify ALK-positive patients in clinical practice. This was a strategy that was endorsed by two professional bodies [ESMO and the Royal College of Pathologists (RCP)] and was also implemented in the economic analysis. No specific tests are detailed in the summary of product characteristics (SmPC) for crizotinib and, therefore, the company provided a description of the specific IHC and FISH assays that are endorsed and validated by other clinical bodies, such as ESMO, RCP and FDA. This approach to diagnosis appears to differ to the strategy that was used in the pivotal trial of crizotinib, for which the identification of ALK patients was based only on a FISH test. However, limited discussion was given as to the implications of the differing testing strategies regarding the patient population identified, although the ERG noted the potential for a two-tiered approach resulting in delays to treatment and patients having a reduced capacity to benefit from treatment if the disease is allowed to progress.
The clinical effectiveness and cost-effectiveness implications of testing strategies using alternative diagnostic tests, such as NGS or RT-PCR, were not explored by the company in this appraisal. The company justified their approach by stating that IHC and FISH represent the significant majority of tests used in the NHS and provided supporting information on the number of IHC tests used in practice. However, the ERG noted that the possibility of using NGS would make the cost of ALK testing less predictable in the near future.
Timing of testing
In this appraisal, crizotinib was evaluated as a first-line treatment for NSCLC. At the time, first-line treatments for NSCLC existed that targeted the EGFR mutation. The company assumed that testing would be carried out upfront at diagnosis, alongside EGFR testing, based on feedback from an advisory board. Upfront testing alongside EGFR tests means that there is no significant increase in the number of tests required and no potential capacity issues. Sequential testing of ALK status (i.e. after EGFR testing) was not acknowledged as an option.
Unit costs of testing
At the time of the appraisal, there was some uncertainty regarding the unit costs of testing because it was unclear whether or not laboratory and overhead costs were included in the cost supplied by the company. The impact to the cost-effectiveness of crizotinib by using alternative unit costs of treatment that were estimated by other sources was explored, with a higher cost of testing being associated with a modest increase to the ICER. However, the committee considered that the true cost remained uncertain and that it was likely to lie between the ranges identified.
Impact on the number of patients identified
The challenges of a new diagnostic process were described as having an impact on the number of patients expected to be eligible for crizotinib treatment, noting that the number of patients who received the treatment while it was available on the CDF for a later line of treatment was smaller than the expected number of eligible patients, given that not all ALK-positive patients were being identified in practice.
Osimertinib
Osimertinib, appraised for NSCLC patients with a T790M mutation (TA416),231 was positioned as a second-line treatment option following treatment with an EGFR TKI, given the low prevalence of T790M mutations at diagnosis. The challenges in the diagnostic pathway with a second-line therapy were discussed, including the increase in service provision and the testing strategy required.
Increase in service provision
The identification of patients eligible for osimertinib was discussed as the main additional resource use to the NHS. The appraisal discusses how not all centres routinely test for the EGFR T790M mutation either at diagnosis or after treatment failure with a first-line EGFR TKI, and its introduction will, therefore, necessitate a change in service provision.
The expansion of testing was not considered to be problematic because the pathway for acquisition, handling and testing of tissue, in addition to mechanisms for reporting of results, was described as being well-established; therefore, no additional costs were associated with the assessment of tumour specimens beyond the increase in testing volumes. Details of the laboratories enrolled to conduct EGFR testing and their current ability to detect T790M mutations using existing platforms were provided to support this assumption.
However, tissue biopsy at disease progression following resistance to EGFR TKI therapy is not routine, and the company provided a detailed description of how the change of pathway to acquire tumour specimens would be implemented. There were a number of challenges highlighted, including the optimal selection of lesions for biopsy owing to tumour heterogeneity and reduced willingness to undergo tissue biopsy. Feasibility studies to validate the pre-analytical steps of the plasma processing pathway were expected to commence shortly after the appraisal.
Testing strategies
Four possible testing strategies to detect T790M mutations were described: (1) tissue biopsy, (2) ctDNA (plasma) test followed by tissue biopsy in patients identified as T790M negative by ctDNA, (3) ctDNA alone and (4) tissue biopsy followed by ctDNA. The company considered that only the first two testing strategies were relevant and in line with the SmPC for osimertinib and included a weighted average of these strategies in their base-case analysis based on the proportion expected to be identified in each way. A number of clinical benefits with the use of ctDNA were described, with it being a less expensive alternative and offering more rapid results, and mitigating the complications associated with the acquisition of lung tissue samples, which may be of particular concern for later-stage disease.
Crizotinib for ROS1-ve non-small cell lung cancer
The appraisal of crizotinib for treating ROS1-positive advanced NSCLC (TA529)255 also highlighted some uncertainty with the introduction of diagnosis into the patient pathway. Diagnostic testing was not routinely carried out in England and Wales to identify ROS1-positive patients at the time of the appraisal; however, there were pre-existing targeted treatments available for NSCLC for which patients were tested for the associated biomarker for EGFR and ALK at diagnosis of NSCLC. The discussions around the challenges of identifying ROS1-positive patients focused on the point in the pathway that ROS1 would be detected and the implications of testing to the time of crizotinib treatment.
Timing of testing
The company presented different scenarios to illustrate the impact of introducing testing at different points in the treatment pathway: one scenario where testing could be carried out upfront on diagnosis alongside testing for other targets associated with treatment for NSCLC (EGFR and ALK) or a scenario where testing would be carried out sequentially after confirmed EGFR negativity and ALK negativity. Upfront testing minimises tissue wastage and avoids delays in the access to therapy by waiting for the patient to complete testing for the targets with existing therapies.
Other parties, including NHS England and the NICE technology appraisal committee (TAC), also considered that upfront testing was more appropriate than sequential testing. The ERG also considered the affect of the timing of testing on the cost that it incurs. For example, there may be a discount available for upfront testing when testing for more than one mutation at the same time. In addition, patients treated in the subsequent line would already have been tested for ALK and/or other mutations, so the cost of testing these (ALK-positive) patients need not be taken into account.
Implications of testing to the timing of treatment
The issue was also raised of the positioning of crizotinib in the pathway. Although the economic analysis considered crizotinib against a comparator that is commonly used as first-line treatment in NSCLC, it was expected that patients treated by crizotinib in clinical practice may be either treatment naive or treatment experienced. If access to diagnostic testing causes delays in the diagnosis of ROS1 positivity, or ROS1 testing had not been carried out prior to initiating first-line therapy, patients would be treatment experienced on starting crizotinib; however, over time it was expected for patients to become predominantly treatment naive as testing becomes more established and diagnosis occurs at an earlier stage in the treatment pathway.
Appendix 9 OpenBUGS code
Appendix 10 Model fit statistics and residual deviance base case
For all analyses, 55,000 iterations were run on two parallel chains and the first 5000 iterations were discarded as ‘burn-in’. Convergence was assessed by visual inspection of the Brooks–Gelman–Rubin plots and assessment of the Ȓ statistic. 156,157
The model fit statistics for the base-case and the sensitivity analyses are shown in Table 35. The results show that all models fit the data well. The inspection of box plots of individual groups’ contributions to the residual deviance support this.
Prior distribution | Posterior mean of the residual deviancea | DIC |
---|---|---|
Base case: uniform (0, 5), 0.3 mean response probability | 11.6 | 30.7 |
Uniform (0, 5), 0.5 mean response probability | 11.9 | 30.9 |
Inverse gamma (2, 20) | 10.4 | 28.9 |
Half-normal (0,0.01)T(0,) | 10.9 | 30.5 |
Half-normal (0,0.1)T(0,) | 12.1 | 31.4 |
Half-normal (0,0.5)T(0,) | 14.8 | 33.1 |
Appendix 11 Bayesian hierarchical model sensitivity analyses
The sensitivity of the BHM results to the prior distribution were assessed. The results showed that the BHM estimated substantial heterogeneity between tumour types irrespective of the prior distribution or the response probability. This can be seen in the estimates of the posterior distributions for the between-group heterogeneity standard deviations in Table 36. The 95% CrIs around all of the results are wide, which indicates considerable uncertainty in these estimates.
Prior distribution | Posterior distributions for the between-group heterogeneity standard deviations (95% CrI) |
---|---|
Base-case: uniform (0,5), 0.3 mean response probability | 2.863 (0.922 to 4.826) |
Uniform (0, 5), 0.5 mean response probability | 2.828 (0.865 to 4.828) |
Inverse gamma (2, 20) | 3.273 (1.879 to 5.901) |
Half-normal (0,0.01)T(0,) | 3.738 (0.970 to 9.544) |
Half-normal (0,0.1)T(0,) | 2.740 (0.812 to 5.814) |
Half-normal (0,0.5)T(0,) | 1.820 (0.455 to 3.466) |
Appendix 12 Estimating the annual eligible population
Diagnostic accuracy illustration
The diagnostic accuracy of a test will vary depending on the prevalence of the genetic alteration within each tumour type, even when the sensitivity and specificity are held constant. This can be expressed by looking at the positive predictive value (PPV) and the negative predictive value (NPV) of a test. The PPV is defined as the likelihood that an individual with a positive test truly has the condition. Alternatively, the NPV is the likelihood that the individual with a negative test truly does not have the condition. The predictive value of a test will differ depending on the prevalence of a genetic alteration.
For example, the sensitivity and specificity of an IHC test for detecting NTRK fusions is 88% and 81%, respectively. If IHC was used to detect NTRK fusions in 2000 patients, 1000 with GIST (NTRK fusion prevalence 0.1%) and 1000 with papillary thyroid tumour (NTRK fusion prevalence 13.30%), the PPV and NPV of a test will differ. Table 37 demonstrates how the prevalence of NTRK fusion changes the PPV and NPV of a test.
Parameter | Papillary thyroid cancer | Gastrointestinal stromal tumour |
---|---|---|
NTRK fusion prevalence | 13% | 1% |
Total population with NTRK fusion | 130 | 10 |
True positives (test positive, NTRK positive) | 114 | 9 |
False positives (test positive, NTRK negative) | 165 | 1 |
True negatives (test negative, NTRK negative) | 705 | 871 |
False negatives (test negative, NTRK positive) | 165 | 119 |
Positive predictive value | 88% | 99% |
Negative predictive value | 7% | 98.9% |
Estimation of the eligible population
Table 38 details the sources used to estimate the annual eligible population.
Tumour type | NTRK fusion prevalence | Annual cancer incidence (England) | Proportion stage III/IV at diagnosis |
---|---|---|---|
Represented tumour types | |||
Appendix cancer | Amatu 2016178 | Based on an incidence of 0.97/100,000267 and the population in England in 2017268 | Marmor 2015267 |
Breast cancer (NOS) | NDA Multidisciplinary Review and Evaluation: Larotrectinib 177 | Cancer Registration Statistics 269 | Cancer Research UK. Breast Cancer Incidence by Stage at Diagnosis270 |
Cholangiocarcinoma | NDA Multidisciplinary Review and Evaluation: Larotrectinib 177 | Rare and Less Common Cancers 271 | Tsuchiya 2015272 (assumed to be the same as hepatocellular carcinoma) |
Colorectal cancer | NDA Multidisciplinary Review and Evaluation: Larotrectinib 177 | Cancer Registration Statistics269 and Thrumurthy 2016273 | Cancer Research UK. Bowel Cancer Incidence by Stage at Diagnosis274 |
GIST | NDA Multidisciplinary Review and Evaluation: Larotrectinib 177 | Starczewska Amelio 2014275 | PDQ Adult Treatment Editorial276 |
IFS | NDA Multidisciplinary Review and Evaluation: Larotrectinib 177 | Based on an incidence of 0.5/100,000177 and the population in England in 2017268 | Orbach 2009277 |
MASC | Skálová 2010278 | Cancer Registration Statistics269 and Luk 2015279 | Sethi 2014280 |
Melanoma | Okamura et al. 2018176 | Cancer Registration Statistics 269 | Cancer Research UK. Melanoma Skin Cancer Incidence by Stage at Diagnosis281 |
NSCLC (NOS) | NDA Multidisciplinary Review and Evaluation: Larotrectinib 177 | National Lung Cancer Audit 282 | National Lung Cancer Audit 282 |
Pancreatic cancer | Okamura et al. 2018176 | Cancer Registration Statistics269 and Pancreatic Cancer UK 2018283 | Cancer Research UK. Pancreatic Cancer Incidence by Stage at Diagnosis284 |
Soft tissue sarcoma | NDA Multidisciplinary Review and Evaluation: Larotrectinib 177 | Cancer Research UK. Soft Tissue Sarcoma Incidence Statistics285 | American Cancer Society 2017286 |
Thyroid | NDA Multidisciplinary Review and Evaluation: Larotrectinib 177 | Cancer Registration Statistics 269 | Deen 2016287 |
Unrepresented tumour types | |||
Cervical cancer | Okamura et al. 2018176 | Cancer Registration Statistics 269 | Cancer Reasearch UK. Cervical Cancer Incidence by Stage at Diagnosis288 |
Congenital mesoblastic nephroma | NDA Multidisciplinary Review and Evaluation: Larotrectinib 177 | Rare and Less Common Cancers271 and Gooskens 2017289 | Gooskens 2017289 |
Gastro–oesophageal junction adenocarcinoma | NDA Multidisciplinary Review and Evaluation: Larotrectinib 177 | NICE290 | Cancer Research UK. Oesophageal Cancer Incidence by Stage at Diagnosis291 |
Head and neck squamous cell carcinoma (NOS) | Okamura et al. 2018176 | Cancer Registration Statistics 269 | Cancer Research UK. Head and Neck Cancer Statistics292 |
High-grade glioma | NDA Multidisciplinary Review and Evaluation: Larotrectinib 177 | Public Health England293 | All high-grade glioma cancers are advanced or metastatic294 |
NET | Sigal 2018175 | UK and Ireland Neuroendocrine Tumour Society295 | UK and Ireland Neuroendocrine Tumour Society295 |
Ovarian cancer (NOS) | Assumption based on average prevalence (Solomon 2019180) | Cancer Registration Statistics 269 | Cancer Research UK. Ovarian Cancer Incidence by Stage at Diagnosis296 |
Paediatric high-grade glioma | Okamura et al. 2018176 | Farrimond 2010297 | Wang 2013294 |
Paediatric melanoma | Okamura et al. 2018176 | Cancer Research UK. Children’s Cancer Incidence Statistics298 | Austin 2013299 |
Papillary thyroid tumoura | NDA Multidisciplinary Review and Evaluation: Larotrectinib 177 | Cancer Registration Statistics269 and Brzeziańska 2006300 | Deen 2016287 |
Prostate cancer (NOS) | Assumption based on average prevalence (Solomon 2020180) | Cancer Registration Statistics 269 | Cancer Research UK. Prostate Cancer Incidence by Stage at Diagnosis301 |
Renal cell carcinoma | Assumption based on average prevalence (Solomon 2020180) | Cancer Registration Statistics269 and Cancer Research UK. Kidney Cancer: Stages, Types and Grades302 | Cancer Research UK. Kidney Cancer: Stages, Types and Grades302 |
Salivary gland (non MASC) | NDA Multidisciplinary Review and Evaluation: Larotrectinib 177 | Cancer Registration Statistics 269 | Assumed to be the same as head and neck squamous cell carcinoma292 |
Secretory breast carcinoma | NDA Multidisciplinary Review and Evaluation: Larotrectinib 177 | Cancer Registration Statistics269 and Horowitz 2012303 | Jacob 2016304 |
Sinonasal adenocarcinoma | Assumption based on average prevalence (Solomon 2020180) | Cancer Registration Statistics269 and Rushton 2010305 | Assumed to be the same as head and neck squamous cell carcinoma292 |
Uterine carcinoma | NDA Multidisciplinary Review and Evaluation: Larotrectinib 177 | Cancer Registration Statistics 269 | Cancer Research UK. Uterine cancer incidence by stage at diagnosis306 |
The prevalence of NTRK fusions for each tumour type was determined from a pragmatic review of the literature. For the majority of tumour types, the prevalence of NTRK fusions was acquired from Foundation Medicine (Cambridge, MA, USA) data in the FDA review for larotrectinib, which assessed 34,476 tumour samples. 177 The frequencies of NTRK fusions for the remaining tumour types were taken from published evidence. 175,176,178 Despite NTRK fusions having been identified in renal cell carcinoma,307 ovarian cancer307 and prostate cancer,308 the exact frequencies are not recorded. Therefore, it was assumed that the NTRK fusion frequency in these tumours was the same as the average frequency of NTRK fusions across all tumours, which is estimated to be 0.26%. 180
In England, the annual incidences of tumours in anatomical sites (e.g. pancreatic cancer) were obtained from the Cancer Registration Statistics269 and Rare and Less Common Cancers: Incidence and Mortality in England271 databases. Where the NTRK fusion has been reported in a specific tumour type (e.g. pancreatic adenocarcinoma) rather than an anatomical site, we used an estimate of the proportion of patients with that cancer type, based on published evidence. The incidence estimates for NSCLC, NETs and soft tissue sarcoma were not available and were obtained from other published sources. 282,295,309 Given that there was no crude incidence available for appendiceal adenocarcinoma, the annual incidence was estimated using an incidence per 100,000 and the annual population within the UK in 2017. 267,268
The proportion of individuals diagnosed with stage III/IV cancer was used as a proxy measure for the proportion of the patients with locally advanced or metastatic cancer. Values for this were primarily obtained from Cancer Research UK. For the tumour types for which data were not available, values were taken from published sources. 267,272,277,280,282,287,289,299,307 For the tumour types for which a known proportion of the patient population had an unknown stage at diagnosis, the unidentified proportion of stage III/IV cancer at diagnosis was assumed to follow the same distribution as the known proportion.
Table 39 presents the calculations of the annual eligible population for each testing strategy.
Tumour type | Prevalence of NTRK fusion (%) | Cancer incidence (England) | Percentage with stage III/IV cancer | Annual TRK-inhibitor eligible population | ||
---|---|---|---|---|---|---|
Hierarchical | RNA-based NGS | Exhaustive | ||||
Tumours represented in the trial | ||||||
Appendix | 4.00 | 540 | 74 | 14.04 | 15.97 | 12.95 |
Breast | 0.07 | 46,102 | 15 | 4.25 | 4.84 | 3.93 |
Cholangiocarcinoma | 0.10 | 556 | 60 | 0.29 | 0.33 | 0.27 |
Colorectal | 0.12 | 34,825 | 55 | 20.20 | 22.98 | 18.64 |
GIST | 1.28 | 734 | 40 | 3.30 | 3.76 | 3.05 |
IFS | 90.90 | 59 | 51 | 24.04 | 27.35 | 22.18 |
MASC | 92.90 | 11 | 22 | 1.80 | 2.25 | 1.82 |
Melanoma | 0.21 | 13,740 | 10 | 2.28 | 2.60 | 2.11 |
NSCLC | 0.09 | 32,576 | 57 | 14.69 | 16.71 | 13.55 |
Pancreatic | 0.26 | 8388 | 78 | 14.95 | 17.01 | 13.80 |
Soft tissue sarcoma | 0.56 | 2740 | 32 | 4.32 | 4.91 | 3.98 |
Thyroid | 0.92 | 2195 | 31 | 5.50 | 6.26 | 5.08 |
Tumours not represented in the trial | ||||||
Cervix | 0.33 | 2591 | 24 | 1.80 | 2.05 | 1.66 |
Congenital mesoblastic nephroma | 60.70 | 2 | 17 | 0.20 | 0.23 | 0.18 |
Gastro–oesophageal junction | 0.10 | 7569 | 73 | 3.40 | 3.87 | 3.14 |
High-grade glioma | 0.05 | 2781 | 100 | 1.18 | 1.34 | 1.09 |
HNSCC | 0.38 | 9946 | 63 | 20.93 | 23.81 | 19.31 |
Neuroendocrine | 0.30 | 4363 | 53 | 6.10 | 6.94 | 5.63 |
Ovarian | 0.25 | 2724 | 55 | 3.29 | 3.75 | 3.04 |
Paediatric high-grade Glioma | 5.30 | 67 | 100 | 3.11 | 3.54 | 2.87 |
Paediatric melanoma | 11.11 | 56 | 34 | 1.83 | 2.08 | 1.68 |
Papillary thyroid tumour | 13.30 | 1057 | 31 | 38.30 | 43.57 | 35.34 |
Prostate | 0.25 | 41,201 | 43 | 38.93 | 44.29 | 35.92 |
Renal cell carcinoma | 0.25 | 7438 | 43 | 7.19 | 8.18 | 6.64 |
Salivary gland | 1.72 | 517 | 63 | 4.92 | 5.60 | 4.54 |
Secretory breast carcinoma | 91.70 | 7 | 9 | 0.46 | 0.58 | 0.47 |
Sinonasal adenocarcinoma | 0.25 | 5 | 63 | 0.01 | 0.01 | 0.01 |
Uterine | 0.10 | 7862 | 18 | 1.24 | 1.42 | 1.15 |
Modelled testing strategies
Tables 40–42 present the testing strategies that would be used to identify NTRK fusions for each tumour type across the three testing strategies. The appropriate test is dependent on NTRK fusion frequency and current testing availability.
Testing strategy | Costs | Tumour type | |
---|---|---|---|
FISH | No incremental costs |
|
|
WGS and confirmatory RNA-based NGS | Cost of confirmatory RNA-based NGS only |
|
|
IHC and RNA-based NGS | Total cost of IHC and RNA-based NGS |
|
|
Testing strategy | Costs | Tumour type | |
---|---|---|---|
First-line RNA-based NGS | Incremental costs of displacing FISH |
|
|
WGS and confirmatory RNA-based NGS | Cost of confirmatory RNA-based NGS only |
|
|
First-line RNA-based NGS | Total cost of RNA-based NGS |
|
|
Testing strategy | Costs | Tumour type | |
---|---|---|---|
DNA-based NGS and confirmatory RNA-based NGS | Incremental costs of displacing FISH |
|
|
WGS and confirmatory RNA-based NGS | Cost of confirmatory RNA-based NGS only |
|
|
DNA-based NGS and confirmatory RNA-based NGS | Total cost of DNA-based NGS and RNA-based NGS |
|
|
DNA-based NGS and confirmatory RNA-based NGS | Cost of confirmatory RNA-based NGS only |
|
|
Appendix 13 Case study: economic model
Survival
The distribution of patients in each health state is determined using observed PFS and OS. Traditionally, the observed TTE data for PFS and OS are utilised for both treatment arms and, depending on the maturity of the data, direct extrapolation is required. However, TTE data for PFS and OS were not available in the literature for either of the approved TRK inhibitors (larotrectinib and entrectinib).
The literature did report the median PFS and OS for both larotrectinib and entrectinib; however, there were significant differences between the median survival estimates of larotrectinib and entrectinib. The median PFS and OS was 28.3 months and 44.4 months, respectively, for patients in the larotrectinib study, and 11.2 months and 20.9 months, respectively, for patients in the entrectinib study. 310,311 Furthermore, the reported OS and PFS data were deemed highly uncertain owing to the significant data immaturity and uncertainty about the extent to which OS is driven by the efficacy of subsequent therapies.
Owing to these uncertainties, hypothetical estimates of PFS and OS were used in the economic model and can be seen in Table 43. Standard errors were assumed to be 10% of the mean.
Parameter | Median PFS (months) (95% CI) | Median OS (months) (95% CI) |
---|---|---|
Responders | 24 (21.6 to 26.4) | 36 (32.4 to 39.6) |
Non-responders | 6 (5.4 to 6.6) | 12 (10.8 to 13.2) |
It is assumed that the survival function of responders and non-responders follows an exponential distribution. Exponential parametric survival curves were, therefore, generated based on median OS and PFS values.
The resulting OS and PFS curves for responders and non-responders can be seen in Figure 16.
FIGURE 16.
Plots showing the stylised (a) progression-free survival and (b) the OS curves for responders and non-responders.
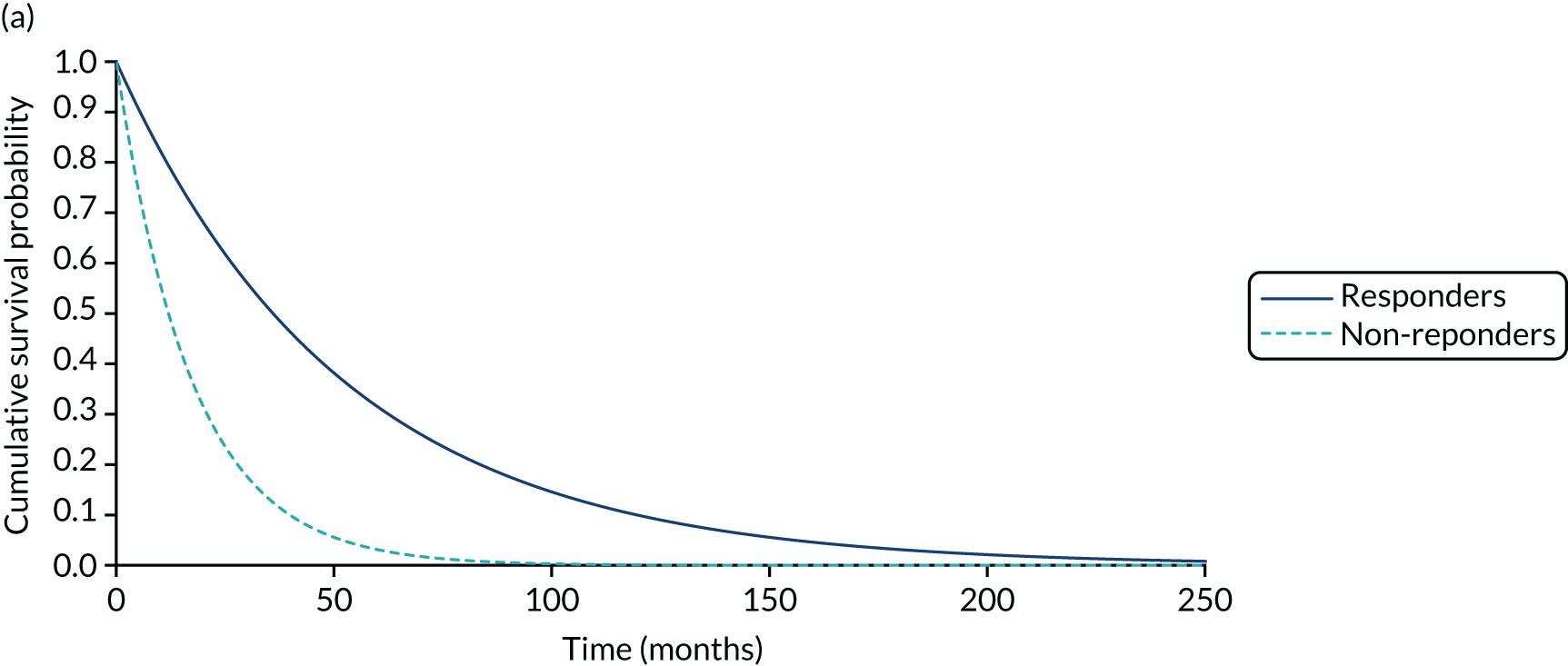
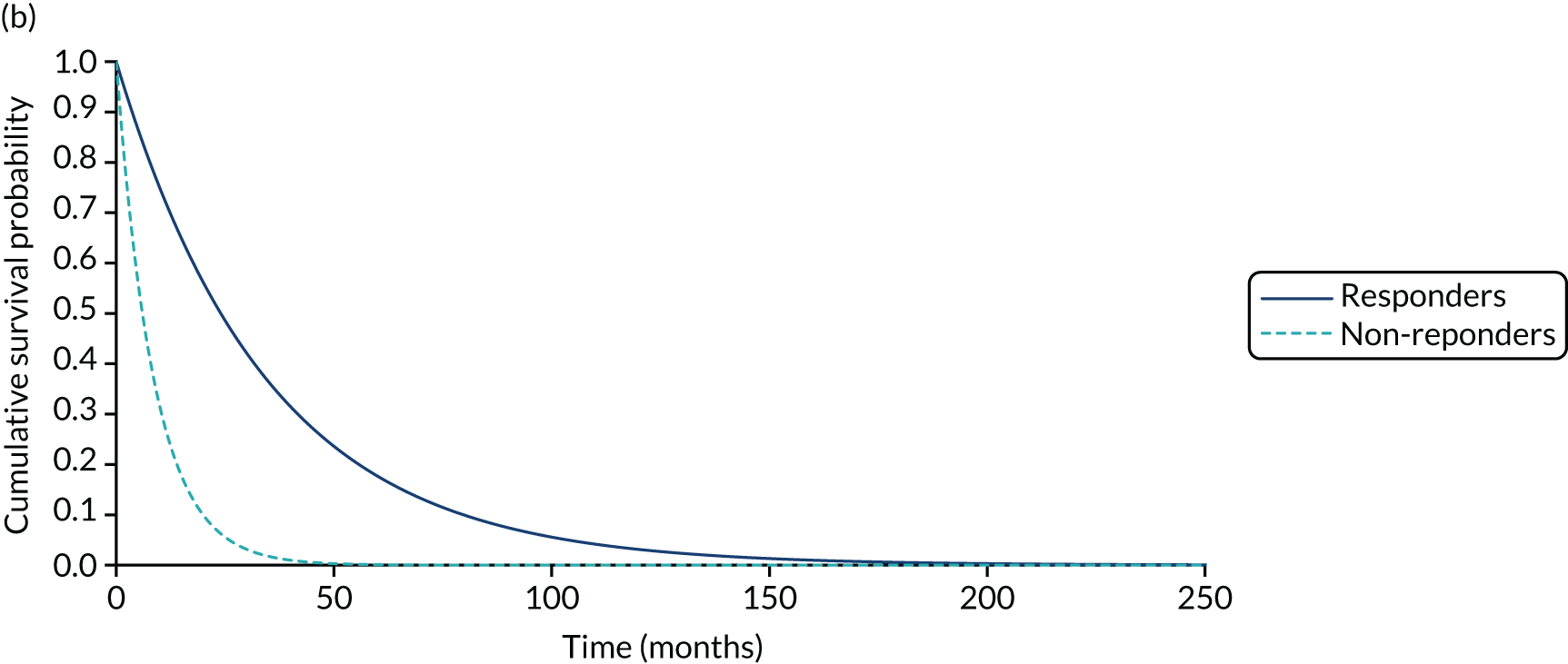
Utilities
Stylised health state utilities were used in the economic model and can be seen in Table 44. The utility values used for progression-free disease for Drug X and progressed disease were based on the mean values reported in the NICE TA of brigatinib for the treatment of patients with ALK-positive advanced NSCLC previously treated with crizotinib. 74,105,260 Given the cytotoxic nature of chemotherapy and the targeted nature of Drug X, the utility value of SoC was assumed to be lower than that of Drug X. As a result, a utility value of 0.72 was used for SoC. This value was based on the utility reported in the NICE TA of crizotinib for treating ROS1-positive advanced NSCLC. 255 The progressed disease health state utilities for Drug X and SoC were assumed to be equivalent because active treatment was assumed to be discontinued on disease progression.
It was assumed that the health state utilities were unchanged across tumour types. To reflect uncertainty in the utilities, standard errors were assumed to be 10% of the mean.
Parameter | Drug X (95% CI) | SoC (95% CI) |
---|---|---|
Progression-free disease | 0.79 (0.71 to 0.87) | 0.72 (0.65 to 0.79) |
Progressed disease | 0.64 (0.57 to 0.71) | 0.64 (0.57 to 0.71) |
Resource use and costs
In the absence of the acquisition cost of any currently available TRK inhibitors inclusive of pricing discounts for the NHS, it is assumed that the manufacturer of Drug X would employ a value-based approach to pricing. This assumes that the drug acquisition cost would be set at a level that results in a histology-independent ICER (inclusive of testing costs and weighted according to the eligible population) at approximately NICE’s decision threshold. For the purpose of the case study, Drug X is assumed to meet NICE’s end-of-life criteria, allowing a maximum willingness-to-pay threshold of £50,000 per QALY.
To ensure generalisability of the results, the preferred approach to generate a weighted average is to use the eligible population rather than the trial population. This should also include the unrepresented tumour types. The threshold analysis used to generate the value-based price of Drug X is conducted using the eligible population, including the unrepresented tumour types. The acquisition cost of SoC is assumed to be £20 per month.
For simplicity, it is assumed that there are no costs associated with administering Drug X or SoC and that there are no adverse event costs. It is also assumed that patients discontinue Drug X and SoC on disease progression.
Health state costs were assumed to be £350 per month per patient in the progression-free disease state and £500 per month per patient in the progressed disease health state. These values were informed by the health state costs reported in the NICE TAs of brigatinib and crizotinib. 255,260
To reflect uncertainty in the health state costs, standard errors were assumed to be 10% of the mean.
A one-off terminal care cost of £6878 is applied on transition from the progressed disease state to the death state. The terminal care cost was obtained from Georghiou and Bardsley. 312
The cost parameters used in the economic model can be seen in Table 45.
Parameter | Costs (£) (95% CI) |
---|---|
Drug acquisition costs | |
Drug X | 1250 |
SoC | 20 |
Health state costs | |
PFS | 350 (315 to 385) |
PPS | 500 (450 to 550) |
End of life | 6878 |
To assess the uncertainty surrounding the variables included in the cost-effectiveness model, a PSA was undertaken using 10,000 samples. All results reported are the mean averages of the 10,000 iterations.
Testing costs for unrepresented tumours
Table 46 provides a summary of the NNS, the annual eligible population and the testing costs for the tumours unrepresented in the trial. This illustrative example assumes that the testing costs equal £50 with a 100% sensitivity and specificity. The average cost to identify one individual eligible for treatment is estimated to be £14,322.
Tumour type | Annual eligible population | NNS | Cost to identify one patient eligible for NTRK treatment (£) |
---|---|---|---|
Cervix | 2 | 303.0 | 15,152 |
Congenital mesoblastic nephroma | 0 | 2.0 | 102 |
Gastro–oesophageal junction | 4 | 1000.0 | 50,000 |
Head and neck squamous cell carcinoma | 24 | 263.2 | 13,158 |
High-grade glioma | 1 | 2000.0 | 100,000 |
Neuroendocrine | 7 | 333.3 | 16,667 |
Ovarian | 4 | 400.0 | 20,000 |
Papillary thyroid tumour | 3 | 23.3 | 1163 |
Paediatric high-grade glioma | 2 | 9.0 | 450 |
Paediatric melanoma | 44 | 461.4 | 23,070 |
Prostate cancer (NOS) | 8 | 400.0 | 20,000 |
Renal cell carcinoma | 6 | 58.1 | 2907 |
Salivary gland | 1 | 1.1 | 55 |
Secretory breast carcinoma | 0 | 400.0 | 20,000 |
Sinonasal adenocarcinoma | 44 | 7.5 | 376 |
Uterine | 1 | 1000.0 | 50,000 |
Total | 151 |
List of abbreviations
- ALK
- anaplastic lymphoma kinase
- BHM
- Bayesian hierarchical model
- BICR
- blinded independent central radiologist
- BRMA
- bivariate random effects meta-analysis
- BSES
- Biomarker-Surrogate Evaluation Schema
- CDF
- Cancer Drugs Fund
- CHF
- congestive heart failure
- CHMP
- Committee for Medicinal Products for Human Use
- CI
- confidence interval
- CNS
- central nervous system
- CR
- complete response
- CRC
- colorectal cancer
- CrI
- credible interval
- ctDNA
- circulating tumour DNA
- dMMR
- deficient mismatch repair
- DoR
- duration of response
- DSU
- Decision Support Unit
- ECOG
- Eastern Cooperative Oncology Group
- EGFR
- epidermal growth factor receptor
- EMA
- European Medicines Agency
- ePAS
- extended patient analysis set
- ERG
- Evidence Review Group
- ESMO
- European Society for Medical Oncology
- EVPPI
- expected value of partial perfect information
- FDA
- Food and Drug Administration
- FISH
- fluorescence in situ hybridisation
- GI
- gastrointestinal
- GIST
- gastrointestinal stromal tumour
- GMI
- growth modulation index
- HER2
- human epidermal growth factor receptor 2
- HR
- hazard ratio
- HRQoL
- health-related quality of life
- HTA
- Health Technology Assessment
- ICER
- incremental cost-effectiveness ratio
- IFS
- infantile fibrosarcoma
- IHC
- immunohistochemistry
- IPD
- individual patient data
- IQR
- interquartile range
- IQWiG
- Institute for Quality and Efficiency in Health Care
- IRC
- Independent Review Committee
- ITT
- intention to treat
- KRAS
- Kirsten rat sarcoma
- LYG
- life-years gained
- MAA
- managed access agreement
- MAIC
- matching-adjusted indirect comparison
- MASC
- mammary analogue secretory carcinoma
- MCC
- Merkelcell carcinoma
- MSI-H
- microsatellite instability high
- NE
- not estimable
- NET
- neuroendocrine tumour
- NGS
- next-generation sequencing
- NHB
- net health benefit
- NHL
- non-Hodgkin’s lymphoma
- NICE
- National Institute for Health and Care Excellence
- NMB
- net monetary benefit
- NNS
- number needed to screen
- NPV
- negative predictive value
- NSCLC
- non-small cell lung cancer
- NTRK
- neurotrophic tyrosine receptor kinase
- OR
- odds ratio
- ORR
- overall response rate
- OS
- overall survival
- PAS
- primary analysis set
- PCR
- polymerase chain reaction
- PD
- progressive disease
- PFS
- progression-free survival
- PPS
- post-progression survival
- PPV
- positive predictive value
- PR
- partial response
- PSA
- probabilistic sensitivity analysis
- PSM
- partitioned survival model
- QALY
- quality-adjusted life-year
- RCP
- Royal College of Pathologists
- RCT
- randomised controlled trial
- RECIST
- Response Evaluation Criteria in Solid Tumours
- RR
- relative risk
- RRcHL
- relapsed or refractory classical Hodgkin’s lymphoma
- RT-PCR
- reverse transcription polymerase chain reaction
- SAG
- Scientific Advisory Group
- SAP
- statistical analysis plan
- SCLC
- small cell lung cancer
- SmPC
- summary of product characteristics
- SoC
- standard of care
- STC
- Simulated Treatment Comparison
- STE
- surrogate threshold effect
- TA
- technology appraisal
- TKI
- tyrosine kinase inhibitor
- TRK
- tyrosine kinase
- TSD
- technical support document
- TTE
- time to event
- TTP
- time to progression
- TTR
- time to response
- VGPR
- very good partial response
- VoH
- value of heterogeneity
- VOI
- value of information
- WGS
- whole genome sequencing