Notes
Article history
The research reported in this issue of the journal was funded by the PHR programme as project number 11/3020/05. The contractual start date was in May 2013. The final report began editorial review in November 2016 and was accepted for publication in June 2017. The authors have been wholly responsible for all data collection, analysis and interpretation, and for writing up their work. The PHR editors and production house have tried to ensure the accuracy of the authors’ report and would like to thank the reviewers for their constructive comments on the final report document. However, they do not accept liability for damages or losses arising from material published in this report.
Declared competing interests of authors
none
Permissions
Copyright statement
© Queen’s Printer and Controller of HMSO 2018. This work was produced by Poortinga et al. under the terms of a commissioning contract issued by the Secretary of State for Health and Social Care. This issue may be freely reproduced for the purposes of private research and study and extracts (or indeed, the full report) may be included in professional journals provided that suitable acknowledgement is made and the reproduction is not associated with any form of advertising. Applications for commercial reproduction should be addressed to: NIHR Journals Library, National Institute for Health Research, Evaluation, Trialsand Studies Coordinating Centre, Alpha House, University of Southampton Science Park, Southampton SO16 7NS, UK.
2018 Queen’s Printer and Controller of HMSO
Chapter 1 Background and aims
Introduction
It has long been recognised that poor housing conditions can have a detrimental impact on health. 1 Early evidence, dating back to the late nineteenth century, suggested a link between cold and damp housing and poor physical health. 2 At around that same time, cold and damp living conditions were also linked to poor mental health. 3 However, it has only been within the past 10–20 years that empirical studies have drawn strong links between housing quality and health. 4
The recent literature on housing and health has shown that low indoor air temperatures are associated with increased risk of stroke, heart attack and respiratory illness in temperate climates, as well as with common mental disorders. 5 Countries with the lowest thermal efficiency standards have the highest levels of excess winter mortality and people living in the least energy-efficient housing are at higher risk of death than those living in more energy-efficient homes. 6–8 Excess winter deaths are almost three times as high in cold homes as in warm homes. 6,8 The World Health Organization (WHO) estimates that 40% of excess winter deaths are caused by living in a cold home. 9
Excess winter mortality is thought to be linked predominantly to circulatory and respiratory conditions. 6,10–12 Prolonged exposure to low temperatures can have an impact on blood pressure and blood chemistry13–15 as well as blood viscosity and vasoconstriction. 16,17 Low temperatures can also suppress the function of the immune system,18 raising the risk of respiratory infection among vulnerable groups. 10 Living in low temperatures has been linked to a higher prevalence of respiratory infections and hospital admissions among the elderly,19–21 as well as to increased severity and frequency of asthmatic symptoms in children. 4 Low indoor temperatures can also encourage the development of damp and mould in the home,22 which are known risk factors for respiratory disease in adults23–26 and children. 22,27
In addition to links with cardiorespiratory conditions and excess winter mortality, living in cold and damp homes has been associated with negative impacts on mental health. 6,28,29 Living in cold and damp homes may be stressful for a number of reasons, including stigmatisation, financial worries, a lack of control over the living environment and prolonged thermal discomfort. 6,30–33 Harris et al. 29 found that people with common mental disorders are also more likely to experience different aspects of fuel-related poverty; living in a cold home, the presence of mould and using less fuel than needed because of worries about costs were all found to be associated with common mental disorders, even after adjusting for financial and socioeconomic factors. Living in a cold home may also have an impact on social interactions:31 it may make people reluctant to invite others into their homes and it may prevent them from going out as a result of financial concerns and fears of returning to a cold home. 4,6,34 Social isolation can increase the risk of depression and coronary heart disease, thereby having the potential to exacerbate the negative effects of living in a cold home. 35
Those affected by fuel poverty often adopt various strategies to deal with their financial circumstances. 36 Households may reduce fuel usage through rationing, or self-disconnect where pre-payment meters are present. 37–41 Studies have found that fuel-poor households reduce lighting usage or limit their consumption of hot water. 42–44 Others may trade warmth for other essentials, such as food. 34,45,46 This phenomenon has become known as the ‘heat-or-eat’ dilemma. 45,46 When heating is prioritised, disposable income and food choices become restricted, affecting both the quantity and quality of foods purchased and consumed. 34,36 Households may also choose not to adopt coping strategies and instead continue their normal spending patterns, which can lead to arrears in fuel payments and the accumulation of debt. 47
Health impacts of energy efficiency improvements
It is clear that housing quality is associated with a range of health and psychosocial outcomes. However, most evidence is derived from cross-sectional research. This is problematic, as those living in poor housing are most likely to be socioeconomically deprived and have long-standing illness. The causal pathways between poor housing and poor health can therefore be determined only by intervention studies. In a systematic review of the literature, Thomson et al. 48 identified 39 housing intervention studies that reported quantitative or qualitative data on health and associated socioeconomic outcomes. Of these, 19 evaluated affordable warmth and energy efficiency improvements relevant to modern-day housing conditions (i.e. post 1985), including five randomised controlled trials, five controlled before-and-after studies and five uncontrolled or retrospective studies. Thomson et al. 48 concluded that affordable warmth and energy efficiency measures may produce improvements in general health, respiratory health and mental health, but that interventions targeting at-risk populations (i.e. those with inadequate warmth and pre-existing conditions) are more likely to be successful than general, area-based, programmes.
Two randomised controlled studies conducted in New Zealand by Howden-Chapman et al. 49,50 found that improving energy efficiency through insulation improved respiratory health in both children and adults, and that improved non-polluting heating systems reduced symptoms of pre-existing asthma in children. In the case of asthmatic children, benefits could be linked to the rise in temperature in both the living room and the child’s bedroom as well as lower levels of nitrogen dioxide. 50 In a randomised trial, Barton et al. 51 found substantial reductions in non-asthma-related chest problems and the number of reported asthma symptoms among the intervention group for both adults and children.
Energy efficiency measures also appear to be beneficial for mental health, although studies have shown mixed results. 48 Howden-Chapman et al. 49 found significant improvements in three subscales of the Short Form questionnaire-36 items (SF-36), namely in the ‘happiness’, ‘vitality’ and ‘role emotional’ scales. However, the authors did not report the overall results for the Mental Health Composite Scale (MCS). Barton et al. 51 found no significant differences between the intervention and control groups regarding the mental health subscales of either the SF-36 or the General Health Questionnaire-12 items. Braubach et al. 52 similarly found non-significant differences in self-reported depression between the intervention and control groups. The authors noted that the follow-up questionnaire was distributed within a few months of the intervention, and that detailed analysis of the data had not been undertaken in order to better understand the impacts of both time and socioeconomic variables such as age. Shortt and Rugkåsa53 reported that stress and mental illness increased significantly in the control group but showed a non-significant decrease in the intervention group.
Psychosocial impacts of energy efficiency improvements
Studies examining the impacts of energy efficiency improvement programmes have thus far focused primarily on a limited number of health outcomes. Few large-scale controlled studies have been undertaken to understand the wider psychosocial impacts of such programmes. 48 The predominantly qualitative literature suggests two inter-related pathways that may link energy efficiency investments to better mental and physical health. 54–56 The first pathway is the process in which energy efficiency improvements to homes lead to better thermal living conditions, through improved indoor air temperature and decreased humidity, both of which contribute to reduced damp-related housing problems. 57 Warmer, drier homes can contribute to improved respiratory health, and also better mental health through improved thermal satisfaction,58 expanded living space and reduced social interactions. 59 The second pathway is the process in which energy efficiency measures lead to improved well-being by making heating more affordable. 6 Reduced spending on heating bills alleviates financial stress and fuel poverty among low-income households,60,61 and helps to free financial resources for better food security45,46 and reduced social isolation. 31 Social isolation may reduce further because people may become less reluctant to invite people into their homes with improved internal conditions. 62
Energy efficiency improvements and internal conditions
The evidence of the impact of energy efficiency improvements on indoor conditions is limited. Overall, based on available studies that have been conducted so far, energy efficiency measures produce modest increases in mean indoor air temperatures and small increases in mean humidity levels. 48 The Warm Front study group reported mean living room temperature increases of 0.58 °C for insulation and 1.36 °C for heating measures, and mean bedroom temperature changes of 1.14 °C for insulation and 1.98 °C for heating measures, with greater changes for dwellings that received both heating and insulation measures (1.52 °C and 2.31 °C for living room and bedroom, respectively). 63 Howden-Chapman et al. 49 reported an average increase in bedroom temperature of 0.50 °C and an average decrease in humidity level of 2.3% relative humidity (RH). The Glasgow Warm Homes Study60 reported increases in mean temperatures of > 2 °C in the living room and almost 3 °C in the bedroom, but no significant changes in RH levels.
More recently, there have been suggestions that increasing the energy efficiency of a home could have detrimental effects on people’s health. 64 Reduced ventilation through insulation and draughtproofing may increase RH levels65 and, as a result, promote mould growth. 66,67 Indeed, low ventilation rates and homes with greater energy efficiency are associated with asthma and allergic symptoms in children. 68–70
The evidence on energy efficiency investments and indoor conditions, however, is scant. Most research in the area has been cross-sectional71 and has not included control households,72 spot measurements8 or short-term monitoring. 60 As previously observed by Oreszczyn et al. ,68 most studies did not correct for external conditions during the monitoring periods. Evidence for increases in RH levels has been anecdotal or inferred from cross-sectional studies only. Raising indoor air temperatures through better insulation should reduce RH levels unless there is inadequate ventilation. 64 Overall, based on the available studies that have been conducted to date, it appears that energy efficiency investments produce modest increases in mean indoor air temperatures and small increases in mean humidity levels. 48
Conclusion
The literature review presented here shows that housing improvements that increase the energy efficiency of homes have the potential to improve the health of residents, in particular if they are targeting at-risk populations with inadequate warmth and pre-existing conditions. 48 However, most research involves observational studies using self-completion questionnaires. Field studies that rely on survey methodologies are vulnerable to biases from low response rates, attrition and self-reporting. Anonymous data linkage of routinely collected health data, which is less vulnerable to such biases, has not, to our knowledge, been used before to examine the impact of energy efficiency improvements (see Chapter 2). 73 In addition, less is known about the wider psychosocial impact of energy efficiency improvements. There is a distinct lack of good-quality quantitative evidence regarding the pathways and processes that may contribute to better health in the longer term (see Chapter 3). 54 Moreover, only a limited number of household monitoring studies have been conducted to examine the impacts of energy efficiency improvements on indoor hydrothermal conditions. Research in this area has been marred by a number of methodological and analytical issues and has relied on studies with relatively small sample sizes (see Chapter 4). Finally, economic evaluations have rarely been carried out alongside housing improvement programmes to assess their health-related quality of life and economic impacts. 74 Health economic evaluations are essential to inform policy-makers about the value for money of energy efficiency investments (see Chapter 5).
Aims of the project
In this study, we make use of a major energy performance investment programme (referred to hereafter as ‘the intervention’) that took place in Wales in two separate phases between 2010 and 2015. Arbed is, along with Nest, part of the Welsh Government Warm Homes programme. It was established to bring environmental, social and economic benefits to Wales through co-ordinated investments in the energy performance of homes located in low-income areas (see The intervention). Our project aimed to examine whether or not the investments provided additional benefits in terms of improved health of residents. More specifically, the project aimed to determine the impact of the intervention on (1) hospital admissions for cardiorespiratory conditions, (2) the prevalence of self-reported respiratory symptoms and mental health status of residents, (3) internal hydrothermal conditions and household energy use and (4) psychosocial outcomes that may be part of the pathways to health. It also aimed to (5) estimate the costs and consequences of the energy performance investments to the health system and (6) undertake a cost–utility analysis (CUA) of these investments.
The intervention
The Welsh Government tries to address fuel poverty through demand-led and area-based elements. 75 The Welsh Government Warm Homes programme provides funding for energy efficiency improvements to low income households on demand (Nest) and to those living in deprived communities across Wales (Arbed). The research reported here focuses on the area-based Arbed (meaning ‘save’ in Welsh) programme.
The Arbed energy performance investment programme was set up in 2009 by the Welsh Government to bring environmental, social and economic benefits to Wales. The programme was designed to improve the energy efficiency of existing homes in low-income areas and aimed to (1) reduce the number of households living in fuel poverty, (2) create jobs and regeneration in Wales and (3) combat climate change by reducing greenhouse gas emissions. The programme consisted of two phases. The first phase (Arbed 1) took place in 2010 and 2011. The second phase (Arbed 2) took place between 2012 and 2015.
Both phases of the intervention programme were Wales-wide, with work prioritised within the Welsh Government’s seven Strategic Regeneration Areas of the Heads of the Valleys, Môn a Menai, North Wales Coast, Western Valleys, Swansea, Aberystwyth and Barry. Many of these schemes were in old slate- and coal-mining areas and in a number of deprived urban areas.
The first phase of the intervention programme focused specifically on energy efficiency improvements to homes in low-income areas as identified by social housing providers. A total of £36.6M was invested by the Welsh Government, with an additional £32M leveraged by energy suppliers, housing associations and local councils. 76 This phase consisted of 28 schemes across 18 local authorities (LAs) in Wales, and helped to improve the energy performance of around 7500 homes. It mainly improved social housing (79%), but also a number of privately rented and owner-occupied houses (21%). The programme funded retrofit measures such as solid wall insulation (> 4000 homes), solar panels (> 1800 homes), solar hot water (> 1080 homes) and heat pumps (> 100 homes). Leveraged funds from energy providers and social housing providers were used to enable homes to receive additional measures, such as boiler upgrades and replacements, window upgrades, roof extensions, structural work and energy saving advice. 77
The second phase of the intervention programme was co-funded by the Welsh Government and the European Regional Development Fund (ERDF), and had an overall budget of £45M. This comprised £33M of ERDF funding and a further £12M from the Welsh Government. The second phase consisted of 32 schemes and helped to improve the energy performance of > 4800 homes. In contrast to the first phase, which primarily focused on social housing, the second phase of the intervention programme specifically targeted mixed-tenure, low-income neighbourhoods that had a high number of hard-to-heat, hard-to-treat homes, and when, as a result, people were at a higher risk of living in fuel poverty. ‘Hard-to-heat, hard-to-treat’ is a term used for low-energy-performance dwellings that, for whatever reason, cannot accommodate staple or cost-effective fabric energy efficiency measures. 78 Typical energy efficiency measures included external wall insulation, heating system upgrades (including gas combination boilers, heating controls and central heating systems), voltage optimisers and connecting communities to the gas mains network.
Both phases of the intervention programme aimed to boost local economies by using local businesses to manufacture, supply and install as many of the measures as possible and provide training and employment opportunities for local workers through a tendering process to recruit contractors and subcontractors. 77 There were, however, a number of differences in the delivery of the programme between the two phases.
The first phase of the programme was managed by LAs and social housing providers themselves, and delivered through contractors and subcontractors. The LAs and social housing providers submitted proposed schemes to the Welsh Government, which decided which were to be funded. The second phase of the programme was managed by two scheme managers: Melin Homes, a housing association, managed schemes in South Wales; and Wilmott Dixon, a private construction company, managed schemes in North and Mid Wales.
Local authorities were invited by the Welsh Government to propose schemes based on scoring criteria using proxies to identify areas at fuel poverty risk, and once a scheme was approved this was passed on to the two scheme managers for work to commence. The selected areas were visited by surveyors to determine the most appropriate and cost-effective measures for the schemes. Contractors were used to deliver the improvement work, overseen by the two scheme managers. The areas were subsequently visited by representatives and community events were set up to encourage households to sign up for the improvement programme.
For both phases, depending on the measures chosen for each home, residents would have been in contact with representatives of the scheme managers who recruited householders to the scheme, chose suitable measures for the property and allocated selected contractors and businesses to undertake the work. Each household would have been in contact with a variety of stakeholders from start to finish, including, but not exclusively, scheme managers, community engagement teams, project managers and contractors including building surveyors, scaffolders, insulation installers and central heating engineers. The process from initial contact with householders through community engagement to finishing the whole scheme could take up to a year or, in a few cases in which the work was delayed, longer.
A TIDieR (Template for Intervention Description and Replication) checklist is provided in Appendix 6.
The project
The project provided a multimethod investigation of the health impacts of energy performance investments in low-income neighbourhoods through four interlinked studies. The project comprised the use of the Secure Anonymised Information Linkage (SAIL) databank, a community-based field study, a household monitoring study and an economic evaluation. In addition, the project included a number of resident engagement activities.
The SAIL databank was used to retrospectively evaluate the first phase of the intervention programme. SAIL is an ethically approved tool, developed and hosted by Swansea University, that ensures that individuals remain anonymous and retain the ability to make links between different data sets. 79 Intervention records of the first phase of the programme were anonymously linked to individual health records to determine the impacts of the intervention on hospital admissions for cardiorespiratory conditions. Data generated in this work package were used in the economic evaluation to calculate the cost savings of the investments to the health system. Findings of the data linkage study are reported in Chapter 2.
A community-based study was set up to evaluate the second phase of the intervention programme. The field study aimed to determine the impact of the intervention on the prevalence of respiratory conditions and mental health status of residents as well as on psychosocial outcomes that may be part of pathways to health. The findings of the community-based study are reported in Chapter 3.
Chapter 4 presents the results from the household monitoring study. The household monitoring study was conducted to determine the impact of the intervention on internal hydrothermal conditions. It further provided evidence on whether or not the intervention resulted in lower household energy use and an expansion of living space. 61 Gas and electricity meter readings were taken during the installation and collection visits to determine the impacts of the programme on household energy use.
The fourth study, reported in Chapter 5, presents the results of an economic evaluation of the energy performance investments. Data from both the data linkage and community-based studies were used to determine whether or not the investments improved public health over and above the other intended benefits of the programme. A cost–consequences analysis (CCA) compared the costs of delivering the intervention to the cost savings as a result of reduced health service use. A CUA estimated the benefits of the programme as a result of improving the quality of life of residents of low-income neighbourhoods.
Resident engagement
A reconvened focus group study was conducted as part of the wider resident engagement of the research project. Three focus groups were held with residents before and after they received energy efficiency improvements in the second phase of the intervention programme. The aims of the focus groups were to obtain a better understanding of the views and experiences of residents living in energy-inefficient (hard-to-heat, hard-to-treat) houses, and the ways in which the programme may have changed those experiences. The protocol of the focus group discussions can be found in Appendix 1. The results of the reconvened focus groups are summarised in Appendix 2, with the consolidated criteria for reporting qualitative research (COREQ) checklist provided in Appendix 3. A number of focus group participants were subsequently invited to form a resident panel, which met on three occasions throughout the project and contributed to the biannual study steering committee (SSC) meetings. The resident panel provided advice on how to disseminate the findings at the end of the project. Findings of the community-based and household monitoring studies were disseminated through an easy-to-understand key findings brochure (see Appendix 4) and three community dissemination meetings. Appendix 5 shows the pull-up banner that was used in meetings.
Chapter 2 The data linkage study
Introduction
This chapter follows the STROBE (Strengthening the Reporting of Observational Studies in Epidemiology) reporting guidelines for observational study designs. 81
Background
This chapter describes the retrospective evaluation of the first phase of the intervention programme that ran in 2010 and 2011. The evaluation used anonymised, routinely collected health records held within the SAIL databank. 82,83 Intervention records of the first phase of the intervention programme were anonymously linked to individual health records to determine the impact of the intervention on hospital admissions for cardiorespiratory conditions and a number of secondary outcomes. The study used data on emergency hospital admissions for cardiovascular and respiratory conditions held in the Patient Episode Database for Wales (PEDW), which contains all hospital records for people treated at hospitals in Wales.
Governance approval (reference SAIL0273) for this research was received from the independent Information Governance Review Panel (IGRP) in March 2014. Membership of the IGRP consists of senior representatives from the British Medical Association, National Research Ethics Service, Public Health Wales, NHS Wales Informatics Service (NWIS) and the SAIL Consumer Panel.
Objectives
The overall aim of this study was to examine the long-term impact on health-care utilisation of energy efficiency investments. In particular, the study aimed to establish the change in the primary outcome of emergency hospital admissions for cardiovascular and respiratory conditions combined for people of all ages following the intervention.
The study had four secondary outcomes:
-
Change in emergency hospital admissions for cardiovascular conditions for people aged ≥ 60 years, and for people of all ages, following the intervention.
-
Change in emergency hospital admissions for respiratory conditions for people aged ≥ 60 years, and for people of all ages, following the intervention.
-
Change in emergency hospital admissions for chronic obstructive pulmonary disease (COPD) conditions for people aged ≥ 60 years, and for people of all ages, following the intervention.
-
Change in excess hospital admissions during the winter months for people aged ≥ 60 years, and for people of all ages, following the intervention.
Methods
Study design
The data linkage study consisted of a quasi-experimental analysis of residents living within homes having undergone energy efficiency improvements. The research utilised individual-level health data held within the SAIL databank anonymously linked with property-level intervention data provided by the housing scheme operators. Intervention and comparator groups were created through retrospective analysis of SAIL data from interventions carried out in 2010 and 2011. Health outcomes were obtained for the entire period that residents were present within the homes.
Setting
In the first phase of the intervention programme, a total of £68M was invested, including leveraged funding. The programme had the joint aims of delivering affordable warmth, alleviating fuel poverty, reducing CO2 (carbon dioxide) emissions and boosting economic development and regeneration in Wales. LAs and registered social landlords (RSLs) submitted project plans to improve the thermal efficiency of homes in Wales. The majority of homes were social homes. Work for 28 schemes was carried out in 2010 and 2011; it covered a wide geographical area across Wales and is referred to as the intervention study group (Figure 1).
FIGURE 1.
Locations (in blue) of (a) the top 10% of deprived areas; and (b) the intervention areas.
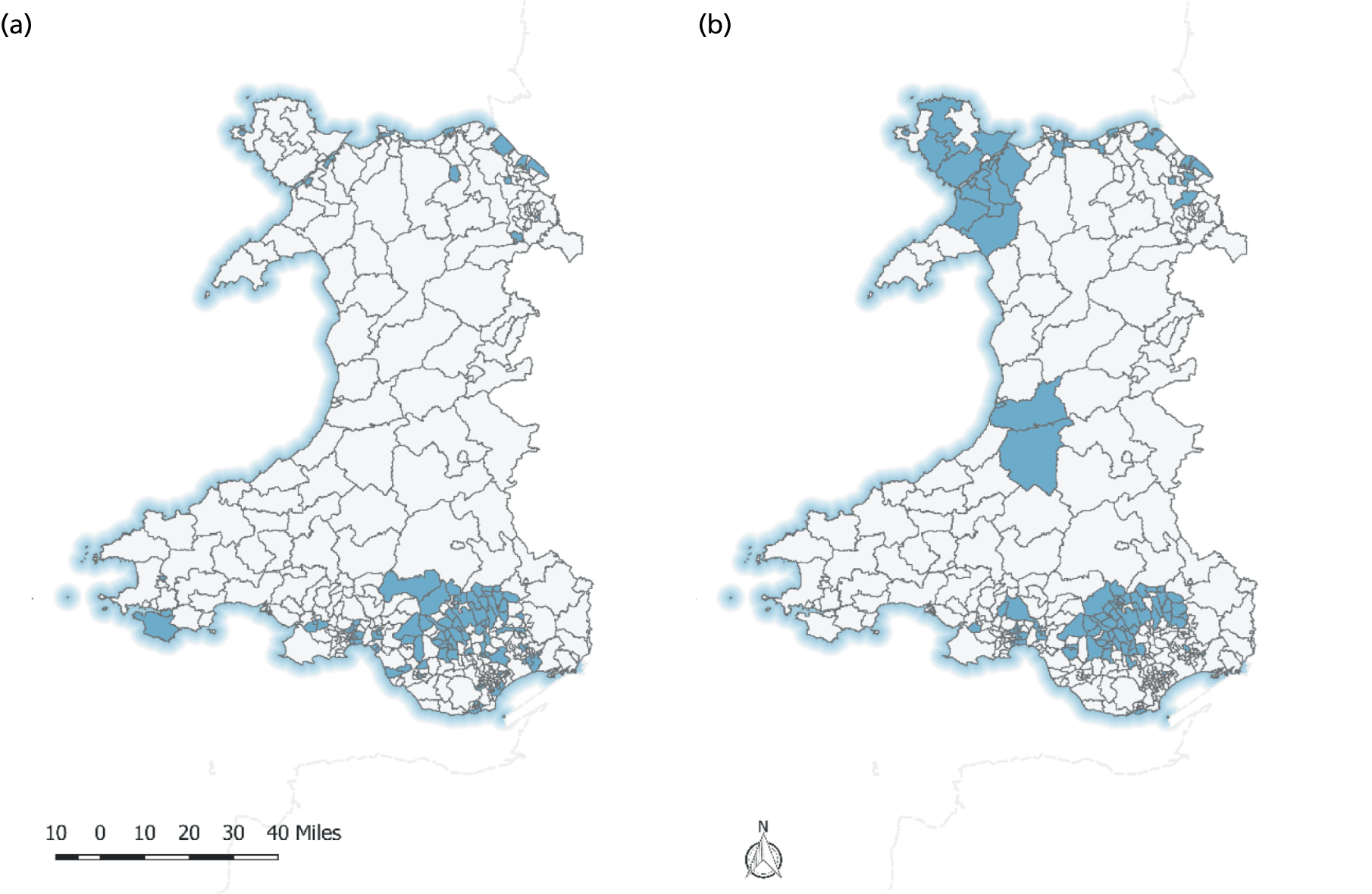
Participants
Intervention home addresses and measures were collated and imported into the SAIL databank through a split file method designed to maintain the anonymity of people living in the properties. 83 The first part of the split file containing addresses and unique property reference numbers was sent to a trusted third party, the NWISs, and replaced with a Residential Anonymous Linking Field (RALF). 79,84 The RALFs were securely transferred to the SAIL databank with a further level of encryption added by the SAIL technical team prior to being made available to researchers under controlled data access agreements. The second part of the split file contained intervention data with no identifiable data. This file was issued directly to SAIL and the two files were re-linked in the SAIL databank after removing the address data.
A similar split file method was followed to create anonymous (individual) linking fields (ALFs) for each person in the SAIL databank. 82 The first part of the split file containing identifiable data (including names and dates of birth) was issued to NWIS and replaced with the ALF prior to a further level of encryption added prior to researcher data access. Data relating to health-care utilisation and other administrative data, as supplied to SAIL in the second half of the split file process, was relinked in the SAIL databank, ensuring that anonymity was maintained.
The encrypted property-level (RALF) data is linked to individual-level (ALF) data in the Welsh Demographic Service (WDS) data set, which contains details of every person registered with a general practitioner (GP) in Wales along with their address histories and are based on addresses registered with a GP. We extracted people living within intervention properties according to our study inclusion criteria: a person needed to be resident in the property for ≥ 60 days during the study period (2005–14). People were retained in the study if they were living in the property during the intervention, moved out pre intervention or moved in post intervention. We used multilevel modelling methods that take into account unbalanced data; people did not need to be in the study for both the before and after phases.
Obtaining individual ALFs registered as occupants of the houses in our study allowed us to link to individual-level health data and mortality records without ever seeing personal, identifiable information or low-level geographical information.
The same method was applied for two groups of comparator homes. Social housing addresses from a Welsh LA were provided for the first comparator group. The second comparator group comprised all homes located in the top 10% of deprived lower super output areas (LSOAs) in Wales, based on the 2011 Welsh Index of Multiple Deprivation (WIMD) income domain.
Data sources
Address and intervention data were collected through contacting and requesting standard information from 28 LAs and RSLs. The supplied information was collated into a master data set by researchers at Cardiff University who had no access to SAIL data. This was important to maintain data privacy so the SAIL data analyst did not have access to both the identifiable address data and the anonymised version. The data were subsequently imported into SAIL through a split file method by our trusted third-party supplier, as described previously. The procedure ensured that the anonymity of homes and, thus, residents was maintained. Intervention dates were received in SAIL in a non-standard, non-codified manner. Data cleansing was carried out within the SAIL databank to reformat intervention dates and descriptions with a number of rules applied, described in Participants.
A Welsh LA provided all addresses of their social housing stock, which were transferred into SAIL to form the first comparator group. A second comparator group was created by selecting LSOAs forming the top 10% of deprived areas in Wales from the WIMD 2011 income domain, then linked to homes within the WDS containing addresses based on GP address registrations.
Homes from the three groups were linked to individual residence records across the study period within WDS, providing start and end dates of residence.
The WIMD 2011 was used to obtain income-related deprivation data at the LSOA level. The urban/rural classification was obtained using the Office for National Statistics (ONS) classification. Person-level demographic data, including age and sex, were obtained from the WDS data set. When relevant, the date of death was obtained by linking the ONS death registration data to the WDS data set. When a conflict existed, the ONS deaths data set was used as the primary data source.
Data sets were set up in a panel design containing one record for each person within a home for each monthly period in which they were present in the study. Study entry dates were defined as the earliest date selected for either the date of moving into the property or the start of the study period (January 2005). Study exit dates were defined as the latest date selected for the month of death, date of moving out of the home or the end of the study period (December 2014). Variables such as comorbidity, outcomes and age were updated to be reflective of the specific period within the study.
Variables
Records of emergency hospital admissions for relevant conditions from 2005 to 2014 were extracted from the PEDW data set, which contains records of all inpatient and day case episodes of care undertaken in NHS Wales plus data on Welsh residents treated in other UK nations (primarily England). NWIS provides SAIL with a de-identified version of PEDW via monthly electronic feeds.
We selected the first episode from each case of emergency admissions, representing continuous periods of inpatient care for a single patient, for diseases indicated by the selection of specific International Classification of Diseases, Tenth Edition (ICD-10)85 codes. Total admissions were counted for each month a person was included in the study. Our primary outcome was defined as any admission for cardiovascular (codes I00–I99) or respiratory (codes J00–J99) reasons. Episodes representing symptoms and signs involving circulatory and respiratory systems (R00–R09) in the first diagnostic position with no subsequent secondary diagnosis codes were also included in the primary outcome.
The secondary cardiovascular outcome consisted of records with ICD-10 codes I00 to I99, with the respiratory outcome identified by ICD-10 codes J00 to J99. Admissions that were attributable to COPD were selected using a combination of age and ICD-10 codes: any age for admissions with codes J40–J44, or R06 primary diagnostic position combined with J40–J49 any position, and codes J45–J46 for those aged ≥ 40 years (see Appendix 7).
Comorbidity was calculated using Bottle and Aylin’s86 algorithm, which was created using English National Health Service Hospital Episode Statistics data. We amended the algorithm, opting not to include ICD-10 code C44 (Non-Melanoma Skin Cancer) within the comorbidity algorithm to avoid potential bias as a result of differential treatment practices, which can be carried out in primary or secondary settings depending on geographical location in Wales.
Bias
We were supplied with address details for 5801 homes, from which we were able to geocode 4968 (86%). The 14% loss of property data was a result of a number of reasons, including incomplete or poor-quality address data, properties not present within WDS or properties with no residents registered within the study period. This loss of properties represents potential selection bias; however, as a result of the anonymised nature of SAIL and information governance, we were unable to explore this in more detail. The largest risk of selection bias arises from the exclusion of 509 properties that we were unable to link in the WDS. An analysis of non-matched properties showed that 70% were multiple-occupancy residences (blocks and flats), with the remaining 30% predominantly in terraced housing. From our experience, these linkage rates are typical of secondary address-level administration data. 87 This indicates a potential issue with source data and classification of properties at the National Land and Property Gazetteer.
Loss to follow-up bias is avoided because of the use of routinely collected data in SAIL. We were able to follow participants over a long period retrospectively, allocating health-care utilisation to individuals for the full period they were resident within an intervention home. Potential bias arising from background trends in emergency hospital admissions was explored by comparing changes in rates of emergency hospital admissions between study groups over a long period, taking into account pre-, mid- and post-intervention health-care utilisation. The trends were comparable and required no specific adjustment.
Study size
The process described in Participants generated our study data set, comprising 25,908 people living within the 4968 intervention homes over the 10-year study period, allowing for people moving in and out of homes. We had comparable data on two comparator groups. The first, a social housing comparator group, contained 48,261 people living within 12,350 homes; the second, drawn from the top 10% of deprived LSOAs in Wales, contained 524,596 people living in 118,982 homes.
Quantitative variables
Two date fields were received in relation to the intervention: start and end date. The quality and extent of these data were varied; some properties had both start and end dates recorded, whereas other properties had either start or end dates or no dates at all. We reviewed the data and imputed intervention dates based on the logic described in this section. We assumed that the month of the intervention end date represented the start of the ‘after’ period, all data prior to this month being allocated to the ‘before’ data collection period. Although we completed a validation exercise to ensure that we were using the correct addresses of intervention properties, there was no available method to validate dates or intervention measure data. We applied rules to allocate property dates by following these steps:
-
reversed dates when end date was prior to start date (n = 22)
-
calculated median days between start and end date for all homes with valid start and end dates (median = 92)
-
for homes with a valid end date only, subtracted the median days to impute a start date
-
for homes with a valid start date only, added the median days to impute an end date
-
calculated median start and end dates for all homes after applying steps 1–4
-
applied the static start and end dates from step 5 across all remaining homes.
Statistical methods
The methodological approach, adopted for the primary and secondary outcomes and the subgroup analyses, is based on initial investigations to assess the nature of any trends present in the observed data over the study period. This observed data is available on a monthly basis and, for both primary and secondary outcomes, comprises counts of events in that month. In the absence of any obvious trend over time, data on each individual’s residence is conflated into two values, comprising the number of events occurring before and after the intervention. To take account of the different time durations in the pre- and post-intervention phases, these aggregated counts were transformed into annualised rates. These annualised rates were summarised and compared using mixed multilevel linear models featuring an indicator variable for phase, adjusting for explanatory factors and covariates, and incorporating random effects to account for clustering.
This approach takes the basic unit for clustering purposes to be a person’s unbroken period of residence in a property, so data on an individual arising from a second residence is regarded as independent of data collected during that individual’s first residence. This applies even if the two residences are the same, that is, when there is a break in a person’s residence at a property. The pre- and post-intervention phases are defined using the assumption that the intervention is effective from its end date. We set the phase indicator to be 0 for pre intervention and 1 for post intervention, so that the coefficient for this variable corresponds to the effect of the intervention.
We included several individual-level covariates and factors in our analyses. Specifically, we considered covariates summarising participant age for each phase, calculated using the mid-point of residence in an intervention study home; a seasonality score for each phase; a single comorbidity score, ranging from 0 to 1, summarising comorbidities recorded across all months; and an indicator of sex (male, female).
We also considered area-based variables recording income deprivation (summarised by five ordered categories ranging from 1 = least deprived to 5 = most deprived) and a measure of rurality (1 = village and hamlet, 2 = town and fringe, 3 = urban), both obtained at LSOA level. 88
We defined sine-based factors for each month, taking negative values for October to March inclusive and positive values for April to September inclusive, resulting in an aggregate factor of zero for each complete year. Our seasonality covariate was based on seasonality scores for each phase, defined as the sum of monthly factors across each phase.
To assess excess winter admissions, we created a binary indicator for winter or non-winter, set to 1 for December, January, February and March, and to 0 for all other months. 89
Our modelling approach, aimed at obtaining the precise estimate of the intervention effect on each variable in turn, used appropriate univariate linear mixed models (implicitly assuming normality) and progressed by eliminating, in turn and starting with the least significant, all covariates and factors with a coefficient with a p-value of > 0.05, concluding when all remaining explanatory variables were statistically significant.
Results
Participants
Address records and intervention details for 5801 intervention homes were collated and supplied to SAIL for the intervention. There were 172 records with insufficient address data to allow data linkage. A further 99 records were excluded as a result of multiple source records matching to individual homes held within SAIL. A further 509 records were removed because there was no record of the home within the WDS data set. Finally, 53 records were removed when there were no residents recorded by the WDS as living in the home over the study period. This left 4968 properties for analyses, as shown in Figure 2. The supplied intervention addresses were validated against a separately sourced list of addresses from the Welsh Government, with 88% of addresses common to both sources. This is a conservative estimate because a number of addresses from both sources had insufficient address details to generate a linkable address. The number of individuals linked to these properties in the study period was 25,908.
FIGURE 2.
The flow diagram for the intervention group.
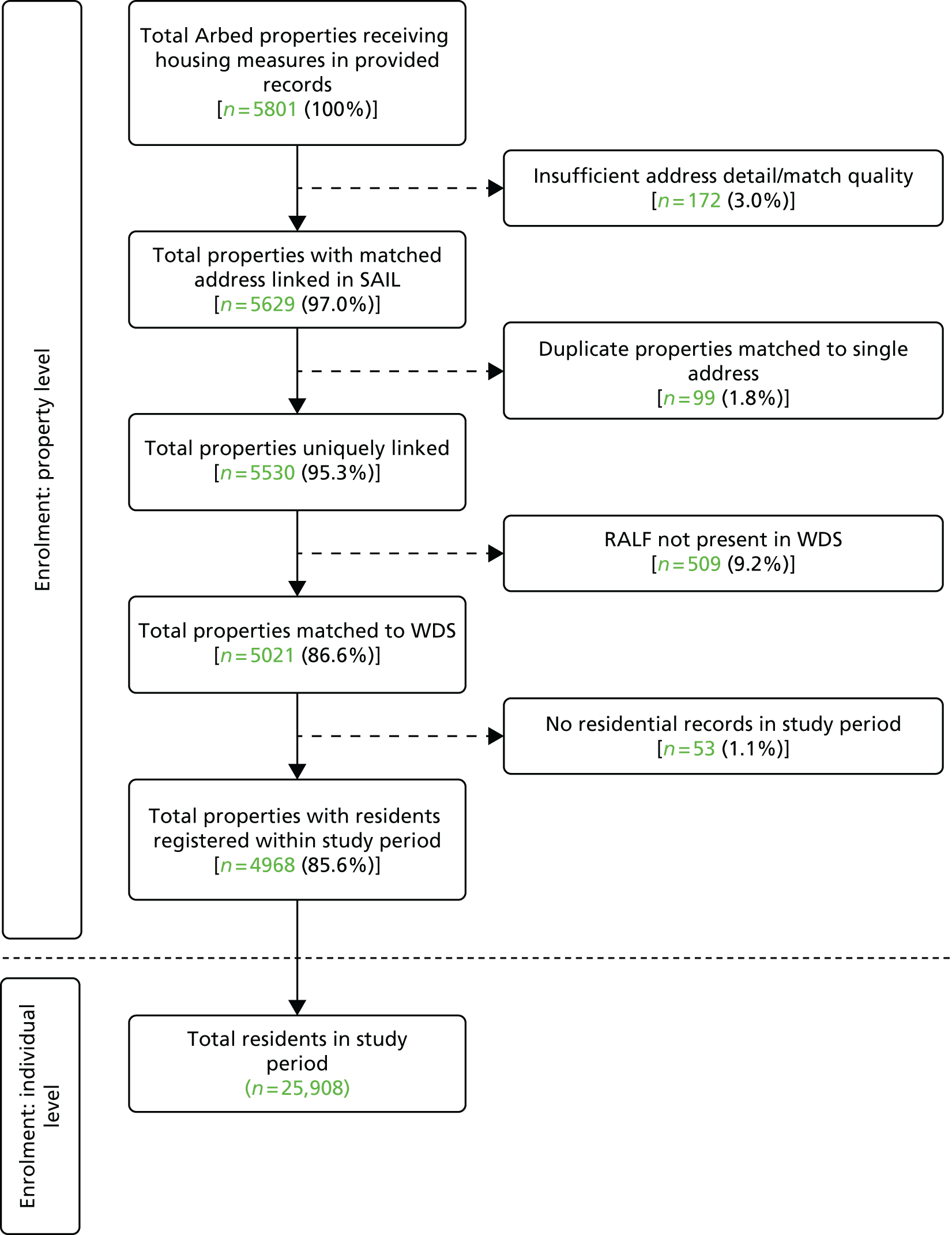
Descriptive data
Our final study size was 25,908 people living within 4968 intervention homes over the study period. We arrived at the study size through the process described in Methods, Participants and Results, Participants.
Properties
Just over 70% of intervention homes received one energy efficiency measure, around 20% received two measures and the remainder received three or more. The most common type of intervention measure carried out on the intervention homes was the installation of external wall insulation. Over 50% of homes received external wall insulation, 30% received photovoltaics, 20% received solar hot water, 15% switched fuels and 5% received an air source heat pump.
Individuals
We summarised the data by property and participant, because the interventions apply to properties but outcomes relate to residents. Each property can house multiple residents, who can be present simultaneously or consecutively, and each study participant can reside in multiple properties continuously or with breaks over the study period. We have demographic data for individuals living within 136,300 distinct study homes, of which 4968 are intervention homes, 12,350 are social housing comparator homes and 118,982 are comparator homes in areas designated in the top 10% of deprived areas by WIMD 2011.
There were 4968 properties that received the intervention (Table 1): for 2021 properties (40.7%), both the start and end dates for the intervention were known and for a further 1739 properties (35.0%), either the start or end date was known and the other was imputed. For the remaining 1208 properties (24.3%), both the start and end dates were imputed. Many people moved home during the intervention period. The extent of residential mobility is indicated by the association of several residents with multiple homes (Table 2).
End date | Start date | |||||||||
---|---|---|---|---|---|---|---|---|---|---|
2010 | 2011 | |||||||||
July | August | September | October | November | December | January | February | March | May | |
2010 | ||||||||||
August | 1 | |||||||||
September | 19 | |||||||||
October | 1 | 68 | ||||||||
November | 20 | 75 | ||||||||
December | 10 | 126 | 2 | |||||||
2011 | ||||||||||
January | 65 | 79 | 68 | 2 | ||||||
February | 83 | 100 | 86 | 4 | 9 | |||||
March | 76 | 257 | 50 | 318 | 116 | 92 | 74 | |||
April | 45 | 40 | ||||||||
May | 1 | |||||||||
June | ||||||||||
July | 8 | |||||||||
August | ||||||||||
September | 3 | |||||||||
October | ||||||||||
November | 80 | |||||||||
December | 2 | |||||||||
2012 | ||||||||||
January | 35 | |||||||||
February | ||||||||||
March | 2 | |||||||||
April | ||||||||||
May | ||||||||||
June | ||||||||||
July | ||||||||||
August | ||||||||||
September | 4 | |||||||||
Total | 19 | 21 | 323 | 793 | 138 | 390 | 167 | 92 | 74 | 4 |
Intervention only | Social housing only | Top 10% deprived areas only | Intervention and social housing | Intervention and top 10% deprived areas | Social housing and top 10% deprived areas | Intervention, social housing and top 10% deprived areas | Total | |
---|---|---|---|---|---|---|---|---|
Sexa | ||||||||
Male | 8972 | 19,188 | 255,021 | 331 | 2995 | 4058 | 131 | 290,696 |
Female | 9545 | 19,874 | 254,667 | 367 | 3410 | 4155 | 157 | 292,175 |
Residents associated with | ||||||||
1 home | 17,097 | 29,644 | 398,788 | 0 | 0 | 0 | 0 | 445,529 |
2 homes | 1265 | 7101 | 79,633 | 454 | 3736 | 4271 | 0 | 96,460 |
3 homes | 127 | 1723 | 21,439 | 183 | 1675 | 2335 | 112 | 27,594 |
4 homes | 25 | 444 | 6472 | 37 | 583 | 966 | 99 | 8626 |
5 homes | 2 | 110 | 2248 | 18 | 254 | 381 | 43 | 3056 |
6 homes | 1 | 29 | 756 | 5 | 98 | 170 | 19 | 1078 |
7 homes | 0 | 6 | 235 | 1 | 36 | 54 | 6 | 337 |
8 homes | 0 | 5 | 69 | 0 | 14 | 22 | 5 | 116 |
9 homes | 0 | 0 | 35 | 0 | 3 | 6 | 3 | 47 |
10 homes | 0 | 0 | 10 | 0 | 4 | 5 | 0 | 19 |
11 homes | 0 | 0 | 5 | 0 | 2 | 2 | 1 | 10 |
12 homes | 0 | 0 | 0 | 0 | 0 | 1 | 0 | 1 |
Total | 18,517 | 39,062 | 509,690 | 698 | 6405 | 8213 | 288 | 582,873 |
Main results
Monthly emergency admission rates per person across the study period offer a visual confirmation of little or no trend in this rate (Figure 3). There were approximately three or four admissions per month per 1000 people throughout the study. Corresponding time plots for the comparator groups showed only weak correlation with the intervention cohort for the primary outcome, and considerable noise (see Appendix 8). Therefore, we did not use the comparator groups to control the analyses.
FIGURE 3.
Monthly emergency admission rates per person (study month 1 is January 2005) for residents within intervention homes.
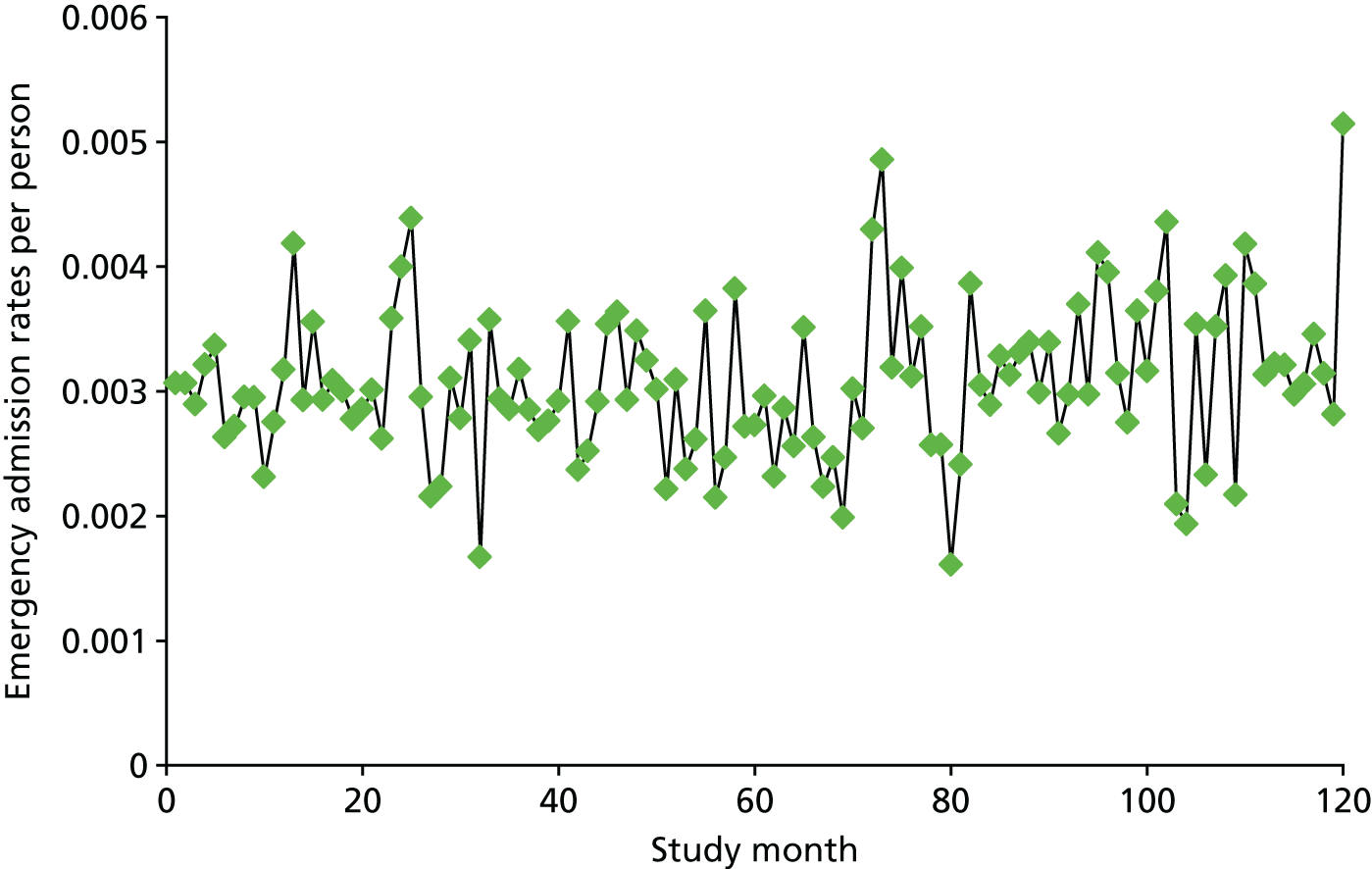
Data and model summaries for our primary outcome and several secondary outcomes are presented, firstly for all ages (Table 3 and Figure 4) and then for older residents who are aged ≥ 60 years (Table 4 and Figure 5). All outcomes possess both more skewness and kurtosis than is consistent with the assumption of normality.
Outcome | Raw data, M (SD) [n] | Adjusted comparison | 95% CI | ||
---|---|---|---|---|---|
Post intervention | Pre intervention | Paired differences | |||
Primary | |||||
Annualised rate of cardiorespiratory emergency admissions per persona | 0.0577 (0.7079) [18,527] | 0.0543 (0.4788) [22,209] | 0.0152 (0.9144) [12,433] | Δ = 0.0011 (p = 0.852) | –0.0103 to 0.0125 |
Secondary | |||||
Annualised rate of COPD-related emergency admissions per personb | 0.0069 (0.1150) [18,527] | 0.0062 (0.1468) [22,209] | 0.0054 (0.1526) [12,433] | Δ = –0.0002 (p = 0.895) | –0.0025 to 0.0022 |
Annualised rate of cardiovascular-related emergency admissions per personc | 0.0171 (0.4377) [18,527] | 0.0179 (0.2709) [22,209] | 0.0108 (0.5264) [12,433] | Δ = –0.0014 (p = 0.694) | –0.0083 to 0.0055 |
Annualised rate of respiratory-related emergency admissions per persond | 0.0371 (0.5430) [18,527] | 0.0324 (0.3720) [22,209] | 0.0045 (0.7374) [12,433] | Δ = 0.0042 (p = 0.348) | –0.0046 to 0.0131 |
FIGURE 4.
Graphical representation of the intervention effects in Table 3 (with 95% CI) by emergency admission category for people of all ages.
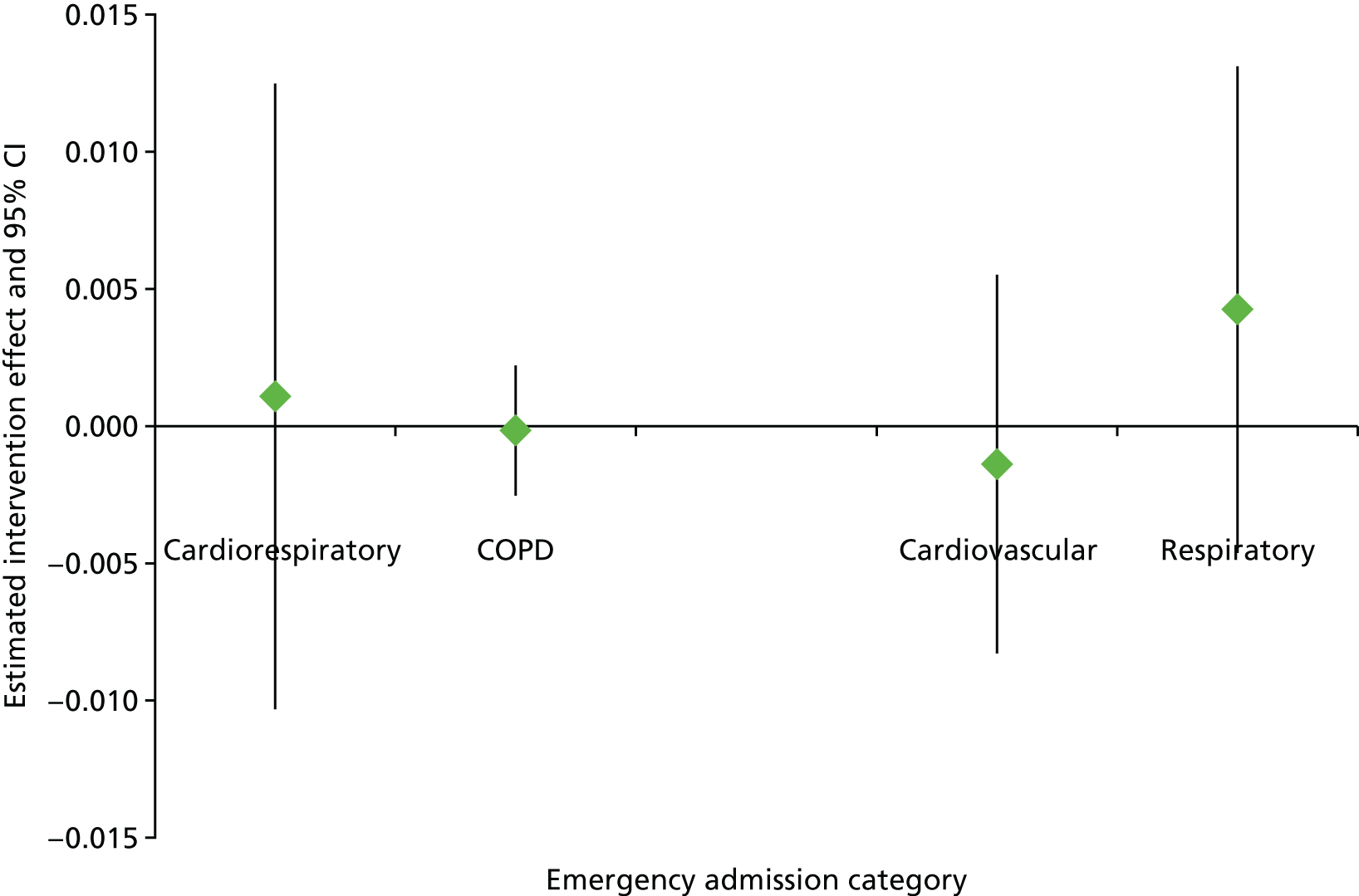
Outcome | Raw data, M (SD) [n] | Adjusted comparison | 95% CI | ||
---|---|---|---|---|---|
Post intervention | Pre intervention | Paired differences | |||
Primary | |||||
Annualised rate of cardiorespiratory emergency admissions per persona | 0.1805 (1.4538) [2226] | 0.1714 (0.8747) [2804] | 0.1152 (1.5524) [1991] | Δ = 0.0490 (p = 0.136) | –0.0153 to 0.1132 |
Secondary | |||||
Annualised rate of COPD-related emergency admissions per personb | 0.0391 (0.2656) [2226] | 0.0386 (0.3945) [2804] | 0.0229 (0.3595) [1991] | Δ = 0.0133 (p = 0.109) | –0.0030 to 0.0295 |
Annualised rate of cardiovascular-related emergency admissions per personc | 0.0704 (0.3725) [2226] | 0.0974 (0.7176) [2804] | 0.0313 (0.3880) [1991] | Δ = 0.0273 (p = 0.009) | 0.0068 to 0.0479 |
Annualised rate of respiratory-related emergency admissions per persond | 0.1074 (1.3990) [2226] | 0.0712 (0.4727) [2804] | 0.0835 (1.4960) [1991] | Δ = 0.0412 (p = 0.144) | –0.0141 to 0.0964 |
FIGURE 5.
Graphical representation of the intervention effects in Table 4 (with 95% CI) by emergency admission category for people aged ≥ 60 years.
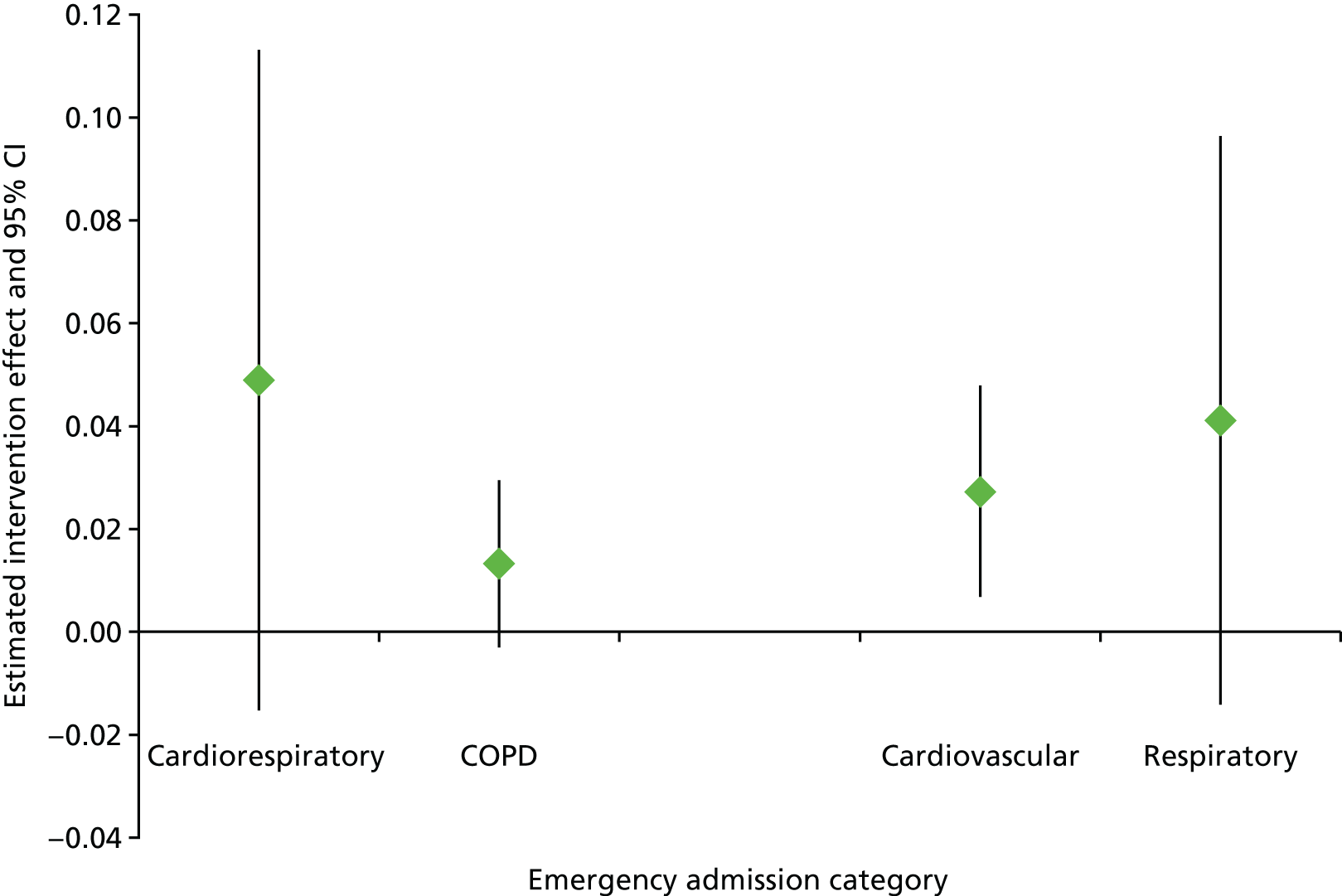
The intervention did not have a statistically significant effect on our primary outcome, neither did it have a significant effect on any of our all-age secondary outcomes. Table 3 and Figure 4 show that the intervention was not significantly associated with a change in cardiorespiratory, COPD-related, cardiovascular or respiratory emergency admissions for people of all ages. The results include summaries of the raw, unadjusted data for the pre- and post-intervention phases, and the paired differences for those people who were in the study for both phases (irrespective of the lengths of residency periods). We subsequently included covariates and factors in univariate linear models for each outcome, and the adjusted comparisons are taken from models that retain all significant covariates and factors (listed at the end of Tables 3 and 4). Age was a significant covariate in all ‘all ages’ models.
Estimates of overall intervention effects are small – for instance, the estimated Δ = 0.0011 for the primary outcome equates to 1.1 further cardiorespiratory emergency admissions per year per 1000 people – and generally consistent with the differences in pre- and post-intervention rates. However, widths of the 95% confidence intervals (CIs) for the intervention effect vary quite considerably across outcomes, reflecting both prevalence and variability of that outcome in study data. There is only moderate agreement between the (adjusted) intervention effects and the (unadjusted) paired differences in rates for those in the study for both phases. More detailed consideration of the characteristics of that subgroup may further reconcile these summaries.
Figure 5 shows the associations of the intervention with changes in cardiorespiratory, COPD-related, cardiovascular or respiratory emergency admissions for people aged ≥ 60 years. This is a relatively small subgroup, so interpretation of these results should take account of the reduced sample size. The intervention was not linked to significant changes in emergency admissions for cardiorespiratory, COPD-related and respiratory conditions. However, it had a significant effect on cardiovascular-related emergency admissions. In contrast to expectations, there was an increase in admissions in the post-intervention period.
In order to determine the excess winter hospital admissions for the primary outcome of the study (the annualised rate of combined cardiovascular or respiratory emergency admissions per person), we classified months as winter (December to March) or non-winter (April to November). Table 5 summarises the observed rates by phase (pre or post intervention) and season (winter or not winter).
Season | Raw data | Adjusted comparison | 95% CI | |
---|---|---|---|---|
Post-intervention phase, M (SD) [n] | Pre-intervention phase, M (SD) [n] | |||
Winter months | 0.0582 (0.6771) [18,147] | 0.0566 (0.5889) [21,750] | ΔPS = 0.0097 (p = 0.074) | –0.0009 to 0.0204 |
Non-winter months | 0.0520 (0.6195) [18,226] | 0.0461 (0.3319) [21,618] |
Table 5 shows that, as per intuition, rates are higher in the winter months than in the non-winter months in both the pre- and post-intervention phases. In this analysis, we are also interested in the interaction between intervention phase and season. This is because the statistical interaction summarises the extent to which differences in admissions occur between seasons for each of the pre- and post-intervention phases separately. Table 5 therefore presents details from the interaction analysis for this term (ΔPS), adjusted for comorbidity score and sex (both with p < 0.001). The interaction between season and intervention phase was not significant.
Discussion
Key results
The study found no intervention effect of reduced admissions for either the primary outcome (combined cardiovascular and respiratory emergency admissions) or any of the secondary outcomes for people of all ages living in an intervention home. It also did not find an intervention effect of reduced cardiorespiratory emergency admissions when we focused analyses on residents who were aged ≥ 60 years. However, we did see a statistically significant intervention effect of increased cardiovascular-related emergency admissions for residents aged ≥ 60 years. Cardiorespiratory emergency admission rates were found to be higher in winter than in non-winter months, in both the pre- and the post-intervention phases. The interaction between season and phase was not statistically significant, so there was no evidence that the intervention had an effect on the difference between winter and non-winter rates.
Limitations
Routine data allowed us to evaluate this intervention using retrospective links from individuals living in intervention homes for the relevant times. We were able to retrospectively analyse health-care utilisation data for a large number of people across a 10-year period and minimise the bias experienced in more-traditional research caused by factors such as recruitment and subsequent loss to follow-up. Problems of recall bias are avoided with routinely collected health data that are based on observed health events and not subjectively reported conditions.
However, we were unable to randomise participants into intervention and control groups and, as with all observational studies, the potential for unmeasured confounding remains. For example, it is challenging to control for the multiple associations between poverty, poor housing and poor health. 90
The study was only able to determine the impact of the intervention on events recorded as hospital admissions. The lack of association with emergency hospital admissions indicated that benefits do not appear in the hospital statistics within the follow-up period and that future evaluations should focus on less-severe conditions that may be treated in primary care settings, although recent research suggests that housing interventions may not necessarily change established patterns in primary health-care utilisation in populations with a long-term lack of well-being. 91
Furthermore, we acknowledge the data limitations, including the lack of depth of the routine data. For instance, using routine data means that we cannot understand how people’s lives have changed in their home; this needed the additional studies that were completed as part of this research (see Chapter 3). Data quality was also an issue. Intervention home addresses and intervention dates required considerable time and effort to collect, and subsequently convert to a structured format that could be used within the databank. There were missing dates for several hundred intervention homes.
We relied on a routinely collected demographic data set (WDS) to link people into homes for the correct time periods. Research by the ONS suggested that some segments of the younger male population are more likely to be missing from this data set. Further work is needed to quantify potential bias in this routine anonymised data set.
Finally, we acknowledge that assumptions implicit in linear models are unlikely to be entirely satisfied by the study data, and that it would be useful to investigate further models that seek to account more explicitly for features observed in this data.
Interpretation
There was no evidence that energy efficiency investments, as implemented in the intervention programme, provided a reduction in health-care utilisation in the short to medium term. Residents were not involved in the intervention implementation and so may have felt a lack of control; this may have contributed to the lack of a significant reduction in health-care events. 92 Longer-term studies, of a decade or longer, may be needed to realise an intervention effect on health-care utilisation recorded using routine data, including hospital as well as primary care outcomes.
Generalisability
The research is generalisable to residents of homes located in temperate regions, with similar socioeconomic characteristics, living in homes in need of thermal efficiency measures. The intervention homes were predominantly social housing, maintained by LAs and RSLs and located within low-income areas. The results may also be generalisable to some extent to residents of private rented homes or homeowners in low-income areas.
Chapter 3 The community-based study
This chapter contains material from Grey et al. 80 This article is distributed under the terms of the Creative Commons Attribution 4.0 International License (http://creativecommons.org/licenses/by/4.0/), which permits unrestricted use, distribution, and reproduction in any medium, provided you give appropriate credit to the original author(s) and the source, provide a link to the Creative Commons license, and indicate if changes were made. The Creative Commons Public Domain Dedication waiver (http://creativecommons.org/publicdomain/zero/1.0/) applies to the data made available in this article, unless otherwise stated. The text below includes minor additions and formatting changes to the original text.
Introduction
This chapter follows the STROBE reporting guidelines for observational study designs. 81
Background
Chapter 2 reported on the impacts of energy efficiency investments on hospital admissions for cardiovascular and respiratory conditions using the SAIL databank. The first phase of the intervention programme, which took place between 2010 and 2012, was evaluated by anonymously linking address and intervention records to the PEDW, containing all hospital admissions and day case activity in Welsh hospitals and Welsh residents being treated in hospitals in England. The strength of this approach is that it can retrospectively evaluate the intervention without the results being biased as a result of low response rates and losses to follow-up. However, although national health records can be used to link the intervention to actual health service use, it does not detect mental health conditions that do not involve the use of health services, as well as more-subjective psychosocial aspects that may be part of pathways to health. The community-based study reported in this chapter specifically focused on mental health and well-being, self-reported respiratory conditions and wider psychosocial impacts of the intervention.
As discussed in Chapter 1, the literature suggests two interrelated pathways between energy efficiency investments and better mental and physical health. 54,56 Energy efficiency investments may improve living conditions through increased indoor air temperatures and fewer damp-related housing problems. Improving living conditions may not only contribute to better respiratory health but can also contribute to better mental health through improved thermal satisfaction. 58 Furthermore, an expansion of living space and improved social interactions (and therefore reduced social isolation) may be beneficial for residents’ mental well-being. 59 Energy efficiency investments also contribute to improved well-being by making heating more affordable. 6 Reduced spending on heating bills alleviates financial stress and fuel poverty among low-income households60,61 and helps to free financial resources for better food security45,46 and social interactions. 31
Objectives
This chapter describes the methodology and findings of the community-based evaluation of the second phase of the intervention programme. The overall aim of the study was to better understand the short-term health and psychosocial impacts of energy efficiency investments in low-income areas. More specifically, the study aimed to determine the impacts of (1) the intervention on the primary health outcomes of self-reported respiratory symptoms and mental health, (2) the intervention on the secondary health outcomes of overall physical quality of life and subjective well-being and (3) energy efficiency investments on secondary psychosocial outcomes that may be part of pathways to health, including self-reported fuel poverty, food security and financial difficulties and stress, as well as self-reported thermal satisfaction, housing conditions, the number of heated rooms and social interactions. An additional aim was the health economic evaluation of the extent to which the intervention changes the health-related quality of life of inhabitants, through the calculation of quality-adjusted life-years (QALYs).
The research described in this chapter received ethics approval from the School Research Ethics Committee (SREC) of the Welsh School of Architecture, Cardiff University (EC1308.160).
Methods
Study design
The community-based study used a quasi-experimental controlled pre-/post-test design and collected data through self-completion questionnaires. Questionnaires were distributed in low-income areas where energy efficiency improvements were scheduled but had not yet started, as well as in matched control areas where no energy efficiency improvements were scheduled. The intervention programme ran for 3 years between 2012 and 2015. The community-based study focused on schemes that were delivered in 2014 and 2015, respectively.
Setting
The energy efficiency investment programme targeted low-income mixed-tenure neighbourhoods. The schemes were selected on the basis of the number of low-income households, the number of households owning or privately renting their house and the number of hard-to-heat, hard-to-treat homes in the area. The schemes mostly took place in Strategic Regeneration Areas with high numbers of hard-to-heat, hard-to-treat homes. Matched control areas were selected using the WIMD and with the assistance of the LAs where the schemes were taking place. In total, 24 intervention and 23 control areas were included in the study. Anglesey was the only scheme that did not have a direct matched control area. Figure 6 shows the locations of the intervention areas that were included in the study.
FIGURE 6.
Locations of the intervention areas.
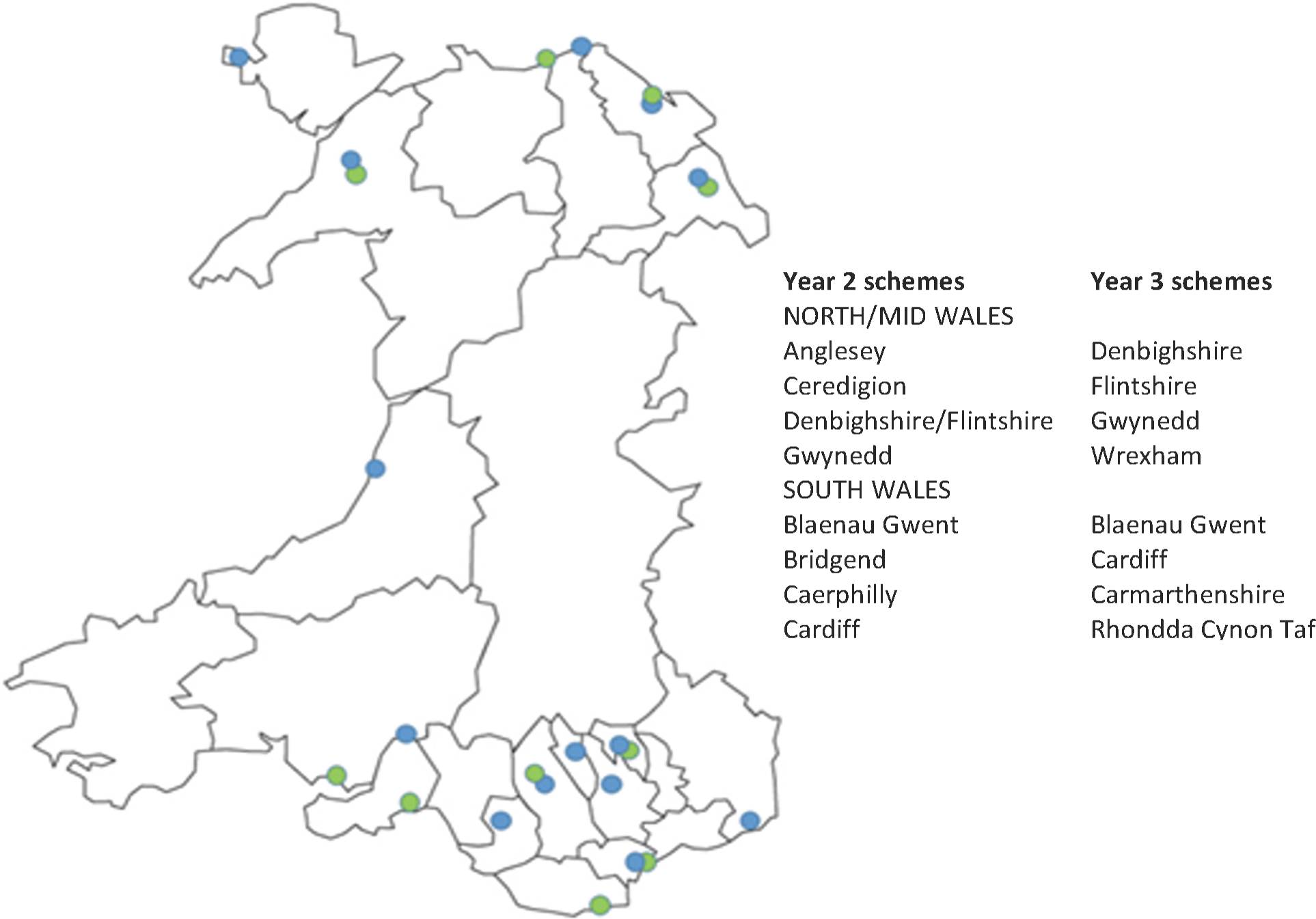
Baseline (pre-intervention) data for year 2 schemes were collected during the 2013/14 heating season, before energy improvement work had started on homes scheduled to receive the intervention. Follow-up (post-intervention) data for year 2 schemes were collected during the 2014/15 heating season, after the work was completed. Baseline and follow-up data for year 3 schemes were collected during the 2014/15 and 2015/16 heating seasons, respectively. Data for the intervention and control areas were collected during the same time periods. Data for one year 2 scheme (Gwynedd) could not be collected in two subsequent heating seasons as a result of delays in the delivery of the improvements. In this case, the follow-up data for both the intervention and matched control area were collected during the 2015/16 heating season, at the same time as the follow-up data for year 3 schemes.
Participants
We used a purposive sampling strategy to recruit residents living in the eligible intervention and control areas. The survey was administered using the drop-off-and-collect method of data collection. 93 Initial contact was made by postal letter, introducing the study and informing residents that they were going to be visited by researchers from the university. Researchers then visited all selected areas to deliver the questionnaires by hand and, when possible, personally invite residents to take part in the study. When at the time of delivery no residents were at home, a pack containing a cover letter, questionnaire and Freepost envelope was left in their letter box. Researchers then returned at a later date, usually within a week, to collect the questionnaires. Similarly, a reminder to return the questionnaire was left if occupants were away at the time of the return visit. The questionnaire could then be returned by Freepost. Participants were asked to provide their contact details and consent to be recontacted for the follow-up phase of the study. Any adult resident currently living in the selected intervention and control areas was eligible for inclusion.
The initial invitation letter and the questionnaire were both translated into Welsh. The Welsh version of the questionnaire was available on request.
All participants who had returned a completed questionnaire in the baseline period and had consented to be recontacted were invited to fill out a second questionnaire for the follow-up. Participants were sent a postal questionnaire that was addressed to them personally. They were subsequently visited by researchers to collect the questionnaire in person when possible. A reminder was left if the participants were not at home during the follow-up visit.
Incentives were used to increase the baseline and follow-up response rates. Participants were entered into a prize draw for one of 25 £50 shopping vouchers (year 2 baseline) or for one of three iPads (Apple Inc., Cupertino, CA, USA) (year 3 baseline and all follow-up stages).
Not every eligible household within the scheme areas elected to have energy efficiency work done to their house. Records from the two scheme managers were used to confirm which properties were upgraded and which improvements were made. Respondents from intervention areas who did not have energy efficiency work done to their home became part of the control group. A small number of properties located within the matched control area of a delayed year 2 scheme (Gwynedd) became part of a year 3 scheme. Respondents from these properties became part of the intervention group.
Variables
Data were collected via a self-completion questionnaire covering the topics of health and well-being, fuel poverty, financial difficulties and stress, food security, social interactions, thermal satisfaction, housing conditions and number of heated rooms. The sociodemographic section of the questionnaire contained questions about sex, age, household composition, marital status, employment status, household income, smoking and housing benefits (see Appendix 9).
The primary health outcomes were changes in mental health status [MCS from the Short Form questionnaire-12 items (SF-12)] and self-reported respiratory and asthma symptoms. Secondary health outcomes were changes in self-reported health-related quality of life [Physical Health Composite Scale (PCS) from the SF-12] and subjective well-being. Other secondary psychosocial outcomes included changes in fuel poverty status, financial difficulties and stress, food security, social interactions, thermal satisfaction and reported housing conditions.
The Short Form questionnaire-12 items
The SF-12 is a validated and widely used measure to assess overall health-related quality of life. 94 The 12-item survey includes questions on physical and mental health, covering the eight subdomains of general health, physical functioning, physical role functioning, bodily pain, vitality, emotional role functioning, mental health and social functioning. The questions consist of 10 five-point items and two three-point items as measured on a Likert scale. The items were combined using a standardised scoring algorithm to form the separate PCS and MCS, which range from 0–100 with a standardised mean score of 50 and a standard deviation (SD) of 10. Higher scores represent better well-being.
Respiratory symptoms
Self-reported respiratory symptoms were measured using items adapted from Fisk et al. 26 and WHO. 95 Respondents were asked if they had experienced any of the following symptoms over the past month: coughing, bringing up phlegm, shortness of breath, wheezing attack, chest tightness, runny nose, blocked nose, sinus swelling, sneezing, sore throat and cold or flu. The respiratory symptoms were summed creating a scale ranging from 0 to 11.
Asthma symptoms
The short version of the European Community Respiratory Health Survey was included in the questionnaire, which contains nine questions about the presence of respiratory symptoms during the previous 12 months (wheezing and whistling, breathlessness, chest tightness, shortness of breath, nocturnal coughing), attacks of asthma during the previous 12 months, current use of asthma medication and nasal allergies including hay fever. The survey was designed to estimate prevalence of asthma, asthma-like symptoms and airway responsiveness. 96 The asthma symptoms were summed creating a scale ranging from 0 to 9.
Subjective well-being
Subjective well-being was measured using four questions developed by the ONS. 97 The questions measure three different aspects of subjective well-being, including respondents’ life satisfaction (an evaluation or global assessment measure), happiness and anxiousness (both hedonic well-being measures, reflecting feelings over short periods of time) and worthwhileness (a eudemonic measure). Each of the four questions could be answered using an 11-point Likert scale. The four responses were combined into a single scale after reversing the anxiousness item (Cronbach’s α = 0.90). The final scale ranged from 0 (low subjective well-being) to 10 (high subjective well-being).
Fuel poverty
One self-reported indicator of subjective fuel poverty was used. Respondents were asked whether or not, within the past 12 months, they had put up with feeling cold to save heating costs. Respondents could answer yes or no. This question has previously been used in the New Zealand Housing, Heating and Health Study. 98
Financial difficulties and stress
The study used a financial stress scale from the Renton-I study99 that was used to measure how often respondents had difficulties meeting the cost of different house-related expenses, such as rent or mortgage, repairs or maintenance of home, fuel bills or credit payments. Each question could be answered using a four-point Likert scale ranging from 1 (never) to 4 (very often). The responses to the four items were averaged to create a single scale (Cronbach’s α = 0.79). Respondents were further asked about their general level of financial stress using a question derived from the INTERHEART study. 100 The question used a five-point Likert scale ranging from none (1) to high (5).
Food security
The questionnaire included three questions from the US Adult Food Security Survey (2012) to determine households’ economic access to food in terms of quantity, quality and variety. 101 Food security is defined as access at all times to enough food for an active, healthy life, including readily available nutritionally adequate and safe foods that can be acquired in socially acceptable ways. 102 The following three questions were asked: (1) ‘In the last 12 months, the food I bought just didn’t last, and I didn’t have money to get more’; (2) ‘In the last 12 months, I couldn’t afford to eat balanced meals’; and (3) ‘In the last 12 months, did you ever cut the size of your meals or skip meals because there wasn’t enough money for food?’. Each question could be answered using a four-point Likert scale ranging from 1 (very often) to 4 (never). The responses were averaged to create a single scale (Cronbach’s α = 0.92).
Thermal satisfaction
Thermal satisfaction was measured by asking respondents how satisfied they are with the temperature in their home on a typical winter day. The five-point response scale ranged from 1 (very dissatisfied) to 5 (very satisfied). This question was previously used in the Carmarthenshire Homes Standard Health Impact Assessment Study. 103
Housing conditions
Housing conditions were assessed in two ways. First, respondents were asked about their satisfaction with the current state of repair of their home. Respondents could answer the question on a five-point Likert scale ranging from 1 (very dissatisfied) to 5 (very satisfied). Second, respondents were asked whether or not they were currently experiencing six housing-related problems. These were condensation, leaking roofs, damp walls and/or floors, rot in windows and door frames, draught and mould. The housing problems were summed, creating a scale ranging from 0 to 6.
Number of heated rooms
Respondents were asked about their heating behaviours, in particular which rooms were heated on a typical winter day and evening. The items were combined to provide a sum of the number of rooms that are typically heated during the day and in the evening, focusing on four key rooms within the home: the kitchen, main living room, main bedroom and bathroom. The sum of the number of heated rooms was used in the analyses.
Social interactions
Social interactions were measured by asking respondents whether or not, in the last year, they had ever felt reluctant to invite friends or family to their home because of difficulties with keeping it warm. The question originated from the Adult Psychiatric Morbidity in England study. 104 Respondents could answer yes or no.
The Short Form questionnaire-6 Dimensions
The Short Form questionnaire-6 Dimensions (SF-6D) utility score was calculated from the SF-12 into the SF-6D using the ‘Sheffield algorithm’. 105 The weighting algorithm was calculated using the preference-based standard gamble approach. The resulting SF-6D index value incorporates all health domains from the SF-12, with higher numbers associated with better health utility. Full health is represented by a value of 1 and death corresponds to a score of 0. The SF-6D utility score allows for the calculation of QALYs. One QALY is equal to living 1 year in full health; the duration in years is multiplied by the utility score.
Bias
The community-based study reported in this chapter used a quasi-experimental, controlled pre-/post-test design to control for time-variable factors such as external hydrothermal conditions and other temporal trends. The assignments of participants to the intervention and control groups was not randomised. Selection bias occurs when selection to the intervention and control groups results in differences in unit characteristics between conditions that may be related to outcome differences. 106 In order to maximise initial comparability, the matched control areas were selected using the same criteria as the intervention schemes.
The drop-off-and-collect method of survey administration and incentives were used to reduce selection bias as a result of non-response as much as possible. 93 The overall response rate was 16.5%, with 1508 of the 9127 distributed questionnaires being completed and returned. Of the 1508 baseline respondents, 1288 consented to be recontacted (85.4%). The attrition rate between baseline and follow-up was 48.1% owing to a lack of consent and loss to follow-up (n = 726), resulting in a final total sample of 782 respondents who filled out both the baseline and follow-up questionnaires (Figure 7).
FIGURE 7.
The flow diagram for the community-based study.
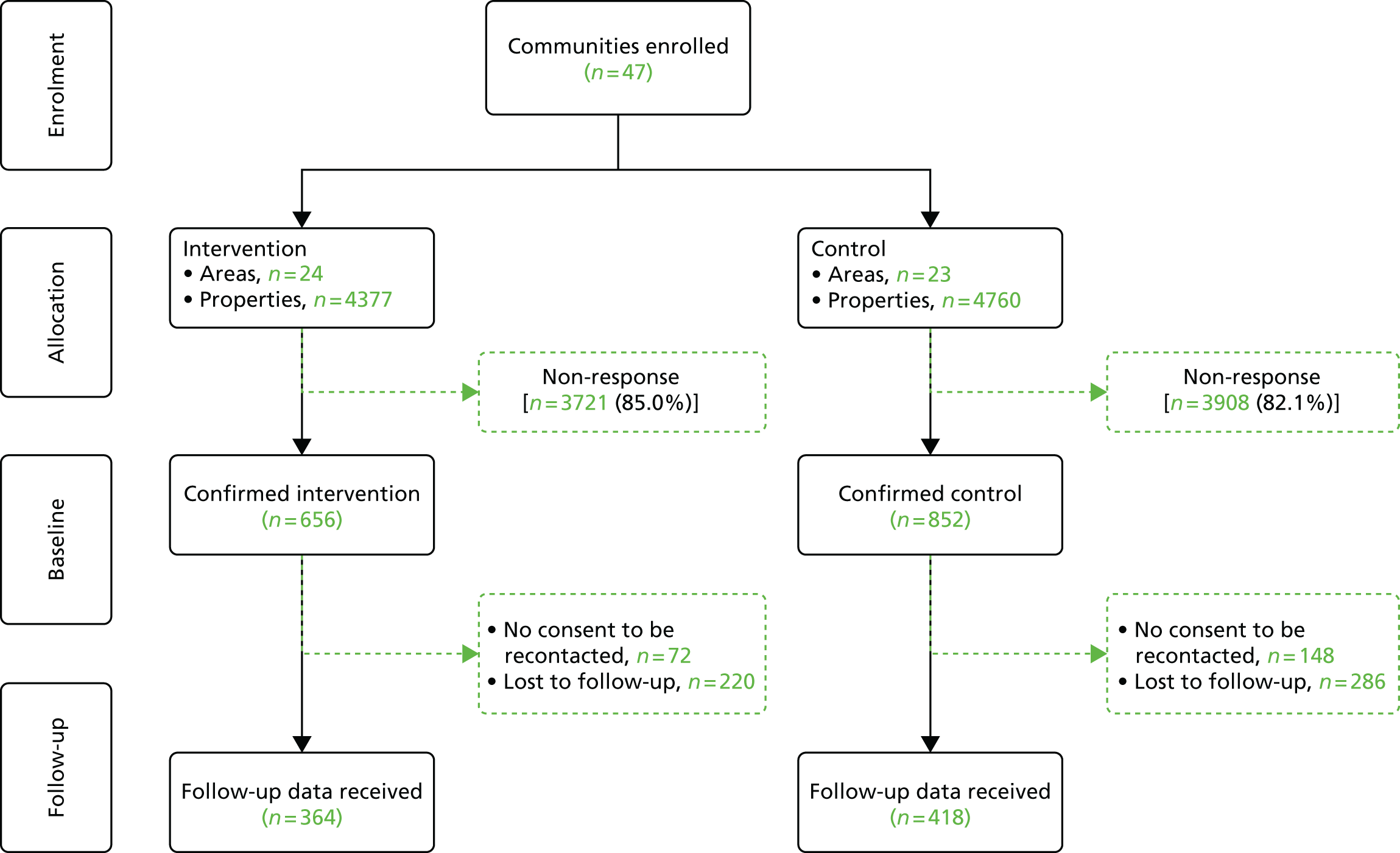
The overall response for the baseline data collection rates were similar for the intervention and control groups (15.0% vs. 17.9%), although there were some differences in attrition (44.5% vs. 50.9%) between the baseline and follow-up phases [χ2(1) = 6.132; p = 0.013]. Differences were also seen in the rate of consent between the intervention and control areas (89.0% vs. 82.6%) [χ2(1) = 12.165; p = 0.000]. The resulting intervention and control populations were comparable in terms of sociodemographics, with chi-squared tests showing non-significant differences between the two groups for all variables listed.
A loss to follow-up analysis showed that there were a number of socioeconomic differences between the respondents included in the final study sample and those who dropped out between baseline and follow-up (Table 6). Respondents lost to follow-up were more likely to be female [χ2(1) = 9.661; p = 0.002], younger in age [χ2(7) = 73.053; p = 0.000] and have children living in the household [χ2(1) = 28.738; p = 0.000]. Respondents lost to follow-up were also more likely to have a lower household income [χ2(8) = 15.950; p = 0.043] and to receive housing benefits [χ2(1) = 8.763; p = 0.003]. In terms of health outcomes, the respondents lost to follow-up were more likely to be at risk of common mental disorders, as measured by the MCS of the SF-12 [χ2(1) = 8.594; p = 0.003]. However, there were no differences with regard to physical health, as measured by the PCS of the SF-12, subjective well-being and self-reported respiratory and asthma symptoms.
Characteristics | Respondents, % (n/N) | |
---|---|---|
Study | Lost to follow-up | |
Sociodemographic | ||
Sex | ||
Male | 40.7 (311/764) | 32.9 (230/700) |
Female | 59.3 (453/764) | 67.1 (470/700) |
Age (years) | ||
≤ 25 | 1.6 (14/776) | 5.7 (41/712) |
26–35 | 6.4 (50/776) | 13.9 (99/712) |
36–45 | 10.1 (78/776) | 15.4 (110/712) |
46–54 | 12.6 (98/776) | 15.3 (109/712) |
55–64 | 27.3 (213/776) | 17.3 (123/712) |
≥ 65 | 41.6 (323/776) | 32.3 (230/712) |
Household composition | ||
Households with no children | 81.2 (623/767) | 69.8 (484/693) |
Households with children | 18.8 (144/767) | 30.7 (209/693) |
Marital status | ||
Single | 12.5 (97/778) | 16.6 (119/716) |
Married/cohabiting | 53.3 (414/778) | 51.5 (369/716) |
Separated/divorced | 19.1 (148/778) | 17.1 (123/716) |
Widowed | 15.0 (117/778) | 13.8 (99/716) |
Household income | ||
£0–4999 | 3.6 (26/731) | 7.6 (49/641) |
£5000–9999 | 24.8 (182/731) | 24.1 (155/641) |
£10,000–19,999 | 35.0 (256/731) | 37.2 (238/641) |
£20,000–29,999 | 16.6 (121/731) | 15.0 (96/641) |
≥ £30,000 | 20.0 (146/731) | 16.1 (103/641) |
Housing benefits | ||
Yes | 24.7 (187/756) | 31.7 (222/700) |
No | 75.3 (569/756) | 68.3 (478/700) |
Fuel poverty (putting up with feeling cold to save heating costs) | ||
Yes | 59.4 (464/774) | 64.4 (457/710) |
No | 40.6 (310/774) | 35.6 (253/710) |
Health | ||
MCS | ||
At risk of CMD | 57.1 (444/777) | 64.6 (461/714) |
Not at risk of CMD | 42.9 (333/777) | 35.4 (253/714) |
PCS | ||
At risk of physical complaints | 58.8 (456/776) | 59.9 (427/714) |
Not at risk of physical complaints | 41.2 (320/776) | 40.1 (287/714) |
Subjective well-being | ||
Very low | 12.2 (95/781) | 13.1 (95/724) |
Low | 24.6 (192/781) | 28.5 (206/724) |
Medium | 29.2 (228/781) | 30.4 (220/724) |
High | 34.1 (226/781) | 28.0 (203/724) |
Respiratory symptoms | ||
0 | 23.4 (183/781) | 21.2 (154/725) |
1 | 14.8 (116/781) | 13.2 (96/725) |
2 | 12.8 (100/781) | 13.2 (96/725) |
3 | 12.9 (101/781) | 10.2 (74/725) |
4 | 10.7 (84/781) | 10.2 (74/725) |
≥ 5 | 25.1 (197/781) | 31.8 (231/725) |
Asthma symptoms | ||
0 | 31.6 (225/713) | 30.8 (199/646) |
1 | 23.0 (164/713) | 20.3 (131/646) |
2 | 11.1 (79/713) | 12.5 (81/646) |
3 | 6.5 (46/713) | 7.3 (47/646) |
4 | 7.2 (51/713) | 7.6 (49/646) |
≥ 5 | 20.9 (41/713) | 21.5 (139/646) |
Recall bias may be another possible source of bias, which was minimised by asking respondents to answer questions regarding the current heating season. Missing data bias is possible when respondents may have chosen not to answer specific questions in either questionnaire. Overall, there were few missing values, except for self-reported asthma symptoms. A missing-values analysis found that data were missing completely at random.
Study size
We aimed to achieve a total sample size of 1000 participants (intervention, n = 500; control group, n = 500) to have 80% statistical power to detect an effect size (d) of 0.16 at the 5% significance level. The final achieved samples of n = 364 for the intervention group and n = 418 for the control group provide 80% statistical power to detect effect sizes of d = n = 0.18 at the 5% significance level, which is in line with effect sizes observed in comparable field studies examining the short-term health effects of housing improvements. 48,51,107
Statistical methods
Statistical analyses were conducted with IBM SPSS Statistics version 20.0 (IBM Corporation, Armonk, NY, USA) and MLwiN version 2.36 (MLwiN, Centre for Multilevel Modelling, Bristol, UK) software packages. 108–110 The effects of the intervention on the primary and secondary health and psychosocial outcomes were initially assessed using mixed design analyses of variance (ANOVAs) as proposed, and subsequently using a multilevel modelling, repeated measures approach. The two types of analyses produced similar results. Here we only present the results of the multilevel modelling analyses for the purpose of clarity.
A longitudinal data set was created with the two measurement occasions (level 1) nested within individuals (level 2), allowing the analyses to take into account non-independence between measurement occasions. The basic model included the intervention group (intervention vs. control) as an individual-level factor and measurement occasion (follow-up vs. baseline) as a within-person factor. Differential changes between the intervention and control groups were assessed with a cross-level interaction between measurement occasion and intervention group. Only the interaction effects indicating the differential changes between the intervention and control groups are reported. All analyses were conducted with and without adjusting for the covariates selected a priori: sex, age, housing benefit, household income and smoking status. Cohen’s d was calculated to determine the size of interaction effects. Cohen’s d is a standardised measure of the magnitude of an effect, with the values of 0.2, 0.5 and 0.8 generally taken to reflect ‘small’, ‘medium’ and ‘large’ effect sizes, respectively. 111,112
Different types of models were constructed depending on the type of outcome variable. Linear regression models were constructed for the primary health outcomes of respiratory symptoms, asthma symptoms, MCS, the secondary health outcomes of PCS and subjective well-being, as well as for the secondary psychosocial outcomes of financial difficulties and food security. Ordered multinomial response models were constructed for the ordinal psychosocial outcome variables of financial stress, thermal satisfaction, satisfaction with the current state of repair of their home, number of reported housing problems and the number of heated rooms during the day and the evening. Logistic regression models were constructed for the two secondary psychosocial outcomes of fuel poverty and social interactions. For the ordered multinomial response and binomial models, a logit link function was used. Parameters in all models were estimated using Markov chain Monte Carlo with 50,000 iterations. All analyses were conducted with and without adjusting for the individual-level covariates of sex, age, housing benefits, household income and smoking status. The five covariates were selected a priori.
For the SF-12, missing data were dealt with using QualityMetric’s Missing Score Estimation algorithm (QualityMetric, Lincoln, RI, USA), for which item response theory and regression methods were used to estimate scores on the health domain scales and component summary measures (MCS and PCS) using QualityMetric’s Health Outcomes Scoring Software (QualityMetric, Lincoln, RI, USA). 113 All other missing data were excluded from the statistical analyses, which were conducted without imputation, as the percentage of missing values were in most cases low (< 3%). The missing values were higher for asthma symptoms (8.8%). Little’s MCAR (missing completely at random) tests found that the data were missing completely at random [primary and secondary health outcomes and sociodemographic characteristics: χ2(26) = 28.745; p = 0.323; psychosocial outcomes: χ2(60) = 63.720; p = 0.347].
For the analysis of health-related quality of life and QALYs, the SF-6D index values were transformed into utilities implementing the area under the curve approach, with these values baseline-adjusted. A linear progression of SF-6D between baseline and follow-up was assumed in the absence of more data. For the main analysis, a multilevel modelling, repeated measures approach was used, adjusting for the individual-level covariates of sex, age, housing benefits, household income and smoking status.
Results
Participants
In total, 9137 questionnaires were distributed to eligible households across 47 intervention and control areas at the start of year 2 and year 3 of the intervention programme (see Figure 6). Of these, 1508 were completed and returned (656 from the intervention and 852 from the control areas), corresponding to an overall response rate of 16.5%. Figure 7 illustrates the flow of participants through the study.
Out of the 1508 baseline participants in both the intervention and control groups, 220 did not consent to be recontacted and a further 506 participants were lost to follow-up, reflecting an overall attrition rate of 48.1%. Eighty-one participants from the original intervention areas did not receive any upgrades to their home as part of the intervention programme. These respondents were added to the control group. Some of the properties in the matched control area for the delayed year 2 scheme in Gwynedd were included in a year 3 scheme before follow-up data could be collected. The 22 respondents from these properties were added to the intervention group.
Descriptive data
Table 7 summarises the final study sample in terms of sociodemographic and building characteristics. The intervention and control populations were comparable in terms of sociodemographic and building characteristics, with chi-squared tests showing no significant differences between the two groups for any of the variables listed in the table. Because the intervention and control group are largely similar at baseline, there is a low likelihood of confounding.
Characteristics | Study cohort, % (n/N) | |
---|---|---|
Intervention | Control | |
Sociodemographic | ||
Sex | ||
Male | 41.2 (145/352) | 40.3 (166/412) |
Female | 58.8 (207/352) | 59.7 (246/412) |
Age (years) | ||
≤ 25 | 1.4 (5/362) | 2.1 (9/414) |
26–35 | 6.9 (25/362) | 6.0 (25/414) |
36–45 | 10.8 (39/362) | 9.4 (39/414) |
46–54 | 14.1 (51/362) | 11.1 (47/414) |
55–64 | 30.1 (109/362) | 25.1 (104/414) |
≥ 65 | 36.7 (133/362) | 45.9 (190/414) |
Household composition | ||
Without children | 79.1 (284/359) | 83.1 (339/415) |
With children | 20.9 (75/359) | 16.9 (76/415) |
Marital status | ||
Single | 13.8 (50/363) | 11.3 (47/412) |
Married/cohabiting | 55.1 (200/363) | 51.6 (214/412) |
Separated/divorced | 16.0 (58/363) | 21.7 (90/412) |
Widowed | 14.9 (54/363) | 15.2 (63/412) |
Household income | ||
£0–4999 | 3.5 (12/339) | 3.6 (14/392) |
£5000–9999 | 23.5 (80/339) | 25.8 (101/392) |
£10,000–19,999 | 33.9 (115/339) | 35.9 (141/392) |
£20,000–29,999 | 18.0 (61/339) | 15.3 (60/392) |
≥ £30,000 | 20.6 (70/339) | 19.4 (76/392) |
Housing benefits | ||
Yes | 25.1 (89/354) | 24.4 (98/402) |
No | 7.9 (265/354) | 75.6 (304/402) |
Tenure | ||
Owner occupied | 77.5 (276/356) | 74.0 (307/415) |
Private rental | 3.9 (14/356) | 6.7 (28/415) |
LA rental | 13.8 (49/356) | 13.3 (55/415) |
Housing association rental | 3.4 (12/356) | 5.3 (22/415) |
Time lived at current address (years) | ||
< 1 | 3.3 (12/360) | 4.3 (18/416) |
1–4 | 9.4 (34/360) | 11.8 (49/416) |
5–9 | 14.4 (52/360) | 12.7 (53/416) |
> 9 | 72.8 (262/360) | 71.2 (296/416) |
Building | ||
Building type | ||
Detached house | 12.5 (45/360) | 12.3 (51/416) |
Semi-detached house | 38.1 (137/360) | 28.8 (120/416) |
Terraced house | 41.1 (148/360) | 46.9 (195/416) |
Bungalow | 5.0 (18/360) | 5.3 (22/416) |
Flat | 1.4 (5/360) | 6.0 (25/416) |
Year of construction | ||
Before 1919 | 44.1 (152/345) | 40.7 (166/405) |
1919–1945 | 26.1 (90/345) | 24.3 (99/405) |
1945–1965 | 17.4 (60/345) | 23.5 (96/405) |
1965–1979 | 7.5 (26/345) | 6.1 (25/405) |
1980 or later | 4.9 (17/345) | 5.4 (22/405) |
Number of bedrooms | ||
1 | 1.7 (6/358) | 5.4 (22/409) |
2 | 19.8 (71/358) | 20.8 (85/409) |
3 | 68.2 (244/358) | 65.3 (267/409) |
≥ 4 | 10.4 (37/358) | 8.5 (35/409) |
Of the 782 respondents who completed both the baseline and follow-up questionnaires, the highest proportion was female, aged ≥ 65 years, did not live with children within their household, was either married or cohabiting and was retired. The highest proportion of respondents had no formal educational qualification, had a combined household income of £10,000–19,999. One-quarter of all respondents received housing benefits, reflecting the overall levels of deprivation of the intervention and control areas. The vast majority of respondents owned the properties they resided in and had lived in the same property for > 9 years. The building type tended to be either semi-detached or terraced, built either before 1919 or before 1945, with an average of three bedrooms.
External wall insulation was by far the most implemented measure (71.7%), followed by voltage optimisers (44.4%) and full central heating systems (new condensing boilers and radiators and pipes when needed; 39.0%). Respondents from three communities received a connection to the mains gas network together with a new heating system (13.9%). Voltage optimisers were only implemented in South Wales schemes.
Main results
The impact of the intervention on the primary health outcomes
The primary health outcomes assessed were self-reported respiratory and asthma symptoms, and the mental health composite score of the SF-12 (MCS). The study found no evidence that the intervention had a significant impact on the primary health outcomes of the study. Table 8 shows that there was a small reduction in the number of reported respiratory symptoms between baseline and follow-up for the intervention group. However, the change does not statistically differ from the one observed for the control group. Similarly, the reduction in the number of reported asthma symptoms for the intervention group did not significantly differ from the change observed in the control group. There was a slight increase in the MCS scores for the intervention group. However, the increase for the intervention group was similar to the increase for the control group.
Outcome | Scale | Model | Study cohort, M (SD) | Effect size | Unadjusted | Adjusteda | |||||||
---|---|---|---|---|---|---|---|---|---|---|---|---|---|
Intervention | Control | ||||||||||||
Baseline | Follow-up | Baseline | Follow-up | Cohen’s d | B | SE | p | B | SE | p | |||
Respiratory symptoms | 0–11 | L | 2.97 (2.74) | 2.91 (2.74) | 2.94 (2.67) | 3.05 (2.77) | 0.061 | –0.155 | 0.192 | 0.419 | –0.141 | 0.202 | 0.485 |
Asthma symptoms | 0–9 | L | 2.34 (2.55) | 2.20 (2.45) | 2.23 (2.50) | 2.24 (2.51) | 0.051 | –0.088 | 0.247 | 0.722 | –0.133 | 0.253 | 0.600 |
MCS | 0–100 | L | 44.81 (12.56) | 45.62 (11.94) | 46.02 (12.06) | 46.85 (12.36) | 0.005 | –0.059 | 0.789 | 0.940 | –0.003 | 0.812 | 1.000 |
The impacts of the intervention on the secondary health outcomes
The secondary health outcomes were the PCS of the SF-12 and subjective well-being. The study found no evidence that the intervention had an impact on physical quality of life, as measured by the PCS. Although there was a slight reduction in PCS scores for the intervention group, the reduction was not significantly different from the reduction observed for the control group.
Table 9 shows an increase in subjective well-being for the intervention group but a slight decrease for the control group. This represents a significant measurement occasion × intervention group interaction effect at the 1% level. The interaction effect remained significant after controlling for the covariates of sex, age, household income, housing benefits and smoking status (B 0.38, 95% CI 0.11 to 0.64). This suggests that the intervention had a small positive impact on people’s overall feelings of well-being (Cohen’s d = 0.20).
Outcome | Scale | Model | Study cohort, M (SD) | Effect size | Unadjusted | Adjusteda | |||||||
---|---|---|---|---|---|---|---|---|---|---|---|---|---|
Intervention | Control | ||||||||||||
Baseline | Follow-up | Baseline | Follow-up | Cohen’s d | B | SE | p | B | SE | p | |||
PCS | 0–100 | L | 42.90 (13.94) | 43.11 (13.80) | 42.25 (14.47) | 41.48 (14.24) | 0.107 | 0.987 | 0.664 | 0.137 | 0.976 | 0.669 | 0.145 |
Subjective well-being | 0–10 | L | 6.55 (2.50) | 6.89 (2.25) | 6.96 (2.42) | 6.92 (2.42) | 0.200 | 0.375 | 0.134 | 0.005 | 0.384 | 0.134 | 0.004 |
The impacts of the intervention on the secondary psychosocial outcomes
Table 10 shows the results for the secondary psychosocial outcomes of the study. Analyses indicate that the intervention had an impact on financial difficulties, thermal satisfaction, satisfaction of the state of repair of the home, the number of housing problems and social interactions.
Outcome | Scale | Model | Study cohort, M (SD) | Effect size | Unadjusted | Adjusteda | |||||||
---|---|---|---|---|---|---|---|---|---|---|---|---|---|
Intervention | Control | ||||||||||||
Baseline | Follow-up | Baseline | Follow-up | Cohen’s d | B | SE | p | B | SE | p | |||
Fuel poverty | |||||||||||||
Putting up with feeling cold to save heating costs | 0–1 | Bin | 0.63 (0.48) | 0.45 (0.50) | 0.57 (0.50) | 0.46 (0.50) | 0.153 | –0.720 | 0.324 | 0.026 | –0.717 | 0.334 | 0.032 |
Financial stress | 1–5 | O | 2.96 (1.40) | 2.60 (1.35) | 2.81 (1.38) | 2.58 (1.36) | 0.108 | –0.381 | 0.213 | 0.074 | –0.403 | 0.220 | 0.067 |
Financial difficulties | 1–4 | L | 1.93 (0.90) | 1.67 (0.73) | 1.74 (0.75) | 1.65 (0.73) | 0.204 | –0.148 | 0.047 | 0.002 | –0.149 | 0.050 | 0.003 |
Food security | 1–4 | L | 3.51 (0.77) | 3.61 (0.73) | 3.55 (0.77) | 3.59 (0.73) | 0.117 | 0.057 | 0.035 | 0.093 | 0.063 | 0.036 | 0.080 |
Thermal satisfaction | 1–5 | O | 3.45 (1.15) | 3.85 (1.10) | 3.71 (1.16) | 3.63 (1.22) | 0.462 | 1.319 | 0.216 | 0.000 | 1.342 | 0.219 | 0.000 |
Housing conditions | |||||||||||||
Satisfaction with state of repair of home | 1–5 | O | 3.26 (1.28) | 4.04 (1.06) | 3.60 (1.26) | 3.82 (1.20) | 0.440 | 1.342 | 0.222 | 0.000 | 1.352 | 0.221 | 0.000 |
Number of housing problems | 0–6 | O | 1.88 (1.53) | 1.20 (1.32) | 1.49 (1.53) | 1.32 (1.45) | 0.386 | –1.065 | 0.221 | 0.000 | –1.097 | 0.225 | 0.000 |
Number of heated rooms | |||||||||||||
Day | 0–4 | O | 2.74 (1.29) | 3.01 (1.21) | 2.69 (1.32) | 2.78 (1.28) | 0.137 | 0.387 | 0.212 | 0.067 | 0.408 | 0.213 | 0.055 |
Evening | 0–4 | O | 2.24 (1.01) | 2.31 (1.04) | 2.21 (1.01) | 2.19 (1.02) | 0.096 | 0.239 | 0.205 | 0.242 | 0.239 | 0.205 | 0.242 |
Social interactions | 0–1 | Bin | 0.24 (0.43) | 0.12 (0.33) | 0.18 (0.39) | 0.14 (0.34) | 0.195 | –1.052 | 0.429 | 0.014 | –1.149 | 0.456 | 0.012 |
The number of respondents reporting putting up with feeling cold to save heating costs decreased for the intervention group. A smaller decrease was observed for the control group. This represents a significant interaction effect at the 5% level. The interaction effect remained significant after controlling for the covariates [odds ratio (OR) 0.49, 95% CI 0.25 to 0.94]. The effect size of the interaction was small (Cohen’s d = 0.15).
A significant interaction effect was observed for self-reported financial difficulties, even after controlling for sex, age, household income, housing benefits and smoking status (B –0.15, 95% CI –0.25 to –0.05). The size of the interaction effect was small (Cohen’s d = 0.20). Table 10 shows that self-reported financial difficulties decreased to a greater extent in the intervention group than in the control group.
A significant interaction effect was observed for thermal satisfaction, which remained after controlling for the covariates (B 1.34, 95% CI 0.91 to 1.77). This is a medium-sized effect (Cohen’s d = 0.46). Although both the intervention and control groups saw an increase in thermal satisfaction, the increase was greater in the intervention group.
Significant interaction effects were observed for the two housing conditions variables. The effects remained significant after adjusting for the covariates for both people’s satisfaction with the current state of repair of their home (B 1.35, 95% CI 0.92 to 1.79) and the number of reported housing problems (B –1.10, 95% CI –1.54 to –0.66). The effects were small-to-medium sized (Cohen’s d = 0.44 and 0.39, respectively). Table 10 shows that satisfaction with the state of repair of their home increased for the intervention group but decreased for the control group. The number of reported housing problems decreased for both the intervention and control groups but decreased to a greater extent in the intervention group.
Finally, a significant interaction effect was found for social interactions, which remained after controlling for the covariates (OR 0.32, 95% CI 0.13 to 0.77). The size of the interaction effect was small (Cohen’s d = 0.19). The proportion of people feeling reluctant to invite friends or family to their home because of difficulties with keeping it warm fell to a greater extent in the intervention group than in the control group.
The impact of the intervention on health-related quality of life and QALYs
Mean baseline SF-6D scores for the intervention and control groups of 0.685 (SD 0.167) and 0.683 (SD 0.164), respectively, were observed. Mean scores for follow-up were higher for both the intervention 0.692 (SD 0.168) and control group 0.695 (SD 0.170). The baseline-adjusted QALY gain for the intervention group was 0.003, whereas the intervention group experienced an increase of 0.007 QALYs. SF-6D score at both time points, the resulting change in SF-6D, and the QALYs difference were all insignificant between groups {B –0.007 [standard error (SE) 0.016], 95% CI –0.04 to 0.02}. Accounting for covariates in a regression analysis framework resulted in an insignificant and negative coefficient for the intervention. These results suggest that the intervention did not have a significant impact on the quality of life or on the utility score of respondents.
Discussion
Key results
This was the first large quasi-experimental field study with a controlled pre-/post-test design to examine both the short-term health and psychosocial impacts of energy efficiency investments in low-income areas. This community-based study found no evidence that investments in energy efficiency improve respiratory or mental health in the short-term. Furthermore, no evidence was found that they provide physical health benefits in the short-term. However, those who received energy efficiency measures reported improved subjective well-being as compared with the controls, as well as improvements in a number of the secondary psychosocial outcomes that are conducive to better health. The study found that respondents who received the intervention also reported fewer financial difficulties, higher thermal satisfaction and higher satisfaction with the improvement of their homes. They also reported less reluctance to invite friends or family to their homes. The results were similar with or without controlling for a number of socioeconomic covariates.
Limitations
The field study in this chapter used self-completion questionnaires to be filled out by the study participants themselves. It is therefore subject to a number of biases. The study had a low initial response rate and a substantial loss to follow-up. However, the response and attrition patterns were similar for the intervention and control areas, and an analysis showed that there were only a small number of differences between study respondents and those lost to follow-up. The final samples appeared largely comparable in terms of their composition. The low response and retention rates are perhaps not completely surprising given the nature of the research. The study was conducted in low-income neighbourhoods, where response rates for mail and door-to-door data collection methods tend to be low. 114 Several strategies were used to increase the response rates, including incentives and door knocking.
In order to make the control group as comparable as possible to the intervention group, the matched control areas were selected using the same criteria as the intervention areas. This proved to be challenging, given that many comparable areas had already received energy efficiency measures through the intervention programme. A number of control areas were subsequently selected to receive energy efficiency investments in the year after the study. The composition of the final samples suggest that the control participants may be used as a robust ‘non-exposed’ comparator group.
A further limitation is the time frame of the study. Outcome measures were collected in the heating seasons directly before and after the intervention. The short follow-up period means that claims can only be made about the short-term effects of the intervention (see Interpretation). Studies with longer follow-up periods are needed to establish the long-term impacts of energy efficiency investments. 48 This has been done in the data linkage study (see Chapter 2).
According to Thomson et al. 48,115 interventions targeting at-risk populations provide clearer health benefits than area-based housing improvement programmes. The benefits of area-based programmes may be less pronounced because they do not target those who are most at need. Unlike other demand-led schemes such as Warm Homes Nest, the Warm Homes Arbed intervention was not tailored according to individual need. However, the intervention did target low-income areas with inadequate warmth owing to a large number of hard-to-heat, hard-to-treat homes within the area. Still, no clear physical health improvements were observed in this study, which may be explained by the limited follow-up period in the research. It would be useful to directly compare area-based (e.g. Warm Homes Arbed) and demand-led, targeted (e.g. Warm Homes Nest) schemes.
Researchers evaluating complex social interventions are faced with several challenges. 116,117 The study involved an evaluation of an external, in this case government-led, programme. That means that the researchers did not have control over the content or delivery of the programme. Furthermore, the intervention effects may be diluted by constant changes in the context. For example, it is possible that respondents may have independently chosen to undertake energy efficiency improvements to their home, therefore diluting the effects of the intervention programme. 117,118 However, there is no evidence that this happened in the study.
Interpretation
The study suggests that, although there is no evidence that energy efficiency investments provide physical health benefits in the short term, they improve social and economic conditions that are conducive to better health. Furthermore, they were found to be beneficial for subjective well-being. Longer-term studies are needed to establish the health impacts of energy efficiency investments. According to Thomson et al. ,48,115 most studies investigating the health impacts of housing improvements have limited follow-up periods. Studies have therefore been unable to detect longer-term health impacts. We would expect that the changes in the psychosocial outcomes are conducive to better health in the longer term. The observed changes in the social outcomes may be a valuable indicator of the potential for longer-term health benefits.
Generalisability
The results are likely to be generalisable to other low-income areas with inadequate warmth and other vulnerable at-risk populations. The public health implications are typical for other deprived areas across the UK with low-quality housing. The results may be less generalisable to other less-deprived areas.
Chapter 4 The household monitoring study
This chapter contains material from Poortinga et al. 119 © 2017 The Author(s). Published by Informa UK Limited, trading as Taylor & Francis Group. This is an Open Access article distributed under the terms of the Creative Commons Attribution License (http://creativecommons.org/licenses/by/4.0/), which permits unrestricted use, distribution, and reproduction in any medium, provided the original work is properly cited.
Introduction
This chapter follows the STROBE reporting guidelines for observational study designs. 81
Background
It is widely acknowledged that living in cold conditions poses various health risks, in particular to low-income, fuel-poor households. 6 Improving the energy efficiency of the housing stock might bring multiple positive health gains through improved hydrothermal conditions and lower financial stress through reduced fuel consumption. More recently, there have been suggestions that increasing the energy efficiency of a home could have detrimental effects on people’s health. 64 Reduced ventilation through insulation and draughtproofing may increase RH levels65 and, as a result, promote mould growth. 66,67
Only a handful of studies to date have attempted to identify and measure the impact of affordable warmth interventions on internal conditions and fuel consumption using a number of monitoring methodologies and data analysis techniques. Most research in this area has been cross-sectional,71 reported means and/or temperature frequencies,49,120 monitored events for a short period only60 or did not include control households. 63,121 Furthermore, most studies did not make use of the granularity of the measurements, consider the non-independent repeated nature of monitoring data or control systematically for external conditions.
This household monitoring study addresses some of these issues through detailed long-term household monitoring of intervention and control households at baseline and follow-up. Both the internal and the external hydrothermal conditions are measured at a higher resolution than previous research and combined into a comprehensive data set that was amenable to multilevel interrupted time series analysis. 122 This approach allows the impact of the energy efficiency interventions to be estimated with a maximum level of statistical power, adjusting for external conditions at the same level as the internal measurements.
Objectives
This chapter describes the methodology and findings of the household monitoring study. The study had the overall aim to examine the impact of the second phase of the intervention programme on internal household conditions and energy use. It set out to determine the impact of (1) the intervention on whole-house indoor air temperature and RH; (2) individual measures on whole-house indoor air temperature and RH; (3) the intervention on indoor temperatures at different times of the day; (4) the intervention on indoor temperatures in different rooms within the home; (5) the intervention under different external hydrothermal conditions; (6) the intervention on the average daily duration and cumulative substandard internal hydrothermal conditions; and (7) the intervention on household energy use, in particular gas usage.
The research described in this chapter received ethics approval from the SREC of the Welsh School of Architecture, Cardiff University (EC1308.160).
Methods
Study design
The household monitoring study uses a quasi-experimental controlled before-and-after design consisting of long-term hydrothermal condition monitoring in two subsequent heating seasons. The monitoring took place in a number of households before and after they received energy efficiency improvements under the intervention programme. The study further included a number of control households that did not receive such improvements. The indoor measurements for the control households were taken at the same time as for the intervention households. All households for the monitoring study were recruited from the community sample described in Chapter 3.
Setting
The study was conducted in five low-income areas where energy performance investments were scheduled and in five matched control areas where no such investments were planned (Figure 8). The baseline measurements were taken in the 2013/14 heating season. The follow-up measurements were taken in the 2014/15 heating season.
FIGURE 8.
Locations of the intervention and control areas.
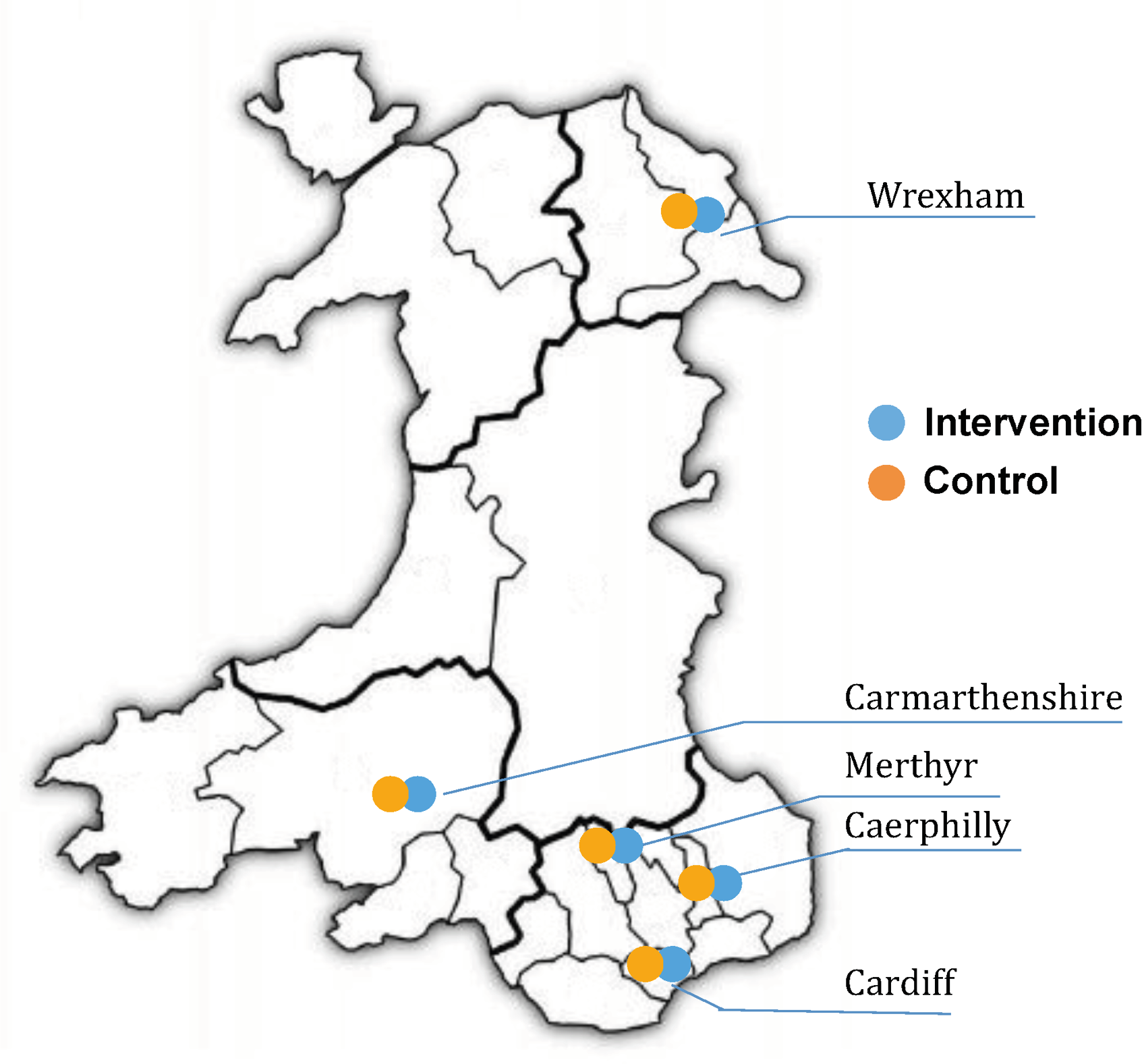
The five intervention and control areas were selected from year 2 schemes included in the community-based study (see Chapter 3). The intervention areas and their matched control areas were in Brynamman (Carmarthenshire), Caerau (Cardiff), Llay (Wrexham), Hollybush (Caerphilly) and Pennydarren (Merthyr Tydfil). The areas were selected based on the scheduling of the intervention work and the returns of the baseline questionnaires from the community-based study. This was to ensure that a sufficient number of households could be recruited in time for the monitoring to take place in the 2013/14 heating season. The communities varied in terms of their location, climatic conditions and dominant housing type. The Cardiff and Merthyr Tydfil areas consisted predominantly of British steel-framed [British Iron and Steel Federation (BISF)] houses, Caerphilly and Carmarthenshire were off-gas areas and Wrexham consisted predominantly of terraced houses with solid walls. The control areas were selected to resemble the intervention areas as much as possible in terms of housing type and level of deprivation (see Chapter 3).
Respondents from the selected areas who provided consent to be recontacted were invited by telephone to have their house monitored. In total, 99 households agreed to take part in the study, of which 50 were located in the intervention areas and 49 in the matched control areas. Households were visited between 11 January and 1 February 2014 to install indoor data loggers, and again between 25 March and 17 April 2014 to collect them. In the baseline period, houses were monitored for a minimum of 28 consecutive days and a maximum of 71 days. On average, the houses were monitored for 46 days, with a SD of 9 days.
The households that took part in the first part of the study were recontacted by letter prior to the 2014/15 heating season for follow-up measurements. The reminder letter was followed up by a telephone call to arrange an installation visit. Households were visited between 1 November and 18 November 2014 to install indoor data loggers, and again between 12 April and 30 April 2015 to collect them. In the follow-up period, houses were monitored for a minimum of 97 days and a maximum 181 days. On average, houses were monitored for 127 days (SD 32 days). Loss to follow-up was low (11%), resulting in a final sample of 88 households (intervention, n = 48; control, n = 40). Local weather stations were installed in or close to the five monitoring areas to record external meteorological conditions during the baseline and follow-up periods. Gas and electricity meter readings were taken during the installation and collection visits.
Participants
Households for the monitoring study were recruited from the community sample described in Chapter 3. The aim was to recruit 100 households for the monitoring study, with a subset of households undergoing energy efficiency improvements (n = 50) and a control group that did not receive such improvements (n = 50).
Variables
The following exposure intervention and outcome variables were included in the analyses.
Intervention measures
The intervention measures included external wall insulation (with mechanical ventilation), new windows and doors, boiler and heating system upgrades, connection to the gas mains network and installation of voltage optimisers. The measures were recorded for each participating household. In this study, 35 households received external wall insulation, nine received new windows and doors, 48 received a new boiler or heating system and 46 had a voltage optimiser installed. Furthermore, 20 properties from two intervention areas were connected to the mains gas network. Figure 9 shows that, on average, the measures increased the Standard Assessment Procedure ratings of the intervention households from 52 (SD 12) to 66 (SD 5). This is an average increase from Energy Performance Certificate rating band E to band D. The Standard Assessment Procedure was developed by the Building Research Establishment and is currently the methodology used by the UK Government to assess the energy performance of dwellings. 123 It presents the energy performance of homes in a figure ranging from 1 to 100. This has been subdivided into seven bands ranging from A to G.
FIGURE 9.
Standard Assessment Procedure ratings of intervention households before and after installation of the energy efficiency measures.
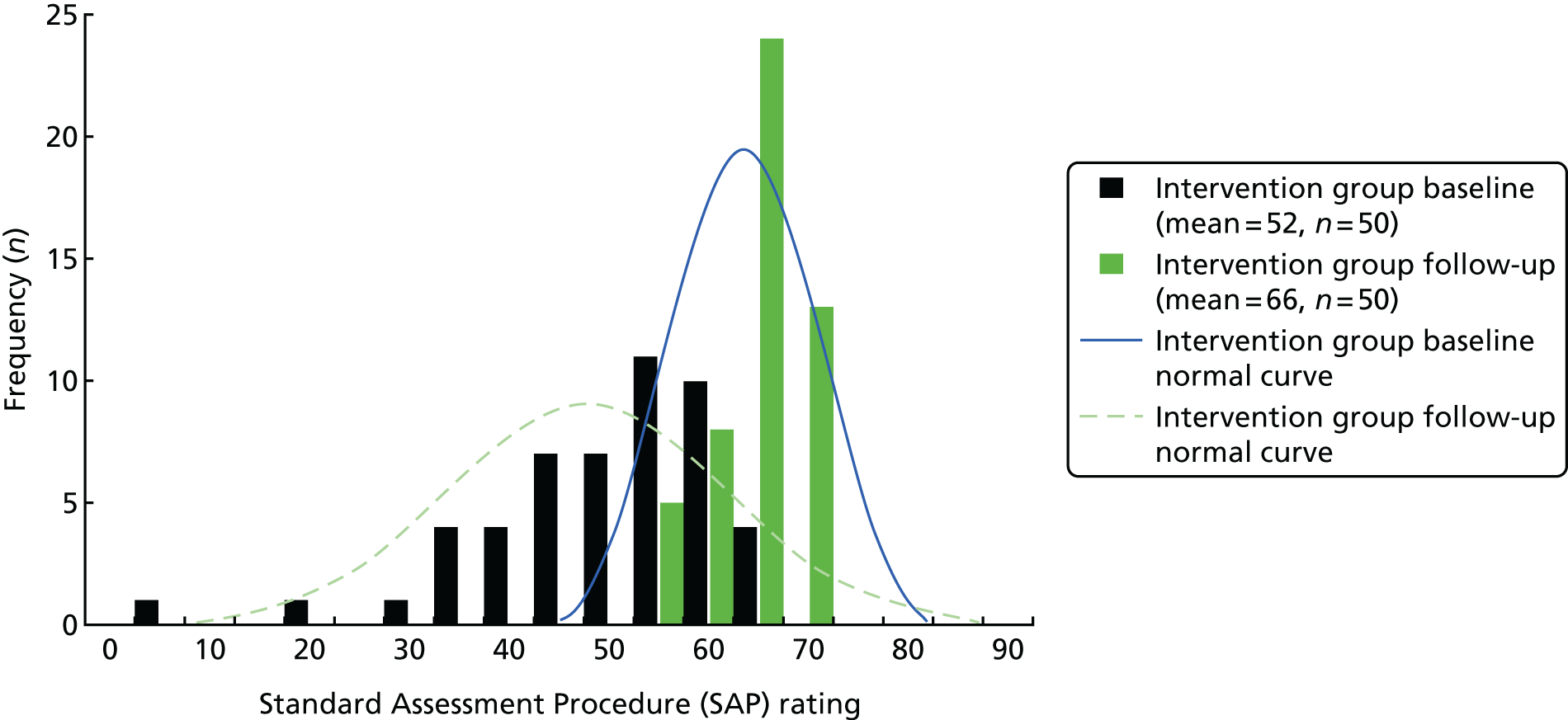
Indoor air temperature and relative humidity
The main outcomes of the household monitoring study were indoor air temperature and RH at different times of the day and in different rooms within the home. These were measured with three Tinytag Ultra 2 data loggers (Gemini Data Loggers, Chichester, West Sussex, UK) that were positioned in the living room, kitchen and main bedroom, respectively. These rooms were selected as they represent the key living spaces in domestic dwellings. The data loggers were positioned away from any direct heat source and external windows, in a location where they would cause the least disturbance to the occupants and were unlikely to get covered, typically on top of a cupboard or shelf at a height of about 2 metres. Owing to practical issues when placing loggers in households with diverse circumstances, furnishings and personal preferences, the exact locations where they were positioned within rooms varied. Indoor air temperature and RH were recorded every 15 minutes.
The data were used to calculate the average daily indoor air temperature and RH, as well as the average indoor air temperature and RH in the morning (06.00–09.00), during the day (09.00–18.00), in the evening (18.00–23.00) and at night (23.00–06.00). The data were used for the three rooms separately and combined to calculate a whole-house average.
The Tinytag Ultra 2 data loggers have an air temperature reading range of –25 °C to 85 °C, with a resolution of 0.01 °C and an error range of ± 0.35 °C, and a RH reading range of 0% to 95%, with a resolution of 0.3% and an error range of ± 3.0% at 25 °C.
Duration and cumulative substandard internal conditions
The study further explored the impact of the intervention on the duration and cumulative substandard internal conditions.
The duration of substandard internal conditions was determined by recording each day the time that the indoor air temperature dropped to < 18 °C and dropped to < 16 °C and that the indoor RH was > 60%. These thresholds were based on the literature, which shows that indoor air temperatures in winter of ≥ 18 °C pose minimal risk to the health of a sedentary person and to people aged > 65 years or with pre-existing medical conditions. 124 Indoor air temperatures of < 18 °C increase the risk of high blood pressure and this risk is heightened in temperatures of < 16 °C. Indoor air temperatures of < 16 °C may further diminish resistance to respiratory diseases. 125 RH levels of > 60% have been linked to respiratory and allergic conditions, as well as fungal growth and house dust mite infestations. 66,67
The cumulative substandard internal conditions is the time intensity integral of substandard conditions beyond the chosen thresholds, thus representing the daily dose of substandard internal conditions to which householders are potentially exposed. The cumulative substandard indoor air temperature reflects the amount of underheating over the period of 1 day. The cumulative substandard indoor RH reflects the total amount of exposure to risky humidity levels.
The cumulative substandard internal conditions are expressed as °C·hour and % RH·hour for indoor air temperature and RH, respectively.
Outdoor air temperature and relative humidity
Outdoor air temperature and RH were measured by local weather stations that were installed in or close to the five monitoring areas, typically in a participating household’s garden. Outdoor air temperature and RH were recorded every 15 minutes by local Delta-T-GP1 weather stations (Delta-T Devices, Burwell, Cambridgeshire, UK). Outdoor air temperature and RH were recorded for the full baseline and follow-up periods for each of the five locations.
The measurements were combined to calculate the average whole-day outdoor air temperature and RH, and to calculate the average outdoor air temperature and RH in the morning (06.00–09.00), during the day (09.00–18.00), in the evening (18.00–23.00) and at night (23.00–06.00).
Delta-T-GP1 weather stations have an air temperature reading range of –20 °C to 70 °C, with a resolution of 0.05 °C and an error range of ± 0.3 °C, and a RH reading range of 0–100%, with a resolution of 0.2% and an error range of ± 2% between 5% and 95% and of ± 2.5% for < 5% and > 95% RH.
Heating demand: heating degree days
The outdoor air temperature measurements were subsequently converted into daily heating degree days (HDDs). 126 HDDs reflect the demand for energy needed to heat buildings over a specific period, in this case 1 day. The heating demand is calculated by summing the differences between the outdoor air temperature and a reference temperature. As such, the HDD measure is an exposure measure reflecting the cumulative amount of degrees the temperature falls below the base temperature over 1 day. The reference temperature, 15.5 °C in the UK, reflects the outdoor temperature at which, generally, no heating is needed to maintain comfortable internal conditions. 127,128 In this study, HDDs are calculated as the mean temperature difference for the 96 daily readings, similar to the mean degree hour method, which is considered the most detailed method of calculating degree days. 126 The outside temperature is only included in the calculations when it is lower than the reference temperature.
Heating degree days provide some advantages over other methods that use mean outdoor temperatures to calculate energy demand: they take account of fluctuations in outdoor air temperature and exclude periods when space heating is not needed, therefore capturing extreme conditions in a way that mean temperature methods cannot. This makes them more reliable in predicting energy consumption, particularly in milder conditions and in periods with fluctuating or extreme cold snaps, when they capture both the magnitude and duration of an event. HDDs also have a number of shortcomings. 129 They are based on assumptions about when additional energy is needed to heat a building and ignore the fact that some buildings are only heated during specific periods. Furthermore, they do not reflect variations in the ability of different buildings to retain heat or to exploit solar gains.
Average daily gas usage
Average daily gas usage was calculated from meter readings taken during the installation and collection visits for both the baseline and follow-up periods. The change in average daily gas usage provides an indication of the effectiveness of the energy performance investments, as most of the metred gas will have been used for space and water heating. It was not possible to take gas meter readings in off-gas areas.
Bias
The household monitoring study was undertaken in a subset of households that had already participated in the community-based study. The biases described in Chapter 3 therefore also apply to this part of the project.
Another potential bias was that the local climate could lead households in different areas to be exposed to different external hydrothermal conditions during the baseline and follow-up. To control for such biases, the study compared internal conditions of the intervention households with the control households located within the same areas. Furthermore, internal hydrothermal conditions were adjusted for external hydrothermal conditions as measured by weather stations installed in or near the different monitoring areas. This design minimised differences between households resulting from external hydrothermal conditions.
Selection and attrition bias have the potential to overestimate and underestimate the effects of the intervention. The household monitoring was undertaken in a subset of households that had already participated in the community-based study (see Chapter 3).
There was a risk of selection bias in the households agreeing to their house being monitored, as well as attrition bias between the baseline and follow-up monitoring. Households that had completed the questionnaire and consented to be recontacted were invited to participate in the monitoring study. The monitoring households and the overall sample from which they were selected were broadly similar in terms of their building and sociodemographic characteristics. The monitoring sample contained a higher percentage of terraced houses (47.9% vs. 29.0%) and private rentals (11.5% vs. 4.3%) than the overall area sample. Figure 10 shows that the response rate for the intervention group (23.3%) was slightly lower than that for the control group (30.2%). However, that was mainly owing to the size of the area sample from which they were recruited. Attrition was higher for the control households (n = 9) than for the intervention households (n = 2), but no monitored area lost more than four households. A loss to follow-up analysis showed that attrition did not bias the samples in a systematic way. The final intervention and control samples were comparable in terms of building age, building type, number of bedrooms, tenure and household composition (i.e. with or without children) (see Table 11).
FIGURE 10.
The flow diagram for the household monitoring study.
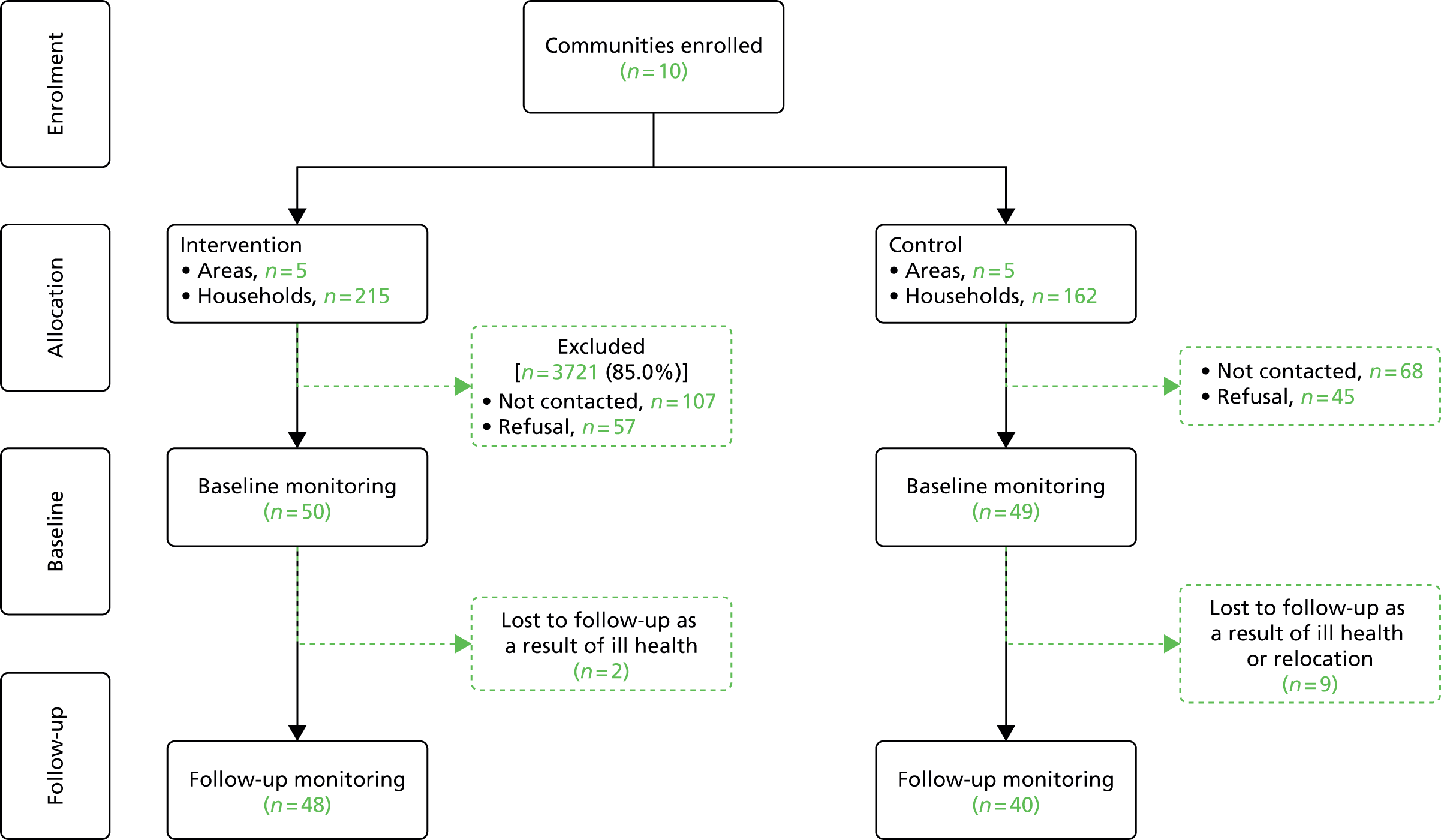
Study size
The study set out to monitor 100 properties over a 4-week period in two subsequent heating seasons. The 15-minute interval readings were combined to calculate whole-day averages, as well as averages for 4-day segments (i.e. morning, day, evening and night). This would ensure that the statistical analyses were based on ≥ 5600 data points clustered within the 100 households.
The eventual sample consisted of 99 households that were monitored for an average of 46 days (SD 9 days) during the baseline period and 88 households that were monitored for an average of 127 days (SD 32 days) for the follow-up period. This created a database with 15,771 data points for the different hydrothermal outcome variables after the removal of missing day values. All measurements were included in the final data set. This means that all 99 households contributed to the baseline estimates, but only the measurements of the 88 households included in both the baseline and follow-up contributed to the estimates for changes over time. This means that the parameters reported in this report were based only on the monitoring data of 88 households for which we had both baseline and follow-up periods.
Statistical methods
The data were analysed by constructing a series of controlled multilevel interrupted time series regression models, with daily internal conditions (level 1) nested within households (level 2) that either received an intervention or did not. The nested multilevel design makes it possible to take account of the clustering of the observations over time using random effects. 130 The approach also enables the handling of unbalanced data when the number of observations differ for the different households and time periods. 130 This makes the approach suitable for analysing monitoring data of multiple properties with different start and end dates.
Analyses were conducted with the MLwiN version 2.36 software package. 109 The software package is specifically designed for fitting multilevel models, in this case an interrupted time series regression analysis. The analysis involved the use of the time series of the daily averaged hydrothermal conditions that were measured during the baseline and follow-up periods in the intervention and control households. The interruption occurred between baseline and follow-up sampling periods when intervention households had improvement work done to their homes. Therefore, the interruption in the ‘interrupted time series’ refers to the energy efficiency improvements undertaken in the intervention households. This was then compared with control households that did not receive the energy efficiency investments during that period.
The basic statistical models included three independent variables: (1) the intervention group, (2) the measurement period and (3) an interaction between measurement period and intervention group. The intervention group variable indicated whether the measurements were taken in an intervention or a control household. The measurement period variable indicated whether the measurements were taken in the baseline or the follow-up period. The interaction term of the measurement period and intervention group variables indicated that the intervention has taken place in the follow-up period for the intervention households. The regression coefficient related to this term shows the level of change in internal conditions for the intervention group relative to the control group.
The statistical models were further controlled for external conditions. This was done by including the daily averaged external measurements as independent variables. The models with indoor air temperature as the outcome variable included daily HDD values as a covariate to control for external thermal conditions. The models with indoor RH as the outcome variable additionally included a measure of the average daily outdoor RH to control for external hydrological conditions.
Interrupted time series analyses typically include a time variable (indicating the time elapsed since the start of the study, as measured in days) and a time after the interruption variable (indicating the time elapsed since the intervention, as measured in days) in order to identify trends over time and changes in the trend after the intervention, respectively. 131 However, as no obvious trend over time was observed within the baseline and follow-up periods, these terms were excluded from the regression models.
One problem with repeated measurement data is that the measurements are often not independent, which violates one of the assumptions of ordinary least squares regression. Autocorrelation within time series, when measurements close to one another are more similar than measurements that are further apart, may lead to increased type 1 errors. The autocorrelation function and partial autocorrelation function in MLwiN indicated autocorrelation with a diminishing lag. Autocorrelation reflects the internal correlation within a time series, showing the degree to which the different measurements are interdependent. 131 An autoregressive model was constructed by adding a weight specifying that the error covariance decreases as the time distance between measurements increases in order to control for the observed dependency. 132
Results
Participants
The household monitoring study took place in five intervention areas and their matched control areas nearby (see Figure 8). Respondents from these areas who provided consent to be recontacted were invited to have their home monitored. In total, 202 households were invited by telephone to participate, of which 99 agreed to have their house monitored (intervention, n = 50; control, n = 49). This corresponds to a response rate of 49%. The rest of the households were not contacted, either because they did not provide a contact telephone number or because the maximum number of households for the area was reached. Eleven households were lost to follow-up owing to ill health or relocation (intervention, n = 2; control, n = 9). This means that 99 households were monitored at baseline and 88 households at follow-up. Figure 10 shows the flow of households through the monitoring study.
Descriptive data
Table 11 summarises the characteristics of the intervention and control households at baseline and follow-up. It shows that there were no statistically significant differences between the two groups in terms of building age, building type, number of bedrooms, tenure and household composition.
Characteristics | Study cohort, % (n/N) | |||
---|---|---|---|---|
Intervention | Control | |||
Baseline | Follow-up | Baseline | Follow-up | |
Year of construction | ||||
Before 1919 | 22.5 (11/49) | 28.9 (13/45) | 39.1 (18/46) | 35.9 (45/39) |
1919–1945 | 28.6 (14/49) | 20.0 (9/45) | 32.6 (15/46) | 33.3 (14/39) |
1945–1965 | 32.65 (16/49) | 37.8 (17/45) | 0.0 (0/46) | 0.0 (0/39) |
1965–1979 | 8.2 (4/49) | 8. 9 (4/45) | 26.1 (12/46) | 28.2 (11/39) |
1980 or later | 8.2 (4/49) | 4.4 (4/45) | 2.2 (1/46) | 2.6 (1/39) |
Building type | ||||
Detached house | 4.0 (2/50) | 4.4 (2/46) | 4.1 (2/49) | 4.8 (2/42) |
Semi-detached house | 42.0 (21/50) | 47.8 (22/46) | 49.0 (24/49) | 40.5 (17/42) |
Terraced house | 52.0 (26/50) | 45.7 (21/46) | 40.82 (20/49) | 47.6 (20/42) |
Bungalow | 0.0 (0/50) | 0.0 (0/46) | 2.0 (1/49) | 2.4 (1/42) |
Purpose-built flat | 2.0 (1/50) | 2.2 (1/46) | 4.1 (2/49) | 4.8 (2/42) |
Construction type | ||||
British steel framed | 30.0 (15/50) | 32.6 (15/46) | 38.8 (19/49) | 42.9 (18/42) |
Masonry solid wall | 60.0 (30/50) | 58.7 (27/46) | 57.1 (28/49) | 54.8 (23/42) |
Masonry cavity wall | 10.0 (5/50) | 8.7 (4/46) | 4.1 (2/49) | 2.4 (1/42) |
Number of bedrooms | ||||
1 | 2.0 (1/49) | 2.2 (1/45) | 4.1 (2/49) | 4.8 (2/42) |
2 | 8.2 (4/49) | 8.9 (4/45) | 10.2 (5/49) | 9.5 (4/42) |
≥ 3 | 89.8 (44/49) | 89.0 (40/45) | 85.7 (42/49) | 85.7 (36/42) |
Tenure | ||||
Owner occupied | 61.2 (30/50) | 57.8 (26/45) | 61.2 (30/49) | 61.9 (26/42) |
Private rental | 14.3 (7/50) | 11.1 (5/45) | 8.2 (4/49) | 11.9 (5/42) |
LA rental | 24.5 (12/50) | 24.4 (11/45) | 28.6 (14/49) | 26.2 (11/42) |
Housing association rental | 2.0 (1/50) | 6.7 (3/45) | 2.0 (1/49) | 0.0 (0/42) |
Household composition | ||||
Without children | 68.8 (33/48) | 67.4 (31/46) | 81.3 (39/48) | 80.5 (33/41) |
With children | 31.3 (15/48) | 32.6 (15/46) | 18.8 (9/48) | 19.5 (8/41) |
Table 12 shows the average internal conditions for the intervention and control households at baseline and follow-up, unadjusted for external conditions. The table suggests that indoor air temperatures increased for the intervention group but decreased for the control group between baseline and follow-up. The changes in indoor RH were less pronounced. Small reductions were observed for both the intervention and the control groups. The internal conditions presented in the table are not adjusted for external hydrothermal conditions, which may differ across the monitoring periods and areas.
Outcome | Study cohort, M (SD) | |||
---|---|---|---|---|
Intervention | Control | |||
Baseline | Follow-up | Baseline | Follow-up | |
Average indoor air temperature (°C) | ||||
Whole house | ||||
Overall | 18.35 (2.99) | 19.03 (2.58) | 17.62 (2.59) | 17.30 (2.87) |
Morning | 17.23 (3.32) | 17.95 (2.91) | 16.27 (2.67) | 16.12 (2.97) |
Day | 18.45 (3.09) | 19.10 (2.59) | 17.64 (2.89) | 17.42 (2.92) |
Evening | 19.09 (3.02) | 19.89 (2.81) | 18.62 (2.81) | 18.12 (3.13) |
Night | 18.18 (3.09) | 18.93 (2.70) | 17.48 (2.46) | 17.06 (2.93) |
Living room daily | 18.82 (3.11) | 19.43 (2.85) | 18.32 (2.51) | 18.12 (3.00) |
Bedroom daily | 18.37 (3.38) | 19.09 (3.11) | 17.63 (3.14) | 16.57 (3.39) |
Kitchen daily | 17.86 (3.54) | 18.69 (3.12) | 16.92 (3.65) | 17.20 (3.63) |
Average indoor RH (%) | ||||
Whole house | ||||
Overall | 55.71 (11.48) | 53.26 (11.35) | 57.86 (11.17) | 56.50 (11.70) |
Morning | 56.42 (12.41) | 53.57 (12.01) | 58.99 (11.18) | 57.00 (11.97) |
Day | 55.22 (11.40) | 53.00 (11.11) | 57.54 (11.20) | 55.89 (11.62) |
Evening | 55.91 (11.48) | 53.52 (11.76) | 57.67 (11.79) | 56.88 (12.12) |
Night | 55.99 (11.95) | 53.27 (11.74) | 57.92 (11.33) | 56.80 (12.03) |
Living room daily | 53.30 (12.44) | 52.17 (11.90) | 55.75 (11.60) | 53.35 (12.54) |
Bedroom daily | 56.78 (12.95) | 53.47 (12.40) | 57.85 (11.55) | 59.32 (12.69) |
Kitchen daily | 57.12 (12.37) | 54.14 (12.72) | 59.96 (14.75) | 56.83 (13.84) |
Table 13 shows the distribution of internal conditions for the intervention and control groups at baseline and follow-up, again unadjusted for external conditions. The figures represent the proportion of measurements falling into the different indoor air temperature and RH bands. The distribution of indoor air temperature was similar for the two groups at baseline [χ2(3) = 1.761; p = 0.623] but differed at follow-up [χ2(3) = 18.231; p = 0.000]. The proportion of substandard indoor air temperature measurements decreased for the intervention group but increased for the control group. In contrast, the proportion of indoor air temperature within the 18–24 °C band (the recommended comfort zone) increased for the intervention group but decreased for the control group.
Outcome | Study cohort (%) | |||
---|---|---|---|---|
Intervention | Control | |||
Baseline | Follow-up | Baseline | Follow-up | |
Indoor air temperature °C | ||||
< 16 | 23.5 | 11.0 | 27.0 | 30.4 |
16–18 | 20.3 | 18.5 | 26.3 | 26.6 |
18–24 | 55.1 | 68.5 | 45.5 | 42.9 |
> 24 | 1.2 | 2.0 | 1.1 | 0.1 |
Indoor RH (%) | ||||
< 40 | 5.2 | 9.8 | 3.5 | 6.1 |
40–50 | 30.3 | 34.3 | 26.5 | 25.3 |
50–60 | 30.7 | 29.3 | 25.1 | 34.8 |
> 60 | 35.4 | 25.0 | 45.0 | 33.8 |
Furthermore, Table 13 shows that the proportion of substandard RH measurements (i.e. > 60% RH) decreased for both the intervention and control groups. The distribution of indoor RH levels was similar at baseline [χ2(3) = 2.659; p = 0.447] and at follow-up [χ2(3) = 3.001; p = 0.391].
Main results
Indoor air temperature
Figure 11 shows the estimates and 95% CIs of the measurement occasion × intervention group interactions for the overall average whole-house temperature, and for the average whole-house temperatures in the morning, day, evening and night, respectively. These interactions indicate the levels of change in indoor air temperature observed in the intervention households in comparison to the control households. The figure shows that, on average, the intervention increased the indoor air temperature by 0.84 °C (95% CI 0.64 to 1.04 °C). The largest changes were observed in the evening (1.17 °C, 95% CI 0.94 to 1.39 °C) and at night (1.01 °C, 95% CI 0.79 to 1.24 °C). Slightly smaller changes were observed in the morning (0.51 °C, 95% CI 0.26 to 0.75 °C) and during the day (0.62 °C, 95% CI 0.40 to 0.85 °C).
FIGURE 11.
Relative change in daily average indoor air temperature during different times of the day for the intervention households.
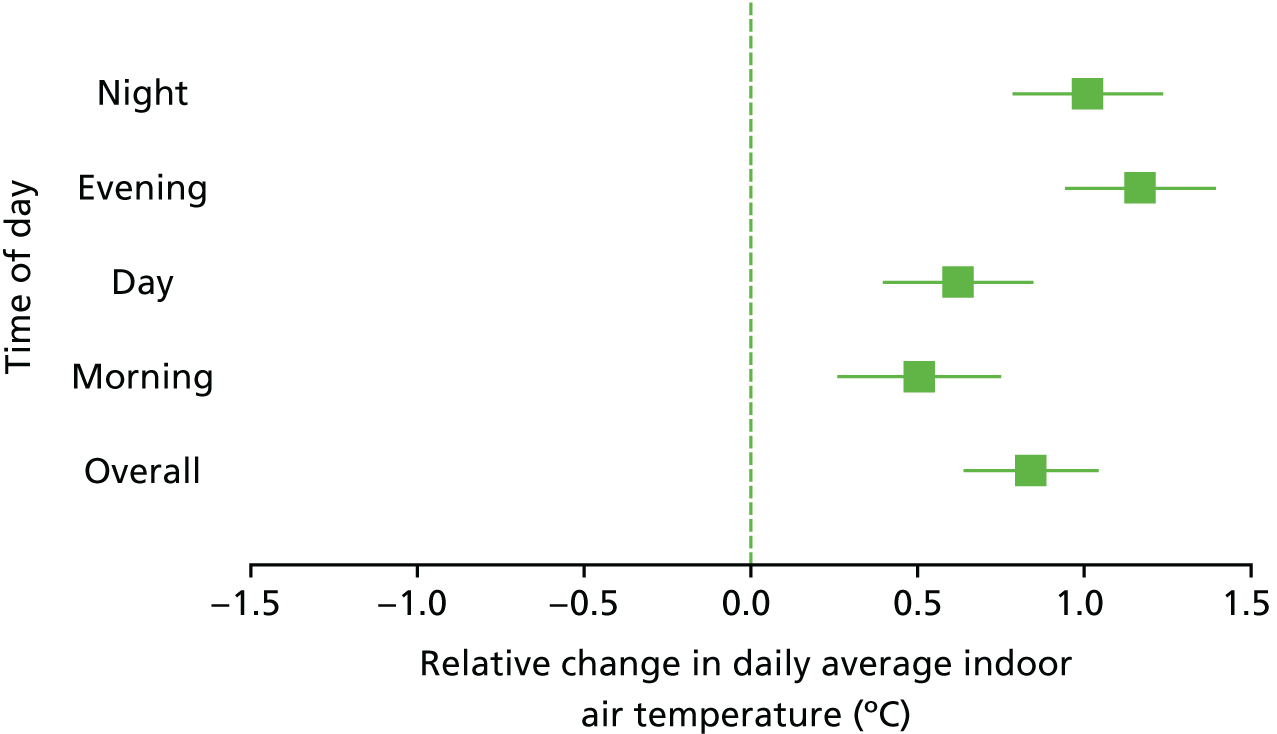
Figure 12 shows that significant increases in indoor air temperatures were found for the living room and bedroom. On average, the intervention increased the indoor air temperature of the living room by 1.01 °C (95% CI 0.78 to 1.23 °C) in comparison to the control households. The largest change was observed in the bedroom: bedroom temperature in intervention households increased by an average of 1.28 °C in comparison to control households (95% CI 1.04 to 1.52 °C). The increases in the kitchen were the smallest and non-significant (0.24 °C, 95% CI –0.01 to 0.48 °C).
FIGURE 12.
Relative change in daily average indoor air temperature for different rooms of the intervention households.
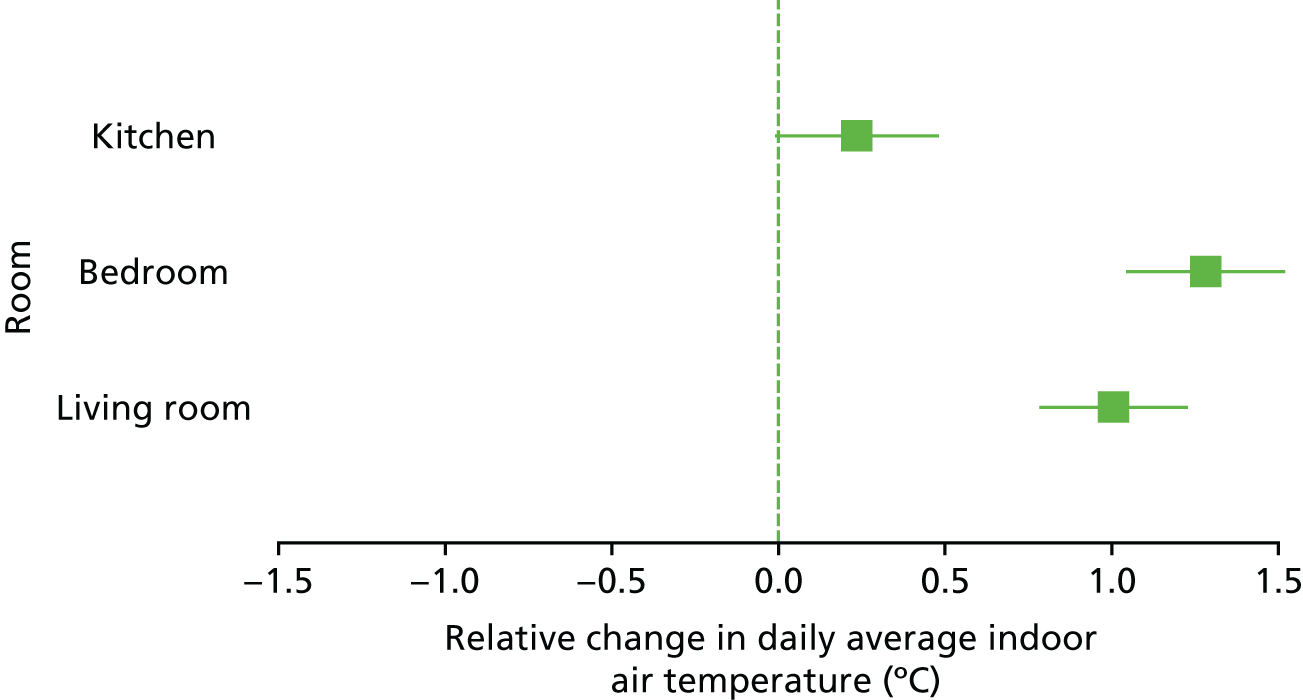
Figure 13 shows that some intervention measures were more effective than others in raising indoor air temperatures. External wall insulation produced the largest increase in indoor air temperature (1.12 °C, 95% CI 0.69 to 1.55 °C). Connecting a property to the gas mains network also increased the indoor air temperature significantly by an average of 0.69 °C (95% CI 0.29 to 1.09 °C). A new boiler or heating system (–0.19 °C, 95% CI –0.69 to 0.31 °C) and new windows and doors (–0.02 °C, 95% CI –0.39 to 0.35 °C) did not increase indoor air temperatures significantly.
FIGURE 13.
Relative change in daily average indoor air temperature for different intervention measures.
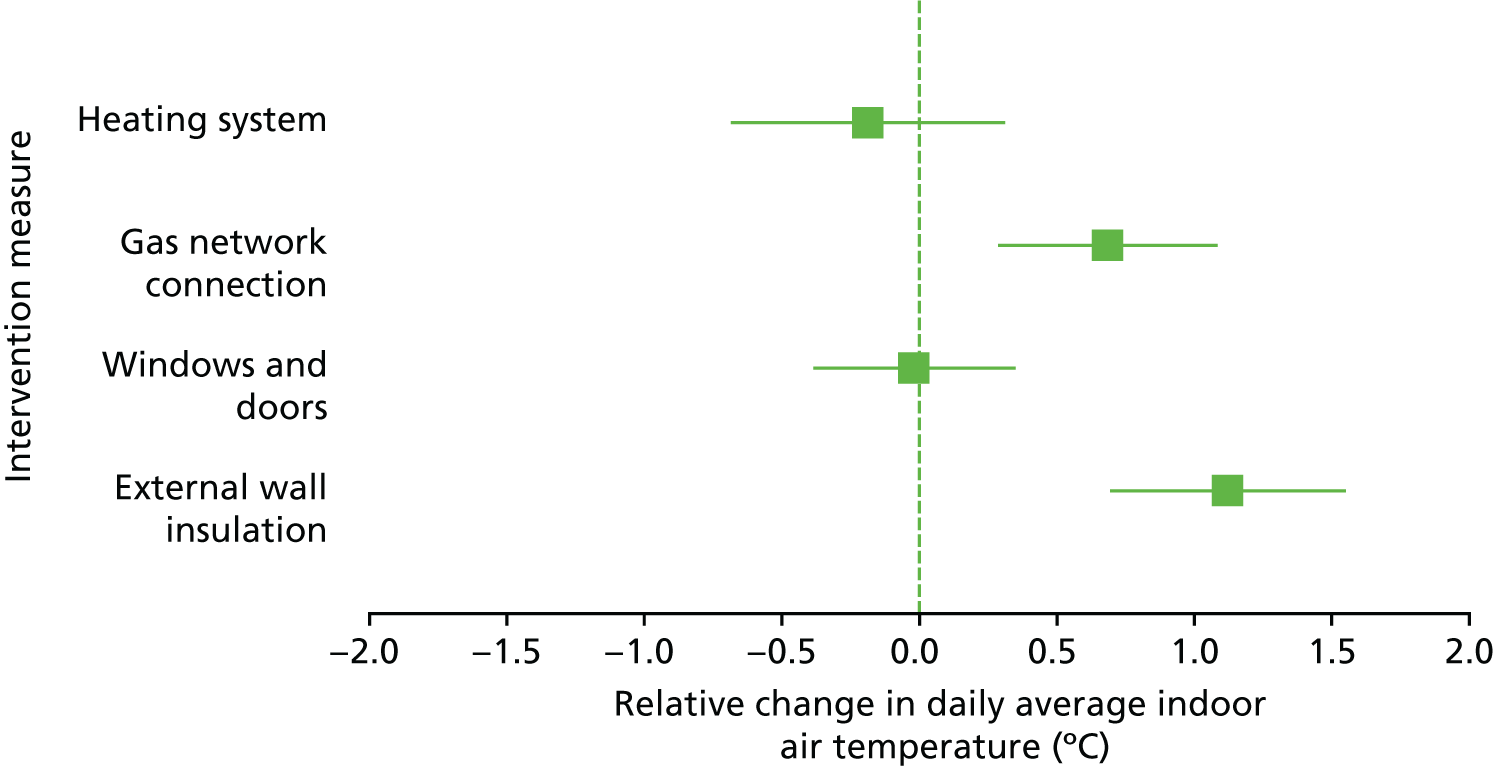
The effects of the intervention on indoor air temperatures were different for the different building construction types. Figure 14 shows that the intervention increased indoor air temperatures in buildings with solid walls (1.54 °C, 95% CI 1.26 to 1.83 °C) and in British steel-framed (BISF) buildings (0.74 °C, 95% CI 0.51 to 0.96 °C). The intervention did not increase indoor air temperature in buildings with cavity walls (–0.17 °C, 95% CI –0.58 to 0.25 °C).
FIGURE 14.
Relative change in daily average indoor air temperature for different construction types.
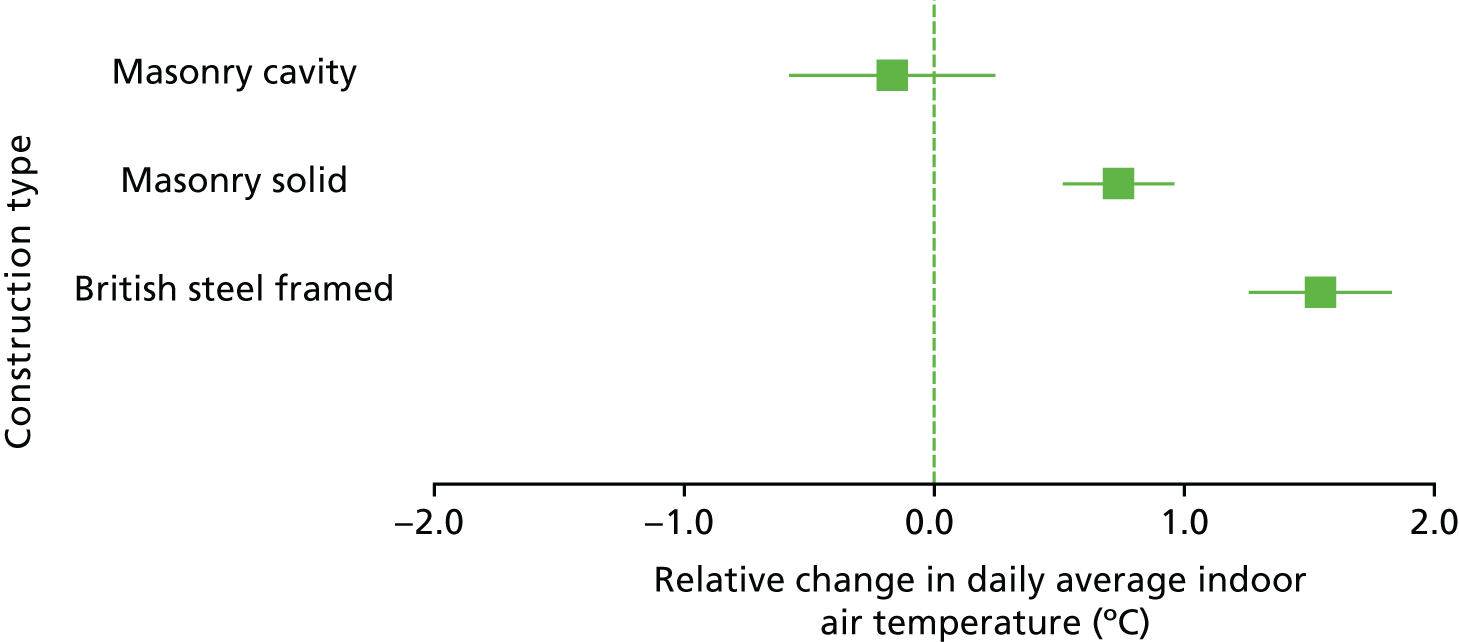
Figure 15 shows the change in indoor air temperatures as a result of the intervention under different heating demand conditions. The increases in indoor air temperature ranged between 0.59 °C and 1.03 °C, and were the highest for the lower heating demand conditions (i.e. below 6 HDDs and between 6 and 8 HDDs).
FIGURE 15.
Relative change in daily average indoor air temperature under different heating demand conditions for the intervention households.
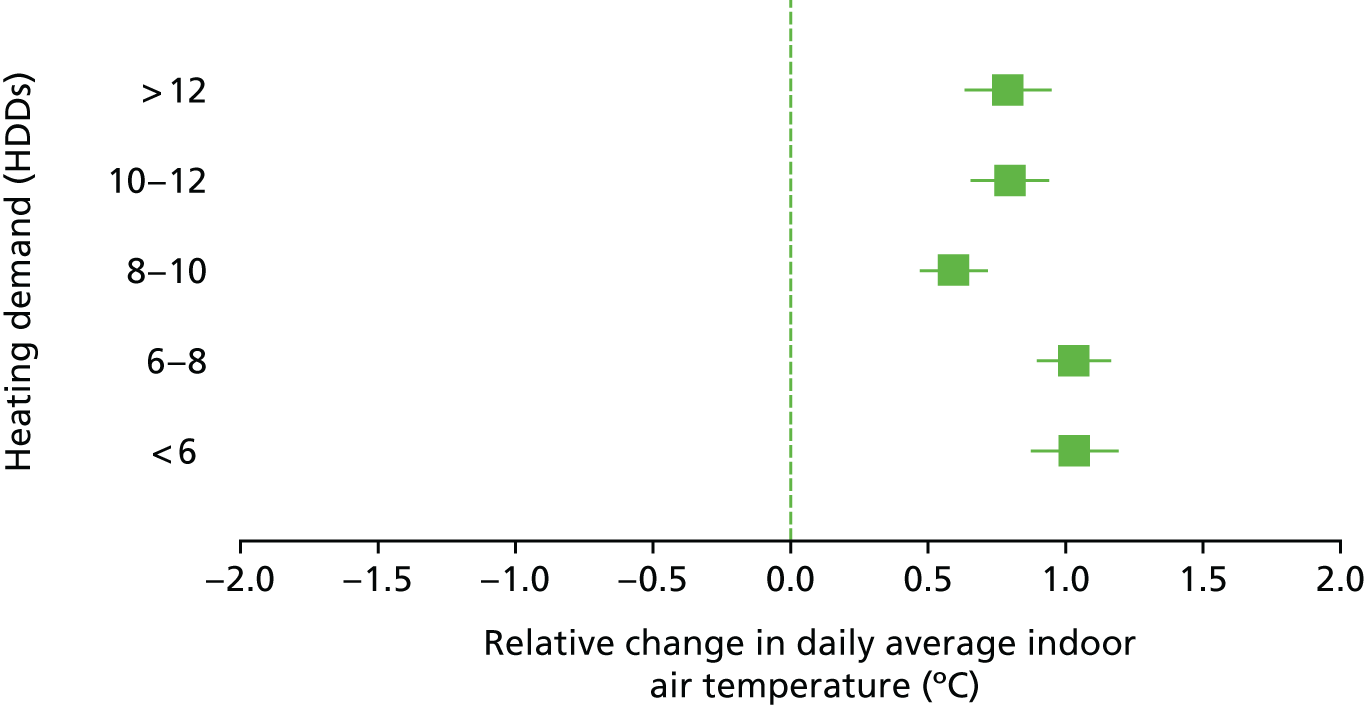
Indoor relative humidity
Figure 16 shows that, on average, the intervention increased indoor humidity levels by 0.04% RH in absolute terms. The increase in the intervention households was not significantly different from the one observed for the control households (95% CI –0.74% to 0.83% RH). The figure further shows that the changes were consistent for different levels of outdoor RH conditions. None of the changes differed significantly from the changes observed for the control households under the same conditions.
FIGURE 16.
Relative change in daily average indoor RH under different external RH conditions for the intervention households.
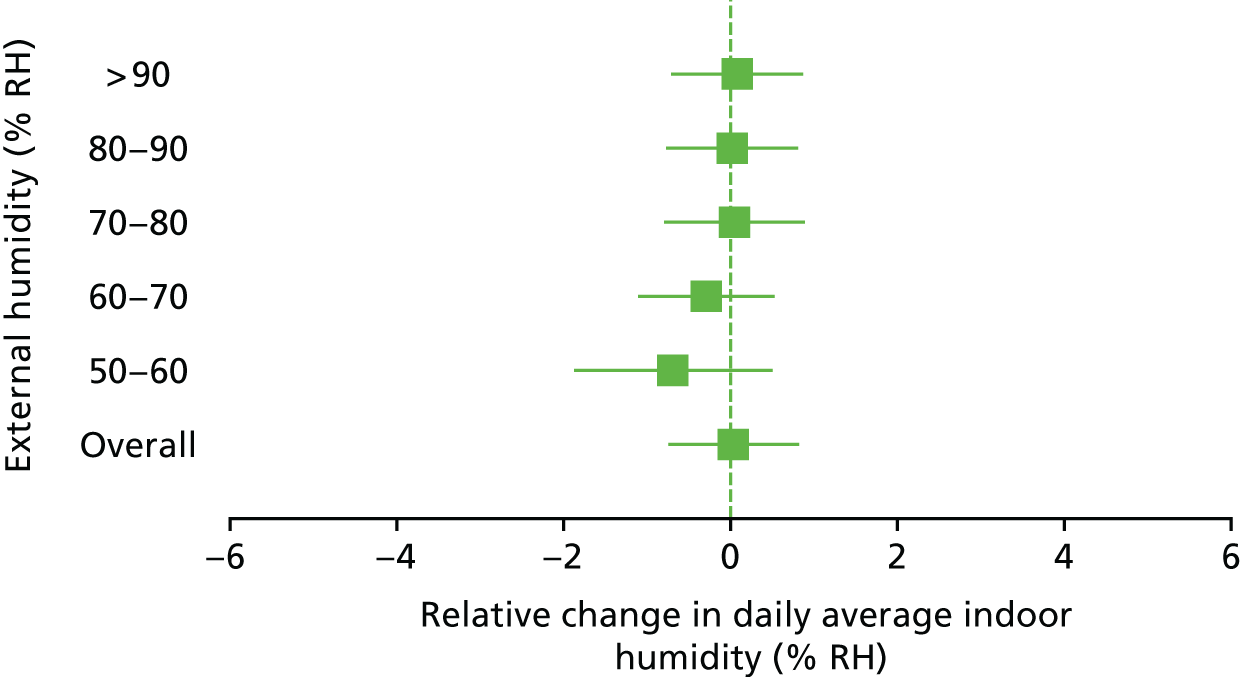
Figure 17 shows that the different intervention measures had differential impacts on internal hydrological conditions. Both a gas network connection (3.86% RH, 95% CI 2.31% to 5.41% RH) and the installation of new windows and doors (5.15% RH, 95% CI 3.73% to 6.57% RH) increased indoor RH levels. However, the increases were small in absolute terms. External wall insulation, which included the installation of mechanical ventilation, did not increase levels of indoor RH (–0.60% RH, 95% CI –2.26% to 1.06% RH) nor did the installation of boilers or heating systems (–1.59% RH, 95% CI –3.52% to –0.34% RH).
FIGURE 17.
Relative change in daily average indoor RH for different energy efficiency measures.
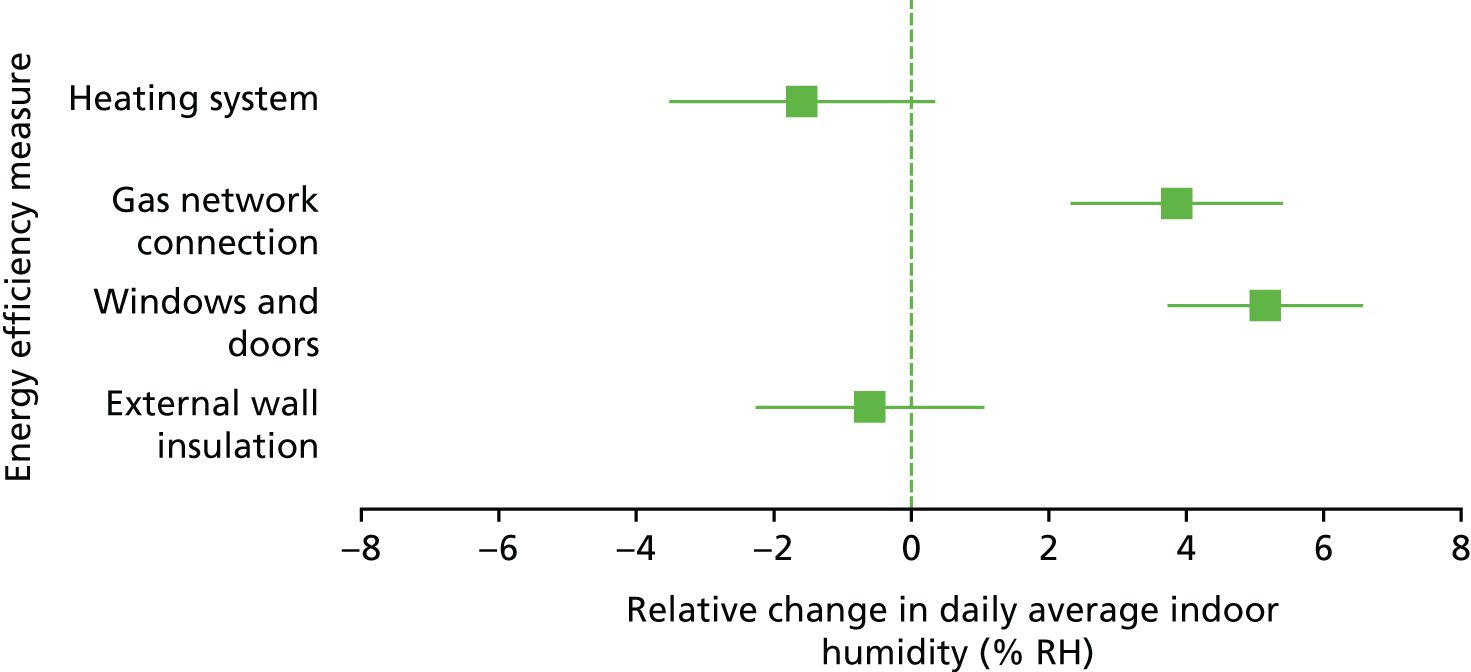
The effects of the intervention on indoor RH levels were different for different building construction types (Figure 18). The intervention increased indoor RH in buildings with cavity walls by 4.57% RH (95% CI 2.94% to 6.20% RH). The intervention decreased indoor RH levels in buildings with solid walls (–0.90% RH, 95% CI –1.76% to –0.03% RH). The intervention did not change indoor RH levels in British steel-framed buildings (–0.35% RH, 95% CI –1.49% to 0.78% RH).
FIGURE 18.
Relative change in daily average indoor RH for different construction types.
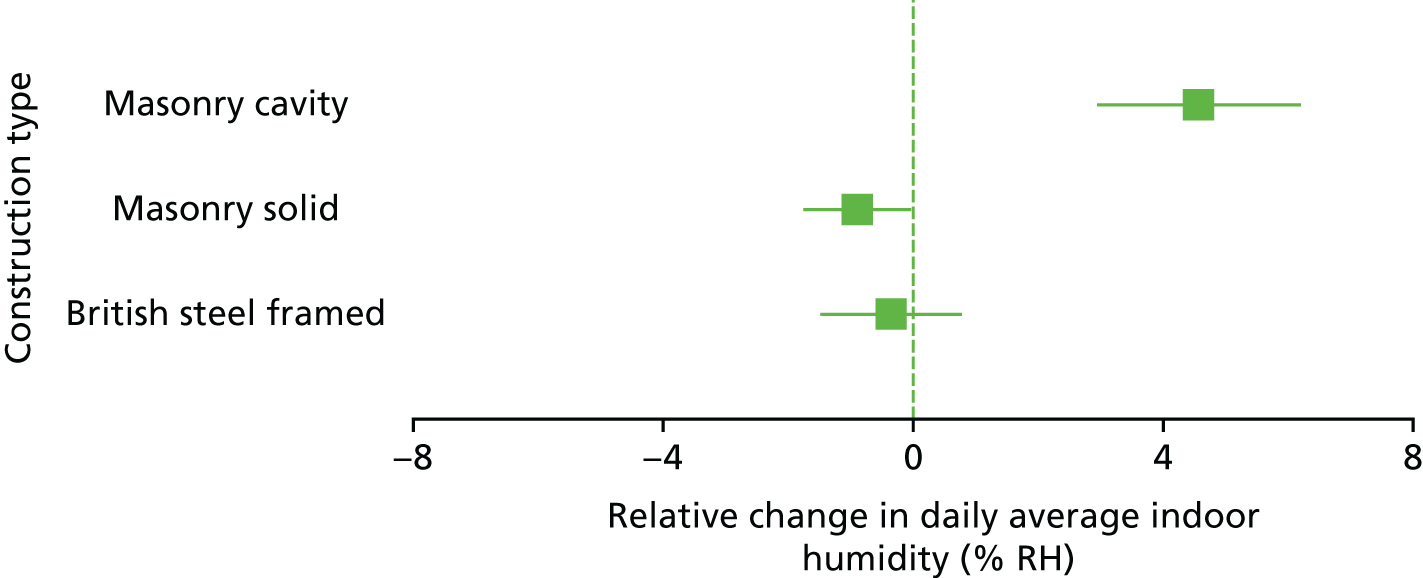
Duration and cumulative substandard internal conditions
The study further explored the impact of the intervention on the average daily duration and cumulative substandard internal conditions. Figure 19 shows that there is no evidence that the intervention reduced the daily duration of temperatures of < 18 °C (0.27 hours, 95% CI –0.49 to 0.96 hours) or <16 °C (0.20 hours, 95% CI –0.48 to 0.88 hours). However, the intervention did reduce the duration of indoor RH levels of > 60% RH by 1.14 hours (95% CI –2.00 to –0.28 hours).
FIGURE 19.
Relative change in daily average duration of substandard internal conditions for the intervention households.
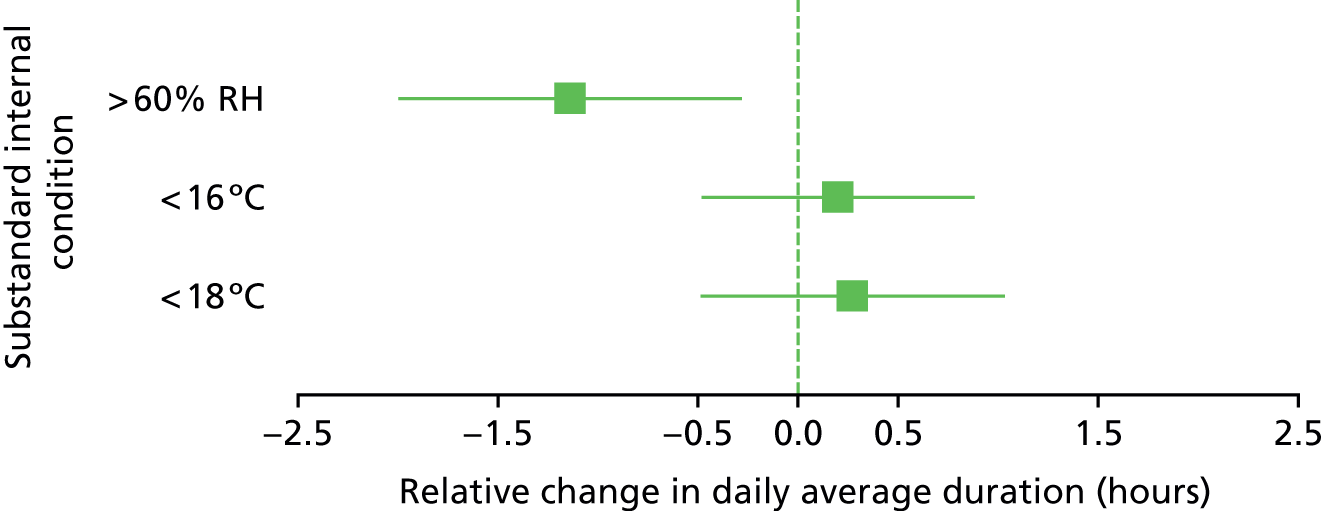
Figure 20 shows that the intervention had a positive effect on the three cumulative substandard internal conditions measures. The cumulative amount of indoor air temperature of < 18 °C was reduced by 3.62 °C·hour (95% CI –6.95 to –0.30 °C·hour). The cumulative amount of the indoor air temperature of < 16 °C was reduced by 4.20 °C·hour (95% CI –6.64 to –1.76 °C·hour). The cumulative amount of the indoor RH levels of > 60% was reduced by 19.32% RH·hour (95% CI –29.68% to –8.96% RH·hour).
FIGURE 20.
Relative change in daily average cumulative substandard internal conditions for the intervention households.
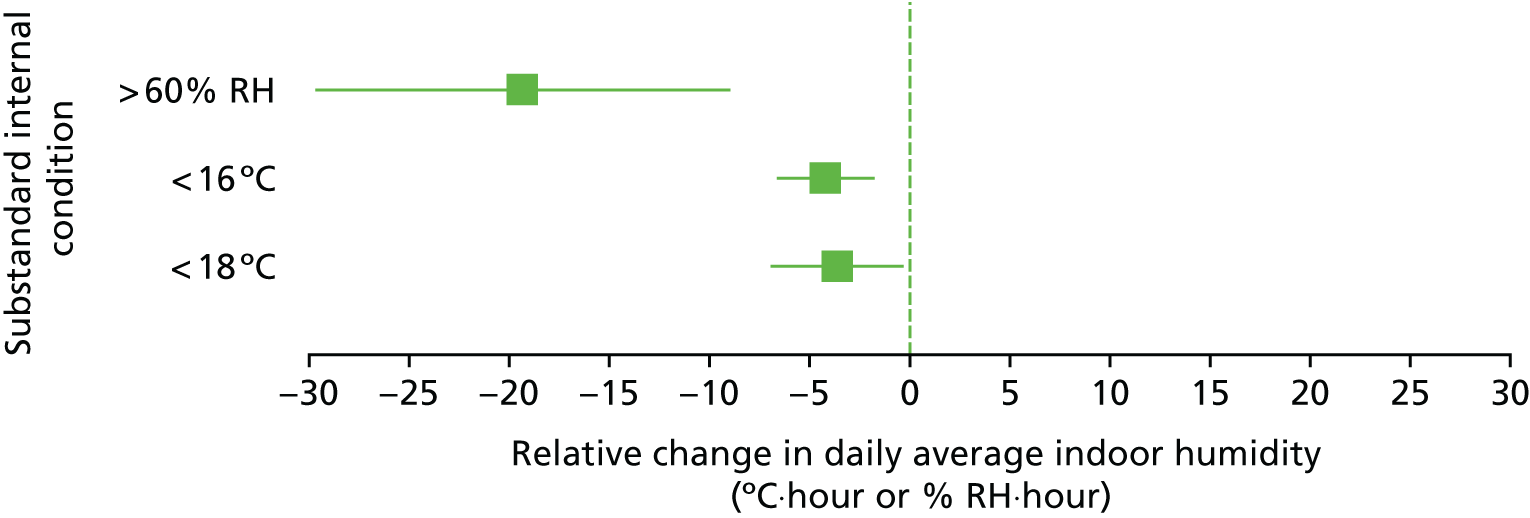
Average daily gas usage
Figure 21 shows the average daily gas usage for the intervention (n = 26) and control households (n = 37) at baseline and follow-up periods. The calculation excluded the households from the off-gas areas for which no gas meter readings could be taken. Average daily gas usage decreased from 3.88 m3 to 2.45 m3 in the intervention households, a decrease of 36.9%. In contrast, the average daily gas usage increased from 4.60 m3 to 4.76 m3 in the control households. Having controlled by individual household heating demand, a repeated measures ANOVA showed that the intervention group × measurement occasion interaction was significant [F(1,60) = 35.985; p = 0.000; η2 = 0.379 (Cohen’s d = 1.41)].
FIGURE 21.
Daily average gas consumption for the intervention and the control households at baseline and follow-up.
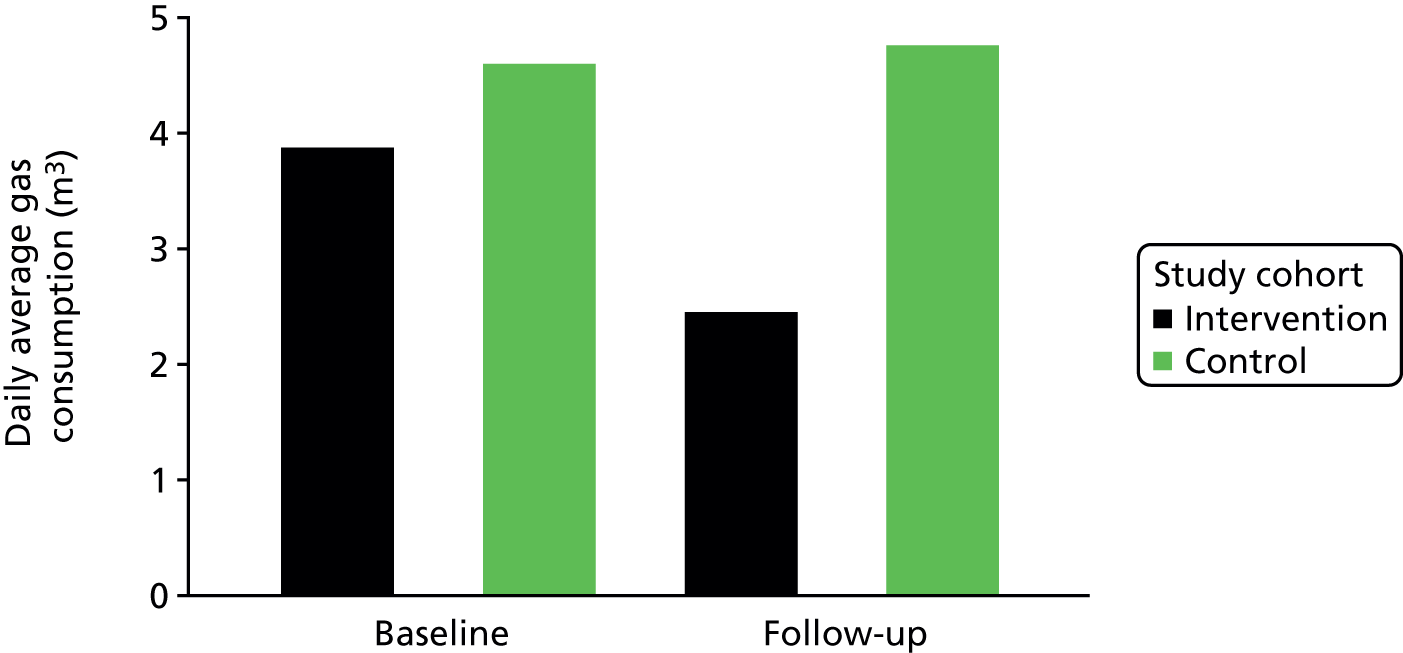
Discussion
Key results
This study provided new evidence on the impact of energy efficiency investments on internal conditions and household energy use through detailed long-term household monitoring. Internal conditions were monitored for a minimum of 28 consecutive days before and after the installation of energy efficiency measures. These were compared with the internal conditions of households that did not receive such measures. The study found that the intervention raised indoor air temperature by an average of 0.84 °C, whereas average daily gas usage dropped by 37%. Similar increases were observed across different heating demand conditions. Furthermore, the intervention reduced the cumulative amount of the indoor air temperature that was substandard. Overall, the intervention did not increase indoor RH levels, although small increases were found for some individual measures. The study found that the intervention reduced the average daily duration and cumulative amount of indoor levels of > 60% RH.
The intervention measures were not equally effective. External wall insulation and connection to the gas mains network significantly increased indoor air temperatures; new windows and doors or a new heating system did not significantly increase indoor air temperatures. The increases in indoor air temperatures in British steel-framed buildings and buildings with solid walls could largely be attributed to the external wall insulation they received. Both new windows and doors and connection to the gas mains network increased indoor RH, although these increases were small in absolute terms. External wall insulation probably did not increase indoor RH because of mechanical ventilation. Increases in indoor RH levels were observed only in buildings with cavity walls, and not in British steel-framed buildings or buildings with solid walls. It shows the importance of using mechanical ventilation when making buildings more airtight. 64
The observed changes in indoor conditions were similar to or somewhat smaller than those found in previous research. The results of these studies are discussed in Chapter 1. However, in order to be able to compare different studies, changes in indoor conditions need to be accompanied by data on energy use. The extent to which the benefits of improved energy efficiency are taken as energy saving or as extra warmth can then be assessed. The latter is also known as the rebound effect. 133,134
Limitations
Although the study involved detailed long-term monitoring before and after the intervention, included a control group and controlled for external hydrothermal conditions, it did not monitor occupancy, heating or occupant behaviour. Occupancy and occupant behaviour have a large impact on the energy consumption and internal conditions of buildings. Including these aspects would improve our understanding of adaptive behaviours resulting from the energy efficiency investments. However, it was beyond the scope of the study to incorporate internal household dynamics into the research. The research uses estimates from meter readings made at the installation and collection of the monitors. This can only provide an indication of the energy savings as a result of the energy efficiency investments. Higher-resolution energy monitoring would allow the energy usage to be attributed to specific purposes and to provide information on occupancy and occupant behaviour.
Limitations regarding the sample are similar to the community-based study, from which participating households were recruited. These limitations are discussed in Chapter 3. The final sample of intervention and control households, however, were largely comparable, and the study had relatively low attrition. Although the study involved only a relatively small number of houses, they were observed for a minimum of 4 weeks before and after the intervention, resulting in a detailed data set with > 15,000 data points. The longitudinal study therefore allowed the model parameters to be estimated with far more precision, using 28–231 observations per household as opposed to just one.
The intervention involved a number of different energy efficiency measures, depending on the type and location of the properties. Although this means that the houses were non-identical and that the intervention differed across the different schemes, it allowed the study to estimate the effects of different energy efficiency measures in different types of buildings.
Interpretation
The study suggests that the intervention successfully increased indoor air temperatures. Although the overall increase was relatively small (in the order of 1.0–1.5 °C), it reflects long-term average increases, reducing the potential exposure of substandard temperatures: it brought the majority of indoor temperatures within the ‘healthy’ comfort zone of 18–24 °C. 124,125 An above-average increase in bedroom temperature suggests that the intervention helped to expand comfortable space within the home. 61 There is no evidence that insulation substantially increases indoor RH levels when accompanied by mechanical ventilation. This suggests that energy efficiency investment programmes such as Arbed will be beneficial primarily by providing improved living conditions that are conducive to good physical and mental health. 54 The reduction in gas usage suggests that the intervention has been successful in reducing financial pressure and improving living conditions in households in low-income areas, which were the main aims of the intervention programme.
Generalisability
The study involved detailed long-term household monitoring of a number of different households receiving a range of different energy efficiency measures through the intervention programme. There is little evidence that selection and attrition have systematically biased the sample, suggesting that the results may be generalised to similar energy performance investment programmes, as may the results regarding the specific measures and construction types. However, the results may not be directly generalisable to non-deprived communities or households with different financial circumstances, occupancy or heating patterns.
Chapter 5 The economic evaluation
Introduction
This chapter follows Consolidated Health Economic Evaluation Reporting Standards (CHEERS) reporting standards (see Appendix 10). 135
Background
Health economic studies are intended to inform policy-makers and health-care decision-makers about which interventions, policies or services provide the best value for money. The challenge for such evaluations is to find the right methodology to evaluate social policy interventions that is not overburdensome in terms of data needs, yet appropriately informs decisions. The National Institute for Health and Care Excellence (NICE), for health technology assessment, defines a reference case that specifies the most appropriate methods consistent with the NHS objective of maximising health gain from limited resources. The reference case involves the use of the QALY as a single index to measure and value the health effects of interventions. 136
Social policy interventions, such as structural energy performance investments, have multiple social, economic and environmental dimensions. This differentiates them from many other types of health intervention. 137 These investments do not always provide an immediate return in terms of health and other social benefits. 138 Therefore, they may not be considered cost-effective using accepted norms. There is some evidence, summarised in a review paper by Fenwick et al. ,74 that investments in the energy efficiency of social housing stock provide wider benefits to the health and lives of residents, and that these investments may furthermore have value in their benefits to the NHS and social services.
This chapter describes the analyses undertaken for the health economic evaluation of the intervention programme. The analyses explore whether or not the structural energy performance investments under the intervention programme have delivered health-related quality-of-life improvements and economic impact. The value for money of a social policy intervention depends on several factors, including the timing and setting of the intervention as well as the perspective of the evaluator. Considerations include the balance between the estimated costs of the intervention and the expected outcomes for the beneficiaries, and the time over which the costs fall and benefits are realised. Although energy efficiency programmes are generally not provided or paid for by the health services – the intervention was funded through ERDF and the Welsh Government – they may provide benefits to the health services in terms of cost avoided through reduced health service usage. Furthermore, the intervention may not only benefit the NHS through changing use of health services and a reduction in contact with health and social services, but it may also result in improved quality of life of residents. To inform decisions about energy performance investments, a comprehensive overview of the benefits of such investments and an understanding of how benefits are realised beyond the perspective of the funder of the investments are required, for example benefits to the health services.
Economic studies for public health
Health economic analyses take many forms but can be broadly classified into two categories: cost studies and economic evaluations. A short description of the different types of cost studies and economic evaluations is provided in Box 1. The difference between the two categories is that cost studies focus on the identification of resources, impact and costs related to providing interventions and any cost offsets that may arise, whereas economic evaluation studies are comparative analyses that take account of both costs and benefits of the intervention and a comparator.
-
Cost–offset analysis establishes whether or not health-care utilisation decreases as a result of a health intervention. A total offset occurs when general health-care savings exceed the cost of the health-care intervention, effectively resulting in the treatment paying for itself.
-
Cost minimisation analysis addresses assessment of costs if the health benefits of competing health-care technologies have been demonstrated to have clinically equivalent outcomes.
-
Cost–consequences analysis considers all the health and non-health benefits of an intervention across different sectors and reports them in a disaggregated form. It accepts that different types of benefit cannot be captured using the same units. All impacts and costs are considered when deciding which interventions represent the best value, even if the impacts cannot be costed.
-
Cost-effectiveness analysis typically involves measuring a specific, one-dimensional health or clinical outcome for competing health technologies, for example ‘asthma attacks averted’.
-
Cost–utility analysis is a special type of cost-effectiveness analysis in which multidimensional health outcomes are reduced to a single dimension reflecting individuals’ preferences for the diverse health outcomes. The most commonly used outcome in CUA is the QALY. For both cost-effectiveness and cost–utility studies, value for money is identified using a measure of the additional cost per additional outcome ratio (e.g. an incremental cost/QALY ratio) and comparing that with an external threshold or with the ratio achieved by alternative policies.
-
Cost–benefit analysis involves the measurement and valuation of all outcomes of interest in monetary terms. Here the value for money is identified by positive net economic benefit associated with the intervention compared with an alternative (i.e. the monetary value of the outcomes exceeds the net costs of the intervention less any cost savings achieved elsewhere).
-
Cost–utility analysis is a form of cost-effectiveness analysis (CEA) that uses the QALY as the measure of benefit and is specified in the NICE reference case136 as a preferred method for conducting health economic evaluations, although it is recognised that it has limitations as well. CUA does not consider wider non-health benefits often associated with social policy interventions. 139 When evaluating social policy interventions, it is important to consider social and economic benefits beyond those for the health service, which cannot easily be captured by the QALY metric. In reality, benefits are measured and valued using different outcome metrics; therefore, an appropriate framework is needed that allows all relevant benefits of an intervention to be taken into account in the analysis. Cost–consequence analysis (CCA) and cost–benefit analysis (CBA) are considered suitable approaches by NICE, given that they allow all relevant benefits to be considered. The difference between CCA and CBA is that the former reports all health and non-health benefits in a disaggregated form, whereas the latter expresses all costs and benefits in monetary terms.
Previous economic studies of the health impact of housing improvement
A number of cost studies and economic evaluations have been conducted to assess the health-related quality of life and economic impacts of housing improvements. In a recent review of the literature, Fenwick et al. 74 identified 45 health economic studies relating to the health impacts of housing. It is clear from the review that only a limited number of health economic evaluations had been conducted during the period considered. Out of the 45 health economic studies, 29 reported cost and/or economic analysis and the majority involved cost studies (n = 25). Only four studies involved an economic analysis, although, in the opinion of the authors, 11 of the reported cost studies had sufficient data to conduct an economic evaluation. 74 Three studies stated that a CBA had been conducted but in fact it had not, and only one study was found to have conducted a CEA. Barton et al. 51 used the SF-36 to capture change in the health-related quality of life of residents as the basis for the economic evaluation. They concluded that the housing improvements did not produce substantial gains in terms of health-related quality of life, health or more schooling. However, they also recognised that there may have been insufficient time for measurable benefits to have materialised.
In addition to the studies reported by Fenwick et al. ,74 a small number of more-recent health economic studies have been conducted. The Gentoo social housing and sustainability group conducted a pilot study140 (n = 12) examining the health economic impact of energy efficiency improvements on people with COPD. The study found that self-reported GP appointments were reduced by 60%, accident and emergency (A&E) attendances by 30%, outpatient appointments by 22% and emergency admissions by 25%. The Warm Homes for Health project141,142 involved similar improvements, mainly focused on heating and insulation. The study suggests that installation of housing improvements produced cost savings to the NHS worth > £50,000. This would equate to an annual saving of £1B if all 4.8 million substandard homes in the UK receive the same energy efficiency measures. However, these two studies are at an early stage and at the time of writing the full results of their final health economic analyses have not yet been published.
Lawson et al. 143 conducted a CUA of a transfer of social and private tenants to new-build social housing rather than regeneration of existing housing stock. The study did not find a significant change in outcomes expressed as utility scores in the intervention group in comparison to a control group over a 2-year period. As a result, they concluded that the intervention was not value for money in health terms, although this was qualified by noting that not all benefits may have been captured and that they may not have been fully realised over the period of the study. 143
The research reported here builds on these prior studies but extends the body of evidence by using routinely collected health data in addition to self-reported survey data. The use of routinely collected health data allowed the intervention to be evaluated retrospectively using actual health-care utilisation data from the SAIL databank. 82–84,144 The study focuses on utilisation of secondary health-care services, including emergency admissions and contacts with primary care within NHS Wales, by people living in homes that received the intervention.
Objective
The objective of the economic analysis was to evaluate the costs and consequences of the intervention from the perspective of NHS Wales. This was undertaken in the form of a CCA and a CUA.
Method
Study design
The health outcomes utilised in the economic analyses are based on the analyses undertaken using routinely collected data held within the SAIL databank. The methods used for these analyses are described in Chapter 2. Based on a literature review and the recommendations of NICE, CCA was considered to be the most appropriate methodological approach for the evaluation as it allows for different types of outcome to be considered. The CCA considers resource use and the related cost impacts of the intervention and benefits of different kinds to be considered and to be reported in a disaggregated form. Health service impacts and costs are thus considered as separate items to enable decision-makers to determine if the intervention represents good value from their perspective.
In addition to the CCA, a CUA was considered as an approach to evaluate the intervention programme. The CUA approach attaches utility values (the ‘Q’ value in QALYs) over time for study outcomes to provide one single measure (i.e. QALYs). The CUA considers resource use and cost impacts of the intervention and the benefits in terms of QALYs to be established for the intervention. In CUA, the intervention outcomes and the overall costs are compared with the relevant comparator. The intervention can ‘dominate’ the control (greater effect/lower cost), in which case it will be unambiguously cost-effective, or, in the event of a non-dominance (greater effect/higher cost), results are reported in the form of an incremental cost-effectiveness ratio (ICER) showing the additional cost per QALY gained.
A simple CUA based on QALYs derived from SF-12 responses is reported in Chapter 3. However, the utility values collected in the community-based study did not represent specific health states; rather, they were valuations of health experienced before and after the intervention was completed.
The economic analyses utilise the outcomes from the SAIL databank analyses described in Chapter 2, and follow a panel analysis approach utilising data available for the study population in the SAIL databank. The data were extracted for the study populations in monthly increments and used to develop a retrospective analysis (see Chapter 2). To fully utilise the SAIL databank outcomes, we looked to the literature and prior research to identify utility values for the health states experienced by individuals experiencing COPD-related emergency admissions.
The overall study period is 10 years, from 2005 to 2014. The intervention was delivered in 2010/11. Information collected prior to the intervention was utilised as a baseline to observe any subsequent changes in hospital admissions. The time horizon for the economic evaluation was 4 years post intervention.
As described in Chapter 2, the intervention programme included external wall insulation, boiler upgrades and replacements, microgeneration (photovoltaics/solar hot water/heat pumps) and general energy-saving advice. The lifespans of the different elements range from 12 years for boiler upgrades and replacements to 42 years for insulation (see Table 14). 91 Considering that the shortest lifespan of an element was 12 years, residents remaining in the property after the improvements will have benefited from the elements installed. Extrapolating beyond the study period seems inappropriate for the base case scenario, given the uncertainty about the health benefits that remain with an individual if they move out of properties over time. We believe that the 4-year time horizon of the study is appropriate and generalisable. To explore this element of uncertainty further, we undertook a sensitivity analysis, using threshold analysis to explore a range of durations of residency. We assumed that the impact was constant and the benefit continued after the observed study period. As the follow-up period after the intervention was completed was longer than 12 months, the costs and benefits beyond 1 year were discounted at a standard rate for public health interventions, currently 3.5%, to bring them into 2013 prices and a sensitivity analysis with 1.5%. 139
Setting
The economic analysis focused on the first phase of the intervention programme that took place in 2010 and 2011, in which a total of £68M was invested, including leveraged funding. The programme improved the energy efficiency of existing homes in low-income areas. LAs and RSLs submitted project plans to improve thermal efficiency of homes in Wales. The majority of homes were social housing. This study was undertaken using data for the intervention group providing their own historical controls. Individually reported baseline and follow-up periods in the intervention cohort were used to calculate the duration of the intervention for each resident and/or household.
Participants
The study participants within this study were individuals who lived within one of the intervention or comparator homes during the period under consideration. Anonymised housing codes were used to link the homes and individuals within the SAIL databank. All individuals who were a resident within a study home for ≥ 60 days were included in the analysis. Baseline data were collected for several years prior to the intervention.
Variables
A range of explanatory variables was collected for each individual and/or household. The variables utilised within this study were chosen for their theoretical power in explaining the levels of emergency admissions experienced. Information was collected at the individual level for age (in 5-year bands), sex and comorbidity (0, 1). Housing-level data were collected for area income deprivation (ordinal scale) and a measure of rurality (urban or rural). Given the seasonal trend observed within the dependent variables, an identifier for seasonality was also included. All outcome variables were grouped into the composite count measure of cardiovascular and respiratory emergency hospital admissions. To account for the variability in the duration over which individuals were observed, each of the count measures is transformed into a yearly rate. Data for each of these emergency admission variables were collected from PEDW. The PEDW data are structured into ICD-10 diagnosis chapters, described in Chapter 2.
Costs
The comparisons in health service resource use related to the study outcomes for the study population were made between the baseline and follow-up intervention period. The resource changes for the health system as a result of changes in health service use were calculated using the methods described in Chapter 2 and standard NHS unit costs. The costs associated with the resources in secondary care health services used by the recipients of the intervention include secondary care admissions for cardiovascular and respiratory conditions. The total cost of the intervention was estimated from the cost of implementing the programme, plus changes in health-care resource costs over the follow-up period. The comparison is made with the pre-intervention period for the study population and no intervention costs and so the total costs are derived solely from the changes in health-care resource use. The overall budget allocated to the intervention programme was derived from records received from the Welsh Government and the 28 LAs and RSLs involved in the scheme. The evaluation was undertaken from a NHS costing perspective; only costs and benefits to NHS Wales were considered. We used 2015 as the base year for costs.
Outcomes
The health outcomes for the CCA were changes in emergency hospital admissions for cardiovascular and respiratory conditions.
The intervention was expected to have an impact on the health-related quality of life of the residents who received the intervention over the follow-up period. As reported in Chapter 3, the results of the community-based study showed no difference in either health-related quality of life or utilities within 12 months of the delivery of the intervention. These results reflect those of similar studies evaluating housing interventions and the subsequent impact on health-related quality of life, in which the authors suggest that the relatively short time period of the follow-up did not allow the full impact of the interventions to be reflected in health-related quality-of-life status. 51,143
In order to undertake a meaningful CUA, we used an economic modelling approach, which instead used literature-based utility values for the health states related to the primary and secondary health outcomes to evaluate the probable impact of the intervention on QALYs. The CUA conducted here used only outcomes relating to COPD. The reason for that is that there is a relative wealth of literature on utility values for hospital admissions for exacerbations of COPD in comparison with the other reasons for hospital admissions. A number of prior studies have examined the impacts of housing interventions on COPD, and COPD forms an important part of the overall burden of disease. 118,145,146 In order to identify utility values for COPD to enable contextualisation of the results, a systematic approach was taken to reviewing the literature. The literature review is provided in Appendix 12.
Economic analysis
Two types of economic analysis were conducted: CCA and CUA.
Cost–consequences analysis
The primary outcome measure for the CCA was the change in cardiovascular or respiratory emergency hospital admissions and utilised the financial costs of the intervention. The findings are reported as the ratio of the cost per person per year and the changes in primary outcome. The statistical approach undertaken to calculate the impact of the intervention on the primary outcome initially assessed the presence of trends over the study period. The data were observed as a monthly count of emergency admissions. In the absence of an evident trend, the data were conflated into a two-period comparison. Our analysis compares the outcome rates before and after the intervention. To account for different exposure durations and data containing incomplete years, the aggregated count data is transformed into annualised rates when possible (see Chapter 2). The resulting annualised rates were compared using a mixed multilevel linear model, which incorporates the covariates and explanatory variables.
The covariates included were age, sex, overall comorbidity score, a seasonality adjustment and area-based deprivation. The age variable takes an average age for each of the two periods. In the event of clustering, random effects modelling was used. Clustering was based on a person’s unbroken residence within a study property. When an individual was observed inhabiting multiple properties within the study period, the data are assumed to be independent owing to the influence of unobserved factors. The independence of residential durations extends to individuals returning to an intervention property they previously inhabited.
A ‘bottom-up’ costing approach was undertaken as part of the economic analyses; details are provided in Appendix 11. The rationale behind this costing approach was the desire to report figures that represent the financial implications of replicating the intervention and enable both bottom-up and top-down costing in the analysis, which may have inflated values owing to the inclusion of managerial, planning and data collection components that may not be required in a replication intervention. The total bottom-up cost of delivering the intervention was £32,484,900 for the 4968 households in the analysable data set (i.e. with complete data on the property intervention and inhabitants). The Welsh Government intervention top-down spend was reported to be between £60M and £68M (personal communication with Welsh Government). However, these figures include additional properties and leveraged funding that were not reported in the initial data set. The top-down costing approach was used for the sensitivity analysis.
Costs were initially estimated on a per-household basis and later adjusted to a per-person per-year level to standardise the outcome measure. Given the range in effective duration for the different components of the intervention, a time-dependent costing approach was adopted. Table 14 shows the frequency of each intervention component alongside the effective duration and cost. Costs are reported at 2013 prices. The resulting cost per household was calculated according to the average overall cost and then adjusted to create a per-year figure. The intervention cost was spread across the effective lifetime using a straight-line depreciation method given the assumption of zero residual value.
Intervention measure | Number delivered | Estimated lifetime (years)147 | Indicative cost (£) (WG report)148 | Average cost (£) per year |
---|---|---|---|---|
External wall insulation | 2709 | 36 | 7300 | 202.78 |
Photovoltaics | 1458 | 25 | 5400 | 216.00 |
Solar water heating | 928 | 25 | 2600 | 104.00 |
Air source heat pumps | 401 | 15 | 6000 | 400.00 |
Loft/rafter insulation | 172 | 42 | 100 | 2.38 |
The bottom-up costing approach provided a per-property per-year cost of £225.76. Given that all the intervention elements have a life expectancy of > 4 years, the cost per household can be assumed to be consistent across all the years included in the analysis. The data suggest an average occupancy of 2.7 individuals, resulting in an average per-person per-year cost of £83.61.
The CCA compared the cost of the intervention with the range of reported outcomes. The primary outcome is the rate of combined cardiovascular and respiratory emergency hospital admission. The secondary outcomes are the contributing subgroups of the primary outcome. The primary and secondary outcomes are illustrated alongside their mean annualised change rates in Table 15.
Outcome | Before, M (SD) [n] | After, M (SD) [n] | Change in rate |
---|---|---|---|
Cardiovascular or respiratory | 0.0543 (0.4788) [22,209] | 0.0577 (0.7079) [18,527] | 0.0034 |
COPD | 0.0062 (0.1468) [22,209] | 0.0069 (0.1150) [18,527] | 0.0007 |
Cardiovascular | 0.0179 (0.2709) [22,209] | 0.0171 (0.4377) [18,527] | –0.0008 |
Respiratory | 0.0324 (0.3720) [22,209] | 0.0371 (0.5430) [18,527] | 0.0043 |
The annualised prevalence rates reported here show that, without accounting for covariates, the primary outcome rate increases by 0.0034 following the intervention. Changes in emergency admissions represent an important component of the cost in CCA as a change in rate represents a change in cost. Using an ‘average duration’ approach and the reported emergency admissions, alongside NHS reference costs,149 results in a representative cost per event of £2734.29.
Cost–utility analysis
We developed the event profile for COPD-related emergency hospital admission based on a structured literature review (see Appendix 12 and the search strategy reported in Appendix 13). The decline and partial recovery profile that characterises a COPD exacerbation was modelled to include five distinct phases as described by Seemungal et al. 150
The baseline utility value was sourced from a systematic review151 that reported UK-based utility scores derived from the EuroQol-5 Dimensions (EQ-5D) instrument for each of the four international severity levels for COPD. 152 The distribution of COPD in UK general practice using the Global Initiative for Chronic Obstructive Lung Disease (GOLD) classification was described in a study by Haughney et al. ,153 in which the combination of the EQ-5D and weighting data provided the baseline COPD utility. The GOLD stages represent varying forced expiratory volumes (FEVs). The baseline COPD utility score represents the value that individuals with COPD experience before an exacerbation occurs, whether severe enough to cause a hospital admission or otherwise. Table 16 reports the combination of the distribution of GOLD COPD stages and the utility scores representing the valuation of the health state. The resulting weighted baseline value was a utility score of 0.750.
GOLD stage of COPD | Distribution of COPD population (%) | Utility score |
---|---|---|
1 | 17.1 | 0.806 |
2 | 52.2 | 0.767 |
3 | 25.5 | 0.704 |
4 | 5.2 | 0.616 |
An emergency hospitalisation attributable to an exacerbation of COPD represents a detrimental impact on an individual’s short-term and possibly long-term health-related quality of life, represented here by health state utility scores. The literature search identified three papers that included utility valuations for individuals on admission and on discharge from a hospital stay for a COPD admission. 154–156 The utility scores for use in this analysis were created using a weighted average of all those reported in these papers, taking into account the number of individuals that the publication reported. Table 17 shows the number of individuals, utility value and publication from which the value was derived. The average utility score, derived from the literature for the COPD patients who experienced a hospital stay, is 0.504.
Research paper | Number of patients | Utility value |
---|---|---|
Esteban et al.157 | 1421 | 0.600 |
Menn et al.155 | 117 | 0.446 |
O’Reilly et al.156 | 222 | –0.077 |
Average | 0.504 |
The COPD events that are serious enough to require hospital admission carry a risk of mortality. To account for this within this model, an inflated relative mortality measure was calculated. The data on COPD hospital admission mortality come from Esteban et al. 157 Given the average hospital stay duration of 7 days, all-cause mortality for this duration provides a baseline rate. The average age of patients in the literature was 72.6 years, whereas the data for COPD events utilised within this analysis show a mean age of 69.2 years. The relative all-cause mortality for these two ages was used to deflate the mortality observed in the literature to a rate representative of COPD hospitalisations for the data used in our analysis. The all-cause mortality was calculated to be 0.031% for the 7-day period, whereas those experiencing a COPD-related hospitalisation had a mortality rate calculated to be 2.507%. The net increase in mortality attributable to COPD hospitalisation is 2.476%. Taking into account this increased mortality when estimating the utility value for a COPD admission for an exacerbation, we estimated a utility value of 0.492.
The estimation of a discharge utility score follows the same approach as estimating the admission value. Hospital discharge values were obtained from the same three papers as the admission figures. 155–157 The data sourced from the literature report 1289 individuals with a mortality-adjusted average utility score of 0.613. As previously mentioned, COPD events are characterised by an initially large reduction of health-related quality of life followed by a recovery phase to prior health. However, some individuals may ultimately never fully recover to the same health and FEV levels they had prior to the exacerbation.
The post-discharge recovery phase was estimated using the recovery statistics reported by Seemungal et al. 150 The duration of an event that began with a hospitalisation attributable to COPD was estimated by Seemungal et al. 150 to be a total of 91 days. Given a 7-day hospital stay, the remaining 84 days are characterised by two phases: first, 28 days following discharge, during which 75.2% of individuals were found to make a full recovery; and, second, the subsequent 56 days, during which 79.8% of those remaining not fully recovered were reported as still experiencing a negative residual impact of their event. The utility score of these two recovery points is calculated according to the percentage of individuals recovering from the discharge utility value back to the initial baseline value. The utility score for individuals 28 days post discharge was calculated as 0.720, increasing to 0.726 at 84 days. The figure of 0.726 is assumed to be the long-term utility score.
Figure 22 plots the five COPD health states and the related utility values, illustrating the sharp decline caused by the hospitalised exacerbation event followed by the gradual recovery that ultimately never reaches the initial baseline value. The area under the curve approach was used to estimate the short- and long-term reduction in QALYs. The short-term calculation assumes that the hospitalisation occurs in the middle of the year with a within-year QALY reduction of 0.022. The long-term impact of a COPD event is a reduction of 0.240 QALYs.
FIGURE 22.
Time course and utility values for a COPD exacerbation event.
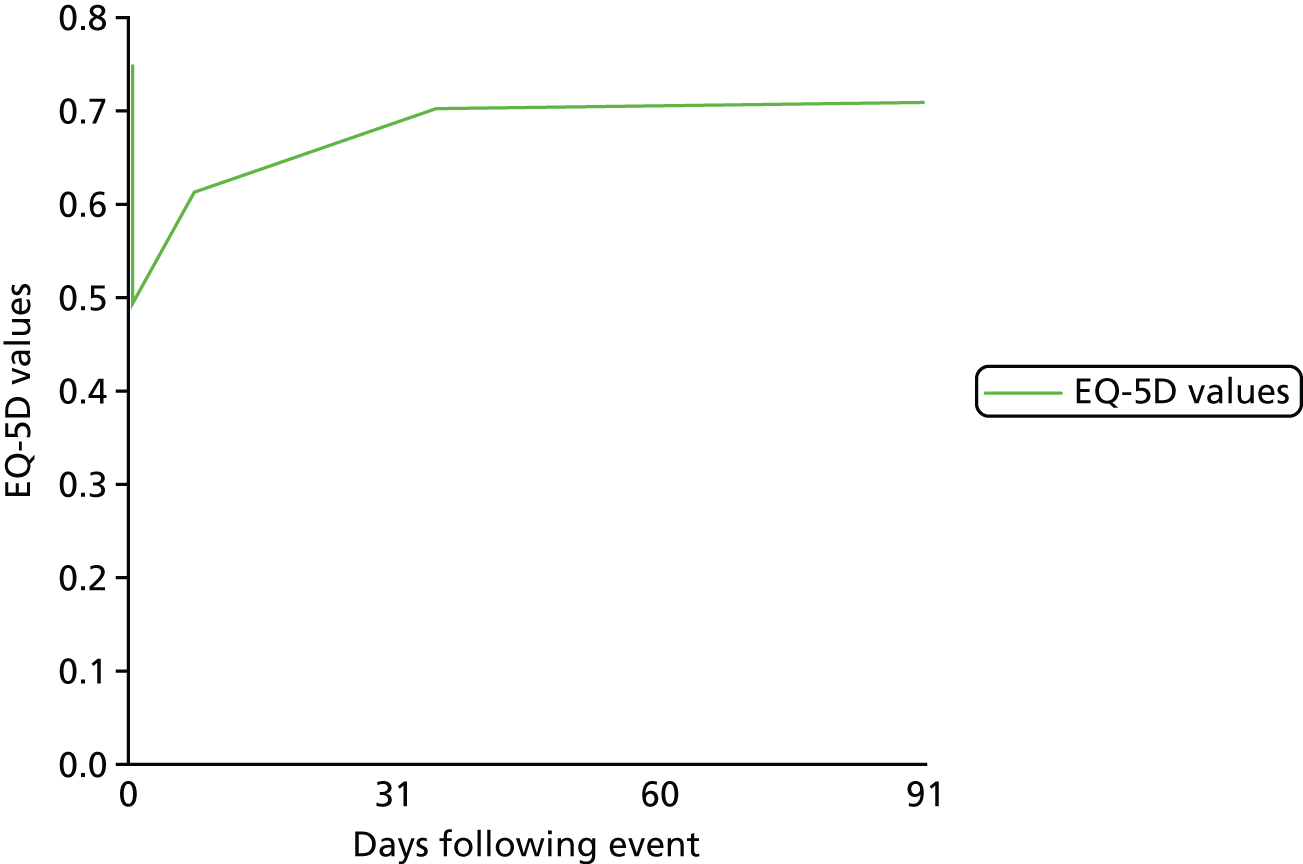
Although commonly used for estimating health valuation over time, utility decrements may fail to account for the full detrimental impact of a COPD event when used to estimate a structured event pathway for a COPD patient. Rutten-van Mölken et al. 158 sought to capture the broader holistic impact of a serious COPD exacerbation using an isolated time trade-off valuation approach. This time trade-off study estimated a single serious exacerbation to reduce health state utility by 0.042. This study viewed an event within the context of 1 year as opposed to the modelling undertaken in this analysis. The extended time frame obtained from the five-point calculation aligns well with the panel data analysis in that it accounts for the longer-term impact of reductions in FEV.
To inform our CUA and economic model, each adverse health event resulting in a hospital admission had a curve mapped from the utility values across the study period. This approach incorporates the initial impact of the event and a time-variable component. The analysis utilised cost associated with the intervention and the improved health following a reduction in COPD events to generate an ICER. 159
Sensitivity analysis
To address uncertainty in our findings, a number of deterministic one-way sensitivity analyses were carried out based on a number of variables that we were aware introduced uncertainty and/or had a substantive impact on the health outcomes, resource use and costs. The CCA results offer a concise package of findings on which a best-case and worst-case scenario evaluation is undertaken for the sensitivity analysis. The uncertainty in the outputs of the CUA was explored using one-way sensitivity analysis, a threshold analysis and a probabilistic sensitivity analysis (PSA).
The threshold analysis estimates the level of reduced COPD hospitalisations required to make the intervention cost-effective at a £30,000 per incremental QALY gained level. Given the importance of the time frame of the analysis, a range of different time horizons were taken. The PSA enabled a range of variables with inherent uncertainty to be investigated to determine the impact on the results.
Uncertainty exists for two main components of the analysis:
-
costs
-
change in adverse health events resulting in hospital admissions.
Probabilistic sensitivity analysis was conducted to assess the uncertainty of each of these two areas. The approach takes into account the uncertainty inherent in the Welsh Government top-down cost estimate of approximately £60M–68M and models the uncertainty using a uniform distribution. The uniform distribution was chosen in line with good practice because there is no centralised tendency and all figures within the distribution are assumed to be as likely as any other. The change in hospitalisation event values are estimated using normally distributed random samples. The normal distribution takes into account the central point estimate used in the deterministic analysis and the SD from this position.
The PSA approach estimates 1000 resamples, which are calculated as individual ICERs (see Equation 1). Findings from the PSA are reported in a cost-effectiveness plane (CEP). The 95% CIs calculated from the PSA samples are reported alongside the main ICER finding. The ICER, CEP and cost-effectiveness acceptability curve (CEAC) were evaluated relative to the NICE willingness-to-pay threshold values. NICE generally considers that interventions costing the NHS < £20,000 per QALY gained are cost-effective. Interventions costing between £20,000 and £30,000 can be deemed cost-effective, depending on the level of uncertainty about the ICER, the impact on health-related quality of life of the intervention, the degree of innovation and level of benefits that may arrive that are not easily quantifiable in terms of health gain:139
Results
Cost–consequence analysis
The CCA outcomes are presented in Table 18. The outcomes for the primary objective are reported separately from the costs. The average change in emergency admissions by year is weighted according to the prevalence levels within each of the 4 post-intervention years. Table 18 reports the primary objective alongside the intervention costs. The costs and outcomes are discounted to present-day values at 3.5%.
Year | Weighting | Change in admissions post intervention, B (SD) | Cost per person per year for the intervention (£) | 3.5% discounted event (discounted event cost, £) |
---|---|---|---|---|
Overall | 0.0010 (0.006) | |||
1 | 1.078 | 0.0012 (0.006) | 83.61 | 0.0012 (3.20) |
2 | 0.919 | 0.0010 (0.005) | 83.61 | 0.0010 (2.64) |
3 | 1.011 | 0.0011 (0.006) | 83.61 | 0.0010 (2.81) |
4 | 0.992 | 0.0011 (0.006) | 83.61 | 0.0010 (2.66) |
Total | 345.75 | 0.0041 |
Table 18 reports four data columns and totals. The ‘weighting’ column offers the calculation needed to transform the overall impact into a yearly value, which is based on the prevalence of the measure in each year. The change in admissions (B) and SD are reported in the second data column; the fourth data column reports the discounted effects and attached treatment costs. The third column includes the cost of the intervention, which is combined with the changes in treatment costs to offer the final cost component. The total effect value is a simple summation of the four yearly changes in admissions.
The results suggest a non-significant increase in hospital admissions for the primary outcome: over the 4 years, the cumulative impact is 0.0041 events per person. The per-person cost over the post-intervention period amounts to £345.75, which is driven by an intervention cost of £334.44 and an increase in treatment costs of £11.31. Given the increase in the primary outcome following the intervention and the positive intervention costs, the intervention is not deemed as cost-effective.
The results using the secondary outcomes are reported in Tables 19–21. The approach is the same as with the primary outcome but leaves out the intervention costs. The intervention costs are not included in the tables to avoid double counting. Table 22 reports the combined outcomes and costs for the four measures.
Year | Weighting | Undiscounted change, B (SD) | 3.5% discounted event (discounted event cost, £) |
---|---|---|---|
Overall | –0.0002 (0.001) | ||
1 | 0.7724 | –0.0001 (0.001) | –0.0001 (–0.34) |
2 | 0.8666 | –0.0001 (0.001) | –0.0001 (–0.36) |
3 | 1.2057 | –0.0002 (0.001) | –0.0002 (–0.49) |
4 | 1.1554 | –0.0002 (0.001) | –0.0002 (–0.45) |
Total | –0.0006 (–1.64) |
Year | Weighting | Undiscounted change, B (SD) | 3.5% discounted event (discounted event cost, £) |
---|---|---|---|
Overall | –0.0014 (0.004) | ||
1 | 1.1196 | –0.0016 (0.004) | –0.0016 (–4.28) |
2 | 0.9067 | –0.0013 (0.003) | –0.0012 (–3.35) |
3 | 0.8947 | –0.0013 (0.003) | –0.0012 (–3.20) |
4 | 1.0790 | –0.0015 (0.004) | –0.0014 (–3.74) |
Total | –0.0053 (–14.56) |
Year | Weighting | Undiscounted change, B (SD) | 3.5% discounted event (discounted event cost, £) |
---|---|---|---|
Overall | 0.0042 (0.005) | ||
1 | 1.0083 | 0.0042 (0.005) | 0.0042 (11.48) |
2 | 0.9460 | 0.0040 (0.004) | 0.0039 (10.57) |
3 | 1.0918 | 0.0046 (0.005) | 0.0043 (11.74) |
4 | 0.9540 | 0.0040 (0.004) | 0.0036 (9.84) |
Total | 0.0160 (43.66) |
Secondary outcomes emergency admissions | Change in emergency admission events | Net financial cost overall per person (£) |
---|---|---|
COPD | –0.0006 | Effect –85.96 |
Cardiovascular | –0.0053 | Intervention 334.44 |
Respiratory | 0.016 | Net cost 248.48 |
Table 19 reports the impact on the COPD-related hospital admissions per person. The reduction in COPD emergency admissions is not significant at a 95% confidence value; the summation of these event reductions over the 4 years totals to –0.0006 per person. The scale of this reduction is very small and suggests a reduction in NHS treatment costs of £1.64. The reduction of 0.0006 events should be considered alongside each of the following impacts. The weighting levels in Table 20 show that COPD events were increasing over the 4-year period.
Table 20 reports the impact on the cardiovascular-related emergency hospital admission events per person. As with the COPD results, the cardiovascular event levels have reduced non-significantly. The scale of the reduction is 0.0053 and the discounted amount saved is £14.56. The first year following the intervention saw the lowest levels of cardiovascular emergency admissions.
Table 21 reports the impact on the respiratory-related emergency admission events per person. The respiratory emergency admission event changes following the intervention are positive but do not achieve statistical significance. However, the scale is much greater than that for either the COPD or the cardiovascular results. Given that the overall respiratory and cardiovascular findings show an increase in frequency, the two relatively small decreases and the finding that one component was positive and dominating are not surprising.
The combinations of each of the secondary outcome measures alongside the intervention costs are reported in Table 22. The intervention reduces COPD and cardiovascular emergency admissions but increases the number of respiratory-related admissions. This is at a net cost of £248.48 per person over a 4-year period.
Cost–utility analysis
The CUA utilises the data from the CCA and develops the analysis further into an economic evaluation using the data identified in the literature review (reported in Appendix 12). Our analysis suggests that a within-year QALY decrease for a single COPD exacerbation hospital admission is 0.022. The residual decrement to utility scores in subsequent years after the initial year of the event results in a reduction in QALYs of 0.024. The inclusion of these QALY valuations in the 4-year model is presented in Table 23.
Year | Undiscounted change, B (SD) | 3.5% discounted event (discounted event cost, £) | Cost per year for the intervention (£) | QALY gains |
---|---|---|---|---|
1 | –0.0001 (0.001) | –0.0001 (–0.34) | 83.61 | –0.000003 |
2 | –0.0001 (0.001) | –0.0001 (–0.36) | 83.61 | –0.000006 |
3 | –0.0002 (0.001) | –0.0002 (–0.49) | 83.61 | –0.000010 |
4 | –0.0002 (0.001) | –0.0002 (–0.45) | 83.61 | –0.000013 |
Total | 332.80 | –0.000032 |
The relatively small decrease in COPD events and the relatively large cost of the intervention programme mean that the ICER is > £10M (£10,485,472.12) per QALY gained. When using a discount rate of 1.5% instead of the base case 3.5%, the ICER reduced to £10,066,540.29. Both of these ICERs are deemed not cost-effective.
Threshold analysis was undertaken to estimate the required reduction in COPD events and related utility scores over the 4-year period to make the intervention cost-effective. The base-case analysis estimates suggest that the intervention is not cost-effective, as it would require a constant yearly per-person reduction in utilities associated with COPD events of 0.028 in order to make the intervention appealing in terms of a cost per incremental QALY gained of £20,000–30,000. Given the long-term investment associated with the intervention, a 10-year threshold analysis was estimated. The benefits of the cumulative health-related quality-of-life benefits require a yearly decrease in utility scores of 0.013 for the intervention to be considered cost-effective. The disparity between these two figures highlights the influence of the duration of analysis. With a condition such as COPD, which has a long-term decrement to health-related quality of life, interventions that deliver benefits consistently over an extended period may be perceived more favourably with extended timelines. Given the relative scale of the required benefits estimated by the threshold analysis in the shorter term compared with those reported in Table 23, time horizons of > 10 years were not investigated.
The PSA varied the yearly reductions in COPD admissions alongside a uniformly distributed cost of intervention. The 1000 replications are plotted on a CEP (Figure 23). Given the scale of reduction observed in the statistical analysis and the costs involved, it is important to note the axis units. The QALY axis reports numbers in the thousandths of a QALY whereas the net cost unit is in hundreds of pounds. The impact of the intervention on COPD events has a 0% chance of the intervention being cost-effective at a £30,000 per incremental QALY gained level. The CEAC is not illustrated owing to the lack of movement at plausible ICERs.
FIGURE 23.
Probabilistic sensitivity analysis results on a CEP.
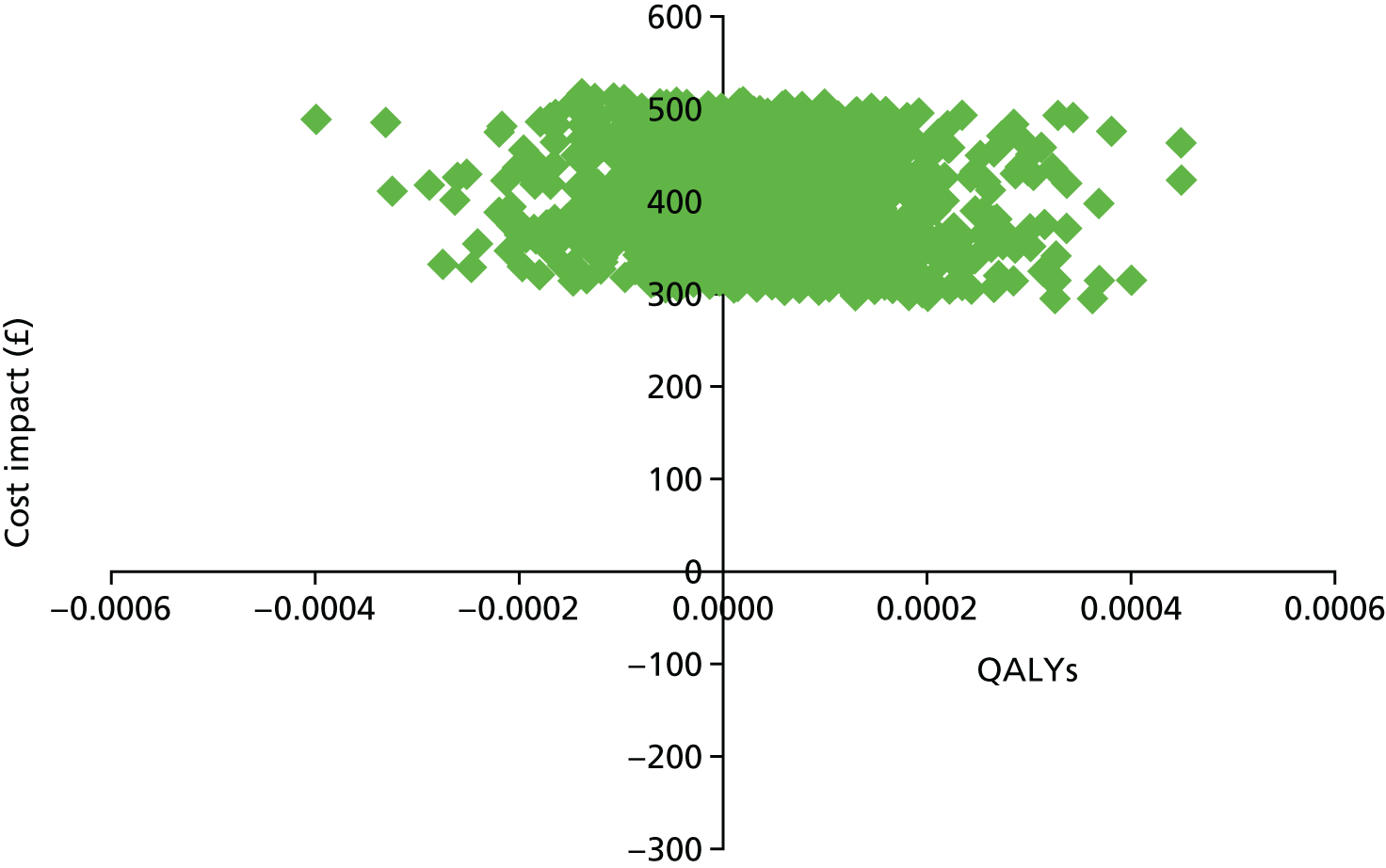
The findings reinforce the uncertainty surrounding the statistical outcomes and highlight the relatively small scale of the impact on COPD event rates in comparison with the intervention costs. The furthest outlier in favour of the intervention saves 0.004 QALYs at a cost of £314.14.
The PSA estimations are also reported as a CEAC in Figure 24. The relatively small-scale impact on the effectiveness of the intervention in terms of improvement of utility scores and relatively high costs of the intervention resulted in a 0% chance of cost-effectiveness using a threshold of £30,000 per QALY gained. The scale of the CEAC relates to the relative cost and effectiveness levels and provides an illustration of the positions where the likelihood of cost-effectiveness changes. The upper left-hand quadrant of the CEP in Figure 23 shows the occurrence of dominated observations (higher costs and worse health outcome), which accounts for 36.1% of the estimations. The maximum percentage of estimations that could be considered cost-effective (i.e. < £30,00 per QALY gained) is 65.9%; the CEAC illustrates up to 54% of these.
FIGURE 24.
Cost-effectiveness acceptability curve for the intervention.
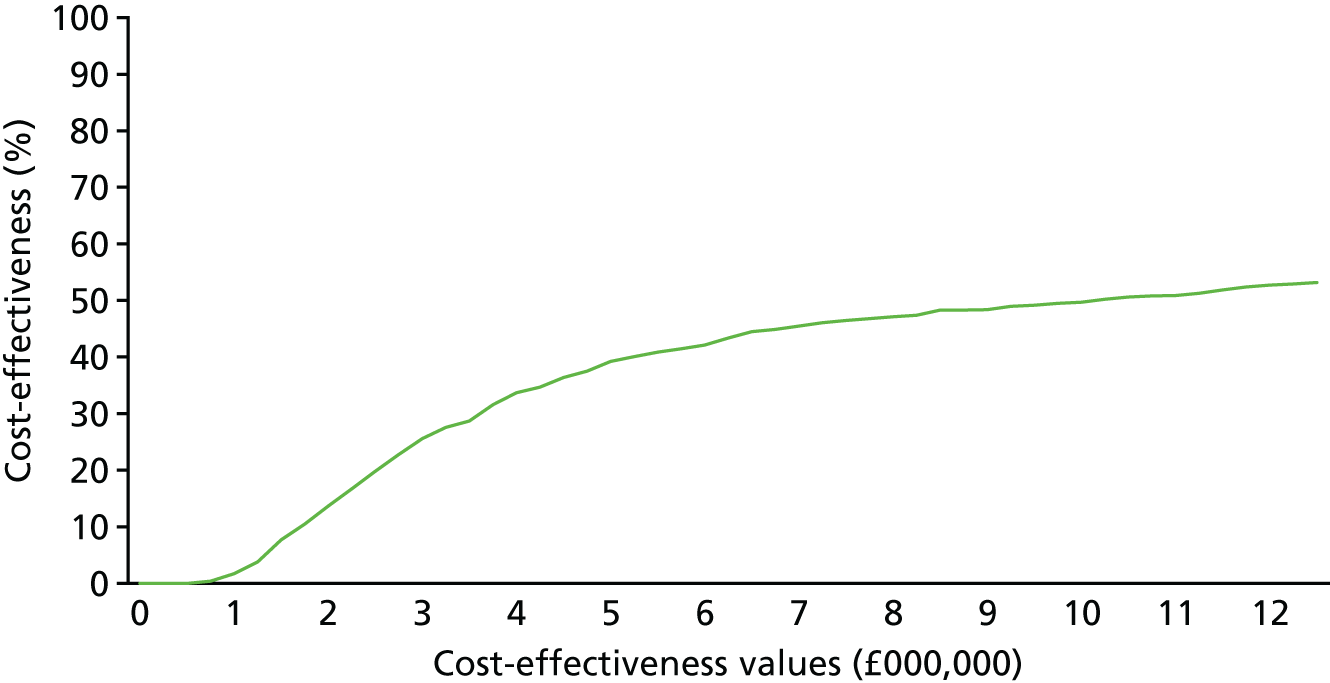
Discussion
Key results
As with all economic evaluations, the outcomes of the analyses are driven by the effects of an intervention as well as the costs. In the case of this analysis, the costs incurred by the intervention are large, overwhelming the benefits. The disaggregated outcomes derived from the intervention were balanced against the costs in the CCA. As shown in Chapter 2, the change in emergency admissions following the intervention was non-significant. This could be interpreted to mean that the investments, whether the lower bottom-up or the higher top-down reported cost, have not delivered identifiable health benefits in terms of reduced costs to the health service. Similarly, in the CUA, which explored the impacts of the intervention on QALYs relating to the change in rate of emergency admissions for people with COPD, the small, non-significant reduction in emergency admissions is overpowered by the cost of the intervention. Therefore, the intervention cannot be considered cost-effective using commonly accepted norms, with or without discounting costs and benefits to present-day values in line with best practice. It is important to consider that this does not mean that the intervention is without benefit, but rather it may be that the benefits were not able to be identified and measured through the approaches we utilised.
Strengths and limitations
A strength of the current study was the use of routine data held within the SAIL databank. Unlike other health economic studies that focused on affordable warmth interventions, this study was able to use actual health-care utilisation data with near-complete data for the study population (see Chapter 2). However, the data set will have included people with a wide range of health problems of different stages of severity and no information was available regarding the initial quality of housing and, therefore, the relative improvement resulting from the intervention.
Another strength of the study was that data were used from both the data linkage and the community-based field study. Although routine data allow calculations to be based on actual health-care utilisation, emergency hospital admissions are only a proxy measure for health status. This type of contact with the health-care system can provide a signal that health is changing but will only be one part of the overall story. The community-based field study provided a more detailed understanding of the health, well-being and health-related quality-of-life impact of the intervention, and was used as a basis for a CUA. The results of that analysis are reported in Chapter 3.
It was not possible to undertake a CBA, an approach recommended by NICE as an option for evaluating public health interventions, because the available data did not facilitate identification and valuation of all of the impacts of the intervention to society and the full societal cost and consequences of the intervention. It could be useful to conduct such analyses in the future, considering that a housing intervention is likely to have a wide range of benefits beyond health improvements alone. The community-based study showed that the intervention provided a wide range of psychosocial and economic benefits to residents. It is recognised by NICE, however, that CBA poses challenges in terms of obtaining all necessary data to undertake analysis. It was beyond the scope of the study to value these benefits.
We explored the impact of the intervention for up to 10 years (i.e. the probable lifespan of the shortest-lived elements of the intervention), but the effects of the intervention are plausibly longer. A study using longer-term data and a longer-term time horizon may deliver different results.
Interpretation
When trying to determine whether or not an intervention delivers good value for money, the intervention does not necessarily have to be cost-sparing or cost-effective in terms of what would be acceptable for a clinical intervention. Public health decision-makers and budget holders may feel that the goals of the intervention – in this case the delivery of affordable warmth, alleviation of fuel poverty and reduction of CO2 emissions – justify the costs of the intervention. The fact that our economic analysis suggests that the intervention incurred major costs but did not lead to detectable cost reductions to the health service or improvements in QALYs for people with COPD does not mean that the intervention is without benefit. Indeed, the community-based study showed that the intervention provided a range of social benefits and made it easier and cheaper for households in low-income areas to heat their homes. In addition, the health benefits may emerge over a longer period than the 4-year horizon used in this study, especially as a prevention intervention. A child growing up in a property may not have health impairments that he or she may otherwise have had without the improvements.
The Gentoo140 and Warm Homes for Health studies,141 which found health improvements and cost savings after housing improvements, were studies that targeted people with poor housing and the capacity to benefit healthwise. The CUA undertaken with the COPD subpopulation may have been limited by a similar problem. COPD that is sufficiently severe enough to present a risk of hospital admission is defined as moderate to severe. 160 People with moderate to severe COPD are prone to frequent exacerbations (three or more exacerbations per year is part of the definition) and these are an important cause of hospital admission and readmission and, therefore, have a considerable impact on health-related quality of life and daily activities. 150 Reducing exacerbation frequency through improved housing for these people might be more valuable than for others with less-severe COPD, and might spare more health-care resources. However, a hospital admission may be precipitated by a lack of social support and comorbidities rather than severity, which our study data were not able to reveal, but an exacerbation avoided by someone who might fall into this category may have additional value. Focusing an analysis on all such patients may reveal a different picture to the one our present analysis suggests.
Generalisability
This economic study and our findings would be generalisable to a developed country in a temperate region where a national health service exists founded on the principles of solidarity and tax-funded health care.
Chapter 6 Conclusion
Introduction
Improving the energy efficiency of existing housing stock not only has the potential to reduce fuel poverty and carbon emission but it can also help to improve the health of residents. 6 Research has shown that housing quality is associated with a range of health and psychosocial outcomes, and that provision of adequate, affordable warmth can lead to health improvements. However, a recent systematic review of warmth and energy efficiency studies concluded that evidence of the effectiveness of housing improvement is inconclusive, and that more robust research is needed to fill existing gaps in the literature. 48 In particular, limited follow-up periods have made it difficult to determine the long-term health impacts of housing interventions, few studies have focused on psychosocial outcomes that may be part of pathways to better health and only limited research has been conducted to determine whether or not affordable warmth interventions produce better indoor hydrothermal conditions.
In this project, we took the opportunity afforded by a major energy performance investment programme in Wales. The core objectives of the investment programme were to deliver affordable warmth, alleviate fuel poverty and reduce CO2 emissions through area-based energy efficiency improvements. We examined whether or not these investments provided additional health benefits to residents living in low-income areas in Wales. This was done through a mixed-methods programme of work, including data linkage (see Chapter 2), a community-based study (see Chapter 3), household monitoring (see Chapter 4) and a health economic assessment (see Chapter 5). In addition, reconvened focus groups were conducted as part of the wider resident engagement in the project (see Appendix 2).
In this final chapter, we will summarise the principal findings of the different studies, followed by a discussion of the main contributions and implications of these findings, as well as the strengths and limitations of the research. The chapter will be concluded with recommendations for further research.
Key findings
The research provided empirical evidence regarding the health and psychosocial impacts of energy performance investments. The key findings of the different studies are reported in the following sections.
The community-based study and the reconvened focus groups
The community-based study found no evidence that the improvements in energy efficiency lead to better mental and physical health in the short term. The intervention was associated with neither improvements in key mental and physical health outcomes nor changes in self-reported respiratory and asthma-related symptoms. However, quantitative evidence was found that the intervention led to improved subjective well-being during the study period, as well as to improvements in a number of psychosocial outcomes that may be part of pathways to better health. This included increased thermal satisfaction, fewer reported financial difficulties, increased satisfaction among participants with the repair of their homes, fewer reported housing-related problems and more social interactions.
These findings were reflected in the focus group discussions that were conducted as part of the resident engagement. The longitudinal focus groups showed the importance of improving the energy efficiency of houses in low-income neighbourhoods. 161 According to residents, the intervention made a great difference to the comfort and warmth of their homes, opened up spaces within the home and reduced their heating bills substantially. This not only helped to relieve financial stress and fuel poverty but made them feel less socially isolated. This confirms that the benefits of the improvements were, at least in the short term, more closely linked to better well-being owing to improved socioeconomic conditions than to physical health outcomes. Experiences with the delivery of the intervention were mixed, which some residents found disempowering and stressful.
The household monitoring study
The household monitoring study demonstrated that the improvements in energy efficiency raised indoor air temperatures and helped households to reduce their energy use. The increase in air temperature was consistent across different heating demand conditions. Although no evidence was found that the intervention reduced the duration of substandard temperatures within the homes, there was a small decrease in the cumulative amount of substandard temperatures (i.e. the time intensity integral of substandard temperatures). However, this reduction was small in absolute terms.
The different energy efficiency measures were not equally effective in raising indoor air temperatures. External wall insulation and connection to the gas mains network were the most effective, whereas new windows and doors or a new heating system did not lead to significant changes in indoor air temperature. The greatest increases in indoor air temperatures were found in British steel-framed (BISF) buildings and buildings with solid walls, which had the lowest energy performance ratings before the intervention. That indoor air temperatures increased most in the evening and at night, as well as in the living room and main bedroom, suggests that the intervention makes the biggest difference when spaces are in use.
Overall, no evidence was found that the intervention increased indoor RH levels. However, some evidence was found that the intervention decreased the duration and cumulative amount of substandard temperatures. Although no evidence was found that the intervention increased indoor RH levels overall, some evidence indicated that individual measures did. Both a connection to the gas mains network and the installation of new windows and doors were associated with small increases in indoor RH levels. However, the increases were small in absolute terms.
The data linkage study
The data linkage study found no evidence that the intervention reduced emergency admissions for either the primary outcome (combined cardiorespiratory emergency hospital admissions) or most secondary outcomes (emergency hospital admissions for cardiovascular, respiratory and COPD conditions, and excess winter admissions) for people of all ages living in an intervention home. The study also did not find evidence of a significant intervention effect on our primary outcome when focused only on residents aged ≥ 60 years at the mid-point of their residence in an intervention home. However, the study did find a statistically significant intervention effect on cardiovascular-related emergency admissions: there was an increase in emergency cardiovascular admissions post intervention for those aged ≥ 60 years. Although we controlled for age in our analyses, it is possible our analyses have not taken all effects of ageing into account. It may be that age does not have a linear response and the older people in this group are increasingly frail, having had poor health conditions set in motion at an earlier age, working to outweigh the potential for reduced admissions as a result of a single thermal efficiency intervention.
The economic evaluation
The CCA undertaken as part of the economic analysis did not find evidence that the intervention delivered explicit reductions in cardiorespiratory-related emergency admissions. The costs of achieving this outcome relate to the costs of delivering the intervention unmodified by savings in emergency admissions. However, the purpose of undertaking CCA is to present costs and benefits separately, allowing the reader or decision-maker to make their own decision about the relative value of the benefits compared with the costs. As reported previously, there were social benefits from the intervention, albeit not demonstrably seen in emergency admissions. The increased warmth and well-being derived from housing improvements may well be considered worthwhile irrespective of the impact on health-care activity.
The CUA explored, through modelling methods, the impact on QALYs of the change in rate of emergency admissions for people with COPD. However, the impact on COPD-related emergency admissions is overpowered by the cost of the intervention and, therefore, cannot be thought of as cost-effective using commonly accepted norms.
Implications of the research
The research found no demonstrable effects of the intervention on health or health service utilisation in the short and medium term. As a result, the health economic analysis concluded that the intervention might not be considered cost-effective in a traditional sense. These findings appear to be in line with a recent systematic review conducted by Thomson et al. 48 in that area-based programmes are less likely to produce improvements than interventions that specifically target at-risk populations. Emerging findings of other research suggest that Nest – the more targeted part of the Welsh Government Warm Homes programme – provides greater health benefits than the broader Arbed scheme that was evaluated in this programme of work. 162 It is nevertheless surprising that no apparent effects were found, given that the intervention programme targeted low-income neighbourhoods with poor-quality housing and where, as a result, residents were at a higher risk of living in fuel poverty.
However, that does not mean that the intervention was without substantial benefit. The main purpose of the programme was to deliver affordable warmth, alleviate fuel poverty and reduce CO2 emissions. The research found clear evidence that this was the case. The research showed that the investments in energy efficiency provide a wide range of benefits to the lives and well-being of residents. It is not unlikely that this may produce value in terms of benefits to the NHS and social services in the longer term. The time horizon of 4 years may still be insufficient for the health benefits of the investments to have materialised. 48 However, even without such demonstrable health improvements, it may be worth investing in the energy efficiency of substandard homes if it provides better living conditions and quality of life for low-income communities. Although it was not possible to undertake a full CBA, future research may consider the wider social benefits of delivered affordable warmth to low-income households, reduced fuel poverty and reduced CO2 emissions.
The project trialled a new approach to analyse household monitoring data, which can serve as a useful model for further evaluations of housing improvement programmes. Variants of interrupted time series analysis can be used to make robust assessments with relatively small sample sizes. It is often unclear whether residents receiving energy efficiency improvements use them for extra warmth or benefit in a different way. 133,134 Researchers have observed greater temperature increases following energy efficiency improvements among low-income groups, most likely because low-income groups may have a greater unmet demand for space heating. 163 The household monitoring study found evidence that the rebound effects following the intervention were relatively small. The benefits of energy efficiency improvements were taken as energy saving as well as extra warmth, suggesting that the potential long-term health effects of energy efficiency improvements may work via the two main pathways of improved thermal living conditions and more-affordable heating. 54–56
The focus groups study highlighted the importance of involving residents in the decision-making regarding affordable warmth interventions. Although residents were overwhelmingly positive about the intervention programme, and felt that it had made a big difference to their lives, they were more critical about its delivery. In line with previous research, some residents reported feelings of disempowerment and stress, which may have partly offset any potential benefits. 39,53,61 Extensive consultation and involvement throughout a housing improvement programme may help to minimise disruption to residents. 91
Strengths and weaknesses of the research
One of the strengths of the research is that it provided a comprehensive evaluation of a policy-led affordable warmth programme through a longitudinal multimethod investigation comprising data linkage, a field study, household monitoring, qualitative focus groups with residents and a health economic assessment. The different elements were combined to highlight areas in which results converge and supplement each other. Although each method has its own strengths and weaknesses, as discussed in the different empirical chapters, together they provide a more complete picture than could be obtained from a single method alone. 164
This research demonstrates the need for detailed assessment of policy-led interventions in order to fully understand their impacts, and provides a toolkit for how this might be done in the future. Although data linkage can be used to link the intervention to actual health service utilisation, the field study covers more-subjective aspects resulting from the intervention. The household monitoring has helped to assess the differential impacts depending on construction type and intervention measure. Furthermore, the research has shown that more-qualitative research methods can help to improve our understanding of the choices people make following affordable warmth interventions.
The research has a number of limitations. Most notably, it was not possible to randomise participation into intervention and control groups and, as with any observational study, the potential for unmeasured confounding remains. Both the community-based and household monitoring data relied on the responsiveness of residents and were therefore subject to a number of biases. Response rates and retention rates were particularly low for the field study. This was countered by the data linkage study, which minimised selection and attrition biases with near-complete follow-up. However, data quality was also an issue. The effort required to validate and clean intervention records received from data providers was considerable, and there were missing data for many intervention homes. Furthermore, the data linkage study was only able to investigate emergency hospital admissions. The lack of association with emergency hospital admissions may indicate that the focus of the research was too narrow and the follow-up time too short. It is possible that the health benefits will emerge over a longer period than the 4-year horizon used in this study, especially as a preventative intervention. The finding that the intervention provides a wide range of psychosocial outcomes suggests that a full CBA needs to be conducted to fully appreciate the benefits of affordable warmth improvements.
The research has highlighted the challenges of evaluating complex social interventions. 116,117 Evaluators generally do not have control over the content and delivery of the programme, nor the resources and costs relating to the programme and data regarding the timing may not be in the format or of the quality that is needed. There are added complexities of house moves and non-standard delivery of the intervention. Early involvement may help to address these challenges by developing designs and data collection that would provide the best quality of evidence. Equally, understanding of the resources deployed, their costs and how they could be influenced or changed might improve the balance of costs and benefits of the programme. Early consideration of evaluation methods may also help to increase response and retention rates.
Recommendations for further research
Evaluating housing interventions is challenging owing to a number of factors that affect their quality. We make the following recommendations for future research.
-
Our research suggests the importance of incorporating health and economic evaluations as part of housing improvement programmes from the start. A stepped wedge randomisation in the delivery of a programme, together with improved reporting standards regarding the timing, delivery and costs of the intervention, would help to improve the quality of both prospective and retrospective evaluations.
-
In the research, each component covered a different aspect of the evaluation. Data linkage in combination with a field study, household monitoring and qualitative work can be used as a model for the evaluation of future housing interventions to provide a comprehensive overview of their impact. Process evaluation should become a core part of testing complex social interventions. 116,165
-
The lack of results in the research presented here may, as suggested by Thomson et al. ,48 indicate that area-based programmes are less likely to produce measurable health improvements than those that specifically target at-risk populations. A direct comparison between different types of programmes, for example between the area-based Arbed and the demand-led Nest schemes, would help to determine which approach leads to the greatest health improvements.
-
The research found no evidence for changes in emergency admissions associated with the intervention. One explanation is that the health benefits of housing interventions do not appear in hospital statistics. Future research may therefore need to focus on lower-level health conditions as recorded in primary health-care consultations.
-
Future evaluations should ideally have longer follow-up periods and use CBA to fully capture the range of benefits resulting from affordable warmth intervention, including both health and non-health outcomes.
-
Long-term monitoring of internal and external hydrothermal conditions, in combination with detailed monitoring of energy consumption and household behaviours, would help to improve our understanding of adaptive responses following energy efficiency investments. 166,167
Acknowledgements
We would like to thank the Welsh Government and the two Arbed scheme managers, Melin Homes and Willmott Dixon, for their support, the LAs involved in the selection of Arbed schemes for their valuable input and the Communities First groups in South Wales for their advice regarding several aspects of the design of the community-based study.
We are grateful to the members of the SSC, John MacLeod, Simon Nicol, Sarah Lowe, John Mason, Tim Mann, Huw Brunt, Hilary Thomson and Paul Wilkinson, for their valuable advice throughout the project. We would also like to thank the members of the resident panel, Carol Ann Anthony, John Power, Karen Harris and Zoe Taylor, for their participation in this project and advice on how to disseminate the findings of the research.
The study made use of anonymised data held in the SAIL databank, which is part of the national health records research infrastructure for Wales. The authors would like to acknowledge all the data providers who make anonymised data available for research. The authors would like to thank Sarah Lowe and John Mason of the Welsh Government for their help with collecting the housing intervention records. Similarly, we are grateful to the Welsh Government and the 28 LAs and RSLs who provided us with their housing intervention records.
The study involved a community-based study, a household monitoring study and a reconvened focus group study that was part of resident engagement. We would like to thank all participants for their time and willingness to fill out the survey, have their house monitored and to share their views in the focus group discussions. Without their help this work would not have been possible.
Finally, we would like to thank Christina Nascimento, Meng Zhang, Tina Schmieder-Gaite and Katerina Chatzivasileiadi for their help with the literature review and analyses of the community-based and household monitoring studies, Toni Cardew for her assistance with the literature review supporting the economic modelling and Martina Stefani and Gabriela Zapata-Lancaster for their help with conducting the focus group interviews.
Contributions of authors
Wouter Poortinga (Professor of Environmental Psychology) conceived the research, managed the overall project, supervised the statistical analyses for the community-based and household monitoring studies and led the writing of the report.
Sarah E Rodgers (Associate Professor in Spatial Epidemiology) conceived the research, led the SAIL data linkage part of the research and contributed to the writing of the report.
Ronan A Lyons (Professor of Public Health) conceived the research and contributed to the use of the record-linked data sets and the writing of the report.
Pippa Anderson (Associate Professor in Health Economics) led the economic evaluation of the project and the writing of Chapter 5.
Chris Tweed (Professor of Sustainable Design, Head of School of the Welsh School of Architecture) conceived the research and provided expert advice on the household monitoring component of the research.
Charlotte Grey (Research Assistant in Public Health) co-ordinated and conducted the fieldwork for the community-based study, contributed to the data collection of the household monitoring study, conducted the statistical analysis of the community-based study and led the writing of Chapter 3. She collected the housing intervention records from the 28 LAs and RSLs that were used for the data linkage, led the reconvened focus group study that was a component of the resident engagement and organised the drop-in dissemination meetings.
Shiyu Jiang (Research Assistant in Household Monitoring) co-ordinated and conducted the fieldwork for the household monitoring study, contributed to the data collection of the community-based study, conducted the statistical analysis of the household monitoring study and led the writing of Chapter 4. He collected the housing intervention records from the 28 LAs and RSLs that were used for the data linkage, contributed to the reconvened focus group study that was part of the resident engagement and organised the drop-in dissemination meetings.
Rhodri Johnson (Data Analyst) contributed to the preparation and coding of the PEDW data sets used for the SAIL data linkage and health economic components of the project and contributed to the writing of the report.
Alan Watkins (Associate Professor in Medical Statistics) led the statistical analyses of the SAIL data linkage, contributed to the preparation and coding of the PEDW data sets and led the writing of Chapter 2.
Thomas G Winfield (Research Assistant in Health Economics) conducted the health economic analyses and contributed to the writing of the report.
Publications
Poortinga W, Grey C, Jiang S, Rodgers SE, Johnson RD, Lyons RA, et al. Short-term health and social impacts of energy-efficiency investments in low-income communities: a controlled field study. Lancet 2016;388:S96.
Grey CNB, Poortinga W, Jiang S, Nascimento C, Rodgers SE, Johnson RD, Lyons RA. The short-term health and psychosocial impacts of domestic energy-efficiency investments in low-income areas: a controlled before-and-after study. BMC Public Health 2017;17:140.
Poortinga W, Jiang S, Grey CNB, Tweed C. Impacts of energy-efficiency investments on internal conditions in low-income households. Building Res Inf 2017;1–15.
Grey CNB, Schmieder-Gaite T, Jiang S, Nascimento C, Poortinga W. Cold homes, fuel poverty and energy efficiency improvements: a longitudinal focus group approach. Indoor Built Environ 2017;26:902–13.
Data sharing statement
All data queries and requests should be submitted to the corresponding author for consideration in the first instance. Please note that data collected as part of the community-based study, household monitoring study and reconvened focus group study can be supplied from the corresponding author directly. However, access to data held within the SAIL databank is governed by the SAIL IGRP and data access requests should be submitted to saildatabank@swansea.ac.uk referencing ’Arbed 0273’. Access to the intervention data will require approval from the appropriate data custodians. The corresponding author can advise as appropriate. In all cases, access to available anonymised data may be granted following review.
Disclaimers
This report presents independent research funded by the National Institute for Health Research (NIHR). The views and opinions expressed by authors in this publication are those of the authors and do not necessarily reflect those of the NHS, the NIHR, NETSCC, the PHR programme or the Department of Health and Social Care. If there are verbatim quotations included in this publication the views and opinions expressed by the interviewees are those of the interviewees and do not necessarily reflect those of the authors, those of the NHS, the NIHR, NETSCC, the PHR programme or the Department of Health and Social Care.
References
- Liddell C, Guiney C. Living in a cold and damp home: frameworks for understanding impacts on mental well-being. Public Health 2015;129:191-9. https://doi.org/10.1016/j.puhe.2014.11.007.
- Reynolds MT. The housing of the poor in American cities. Publ Am Econ Assoc 1893;8:9-132.
- Weber G. Improved tenement for American cities. Munic Aff 1897;1:745-62.
- Liddell C, Morris C. Fuel poverty and human health: a review of recent evidence. Energy Policy 2010;38:2987-97. https://doi.org/10.1016/j.enpol.2010.01.037.
- Roys M, Davidson M, Nicol S, Ormandy D, Ambrose P. The Real Cost of Poor Housing. Bracknell: BRE Press; 2009.
- The Health Impacts of Cold Homes and Fuel Poverty. London: Friends of the Earth and the Marmot Review Team; 2011.
- Healy JD. Excess winter mortality in Europe: a cross country analysis identifying key risk factors. J Epidemiol Community Heal 2003;57:784-9. https://doi.org/10.1136/jech.57.10.784.
- Wilkinson P, Landon M, Armstrong B, Stevenson S, McKee M. Cold Comfort: The Social and Environmental Determinants of Excess Winter Deaths in England, 1986–96. Bristol: The Policy Press and the Joseph Rowntree Foundation; 2001.
- Housing, Energy and Thermal Comfort: A Review of 10 Countries Within the WHO European Region. Copenhagen: World Health Organization; 2007.
- Health and Winter Warmth: Reducing Health Inequalities. London: Department of Health; 2007.
- Excess Winter Deaths and Illness and the Health Risks Associated with Cold Homes. London: National Institute for Health and Care Excellence; 2015.
- Excess Winter Mortality in England and Wales: 2014/15 (Provisional) and 2013/14 (Final). London: Office for National Statistics; 2015.
- Mitchell R, Blane D, Bartley M. Elevated risk of high blood pressure: climate and the inverse housing law. Int J Epidemiol 2002;31:831-8. https://doi.org/10.1093/ije/31.4.831.
- Woodhouse PR, Khaw KT, Plummer M. Seasonal variation of blood pressure and its relationship to ambient temperature in an elderly population. J Hypertens 1993;11:1267-74. https://doi.org/10.1097/00004872-199311000-00015.
- Crawford VL, McCann M, Stout RW. Changes in seasonal deaths from myocardial infarction. QJM 2003;96:45-52. https://doi.org/10.1093/qjmed/hcg005.
- Keatinge WR. Winter mortality and its causes. Int J Circumpolar Health 2002;61:292-9. https://doi.org/10.3402/ijch.v61i4.17477.
- Fares A. Winter cardiovascular diseases phenomenon. N Am J Med Sci 2013;5:266-79. https://doi.org/10.4103/1947–2714.110430.
- Howieson SG, Hogan M. Multiple deprivation and excess winter deaths in Scotland. J R Soc Promot Health 2005;125:18-22. https://doi.org/10.1177/146642400512500110.
- Rudge J, Gilchrist R. Excess winter morbidity among older people at risk of cold homes: a population-based study in a London borough. J Public Health 2005;27:353-8. https://doi.org/10.1093/pubmed/fdi051.
- Rudge J, Gilchrist R. Measuring the health impact of temperatures in dwellings: investigating excess winter morbidity and cold homes in the London Borough of Newham. Energy Build 2007;39:847-58. https://doi.org/10.1016/j.enbuild.2007.02.007.
- Webb E, Blane D, de Vries R. Housing and respiratory health at older ages. J Epidemiol Community Health 2013;67:280-5. https://doi.org/10.1136/jech-2012-201458.
- Platt SD, Martin CJ, Hunt SM, Lewis CW. Damp housing, mould growth, and symptomatic health state. BMJ 1989;298:1673-8. https://doi.org/10.1136/bmj.298.6689.1673.
- Dales RE, Burnett R, Zwanenburg H. Adverse health effects among adults exposed to home dampness and molds. Am Rev Respir Dis 1991;143:505-9. https://doi.org/10.1164/ajrccm/143.3.505.
- Pirhonen I, Nevalainen A, Husman T, Pekkanen J. Home dampness, moulds and their influence on respiratory infections and symptoms in adults in Finland. Eur Respir J 1996;9:2618-22. https://doi.org/10.1183/09031936.96.09122618.
- Gunnbjörnsdóttir MI, Franklin KA, Norbäck D, Björnsson E, Gislason D, Lindberg E, et al. Prevalence and incidence of respiratory symptoms in relation to indoor dampness: the RHINE study. Thorax 2006;61:221-5. https://doi.org/10.1136/thx.2005.057430.
- Fisk WJ, Lei-Gomez Q, Mendell MJ. Meta-analyses of the associations of respiratory health effects with dampness and mold in homes. Indoor Air 2007;17:284-96. https://doi.org/10.1111/j.1600-0668.2007.00475.x.
- Peat JK, Dickerson J, Li J. Effects of damp and mould in the home on respiratory health: a review of the literature. Allergy 1998;53:120-8. https://doi.org/10.1111/j.1398-9995.1998.tb03859.x.
- Hopton J, Hunt S. The health effects of improvements to housing: a longitudinal study. Hous Stud 1996;11:271-86. https://doi.org/10.1080/02673039608720856.
- Harris J, Hall J, Meltzer H, Jenkins R, Oreszczyn T, McManus S. Health, Mental Health and Housing Conditions in England. London: National Centre for Social Research and Eaga Charitable Trust; 2010.
- Evans J, Hyndman S, Stewart-Brown S, Smith D, Petersen S. An epidemiological study of the relative importance of damp housing in relation to adult health. J Epidemiol Community Health 2000;54:677-86. https://doi.org/10.1136/jech.54.9.677.
- Ormandy D, Ezratty V. Thermal discomfort and health: protecting the susceptible from excess cold and excess heat in housing. Adv Build Energy Res 2015;10:84-98. https://doi.org/10.1080/17512549.2015.1014845.
- Evans GW. The built environment and mental health. J Urban Health 2003;80:536-55. https://doi.org/10.1093/jurban/jtg063.
- Shenassa ED, Daskalakis C, Liebhaber A, Braubach M, Brown M-J. Dampness and mold in the home and depression: an examination of mold-related illness and perceived control of one’s home as possible depression pathways. Am J Public Health 2007;97:1893-9. https://doi.org/10.2105/AJPH.2006.093773.
- Anderson W, White V, Finney A. Coping with low incomes and cold homes. Energy Policy 2012;49:40-52. https://doi.org/10.1016/j.enpol.2012.01.002.
- Press V. Fuel Poverty and Health: A Guide for Primary Care Organisations, and Public Health and Primary Care Professionals. London: National Heart Forum; 2003.
- Gibbons D, Singler R. Cold comfort: a review of coping strategies used by households in fuel poverty. Diabetes Forecast 2008;61:57-61.
- Wright F. Old and cold: older people and policies failing to address fuel poverty. Soc Policy Adm 2004;38:488-503. https://doi.org/10.1111/j.1467-9515.2004.00403.x.
- O’Neill T, Jinks C, Squire A. Heating is more important than food. J Hous Elderly 2006;20:95-108. https://doi.org/10.1300/J081v20n03_07.
- Harrington BE, Heyman B, Merleau-Ponty N, Stockton H, Ritchie N, Heyman A. Keeping warm and staying well: findings from the qualitative arm of the Warm Homes Project. Health Soc Care Community 2005;13:259-67. https://doi.org/10.1111/j.1365-2524.2005.00558.x.
- Speak S. Back to the well: the hidden costs of service exclusion for the network poor. J Consum Stud Home Econ 2000;24:49-5. https://doi.org/10.1046/j.1365-2737.2000.00124.x.
- Doble M. A regulatory policy for self-disconnection: an examination of the reasons for and implications of pre-payment meter stoppages. Policy Stud 2010;21:229-43. https://doi.org/10.1080/01442870020019516.
- Day R, Hitchings R. ‘Only old ladies would do that’: age stigma and older people’s strategies for dealing with winter cold. Heal Place 2011;17:885-94. https://doi.org/10.1016/j.healthplace.2011.04.011.
- Brunner K-M, Spitzer M, Christanell A. Experiencing fuel poverty: coping strategies of low-income households in Vienna/Austria. Energy Policy 2012;49:53-9. https://doi.org/10.1016/j.enpol.2011.11.076.
- Brunner K-M, Mandl S, Christanell A. ECEEE Summer Study Proceedings. Stockholm: European Council for an Energy Efficient Economy; 2013.
- Bhattacharya J, DeLeire T, Haider S, Currie J. Heat or eat? Cold-weather shocks and nutrition in poor American families. Am J Public Health 2003;93:1149-54. https://doi.org/10.2105/AJPH.93.7.1149.
- Beatty TKM, Blow L, Crossley TF. Is there a ‘heat-or-eat’ trade-off in the UK?. J R Stat Soc Ser A Stat Soc 2014;177:281-94. https://doi.org/10.1111/rssa.12013.
- Kempson E, McKay S, Willets M. Characteristics of Families in Debt and the Nature of Indebtedness. London: Department for Work and Pensions; 2004.
- Thomson H, Thomas S, Sellstrom E, Petticrew M. Housing improvements for health and associated socio-economic outcomes. Cochrane Database Syst Rev 2013;2. https://doi.org/10.1002/14651858.CD008657.pub2.
- Howden-Chapman P, Matheson A, Crane J, Viggers H, Cunningham M, Blakely T, et al. Effect of insulating existing houses on health inequality: cluster randomised study in the community. BMJ 2007;334. https://doi.org/10.1136/bmj.39070.573032.80.
- Howden-Chapman P, Pierse N, Nicholls S, Gillespie-Bennett J, Viggers H, Cunningham M, et al. Effects of improved home heating on asthma in community dwelling children: randomised controlled trial. BMJ 2008;337. https://doi.org/10.1136/bmj.a1411.
- Barton A, Basham M, Foy C, Buckingham K, Somerville M. Torbay Healthy Housing Group . The Watcombe Housing Study: the short term effect of improving housing conditions on the health of residents. J Epidemiol Community Health 2007;61:771-7. https://doi.org/10.1136/jech.2006.048462.
- Braubach M, Heinen D, Dame J. Preliminary Results of the WHO Frankfurt Housing Intervention Project. Copenhagen: World Health Organization; 2008.
- Shortt N, Rugkåsa J. ‘The walls were so damp and cold’ fuel poverty and ill health in Northern Ireland: results from a housing intervention. Health Place 2007;13:99-110. https://doi.org/10.1016/j.healthplace.2005.10.004.
- Thomson H, Thomas S. Developing empirically supported theories of change for housing investment and health. Soc Sci Med 2015;124:205-14. https://doi.org/10.1016/j.socscimed.2014.11.043.
- Willand N, Ridley I, Maller C. Towards explaining the health impacts of residential energy efficiency interventions – a realist review. Part 1: pathways. Soc Sci Med 2015;133:191-20. https://doi.org/10.1016/j.socscimed.2015.02.005.
- Gilbertson J, Grimsley M, Green G. Psychosocial routes from housing investment to health: evidence from England’s home energy efficiency scheme. Energy Policy 2012;49:122-33. https://doi.org/10.1016/j.enpol.2012.01.053.
- Jiang S, Grey C, Poortinga W, . Winter Indoor Air Temperature and Relative Humidity in Hard-To-Heat, Hard-To-Treat Houses in Wales: Results from a Household Monitoring Study. Cardiff: Welsh School of Architecture, Cardiff University; 2015.
- Hills J. Getting the Measure of Fuel Poverty. London: Department of Energy and Climate Change; 2012.
- Bonnefoy X. Inadequate housing and health: an overview. Int J Environ Pollut 2007;3/4:411-29. https://doi.org/10.1504/IJEP.2007.014819.
- Caldwell J, McGowan S, McPhail J, McRae C, Morris G, Murray K, et al. Glasgow Warm Homes Study: Final Report. Glasgow: Glasgow City Council Housing Services; 2001.
- Gilbertson J, Stevens M, Stiell B, Thorogood N. Home is where the hearth is: grant recipients’ views of England’s Home Energy Efficiency Scheme (Warm Front). Soc Sci Med 2006;63:946-56. https://doi.org/10.1016/j.socscimed.2006.02.021.
- Platt S, Mitchell R, Pettigrew M, Walker J, Hopton J, Martin C, et al. The Scottish Executive Central Heating Programme: Assessing Impacts on Health. Edinburgh: Scottish Executive Social Research; 2007.
- Oreszczyn T, Hong SH, Ridley I, Wilkinson P. The Warm Front Study Group . Determinants of winter indoor temperatures in low income households in England. Energy Build 2006;38:245-52. https://doi.org/10.1016/j.enbuild.2005.06.006.
- Bone A, Murray V, Myers I, Dengel A, Crump D. Will drivers for home energy efficiency harm occupant health?. Perspect Public Health 2010;130:233-8. https://doi.org/10.1177/1757913910369092.
- Laverge J, Van Den Bossche N, Heijmans N, Janssens A. Energy saving potential and repercussions on indoor air quality of demand controlled residential ventilation strategies. Build Environ 2011;46:1497-503. https://doi.org/10.1016/j.buildenv.2011.01.023.
- Arundel AV, Sterling EM, Biggin JH, Sterling TD. Indirect health effects of relative humidity in indoor environments. Environ Health Perspect 1986;65:351-61.
- Baughman AV, Arens EA. lndoor humidity and human health – Part I: Literature review of health effects of humidity-influences indoor pollutants. ASHRAE Trans 1996;102:192-211.
- Sharpe RA, Thornton CR, Nikolaou V, Osborne NJ. Fuel poverty increases risk of mould contamination, regardless of adult risk perception and ventilation in social housing properties. Environ Int 2015;79:115-29. https://doi.org/10.1016/j.envint.2015.03.009.
- Bornehag CG, Sundell J, Hägerhed-Engman L, Sigsgaard T. Association between ventilation rates in 390 Swedish homes and allergic symptoms in children. Indoor Air 2005;15:275-80. https://doi.org/10.1111/j.1600-0668.2005.00372.x.
- Hägerhed-Engman L, Sigsgaard T, Samuelson I, Sundell J, Janson S, Bornehag CG. Low home ventilation rate in combination with moldy odor from the building structure increase the risk for allergic symptoms in children. Indoor Air 2009;19:184-92. https://doi.org/10.1111/j.1600-0668.2008.00573.x.
- Green G, Ormandy D, Brazier J, Gilbertson J, Nicol F, Rudge J. Cutting the Cost of Cold: Affordable Warmth for Healthier Homes. London: E & FN Spon; 2000.
- Hong SH, Ridley I, Oreszczyn T. The Warm Front Study Group . The impact of energy efficient refurbishment on the airtightness in English dwellings. 25th AVIC Conference 2004;221:7-12.
- Rodgers SE, Heaven M, Lacey A, Poortinga W, Dunstan FD, Jones KH, et al. Cohort profile: the housing regeneration and health study. Int J Epidemiol 2014;43:52-60. https://doi.org/10.1093/ije/dys200.
- Fenwick E, Macdonald C, Thomson H. Economic analysis of the health impacts of housing improvement studies: a systematic review. J Epidemiol Community Health 2013;67:835-45. https://doi.org/10.1136/jech-2012-202124.
- Fuel Poverty Strategy. Cardiff: Welsh Government; 2010.
- The Arbed Programme: Background. Cardiff: Welsh Government; 2012.
- Arbed – Strategic Energy Performance Investment Programme. Cardiff: Welsh Government; 2014.
- A Study of Hard to Treat Homes Using the English House Condition Survey. Part I: Dwelling and Household Characteristics of Hard to Treat Homes. London: Defra and Energy Saving Trust; 2008.
- Rodgers SE, Lyons RA, Dsilva R, Jones KH, Brooks CJ, Ford DV, et al. Residential Anonymous Linking Fields (RALFs): a novel information infrastructure to study the interaction between the environment and individuals’ health. J Public Health 2009;31:582-8. https://doi.org/10.1093/pubmed/fdp041.
- Grey CNB, Poortinga W, Jiang S, Nascimento C, Rodgers SE, Johnson RD, et al. The short-term health and psychosocial impacts of domestic energy-efficiency investments in low-income areas: a controlled before-and-after study. BMC Public Health 2017;17. https://doi.org/10.1186/s12889-017-4075-4.
- Von Elm E, Altman DG, Egger M, Popock SJ, Gøtzsche PC, Vandenbroucke JP. Strengthening the Reporting of Observational Studies in Epidemiology (STROBE) statement: guidelines for reporting observational studies. BMJ 2007;335:806-8. https://doi.org/10.1136/bmj.39335.541782.AD.
- Ford DV, Jones KH, Verplancke JP, Lyons RA, John G, Brown G, et al. The SAIL databank: building a national architecture for e-health research and evaluation. BMC Health Serv Res 2009;9. https://doi.org/10.1186/1472-6963-9-157.
- Lyons RA, Jones KH, John G, Brooks CJ, Verplancke JP, Ford DV, et al. The SAIL databank: linking multiple health and social care datasets. BMC Med Inform Decis Mak 2009;9. https://doi.org/10.1186/1472–6947–9-3.
- Rodgers SE, Demmler JC, Dsilva R, Lyons RA. Protecting health data privacy while using residence-based environment and demographic data. Heal Place 2011;18:209-17. https://doi.org/10.1016/j.healthplace.2011.09.006.
- International Classification of Diseases, Tenth Edition (ICD-10). Geneva: World Health Organization; 1992.
- Bottle A, Aylin P. Comorbidity scores for administrative data benefited from adaptation to local coding and diagnostic practices. J Clin Epidemiol 2011;64:1426-33. https://doi.org/10.1016/j.jclinepi.2011.04.004.
- Fone D, Morgan J, Fry R, Rodgers SE, Orford S, Farewell D, et al. Change in alcohol outlet density and alcohol-related harm to population health (CHALICE): a comprehensive record-linked database study in Wales. Public Heal Res 2016;4:1-184. https://doi.org/10.3310/phr04030.
- Rural/Urban Definition (England and Wales. London: Office for National Statistics; 2004.
- Excess Winter Mortality in England and Wales Statistical Bulletins. London: Office for National Statistics; 2014.
- Wilkinson P, Pattenden S, Armstrong B, Fletcher A, Kovats RS, Mangtani P, et al. Vulnerability to winter mortality in elderly people in Britain: population based study. BMJ 2004;329. https://doi.org/10.1136/bmj.38167.589907.55.
- Rodgers SE, Bailey R, Johnson R, Poortinga W, Smith R, Berridge D, et al. Health impact, and economic value, of meeting housing quality standards: a retrospective, longitudinal data linkage study. Public Health Res 2018.
- Rogers A, Huxley P, Evans S, Gately C. More than jobs and houses: mental health, quality of life and the perceptions of locality in an area undergoing urban regeneration. Soc Psychiatry Psychiatr Epidemiol 2008;43:364-72. https://doi.org/10.1007/s00127-008-0316-2.
- Steele J, Bourke L, Luloff AE, Liao PS, Theodori GL, Krannich RS. The drop-off/pick-up method for household survey research. J Community Dev Soc 2001;32:238-50. https://doi.org/10.1080/15575330109489680.
- Ware J, Kosinski M, Turner-Bowker D, Gandek B. How to Score Version 2 of the SF-12 Health Survey. Lincoln, RI: Quality Metric Incorporated; 2005.
- WHO Guidelines for Indoor Air Quality: Dampness and Mould. Copenhagen: World Health Organization; 2009.
- Burney PG, Luczynska C, Chinn S, Jarvis D. The European Community Respiratory Health Survey. Eur Respir J 1994;7:954-60. https://doi.org/10.1183/09031936.94.07050954.
- Dolan P, Layard R, Metcalfe R. Measuring Subjective Well-being for Public Policy. London: Office for National Statistics; 2011.
- Howden-Chapman PL, Crane J, Baker M, Pierse N, Nicholls S, Viggers H, et al. Housing, Heating and Health Study: 2005/2006 Report One. Aims and Methods. Wellington: Wellington School of Medical and Health Science, University of Otago; 2006.
- Thomson H, Morrison D, Petticrew M. The health impacts of housing-led regeneration: a prospective controlled study. J Epidemiol Community Health 2007;61:211-14. https://doi.org/10.1136/jech.2006.049239.
- Rosengren A, Hawken S, Ounpuu S, Sliwa K, Zubaid M, Almahmeed WA, et al. Association of psychosocial risk factors with risk of acute myocardial infarction in 11119 cases and 13648 controls from 52 countries (the INTERHEART study): case-control study. Lancet 2004;364:953-62. https://doi.org/10.1016/S0140-6736(04)17019-0.
- Six-Item Short Form of the Food Security Survey Module. Washington, DC: US Department of Agriculture; 2012.
- Bickel G, Nord M, Price C, Hamilton W, Cook J. Guide to Measuring Household Food Security Revised 2000. Washington, DC: US Department of Agriculture; 2000.
- Poortinga W. The Carmarthenshire Homes Standard Health Impact Assessment Study: Technical Interim Report. Cardiff: Welsh School of Architecture; 2011.
- McManus S, Meltzer H, Brugha TS, Bebbington PE, Jenkins R. Adult Psychiatric Morbidity in England, 2007. Results of a Household Survey. London: NHS Information Centre for Health and Social Research; 2009.
- Brazier J, Roberts J, Deverill M. The estimation of a preference-based measure of health from the SF-36. J Health Econ 2002;21:271-92. https://doi.org/10.1016/S0167-6296(01)00130-8.
- Harris AD, McGregor JC, Perencevich EN, Furuno JP, Zhu J, Peterson DE, et al. The use and interpretation of quasi-experimental studies in medical informatics. J Am Med Inform Assoc 2006;13:16-23. https://doi.org/10.1197/jamia.M1749.
- Howden-Chapman P. How real are the health effects of residential energy efficiency programmes?. Soc Sci Med 2015;133:189-90. https://doi.org/10.1016/j.socscimed.2015.03.017.
- Field A. Discovering Statistics Using IBM SPSS Statistics. London: Sage; 2013.
- Rasbash J, Charlton C, Browne WJ, Goldstein H. MLwiN Version 2.36. Bristol: Centre for Multilevel Modelling, University of Bristol; 2016.
- Browne WJ. MCMC Estimation in MLwiN v2.1. Bristol: Centre for Multilevel Modelling, University of Bristol; 2009.
- Cohen J. Statistical Power Analysis for the Behavioral Sciences. Hillsdale, NJ: Lawrence Erlbaum Associates; 1988.
- Rice ME, Harris GT. Comparing effect sizes in follow-up studies: ROC Area, Cohen’s d, and r. Law Hum Behav 2005;29:615-20. https://doi.org/10.1007/s10979-005-6832-7.
- Mark M. User’s Manual for the SF-12v2 Health Survey. Lincoln, RI: QualityMetric Incorporated; 2012.
- Parry O, Bancroft A, Gnich W, Amos A. Nobody home? Issues of respondent recruitment in areas of deprivation. Crit Public Health 2001;11:305-17. https://doi.org/10.1080/09581590110094585.
- Thomson H, Thomas S, Sellstrom E, Petticrew M. The health impacts of housing improvement: a systematic review of intervention studies from 1887 to 2007. Am J Public Health 2009;99:681-92. https://doi.org/10.2105/AJPH.2008.143909.
- Craig P, Dieppe P, Macintyre S, Michie S, Nazareth I, Petticrew M. Medical Research Council Guidance . Developing and evaluating complex interventions: the new Medical Research Council guidance. BMJ 2008;337. https://doi.org/10.1136/bmj.a1655.
- Lindholm L, Rosén M. What is the ‘golden standard’ for assessing population-based interventions? Problems of dilution bias. J Epidemiol Community Health 2000;54:617-22. https://doi.org/10.1136/jech.54.8.617.
- Osman LM, Ayres JG, Garden C, Reglitz K, Lyon J, Douglas JG. A randomised trial of home energy efficiency improvement in the homes of elderly COPD patients. Eur Respir J 2010;35:303-9. https://doi.org/10.1183/09031936.00187708.
- Poortinga W, Jiang S, Grey CNB, Tweed C. Impacts of energy-efficiency investments on internal conditions in low-income households. Building Res Inf 2017:1-15.
- Richardson G, Barton A, Basham M, Foy C, Eick SA, Somerville M, et al. The Watcombe housing study: the short-term effect of improving housing conditions on the indoor environment. Sci Total Environ 2006;361:73-90. https://doi.org/10.1016/j.scitotenv.2005.05.007.
- Hong SH, Oreszczyn T, Ridley I. The impact of energy efficient refurbishment on the space heating fuel consumption in English dwellings. Energy Build 2006;38:1171-81. https://doi.org/10.1016/j.enbuild.2006.01.007.
- Reddy TA. Applied Data Analysis and Modeling for Energy Engineers and Scientists. Boston, MA: Springer; 2011.
- Building Research Establishment Domestic Energy Model (BREDEM). Watford: Building Research Energy Conservation Support Unit; 1997.
- Evidence Review and Economic Analysis of Excess Winter Deaths. Review 3: Delivery and Implementation of Approaches for the Prevention of Excess Winter Deaths and Morbidity. London: National Institute for Health and Care Excellence; 2014.
- Cold Weather Plan for England 2013. London: Public Health England; 2013.
- Degree-Days: Theory and Application. TM41. London: Chartered Institution of Building Services Engineers; 2006.
- Hitchin ER. Estimating monthly degree-days. Build Serv Eng Res Technol 1983;4:159-62. https://doi.org/10.1177/014362448300400404.
- Hong SH, Gilbertson J, Oreszczyn T, Green G, Ridley I. The Warm Front Study Group . A field study of thermal comfort in low-income dwellings in England before and after energy efficient refurbishment. Build Environ 2009;44:1228-36. https://doi.org/10.1016/j.buildenv.2008.09.003.
- Valor E, Meneu V, Caselles V. Daily air temperature and electricity load in Spain. J Appl Meteorol 2001;40:1413-21. https://doi.org/10.1175/1520-0450(2001)040<1413:DATAEL>2.0.CO;2.
- Goldstein H. Multilevel Statistical Models. London: John Wiley & Sons; 2011.
- Lopez Bernal J, Cummins S, Gasparrini A. Interrupted time series regression for the evaluation of public health interventions: a tutorial. Int J Epidemiol 2017;46:348-55.
- Jones K, Subramanian SV. Developing Multilevel Models for Analysing Contextuality, Heterogeneity and Change Using MLwiN, Volume 2. Bristol: Centre for Multilevel Modeling, University of Bristol; 2011.
- Milne G, Boardman B. Making cold homes warmer: the effect of energy efficiency improvements in low-income homes. A report to the Energy Action Grants Agency Charitable Trust. Energy Policy 2000;28:411-24. https://doi.org/10.1016/S0301-4215(00)00019-7.
- Chitnis M, Sorrell S. Living up to expectations: estimating direct and indirect rebound effects for UK households. Energy Econ 2015;52:S100-S116. https://doi.org/10.1016/j.eneco.2015.08.026.
- Husereau D, Drummond M, Petrou S, Carswell C, Moher D, Greenberg D, et al. Consolidated Health Economic Evaluation Reporting Standards (CHEERS) statement. BMJ 2013;346. https://doi.org/10.1136/bmj.f1049.
- Guide to the Methods of Technology Appraisal 2013. London: National Institute for Health and Care Excellence; 2013.
- Roberto CA, Kawachi I. Behavioral Economics and Public Health. Oxford: Oxford University Press; 2015.
- Phillips CJ, Fordham R, Marsh K, Bertranou E, Davies S, Hale J, et al. Exploring the role of economics in prioritization in public health: what do stakeholders think?. Eur J Public Health 2011;21:578-84. https://doi.org/10.1093/eurpub/ckq121.
- Methods for the development of NICE public health guidance. London: National Institute for Health and Care Excellence; 2012.
- Burns P, Coxon J. Boiler on Prescription. Closing Report. Sunderland: Gentoo; 2016.
- Tudor-Edwards R, Bray N. The Warm Homes for Health project: exploring the cost-effectiveness of improving population health through better housing. Lancet 2014;384. https://doi.org/10.1016/S0140-6736(14)62206-6.
- Tudor-Edwards R, Bray N, Burns P, Jones A. Warm Homes for Health: Exploring the Costs and Outcomes of Improving Population Health Through Better Housing. Sunderland: Centre for Health Economics and Medicines Evaluation; 2016.
- Lawson KD, Kearns A, Petticrew M, Fenwick EA. Investing in health: is social housing value for money? A cost–utility analysis. J Epidemiol Community Health 2013;67:829-34. https://doi.org/10.1136/jech-2012-202137.
- Jones KH, Ford DV, Jones C, Dsilva R, Thompson S, Brooks CJ, et al. A case study of the Secure Anonymous Information Linkage (SAIL) Gateway: a privacy-protecting remote access system for health-related research and evaluation. J Biomed Inform 2014;50:196-204. https://doi.org/10.1016/j.jbi.2014.01.003.
- Viggers H, Howden-Chapman P, Ingham T, Chapman R, Pene G, Davies C, et al. Warm homes for older people: aims and methods of a randomised community-based trial for people with COPD. BMC Public Health 2013;13. https://doi.org/10.1186/1471-2458-13-176.
- Osman LM, Ayres JG, Garden C, Reglitz K, Lyon J, Douglas JG. Home warmth and health status of COPD patients. Eur J Public Health 2008;18:399-405. https://doi.org/10.1093/eurpub/ckn015.
- Information for the Supply Chain on Green Deal Measures. London: Department of Energy and Climate Change; 2013.
- Burrell D, Heath S. Arbed Phase 1 – Post Installation Review. Cardiff: Welsh Government; 2011.
- Curtis L, Burns A. Unit Costs of Health and Social Care 2015. Canterbury: Personal Social Services Research Unit; 2015.
- Seemungal TA, Donaldson GC, Bhowmik A, Jeffries DJ, Wedzicha JA. Time course and recovery of exacerbations in patients with chronic obstructive pulmonary disease. Am J Respir Crit Care Med 2000;161:1608-13. https://doi.org/10.1164/ajrccm.161.5.9908022.
- Moayeri F, Hsueh Y-S, Clarke P, Dunt D. Do model-based studies in chronic obstructive pulmonary disease measure correct values of utility? A meta-analysis. Value Heal 2016;19:363-73. https://doi.org/10.1016/j.jval.2016.01.012.
- Global Strategy for the Diagnosis, Management, and Prevention of Chronic Obstructive Pulmonary Disease: GOLD Executive Summary. Bethesda, MD: Global Initiative for Chronic Obstructive Lung Disease; 2016.
- Haughney J, Gruffydd-Jones K, Roberts J, Lee AJ, Hardwell A, McGarvey L. The distribution of COPD in UK general practice using the new GOLD classification. Eur Respir J 2014;43:993-1002. https://doi.org/10.1183/09031936.00065013.
- Esteban C, Quintana JM, Garcia-Gutierrez S, Anton-Ladislao A, Gonzales N, Baré M, et al. Determinants of change in physical activity during moderate-to-severe COPD exacerbation. Int J COPD 2016;11:251-61. https://doi.org/10.2147/COPD.S79580.
- Menn P, Weber N, Holle R. Health-related quality of life in patients with severe COPD hospitalized for exacerbations – comparing EQ-5D, SF-12 and SGRQ. Health Qual Life Outcomes 2010;8. https://doi.org/10.1186/1477–7525–8-39.
- O’Reilly JF, Williams AE, Rice L. Health status impairment and costs associated with COPD exacerbation managed in hospital. Int J Clin Pract 2007;61:1112-20. https://doi.org/10.1111/j.1742-1241.2007.01424.x.
- Esteban C, Arostegui I, Garcia-Gutierrez S, Gonzalez N, Lafuente I, Bare M, et al. A decision tree to assess short-term mortality after an emergency department visit for an exacerbation of COPD: a cohort study. Respir Res 2015;16. https://doi.org/10.1186/s12931-015-0313-4.
- Rutten-van Mölken MPMH, Hoogendoorn M, Lamers LM. Holistic preferences for 1-year health profiles describing fluctuations in health: the case of chronic obstructive pulmonary disease. PharmacoEconomics 2009;27:465-77. https://doi.org/10.2165/00019053-200927060-00003.
- Weinstein MC, Stason WB. Foundations of cost-effectiveness analysis for health and medical practices. N Engl J Med 1977;296:716-21. https://doi.org/10.1056/NEJM197703312961304.
- Burge S, Wedzicha JA. COPD exacerbations: definitions and classifications. Eur Respir J Suppl 2003;41:46s-53s. https://doi.org/10.1183/09031936.03.00078002.
- Grey C, Schmieder-Gaite T, Jiang S, Nascimento C, Poortinga W. Cold homes, fuel poverty and energy-efficiency improvements: a longitudinal focus group approach. Indoor Built Environ 2017;26:902-13. https://doi.org/10.1177/1420326X17703450.
- Morrison-Rees S. The Fuel Poverty Data Linking Project. Emerging Findings Report. Cardiff: Welsh Government, Knowledge and Analytical Services; 2016.
- Gavankar S, Geyer R. The Rebound Effect: State of the Debate and Implications for Energy Efficiency Research. Santa Barbara, CA: Bren School of Environmental Science and Management; 2010.
- Johnson RB, Onwuegbuzie AJ, Turner LA. Toward a definition of mixed methods research. J Mix Methods Res 2007;1:112-33. https://doi.org/10.1177/1558689806298224.
- Oakley A, Strange V, Bonell C, Allen E, Stephenson J. The RIPPLE Study Team . Health services research – process evaluation in randomised controlled trials of complex interventions. BMJ 2006;332:413-16. https://doi.org/10.1136/bmj.332.7538.413.
- Guerra Santin O, Itard L, Visscher H. The effect of occupancy and building characteristics on energy use for space and water heating in Dutch residential stock. Energy Build 2009;41:1223-32. https://doi.org/10.1016/j.enbuild.2009.07.002.
- Yohanis YG, Mondol JD, Wright A, Norton B. Real-life energy use in the UK: how occupancy and dwelling characteristics affect domestic electricity use. Energy Build 2008;40:1053-9. https://doi.org/10.1016/j.enbuild.2007.09.001.
- Allen T. Housing renewal, doesn’t it make you sick?. Hous Stud 2000;15:443-61. https://doi.org/10.1080/02673030050009276.
- Bo M, Fonte G, Pivaro F, Bonetto M, Comi C, Giorgis V, et al. Prevalence of and factors associated with prolonged length of stay in older hospitalized medical patients. Geriatr Gerontol Int 2016;16:314-21. https://doi.org/10.1111/ggi.12471.
- Wu HY, Sahadevan S, Ding YY. Factors associated with functional decline of hospitalised older persons following discharge from an acute geriatric unit. Ann Acad Med Singapore 2006;35:17-23.
- Cornwell J, Levenson R, Sonola L, Poteliakhoff E. Continuity of Care for Older Hospital Patients: A Call for Action. London: The King’s Fund; 2012.
- Petrillo J, Van Nooten F, Jones P, Rutten-van Mölken M. Utility estimation in chronic obstructive pulmonary disease: a preference for change?. PharmacoEconomics 2011;29:917-32. https://doi.org/10.2165/11589280-000000000-00000.
- Pickard AS, Yang Y, Lee TA. Comparison of health-related quality of life measures in chronic obstructive pulmonary disease. Health Qual Life Outcomes 2011;9. https://doi.org/10.1186/1477–7525–9-26.
- Boland MRS, Van Boven JFM, Kruis AL, Chavannes NH, Van der Molen T, Goossens LMA, et al. Investigating the association between medication adherence and health-related quality of life in COPD: methodological challenges when using a proxy measure of adherence. Respir Med 2016;110:34-45. https://doi.org/10.1016/j.rmed.2015.11.008.
- Kruis AL, Boland MR, Schoonvelde CH, Assendelft WJ, Mölken MP, Gussekloo J, et al. RECODE: design and baseline results of a cluster randomized trial on cost-effectiveness of integrated COPD management in primary care. BMC Pulm Med 2013;13. https://doi.org/10.1186/1471-2466-13-17.
- Kruis AL, Boland MR, Assendelft WJ, Gussekloo J, Tsiachristas A, Stijnen T, et al. Effectiveness of integrated disease management for primary care chronic obstructive pulmonary disease patients: results of cluster randomised trial. BMJ 2014;349. https://doi.org/10.1136/bmj.g5392.
- Burns DK, Wilson ECF, Browne P, Olive S, Clark A, Galey P, et al. The cost effectiveness of maintenance schedules following pulmonary rehabilitation in patients with chronic obstructive pulmonary disease: an economic evaluation alongside a randomised controlled trial. Appl Health Econ Health Policy 2016;14:105-15. https://doi.org/10.1007/s40258-015-0199-9.
- Wilson AM, Browne P, Olive S, Clark A, Galey P, Dix E, et al. The effects of maintenance schedules following pulmonary rehabilitation in patients with chronic obstructive pulmonary disease: a randomised controlled trial. BMJ Open 2015;5. https://doi.org/10.1136/bmjopen-2014-005921.
- Cramm JM, Nieboer AP. Self-management abilities and quality of life among frail community-dwelling individuals: the role of community nurses in the Netherlands. Heal Soc Care Community 2017;25:394-401. https://doi.org/10.1111/hsc.12318.
- Cramm JM, Nieboer AP. The effects of social and physical functioning and self-management abilities on well-being among patients with cardiovascular diseases, chronic obstructive pulmonary disease, and diabetes. Appl Res Qual Life 2014;9:113-21. https://doi.org/10.1007/s11482-013-9216-z.
- Nieboer AP, Lötters F, Cramm JM. De wijkverpleegkundige als Zichtbare schakel in de wijk in Rotterdam. Rotterdam: Erasmus Universiteit Rotterdam; 2013.
- Ding B, DiBonaventura M, Karlsson N, Ling X. Asthma-chronic obstructive pulmonary disease overlap syndrome in the urban Chinese population: prevalence and disease burden using the 2010, 2012, and 2013 China National Health and Wellness Surveys. Int J Chron Obstruct Pulmon Dis 2016;11:1139-50.
- Langley PC, Mu R, Wu M, Dong P, Tang B. The impact of rheumatoid arthritis on the burden of disease in urban China. J Med Econ 2011;14:709-19. https://doi.org/10.3111/13696998.2011.611201.
- Dritsaki M, Johnson-Warrington V, Mitchell K, Singh S, Rees K. An economic evaluation of a self-management programme of activity, coping and education for patients with chronic obstructive pulmonary disease. Chron Respir Dis 2016;13:48-56. https://doi.org/10.1177/1479972315619578.
- Mitchell KE, Johnson-Warrington V, Apps LD, Bankart J, Sewell L, Williams JE, et al. A self-management programme for COPD: a randomised controlled trial. Eur Respir J 2014;44:1538-47. https://doi.org/10.1183/09031936.00047814.
- Apps LD, Mitchell KE, Harrison SL, Sewell L, Williams JE, Young HM, et al. The development and pilot testing of the self-management programme of activity, coping and education for chronic obstructive pulmonary disease (SPACE for COPD). Int J Chron Obstruct Pulmon Dis 2013;8:317-27. https://doi.org/10.2147/COPD.S40414.
- Eklund O, Afzal F, Borgström F, Flavin J, Ternouth A, Ojanguren ME, et al. Cost-effectiveness of tiotropium versus glycopyrronium in moderate to very severe chronic obstructive pulmonary disease in Canada, Spain, Sweden, and the UK. Clin Outcomes Res 2016;8:243-52. https://doi.org/10.2147/CEOR.S105579.
- Wedzicha JA, Decramer M, Ficker JH, Niewoehner DE, Sandström T, Taylor AF, et al. Analysis of chronic obstructive pulmonary disease exacerbations with the dual bronchodilator QVA149 compared with glycopyrronium and tiotropium (SPARK): a randomised, double-blind, parallel-group study. Lancet Respir Med 2013;1:199-20. https://doi.org/10.1016/S2213-2600(13)70052-3.
- Tashkin DP, Celli B, Senn S, Burkhart D, Kesten S, Menjoge S, et al. A 4-year trial of tiotropium in chronic obstructive pulmonary disease. N Engl J Med 2008;359:1543-54. https://doi.org/10.1056/NEJMoa0805800.
- Quintana JM, Esteban C, Barrio I, Garci-Gutierrez S, Gonzalez N, Arostegui I, et al. The IRYSS-COPD appropriateness study: objectives, methodology, and description of the prospective cohort. BMC Health Serv Res 2011;11. https://doi.org/10.1186/1472-6963-11-322.
- Hoyle CK, Tabberer M, Brooks J. Mapping the COPD Assessment Test onto EQ-5D. Value Health 2016;19:469-77. https://doi.org/10.1016/j.jval.2016.01.005.
- Decramer M, Anzueto A, Kerwin E, Kaelin T, Richard N, Crater G, et al. Efficacy and safety of umeclidinium plus vilanterol versus tiotropium, vilanterol, or umeclidinium monotherapies over 24 weeks in patients with chronic obstructive pulmonary disease: results from two multicentre, blinded, randomised controlled trials. Lancet Respir Med 2014;2:472-86. https://doi.org/10.1016/S2213-2600(14)70065-7.
- Jia H, Lubetkin EI. Impact of nine chronic conditions for US adults aged 65 years and older: an application of a hybrid estimator of quality-adjusted life years throughout remainder of lifetime. Qual Life Res 2016;25:1921-9. https://doi.org/10.1007/s11136-016-1226-5.
- Korea Centers for Disease Control and Prevention . KCDC Leads the World Free of Diseases n.d. www.cdc.go.kr/CDC/eng/main.jsp (accessed 22 February 2018).
- National Center for Health Statistics (NCHS) . National Health and Nutrition Examination Survey Data 2005–2006 n.d. www.cdc.gov/nchs/nhanes/search/nhanes05_06.aspx (accessed 20 July 2015).
- Centers for Disease Control and Prevention (CDC). National Center for Health Statistics (NCHS) . NCHS Data Linked to Mortality Files 2011. www.cdc.gov/nchs/data_access/data_linkage/mortality.htm (accessed 20 July 2015).
- Kwon HY, Kim E. Factors contributing to quality of life in COPD patients in South Korea. Int J Chron Obstruct Pulmon Dis 2016;11:103-9. https://doi.org/10.2147/COPD.S90566.
- The Fourth Korea National Health and Nutrition Examination Survey (KNHANES IV) 2007–2009. Seoul: Ministry of Health and Welfare and Korea Centers for Disease Control and Prevention; 2007.
- The Fifth Korea National Health and Nutrition Examination Survey (KNHANES V) 2010–2012. Seoul: Ministry of Health and Welfare and Korea Centers for Disease Control and Prevention; 2010.
- Lee H, Rhee CK, Lee BJ, Choi DC, Kim JA, Kim SH, et al. Impacts of coexisting bronchial asthma on severe exacerbations in mild-to-moderate COPD: results from a national database. Int J Chron Obstruct Pulmon Dis 2016;11:775-83. https://doi.org/10.2147/COPD.S95954.
- Lee SH, Hwang ED, Lim JE, Moon S, Kang YA, Jung JY, et al. The risk factors and characteristics of COPD among nonsmokers in Korea: an analysis of KNHANES IV and V. Lung 2016;194:353-61. https://doi.org/10.1007/s00408-016-9871-6.
- Martinez Rivera C, Costan Galicia J, Alcázar Navarrete B, Garcia-Polo C, Ruiz Iturriaga LA, Herrejón A, et al. Factors associated with depression in COPD: a multicenter study. Lung 2016;194:335-43. https://doi.org/10.1007/s00408-016-9862-7.
- Alcázar B, García-Polo C, Herrejón A, Ruiz LA, de Miguel J, Ros JA, et al. Factors associated with hospital admission for exacerbation of chronic obstructive pulmonary disease. Arch Bronconeumol 2012;48:70-6. https://doi.org/10.1016/j.arbres.2011.10.009.
- García-Polo C, Alcázar-Navarrete B, Ruiz-Iturriaga LA, Herrejón A, Ros-Lucas JA, García-Sidro P, et al. Factors associated with high resource utilisation among COPD patients. Respir Med 2012;106:1734-42. https://doi.org/10.1016/j.rmed.2012.09.009.
- Roncero C, Campuzano AI, Quintano JA, Molina J, Pérez J, Miravitlles M. Cognitive status among patients with chronic obstructive pulmonary disease. Int J Chron Obstruct Pulmon Dis 2016;11. https://doi.org/10.2147/COPD.S100850.
- Miravitlles M, Molina J, Quintano JA, Campuzano A, Perez J, Roncero C. Factors associated with depression and severe depression in patients with COPD. Respir Med 2014;108:1615-25. https://doi.org/10.1016/j.rmed.2014.08.010.
- Selya-Hammer C, Gonzalez-Rojas Guix N, Baldwin M, Ternouth A, Miravitlles M, Rutten-van Mölken M, et al. Development of an enhanced health-economic model and cost-effectiveness analysis of tiotropium + olodaterol Respimat® fixed-dose combination for chronic obstructive pulmonary disease patients in Italy. Ther Adv Respir Dis 2016;10:391-40. https://doi.org/10.1177/1753465816657272.
- Rutten-van Mölken MP, Oostenbrink JB, Miravitlles M, Monz BU. Modelling the 5-year cost effectiveness of tiotropium, salmeterol and ipratropium for the treatment of chronic obstructive pulmonary disease in Spain. Eur J Health Econ 2007;8:123-35. https://doi.org/10.1007/s10198-007-0039-4.
- Wacker ME, Jörres RA, Karch A, Koch A, Heinrich J, Karrasch S, et al. Relative impact of COPD and comorbidities on generic health-related quality of life: a pooled analysis of the COSYCONET patient cohort and control subjects from the KORA and SHIP studies. Respir Res 2016;17. https://doi.org/10.1186/s12931-016-0401-0.
- Rutten-van Mölken M, Oostenbrink JB, Tashkin DP, Burkhart D, Monz BU. Does quality of life of COPD patients as measured by the generic EuroQol five-dimension questionnaire differentiate between COPD severity stages?. Chest 2006;130:1117-28. https://doi.org/10.1378/chest.130.4.1117.
- Zanaboni P, Hoaas H, Aarøen Lien L, Hjalmarsen A, Wooton R. Long-term exercise maintenance in COPD via telerehabilitation: a two-year pilot study. J Telemed Telecare 2016.
- Boland MRS, Van Boven JFM, Kocks JWH, Van der Molen T, Goossens LM, Chavannes NH, et al. Mapping the clinical chronic obstructive pulmonary disease questionnaire onto generic preference-based EQ-5D values. Value Heal 2015;18:299-307. https://doi.org/10.1016/j.jval.2014.11.006.
- Boland MR, Kruis AL, Tsiachristas A, Assendelft WJ, Gussekloo J, Blom CM, et al. Cost-effectiveness of integrated COPD care: the RECODE cluster randomised trial. BMJ Open 2015;5. https://doi.org/10.1136/bmjopen-2014-007284.
- Utens CM, Goossens LM, Smeenk FW, van Schayck OC, van Litsenburg W, Janssen A, et al. Effectiveness and cost-effectiveness of early assisted discharge for chronic obstructive pulmonary disease exacerbations: the design of a randomised controlled trial. BMC Public Health 2010;10. https://doi.org/10.1186/1471-2458-10-618.
- Kocks J, de Jong C, Berger MY, Kerstjens HA, van der Molen T. Putting health status guided COPD management to the test: protocol of the MARCH study. BMC Pulm Med 2013;13. https://doi.org/10.1186/1471-2466-13-41.
- Fishwick D, Lewis L, Darby A, Young C, Wiggans R, Waterhouse J, et al. Determinants of health-related quality of life among residents with and without COPD in a historically industrialised area. Int Arch Occup Environ Health 2015;88:799-805. https://doi.org/10.1007/s00420-014-1008-8.
- Darby A, Waterhouse J, Stevens V, Billings CG, Billings CG, Burton CM, et al. Chronic obstructive pulmonary disease among residents of a historically industrialised area. Thorax 2012;67:901-7. https://doi.org/10.1136/thoraxjnl-2011-200543.
- Hong JY, Kim SY, Chung KS, Kim EY, Jung JY, Park MS, et al. Factors associated with the quality of life of Korean COPD patients as measured by the EQ-5D. Qual Life Res 2015;24:2549-58. https://doi.org/10.1007/s11136-015-0979-6.
- Kim J, Kim K. Gender differences in health-related quality of life of Korean patients with chronic obstructive lung disease. Public Health Nurs 2015;32:191-200. https://doi.org/10.1111/phn.12129.
- Miravitlles M, Barrecheguren M, Romain-Rodriguez M. Frequency and characteristics of different clinical phenotypes of chronic obstructive pulmonary disease. Int J Tuberc Lung Dis 2015;19:992-8. https://doi.org/10.5588/ijtld.15.0021.
- Miravitlles M, Huerta A, Valle M, García-Sidro P, Forné C, Crespo C, et al. Clinical variables impacting on the estimation of utilities in chronic obstructive pulmonary disease. Int J Chron Obstruct Pulmon Dis 2015;10:367-77. https://doi.org/10.2147/COPD.S76397.
- Stoddart A, van der Pol M, Pinnock H, Hanley J, McCloughan L, Todd A, et al. Telemonitoring for chronic obstructive pulmonary disease: a cost and cost-utility analysis of a randomised controlled trial. J Telemed Telecare 2015;21:108-18. https://doi.org/10.1177/1357633X14566574.
- Pinnock H, Hanley J, Lewis S, MacNee W, Pagliari C, van der Pol M, et al. The impact of a telemetric chronic obstructive pulmonary disease monitoring service: randomised controlled trial with economic evaluation and nested qualitative study. Prim Care Respir J 2009;18:233-5. https://doi.org/10.4104/pcrj.2009.00040.
- Pinnock H, Hanley J, McCloughan L, Todd A, Krishan A, Lewis S, et al. Effectiveness of telemonitoring integrated into existing clinical services on hospital admission for exacerbation of chronic obstructive pulmonary disease: researcher blind, multicentre, randomised controlled trial. BMJ 2013;347. https://doi.org/10.1136/bmj.f6070.
- Sundh J, Johansson G, Larsson K, Lindén A, Löfdahl CG, Janson C, et al. Comorbidity and health-related quality of life in patients with severe chronic obstructive pulmonary disease attending Swedish secondary care units. Int J Chron Obstruct Pulmon Dis 2015;10:173-83. https://doi.org/10.2147/COPD.S74645.
- Wu M, Zhao Q, Chen Y, Fu C, Xu B. Quality of life and its association with direct medical costs for COPD in urban China. Health Qual Life Outcomes 2015;13. https://doi.org/10.1186/s12955-015-0241-5.
- Abdin E, Subramaniam M, Vaingankar JA, Luo N, Chong SA. Population norms for the EQ-5D index scores using Singapore preference weights. Qual Life Res 2015;24:1545-53. https://doi.org/10.1007/s11136-014-0859-5.
- Subramaniam M, Vaingankar J, Heng D, Kwok KW, Lim YW, Yap M, et al. The Singapore Mental Health Study: an overview of the methodology. Int J Methods Psychiatr Res 2012;21:149-57. https://doi.org/10.1002/mpr.1351.
- van Boven JF, Tommelein E, Boussery K, Mehuys E, Vegter S, Brussele GGO, et al. Improving inhaler adherence in patients with chronic obstructive pulmonary disease: a cost-effectiveness analysis. Respir Res 2014;15. https://doi.org/10.1186/1465-9921-15-66.
- Tommelein E, Mehuys E, Van Hees T, Adriaens E, Van Bortel L, Christiaens T, et al. Effectiveness of pharmaceutical care for patients with chronic obstructive pulmonary disease (PHARMACOP): a randomized controlled trial. Br J Clin Pharmacol 2014;77:756-66. https://doi.org/10.1111/bcp.12242.
- Chen J, Wong CK, McGhee SM, Pang PK, Yu WC. A comparison between the EQ-5D and the SF-6D in patients with chronic obstructive pulmonary disease (COPD). PLOS ONE 2014;9. https://doi.org/10.1371/journal.pone.0112389.
- García-Rio F, Soriano JB, Miravitlles M, Muñoz L, Duran-Tauleria E, Sánchez G, et al. Impact of obesity on the clinical profile of a population-based sample with chronic obstructive pulmonary disease. PLOS ONE 2014;9. https://doi.org/10.1371/journal.pone.0105220.
- Ancochea J, Badiola C, Duran-Taulería E, García Río F, Miravitlles M, Muñoz L, et al. Estudio EPI-SCAN: resumen del protocolo de un estudio para estimar la prevalencia de EPOC en personas de 40 a 80 años de edad en España. Arch Bronconeumol 2009;45:41-7. https://doi.org/10.1016/j.arbres.2008.06.001.
- Miravitlles M, Soriano JB, García Río F, Muñoz L, Duran-Taulería E, Sanchez G, et al. Prevalence of COPD in Spain: impact of undiagnosed COPD on quality of life and daily life activities. Thorax 2009;64:863-8. https://doi.org/10.1136/thx.2009.115725.
- Karabis A, Mocarski M, Eijgelshoven I, Bergman G. Economic evaluation of aclidinium bromide in the management of moderate to severe COPD: an analysis over 5 years. Clin Outcomes Res 2014;6:175-85. https://doi.org/10.2147/CEOR.S57904.
- Kim SH, Oh YM, Jo MW. Health-related quality of life in chronic obstructive pulmonary disease patients in Korea. Health Qual Life Outcomes 2014;12. https://doi.org/10.1186/1477-7525-12-57.
- Lin F-J, Pickard AS, Krishnan JA, . Measuring health-related quality of life in chronic obstructive pulmonary disease: properties of the EQ-5D-5L and PROMIS-43 short form. BMC Med Res Methodol 2014;14. https://doi.org/10.1186/1471-2288-14-78.
- COPD Outcomes-based Network for Clinical Effectiveness and Research Translation . CONCERT 2010. www.kpchr.org/concert/public/index.aspx?pageid=1 (accessed 16 March 2017).
- Miravitlles M, Huerta A, Fernández-Villar JA, Alcázar B, Villa G, Forné C, et al. Generic utilities in chronic obstructive pulmonary disease patients stratified according to different staging systems. Health Qual Life Outcomes 2014;12. https://doi.org/10.1186/s12955-014-0120-5.
- Miravitlles M, Cantoni J, Naberan K. Factors associated with a low level of physical activity in patients with chronic obstructive pulmonary disease. Lung 2014;192:259-65. https://doi.org/10.1007/s00408-014-9557-x.
- Naberan K, Azpeitia A, Cantoni J, Miravitlles M. Impairment of quality of life in women with chronic obstructive pulmonary disease. Respir Med 2012;106:367-73. https://doi.org/10.1016/j.rmed.2011.09.014.
- Mullen K, Coyle D, Manuel D, Nguyen HV, Pham B, Pipe AL, et al. Economic evaluation of a hospital-initiated intervention for smokers with chronic disease, in Ontario, Canada. Tob Control 2015;24:489-96.
- Reid RD, Mullen KA, Slovinec D’Angelo ME, Aitken DA, Papadakis S, Haley PM, et al. Smoking cessation for hospitalized smokers: an evaluation of the ‘Ottawa Model’. Nicotine Tob Res 2010;12:11-8. https://doi.org/10.1093/ntr/ntp165.
- Peters M, Crocker H, Jenkinson C, Doll H, Fitzpatrick R. The routine collection of patient-reported outcome measures (PROMs) for long-term conditions in primary care: a cohort survey. BMJ Open 2014;4. https://doi.org/10.1136/bmjopen-2013-003968.
- Peters M, Crocker H, Dummett S, Jenkinson C, Doll H, Fitzpatrick R. Change in health status in long-term conditions over a one year period: a cohort survey using patient-reported outcome measures. Health Qual Life Outcomes 2014;12. https://doi.org/10.1186/s12955-014-0123-2.
- Samyshkin Y, Kotchie RW, Mörk AC, Briggs AH, Bateman ED. Cost-effectiveness of roflumilast as an add-on treatment to long-acting bronchodilators in the treatment of COPD associated with chronic bronchitis in the United Kingdom. Eur J Health Econ 2014;15:69-82. https://doi.org/10.1007/s10198-013-0456-5.
- Rabe KF. Update on roflumilast, a phosphodiesterase 4 inhibitor for the treatment of chronic obstructive pulmonary disease. Br J Pharmacol 2011;163:53-67. https://doi.org/10.1111/j.1476-5381.2011.01218.x.
- Tsiachristas A, Cramm JM, Nieboer AP, Rutten-van Mölken MP. Changes in costs and effects after the implementation of disease management programs in the Netherlands: variability and determinants. Cost Eff Resour Alloc 2014;12. https://doi.org/10.1186/1478-7547-12-17.
- Lemmens KM, Rutten-van Mölken MP, Cramm JM, Huijsman R, Bal RA, Nieboer AP. Evaluation of a large scale implementation of disease management programmes in various Dutch regions: a study protocol. BMC Health Serv Res 2011;11. https://doi.org/10.1186/1472-6963-11-6.
- Gillespie P, O’Shea E, Casey D, Murphy K, Devane D, Cooney A, et al. The cost-effectiveness of a structured education pulmonary rehabilitation programme for chronic obstructive pulmonary disease in primary care: the PRINCE cluster randomised trial. BMJ Open 2013;3. https://doi.org/10.1136/bmjopen-2013-003479.
- Murphy K, Casey D, Devane D, Cooney A, McCarthy B, Mee L, et al. A cluster randomised controlled trial evaluating the effectiveness of a structured pulmonary rehabilitation education programme for improving the health status of people with chronic obstructive pulmonary disease: the PRINCE Study protocol. BMC Pulm Med 2011;11. https://doi.org/10.1186/1471-2466-11-4.
- Samyshkin Y, Schlunegger M, Haefliger S, Ledderhose S, Radford M. Cost-effectiveness of roflumilast in combination with bronchodilator therapies in patients with severe and very severe COPD in Switzerland. Int J Chron Obstruct Pulmon Dis 2013;8:79-87. https://doi.org/10.2147/COPD.S37486.
- Solem CT, Sun SX, Sudharshan L, Macahilig C, Katyal M, Gao X. Exacerbation-related impairment of quality of life and work productivity in severe and very severe chronic obstructive pulmonary disease. Int J Chron Obstruct Pulmon Dis 2013;8:641-52. https://doi.org/10.2147/COPD.S51245.
- Asukai Y, Baldwin M, Mungapen L. P84 utility values for COPD patients based on the EQ-5D questionnaire from three indacaterol Phase III studies. Thorax 2012;67:A100-A101. https://doi.org/10.1136/thoraxjnl-2012-202678.326.
- Dahl R, Chung KF, Buhl R, Magnussen H, Nonikov V, Jack D, et al. Efficacy of a new once-daily long-acting inhaled beta2-agonist indacaterol versus twice-daily formoterol in COPD. Thorax 2010;65:473-9. https://doi.org/10.1136/thx.2009.125435.
- Barnes PJ, Pocock SJ, Magnussen H, Iqbal A, Kramer B, Higgins M, et al. Integrating indacaterol dose selection in a clinical study in COPD using an adaptive seamless design. Pulm Pharmacol Ther 2010;23:165-71. https://doi.org/10.1016/j.pupt.2010.01.003.
- Kornmann O, Dahl R, Centanni S, Dogra A, Owen R, Lassen C, et al. Once-daily indacaterol versus twice-daily salmeterol for COPD: a placebo-controlled comparison. Eur Respir J 2011;37:273-9. https://doi.org/10.1183/09031936.00045810.
- DiBonaventura M, Paulose-Ram R, Su J, McDonald M, Zou KH, Wagner JS, et al. The burden of chronic obstructive pulmonary disease among employed adults. Int J Chron Obstruct Pulmon Dis 2012;7. https://doi.org/10.2147/COPD.S29280.
- Centers for Disease Control and Prevention National Center for Health Statistics . National Health and Nutrition Examination Survey 2017. www.cdc.gov/nchs/nhanes/index.htm (accessed 20 July 2015).
- Goodacre SW, Wilson RW, Bradburn M, Santarelli M, Nicholl JP. Health utility after emergency medical admission: a cross-sectional survey. Health Qual Life Outcomes 2012;10. https://doi.org/10.1186/1477-7525-10-20.
- Taylor SJC, Sohanpal R, Bremner SA, Devine A, McDaid D, Fernández J-L, et al. Self-management support for moderate-to-severe chronic obstructive pulmonary disease: a pilot randomised controlled trial. Br J Gen Pract 2012;62:687-95. https://doi.org/10.3399/bjgp12X656829.
- Fletcher MJ, Upton J, Taylor-Fishwick J, Buist SA, Jenkins C, Hutton J, et al. COPD uncovered: an international survey on the impact of chronic obstructive pulmonary disease [COPD] on a working age population. BMC Public Health 2011;11. https://doi.org/10.1186/1471-2458-11-612.
- Goossens LM, Nivens MC, Sachs P, Monz BU, Rutten-van Mölken MP. Is the EQ-5D responsive to recovery from a moderate COPD exacerbation?. Respir Med 2011;105:1195-202. https://doi.org/10.1016/j.rmed.2011.02.018.
- Monz BU, Sachs P, McDonald J, Crawford B, Nivens MC, Tetzlaff K. Responsiveness of the cough and sputum assessment questionnaire in exacerbations of COPD and chronic bronchitis. Respir Med 2010;104.
- Janssen DJ, Franssen FM, Wouters EF, Schols JM, Spruit MA. Impaired health status and care dependency in patients with advanced COPD or chronic heart failure. Qual Life Res 2011;20:1679-88. https://doi.org/10.1007/s11136-011-9892-9.
- Janssen DJ, Wouters EF, Schols JM, Spruit MA. Self-perceived symptoms and care needs of patients with severe to very severe chronic obstructive pulmonary disease, congestive heart failure or chronic renal failure and its consequences for their closest relatives: the research protocol. BMC Palliat Care 2008;7. https://doi.org/10.1186/1472-684X-7-5.
- Janssen DJ, Spruit MA, Uszko-Lencer NH, Schols JM, Wouters EF. Symptoms, co-morbidities and healthcare in advanced COPD or chronic heart failure. J Palliat Med 2011;14:735-43. https://doi.org/10.1089/jpm.2010.0479.
- Janssen DJ, Spruit MA, Schols JM, Wouters EF. A call for high-quality advance care planning in outpatients with severe COPD or chronic heart failure. Chest 2011;139:1081-8. https://doi.org/10.1378/chest.10-1753.
- Khdour MR, Agus AM, Kidney JC, Smyth BM, McElnay JC, Elnay JC, et al. Cost-utility analysis of a pharmacy-led self-management programme for patients with COPD. Int J Clin Pharm 2011;33:665-73. https://doi.org/10.1007/s11096-011-9524-z.
- Khdour MR, Kidney JC, Smyth BM, McElnay JC. Clinical pharmacy-led disease and medicine management programme for patients with COPD. Br J Clin Pharmacol 2009;68:588-98. https://doi.org/10.1111/j.1365-2125.2009.03493.x.
- Miravitlles M, Naberan K, Cantoni J, Azpeitia A. Socioeconomic status and health-related quality of life of patients with chronic obstructive pulmonary disease. Respiration 2011;82:402-8. https://doi.org/10.1159/000328766.
- Joo MJ, Lee TA, Bartle B, van de Graaff WB, Weiss KB. Patterns of healthcare utilization by COPD severity: a pilot study. Lung 2008;186:307-12. https://doi.org/10.1007/s00408-008-9095-5.
- Starkie HJ, Briggs AH, Chambers MG, Jones P. Predicting EQ-5D values using the SGRQ. Value Heal 2011;14:354-60. https://doi.org/10.1016/j.jval.2010.09.011.
- Calverley PMA, Anderson JA, Celli B, Ferguson GT, Jenkins C, Jones PW, et al. Salmeterol and fluticasone propionate and survival in chronic obstructive pulmonary disease. N Engl J Med 2007;356:775-89. https://doi.org/10.1056/NEJMoa063070.
- Hoogendoorn M, van Wetering CR, Schols AM, Rutten-van Mölken MP. Is INTERdisciplinary COMmunity-based COPD management (INTERCOM) cost-effective?. Eur Respir J 2010;35:79-87. https://doi.org/10.1183/09031936.00043309.
- Szende A, Leidy NK, Ståhl E, Svensson K. Estimating health utilities in patients with asthma and COPD: evidence on the performance of EQ-5D and SF-6D. Qual Life Res 2009;18:267-72. https://doi.org/10.1007/s11136-008–9429-z.
- Szende A, Svensson K, Stahl E, Meszaros A, Berta GY. Psychometric and utility-based measures of health status of asthmatic patients with different disease control level. PharmacoEconomics 2004;22:537-47. https://doi.org/10.2165/00019053-200422080-00005.
- Stáhl E, Lindberg A, Ronmark E, Svensson K, Jansson S-A, Andersson F, et al. The level of health-related quality of life in patients with COPD and its dependence on age, gender, and disease severity. Eur Respir J 2001;7.
- Punekar YS, Rodriguez-Roisin R, Sculpher M, Jones P, Spencer M. Implications of chronic obstructive pulmonary disease (COPD) on patients’ health status: a western view. Respir Med 2007;101:661-9. https://doi.org/10.1016/j.rmed.2006.06.001.
- Casaburi R, Mahler DA, Jones PW, Wanner A, San PG, ZuWallack RL, et al. A long-term evaluation of once-daily inhaled tiotropium in chronic obstructive pulmonary disease. Eur Respir J 2002;19:217-24. https://doi.org/10.1183/09031936.02.00269802.
- Vincken W, van Noord JA, Greefhorst AP, Bantje TA, Kesten S, Korducki L, et al. Improved health outcomes in patients with COPD during 1 year’s treatment with tiotropium. Eur Respir J 2002;19:209-16. https://doi.org/10.1183/09031936.02.00238702.
- Brusasco V, Hodder R, Miravitlles M, Korducki L, Towse L, Kesten S. Health outcomes following treatment for six months with once daily tiotropium compared with twice daily salmeterol in patients with COPD. Thorax 2003;58:399-404. https://doi.org/10.1136/thorax.58.5.399.
- Oostenbrink JB, Rutten-van Mölken MPMH, Monz BU, FitzGerald JM. Probabilistic Markov model to assess the cost-effectiveness of bronchodilator therapy in COPD patients in different countries. Value Health 2005;8:32-46. https://doi.org/10.1111/j.1524-4733.2005.03086.x.
- Decramer M, Celli B, Tashkin DP, . Clinical trial design considerations in assessing long-term functional impacts of tiotropium in COPD: the UPLIFT trial. COPD 2004;1:303-12. https://doi.org/10.1081/COPD-200026934.
- Spencer M, Briggs AH, Grossman RF, Rance L. Development of an economic model to assess the cost effectiveness of treatment interventions for chronic obstructive pulmonary disease. PharmacoEconomics 2005;23:619-37. https://doi.org/10.2165/00019053-200523060-00008.
- Prescott-Clarke P, Primatesta P. Health Survey for England 1996. London: HMSO; 1998.
- Spencer S, Jones PW. GLOBE Study Group . Time course of recovery of health status following an infective exacerbation of chronic bronchitis. Thorax 2003;58:589-93. https://doi.org/10.1136/thorax.58.7.589.
- Ståhl E, Lindberg A, Jansson SA, Rönmark E, Svensson K, Andersson F, et al. Health-related quality of life is related to COPD disease severity. Health Qual Life Outcomes 2005;3. https://doi.org/10.1186/1477-7525-3-56.
- Lundbäck B, Lindberg A, Lindstrom M, Ronmark E, Jonsson AC, Jonsson E, et al. Not 15 but 50% of smokers develop COPD? Report from the Obstructive Lung Disease in Northern Sweden Studies. Respir Med 2003;97:115-22. https://doi.org/10.1053/rmed.2003.1446.
- Lindstrom M, Jonsson E, Larsson K, Lundback B. Underdiagnosis of chronic obstructive pulmonary disease in Northern Sweden. Int J Tuberc Lung Dis 2002;6:76-84.
- Jansson SA, Andersson F, Borg S, Ericsson A, Jonsson E, Lundback B. Costs of COPD in Sweden according to disease severity. Chest 2002;122:1994-2002. https://doi.org/10.1378/chest.122.6.1994.
- Sullivan PW, Lawrence WF, Ghushchyan V. A national catalog of preference-based scores for chronic conditions in the United States. Med Care 2005;43:736-49. https://doi.org/10.1097/01.mlr.0000172050.67085.4f.
- Agency for Healthcare Research and Quality . Medical Expenditure Panel Survey 2003. www.meps.ahrq.gov/mepsweb/ (accessed 10 November 2003).
- Borg S, Ericsson A, Wedzicha J, Gulsvik A, Lundbäck B, Donaldson GC, et al. A computer simulation model of the natural history and economic impact of chronic obstructive pulmonary disease. Value Heal 2004;7:153-67. https://doi.org/10.1111/j.1524-4733.2004.72318.x.
- Lundbäck B, Nyström L, Rosenhall L, Stjernberg N. Obstructive Lung Disease in Northern Sweden: respiratory symptoms assessed in a postal survey. Eur Respir J 1991;4:257-66.
- Lundbäck B, Rönmark E, Jönsson E. 10 year follow-up of the Obstructive Lung Disease in Northern Sweden study’s (OLIN) first cohort. Am J Respir Crit Care Med 1999;159.
- Brazier J, Roberts J, Tsuchiya A, Busschbach J. A comparison of the EQ-5D and SF-6D across seven patient groups. Health Econ 2004;13:873-84. https://doi.org/10.1002/hec.866.
- Thomas K, MacPherson H, Ratcliffe J, Thorpe L, Brazier J, Campbell M, et al. Longer term clinical and economic benefits of offering acupuncture to patients with chronic low back pain. Health Technol Assess 2005;9. https://doi.org/10.3310/hta9320.
- Kaplan RM, Ries AL, Reilly J, Mohsenifar Z. Measurement of health-related quality of life in the National Emphysema Treatment Trial. Chest 2004;126:781-9. https://doi.org/10.1378/chest.126.3.781.
- The National Emphysema Treatment Trial Research Group . Rationale and design of The National Emphysema Treatment Trial: a prospective randomized trial of lung volume reduction surgery. Chest 1999;116:1750-61. https://doi.org/10.1378/chest.116.6.1750.
- Hazell M, Frank T, Frank P. Health related quality of life in individuals with asthma related symptoms. Respir Med 2003;97:1211-18. https://doi.org/10.1016/S0954-6111(03)00249-X.
- Stavem K, Jodalen H. Reliability and validity of the COOP/WONCA health status measure in patients with chronic obstructive pulmonary disease. Qual Life Res 2002;11:527-33. https://doi.org/10.1023/A:1016345428638.
- Stavem K. Reliability, validity and responsiveness of two multiattribute utility measures in patients with chronic obstructive pulmonary disease. Qual Life Res 1999;8:45-54. https://doi.org/10.1023/A:1026475531996.
- Paterson C, Langan CE, Mckaig GA, Anderson PM, Maclaine GDH, Rose LB, et al. Assessing patient outcomes in acute exacerbations of chronic bronchitis: the Measure Your Medical Outcome Profile (MYMOP), Medical Outcomes Study 6-Item General Health Survey (MOS-6A) and EuroQol (EQ5D). Qual Life Res 2000;9:521-7. https://doi.org/10.1023/A:1008930521566.
- Torrance G, Walker V, Grossman R, Mukherjee J, Vaughan D, La Forge J, et al. Economic evaluation of ciprofloxacin compared with usual antibacterial care for the treatment of acute exacerbations of chronic bronchitis in patients followed for 1 year. PharmacoEconomics 1999;16:499-520. https://doi.org/10.2165/00019053-199916050-00007.
- Harper R, Brazier JE, Waterhouse JC, Walters SJ, Jones NM, Howard P. Comparison of outcome measures for patients with chronic obstructive pulmonary disease (COPD) in an outpatient setting. Thorax 1997;52:879-87. https://doi.org/10.1136/thx.52.10.879.
- Jaeschke R, Guyatt GH, Willan A, Cook D, Harper S, Morris J, et al. Effect of increasing doses of beta agonists on spirometric parameters, exercise capacity, and quality of life in patients with chronic airflow limitation. Thorax 1994;49:479-84. https://doi.org/10.1136/thx.49.5.479.
- Wallentin L, Becker RC, Budaj A, Cannon CP, Emanuelsson H, Held C, et al. Ticagrelor versus clopidogrel in patients with acute coronary syndromes. N Engl J Med 2009;361:1045-57. https://doi.org/10.1056/NEJMoa0904327.
- Pickard AS, Wilke C, Jung E, Patel S, Stavem K, Lee TA. Use of a preference-based measure of health (EQ-5D) in COPD and asthma. Respir Med 2008;102:519-36. https://doi.org/10.1016/j.rmed.2007.11.016.
- Sullivan PW, Ghushchyan V. Preference-based EQ-5D index scores for chronic conditions in the United States. Med Decis Making 2006;26:410-20. https://doi.org/10.1177/0272989X06290495.
- Tillmann M, Silcock J. A comparison of smokers’ and ex-smokers’ health-related quality of life. J Public Health Med 1997;19:268-73. https://doi.org/10.1093/oxfordjournals.pubmed.a024629.
Appendix 1 Resident engagement: focus group protocol
Introduction
Introduction (moderator): thank people for coming, introduce self, briefly outline goals for research, reasons for recording the session given, ‘rules’: only one person to talk at a time, all data are treated confidentially and will be anonymised, the session is open and everyone’s views are important, amount of time that will be taken up. Aim for people to talk to each other, not moderator.
-
Tell me a little bit about how long you have lived in this area, and what are the good and bad things about living here.
-
What are the good and bad things about living in your home?
-
Tell me a little bit about your home – do you have any problems with it (e.g. condensation/mould)?
Before intervention (round 1, 2014)
-
THERMAL COMFORT: I’d like to talk to you about what conditions are like in your home (before any Arbed measures were installed). Would you tell me what a typical winter’s day is like in your home?
Probe differences between household members (especially very young and very old): what was it like getting up in the morning, washing/bathing, doing housework, going to bed, use of rooms/house space, draughty/cold parts of the house, warmth and comfort. What would you like your home to be like? What changes would you make if you had the resources?
-
KEEPING YOUR HOUSE WARM: How adequate is the heating system that you use to warm your house (before Arbed)? How do you use it? What would you change about your home in order for you to be comfortable in winter? What are the factors stopping you from doing this?
Probe: timing, warmth, cost/affordability, convenience, efficiency, ease of use. Have you put up with feeling cold because of cost? Are there any rooms you do not heat?
-
USE OF LIVING SPACE: Does your ability to keep your house warm enough affect your use of different rooms in your house? Does it ever stop you going out or inviting people home during the coldest days in the winter?
-
FUEL POVERTY: Do you feel that paying for heating your home in winter is difficult? What would you spend money on if fuel was less expensive? What is more important, eating well or warmth?
Probe (written on board): rent/mortgage; repairs to home; telephone bill; food and other necessities; going out/treats; credit payments; occasional bills (e.g. insurance); stress; heat or eat.
-
HEALTH AND WELL-BEING: How do you think that living in a home that is not warm enough in winter would affect a person’s feeling of health and well-being? (generally)
How do you think it affects different people: elderly, children, people with pre-existing health problems, fit adults? Probe: effects on mood, state of mind, overall well-being, physical health? Respiratory health: colds/flu?
Alternative: if you are cold in your home what effect has that on your life in general? AND do you think being cold is connected to your health?
-
EXPECTATIONS AND EXPERIENCES OF ARBED UP TO DATE: What did you think the benefits of Arbed might be? Do you think it will have a particularly big effect for any particular family member?
Probe: physical health and mental/well-being issues for self and other family members, use of home (e.g. increase in living space), social interactions, effects of cold home as age, financial benefits
Imagine that this Arbed Scheme did not exist and the government would send you a cheque of the same value for you to spend on absolutely anything you would like. How would you have spent it? Just say the first thing that comes into your mind. Why that? Probe: the money or having the work done: which do you think would benefit you more and why? If you had to spend it on improving your home, what would you spend it on?
End (moderator): thank everyone for their participation, explain what will happen to the data they have given, tell them about further session next year.
After intervention (round 2, 2015)
-
THERMAL COMFORT: I’d like to talk to you about what conditions are like in your home (after any measures were installed). Would you tell me what a typical winter’s day is like in your home now? Are you satisfied with the temperature of your home?
Probe: differences between household members (especially young and old): what was it like getting up in the morning, washing/bathing, doing housework, going to bed, use of rooms/house space, draughty/cold parts of the house, convenience, efficiency, warmth and comfort.
-
KEEPING YOUR HOUSE WARM: How adequate is the heating system that you use to warm your house (after Arbed)? Do you use the heating/hot water any differently now? If yes, how and why?
Probe: timing, warmth, cost/affordability, convenience, efficiency, ease of use. Have you put up with feeling cold because of cost?
-
USE OF LIVING SPACE: After Arbed, do you use/heat your home differently? Are there any rooms you do not heat? Have you changed your habits of going out or inviting people into your home?
-
FUEL POVERTY: What difference has the Arbed work had on your energy bills? Do you find it easier affording to heat your home? Have you changed the way you heat your home? What do you spend any saved money on?
-
HEALTH AND WELL-BEING: After the Arbed work have there been any changes in the way you and your family feel in terms of health or well-being?
In what ways do you think the new heating/hot water has affected your health/illness? Other household member’s health/illness?
Has it affected your/their use of health services (GP visits and callouts; A&E, NHS Direct)? Probe: colds, flu, asthma, other, feelings of well-being? Probe: effects on mood, state of mind, overall well-being, physical health? Respiratory health – colds/flu?
-
EXPECTATIONS AND EXPERIENCES OF ARBED: Now you have had the Arbed work done, would you say it has made a difference? To you? To other members of the household? In what ways?
Probe: the difference made by the most recent work, relative to the effects of any other previous work (damp proofing, cavity wall insulation, loft insulation, double glazing etc.)? What made the biggest difference?
Overall, would you say your experiences with Arbed were positive? Have you had any problems since the work was carried out? If yes, what and how did you deal with it? If no, who would you contact if you did have a problem? Probe: how contacted, choice, control, implementation, core components everyone received.
Can you think of any other benefits from Arbed? Probe: what about in relation to the environment? How important is it to you that your new heating is less harmful to the environment?
Imagine that this Arbed Scheme did not exist and the government would send you a cheque of the same value for you to spend on absolutely anything you would like. How would you have spent it? Just say the first thing that comes into your mind. Why that?
End (moderator): thank everyone for their participation, explain what will happen to the data they have given.
Appendix 2 Resident engagement: summary results of the reconvened focus group study
This appendix contains material from Grey et al. 161 This article is distributed under the terms of the Creative Commons Attribution 3.0 License (http://www.creativecommons.org/licenses/by/3.0/) which permits any use, reproduction and distribution of the work without further permission provided the original work is attributed as specified on the SAGE and Open Access pages (https://us.sagepub.com/en-us/nam/open-access-at-sage).
Introduction and aims
A reconvened focus group study was conducted as part of the wider residents’ engagement with the research project. Three focus groups were held with residents before and again after they received energy efficiency improvements under the second phase of the intervention programme. The aims of the focus groups were to obtain a better understanding of the views and experiences of residents living in energy inefficient (hard-to-heat, hard-to-treat) houses, and then to explore in what way the intervention may have improved these experiences. The qualitative approach used in the study allows the residents to express experiences in their own words and in greater detail than is possible in a quantitative survey. To date, only a small number of qualitative studies have been conducted to examine psychosocial aspects of warmth and energy efficiency improvement. 39,53,61 This reconvened focus group study adds to the evidence of residents’ experiences of living in cold homes, the stresses and consequences of living in fuel poverty, and the ways that energy efficiency improvements may change those experiences.
Methods
The focus groups were conducted in three geographically distinct communities in South Wales that had been selected to receive energy efficiency measures under the programme.
Box 2 provides details of the three communities, as well as specifics of the different schemes. The first round of focus groups was held in March 2014 and lasted just under 1.5 hours. In total, 28 people took part in the study (8 in Caerau, 9 in Brynamman and 11 in Hollybush). Participants were recruited from 30 households whose homes were being monitored as part of the project (see Chapter 4). They were contacted by telephone to invite them to take part in the discussions. Therefore, one of the researchers (SJ) had been in contact with the participants a number of times before the start of the focus groups. The participants were made aware of the goals of the focus groups as well as the overall project. The focus groups were held at a convenient location and took place either at lunchtime or in the early evening. In one of the focus groups, three participants had already received energy efficiency improvements under the intervention programme. Another three participants had recently received external wall insulation, but not through the intervention programme.
Caerau (Cardiff) is a suburb located 3 miles to the west of Cardiff city centre. Housing is a mixture of housing association flats, bricked terraced houses, traditionally built semi-detached houses and semi-detached BISF houses. The work that took place was external wall insulation and boiler/heating system upgrades. Caerau has a WIMD rank of 170 and is part of the Cardiff West Communities First regeneration cluster.
Brynamman (Carmarthenshire) is a village located on the south-facing side of the Black Mountain in an old coal mining area within the Brecon Beacons National Park boundaries. Housing is predominantly small, stone terraces. Before the first focus group was held, the area was not connected to the mains gas network. The mains gas network was extended to homes in the village and boiler and central heating upgrades were provided. The area has a WIMD rank of 651.
Hollybush (Caerphilly) is an old coal mining village situated between Blackwood and Tredegar, above the Sirhowy Valley. Before the first focus group was held, the village was not connected to the mains gas network. Housing is a combination of older small stone terraced houses (pre 1919) and post-1965 and 1980s detached homes. The work extended the mains gas network to homes in the village and provided boiler and central heating upgrades. The area has a WIMD rank of 565 and is located within the Mid Valleys East Communities First regeneration cluster.
The second round of focus groups was held in March and April 2015 after all improvement work was complete in the three communities. The focus groups again lasted just under 1.5 hours. All participants who took part in the first round were invited to attend. In total, 22 people took part in the reconvened focus groups (5 in Caerau, 3 in Brynamman and 14 in Hollybush). This included three additional residents from the Hollybush area who expressed interest in attending the discussions. A number of previous participants were not available for the second round owing to other commitments or relocation.
Out of the 31 residents who participated in the focus group discussions, 14 were male and 17 female; 24 owned their home and 7 rented; 4 were aged between 18 and 40 years, 9 were aged between 40 and 60 years and 18 were aged > 60 years. Eighteen residents occupied the home on their own, 19 occupied the home as a couple and 4 could be considered a family (i.e. a couple plus children).
Topics for the focus group discussions were based on the themes from the household survey used for the community-based study (see Chapter 3). The same themes were discussed in the before and after focus groups. These included the topics of (1) health and well-being; (2) thermal comfort, staying warm and the use of living space; (3) fuel poverty; and (4) experiences with the intervention programme (see Appendix 1 for the focus group protocol).
The discussions were led by two researchers (CG and SJ) and supported by Gabriela Zapata-Lancaster and Martina Stefani (see Acknowledgements). All focus group discussions were recorded and fully transcribed. The resulting discussions were analysed thematically, using the identified themes as organising concepts. Ethics approval was received from the Welsh School of Architecture’s Research Ethics Committee on 15 March 2014 (EC1403.184). Participants were given a £30 voucher as compensation for their time.
Analysis
A structured approach was applied for the analysis. The focus groups were recorded, transcribed and coded using computer-assisted qualitative data analysis software. NVivo 11 (QSR International, Warrington, UK) was used to code the transcripts using the key topics, described above, which were based on identified themes within the context of the existing fuel poverty literature and policies. Transcripts were coded separately by two researchers (CG and Tina Schmieder-Gaite) to ensure consistency. Figure 25 shows the coding tree that was used to analyse the focus group transcripts. After coding, each parent and child node was analysed thematically and emerging patterns were refined and cross-compared. The results are described according to these themes.
FIGURE 25.
Coding tree with coding parent nodes and child nodes.
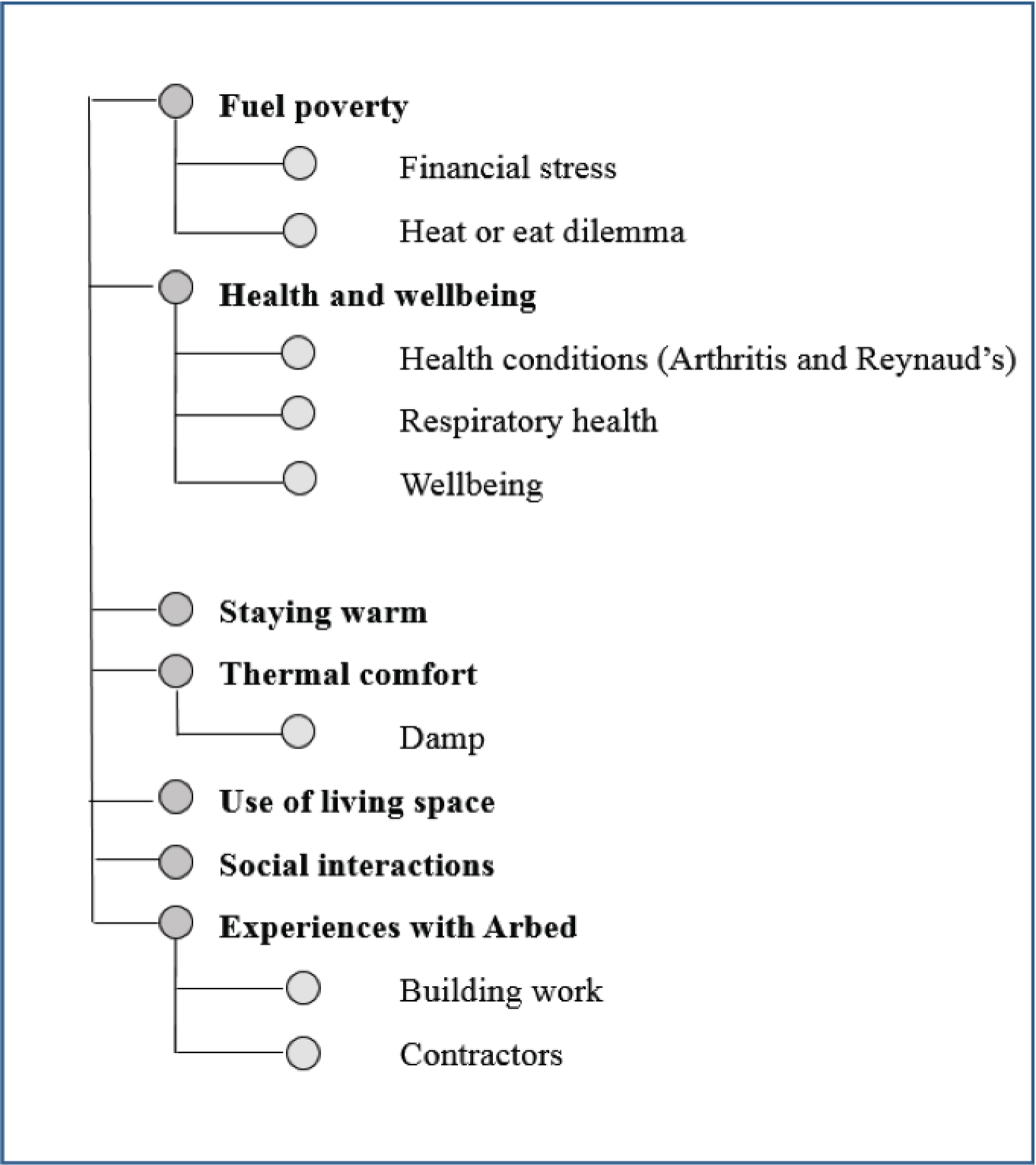
Results
The main findings of the focus groups are presented here under the key themes investigated. The results for the before and after focus groups are discussed separately. A more detailed description of the results can be found in Grey et al. 161
Round 1: before installation (2014)
Health and well-being
There was strong agreement among the focus group participants that living in a cold home is depressing and detrimental to both mental and physical health. In line with the findings of Harrington et al. ,39 participants thought that living in a cold home exacerbates ill health rather than causes it; in particular, that a cold home may make it more difficult to live with or recover from pre-existing chronic conditions. Many described how living in the cold affected their respiratory health and it was felt that the cold environment exacerbated a range of conditions, including diabetes, arthritis and circulatory problems. A cold home was thought to increase both the length and severity of illness. In addition, participants felt that living in a cold home contributes to poor emotional well-being. The physical effects of exposure to poor internal conditions and psychological stress resulting from fuel bills were felt to trigger feelings of depression and anxiety. It was generally believed that a warmer home environment would contribute to better mental and physical well-being.
Thermal comfort, staying warm and use of living space
Participants commented on how unpleasantly cold their homes could be during the winter and expressed concerns about the lack of thermal comfort and the negative consequences this may have for their health. They described how difficult it is to stay warm in an energy inefficient house, in particular if it is non-traditional housing (e.g. a steel frame construction). Respondents used a number of strategies to stay warm and avoid turning on the heating. This included the use of heaters, hot water bottles and blankets, or only heating a limited number of rooms. Similar strategies were reported in Harrington et al. ,39 Gilbertson et al. 61 and Shortt and Rugkåsa. 53 However, these strategies were seen as temporary stopgaps and were considered unsustainable in the longer term. The lack of thermal comfort and living space was thought to put a strain on social interactions within the households and, as a result, participants’ enjoyment of their home. This also meant that some householders tried to avoid being at home for certain periods of time.
Fuel poverty
The focus group participants repeatedly mentioned how expensive it is to heat their homes and discussed how easy it is for low-income households living in an energy inefficient house to fall into fuel poverty. High fuel bills are not only stressful but also force householders to make difficult choices in their daily lives. Participants frequently had to make compromises on how to spend their limited household budget. Just as reported by Harrington et al. ,39 households either economised on fuel or refrained from other activities or expenditures in order to stay warm. Many considered the ‘heat-or-eat’ dilemma a reality in their own lives as well as in their wider community. Some participants reported episodes in which they would not eat because they had to heat their home. The findings support the conclusion of Harrington et al. 39 that the health impacts of fuel poverty involves more than the direct physical effects of exposure to poor internal conditions. The cumulative stresses associated with fuel poverty were found to be particularly damaging. Living in a cold, damp house is more depressing when you are not able to eat properly or go out. An inadequate diet may not only lead to problems associated with food poverty but can also worsen feelings of poor well-being in a cold home. Furthermore, the discussions showed how socially isolating fuel poverty can be. Some of the participants described how friends and family were reluctant to come and visit them in their homes because of the cold, even in summer.
Experiences with the intervention programme
All participants of the focus groups welcomed the energy efficiency measures they were expecting to receive under the intervention programme. The participants who had already received some of the measures felt grateful for them, not only because they were provided for free, but also because they had made a noticeable difference to their comfort, finances and overall quality of life. These results are in line with the findings of previous qualitative studies. Gilbertson et al. 61 reported that recipients of Warm Front energy efficiency work were generally positive about the upgrades and felt that the upgrades had improved thermal comfort, use of living space and feelings of well-being. They also found that greater warmth and comfort further enhanced emotional security and social relations within the home and eased symptoms of chronic illness.
Although the participants of the first round of focus groups were generally positive about the intervention programme and the provided energy efficiency upgrades, they felt that the communication with the recipients could be improved and households themselves should have a greater say in the delivery of the programme. The ‘one-size-fits-all’ approach was generally disliked and many questioned the motives for taking this approach and the usefulness of some of the measures.
Round 2: after installation (2015)
Health and well-being
Improvements to health and well-being were discussed again in the second round of focus groups after all improvement work was complete. The participants generally felt that the improvements had had some effect on their health, in particular their respiratory health. However, participants did not discuss the topic in as much detail as in the first round of focus groups. It appeared that health had become a lower priority, and it proved quite difficult to sustain discussion on the topic. Participants did feel that better thermal comfort and aesthetic aspects of the improvements had had a positive effect on their general well-being. The results of the focus groups suggest that the health benefits of the improvements were, at least in the short term, more closely linked to psychological well-being – resulting from better thermal comfort, having more control over of the heating in their homes and reduced stress from financial pressure – than to the physical effects of exposure to improved internal conditions.
Thermal comfort, staying warm and use of living space
Participants of the second round of focus groups agreed that their homes were warmer and more comfortable following the energy efficiency upgrades to their homes, which meant that they no longer needed to find ways to economise on their fuel bills, for example by restricting their use of heating or finding alternative ways of keeping warm. They discussed that they could now more easily afford to maintain their whole house at a more comfortable temperature using their central heating. This negated the need to use alternative ‘stay warm’ strategies such as the use of blankets. The energy efficiency work not only improved the overall quality of the indoor environment but also opened up space that was previously used less, effectively increasing the amount of usable living space within the home. Similar to the findings of Harrington et al. ,39 participants attached a great importance to their increased ability to keep their homes warm. Most of the participants stated that their homes were now much warmer during the winter and cheaper to heat. A few participants discussed how they now felt more comfortable inviting family or friends into their homes, making them feel less socially isolated.
Fuel poverty
In contrast to Gilbertson et al. ,61 who found that many participants had difficulties assessing whether or not their fuel bills were lower, our study found that participants were generally aware of their reduced fuel bills. Indeed, participants spent a large amount of time discussing their financial savings in detail and how much easier it was to pay their fuel bills; particularly being able to pay a competitive dual-fuel energy tariff, allowing for further discounts and savings. Gilbertson et al. 61 stated that in the Warm Front programme it was likely that householders were experiencing warmer homes but generally not also the dual benefit of reduced bills. This is likely owing to differences in the two energy efficiency schemes: Arbed 2 invested more financially into each home within the scheme, taking a whole-house approach, and the criteria for eligibility to the programmes were different. Shortt and Rugkåsa53 further found that, although energy efficiency measures helped to improve warmth, some households remained in fuel poverty after the measures were installed, suggesting that, although homes might become easier to heat, this does not always translate to lifting households out of fuel poverty and thereby potentially concealing possible health gains from such an intervention. Post intervention, the ‘heat-or-eat’ dilemma was not discussed except by one participant who mentioned that savings made on fuel costs meant they did have more money now to spend on a specialised health diet, although this also meant that the savings did not make them feel better off.
Experiences with the intervention programme
Participants in the second round of focus groups appreciated that the energy efficiency improvements were provided for free and were generally happy about the results. According to the participants, the improvements made a big difference to the warmth of their homes. One occupant, who had received energy efficiency measures through a different scheme, felt that the work had been much more effective in making their home warmer. The work was not only seen to be beneficial in terms of providing affordable warmth but it was also felt that the external wall insulation had improved the aesthetics of their homes and the local environment. Participants discussed in detail how this improved their general sense of well-being and pride. Participants in one focus group discussed the importance of energy efficiency schemes for Wales and, in particular, for local communities such as theirs.
The participants were more critical of the delivery of the energy efficiency measures themselves. They expressed some dissatisfaction about the quality of communication, work conducted by contractors and a lack of involvement in the selection of energy efficiency measures that they felt would be the most beneficial. Participants discussed that regular public meetings both before and during the improvement programme, as well as more personal contact between households and scheme managers, could have improved communication. This would have helped to clarify the aims of the programme, reduced misunderstandings about the measures households were to receive and enabled them to report issues with contractors more easily. Finally, participants discussed that they felt that they had too little say over the measures they would receive and expressed some frustration over the fact that they were expected to simply accept the measures because they were free. In particular, participants who rented their home privately or socially felt that they had little or no control over the whole process.
The results regarding the delivery of the programme resonate with previous research. Gilbertson et al. 61 also found that recipients of a similar warm homes programme expressed frustration about the delivery of the work. Some found the process of installation disempowering and stressful, which could undermine the well-being of an already vulnerable population. Harrington et al. 39 argued that people in fuel poverty should not be viewed as passive targets for benevolence and that government-funded interventions will confer far greater benefit if recipients are made to feel empowered. A greater emphasis on partnership with the community could help address individual concerns, improve feelings of control and, as a result, alleviate stress associated with the delivery of a housing improvement programme. 168 Marmot et al. 6 argued in their review that it is important for programmes to create opportunities for individuals and communities to set the agenda for change and identify local solutions. Indeed, participants of the reconvened focus groups felt that the benefits of the programme would have been greater if they had been more closely involved in the decision-making process.
Discussion
The reconvened focus group study that was conducted as part of the residents’ engagement proved to be a useful addition to the research project. The study provided a better understanding of the views and experiences of residents undergoing energy efficiency improvements, which resonated with the results of the research project. The study also gained from resident involvement in the research project itself. A number of participants were recruited to a resident panel to provide feedback and contribute to the SSC of the research project. This helped to ensure that the views of residents were represented in the research.
The reconvened focus groups showed the importance of structural energy performance investments of hard-to-heat, hard-to-treat houses in low-income neighbourhoods. The study found that living in a cold home was generally viewed as depressing, stressful and detrimental to both mental and physical health. According to the participants, the energy efficiency work made great improvements to the comfort and warmth of their homes, opened up spaces within the home and substantially reduced their heating bills. This not only helped to relieve financial stress and fuel poverty but also made them feel less socially isolated. Participants felt that physical health improvements following the work were secondary to improvements in thermal comfort and their ability to invite friends and family into their homes. The lack of discussions about physical health following the completion of the work suggests that adverse physical conditions inside the home are no longer experienced as urgent.
Although the improvements were, for the most part, positively received by occupants and clearly fulfilled the goal of the programme to make homes warmer and cheaper to heat, there were some complaints about the delivery of the work itself. This adds weight to the need to consider community engagement and communication to involve residents more closely in the decision-making and delivery of affordable warmth programmes.
Appendix 3 Consolidated criteria for reporting qualitative research checklist
Item | Guide questions/description | Reported on page |
---|---|---|
Domain 1: research team and reflexivity | ||
Personal characteristics | ||
1. Interviewer/facilitator | Which author/s conducted the interview or focus group? | 104, Methods |
2. Credentials | What were the researcher’s credentials? (e.g. PhD, MD) | NA |
3. Occupation | What was their occupation at the time of the study? | NA |
4. Gender | Was the researcher male or female? | NA |
5. Experience and training | What experience or training did the researcher have? | NA |
Relationship with participants | ||
6. Relationship established | Was a relationship established prior to study commencement? | 104, Methods |
7. Participant knowledge of the interviewer | What did the participants know about the researcher? (e.g. personal goals, reasons for doing the research) | 104, Methods |
8. Interviewer characteristics | What characteristics were reported about the interviewer/facilitator? (e.g. bias, assumptions, reasons and interests in the research topic) | 104, Methods |
Domain 2: study design | ||
Theoretical framework | ||
9. Methodological orientation and theory | What methodological orientation was stated to underpin the study? (e.g. grounded theory, discourse analysis, ethnography, phenomenology, content analysis) | 104, Analysis |
Participant selection | ||
10. Sampling | How were participants selected? (e.g. purposive, convenience, consecutive, snowball) | 103–4, Methods |
11. Method of approach | How were participants approached? (e.g. face-to-face, telephone, mail, e-mail) | 104, Methods |
12. Sample size | How many participants were in the study? | 103, Methods |
13. Non-participation | How many people refused to participate or dropped out? Reasons? | 104, Methods |
Setting | ||
14. Setting of data collection | Where were the data collected? (e.g. home, clinic, workplace) | 103, Box 2 |
15. Presence of non-participants | Was anyone else present besides the participants and researchers? | NA |
16. Description of sample | What are the important characteristics of the sample? (e.g. demographic data, date) | 104, Methods |
Data collection | ||
17. Interview guide | Were questions, prompts, guides provided by the authors? Was it pilot tested? | Appendix 1, 101–2 |
18. Repeat interviews | Were repeat interviews carried out? If yes, how many? | 104, Methods |
19. Audio/visual recording | Did the research use audio or visual recording to collect the data? | 104, Methods |
20. Field notes | Were field notes made during and/or after the interview or focus group? | NA |
21. Duration | What was the duration of the interviews or focus group? | Pages 103 and 104, Methods |
22. Data saturation | Was data saturation discussed? | NA |
23. Transcripts returned | Were transcripts returned to participants for comment and/or correction? | NA |
Domain 3: analysis and findings | ||
Data analysis | ||
24. Number of data coders | How many data coders coded the data? | 104, Analysis |
25. Description of the coding tree | Did authors provide a description of the coding tree? | 105, Figure 25 |
26. Derivation of themes | Were themes identified in advance or derived from the data? | 104, Methods |
27. Software | What software, if applicable, was used to manage the data? | 104 (NVivo) |
28. Participant checking | Did participants provide feedback on the findings? | NA |
Reporting | ||
29. Quotations presented | Were participant quotations presented to illustrate the themes/findings? Was each quotation identified? (e.g. participant number) | NA, see page 104 for a reference to Grey et al.161 |
30. Data and findings consistent | Was there consistency between the data presented and the findings? | 105–8, Results |
31. Clarity of major themes | Were major themes clearly presented in the findings? | 105–8, Results |
32. Clarity of minor themes | Is there a description of diverse cases or discussion of minor themes? | 108, Discussion |
Appendix 4 Resident engagement: key findings brochure
Appendix 5 Resident engagement: pull-up banner
Appendix 6 Template for Intervention Description and Replication checklist
Item number | Item | Page number | Further details |
---|---|---|---|
BRIEF NAME | |||
1 | Provide the name or a phrase that describes the intervention | 3 | |
WHY | |||
2 | Describe any rationale, theory or goal of the elements essential to the intervention | 3 | |
WHAT | |||
3 | Materials: describe any physical or informational materials used in the intervention, including those provided to participants or used in intervention delivery or in training of intervention providers. Provide information on where the materials can be accessed (e.g. online appendix, URL) | 4 | There was a large amount of variation in materials in the two phases of the intervention programme; a list of materials may be available from the scheme managers of the different schemes |
4 | Procedures: describe each of the procedures, activities and/or processes used in the intervention, including any enabling or support activities | 4–5 | |
WHO PROVIDED | |||
5 | For each category of intervention provider (e.g. psychologist, nursing assistant), describe their expertise, background and any specific training given | 5 | |
HOW | |||
6 | Describe the modes of delivery (e.g. face-to-face or by some other mechanism, such as internet or telephone) of the intervention and whether it was provided individually or in a group | 5 | |
WHERE | |||
7 | Describe the type(s) of location(s) where the intervention occurred, including any necessary infrastructure or relevant features | 4 | |
WHEN and HOW MUCH | |||
8 | Describe the number of times the intervention was delivered and over what period of time, including the number of sessions, their schedule and their duration, intensity or dose | 4–5 | |
TAILORING | |||
9 | If the intervention was planned to be personalised, titrated or adapted, then describe what, why, when and how | 5 | |
MODIFICATIONS | |||
10 | If the intervention was modified during the course of the study, describe the changes (what, why, when and how) | NA | |
HOW WELL | |||
11 | Planned: if intervention adherence or fidelity was assessed, describe how and by whom, and if any strategies were used to maintain or improve fidelity, describe them | NA | |
12 | Actual: if intervention adherence or fidelity was assessed, describe the extent to which the intervention was delivered as planned | NA |
Appendix 7 Emergency-hospital-admission-based outcome classification
The method of classifying hospital admissions in PEDW as primary outcomes, and as COPD outcomes for a subanalysis, is shown in Figure 26. Emergency admissions were identified using the admission method of the first episode within a person spell (representing continuous periods of inpatient care for a single patient), with primary diagnosis of cardiovascular or respiratory conditions contained within chapters ‘I’ (‘Diseases of the Circulatory System’) or ‘J’ (‘Diseases of the Respiratory System’) of the ICD-10. 85 Episodes representing symptoms and signs involving the circulatory and respiratory systems (R00–R09) in the first diagnostic position with no subsequent secondary diagnosis codes were also classified as a primary outcome.
FIGURE 26.
Flow chart to define emergency hospital admission outcomes. FPD, first non-RZ, missing diagnosis code; PD, first diagnosis code (position 1); RZ, R or Z codes (from ICD-10 Chapters 18 or 21, respectively); SD, second to fourteenth position diagnosis code.
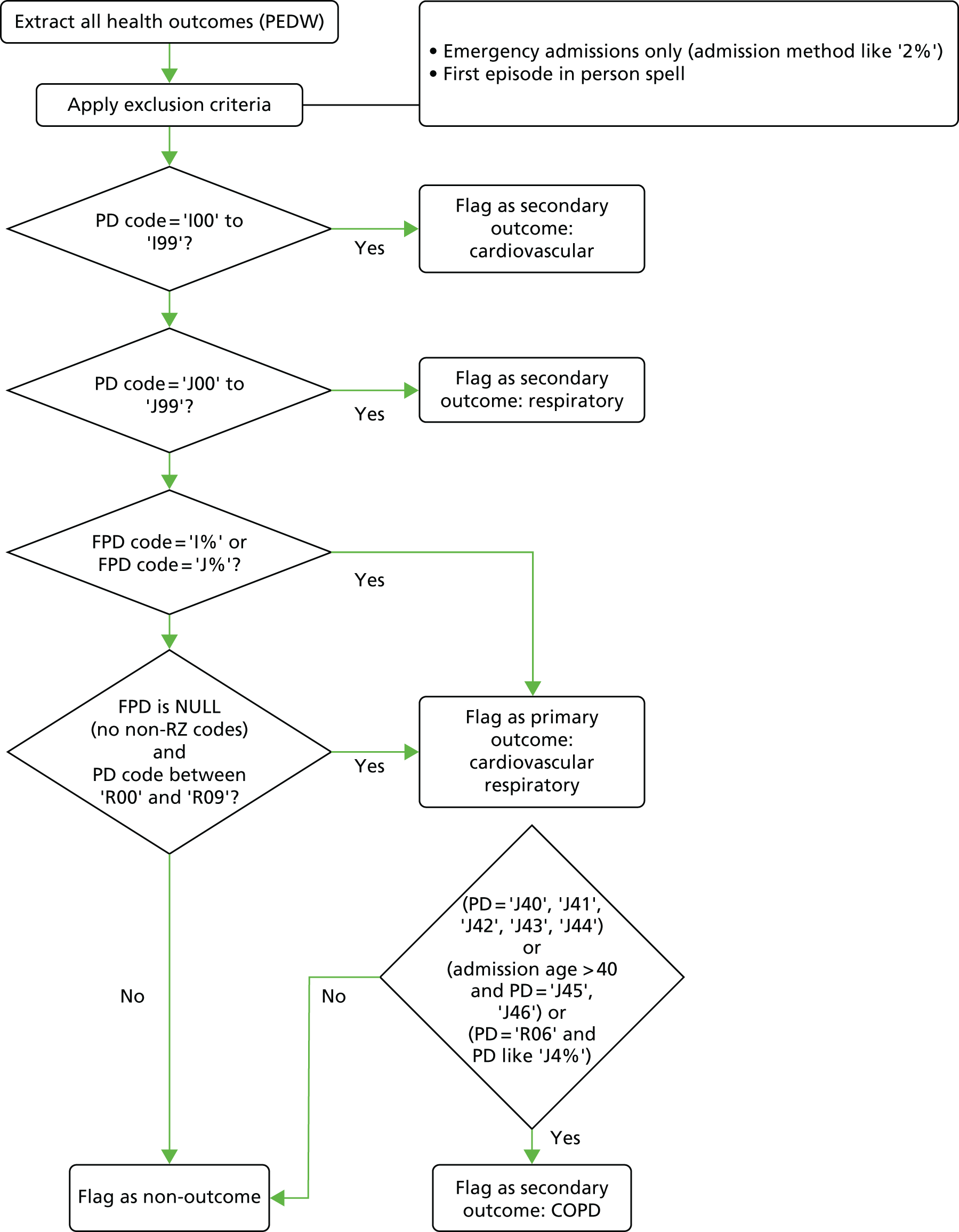
The COPD outcomes using ICD-10 codes as a subgroup of the primary outcome emergency admissions were selected, measured separately for people of all ages and those aged > 60 years. The codes and age-based rules are shown below.
All admissions for any age for codes
-
J40 (bronchitis, not specified as acute or chronic).
-
J41 (simple and mucopurulent chronic bronchitis).
-
J42 (unspecified chronic bronchitis).
-
J43 (emphysema).
-
J44 (other chronic obstructive pulmonary disease).
Admissions for which age at admission is > 40 years for codes
-
J45 (asthma).
-
J46 (status asthmaticus).
All admissions for any age for codes
-
R06 primary code, with any ICD-10 code starting with J4.
Subgroups of the primary outcome were created for cardiovascular (codes J00–J99) and respiratory (codes I00–I99) admissions.
Appendix 8 Data for the two comparator groups
The data on the two comparator groups are potentially useful in assessing trends in the study window, following the usual assumption that trends are common across groups. Figures 27 and 28 show the monthly emergency admission rates per person across the study window for the intervention groups, and for the social housing and the top 10% deprivation groups, respectively. These time plots provide evidence of little or no trend in these rates across the study window data; the corresponding correlations between rates across the study window are 0.296 for intervention and social housing groups (conventionally regarded as weak) and 0.506 for intervention and the top 10% deprivation groups (somewhat stronger but conventionally only regarded as moderate).
FIGURE 27.
Monthly emergency admission rates per person for intervention and social housing groups (study month 1 is January 2005).
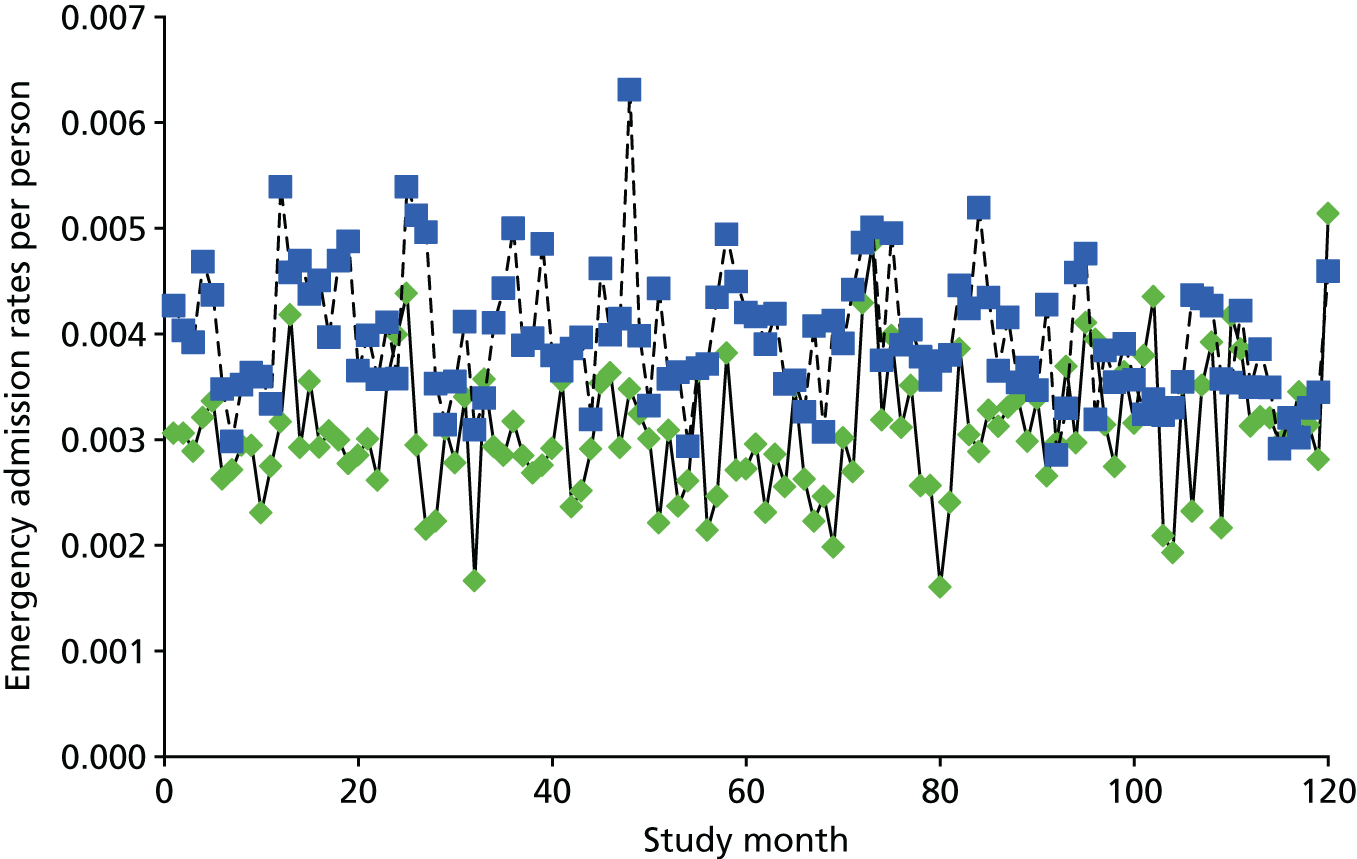
FIGURE 28.
Monthly emergency admission rates per person for intervention and top 10% deprivation groups (study month 1 is January 2005).
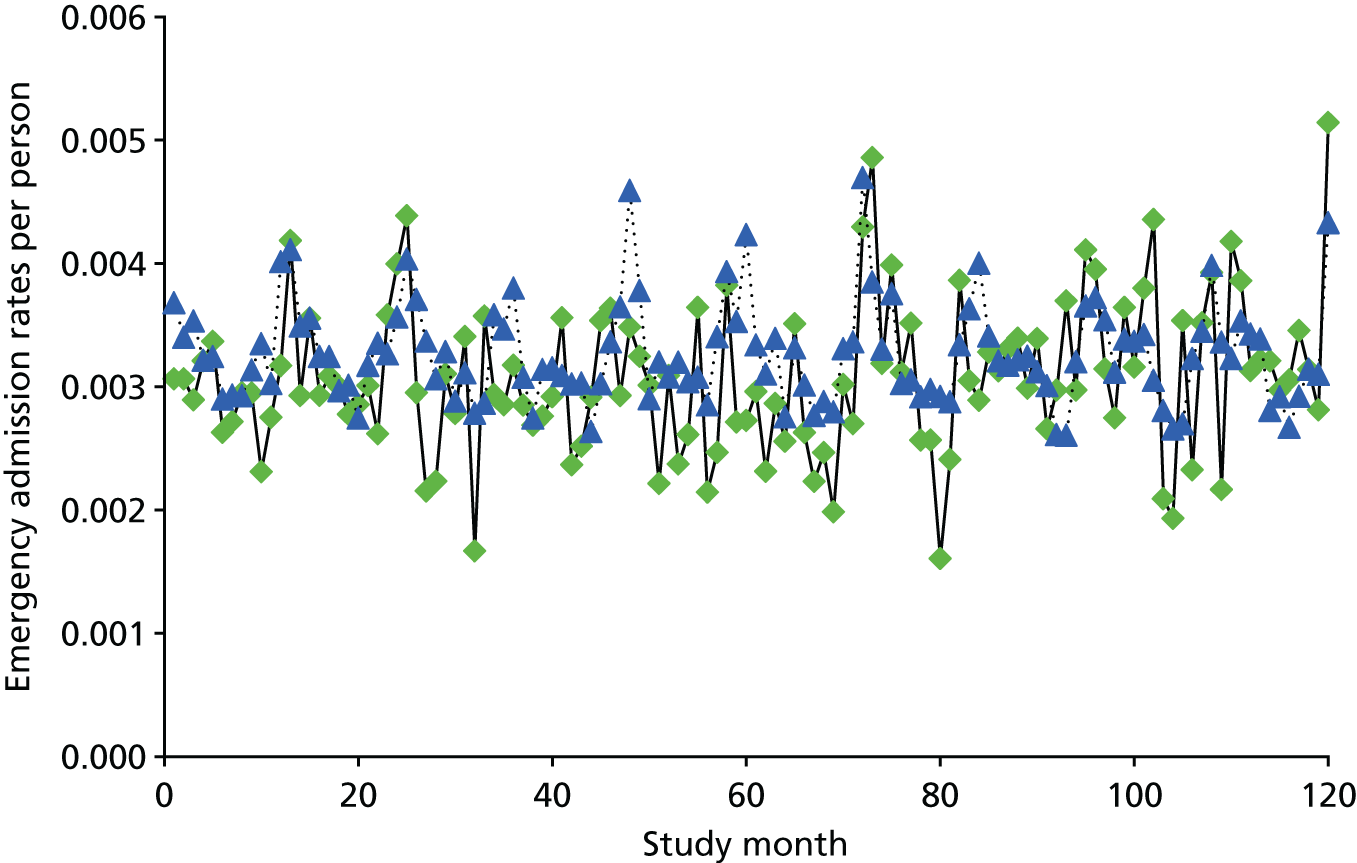
The time plots and correlations offer only limited support for using data from either comparator group in the analysis of the intervention group rates.
Appendix 9 Community-based study: the health questionnaire
Questions 1–7 were from the SF-12 version 2 and have been redacted from this appendix.
Appendix 10 Consolidated Health Economic Evaluation Reporting Standards checklist
Section/item | Item number | Recommendation | Page number |
---|---|---|---|
Title and abstract | |||
Title | 1 | Identify the study as an economic evaluation or use more-specific terms such as ‘cost-effectiveness analysis’, and describe the interventions compared | XX |
Abstract | 2 | Provide a structured summary of objectives, perspective, setting, methods (including study design and inputs), results (including base-case and uncertainty analyses) and conclusions | XX |
Introduction | |||
Background and objectives | 3 | Provide an explicit statement of the broader context for the study | XX |
Present the study question and its relevance for health policy or practice decisions | |||
Methods | |||
Target population and subgroups | 4 | Describe characteristics of the base-case population and subgroups analysed, including why they were chosen | XX |
Setting and location | 5 | State relevant aspects of the system(s) in which the decision(s) need(s) to be made | XX |
Study perspective | 6 | Describe the perspective of the study and relate this to the costs being evaluated | XX |
Comparators | 7 | Describe the interventions or strategies being compared and state why they were chosen | XX |
Time horizon | 8 | State the time horizon(s) over which costs and consequences are being evaluated and say why appropriate | XX |
Discount rate | 9 | Report the choice of discount rate(s) used for costs and outcomes and say why appropriate | XX |
Choice of health outcomes | 10 | Describe what outcomes were used as the measure(s) of benefit in the evaluation and their relevance for the type of analysis performed | XX |
Measurement of effectiveness | 11a | Single study-based estimates: describe fully the design features of the single effectiveness study and why the single study was a sufficient source of clinical effectiveness data | XX |
11b | Synthesis-based estimates: describe fully the methods used for identification of included studies and synthesis of clinical effectiveness data | XX | |
Measurement and valuation of preference-based outcomes | 12 | If applicable, describe the population and methods used to elicit preferences for outcomes | NA |
Estimating resources and costs | 13a | Single study-based economic evaluation: describe approaches used to estimate resource use associated with the alternative interventions. Describe primary or secondary research methods for valuing each resource item in terms of its unit cost. Describe any adjustments made to approximate to opportunity costs | XX, Appendix 12 |
13b | Model-based economic evaluation: describe approaches and data sources used to estimate resource use associated with model health states. Describe primary or secondary research methods for valuing each resource item in terms of its unit cost. Describe any adjustments made to approximate to opportunity costs | XX | |
Currency, price date, and conversion | 14 | Report the dates of the estimated resource quantities and unit costs. Describe methods for adjusting estimated unit costs to the year of reported costs if necessary. Describe methods for converting costs into a common currency base and the exchange rate | XX, Table XX, Appendix 11 |
Choice of model | 15 | Describe and give reasons for the specific type of decision-analytic model used. Providing a figure to show model structure is strongly recommended | XX |
Assumptions | 16 | Describe all structural or other assumptions underpinning the decision-analytic model | XX |
Analytical methods | 17 | Describe all analytical methods supporting the evaluation. This could include methods for dealing with skewed, missing or censored data; extrapolation methods; methods for pooling data; approaches to validate or make adjustments (such as half cycle corrections) to a model; and methods for handling population heterogeneity and uncertainty | XX |
Results | |||
Study parameters | 18 | Report the values, ranges, references and, if used, probability distributions for all parameters. Report reasons or sources for distributions used to represent uncertainty where appropriate. Providing a table to show the input values is strongly recommended | XX |
Incremental costs and outcomes | 19 | For each intervention, report mean values for the main categories of estimated costs and outcomes of interest, as well as mean differences between the comparator groups. If applicable, report incremental cost-effectiveness ratios | XX |
Characterising uncertainty | 20a | Single study-based economic evaluation: describe the effects of sampling uncertainty for the estimated incremental cost and incremental effectiveness parameters, together with the impact of methodological assumptions (such as discount rate, study perspective) | XX |
20b | Model-based economic evaluation: describe the effects on the results of uncertainty for all input parameters, and uncertainty related to the structure of the model and assumptions | XX | |
Characterising heterogeneity | 21 | If applicable, report differences in costs, outcomes or cost-effectiveness that can be explained by variations between subgroups of patients with different baseline characteristics or other observed variability in effects that are not reducible by more information | NA |
Discussion | |||
Study findings, limitations, generalisability and current knowledge | 22 | Summarise key study findings and describe how they support the conclusions reached. Discuss limitations and the generalisability of the findings and how the findings fit with current knowledge | XX |
Other | |||
Source of funding | 23 | Describe how the study was funded and the role of the funder in the identification, design, conduct and reporting of the analysis. Describe other non-monetary sources of support | XX |
Conflicts of interest | 24 | Describe any potential for conflict of interest of study contributors in accordance with journal policy. In the absence of a journal policy, we recommend authors comply with International Committee of Medical Journal Editors recommendations | XX |
Appendix 11 Costs of intervention measures and their estimated lifetime
Intervention measure | Number delivered | Estimated lifetime-years (DECC)147 | Cost (£) | ||
---|---|---|---|---|---|
Indicative (WG)148 | Intervention measure | Yearly per measure | |||
External wall insulation | 2709 | 36 | 7300 | 19,775,700 | 549,325 |
Photovoltaics | 1458 | 25 | 5400 | 7,873,200 | 314,928 |
Solar water heating | 928 | 25 | 2600 | 2,412,800 | 96,512 |
Air source heat pumps | 401 | 15 | 6000 | 2,406,000 | 160,400 |
Loft/rafter insulation | 172 | 42 | 100 | 17,200 | 409.52 |
Total cost | 32,484,900.00 | 1,121,574.52 |
The total yearly cost of £1,121,574.52 represents each of the individual measures’ totals divided by their estimated lifetime. The per-house yearly cost is the total yearly cost divided by the number of properties (n = 4968), which results in a figure of £225.76.
Appendix 12 Chronic obstructive pulmonary disease utilities literature review
Introduction
The review was designed as a structured narrative literature review. It sought to capture and draw conclusions from the main body of literature reporting health state utility values (HSUVs) for patients with COPD in order to build a profile of HSUVs before, during and after admission to hospital with an exacerbation, including the longer-term residual effect.
The focus was on secondary health-care utilisation as the majority of patients with COPD are elderly and thus are at risk of prolonged hospital stays. 169 Hospital admission can have a considerable impact on subsequent quality of life, often disempowering individuals and reducing functional independence. 170,171
The evidence was compiled following systematic procedures and, when possible, adhered to PRISMA (Preferred Reporting Items for Systematic Reviews and Meta-Analyses; see Appendix 15). PRISMA is an evidence-based minimum set of items for reporting in systematic reviews and meta-analyses. PRISMA focuses on the reporting of reviews evaluating randomised trials, but can also be used as a basis for reporting systematic reviews of other types of research, particularly evaluations of interventions. The protocol for the review was devised and included an initial scoping of the literature to identify existing reviews, a comprehensive search strategy to identify relevant studies and a narrative data synthesis to provide a summary of results in relation to each objective.
Aim and objectives
To review the literature in order to build a profile of HSUVs for patients with COPD before, during and after admission to hospital with an exacerbation of COPD:
-
to quantify HSUVs for stable COPD patients
-
to quantify the change in HSUV from hospital admission to discharge
-
to quantify the residual effect of a hospital admission
-
to identify other confounding variables that cause variation in HSUVs.
Methods
A scoping exercise identified several existing reviews that provided pooled HSUVs for patients with COPD, including three open access reviews and one review of which only the abstract was available. 151,172,173 The scoping search revealed the availability of many recent systematic reviews/meta-analyses in relation to HSUVs in patients with stable COPD (objective i). The focus of this review was to capture any additional reviews relevant to stable COPD (objective i) and to identify studies reporting HSUVs at admission and discharge (objective ii), the residual effect of a hospital admission (objective iii) and confounding variables (objective iv).
The review was designed using a two-stage process. First, a broad overview of HSUV studies for patients with COPD was undertaken to identify the number and type of studies that are currently available in the literature. The second selection process used additional inclusion and exclusion criteria to identify those studies relevant to each objective. Primary inclusion and exclusion criteria were designed to incorporate all studies reporting HSUVs for patients with COPD. The study used the inclusion and exclusion criteria reported in Table 24.
Inclusion | Exclusion | |
---|---|---|
Population |
|
|
Study type |
|
|
Context |
|
NA |
Outcomes |
|
|
Secondary inclusion and exclusion criteria were developed to extract studies relevant to each objective (Table 25). Based on the results of the first-stage search, it was established that the majority of studies used EQ-5D-generated utility values; therefore, this was included in the secondary inclusion criteria in order to ensure consistency and validity of the HSUV profile.
Objective | Inclusion | Exclusion |
---|---|---|
Change in HSUV during hospital admission |
|
|
Residual HSUV post hospital admission |
|
NA |
Confounding variables |
|
|
The review was restricted to articles published in academic journals. These were sourced from both electronic databases and reference searching of relevant review papers. Searches were conducted on 27 July 2016. Advice was sought from subject librarians with regard to relevant electronic databases and search terms for the review.
The following electronic databases were searched: (1) PubMed (via NCBI), (2) Web of Science (via Thompson Reuters), (3) Scopus (via Elsevier), (4) The Cochrane Library (via Wiley Online Library), which includes the Cochrane Database of Systematic Reviews, the Database of Abstracts of Reviews of Effectiveness, the Cochrane Central Register of Controlled Trials and the Cochrane Methodology Register, (5) ProQuest Health Management and (6) Cumulative Index to Nursing and Allied Health Literature (CINAHL) (via EBSCOhost).
Search strategy
The search strategy was developed around the main themes of the review aim: ‘chronic obstructive pulmonary disease’ and ‘HSUV’. Therefore, the search focused on all generic instruments for measuring health-related quality of life that produce HSUVs [EQ-5D, SF-6D, Health Utilities Index (HUI)-2, HUI-3, Quality of Well-Being (QWB), Assessment of Quality of Life (AQoL), AQoL2] and related terms (i.e. EuroQol and Health Utilities Index), as well as all terms that could represent COPD.
The final search terms were:
COPD OR COAD OR “chronic obstructive pulmonary disease” OR “chronic obstructive lung disease” OR “chronic lung disease” OR “chronic respiratory” OR “chronic obstructive airway disease” OR emphysema OR bronchitis
AND
“health state” OR “health states” OR “health preference” OR “health preferences” OR “health utility” OR “health utilities” OR “utility index” OR “utilities index” OR “utility indices” OR “utility measure” OR “utility measures” OR “utility value” OR “utility values” OR “utility score” OR “utility scores” OR “utility outcome” OR “utility outcomes” OR “utility weight” OR “utility weights” OR “utility evaluation” OR “utility evaluations” OR HUI OR HSUV OR eq5d OR euroqol* OR “eq 5d” OR “euro qol” OR “euro qols” OR “utility analysis” OR “utility analyses” OR “cost utility” OR “cost utilities” OR CUA OR QALY OR “quality adjusted life year” OR “quality adjusted life years” OR “time trade off” OR “time tradeoff” OR “timetrade off” OR TTO OR “standard gamble” OR sf6d OR “sf 6d” OR “short form 6 dimension” OR “short form 6 dimensions” OR “short form 6” OR AQoL OR “assessment of quality of life” OR HUI2 OR HUI3 OR QWB OR QWB-SA OR “quality of well-being” OR 15D OR “15 dimensional”
A combination of title, abstract or topic searches were adapted for use with each database depending on the options available. Limitations included papers published in the English language and no date restrictions were set. The full, unedited search strategies for each database can be found in Appendix 13. All studies that were identified via electronic database searches were exported into EndNoteWeb [Clarivate Analytics (formerly Thomson Reuters), Philadelphia, PA, USA] and duplicates removed. The remaining references were then exported to Microsoft Excel® (Microsoft Corporation, Redmond, WA, USA).
Study selection
A multistage selection process was undertaken by the reviewer. First, all titles and abstracts were screened against the inclusion criteria with a preference towards overinclusion in cases that were unclear. The reviewer was not blinded to author and journal details. Next, full papers were obtained and, owing to time constraints, those that were not available as open access sources were excluded from the review. Following this, full studies were assessed against both the inclusion and exclusion criteria. Many of the studies include duplicate data sets and these were therefore grouped together to ensure that information was not reported twice.
Seventy-eight studies were accepted after the primary inclusion and exclusion criteria were applied (see Table 24 and Figure 29). An overview of these studies was given, including country of origin, instruments used to measure HSUVs, tariff values used and data sets used (Table 26). Subsequently, secondary inclusion and exclusion criteria (see Table 25) were applied to filter these further, so that papers relevant to each objective only were selected. The full review process was documented using the PRISMA flow diagram (see Figure 29).
FIGURE 29.
Flow diagram: study selection process following PRISMA guidelines.
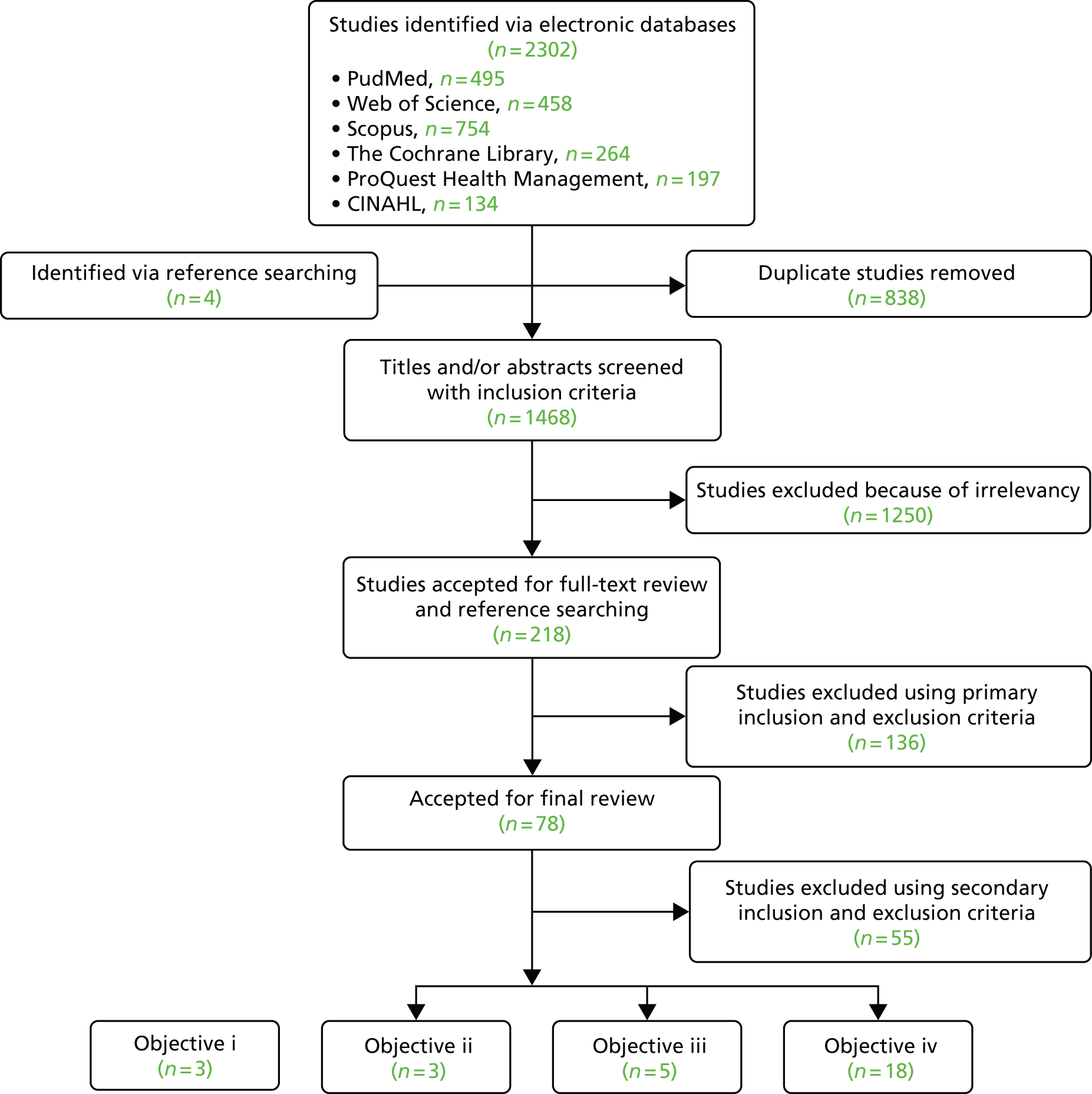
Author | Country | Instrument | Tariff values | Data set |
---|---|---|---|---|
Boland et al.174 | The Netherlands | EQ-5D | – | RECODE trial175,176 |
Burns et al.177 | UK | EQ-5D | UK | Wilson et al.178 |
Cramm and Nieboer179 | The Netherlands | EQ-5D | – | Zichtbare visible link programme180,181 |
Ding et al.182 | China | SF-36v2 (SF-6D), SF-12v2 (SF-6D) | – | CNHW survey183 |
Dritsaki et al.184 | UK | EQ-5D | UK | SPACE trial185,186 |
Eklund et al.187 | Canada, Spain, Sweden, UK | EQ-5D | – | SPARK188 and UPLIFT189 trial |
Esteban et al.154 | Spain | EQ-5D | – | IRYSS-CAS190 |
Hoyle et al.191 | UK | EQ-5D | UK | ClinicalTrials.gov and GlaxoSmithKline studies192 |
Jia et al.193,194 | USA | EQ-5D | – | NHANES 2005–2008195,196 |
Kwon and Kim194,197 | South Korea | EQ-5D | South Korean | KNHANES 2007–2012198,199 |
Lee et al.194,200 | South Korea | EQ-5D | – | KNHANES 2007–2012194 |
Lee et al.194,201 | South Korea | EQ-5D | South Korean | KNHANES 2007–2012194 |
Martinez Rivera et al.202 | Spain | EQ-5D | – | Original data203,204 |
Roncero et al.205 | Spain | EQ-5D | – | Original data206 |
Selya-Hammer et al.207 | Italy | EQ-5D | – | Rutten-van Mölken et al.208 |
Wacker et al.209 | Germany | EQ-5D | German | Rutten-van Mölken et al.210 |
Zanaboni et al.211 | Norway | EQ-5D | – | Original data211 |
Boland et al.212 | The Netherlands | EQ-5D | Dutch | RECODE trial175,176 |
Boland et al.213 | The Netherlands | EQ-5D | – | RECODE,175,176 GO-AHEAD,214 MARCH215 trials |
Fishwick et al.216 | UK | EQ-5D | UK | Original data217 |
Hong et al.194,218 | South Korea | EQ-5D | South Korean | KNHANES 2007–2010199 |
Kim et al.194,219 | South Korea | EQ-5D | South Korean | KNHANES 2007–2010199 |
Miravitlles et al.220 | Spain | EQ-5D | Spanish | Original data – same data as Miravitlles et al.221 |
Miravitlles et al.221 | Spain | EQ-5D | Spanish | Original data – same data as Miravitlles et al.220 |
Stoddart et al.222 | UK | EQ-5D | UK | Original data223,224 |
Sundh et al.225 | Sweden | EQ-5D | UK | Original data225 |
Wilson et al.178 | UK | EQ-5D | – | Original data178 |
Wu et al.226 | China | EQ-5D | – | Original data226 |
Abdin et al.227 | Singapore | EQ-5D | UK | SMHS228 |
van Boven et al.229 | UK, Sweden | EQ-5D | – | PHARMACOP230 |
Chen et al.231 | Hong Kong | EQ-5D | UK | Original data231 |
García-Rio et al.232 | Spain | EQ-5D | – | EPI-SCAN study233,234 |
Karabis et al.235 | USA | EQ-5D | – | Rutten-van Mölken et al.210 |
Kim et al.236 | South Korea | EQ-5D | South Korean | Original data236 |
Lin et al.237 | USA | EQ-5D | USA | CONCERT study238 |
Miravitlles et al.239 | Spain | EQ-5D | Spanish | Original data239 |
Miravitlles et al.206 | Spain | EQ-5D | Spanish | Original data – DEPREPOC study206 |
Miravitlles et al.240 | Spain | EQ-5D | Spanish | Original data – INSEPOC study241,271 |
Mullen et al.242 | Canada | EQ-5D | USA | Combined data set243 |
Peters et al.244 | UK | EQ-5D | – | Original data – same data as Peters et al.245 |
Peters et al.245 | UK | EQ-5D | – | Original data – same data as Peters et al.244 |
Samyshkin et al.246 | UK | EQ-5D | – | Studies M2–124 and M2–125, Rabe247 and Rutten-van Mölken et al.158 |
Tsiachristas et al.248 | The Netherlands | EQ-5D | Dutch | Original data249 |
Gillespie et al.250 | Ireland | EQ-5D | UK | PRINCE trial251 |
Samyshkin et al.252 | Switzerland | EQ-5D | – | Rutten-van Mölken et al.158 and Takeda Pharma AG (Pfäffikon, Switzerland) |
Solem et al.253 | USA | EQ-5D | USA | Original data253 |
Asukai et al.254 | UK | EQ-5D | UK | INVOLVE,255 INHANCE256 and INLIGHT-2257 trials |
DiBonaventura et al.258 | USA | SF-12v2 (SF-6D) | – | NHWS259 |
Goodacre et al.260 | UK | EQ-5D | UK (using pilot-study data) | Original data260 |
Taylor et al.261 | UK | EQ-5D | UK | Original data261 |
Fletcher et al.262 | Brazil, China, Germany, Turkey, USA, UK | EQ-5D | Germany, UK and USA | Original data262 |
Goosens et al.263 | USA | EQ-5D | USA | CASA-Q264 |
Janssen et al.265 | The Netherlands | EQ-5D | – | Original data266–268 |
Khdour et al.269 | UK | EQ-5D | UK | Khdour et al.270 |
Miravitlles et al.271 | Spain | EQ-5D | Spanish | Original data – INSEPOC study241,271 |
Pickard et al.173 | USA | SF-36, EQ-5D | UK and USA | Joo et al.272 |
Starkie et al.273 | Worldwide | EQ-5D | UK | TORCH study274 |
Hoogensoorn et al.275 | The Netherlands | EQ-5D | – | Original data275 |
Menn et al.155 | Germany | EQ-5D, SF-12 (SF-6D) | UK | Original data155 |
Rutten-van Mölken et al.158 | The Netherlands | EQ-5D | In-study valuation | Original data158 |
Szende et al.276 | Sweden | EQ-5D | UK | Combined data – Szende et al.277 and OLIN278 |
Punekar et al.279 | USA, France, Germany, Italy, Spain, UK | EQ-5D | UK | Original data – the Respiratory Disease Specific Programme III279 |
O’Reilly et al.156 | Germany | EQ-5D | UK | Original data156 |
Rutten-van Mölken et al.208 | Spain | EQ-5D | Spanish | Six clinical trials and pooled data280–283 |
Rutten-van Mölken et al.210 | 13 countries (% participants): USA (34.5%), Czechia (17.5%), Spain (11.9%), Denmark (8.4%), Germany (4.9%), Poland (4.8%), the Netherlands (4.4%), Italy (4.4%), France (3.1%), Hungary (2.5%), the Russian Federation (1.5%), Belgium (1.4%), Australia (0.8%) | EQ-5D | UK and USA | UPLIFT trial284 |
Spencer et al.285 | UK | EQ-5D | – | Prescott-Clarke et al.,286 Brazier et al.,105 Spencer et al.287 |
Ståhl et al.288 | Sweden | EQ-5D, SF-36 (SF-6D) | – | OLIN studies289–291 |
Sullivan et al.292 | USA | EQ-5D | USA | MEPS293 |
Borg et al.294 | Sweden, UK | EQ-5D | UK | OLIN studies289,295,296 |
Brazier et al.297 | UK | EQ-5D, SF-6D | UK | Thomas et al.298 |
Kaplan et al.299 | USA | SF-36 (SF-6D), QWB-SA | – | NETT300 |
Hazell et al.301 | UK | EQ-5D | UK | Original data301 |
Stavem et al.302 | The Netherlands | EQ-5D | UK | Original data – same data as Stavem303 |
Paterson et al.304 | UK | EQ-5D | UK | Original data304 |
Stavem303 | Norway | EQ-5D, 15D, SF-36 (SF-6D) | UK | Original data – same data as Stavem et al.302 |
Torrance et al.305 | Canada | HUI | – | Original data305 |
Harper et al.306 | UK | EQ-5D, SF-36 (SF-6D) | – | Original data306 |
Jaeschke et al.307 | Canada | QWB | – | Original data307 |
Data collection process
Data were extracted from the final selection of papers in a systematic manner in which each study was scanned for the same fields. When multiple papers reported the same data set(s), only one paper was included. Several criteria were used to prioritise the paper chosen in the following order: first, studies using patient-reported data were prioritised (as opposed to secondary data used in modelling studies); second, the papers with the most-recent data set were chosen (e.g. a study reporting 2014–16 data instead of 2013–15 data); and third, the study reporting the largest sample size and/or most information was selected.
Data items
The data items extracted included the following: author, year, country of origin (data), sample size, age, sex, COPD GOLD stage/severity level, study type, time of measurement of EQ-5D, data source, EQ-5D tariff value and HSUVs. Risk of bias was assessed at outcome level only. The primary outcome was HSUV.
Overview of all health state utility values studies for patients with chronic obstructive pulmonary disease
After the initial search, 79 studies were identified to include HSUVs for patients with COPD. The studies incorporated data from the UK, Spain, France, the Netherlands, Germany, Switzerland, Italy, Norway, Sweden, Turkey, Brazil, China, the USA and Canada. In addition, several studies involved multicountry data sets in which individual countries were not identified. 210,273
The majority of studies reported HSUVs based on the EQ-5D (73 studies154–156,158,173,174,177–179,184,187,191,193,194,197,200–202,205–213,216,218–222,225–227,229,231,232,235–237,239,240,242,244–246,248,250,252–254,260–262,265,269,271,273,275,276,279,285,288,292,294,297,301–304,306); some studies also reported utility values based on the SF-36/SF-12/SF-6D (nine studies155,173,182,258,288,297,299,303,306). In addition, one study used HUI,305 one study used QWB307 and one study used the 15-dimensional measure of health-related quality of life. 303
Eighteen studies177,178,184,191,216,222,244–246,254,260,261,269,286,297,301,304,306 were UK based (UK data set or, if secondary data were used, UK lead authors), five studies included UK data in pooled analysis and 27 studies155,156,173,177,184,191,210,216,222,225,227,231,250,254,260–262,269,273,276,279,294,297,301–304 used UK tariff values. Not all studies explicitly stated the tariff values used so instead, when possible, this was deduced by the references used.
Although a large number of studies were identified, many used overlapping data sets that were not always initially obvious. For instance, modelling studies often used secondary data from the same clinical trials; for example, Eklund et al. 187 and Karabis et al. 235 used data from the UPLIFT trial. 308 Other studies also reported secondary data in other studies; for example, Wacker et al. 209 report data from Burns et al. 177 and Rutten-van Mölken et al. 210 report data from Wilson et al. 178 Many studies used pooled data from several trials; for example, Boland et al. 212 used pooled data from the Randomised Clinical Trial on Effectiveness of Integrated COPD Management in Primary Care (RECODE),175,176 the Assessment Of Going Home Under Early Assisted Discharge (GO-AHEAD) trial214 and the Health Status Guided COPD Care (MARCH) trial. 215 Other studies used data from the same national surveys for different and/or overlapping years; for example, Kwon et al. ,194,197 Lee et al. ,194,201 Hong et al. 194,218 and Kim et al. 194,219 use data from the Korean National Health and Nutrition Examination Survey (KNHANES). 199
The initial scan found three review papers with full-text availability in relation to COPD patients with stable COPD151,172,309 and the full search did not identify any additional reviews (Table 27).
Author | Country | Population | Methods | Instrument | HSUV |
---|---|---|---|---|---|
Pickard et al.309 | All countries considered | COPD and asthma |
Papers published from January 1988 to January 2007 COPD |
EQ-5D | Mean EQ-5D-generated utilities using UK tariff and GOLD stages (SD)
|
Petrillo et al.172 | All countries considered | COPD |
Papers published from 1997 to August 2009 COPD |
All instruments considered (generic and specific) | Range EQ-5D-generated utilities based on GOLD stages for UK studies
|
Moayeri et al.151 | All countries considered | COPD |
Papers published before 2014 COPD English-language studies |
EQ-5D (UK tariff) | Mean EQ-5D-generated utilities using UK tariff and GOLD stages (95% CI)
|
Pickard et al. 309 estimated average HSUVs by disease severity based on pooled data from 1988 to 2007. Petrillo et al. 172 updated the search by Pickard et al. 309 and provided HSUV ranges by disease severity and a summary of the HSUV change during an exacerbation (community or hospital based). Moayeri et al. 151 conducted a meta-analysis on COPD HSUV studies that were published prior to 2014 using UK tariff values and produced average utilities by disease severity.
Change in health state utility values during a hospital admission for an exacerbation of chronic obstructive pulmonary disease
Three studies considered change in EQ-5D-generated HSUVs from hospital admission to discharge,154–156 two of which used UK tariff values. 155,156
There was large disparity in HSUVs between the studies using UK tariff values, particularly in relation to HSUVs on admission. For instance, mean admission and discharge HSUVs for O’Reilly et al. 156 were –0.077 and 0.576, respectively; whereas, for Menn et al. ,155 the reported weighted average admission and discharge HSUVs were 0.61 and 0.78, respectively. However, differences can be explained by the timing of the measurement; whereas patients in O’Reilly et al. 156 completed the EQ-5D ‘on admission’ (i.e. at the most severe part of their exacerbation), patients in Menn et al. 155 completed the EQ-5D ‘within 3 days after admission’ (i.e. after treatment had started, when it is likely symptoms had improved). Similarly, Esteban et al. 154 reported similar values (0.60 on admission and 0.64 on discharge) to those reported by Menn et al. ,155 when data were collected ‘on the first day after admission’, again after treatment had started (Table 28).
Author | Country | Population | Methods | Instrument | HSUV, mean (SD) |
---|---|---|---|---|---|
Esteban et al.154 | Spain | 1421 COPD (all stages) patients admitted to hospital
|
Prospective cohort study; patients recruited on admission to hospital. EQ-5D score measured in an interview at admission (24 hours after ED) and discharge (next day or week after) | EQ-5D |
Admission (n = 1421) = 0.60 (0.28) Discharge (n = 950) = 0.64 (0.29) |
Menn et al.155 | Germany |
117 patients Mean age, years (SD)Sex, male (%) |
Prospective observational study, data collected via patient-completed questionnaire during hospital stay within 3 days of admission and discharge |
EQ-5D SF-12 (SF-6D) German and UK tariff |
German tariffUK tariff GOLD stage 3 (severe) (n = 34): admission = 0.46 (0.31); discharge = 0.72 (0.23) GOLD stage 4 (very severe) (n = 83): admission = 0.44 (0.31); discharge = 0.61 (0.28) Sensitivity to change |
O’Reilly et al.156 | Germany |
All (n = 222) GOLDFollow-up (n = 40) |
Prospective observation study, data collected via self-administered EQ-5D and SF-12 during hospital admission, at discharge and at 3-month follow-up |
EQ-5D (UK tariff) SF-12 |
All (n = 222)
|
Residual effect on utility values after an admission to hospital with an exacerbation of chronic obstructive pulmonary disease
Five studies (Table 29) report the residual effect of EQ-5D-generated HSUVs after an exacerbation requiring hospital admission,156,158,246,252,253 three of which used the same data set. 158,246,252 The residual effect was tracked over three timescales: 3 months,156 12 months158 and a varied timescale related to previous exacerbation. 253 Only one study used UK tariff values. 156
Author | Country | Population | Methods | Instrument | HSUV |
---|---|---|---|---|---|
Solem et al.253 | USA | Overall (n = 314)
|
Cohort study, data collected in a patient interview (including EQ-5D), recruitment via primary care physicians/pulmonologists | EQ-5D (USA tariff) | Overall (n = 314), mean (SD)
|
Rutten-van Mölken et al.158 (same data as in Samyshkin et al.246,252) | The Netherlands | 229 TTO data available
|
Cohort study, recruited via advertisement in local newspaper; health states valued during group session or at home | EQ-5D (Dutch tariff) | Estimated annual utility decrements, mean (SE)
|
O’Reilly et al.156 | Germany | Follow-up (n = 40), GOLD stages
|
Prospective observation study, data collected from self-administered EQ-5D and SF-12 during hospital admission, at discharge and at 3-month follow-up |
EQ-5D (UK tariff) SF-12 |
HSUV, mean (SD)
|
Confounding variables
The studies reported HSUV in a range of different subgroups, the most common being severity level, phenotype, age, sex, number and severity of exacerbations, number and type of comorbidities, smoking history, body mass index (BMI) and socioeconomic status. Tables for the confounding variables can be found in Appendix 14.
Phenotype
Two studies reported utility values by phenotype, although each considered different subgroups. Mean utility values in each group ranged from 0.63 to 0.69 in Ding et al. 182 and from 0.52 to 0.69 in Miravittles et al. 220
Ding et al. 182 found patients with asthma–COPD overlap syndrome (ACOS) and asthma only to have the lowest HSUVs in comparison with COPD only (COPD, chronic bronchitis or emphysema) or controls (those without asthma, COPD, chronic bronchitis or emphysema). In contrast, Miravitlles et al. 220 studied a cohort of COPD patients and found the ‘excerbators with chronic bronchitis’ group to have the lowest HSUV in comparison with ‘excerbators without chronic bronchitis’, ACOS or ‘non-excerbators’.
Age
Four studies considered HSUV by age, with mean HSUV ranging from 0.42 to 0.94. 194,219,262,279,301 All studies showed a general trend towards a decrease in HSUV with age, with a slightly greater decrease seen in females than in males. 194,219,301 However, this sex difference is particularly pronounced in the South Korean study, which, as mentioned previously, has a high percentage of males, meaning differences may be exaggerated by a small female sample size. 194,219
Sex
Six studies reported sex differences in HSUV, with overall mean utility values ranging from 0.60 to 0.93. 191,194,219,225,236,262,279 Four out of six of the studies reported that females have a lower HSUV, with differences ranging from 0.03 to 0.11. 191,225 Two studies reported that males have a higher HSUV; however, both studies have male-dominated cohorts [Kim et al. 236 (91.5% male) and Kim et al. 194,219 (74.0% male)] and are based on a specific population (i.e. South Korean).
Number and severity of exacerbations
Two studies looked at HSUV by number of exacerbations, reporting a reduction in HSUV with an increasing number of exacerbations,221,279 and one study considered utility decrements by severity of exacerbations, reporting greater decrements in HSUVs with increasing severity. 158
Number and type of comorbidities
Three studies considered the effect of specific comorbidities on HSUV. 202,206,221 The greatest differences were found between those with and without depression. Both Martinez Rivera et al. 202 and Miravitlles et al. 206 found that patients with COPD and depression had a lower mean HSUV than patients with COPD only (0.40 and 0.55 vs. 0.76 and 0.83, respectively). Miravitlles et al. 221 also found that those with comorbid cardiovascular disease had a lower mean HSUV (0.78) than patients with COPD only (0.82); however, no differences were found for patients with and without diabetes, and patients with comorbid haematological malignancies had similar HSUVs.
One study measured HSUVs in relation to a number of comorbid diseases in patients with COPD and found that HSUV decreased as the number of comorbidities increased. 194,218 In addition, Miravitlles et al. 221 also considered HSUV in relation to the Charlson index, with mixed results: those scoring 0, 1 and 3 had similar utility scores (0.82, 0.82 and 0.81, respectively), whereas those scoring 2 had a lower HSUV of 0.74.
Smoking history
Three studies assessed smoking status in relation to HSUV in patients with COPD, with mixed results. The studies report smokers with HSUVs higher than,194,201 lower than242 and the same as221 non-smokers.
Appendix 13 Search strategy
PubMed: 495 (date of search: 27 July 2016).
Web of Science: 458 (date of search: 27 July 2016).
Scopus: 754 (date of search: 27 July 2016).
The Cochrane Library: 264 (date of search: 27 July 2016).
ProQuest Health Management: 197 (date of search: 27 July 2016).
CINAHL: 134 (date of search: 27 July 2016).
PubMed | |
---|---|
Search terms |
#1 “Lung Diseases, Obstructive”[Mesh] #2 COPD[tiab] OR COAD[tiab] OR “chronic obstructive pulmonary disease”[tiab] OR “chronic obstructive lung disease”[tiab] OR “chronic lung disease”[tiab] OR “chronic respiratory”[tiab] OR “chronic obstructive airway disease” [tiab] OR emphysema[tiab] OR bronchitis[tiab] #3 “health state”[tiab] OR “health states”[tiab] OR “health preference”[tiab] OR “health preferences”[tiab] OR “health utility”[tiab] OR “health utilities”[tiab] OR “utility index”[tiab] OR “utilities index”[tiab] OR “utility indices”[tiab] OR “utility measure”[tiab] OR “utility measures”[tiab] OR “utility value”[tiab] OR “utility values”[tiab] OR “utility score”[tiab] OR “utility scores”[tiab] OR “utility outcome”[tiab] OR “utility outcomes”[tiab] OR “utility weight”[tiab] OR “utility weights”[tiab] OR “utility evaluation”[tiab] OR “utility evaluations”[tiab] OR HUI[tiab] OR HSUV[tiab] OR eq5d[tiab] OR euroqol*[tiab] OR “eq 5d”[tiab] OR “euro qol” [tiab] OR “euro qols” [tiab] OR “utility analysis”[tiab] OR “utility analyses”[tiab] OR “cost utility”[tiab] OR “cost utilities”[tiab] OR CUA[tiab] OR QALY[tiab] OR “quality adjusted life year”[tiab] OR “quality adjusted life years”[tiab] OR “time trade off”[tiab] OR “time tradeoff” [tiab] OR “timetrade off”[tiab] OR TTO[tiab] OR “standard gamble”[tiab] OR sf6d[tiab] OR “sf 6d”[tiab] OR “short form 6 dimension”[tiab] OR “short form 6 dimensions”[tiab] OR “short form 6”[tiab] OR AQoL[tiab] OR “assessment of quality of life”[tiab] OR HUI2[tiab] OR HUI3[tiab] OR QWB[tiab] OR QWB-SA[tiab] OR “quality of well-being”[tiab] OR 15D[tiab] OR “15 dimensional”[tiab] #4 #1 OR #2 #5 #3 AND #4 |
Filters |
Species: Human Language: English |
SCOPUS | |
Search terms |
Title-Abstract-Key Words: COPD OR COAD OR “chronic obstructive pulmonary disease” OR “chronic obstructive lung disease” OR “chronic lung disease” OR “chronic respiratory” OR “chronic obstructive airway disease” OR emphysema OR bronchitis AND Title-Abstract-Key Words: “health state” OR “health states” OR “health preference” OR “health preferences” OR “health utility” OR “health utilities” OR “utility index” OR “utilities index” OR “utility indices” OR “utility measure” OR “utility measures” OR “utility value” OR “utility values” OR “utility score” OR “utility scores” OR “utility outcome” OR “utility outcomes” OR “utility weight” OR “utility weights” OR “utility evaluation” OR “utility evaluations” OR HUI OR HSUV OR eq5d OR euroqol* OR “eq 5d” OR “euro qol” OR “euro qols” OR “utility analysis” OR “utility analyses” OR “cost utility” OR “cost utilities” OR CUA OR QALY OR “quality adjusted life year” OR “quality adjusted life years” OR “time trade off” OR “time tradeoff” OR “timetrade off” OR TTO OR “standard gamble” OR sf6d OR “sf 6d” OR “short form 6 dimension” OR “short form 6 dimensions” OR “short form 6” OR AQoL OR “assessment of quality of life” OR HUI2 OR HUI3 OR QWB OR QWB-SA OR “quality of well-being” OR 15D OR “15 dimensional” |
Filters | Language: English |
CINAHL | |
Search terms |
S1 (MH “Lung Diseases, Obstructive+”) S2 AB COPD OR COAD OR “chronic obstructive pulmonary disease” OR “chronic obstructive lung disease” OR “chronic lung disease” OR “chronic respiratory” OR “chronic obstructive airway disease” OR emphysema OR bronchitis S3 AB “health state” OR “health states” OR “health preference” OR “health preferences” OR “health utility” OR “health utilities” OR “utility index” OR “utilities index” OR “utility indices” OR “utility measure” OR “utility measures” OR “utility value” OR “utility values” OR “utility score” OR “utility scores” OR “utility outcome” OR “utility outcomes” OR “utility weight” OR “utility weights” OR “utility evaluation” OR “utility evaluations” OR HUI OR HSUV OR eq5d OR euroqol* OR “eq 5d” OR “euro qol” OR “euro qols” OR “utility analysis” OR “utility analyses” OR “cost utility” OR “cost utilities” OR CUA OR QALY OR “quality adjusted life year” OR “quality adjusted life years” OR “time trade off” OR “time tradeoff” OR “timetrade off” OR TTO OR “standard gamble” OR sf6d OR “sf 6d” OR “short form 6 dimension” OR “short form 6 dimensions” OR “short form 6” OR AQoL OR “assessment of quality of life” OR HUI2 OR HUI3 OR QWB OR QWB-SA OR “quality of well-being” OR 15D OR “15 dimensional” S4 S1 OR S2 S5 S3 AND S4 Note: AB = abstract, MH = Cinahl Heading |
Filters |
Language: English Species: Human |
Web of Science | |
Search terms |
Topic: COPD OR COAD OR “chronic obstructive pulmonary disease” OR “chronic obstructive lung disease” OR “chronic lung disease” OR “chronic respiratory” OR “chronic obstructive airway disease” OR emphysema OR bronchitis AND Topic: “health state” OR “health states” OR “health preference” OR “health preferences” OR “health utility” OR “health utilities” OR “utility index” OR “utilities index” OR “utility indices” OR “utility measure” OR “utility measures” OR “utility value” OR “utility values” OR “utility score” OR “utility scores” OR “utility outcome” OR “utility outcomes” OR “utility weight” OR “utility weights” OR “utility evaluation” OR “utility evaluations” OR HUI OR HSUV OR eq5d OR euroqol* OR “eq 5d” OR “euro qol” OR “euro qols” OR “utility analysis” OR “utility analyses” OR “cost utility” OR “cost utilities” OR CUA OR QALY OR “quality adjusted life year” OR “quality adjusted life years” OR “time trade off” OR “time tradeoff” OR “timetrade off” OR TTO OR “standard gamble” OR sf6d OR “sf 6d” OR “short form 6 dimension” OR “short form 6 dimensions” OR “short form 6” OR AQoL OR “assessment of quality of life” OR HUI2 OR HUI3 OR QWB OR QWB-SA OR “quality of well-being” OR 15D OR “15 dimensional” |
Filters |
Language: English Databases: Web of ScienceTM Core Collection (SCI-EXPANDED, SSCI, CPCI-S, CPCI-SSH, ESCI) |
The Cochrane Library | |
Search terms |
#1 MeSH descriptor: [Lung Diseases, Obstructive] explode all trees COPD OR COAD OR “chronic obstructive pulmonary disease” OR “chronic obstructive lung disease” OR “chronic lung disease” OR “chronic respiratory” OR “chronic obstructive airway disease” OR emphysema OR bronchitis :ti,ab,kw #3 “health state” OR “health states” OR “health preference” OR “health preferences” OR “health utility” OR “health utilities” OR “utility index” OR “utilities index” OR “utility indices” OR “utility measure” OR “utility measures” OR “utility value” OR “utility values” OR “utility score” OR “utility scores” OR “utility outcome” OR “utility outcomes” OR “utility weight” OR “utility weights” OR “utility evaluation” OR “utility evaluations” OR HUI OR HSUV OR eq5d OR euroqol* OR “eq 5d” OR “euro qol” OR “euro qols” OR “utility analysis” OR “utility analyses” OR “cost utility” OR “cost utilities” OR CUA OR QALY OR “quality adjusted life year” OR “quality adjusted life years” OR “time trade off” OR “time tradeoff” OR “timetrade off” OR TTO OR “standard gamble” OR sf6d OR “sf 6d” OR “short form 6 dimension” OR “short form 6 dimensions” OR “short form 6” OR AQoL OR “assessment of quality of life” OR HUI2 OR HUI3 OR QWB OR QWB-SA OR “quality of well-being” OR 15D OR “15 dimensional” :ti,ab,kw #4 #1 OR #2 #5 #4 AND #3 |
ProQuest Health Management | |
Search Terms |
Anywhere except full text: COPD OR COAD OR “chronic obstructive pulmonary disease” OR “chronic obstructive lung disease” OR “chronic lung disease” OR “chronic respiratory” OR “chronic obstructive airway disease” OR emphysema OR bronchitis AND Anywhere except full text: “health state” OR “health states” OR “health preference” OR “health preferences” OR “health utility” OR “health utilities” OR “utility index” OR “utilities index” OR “utility indices” OR “utility measure” OR “utility measures” OR “utility value” OR “utility values” OR “utility score” OR “utility scores” OR “utility outcome” OR “utility outcomes” OR “utility weight” OR “utility weights” OR “utility evaluation” OR “utility evaluations” OR HUI OR HSUV OR eq5d OR euroqol* OR “eq 5d” OR “euro qol” OR “euro qols” OR “utility analysis” OR “utility analyses” OR “cost utility” OR “cost utilities” OR CUA OR QALY OR “quality adjusted life year” OR “quality adjusted life years” OR “time trade off” OR “time tradeoff” OR “timetrade off” OR TTO OR “standard gamble” OR sf6d OR “sf 6d” OR “short form 6 dimension” OR “short form 6 dimensions” OR “short form 6” OR AQoL OR “assessment of quality of life” OR HUI2 OR HUI3 OR QWB OR QWB-SA OR “quality of well-being” OR 15D OR “15 dimensional” |
Filters |
Language: English Databases: ProQuest Central, Health & Medical Collection, Nursing & Allied Health Database, Public Health Database, Health Management Database, Family Health Database, Research Library, Research Library: Health & Medicine |
Appendix 14 Additional tables for objective IV
Author | Country | Population | Methods | Instrument | HSUV |
---|---|---|---|---|---|
Ding et al.182 | China |
ACOS = 350 Asthma only = 822 COPD only = 1598 Matched control = 2770 Mean age, years (SD)Sex, male (%)COPD (%) (n = 1602)ACOS (%) (n = 366) |
Cross-sectional cohort study. China National Health and Wellness Survey data (self-administered online survey). Data from 2010, 2012 and 2013. Participants recruited using online and offline methods Control = no diagnosis of asthma, COPD, chronic bronchitis or emphysema COPD only = COPD, chronic bronchitis or emphysema |
SF-36v2, SF-12v2 (SF-6D algorithm applied to get utility values) |
Adjusted mean (SE) ED visits in the past 6 months, adjusted mean (SE) Hospitalisations in the past 6 months, adjusted mean (SE) |
Miravitlles et al.220 | Spain |
3125 patients COPD (1974 primary care, 1151 pneumology departments) ACOS (n = 496)Non-exacerbators (n = 1894)Exacerbators with chronic bronchitis (n = 602)Excerbators without chronic bronchitis (n = 133) |
Transversal observational study; 875 primary care physicians, 258 pneumologists recruited 3/4 patients each | EQ-5D (Spanish tariff) | Unadjusted mean (95% CI)
|
Author | Country | Population | Methods | Instrument | HSUV |
---|---|---|---|---|---|
Kim and Kim194,219 | South Korea |
COPD patients 556 male195 female |
Data from KNHANES | EQ-5D (Korean tariff) | Male, mean (SE)
|
Fletcher et al.262 | Brazil, China, Germany, Turkey, USA, UK |
2426 participants Age (years)MRC dyspnoea scale severity |
Cross-sectional study, participants recruited by a contract research organisation and data collected in interviews that included the EQ-5D | EQ-5D (German, UK and USA tariff) | Age (years), mean (SD)
|
Punekar et al.279 | USA, France, Germany, Italy, Spain, UK | 2703 patients
|
Cross-sectional survey; physicians recruited and recorded information for six patients during consultations | EQ-5D (UK tariff) | Patient seen by PCP (n = 1381) age, mean (95% CI)
|
Hazell et al.301 | UK |
10,471 patients (aged ≥ 16 years)Mean age, years (SD) = 48.5 (19.4) Sex, male (%) = 45.1 (1054 with likely OAD) |
Observational study; data gained from postal questionnaire that includes EQ-5D. Recruitment via two general practices | EQ-5D (UK tariff) |
Likely OAD, mean = 0.63 Female, mean (SD) |
Author | Country | Population | Methods | Instrument | HSUV |
---|---|---|---|---|---|
Hoyle et al.191 | UK | COPD patients
|
Data from two Phase III, longitudinal, randomised controlled trials. All patients randomised to treatment. EQ-5D conducted at baseline and two follow-up visits | EQ-5D (UK tariff) | Baseline of trial, mean (SD)
|
GOLD stage
|
|||||
Kim and Kim194,219 | South Korea | COPD patients | Data from KNHANES | EQ-5D (Korean tariff) | Sex, mean (SE)
|
556 male
|
|||||
195 female
|
|||||
Sundh et al.225 | Sweden | 373 COPD patients
|
27 hospital-based secondary care respiratory units enrolled ≈10 patients each. EQ-5D completed by patient during visit or later at home | EQ-5D (UK tariff) | Sex, mean (SD)
|
Kim et al.236 | South Korea | 200 COPD patients (GOLD stages 1–4); age, years
|
Interview, including EQ-5D. Recruitment by respiratory specialists via outpatient departments | EQ-5D (Korean tariff) | Sex, mean (SE)
|
Fletcher et al.262 | Brazil, China, Germany, Turkey, USA, UK |
2426 patients Age, yearsMRC dyspnoea scale severity |
Cross-sectional study. Data gained from interviews with participants (including EQ-5D). Recruited by a contract research organisation. Participants recruited to represent wide range of demographics and disease severity | EQ-5D (Germany, UK and USA tariff) | Sex, mean (SE)
|
Punekar et al.279 | USA, France, Germany, Italy, Spain, UK | 2703 patients
|
Cross-sectional survey. Physicians recruited and recorded information for six patients during consultations | EQ-5D (UK tariff) | Sex (seen by PCP), mean (95% CI)
|
Mean age, years (SD)
|
Author | Country | Population | Methods | Instrument | HSUV |
---|---|---|---|---|---|
Miravitlles et al.206 | Spain |
346 COPD patients Mean age, years (SD) = 67.9 (9.7) Sex, male (%) = 85.5 |
Multicentre observational cross-sectional study; 15 hospitals. Patients recruited at outpatient clinic. All patients in stable condition | EQ-5D (Spanish tariff) | Exacerbations in previous year, median (IQR)
|
Punekar et al.279 | USA, France, Germany, Italy, Spain, UK | 2703 patients
|
Cross-sectional survey. Physicians recruited and recorded information for six patients during consultations | EQ-5D (UK tariff) | Exacerbations (seen by PCP), mean (95% CI)
|
Author | Country | Population | Methods | Instrument | HSUV |
---|---|---|---|---|---|
Martinez Rivera et al.202 | Spain | 115 COPD patients
|
Multicentre observational cross-sectional study; 13 hospitals. Data also used in Alcazar et al.204 and García-Polo et al.205 | EQ-5D (Spanish tariff) | Mean (SD)
|
Hong et al.194,218 | South Korea |
1178 COPD patientsGOLD stage 1 (mild) COPD (n = 497)GOLD stage 2 (moderate) COPD (n = 612) GOLD stage 3 (severe) or 4 (very severe) COPD (n = 69) 1178 control subjects |
Data from KNHANES | EQ-5D (Korean) | Mean (SD)
|
Miravitlles et al.221 | Spain | 346 COPD patients
|
Multicentre observational cross-sectional study; 15 hospitals. Patients recruited at outpatient clinic. All patients in stable condition | EQ-5D (Spanish tariff) | Median (IQR)
|
Miravitlles et al.206 | Spain | 713 COPD patients
|
DEPREPOC – a multicentre observational cross-sectional study | EQ-5D (Spanish tariff) | Mean (SD)
|
Author | Country | Population | Methods | Instrument | HSUV |
---|---|---|---|---|---|
Lee et al.194,201 | South Korea | Non-smoker (n = 399)
|
Data from KNHANES | EQ-5D (Korean tariff) | Mean (SD)
|
Miravitlles et al.221 | Spain | 346 COPD patients
|
Multicentre, observational, cross-sectional study, 15 hospitals. Patients recruited at outpatient clinic. All patients in stable condition | EQ-5D (Spanish tariff) | Median (IQR)
|
Mullen et al.242 | Canada |
956 COPD Smokers = smoked daily in the last 6 months Mean age, years (SD) |
Smokers hospitalised for one of four selected diagnoses. Intervention: OMSC intervention. Comparator: usual care. Utility scores calculated from Sullivan et al.310 and Tillman et al.311 | EQ-5D (USA tariff) |
Former smoker = 0.52 Current smoker = 0.50 |
Author | Country | Population | Methods | Instrument | HSUV |
---|---|---|---|---|---|
Miravitlles et al.221 | Spain | 346 COPD patients
|
Multicentre observational cross-sectional study; 15 hospitals. Patients recruited at outpatient clinic. All patients in stable condition | EQ-5D (Spanish tariff) | BMI, median (IQR)
|
García-Rio et al.232 | Spain |
3797 COPD patients, aged 40–80 years Normal weightOverweightObese |
EPI-SCAN study – multicentre cross-sectional population-based study | EQ-5D (Spanish tariff) | BMI, mean (SD)
|
Author | Country | Population | Methods | Instrument | HSUV |
---|---|---|---|---|---|
Kim and Kim194,219 | South Korea |
COPD patients 556 male195 female |
Data from KNHANES | EQ-5D (Korean tariff) | Mean (SE)
|
Miravitlles et al.271 | Spain | 4574 COPD patients
|
Patients recruited from GPs and respiratory specialists | EQ-5D (Spanish tariff) | Socioeconomic status – unadjusted
|
Appendix 15 Preferred Reporting Items for Systematic Reviews and Meta-Analyses checklist
Section/topic | Number | Checklist item | Reported on page number |
---|---|---|---|
Title | |||
Title | 1 | Identify the report as a systematic review, meta-analysis or both | The report was identified as a structured narrative literature review, which followed systematic procedures and, where possible, adhered to the PRIMSA checklist (see Appendix 12, page 141) |
Abstract | |||
Structured summary | 2 | Provide a structured summary including, as applicable: background; objectives; data sources; study eligibility criteria, participants and interventions; study appraisal and synthesis methods; results; limitations; conclusions and implications of key findings; systematic review registration number | The literature review was conducted as part of the economic evaluation section (see Chapter 5) of the report and thus inclusion of an abstract was not applicable |
Introduction | |||
Rationale | 3 | Describe the rationale for the review in the context of what is already known | The rationale for the review was included as part of the methodology of the report on Chapter 5, page 61 |
Objectives | 4 | Provide an explicit statement of questions being addressed with reference to participants, interventions, comparisons, outcomes, and study design (PICOS) | Four objectives for the review were stated (see Appendix 12, page 141) |
Methods | |||
Protocol and registration | 5 | Indicate if a review protocol exists, if and where it can be accessed (e.g. web address) and, if available, provide registration information including registration number | The review protocol is described in Appendix 12. No formal registration was made |
Eligibility criteria | 6 | Specify study characteristics (e.g. PICOS, length of follow-up) and report characteristics (e.g. years considered, language, publication status) used as criteria for eligibility, giving rationale | The review was designed using a two-stage process. Eligibility criteria were developed for each stage based around the PICOS format (when relevant) and included language and year of publication (see Tables 24 and 25, pages 142 and 143) |
Information sources | 7 | Describe all information sources (e.g. databases with dates of coverage, contact with study authors to identify additional studies) in the search and date last searched | All information sources were described in Appendix 12 (page 142) and databases, with access dates were included in Appendix 13 |
Search | 8 | Present full electronic search strategy for at least one database, including any limits used, such that it could be repeated | Full electronic search strategies are presented in Appendix 12 (page 143) and all individual database searches are included in Appendix 13 |
Study selection | 9 | State the process for selecting studies (i.e. screening, eligibility, included in systematic review, and, if applicable, included in the meta-analysis) | The process of study selection, including use of inclusion and exclusion criteria, was described in Appendix 12, pages 144 and 148 |
Data collection process | 10 | Describe method of data extraction from reports (e.g. piloted forms, independently, in duplicate) and any processes for obtaining and confirming data from investigators | The process of data extraction, including how duplicate information was dealt with, was described in Appendix 12, page 148 |
Data items | 11 | List and define all variables for which data were sought (e.g. PICOS, funding sources) and any assumptions and simplifications made | The data items extracted were listed in Appendix 12, page 148 |
Risk of bias in individual studies | 12 | Describe methods used for assessing risk of bias of individual studies (including specification of whether this was done at the study or outcome level) and how this information is to be used in any data synthesis | Not applicable (risk of bias was not formally assessed, although potential bias was considered when selecting studies for the economic evaluation) |
Summary measures | 13 | State the principal summary measures (e.g. risk ratio, difference in means) | The principal summary measures were differences in HSUVs |
Synthesis of results | 14 | Describe the methods of handling data and combining results of studies, if done, including measures of consistency (e.g. I2) for each meta-analysis | The studies were used individually for the economic evaluation, except in one case in which a weighted average of the results were used (see Chapter 5, pages 63–5) |
Risk of bias across studies | 15 | Specify any assessment of risk of bias that may affect the cumulative evidence (e.g. publication bias, selective reporting within studies) | Not applicable (risk of bias was not formally assessed, although potential bias was considered when selecting studies for the economic evaluation) |
Additional analyses | 16 | Describe methods of additional analyses (e.g. sensitivity or subgroup analyses, meta-regression), if done, indicating which were prespecified | Not applicable (no additional analysis was undertaken for this review as it was deemed outside the scope of the project) |
Results | |||
Study selection | 17 | Give numbers of studies screened, assessed for eligibility, and included in the review, with reasons for exclusions at each stage, ideally with a flow diagram | The study selection process was presented in a PRIMSA flow diagram (see Appendix 12, Figure 29, page 144) |
Study characteristics | 18 | For each study, present characteristics for which data were extracted (e.g. study size, PICOS, follow-up period) and provide the citations | Study characteristics for all selected studies were presented in Table 27–29 (see Appendix 12, pages 149–53) |
Risk of bias within studies | 19 | Present data on risk of bias of each study and, if available, any outcome level assessment (see item 12) | Not applicable (risk of bias was not formally assessed, although potential bias was considered when selecting studies for the economic evaluation) |
Results of individual studies | 20 | For all outcomes considered (benefits or harms), present, for each study: (a) simple summary data for each intervention group (b) effect estimates and confidence intervals, ideally with a forest plot | For all selected studies, HSUVs (means and SDs) were presented in Tables 27–29 (see Appendix 12, pages 149–53) |
Synthesis of results | 21 | Present results of each meta-analysis done, including confidence intervals and measures of consistency | Not applicable (meta-analysis was not included as part of the data synthesis, instead results were presented as a narrative summary) |
Risk of bias across studies | 22 | Present results of any assessment of risk of bias across studies (see item 15) | Not applicable (risk of bias was not formally assessed, although potential bias was considered when selecting studies for the economic evaluation) |
Additional analysis | 23 | Give results of additional analyses, if done [e.g. sensitivity or subgroup analyses, meta-regression (see item 16)] | Not applicable (no additional analyses were conducted) |
Discussion | |||
Summary of evidence | 24 | Summarise the main findings including the strength of evidence for each main outcome; consider their relevance to key groups (e.g. health-care providers, users, and policy-makers) | Brief summaries of the results in relation to each objective are provided in Appendix 12, pages 148, 149, 154 and 155 |
Limitations | 25 | Discuss limitations at study and outcome level (e.g. risk of bias), and at review-level (e.g. incomplete retrieval of identified research, reporting bias) | The purpose of the review was to identify studies to inform the economic evaluation; therefore, it was not written as a full review, so a section on limitations was not applicable. However, risk of bias and limitations of individual studies were considered when selecting studies for the economic evaluation |
Conclusions | 26 | Provide a general interpretation of the results in the context of other evidence, and implications for future research | The review was designed specifically to identify papers to support the economic evaluation; therefore, this section was not applicable |
Funding | |||
Funding | 27 | Describe sources of funding for the systematic review and other support (e.g. supply of data); role of funders for the systematic review | The literature review was conducted as part of the economic evaluation section (see Chapter 5) of the report. Funding details can be found on pages vi and xxvii |
Glossary
- Arbed
- The area-based, supply-led part of the Welsh Government Warm Homes programme. The scheme took place in two separate phases between 2010 and 2015 and aimed to improve the energy efficiency of existing homes in low-income areas. Arbed means ‘save’ in Welsh.
- Nest
- The demand-led part of the Welsh Government Warm Homes programme. The scheme was established in 2011 and offers free advice and support to low-income households with energy-efficient homes. Free home energy efficiency improvements are offered to eligible households.
List of abbreviations
- A&E
- accident and emergency
- ACOS
- asthma–COPD overlap syndrome
- ALF
- Anonymous (Individual) Linking Field
- ANOVA
- analysis of variance
- AQoL
- Assessment of Quality of Life
- BISF
- British Iron and Steel Federation
- BMI
- body mass index
- CBA
- cost–benefit analysis
- CCA
- cost–consequences analysis
- CEA
- cost-effectiveness analysis
- CEAC
- cost-effectiveness acceptability curve
- CEP
- cost-effectiveness plane
- CI
- confidence interval
- CINAHL
- Cumulative Index to Nursing and Allied Health Literature
- COPD
- chronic obstructive pulmonary disease
- CUA
- cost–utility analysis
- EQ-5D
- EuroQol-5 Dimensions
- ERDF
- European Regional Development Fund
- FEV
- forced expiratory volume
- GOLD
- Global Initiative for Chronic Obstructive Lung Disease
- GP
- general practitioner
- HDD
- heating degree day
- HSUV
- health state utility value
- HUI
- Health Utilities Index
- ICD-10
- International Classification of Diseases, Tenth Edition
- ICER
- incremental cost-effectiveness ratio
- IGRP
- Information Governance Review Panel
- LA
- local authority
- LSOA
- lower super output area
- MCS
- Mental Health Composite Scale
- NICE
- National Institute for Health and Care Excellence
- NWIS
- NHS Wales Informatics Service
- ONS
- Office for National Statistics
- OR
- odds ratio
- PCS
- Physical Health Composite Scale
- PEDW
- Patient Episode Database for Wales
- PRISMA
- Preferred Reporting Items for Systematic Reviews and Meta-Analyses
- PSA
- probabilistic sensitivity analysis
- QALY
- quality-adjusted life-year
- QWB
- Quality of Well-Being
- RALF
- Residential Anonymous Linking Field
- RH
- relative humidity
- RSL
- registered social landlord
- SAIL
- Secure Anonymised Information Linkage
- SD
- standard deviation
- SE
- standard error
- SF-6D
- Short Form questionnaire-6 Dimensions
- SF-12
- Short Form questionnaire-12 items
- SF-36
- Short Form questionnaire-36 items
- SREC
- School Research Ethics Committee
- SSC
- study steering committee
- STROBE
- Strengthening the Reporting Observational Studies in Epidemiology
- WDS
- Welsh Demographic Service
- WHO
- World Health Organization
- WIMD
- Welsh Index of Multiple Deprivation