Notes
Article history
The research reported in this issue of the journal was funded by the HS&DR programme or one of its preceding programmes as project number 12/128/41. The contractual start date was in January 2014. The final report began editorial review in June 2019 and was accepted for publication in February 2020. The authors have been wholly responsible for all data collection, analysis and interpretation, and for writing up their work. The HS&DR editors and production house have tried to ensure the accuracy of the authors’ report and would like to thank the reviewers for their constructive comments on the final report document. However, they do not accept liability for damages or losses arising from material published in this report.
Declared competing interests of authors
Rob Simister is lead consultant of the hyperacute stroke unit at the University College London Hospitals NHS Foundation Trust. Rob Simister is now Joint Clinical Director of the London Stroke Clinical Network and is part funded by the National Institute for Health Research (NIHR) Biomedical Research Centre at University College London Hospitals NHS Foundation Trust and University College London. Angus IG Ramsay was an associate board member of the National Institute for Health Research (NIHR) Health Services and Delivery Research (HSDR) Board (2015–18). Anthony Rudd was the National Clinical Director for Stroke in England from 2010 to 2019 and was the London Stroke Clinical Director from 2009 to 2019. Steve Morris was on the NIHR HSDR Funding Committee (2014–19). Naomi Fulop was a member of the NIHR HSDR Funding Committee (2013–18) and is a NIHR Senior Investigator.
Permissions
Copyright statement
© Queen’s Printer and Controller of HMSO 2020. This work was produced by Simister et al. under the terms of a commissioning contract issued by the Secretary of State for Health and Social Care. This issue may be freely reproduced for the purposes of private research and study and extracts (or indeed, the full report) may be included in professional journals provided that suitable acknowledgement is made and the reproduction is not associated with any form of advertising. Applications for commercial reproduction should be addressed to: NIHR Journals Library, National Institute for Health Research, Evaluation, Trials and Studies Coordinating Centre, Alpha House, University of Southampton Science Park, Southampton SO16 7NS, UK.
2020 Queen’s Printer and Controller of HMSO
Chapter 1 Context
Context and rationale for the research
The ‘weekend effect’ and 7-day working policies
In 2001, Bell and Redelmeier1 published a landmark study showing that mortality was higher among people admitted to hospital during weekends or ‘out of hours’ than among those admitted during normal hours on weekdays, based on the health records of 3.8 million Canadian patients. This effect has been repeatedly demonstrated across a range of acute conditions, patient populations and health systems,1–3 such as myocardial infarction4 and stroke,5–9 patients undergoing elective surgery10 and admissions to emergency departments (EDs)11 or intensive care units. 12 However, a number of studies report equivalent or better outcomes in some patients treated outside normal working hours. 10,12–14 Although contested, such studies indicate that high-quality out-of-hours care is possible and that changes in organisation of services might address the current performance gap.
The Secretary of State for Health made this issue a priority in 2015 by suggesting that ‘someone is 15% more likely to die if admitted on a Sunday than on a Wednesday because we do not have as many doctors in our hospitals at the weekends as we have mid-week’ (House of Commons, 2015. Contains Parliamentary information licensed under the Open Parliament Licence v3.0. See https://www.parliament.uk/site-information/copyright-parliament/open-parliament-licence/). 15 This led to controversial changes to doctors’ work contracts, provoking large-scale strikes16 and an NHS England policy for a 7-day NHS by 2020. 17 The NHS Long Term Plan18 has identified, in particular, that all stroke units will, over the next 5 years, meet the NHS 7-day standards for stroke care and the national clinical guidelines for stroke over the next 5 years.
Several possible causes for an ‘out-of-hours effect’ are proposed, but many relevant factors are likely to be associated with out-of-hours working, including differences in patient case mix affecting outcomes among patients admitted at different times of the week;19 reduced staffing levels;2,11,20 reduced access to supporting investigations;2,11 lower seniority and clinical experience of available staff;1,2 less familiarity of staff with patients;1 less familiarity with unit-specific processes and policies, and insufficient training to address this;2 and fewer supervisory staff and less supervisor familiarity with the staff they supervise. 1 Systems by which such issues may be identified and addressed, such as clinical governance, and cultures that support staff engagement in these systems, may play an important role in how the out-of-hours effect manifests. Ward and organisational leadership may also represent significant factors in supporting service development, learning and engagement. 21
The controversy raised a number of relevant issues for research: first, that exposure of differences in outcomes across the week was having an impact politically, and that it was necessary to answer the question of whether this was a true effect, or an artefact of the patient mix or the quality of the data; second, that it was important to measure other outcomes and care processes, as well as mortality; and, finally, to change the approach towards acknowledging patterns of temporal variation. 22–25 This movement is characterised by the idea that certain times of the day, or days of the week, are associated with poorer outcomes for the patient, but that this is not necessarily limited to the weekend or night-time. 22 As a result, the concept of ‘out-of-hours’ working or the so-called ‘weekend effect’, which once dominated the discourse of the literature on temporal variation,22,26–28 has been re-evaluated.
It is now recognised that temporal variation results, at least in part, from delivery models of care and the cultural values of the health-care sector, enshrined in everyday practice, funding models, staffing practices and wider policies. 29 Recent research, such as the High-Intensity Specialist-Led Acute Care study,30,31 have taken this approach to examine the intensity of specialist-led care in emergency medical admissions, focusing particularly on the organisation and delivery of care, and medical staffing. 30,31
In the case of urgent stroke care, recent studies have shown that temporal variation in mortality is now decreasing,32,33 including in London’s hyperacute stroke unit (HASU) model. 34 However, there has been no large-scale, organisational research to understand what creates temporal in consistency, particularly in services that aim to operate consistently over 7 days, such as the London stroke services. The current study adds a wealth of information to this gap.
Reconfiguration of stroke services and the aspiration of 7-day working
In 2010, acute stroke services in London (population 8.2 million) were centralised. Hospitals were selected to become sites for specialist stroke care (over the first hours following stroke) in a hub-and-spoke network. 35 In London, prior to the reorganisation, 30 hospitals provided acute stroke care. After the reorganisation, initial specialist stroke care was routinely provided to all patients with suspected acute stroke at eight designated HASUs, with all patients being assessed shortly after arrival at the designated hospital by specialised stroke assessment teams able to provide access to immediate brain imaging in all patients and delivering immediate intravenous thrombolysis, when appropriate (Figure 1). The HASU model involved the London Ambulance Service taking all patients with suspected stroke within 48 hours of onset to one of the eight HASUs. 36 Acute stroke patients seen at other medical facilities were similarly transferred as an emergency to a HASU. The aim of the HASUs was to provide specialised care for all acute stroke patients during the first 72 hours after onset of stroke. Twenty-four acute stroke units in London, linked to HASUs, were designated to provide specialist acute stroke care beyond 72 hours for those patients still requiring inpatient treatment. Eight of these were in the same hospital trust as a HASU. 37 On average, across all patients, the quality of acute stroke care in London was better than in the rest of England and increased as a result of the centralisation,38 with a decrease in mortality. 35
FIGURE 1.
Summary of London’s stroke service model before and after reconfiguration. A&E, accident and emergency; ASU, acute stroke unit; MAU, medical admission unit; SU, stroke unit.
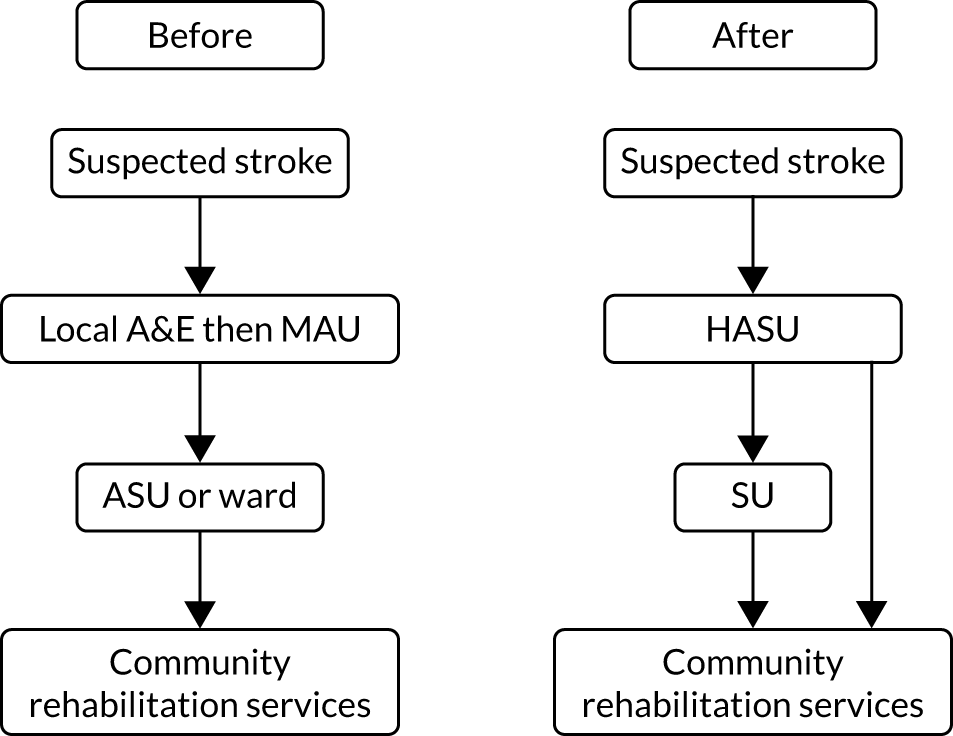
Performance standards for HASUs, linked to payments, were initially set by Healthcare for London39,40 and subsequently the London Strategic Clinical Networks to maintain high quality of care across the HASU stay. Standards include staffing quotas, required infrastructure and delivery of care. Some standards were set to provide rapid access to time-critical ‘front-door’ measures [e.g. dysphagia screen within 4 hours of admission, computerised tomography (CT) scan within 1 hour of admission and thrombolysis administration within 60 minutes]. Other standards were set with less stringent time constraints (e.g. stroke specialist consultant physician assessment within 24 hours and physiotherapist assessment within 72 hours). An evaluation of London’s stroke centralisation found that mortality and length of stay (LOS) were reduced, if all stroke patients receive hyperacute care. 35 It has also been shown that these changes are sustainable over time. 41
The London model has been shown to improve clinical outcomes and care provision,35,37,38 and is cost-effective. 42 Beyond London, many regions in the NHS are currently seeking to centralise stroke service organisation in different ways. 43,44 The development of mechanical thrombectomy (surgical removal of a blood clot from a blood vessel) services is an important new development for a subset of stroke patients. As of May 2020, further reorganisation of the London model is currently in progress with the designation of a subset of HASUs that can provide thrombectomy. At the time of our study (2014–15), no HASU was providing a formal thrombectomy service for stroke associated with large vessel occlusion. Therefore, 24/7 availability of scans and assessments by specialists will be vital to enable the correct patients to be transferred to a thrombectomy centre.
Aims and research objectives
Research aims
This study aimed to identify the extent to which London stroke services were providing care 24 hours a day, 7 days a week (24/7), and, if they were, how this was being achieved. The research provides an opportunity to examine how and if a range of organisational factors influence 24/7 provision of stroke care, and to assess the extent to which temporal patterns of provision are reflected in patient outcomes, including mortality, LOS and functional independence. The research also aimed to explore service developments related to temporal consistency. These lessons are of potential value to people who commission, organise and run stroke services, both in London and across any area of the English NHS that seeks to develop or provide 24/7 hyperacute stroke care. They may also have relevance to research in, and provision of, 24/7 care in other health-care settings, such as emergency and intensive care, treatment of myocardial infarction and elective surgery.
Research objectives
Objective 1
To investigate the extent to which evidence-based care received by stroke patients differs between in-hours and out-of-hours services in London HASUs.
Objective 2
To investigate the extent to which clinical outcomes differ between in-hours and out-of-hours services in the London HASUs.
Objective 3
To investigate how in-hours and out-of-hours services are organised and provided in London HASUs.
Objective 4
To investigate how these services are perceived by stakeholders (including stroke clinicians, patients and carers).
Objective 5
To investigate the costs of acute stroke care in London HASUs on different days and at different times of admission.
Overview of the research project
This project was funded by the National Institute for Health Research Health Services and Delivery Research programme, from January 2014, to study the development, provision, effects and cost of 24/7 stroke care in the London HASU system as part of an open call. The project was extended from its original end date of December 2016 to April 2019 due to delays and the eventual inability to obtain the Sentinel Stroke National Audit Programme (SSNAP) data linked to mortality data from the Office for National Statistics (ONS). The reason for this delay was difficulties in formalising data-sharing agreements between the Health and Social Care Information Centre (now NHS Digital, data controller for ONS data) and Healthcare Quality Improvement Partnership (data controller for SSNAP data). It was agreed with the funder that we would publish the results based on the SSNAP data we did have, rather than continue to delay the publication of the study. At an early stage of our investigation, we recognised the pivotal roles undertaken by the HASU lead nurses. Understanding these activities and their impact on the functioning of the HASUs required the additional capture of the nursing rotas for each HASU for the period of the study. Our analysis of the impact of nursing numbers and seniority in relationship to temporal variation and clinical outcomes is presented here (see Chapters 3 and 5), and strongly supports our initial observations on the central importance of nurse-led activities to our work. This enabled us to undertake new and originally unplanned analyses to look at the impact of nursing numbers and seniority (see Chapter 5) in relation to temporal variation and clinical outcomes (see Chapter 3).
Structure of the report
The report is structured as follows.
See Chapter 2 for:
-
an overview of the study sites
-
data and analysis methods.
(Detailed methods are provided within each findings chapter.)
See Chapter 3 for:
-
costs of acute stroke care in London HASUs on different days and at different times of admission.
See Chapter 4 for factors that influence, temporally:
-
consistent care
-
inconsistent care.
See Chapter 5 for:
-
nursing roles on the HASU
-
temporal variation in nursing provision
-
associated processes and outcomes.
See Chapter 6 for:
-
temporal structures acting on the HASU patient pathway
-
how this can have an impact on measurement of temporal variation.
See Chapter 7 for:
-
discussion and summary of our findings in relation to our research questions
-
implications for health services, research and 7-day working policies.
Changes to the protocol
We made some changes to the protocol because of difficulties in obtaining access to all our data sources, especially mortality data, as noted in Overview of the research project. Initially, we experienced difficulties gaining access to the HASUs, and later on it proved difficult to recruit patients and carers to our interviews. This is partly because of the long delay periods between recruitment on the wards and patients returning to their homes or care facilities when they would be ready to complete the interview. In addition, there was a significant dropout rate between patients expressing interest in taking part and completing interviews: 80 patients expressed initial interest, but either declined later on or would not respond to communications. Patient recruitment improved with registration of the study on the Clinical Research Network portfolio, which allowed access to the Clinical Research Network research practitioner teams in each HASU and a more systematic approach to patient identification. This released our team to discuss the study with patients who had already expressed interest in taking part.
Lack of access to mortality data or long-term dependency data
The most substantial change made to our protocol is in the absence of 3-month mortality and 6-month modified Rankin Scale (mRS) data. The basis of our data set is the SSNAP. The SSNAP is one of several ongoing mandatory national clinical audits and has contributed significantly to the debates around quality of stroke care at the weekends, by providing a detailed data set to several studies. We had hoped to link this data set to Hospital Episode Statistics and ONS data on mortality after discharge. Similar analyses have been achieved by other research teams (e.g. Bray et al. 22). We estimate that we waited for these linked data for 24 months before we decided to drop these analyses from our study. The delay was due to an ongoing disagreement between NHS Digital and Healthcare Quality Improvement Partnership about formalising data-sharing arrangements for our study and similar studies. Follow-up data after discharge from HASU or hospital were collected in the SSNAP for a very small number of patients and it was not feasible to run this analysis.
Without these data our final outputs are weaker, but still important. We are still taking a unique approach to this problem by including within-hospital mortality data, mRS data and nursing rota data, by looking at patterns of temporal variation, rather than binary weekday or weekend patterns, and by incorporating interview and observational data.
Lack of access to carotid surgery data
The consultations about carotid surgery data were made with National Vascular Registry managers. According to the information provided, there was a lag of approximately 18 months between recording of carotid surgery data and their release (as of the end of January 2015 the carotid surgery data were available for linkage up to September 2013). In addition, the SSNAP data requested did not contain patient identifiers and it was not possible to assure the quality of linkage between the SSNAP and the National Vascular Registry data (Table 1).
In the protocol | What could not be achieved within the term of the grant | Additional content |
---|---|---|
Sampling | ||
Covering the period 1 January 2014 to 31 December 2014:
|
|
|
Analysis | ||
We regress our primary and secondary outcomes against different combinations of a series of covariates of interest Primary outcomes:Secondary outcomes: |
We were not able to obtain outcome data on:
|
Additional outcomes:
|
Independent variables | ||
|
Additional independent variables:
|
|
Economic element | ||
The cost components will include:
|
Imaging interventions: Surgical and neuroradiological interventions:Post-discharge care: |
|
Chapter 2 Research methods
Overview
In this chapter we provide an overview of our study design, and we outline the quantitative and qualitative data and methods used (note that detailed methods are provided in Chapters 3–6).
Study design
This study used a mixed-methods design, with findings from planned qualitative and quantitative analyses informing further investigation and analysis throughout the study (see Chapter 1, Structure of the report and Figure 2). 45 The case study method permits development and testing of theories on how efforts to bring about change interact with the context in which they take place. A multiple case study approach, in this case, in which each HASU is a case study, allows the development and testing of theories in several organisational contexts. 46–48 Our large qualitative data set was derived from interviews with HASU staff and patients, and observations on the ward and elsewhere in the eight hospitals. After gaining access to the SSNAP data, we looked at temporal variation (objectives 1 and 2) in the processes and clinical outcomes of HASU care for a 1-year period (from 1 January 2014 to 31 December 2014) (see Chapter 3). Chapter 4 is an analysis of the reasons for the patterns of variation found in our SSNAP data set, derived from dialogues between the quantitative and the qualitative research teams in the study (objective 3). Our third study was informed, in part, by qualitative data, with interviews and observations suggesting that nursing seniority and variation in providing key roles (such as nurse in charge) was perceived to be more important than the number of nurses on the ward (objectives 1–3). Therefore, we examined how nursing roles were provided through different time periods, and the impact on clinical outcomes and processes (see Chapter 5). Chapter 6 reflects the development of our conceptual thinking as a mixed-methods team throughout this project (objectives 3 and 4). We have recognised the limitations of using the SSNAP to assess temporal variation, leading to a qualitative analysis of the forces or structures driving temporal variation, and how this affects measurement of variation in stroke research. Our methods are reported in accordance with the COnsolidated criteria for REporting Qualitative studies (COREQ) (see Appendix 1) and STrengthening the Reporting of OBservational studies in Epidemiology (STROBE) reporting guidelines. 49
FIGURE 2.
Interlinkages of the mixed-methods analysis, including deviation from the protocol.
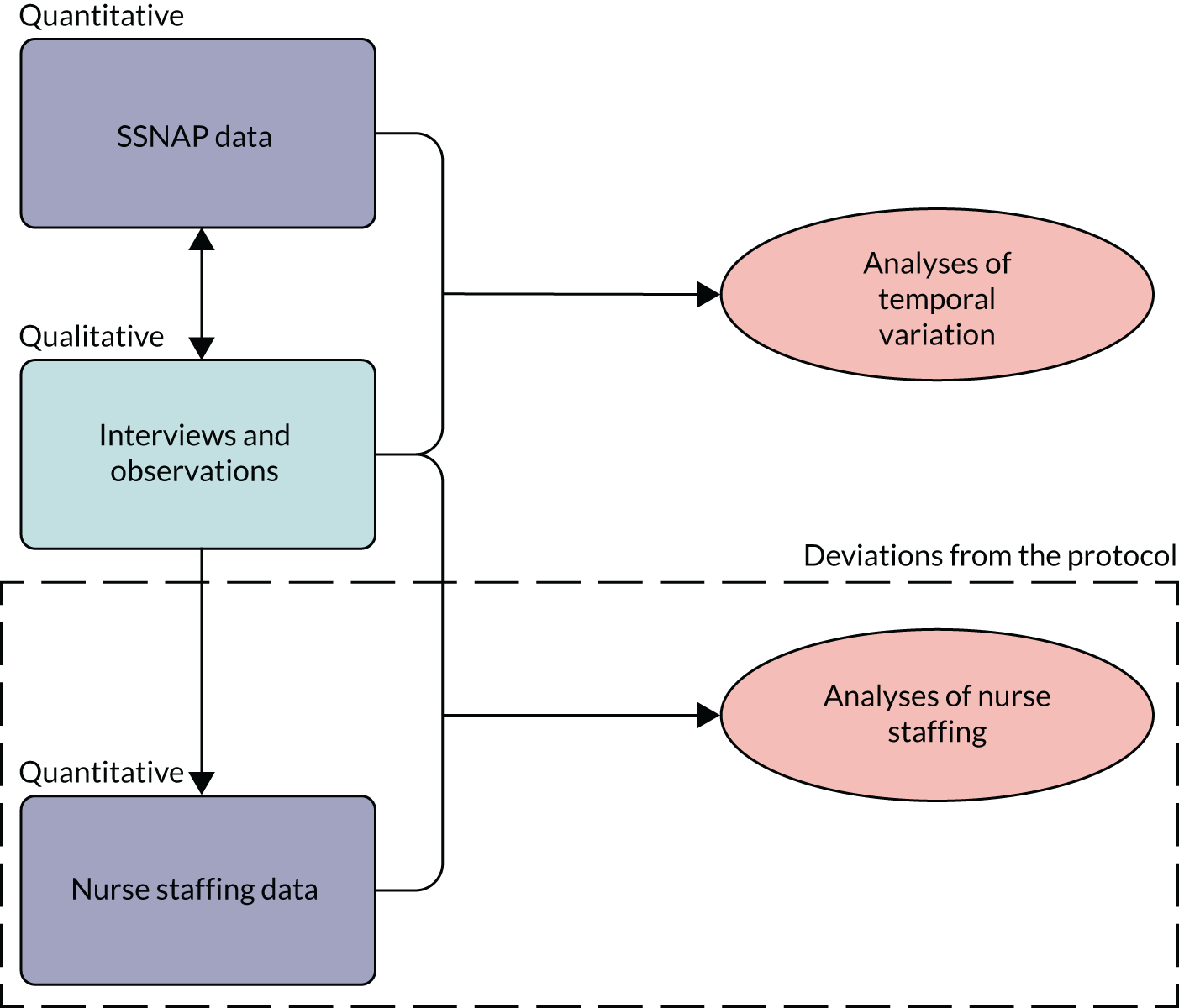
Perspective
We approached this research with a commitment to the study of the HASU system from both a quantitative and a qualitative perspective. Although not an ethnographic study, we adopted some principles of multisited ethnography, such as following people and objects through systems. 50 For example, we based some of our observations around the journey being followed by a stroke patient all the way from the emergency room, through scans and tests, and up to the HASU ward itself. We followed members of staff, such as consultants on their ward rounds and nurses around the hospital. We also focused on relative cultural meanings, such as what 24/7 means from a variety of professional and patient perspectives. We committed to be reflexive and iterative in our approach to data collection, regularly meeting to debrief and make decisions about future plans for data collection, and quantitative and qualitative analysis. 51 Finally, we sought to understand both our qualitative and our quantitative data through our immersion in the services. This led to new additional quantitative analyses being undertaken, such as our analysis of HASU nursing data.
Setting
Our study was set in all eight of the London HASUs. These are dedicated wards, often sited in close proximity to relevant specialties, such as neurology, stroke wards, gerontology, etc. The wards range in size from 18 to 50 beds. We did HASU-specific profiles, noting bed numbers, shift hours, access to scans (e.g. magnetic resonance imaging) (see Tables 11 and 18). In addition to the ward, the HASU teams work in the ED to assess and treat incoming patients. This also includes moving them to scanning facilities and working with radiologists to interpret CT scans. Some patients are either assessed or temporarily situated in other wards when the HASU has inadequate capacity; these are known as ‘outliers’. Therefore, our observational work occurred in all of these locations, but not outside the hospital.
The London HASUs are in the following hospitals:
-
Charing Cross Hospital
-
The Royal London Hospital
-
King’s College Hospital
-
St George’s Hospital
-
Northwick Park Hospital
-
Princess Royal University Hospital
-
Queen’s Hospital
-
University College London Hospital.
Ethics approval
The study received approval from the National Research Ethics Service Committee London, Westminster Branch (reference number 14/LO/1355) and local approvals at each participating trust.
Quantitative data collection
Sentinel Stroke National Audit Programme
We obtained anonymised patient-level data from the SSNAP for all patients treated in London HASUs with a primary diagnosis of stroke (ischaemic stroke or primary intracerebral haemorrhage) between 1 January 2014 and 31 December 2014. The SSNAP collects data on clinical characteristics, care quality (from the time of admission up to 6 months after stroke) and outcomes for all stroke patients admitted to acute care hospitals in England. Data are entered into the SSNAP database by local clinical teams via a secure web interface. During our study period, the case ascertainment in the SSNAP, which is calculated as the proportion of all acute stroke patients admitted to hospitals, was estimated to be 90% for England. 52
Primary outcomes: health outcome measures
Health outcome measures were (1) whether or not the patient died within 3 days of admission to the hospital and (2) functional outcome, measured by a mRS53 score of 3–6 (moderate, moderately severe, severe disability or death) at the end of the inpatient spell. Mortality data in the SSNAP are not available after hospital discharge; therefore, we measured mortality up to 3 days after admission to minimise the number of missed deaths. LOS was measured in the HASU and total LOS in hospital.
Secondary outcomes: quality-of-care measures
Quality-of-care indicators were measured from time of hospital admission or onset of stroke symptoms (the latter used for those who were already in hospital when they had their stroke) (Box 1).
Brain imaging within 1 houra,b and within 12 hours. a,b
Admission to a stroke unit within 4 hours. b
Dysphagia screen within 4 hours. a,b
Assessment by a nurse trained in stroke management within 24 hours. a,b
Administration of intravenous thrombolysis to eligible patients. a,b
Door-to-needle time < 1 hour in patients receiving thrombolysis. a,b
Assessment by a stroke specialist consultant physician within 12 hours and within 24 hours. b
Assessments by a physiotherapist, occupational therapist and SLT within 24 hours and within 72 hours. b
SLT, speech and language therapist.
Measures directly related to stroke nursing care (although not a formal nursing role, dysphagia screening is normally undertaken by a trained nurse), brain scanning and thrombolysis.
Pre-existing quality indicators routinely reported by the SSNAP.
We also included measures with more stringent time constraints to reflect the time-critical nature of acute stroke care.
The denominators used for each measure were consistent with the SSNAP key indicators. 54 Most outcomes were measured for all patients, but there were exceptions: patients who were medically unwell or refused to be screened were excluded from the dysphagia screen measure; patients with only ischaemic stroke who met the Royal College of Physicians’ guideline minimum threshold for thrombolysis were included in the thrombolysis rate; door-to-needle times included only those patients who received thrombolysis; patients who were medically unwell, refused to be assessed or had no relevant deficit were excluded from the therapist measures.
To inform our cost analysis we also created alternative versions of some of these variables that were not time limited, that is whether or not the patient:
-
had brain imaging
-
was admitted to a stroke unit
-
had dysphagia screening
-
was assessed by a nurse trained in stroke management
-
was assessed by a stroke specialist consultant physician
-
was assessed by a physiotherapist, occupational therapist or speech and language therapist (SLT).
Independent variables
In-hours and out-of-hours measures
To examine variations across the week, we initially used a flexible specification of time of admission, measured in six 4-hour periods, 00.00 (midnight) to 03.59, 04.00 to 07.59, 08.00 to 11.59, 12.00 (noon) to 15.59, 16.00 to 19.59 and 20.00 to 23.59, every day of the week (42 periods). We also created a more restrictive measure to examine broad trends across the week: Monday to Friday 08.00 to 19.59, Monday to Friday 20.00 to 07.59, Saturday and Sunday 08.00 to 19.59, and Saturday and Sunday 20.00 to 07.59 (four periods). These specifications were also used by Bray et al. 22
Patient characteristics
In every model we controlled for sex, age (continuous variable), ethnic group (six categories), type of stroke (infarction or primary intracerebral haemorrhage), comorbidities prior to admission, mRS before stroke (0–2, 3–5), level of consciousness on arrival at the hospital (four categories), method of admission to the hospital (three categories), time from onset of stroke symptoms to admission (four categories), month of admission (12 categories) and stroke unit. When analysing mRS scores of 0–2 compared with scores of 3–5 at the end of the inpatient spell, we additionally controlled for the number of days after admission at which the mRS score was measured.
We also computed data from the SSNAP on the number of patients arriving at the hospital in each time period.
Nurse staffing data
Comprehensive data on nurse staffing were collected from nursing rotas from each of the London HASUs for every time of the day across the 12-month study period (between 1 January and 31 December 2014). Nurse staffing measures comprised the band of the nurse in charge [bands 5–8 and registered bank (or agency) nurse] and whether the nurse in charge was also responsible for administering intravenous thrombolysis or whether there was an additional nurse to perform this activity. We also collected data on the total number of specialised stroke nurse staffing on duty in the HASU by band (as above), the number of beds in each HASU and the times of the nursing shift handover period. These data were combined with the SSNAP data set based on the time of arrival at the hospital, so that for every patient who was admitted to a London HASU during the study period we also knew the characteristics of the nurse staffing at the time that they were admitted to the hospital. Note that we were unable to collect medical staffing data and so did account for this in our analyses (see Chapter 5).
Qualitative data collection
Sampling
Staff were sampled purposively to ensure coverage of a range of professional roles found in the HASUs (Table 2), including medical, nursing, therapy, administrative and managerial staff. Patients were also recruited purposively to reflect variations in sex, age, stroke type, discharge destination and carer perspectives (Table 3). The recruitment procedure was as follows: HASU staff provided researchers with a list of eligible patients in the HASU or stroke unit previously admitted to the HASU (see Recruitment and consent). Patients were introduced by clinical staff and given study information to consider. They were told that the interviewers were not members of current clinical staff and that their participation would not affect their care. Full and informed consent was obtained at the time of the interview. Interviews were conducted at participants’ homes, public places or clinical settings, according to their preference. The interviews were conducted between 6 and 99 days after admission to the HASU (average 38 days, median 33 days).
Profession | HASU site ID (n) | Total (n) | |||||||
---|---|---|---|---|---|---|---|---|---|
H1 | H2 | H3 | H4 | H5 | H6 | H7 | H8 | ||
Lead consultant physician | 1 | 1 | 1 | 1 | 1 | 1 | 1 | 1 | 8 |
Consultant physiciana | 1 | 1 | 1 | 3 | |||||
Registrar | 1 | 1 | 1 | 1 | 1 | 1 | 2 | 8 | |
Senior nurse/ward manager | 1 | 1 | 1 | 1 | 1 | 1 | 1 | 7 | |
Senior house officer | 1 | 1 | 1 | 1 | 1 | 1 | 1 | 7 | |
Occupational therapist | 1 | 1 | 1 | 1 | 1 | 1 | 1 | 1 | 8 |
Physiotherapist | 2 | 1 | 1 | 1 | 2 | 1 | 1 | 1 | 10 |
SLT | 1 | 1 | 1 | 1 | 1 | 1 | 1 | 1 | 8 |
Administrator/SSNAP | 1 | 1 | 2 | ||||||
Nurse | 1 | 1 | 1 | 1 | 1 | 1 | 1 | 1 | 8 |
Stroke co-ordinator/facilitator | 1 | 1 | 1 | 1 | 1 | 1 | 6 | ||
HASU lead research nurse | 1 | 1 | |||||||
Total completed | 11 | 9 | 9 | 9 | 9 | 10 | 9 | 10 | 76 |
Characteristic | Value |
---|---|
Age in years, mean (range) | 66 (33–90) |
Female, n/N (%) | 15/31 (48) |
Interviewee, n/N (%) | |
Patient and carer | 10/31 (32) |
Patient alone | 19/31 (62) |
Carer alone | 2/31 (6) |
Stroke type, n/N (%) | |
Ischaemic | 19/31 (61) |
Haemorrhagic | 2/31 (6) |
Stroke type not recorded | 10/31 (33) |
Transfer destination on repatriation from HASU, n/N (%) | |
Home | 15/31 (48) |
Stroke unit | 10/31 (32) |
Rehabilitation unit | 4/31 (12) |
Died in hospital | 1/31 (3) |
Discharge unclear | 1/31 (3) |
Underwent thrombolysis, n/N (%) | 7/31 (23) |
Recruitment and consent
To analyse the organisation of all eight London HASUs during the day and during the night, on weekdays and at weekends, authors GB, ABL, JE and PX conducted 76 interviews with HASU staff and 31 interviews with stroke patients and carers, and undertook 41 non-participant observations of HASU activity over approximately 85 hours. JE also gathered data through informal discussion on staff numbers and seniority on the ward, the composition of staff attending suspected stroke calls in the emergency room and the availability of scans across the week from each site. All interviewers were female and employed as research staff on the project. GB, ABL and PX are postdoctoral researchers with significant interviewing experience and no prior experience of working in stroke. JE was a stroke co-ordinator in a HASU prior to joining the project, with an occupational therapy background. She received research interview and analysis training and feedback from the rest of the team. Qualitative work was overseen by NJF and AIGR, who are experienced in both qualitative research and the London stroke system through previous work.
Patients were approached if they had a provisional or tentative stroke diagnosis and experience of receiving care in the HASU. We excluded patients and carers unable to sufficiently understand spoken or written English, and those who were unable to give informed consent by written or oral methods. Carers were interviewed alone or alongside patients, if patients requested it, and when the patient suffered from severe cognitive impairment or speech difficulty as well as when a patient had died during, or after, admission to a HASU.
The staff interviews were conducted according to a semistructured topic guide. The guide comprised questions about a typical day (see Report Supplementary Material 1), the perceived differences in and attitudes towards working in and out of hours, team working and the HASU environment. The patient interviews followed a broadly biographical topic guide, asking about their journey up to, during and after their HASU stay. We also prompted them to reflect on their experiences in and out of hours. All participants were interviewed only once and interviews were recorded digitally.
Observations
Global written consent for observations was gained initially from service leads and from a selection of staff at team meetings. Thereafter, verbal consent was given by staff members for researchers to make observations of staff meetings and of the stroke care pathway.
Non-participant observations were conducted at least four times at each HASU site, two visits on weekdays, one in the evening during the week and one at the weekend, with some extra visits to certain HASUs to confirm or add to our findings. We aimed to collect information about two dimensions of HASU service organisation and delivery, how (1) a patient progresses through the stroke pathway, assessment in the ED, care on the HASU and HASU repatriation; and (2) HASU staff organise themselves to meet the London specifications in day-to-day delivery operations [e.g. thrombolysis for at least 90% of eligible patients, timely brain scans, direct admission to HASU within 4 hours, daily multidisciplinary team (MDT) meetings]. This research design drew on ongoing research to identify likely service elements and factors influencing the design and implementation of HASU care (Table 4).
HASU site ID | Ward round | MDT meeting | Admission/thrombolysis | 16.00 catch up | Nurse handover | Bed meeting | Discharge |
---|---|---|---|---|---|---|---|
H1 | ✓ | ✗ | ✗ | n/a | ✓ | ✗ | ✗ |
H2 | ✓ | ✓ | ✓ | ✗ | ✓ | ✓ | ✓ |
H3 | ✓ | ✓ | ✓ | ✓ | ✓ | ✓ | ✓ |
H4 | ✓ | ✓ | ✓ | n/a | ✓ | ✓ | ✓ |
H5 | ✓ | ✓ | ✓ | n/a | ✓ | ✓ | ✗ |
H6 | ✗ | ✓ | ✓ | n/a | ✓ | ✗ | ✓ |
H7 | ✗ | ✓ | ✓ | ✓ | ✓ | ✓ | ✗ |
H8 | ✓ | ✓ | ✓ | ✓ | ✓ | ✓ | ✗ |
Total | 6/8 | 7/8 | 7/8 | 3/8 | 8/8 | 6/8 | 4/8 |
Modes of analysis
Quantitative analysis
We investigated variations in quality of acute stroke care and outcomes by day and time of admission in London HASUs and evaluated whether or not nurse staffing affected 24/7 access to high-quality acute stroke care in eight London HASUs. For the former, we ran patient-level regressions, regressing each measure against time period of admission. To inform our cost analysis we also tabulated the non-time-dependent measures described above against time period of admission. For the latter, we also included nurse staffing measures in models for London HASUs. In every model we controlled for baseline patient characteristics (sex, age and ethnic group) and case mix (type of stroke, comorbidities prior to admission, mRS before stroke, level of consciousness on arrival at the hospital, method of admission to the hospital, time from onset of stroke symptoms to admission and month of admission). We tested for statistically significant variations using Wald tests and likelihood ratio tests.
Qualitative analysis
The investigation was driven by understanding how London HASUs organise and deliver 24/7 stroke care in practice, how these service patterns have been influenced by workforce and organisational factors, and how stakeholders, including staff, patients and carers, experience these services.
The qualitative analysis drew together interview and observation data using a composite, thematic analysis across HASUs, as well as a comparative case study approach. The thematic approach55 allowed for developing an understanding of the central issues HASUs, as a particular type of service delivery model, face when delivering care in acute trust settings across 24/7 time scales. The comparative case study approach46–48 illuminated similarities and differences between HASUs and across professional groupings.
We used an iterative approach to analysis, following a five-step process to generate thematic and case study insights.
Step 1: data familiarisation
Data were compressed into workable, standardised forms (i.e. transcription formatting) using several initial mapping methods, allowing team members to become familiar with the types of data being collected (e.g. producing tables showing distribution of participants, making lists of information gathered through observations, key components of the service). These were adapted and refined as we became more familiar with the data. Simultaneously, we filled some of the gaps through ongoing data collection.
Step 2: initial framework coding guided by protocol
Three large groupings based on the central objectives of the study were developed as a way to initially sift through data focused by the protocol questions. A handful of patient, carer and staff transcripts were read by each of the four researcher team members (approximately five per researcher), with the groupings then applied and relevant data extracted.
Step 3: initial coding and framework development
The qualitative team met and discussed central issues emerging under the three-capture category rubric. Together, we developed a preliminary framework of codes reflecting central ideas, grouped into seven categories. Each category was defined along with the assigned codes. Codes then guided the extraction of relevant pieces of data in the interviews and observations. Code extracts were sorted by HASU to begin to identify differences by HASU and professional grouping.
Step 4: refining codes and coding framework
The research team met regularly to discuss extracts and codes to both refine and deepen our understanding of ideas emerging from the data. Some ideas led to new or redefined codes and the collapsing of multiple similar codes into one code. It also began the process of prioritising the most important of the codes, getting to the heart of the study’s driving research questions.
The team regularly met with the overall team (principal investigator, advisors and quantitative team) to feed back learning and solicit insights to further develop analytical threads.
Step 5: ‘headlines’ and written memos
The research team moved from the refined codes in iterative fashion to linking them into arguments, combining pieces of evidence to generate convincing answers to the driving research questions. In this process, we elaborated on the comparative case studies by professional groups and HASUs.
Synthesis of approaches
We used our monthly project meetings to bring together observations, queries and suggestions between the qualitative and the quantitative researchers. Interactions centred on key ideas such as:
-
Can we explain why certain variables do and do not show temporal variation?
-
What are the best ways to capture temporal variation?
-
What factors would we expect to influence temporal variation?
-
What further data can we gather or analyse to expand our findings?
Patient and public involvement
Two stroke patient representatives contributed to development of our study protocol and research questions. Over the course of the study patient representatives participated in three Study Steering Committee meetings (in June 2014, June 2015 and July 2016), in which they participated in discussions of interim findings and ongoing development of the study. Lay summaries of our work were produced for each meeting and representatives were offered individual meetings to discuss matters alone before the main Study Steering Committee meeting. Our patient representatives raised issues related to staff handover and confirmed the importance of the interface between hospital and social services, which we incorporated into our analysis.
Chapter 3 Variation in quality of acute stroke care by day and time of admission: prospective cohort study of weekday and weekend centralised hyperacute stroke unit care
What is known about this topic?
-
There is conflicting evidence whether patients admitted at weekends have higher or lower quality of acute stroke care and better or worse outcomes than those admitted at other times of the week.
-
Recent analyses have shown that analyses of weekend compared with weekday effects or in-hours effects compared with out-of-hours effects may be flawed, as they do not account for variations by day of the week and time of day.
-
The centralisation of acute stroke services in London was associated, on average, with higher likelihood of providing evidence-based care and greater reductions in mortality.
What this analysis adds
-
London HASUs operate a uniform service across the week, with respect to rapid access to stroke nurse review, brain scans and administration of thrombolysis to eligible patients.
-
Three-day mortality and disability at hospital discharge do not vary by day and time of admission in London HASUs.
-
Other aspects of care are not constant across the week in London HASUs.
-
Costs of acute stroke care in London HASUs are higher for patients admitted at the weekend than for those admitted during the week.
Introduction
There is conflicting evidence whether or not patients presenting with acute stroke symptoms receive lower quality of care and have worse outcomes if admitted to hospital outside normal weekday working hours or at weekends (the so-called ‘weekend effect’). Some studies have shown that acute stroke patients admitted at weekends have lower quality of care and higher mortality,56 whereas others have shown the opposite,10,12,13,57 although there is little evidence in the context of acute stroke care. Evaluation of these studies is further complicated by recent evidence that stroke incidence reporting at the weekend may be unreliable in older studies,58 although this is based on data on patients admitted to a single hospital trust. Recent work based on data from the SSNAP data set further found that care quality and outcomes in acute stroke vary across the week, and concluded that binary comparisons of weekend and weekday, or of in-hours and out-of-hours, processes and effects oversimplify more likely variations by day of week and time of day. 22 Furthermore, no studies have investigated the impact of time of admission on disability following a stroke.
If there is lower quality of care and there are worse outcomes at the weekend, then these could be linked to reduced staffing levels. 59 Nurse staffing levels at weekends have been shown to be a significant predictor of mortality from acute stroke,60 whereas evidence from the USA suggests that specialised stroke units, with round-the-clock availability of specialist stroke teams and rapid access to imaging and thrombolysis, reduce variation in quality of care and outcomes across the week. 61–63 Following these findings, the aim of this study was to investigate variations in the quality of acute stroke care and outcomes by day and time of admission in London HASUs (objectives 1 and 2). We used national audit data for all patients in London HASUs who had a stroke during a 12-month period recorded by the SSNAP. 64 We hypothesised where there would be non-significant variation across the week in care quality measures in London HASUs and that this would also translate into non-significant variation in outcomes.
Methods
This section is based on a paper by Melnychuk et al. 65 This is an Open Access article distributed in accordance with the terms of the Creative Commons Attribution (CC BY 4.0) license, which permits others to distribute, remix, adapt and build upon this work, for commercial use, provided the original work is properly cited. See: http://creativecommons.org/licenses/by/4.0/. The text below includes minor additions and formatting changes to the original text.
Data and measures
As outlined in Chapter 2, Quantitative data collection, we used anonymised patient-level data from the SSNAP for all patients in London HASUs with a primary diagnosis of stroke between 1 January and 31 December 2014.
Statistical analysis
We ran patient-level logistic regressions by regressing each measure against the time period of admission. For LOS, we used parametric survival models (modelled as time to event of discharge), assuming a log-normal survival distribution. In every model we controlled for baseline patient and case mix characteristics, month of admission (12 categories) and hospital trust. When analysing mRS scores 0–2 compared with 3–5 at the end of the inpatient spell, we additionally controlled for the number of days after admission the mRS score was measured. We were unable to do this for the analysis of mRS score 0–2 compared with 3–6, as date of death was not available. We tested for statistically significant variations across the week using Wald tests and reported the results as joint p-values under the null hypothesis that the regression coefficients for every time period relative to the omitted time period were zero. We calculated the average predicted probability of each outcome (predicted median LOS in the case of the LOS variables) in each time period controlling for the covariates. Patients admitted with a diagnosis of acute stroke in London who were not treated in a HASU were excluded (6% of all London patients in our data set were not treated in a HASU). p-values < 0.05 were considered to be statistically significant. Data on National Institutes of Health Stroke Scale (NIHSS) score, a validated measure of stroke severity on a scale from 0 (no stroke symptoms) to 42 (severe stroke), were available for 93% of patients in London HASUs. Owing to the extent of missing NIHSS data, in our main analysis we controlled for stroke severity using level of consciousness on arrival at the hospital (one component of NIHSS, which was available for all patients in London HASUs). We then re-ran all analyses controlling for NIHSS on arrival at the hospital on the smaller sample, instead of level of consciousness on arrival. The findings using NIHSS score on arrival were qualitatively the same and are presented in the Report Supplementary Material 2, Figures S1–6.
Cost analysis
To investigate the costs of acute stroke care in London HASUs on different days and at different times of admission, we explored whether or not mean costs per patient in the HASU varied by time period of admission. Our cost perspective was the HASU. We examined costs associated with variations in LOS in the HASU. In a secondary analysis, we also included total LOS in the hospital. We re-ran the LOS models, described above, using ordinary least squares and computed adjusted mean HASU and total LOS by time period of admission (although mean costs are affected by skewness and median costs are not, it is the arithmetic mean costs that are most informative for health-care policy decision). 66 We also examined costs associated with variations in the non-time-dependent quality-of-care measures described in Chapter 2 (see Box 1) for patients in London HASUs (whether or not the patient had brain imaging; was admitted to a stroke unit; had dysphagia screening; was assessed by a nurse trained in stroke management, or a stroke specialist consultant physician, physiotherapist, occupational therapist or a SLT). Note that the reason for focusing on the non-time-limited measures was that costs are driven by whether or not the patient received these processes, rather than whether or not they received them within a given time period. We also included receipt of thrombolysis (administration of intravenous thrombolysis to eligible patients). As it was simpler to identify trends, we focused on variations between the four broad time periods across the week (Monday to Friday, 08.00 to 19.59; Monday to Friday, 20.00 to 07.59; Saturday and Sunday, 08.00 to 19.59; and Saturday and Sunday, 20.00 to 07.59). Measures for which there were variations by time period of admission were included in the cost analysis. In this case, we multiplied the incremental volume of resource use associated with each measure for the three other time periods compared with Monday to Friday 08.00 to 19.59 by its unit cost, taken from two published economic evaluations of acute stroke care in London HASUs67,68 and inflated to 2017/18 prices. 69 We assumed that unit costs of the non-time-dependent measures did not vary by the time period of admission, because these are typically provided during normal working hours (see Chapter 6, What this analysis adds).
Results
This section is based on a paper by Melnychuk et al. 65 This is an Open Access article distributed in accordance with the terms of the Creative Commons Attribution (CC BY 4.0) license, which permits others to distribute, remix, adapt and build upon this work, for commercial use, provided the original work is properly cited. See: http://creativecommons.org/licenses/by/4.0/. The text below includes minor additions and formatting changes to the original text.
The study cohort comprised 7094 patients from eight London HASUs. The number of admissions varied across the week: admissions peaked during the day then fell at night; there were more admissions during the day through the week than during the day at the weekend; there were similar numbers of admissions during the night each day; and the highest number of admissions was during the day on Monday (Figure 3).
FIGURE 3.
Number of admissions in London HASUs across the 42 time periods in the week. This figure is adapted from Melnychuk et al. 65 This is an Open Access article distributed in accordance with the terms of the Creative Commons Attribution (CC BY 4.0) license, which permits others to distribute, remix, adapt and build upon this work, for commercial use, provided the original work is properly cited. See: http://creativecommons.org/licenses/by/4.0/. This figure includes minor additions and formatting changes to the original figure.
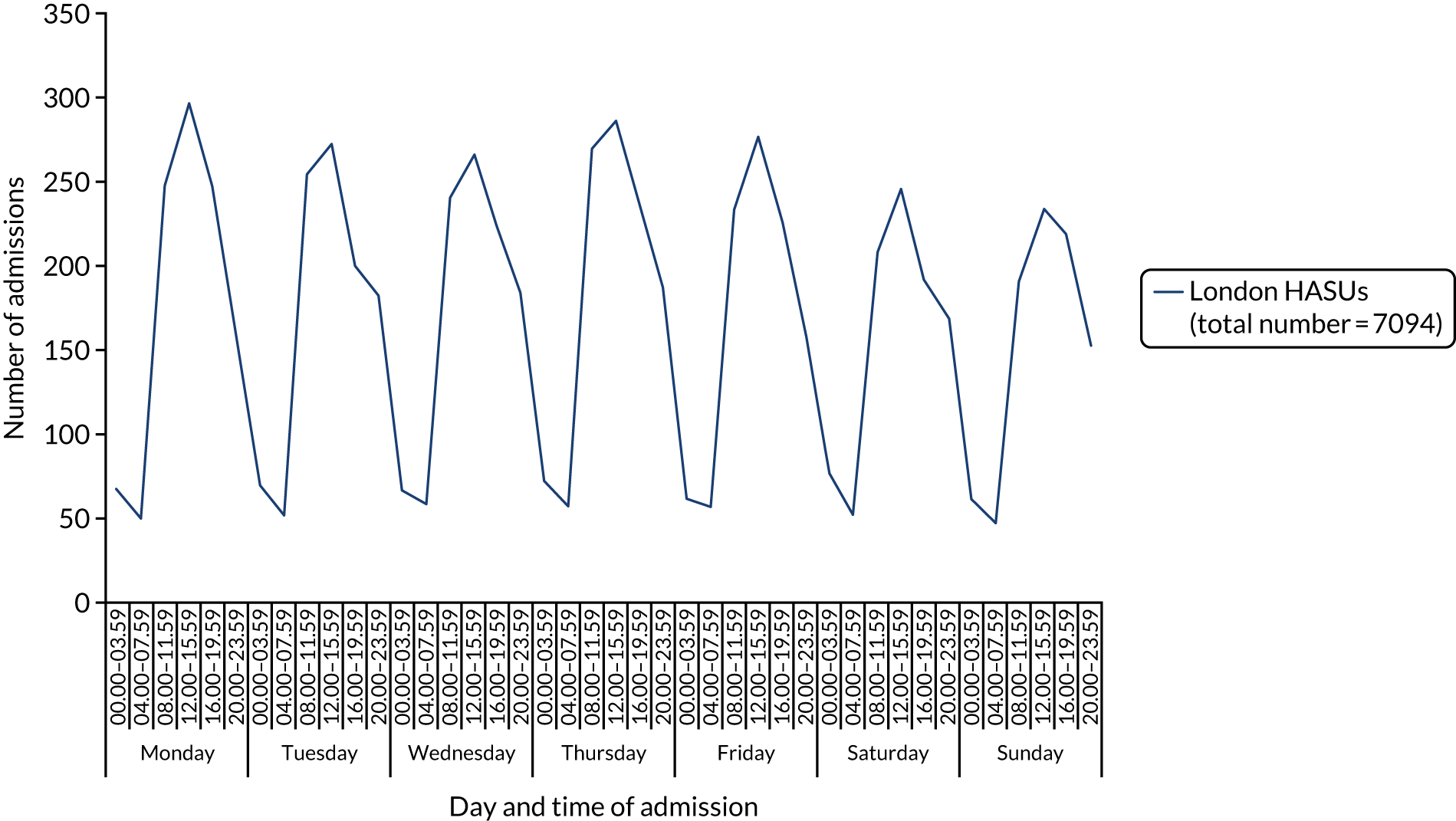
The mean number of admissions per HASU per week in each of the 42 4-hour time periods ranged from 1.03 to 1.44. There was slightly higher proportion of men than women, the mean age was 72 years and 38% of patients were non-white (Table 5). London HASUs case mix was characterised by a large proportion of people with congestive heart failure, hypertension and diabetes. Twenty-two per cent of patients had at least moderate disability according to their mRS score before stroke and 84% of patients arrived at the hospital in an ambulance. Thirty-nine per cent of patients were admitted to the hospital within 3 hours from onset of stroke symptoms, but for 29% of patients the time of symptom onset was unknown.
Characteristic | Value |
---|---|
Sex, n (%) | |
Male | 3719 (52) |
Female | 3375 (48) |
Age (years), mean (SD) | 72 (15) |
Ethnic group, n (%) | |
White | 4332 (61) |
Mixed | 72 (1) |
Black | 650 (9) |
Asian | 505 (7) |
Other | 526 (7) |
Not available | 1009 (14) |
Type of stroke, n (%) | |
Infarction | 6252 (88) |
Primary intracerebral haemorrhage | 842 (12) |
Comorbidities prior to admission, n (%) | |
Congestive heart failure | 439 (6) |
Hypertension | 4284 (60) |
Atrial fibrillation | 1229 (17) |
Diabetes | 1705 (24) |
Stroke/TIA | 1688 (24) |
mRS score before stroke, n (%) | |
Slight or no disability (0–2) | 5552 (78) |
At least moderate disability (3–5) | 1542 (22) |
Level of consciousness on arrival at the hospital, n (%)a | |
Alert | 5991 (84) |
Not alert, but respond to minor stimulation | 663 (9) |
Not alert, requires repeated stimulation | 281 (4) |
Unresponsive | 159 (2) |
NIHSS score on arrival at the hospital, median (IQR) | 5 (2–11) |
Method of admission to the hospital, n (%) | |
Already inpatient | 173 (2) |
Ambulance | 5966 (84) |
Walk-in | 955 (14) |
Time (minutes) from onset of stroke symptoms to admission, n (%) | |
< 180 | 2741 (39) |
180–359 | 759 (11) |
≥ 360 | 1516 (21) |
Time of onset not known | 2078 (29) |
There was no significant variation in care quality across the 42 time periods in any of the measures relating to brain scanning, stroke nursing care and thrombolysis in London HASUs (all p-values > 0.05) (Figure 4).
FIGURE 4.
Quality of care across the 42 time periods in the week: measures linked to performance standards for London HASUs. (a) Brain scan within 1 hour (p = 0.1372); (b) brain scan within 12 hours (p = 0.1757); (c) dysphagia screen within 4 hours (p = 0.2217); (d) nurse assessment within 24 hours (p = 0.3318); (e) administration of intravenous thrombolysis to eligible patients (p = 0.9233); and (f) door-to-needle time < 1 hour (p = 0.5400). This figure is adapted from Melnychuk et al. 65 This is an Open Access article distributed in accordance with the terms of the Creative Commons Attribution (CC BY 4.0) license, which permits others to distribute, remix, adapt and build upon this work, for commercial use, provided the original work is properly cited. See: http://creativecommons.org/licenses/by/4.0/. This figure includes minor additions and formatting changes to the original figure.
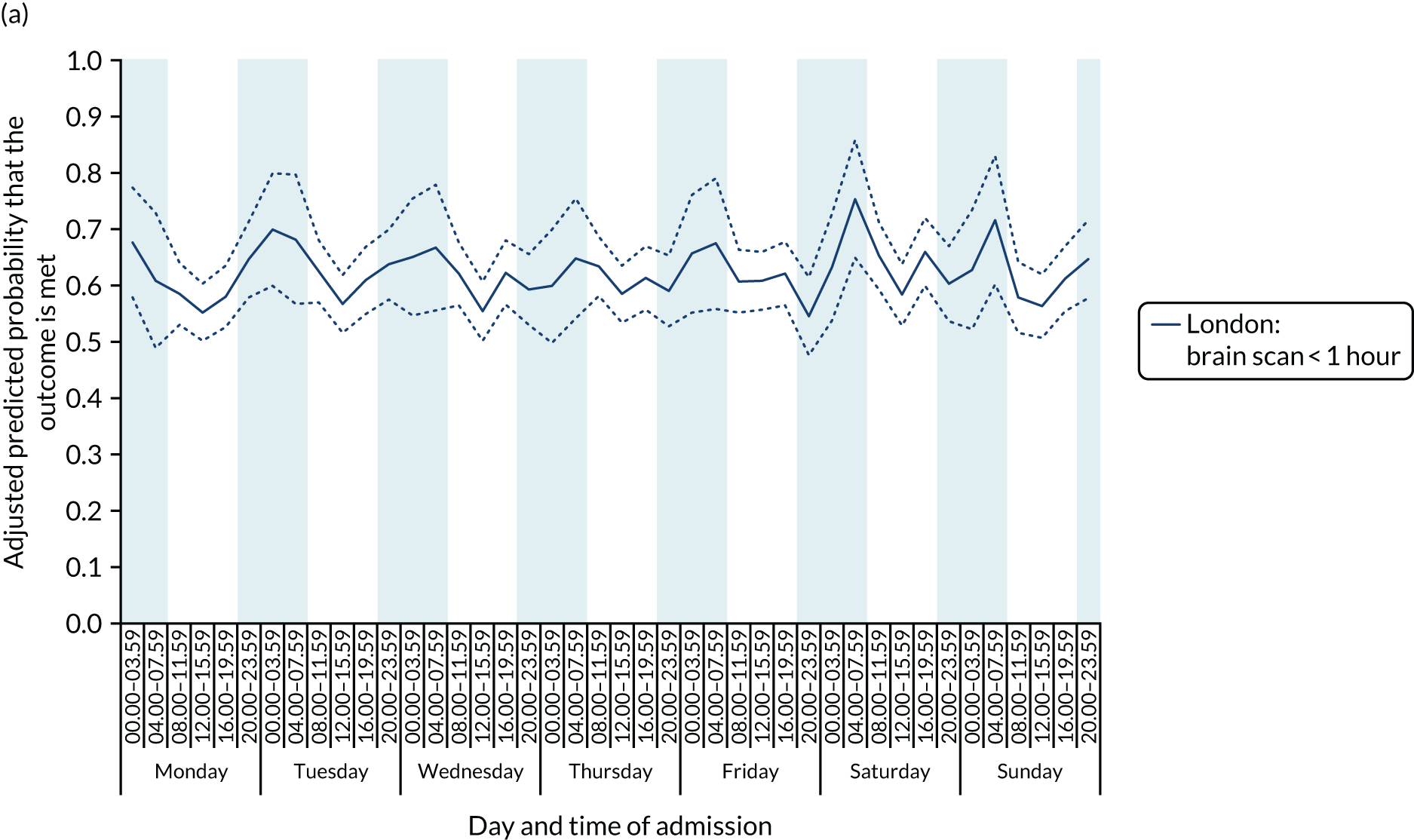
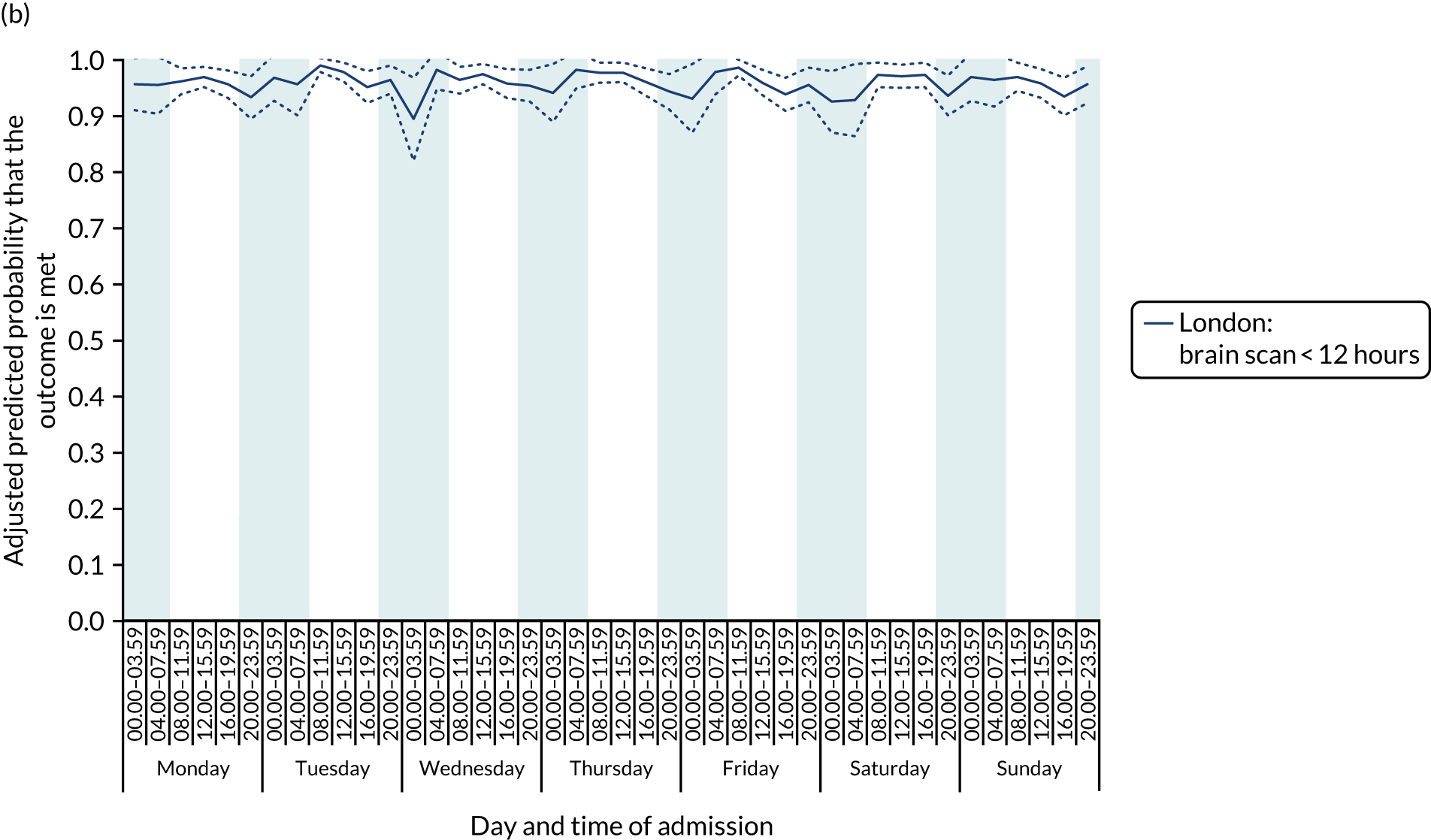
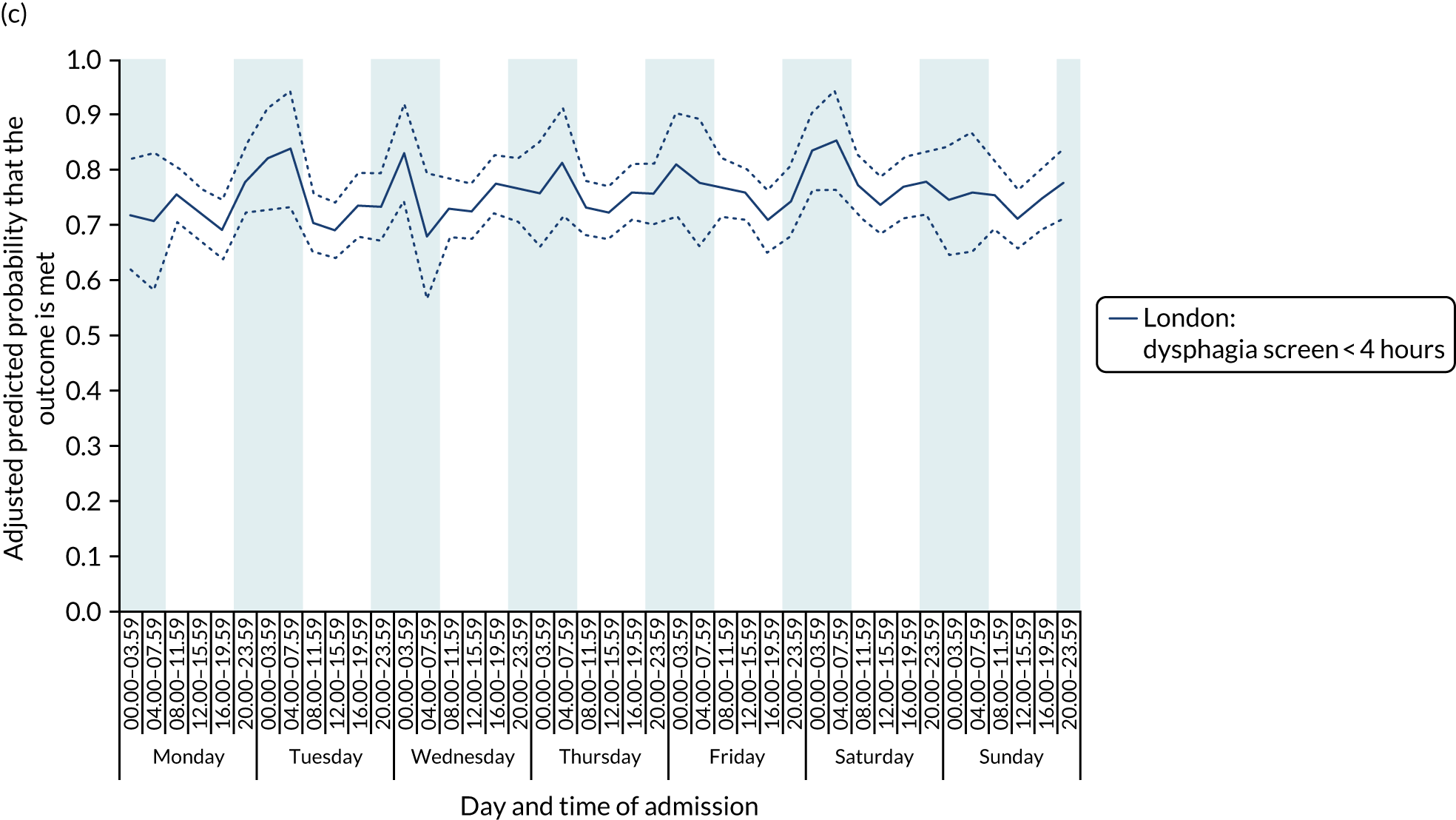
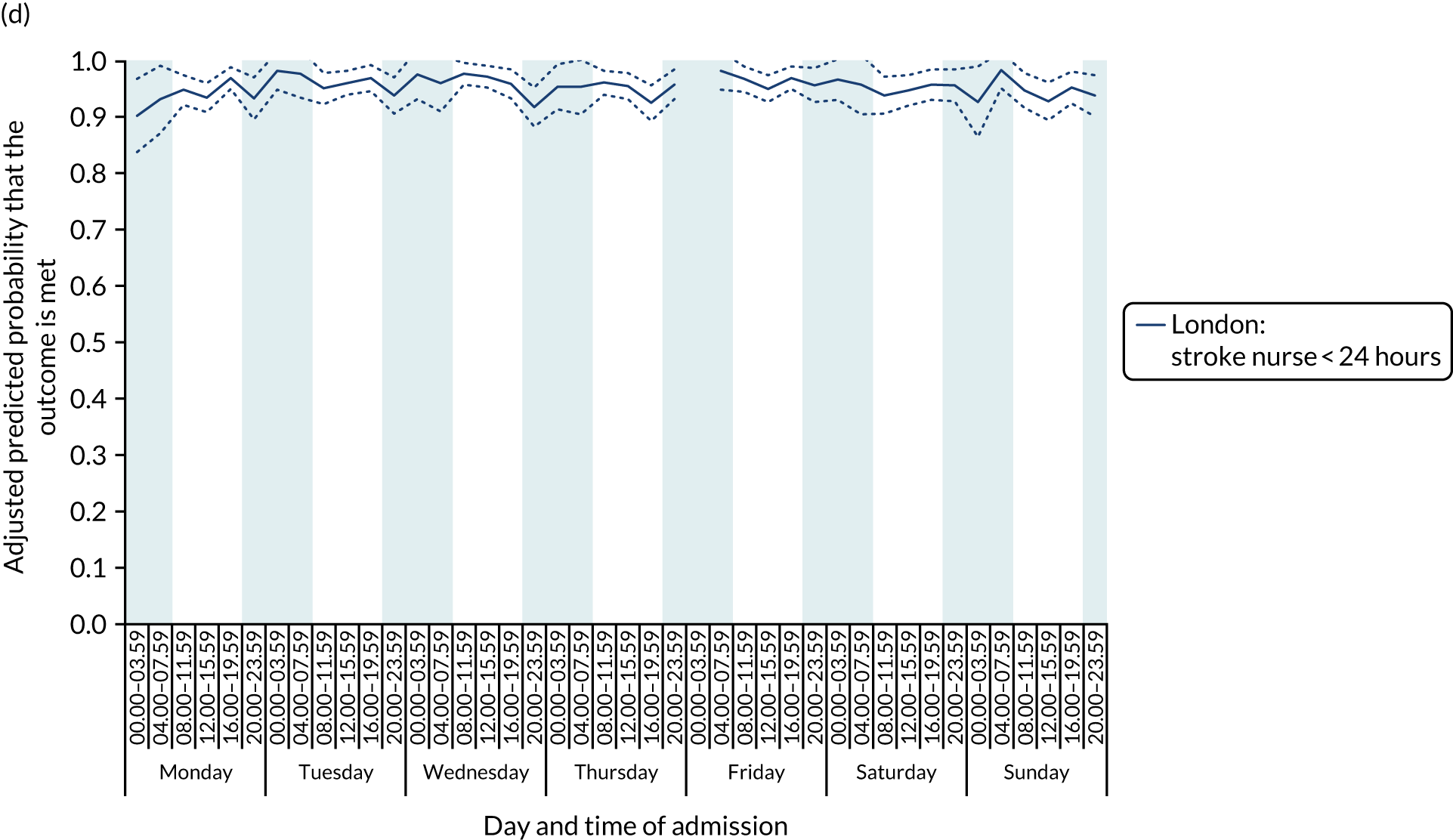
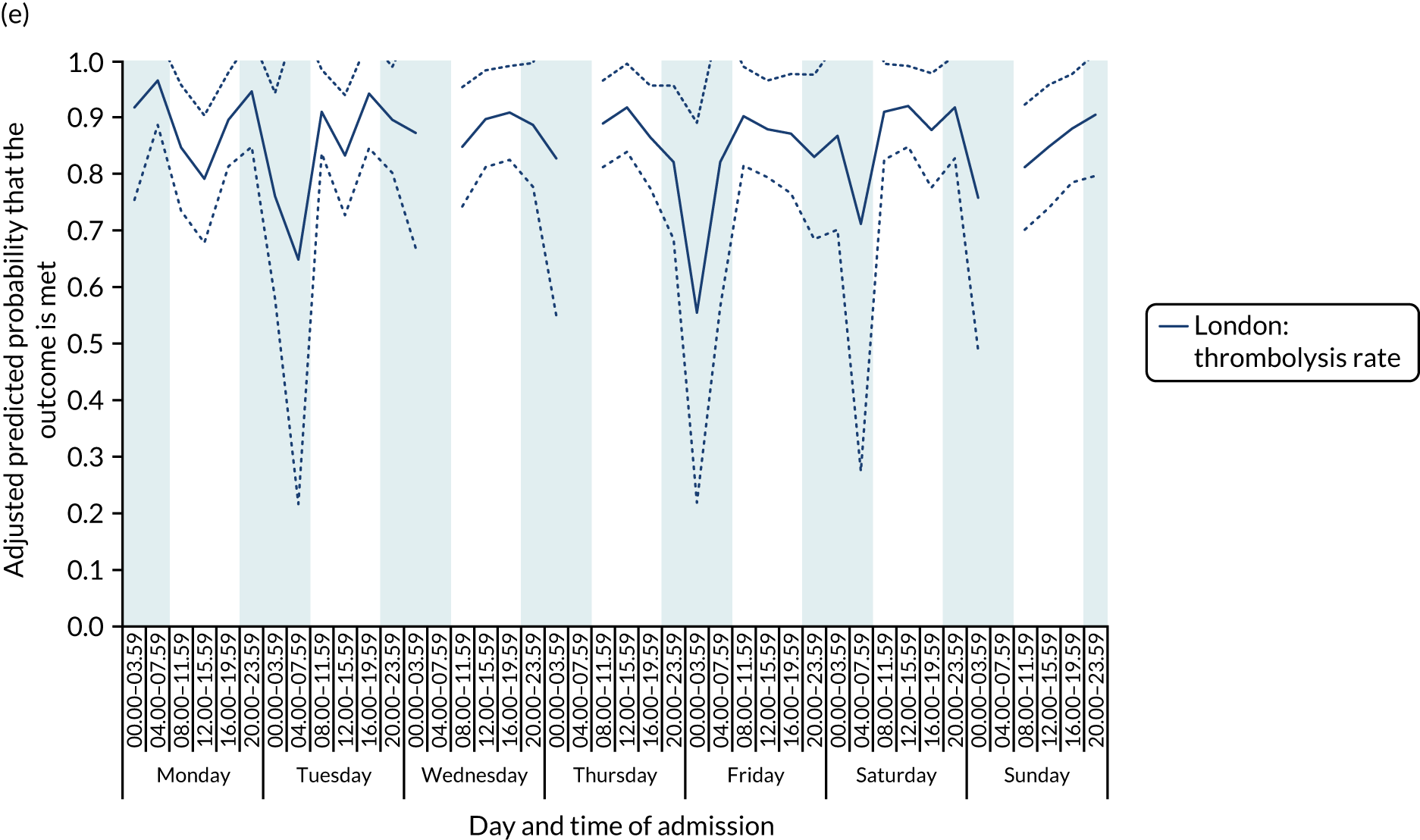
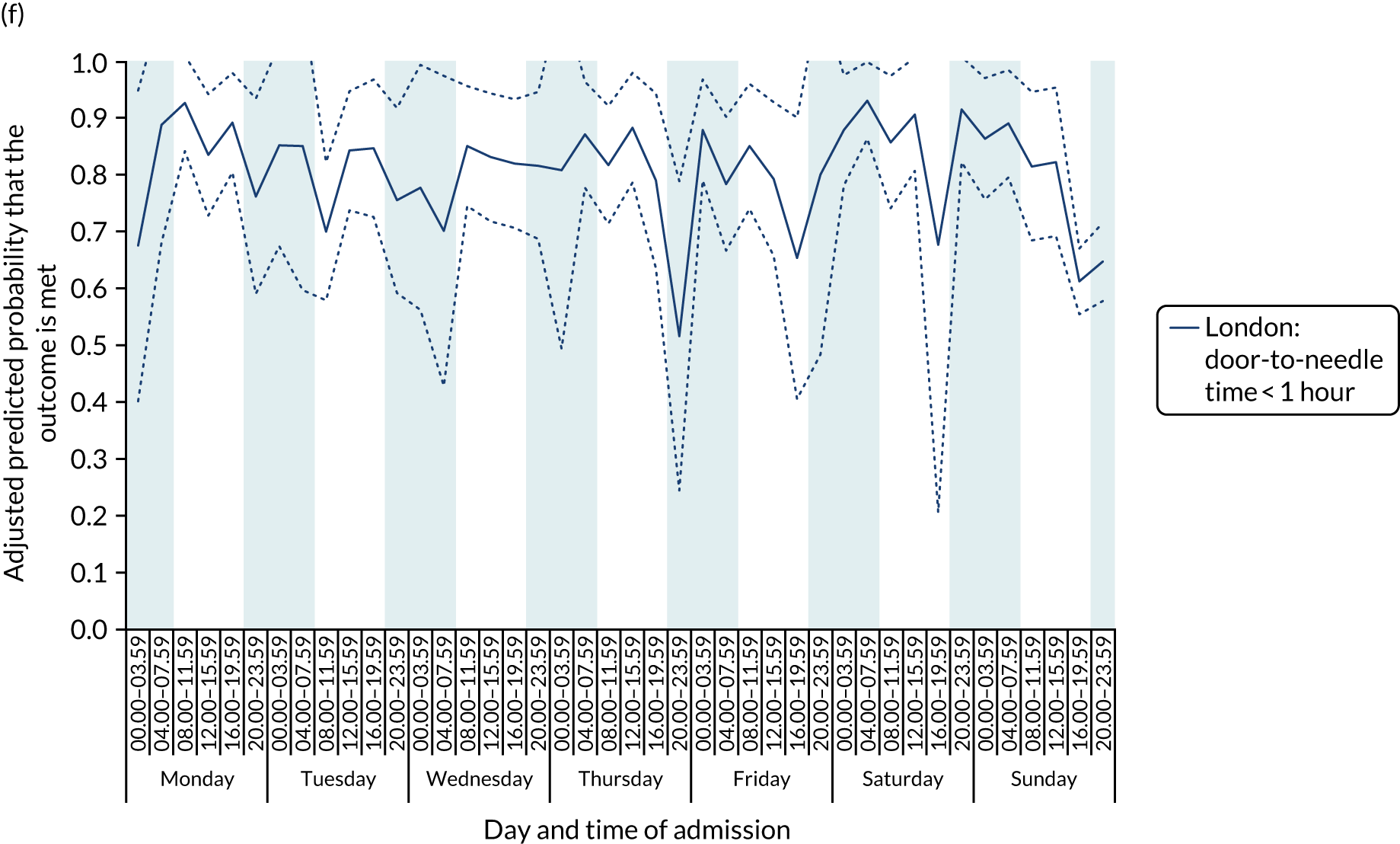
For all the other quality-of care-measures, there was significant variation by time period of admission across the week (all p-values < 0.01). There were three patterns of variation. First, variation by time of day but not day of the week was observed for assessment by a stroke specialist consultant physician within 12 hours and within 24 hours in London HASUs, and admission to a stroke unit within 4 hours (Figure 5).
FIGURE 5.
Quality of care across the 42 time periods in the week in London HASUs: variation by time of day but not day of the week. (a) Assessment by a stroke consultant (stroke physician < 12 hours, p < 0.0001; stroke physician < 24 hours, p = 0.01); and (b) admission to a stroke unit within 4 hours (stroke unit admission < 4 hours, p < 0.0001). This figure is adapted from Melnychuk et al. 65 This is an Open Access article distributed in accordance with the terms of the Creative Commons Attribution (CC BY 4.0) license, which permits others to distribute, remix, adapt and build upon this work, for commercial use, provided the original work is properly cited. See: http://creativecommons.org/licenses/by/4.0/. This figure includes minor additions and formatting changes to the original figure.
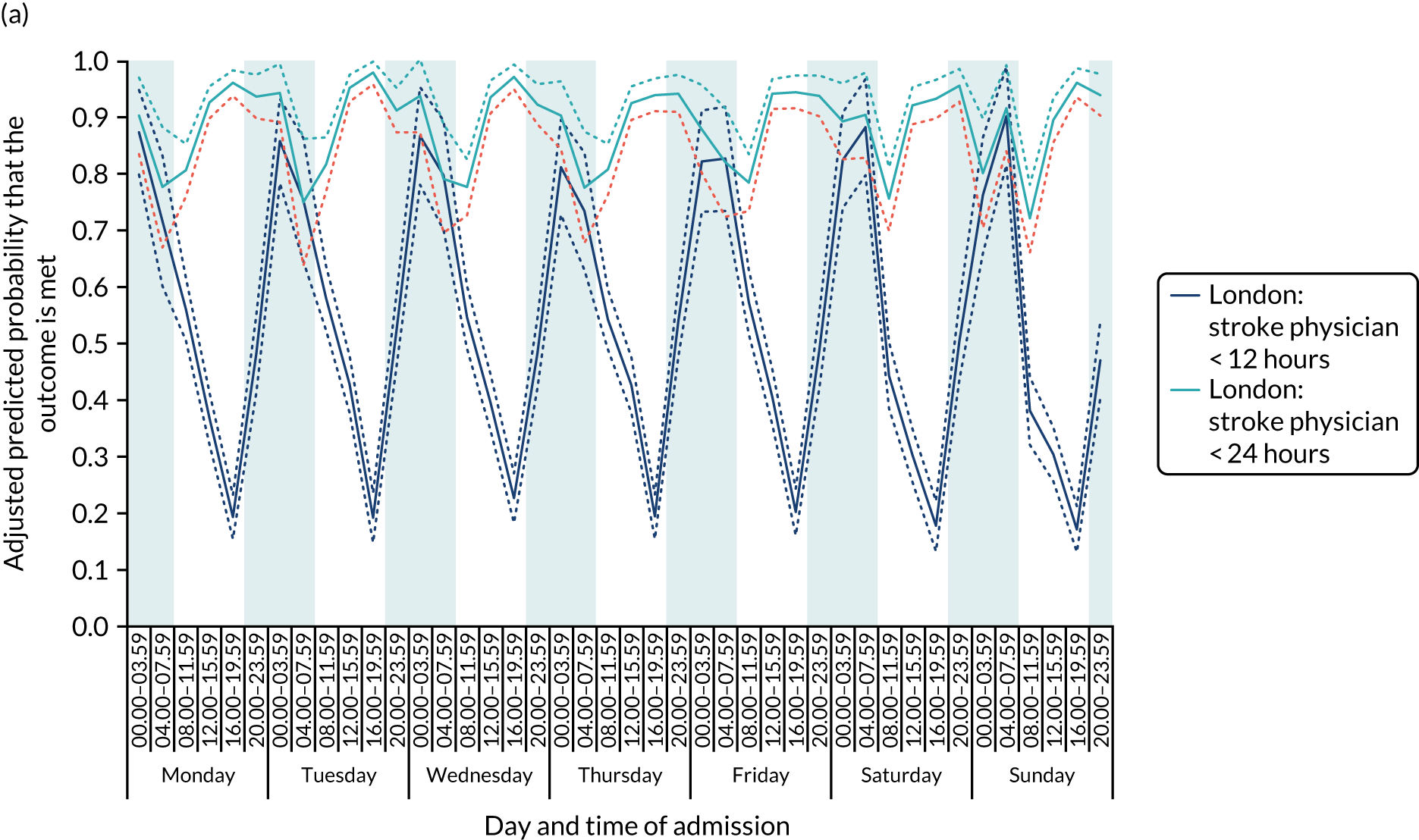
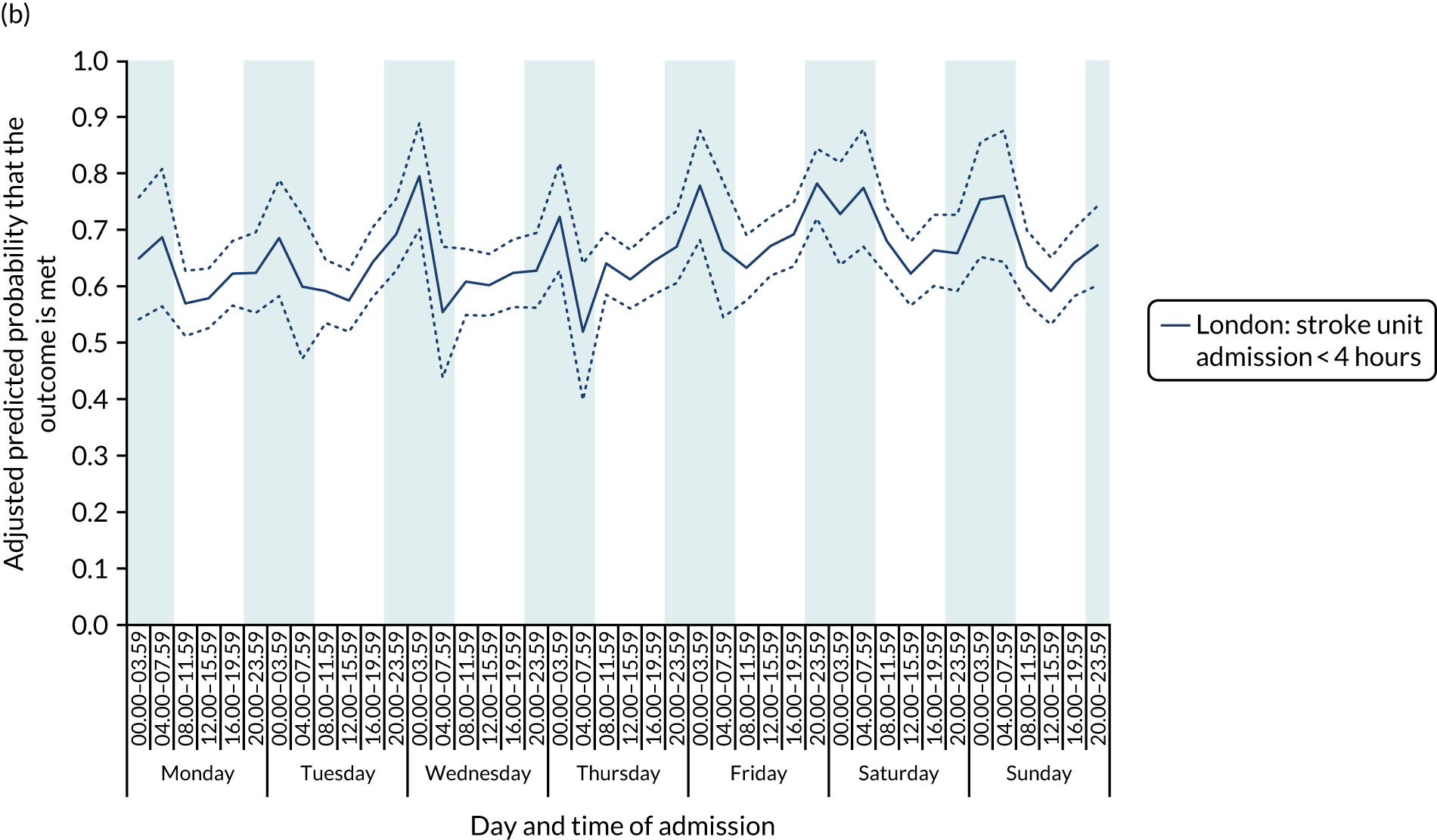
With this pattern, similar variations during the day were found each day of the week. Second, variation by day of the week but not time of day was observed for assessments by a physiotherapist, occupational therapist and SLT within 72 hours in London HASUs (Figure 6).
FIGURE 6.
Quality of care across the 42 time periods in the week in London HASUs: variation by day of the week but not time of day. (a) Physiotherapist assessment within 72 hours (p < 0.0001); (b) occupational therapist assessment within 72 hours (p < 0.0001); (c) swallow assessment by a SLT within 72 hours (p < 0.0001); and (d) communication assessment by a SLT within 72 hours (p < 0.0001). This figure is adapted from Melnychuk et al. 65 This is an Open Access article distributed in accordance with the terms of the Creative Commons Attribution (CC BY 4.0) license, which permits others to distribute, remix, adapt and build upon this work, for commercial use, provided the original work is properly cited. See: http://creativecommons.org/licenses/by/4.0/. This figure includes minor additions and formatting changes to the original figure.
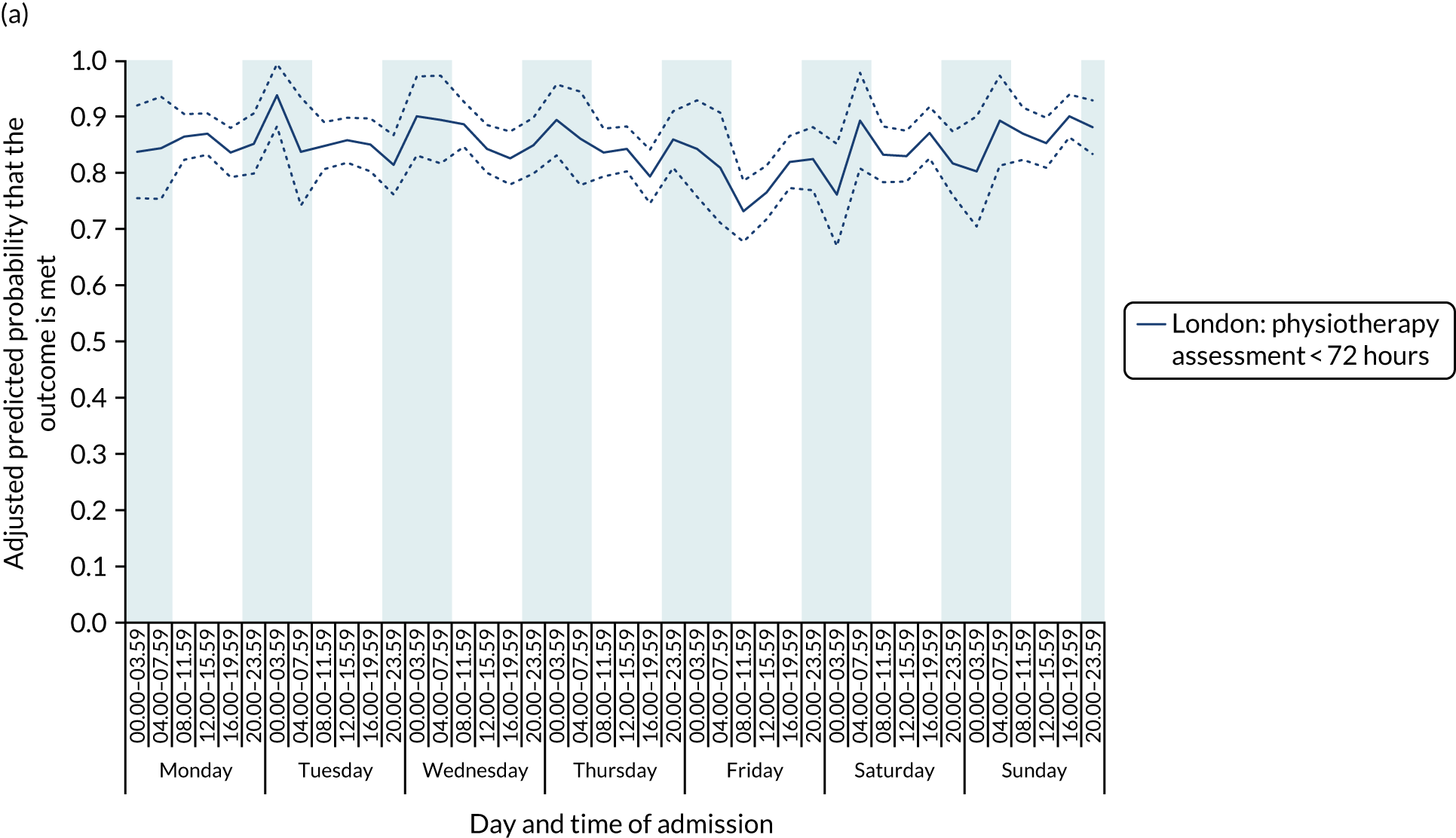
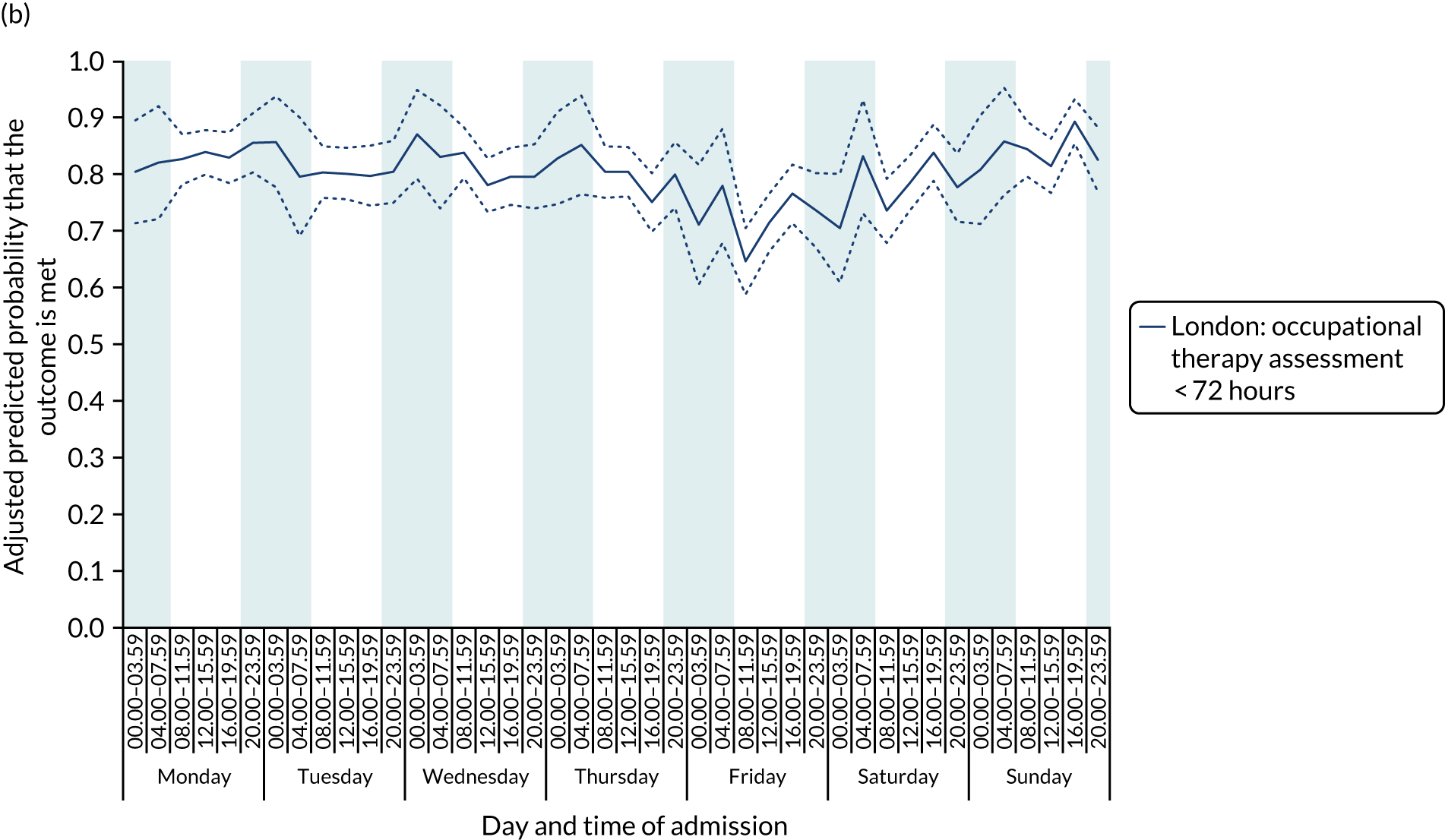
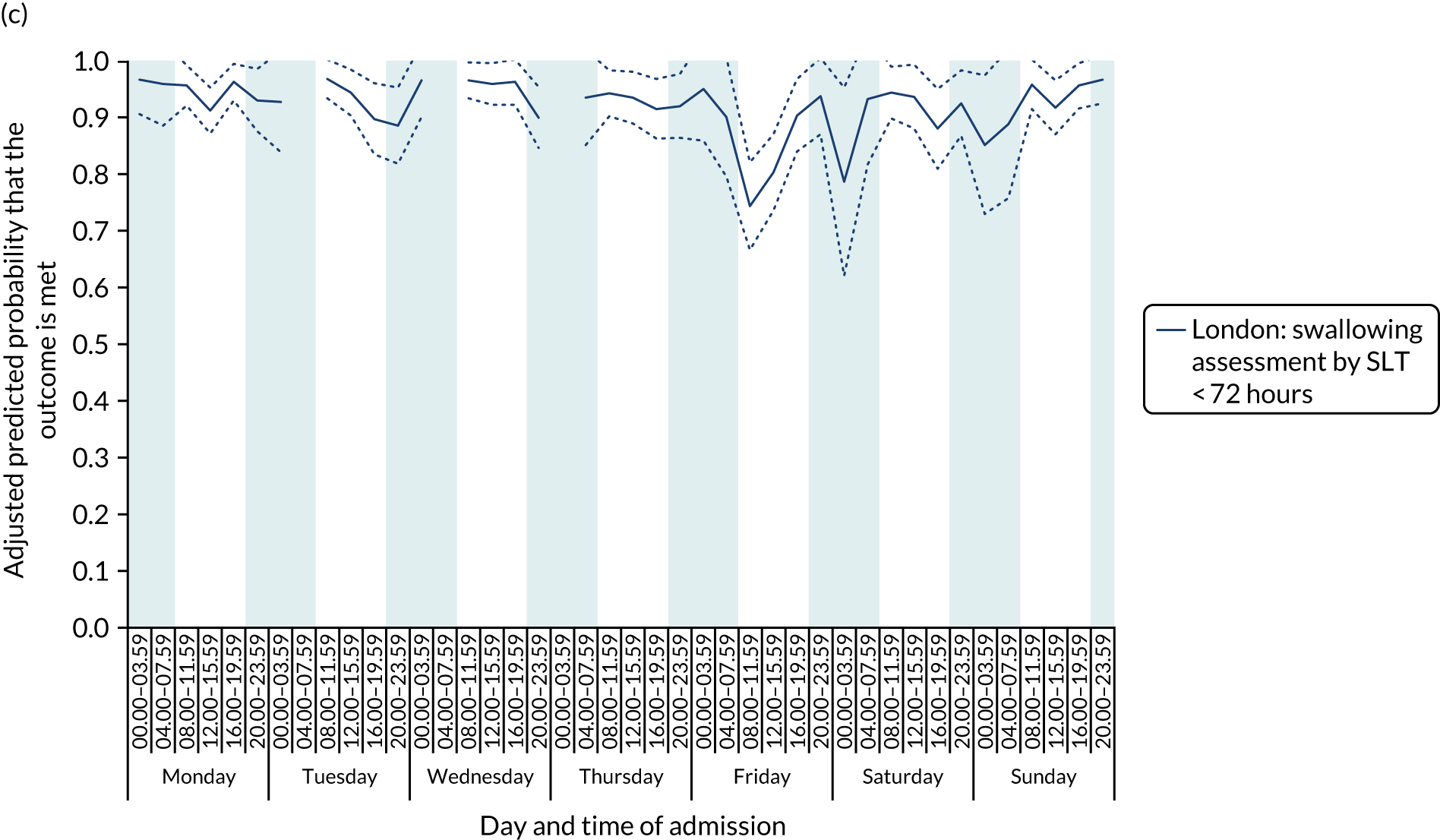
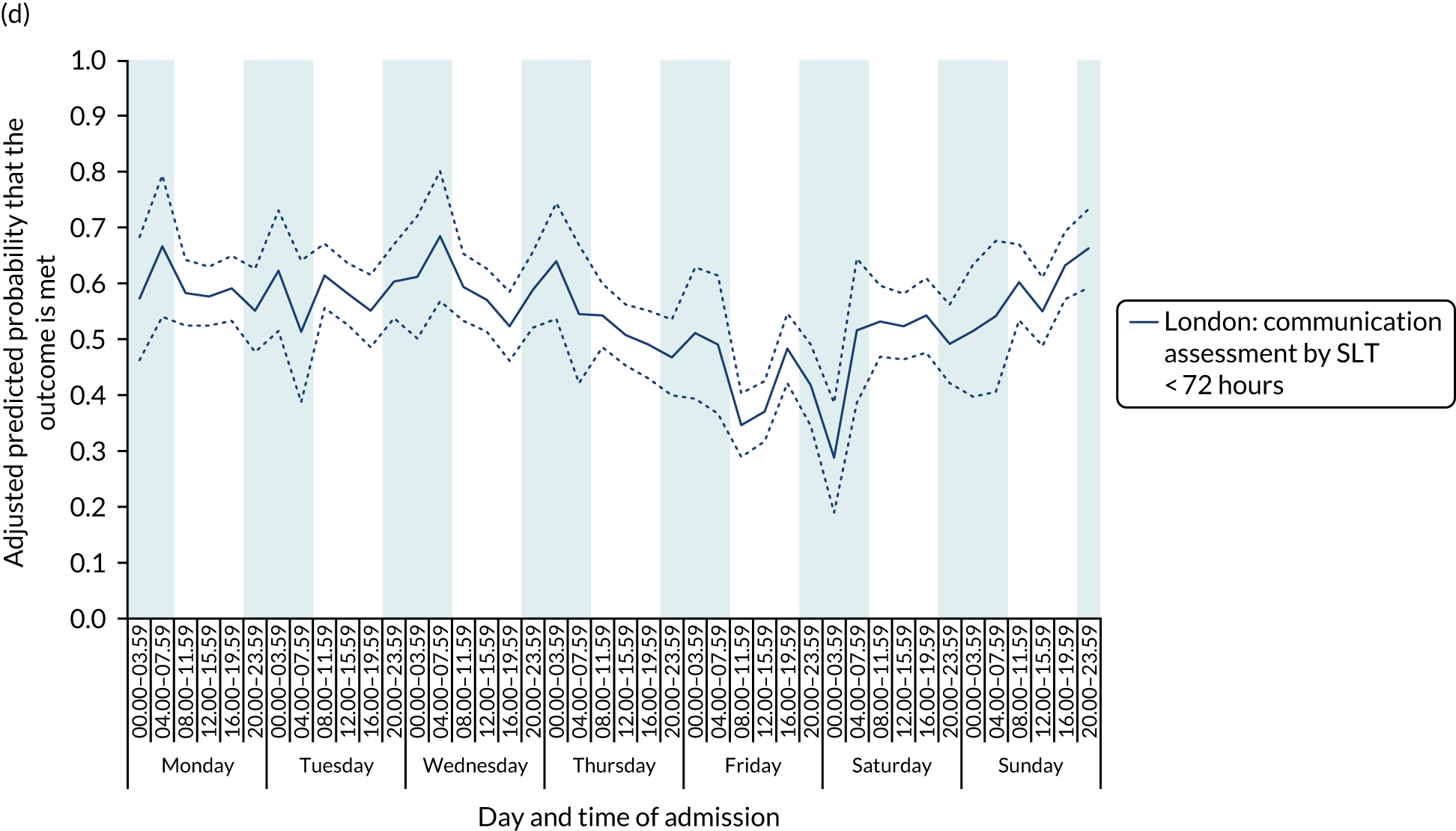
With this pattern, care quality was worse for patients admitted on a Friday. Third, variation by time of day and day of the week was observed for therapist assessments within 24 hours (Figure 7). With this pattern, there was variation during the day on Monday to Friday and care quality was worse at weekends.
FIGURE 7.
Quality of care across the 42 time periods in the week in London HASUs: variation by time of day and day of the week. (a) Physiotherapist assessment within 24 hours (p < 0.0001); (b) occupational therapist assessment within 24 hours (p < 0.0001); and (c) communication assessment by a SLT within 24 hours (p < 0.0001). This figure is adapted from Melnychuk et al. 65 This is an Open Access article distributed in accordance with the terms of the Creative Commons Attribution (CC BY 4.0) license, which permits others to distribute, remix, adapt and build upon this work, for commercial use, provided the original work is properly cited. See: http://creativecommons.org/licenses/by/4.0/. This figure includes minor additions and formatting changes to the original figure.
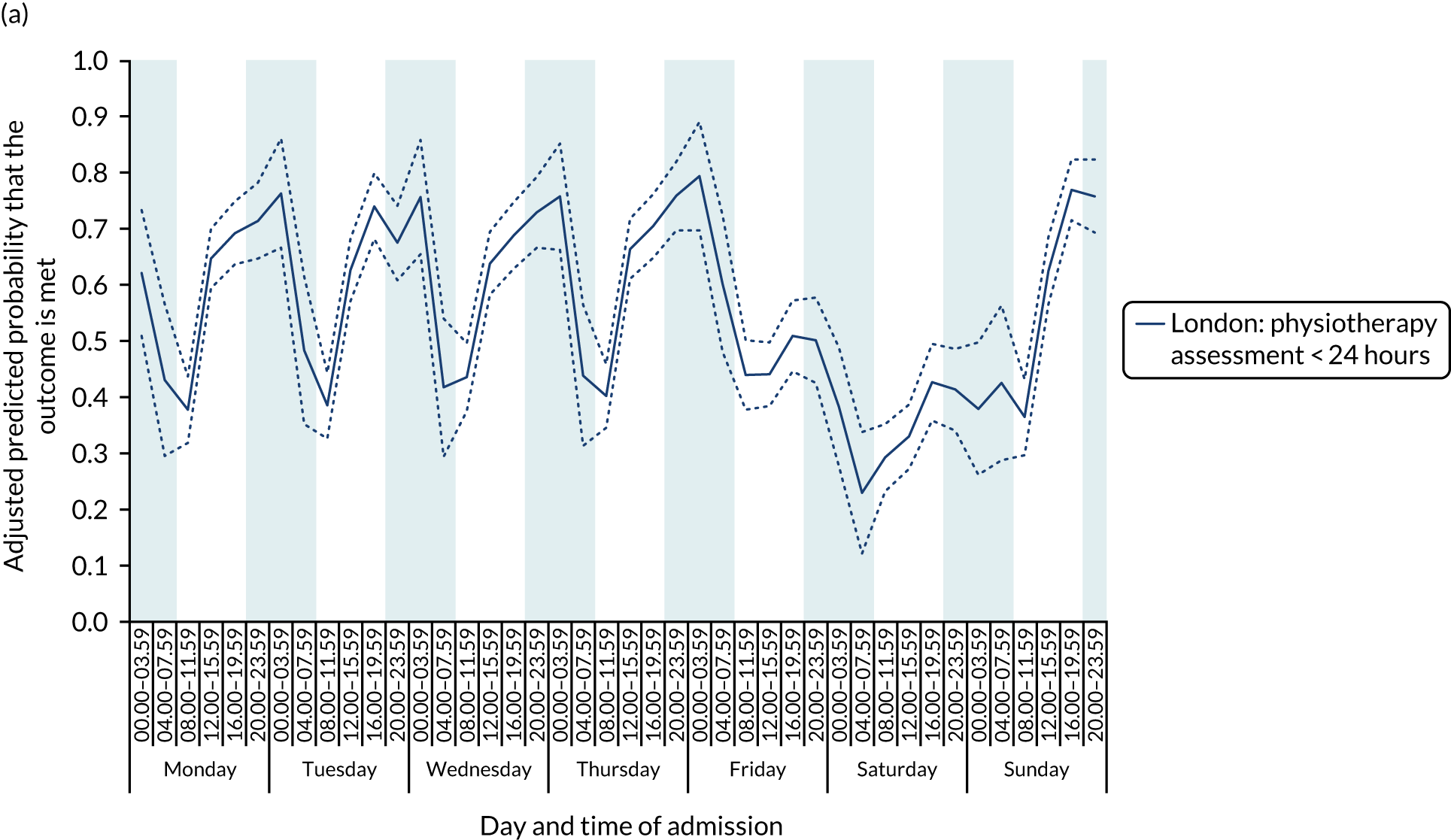
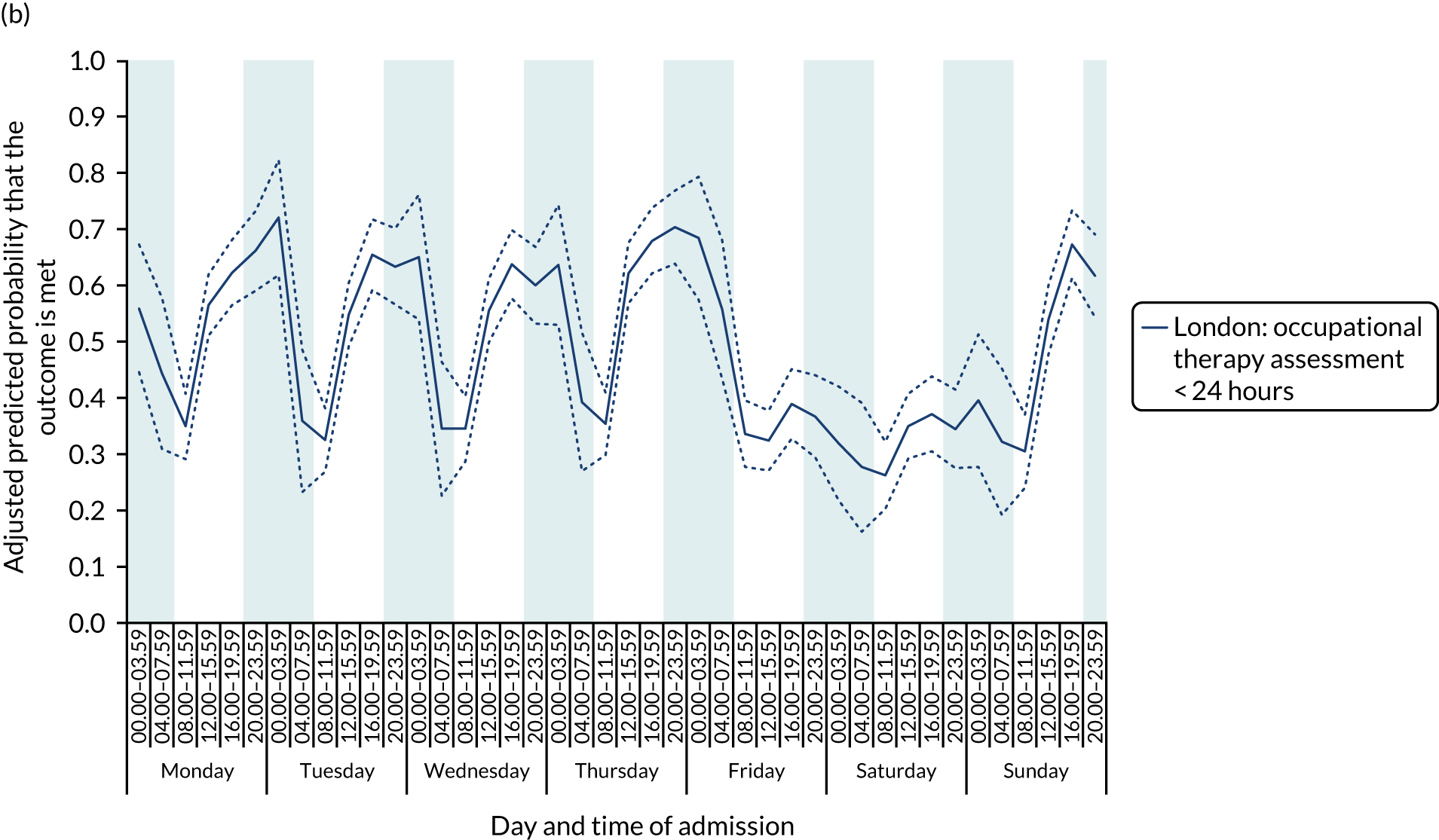
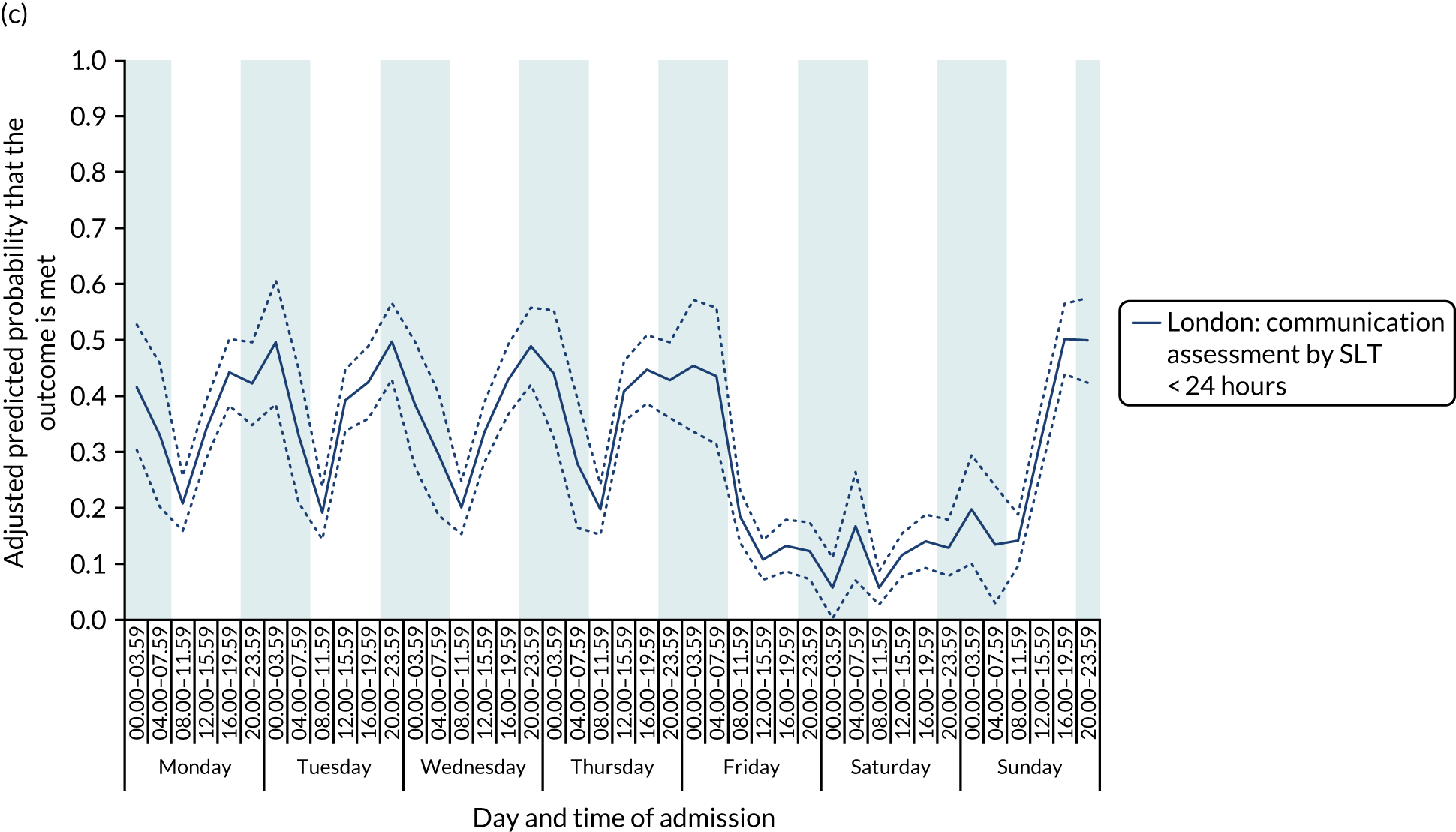
There was no significant variation in outcomes across the 42 time periods in London HASUs (all p > 0.05) (Figure 8).
FIGURE 8.
Outcomes across the 42 time periods in the week in London HASUs. (a) Mortality at 3 days (p = 0.9230); (b) mRS score 3–6 (p = 0.1777); and (c) mRS score 3–5 (p = 0.2015). This figure is adapted from Melnychuk et al. 65 This is an Open Access article distributed in accordance with the terms of the Creative Commons Attribution (CC BY 4.0) license, which permits others to distribute, remix, adapt and build upon this work, for commercial use, provided the original work is properly cited. See: http://creativecommons.org/licenses/by/4.0/. This figure includes minor additions and formatting changes to the original figure.
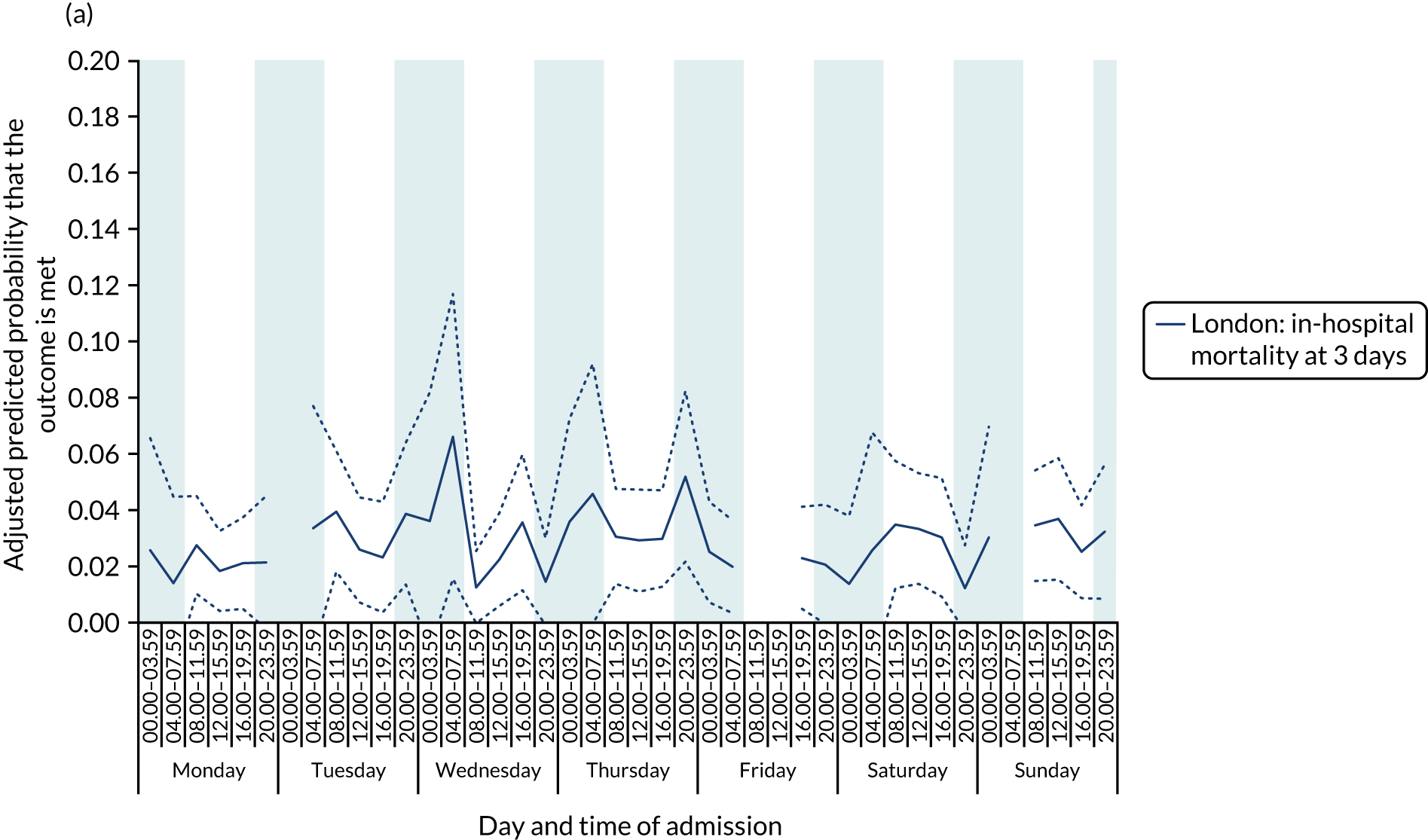
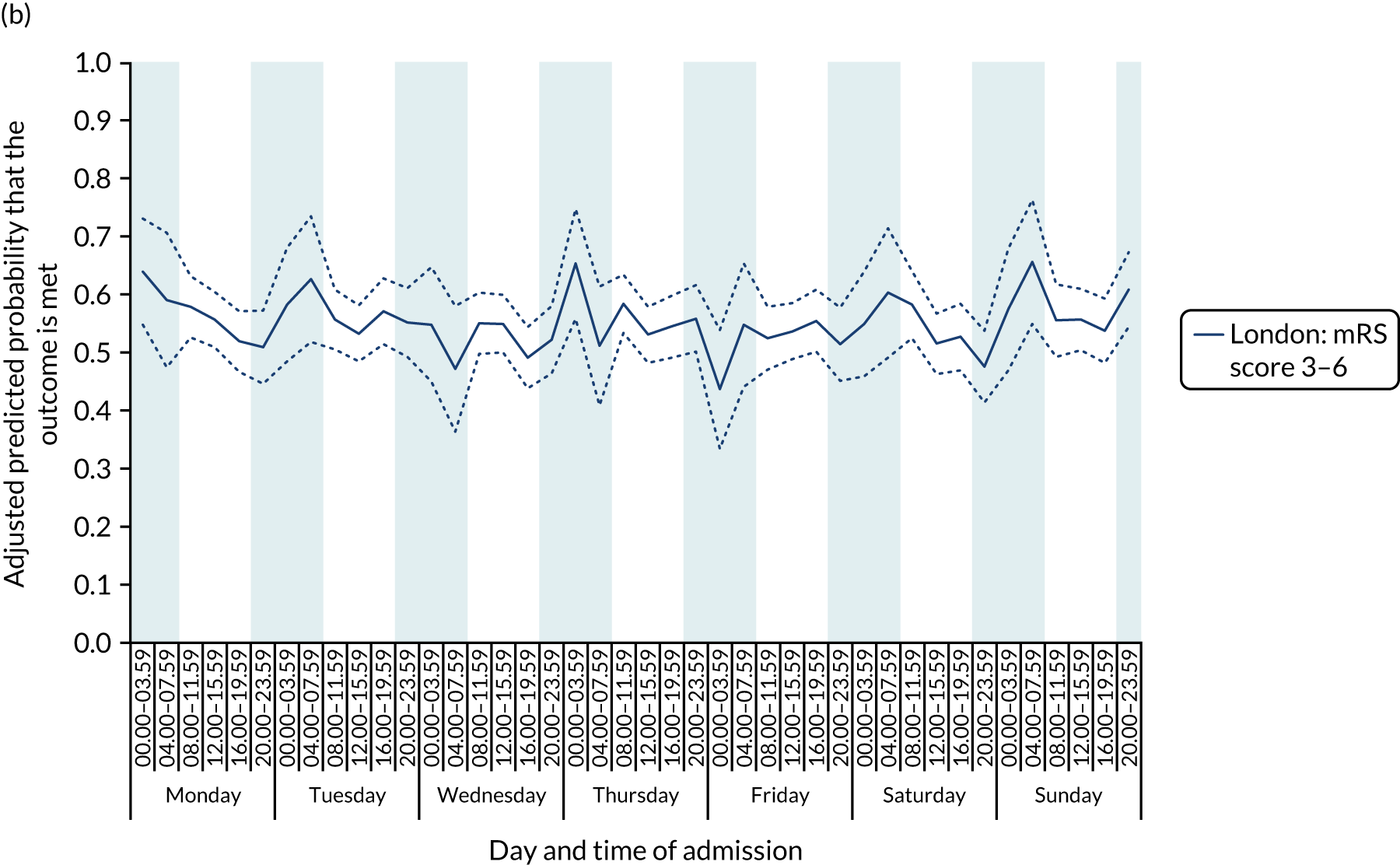
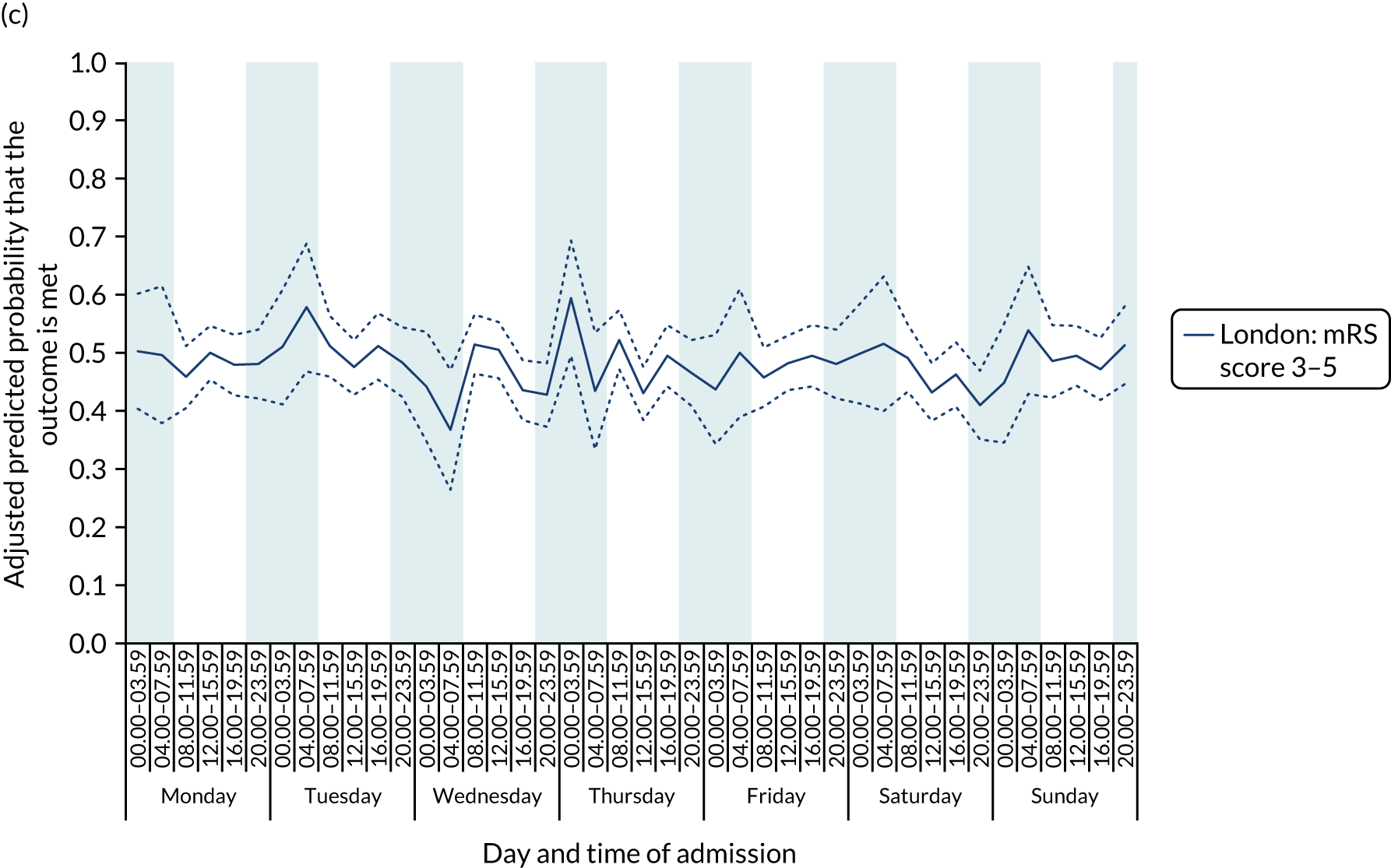
There was significant variation in LOS across the 42 time periods, in terms of both HASU LOS and total inpatient LOS (p < 0.001 for HASU LOS and p = 0.005 for total LOS in hospital) (Figure 9). Median HASU LOS varied between 2.6 and 3.6 days across the 42 time periods. It was difficult to detect a trend by day and time of admission in HASU LOS and inpatient LOS.
FIGURE 9.
Length of stay across the 42 time periods in the week in London HASUs. (a) LOS in HASU (p < 0.0001); and (b) LOS in hospital (p < 0.0001). This figure is adapted from Melnychuk et al. 65 This is an Open Access article distributed in accordance with the terms of the Creative Commons Attribution (CC BY 4.0) license, which permits others to distribute, remix, adapt and build upon this work, for commercial use, provided the original work is properly cited. See: http://creativecommons.org/licenses/by/4.0/. This figure includes minor additions and formatting changes to the original figure.
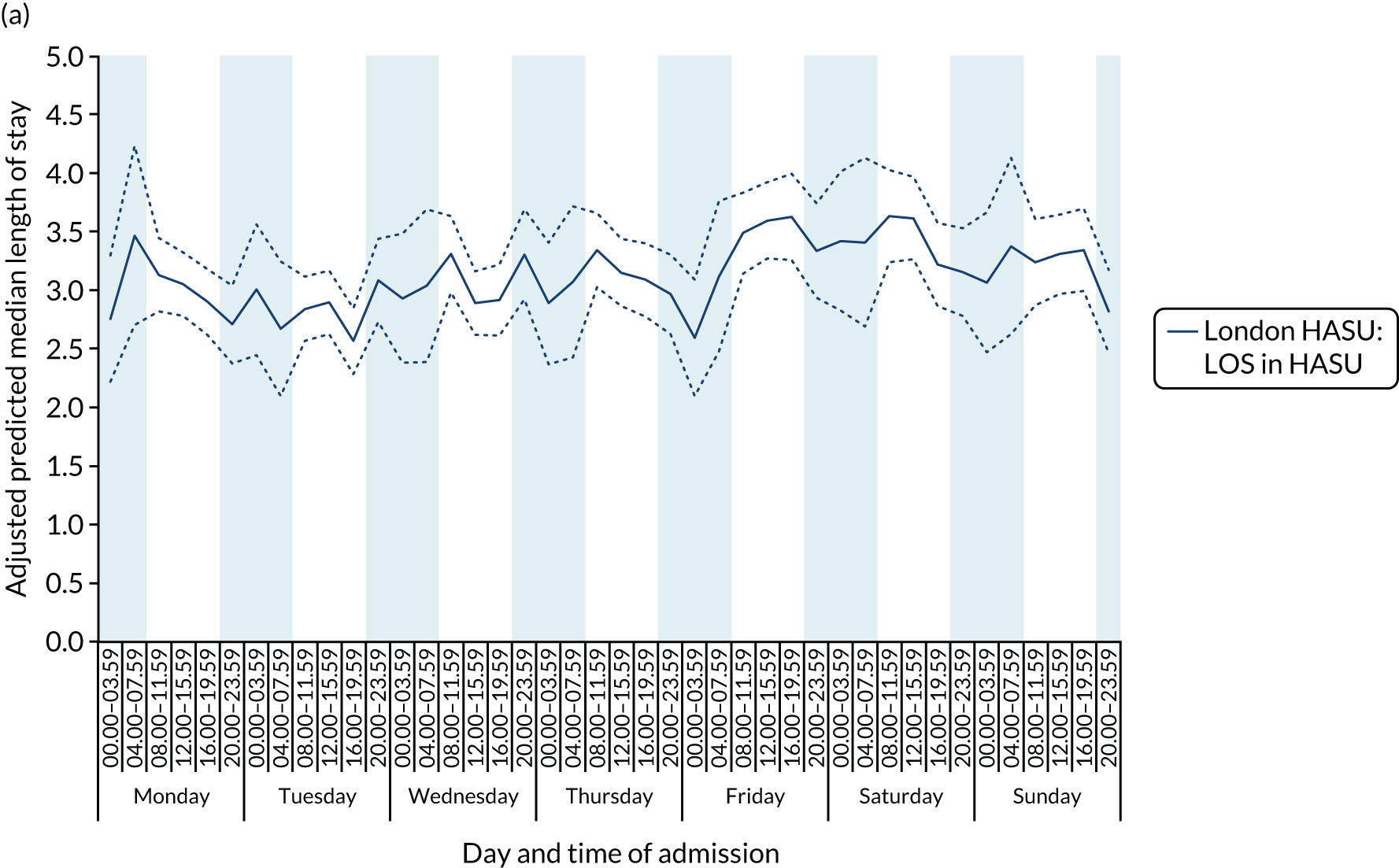
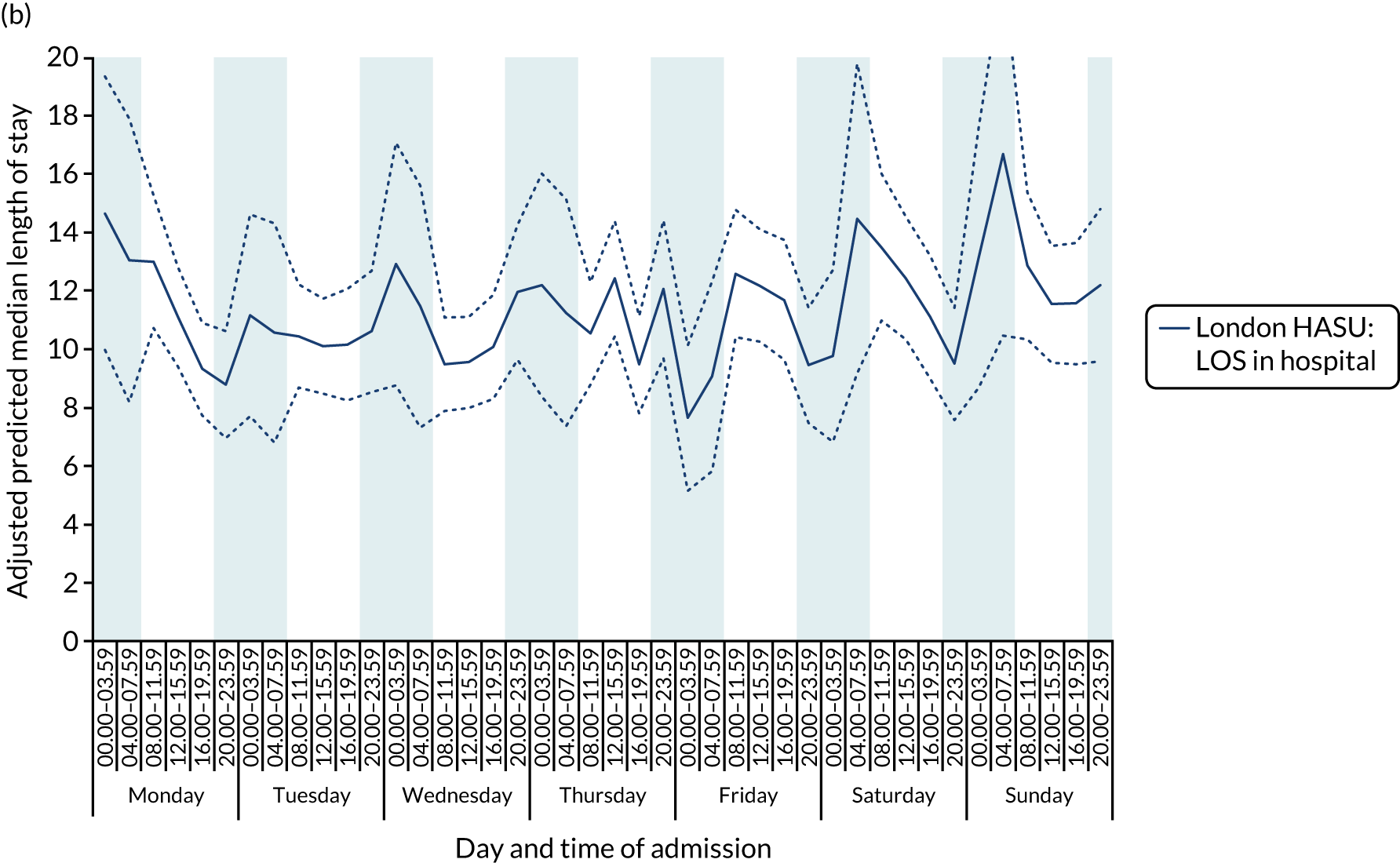
Results using the four time period specifications (Table 6) were broadly similar to those with the 42 time periods, but pooling time periods meant that the extent of variation during the week for some of the quality-of-care measures was reduced (see Report Supplementary Material 2, Tables S1 and S2, for unadjusted figures and p-values, respectively). In these analyses, there was no significant variation in London HASUs in quality-of-care measures linked to specialist stroke nurse assessments, rapid access to brain scans and administration of thrombolysis to eligible patients for London HASUs, nor was there in the outcome measures. LOS varied significantly for London HASUs: pooling time periods more clearly indicates longer LOS among patients admitted at the weekend.
Measures | 08.00–19.59 | 20.00–07.59 | p-value | ||
---|---|---|---|---|---|
Weekday | Weekend | Weekday | Weekend | ||
Quality-of-care measures that do not vary across the week | |||||
Brain scan within 1 hour | 0.60 (0.58 to 0.61) | 0.61 (0.58 to 0.63) | 0.63 (0.60 to 0.65) | 0.65 (0.61 to 0.68) | 0.0344 |
Brain scan within 12 hours | 0.97 (0.96 to 0.97) | 0.96 (0.95 to 0.97) | 0.95 (0.94 to 0.96) | 0.95 (0.93 to 0.96) | 0.0093 |
Dysphagia screen within 4 hours | 0.74 (0.72 to 0.75) | 0.75 (0.73 to 0.77) | 0.77 (0.75 to 0.79) | 0.79 (0.76 to 0.82) | 0.0029 |
Assessment by a nurse trained in stroke management within 24 hours | 0.96 (0.95 to 0.96) | 0.94 (0.93 to 0.96) | 0.95 (0.94 to 0.96) | 0.95 (0.94 to 0.97) | 0.1872 |
Administration of intravenous thrombolysis to eligible patients | 0.88 (0.86 to 0.90) | 0.88 (0.84 to 0.92) | 0.86 (0.82 to 0.91) | 0.88 (0.82 to 0.95) | 0.9327 |
Door-to-needle time < 1 hour in patients receiving thrombolysis | 0.84 (0.81 to 0.87) | 0.89 (0.85 to 0.93) | 0.79 (0.74 to 0.84) | 0.84 (0.77 to 0.91) | 0.0269 |
Quality-of-care measures that vary significantly across the week | |||||
Assessment by a stroke specialist consultant physician within 12 hours | 0.39 (0.38-.40) | 0.30 (0.27 to 0.32) | 0.63 (0.61 to 0.66) | 0.64 (0.60 to 0.68) | < 0.0001 |
Assessment by a stroke specialist consultant physician within 24 hours | 0.90 (0.89 to 0.91) | 0.87 (0.85 to 0.89) | 0.90 (0.88 to 0.91) | 0.92 (0.90 to 0.94) | 0.0043 |
Admission to a stroke unit within 4 hours | 0.62 (0.60 to 0.63) | 0.64 (0.61 to 0.66) | 0.67 (0.65 to 0.70) | 0.70 (0.67 to 0.74) | < 0.0001 |
Physiotherapist assessment within 72 hours | 0.83 (0.82 to 0.84) | 0.86 (0.84 to 0.88) | 0.85 (0.83 to 0.87) | 0.84 (0.81 to 0.87) | 0.0693 |
Occupational therapist assessment within 72 hours | 0.79 (0.78 to 0.80) | 0.82 (0.80 to 0.84) | 0.81 (0.79 to 0.82) | 0.80 (0.76 to 0.83) | 0.0967 |
Dysphagia assessment by a SLT within 72 hours | 0.92 (0.91 to 0.93) | 0.93 (0.91 to 0.95) | 0.93 (0.91 to 0.95) | 0.91 (0.88 to 0.95) | 0.5838 |
Communication assessment by a SLT within 72 hours | 0.53 (0.51 to 0.54) | 0.56 (0.54 to 0.59) | 0.55 (0.53 to 0.58) | 0.52 (0.48 to 0.56) | 0.0739 |
Physiotherapist assessment within 24 hours | 0.56 (0.54 to 0.57) | 0.47 (0.45 to 0.50) | 0.65 (0.63 to 0.68) | 0.48 (0.44 to 0.52) | < 0.0001 |
Occupational therapist assessment within 24 hours | 0.49 (0.47 to 0.50) | 0.42 (0.40 to 0.45) | 0.58 (0.55 to 0.60) | 0.41 (0.37 to 0.45) | < 0.0001 |
Communication assessment by a SLT within 24 hours | 0.29 (0.28 to 0.31) | 0.22 (0.20 to 0.24) | 0.39 (0.37 to 0.42) | 0.23 (0.20 to 0.27) | < 0.0001 |
Outcome measures | |||||
Mortality at 3 days | 0.03 (0.02 to 0.03) | 0.03 (0.02 to 0.04) | 0.03 (0.02 to 0.04) | 0.02 (0.01 to 0.03) | 0.3310 |
mRS score 3–6 | 0.55 (0.53 to 0.56) | 0.55 (0.52 to 0.57) | 0.55 (0.52 to 0.57) | 0.56 (0.53 to 0.59) | 0.8672 |
mRS score 3–5a | 0.49 (0.47 to 0.50) | 0.47 (0.45 to 0.50) | 0.48 (0.45 to 0.50) | 0.48 (0.44 to 0.51) | 0.7497 |
LOS | |||||
LOS in HASU (median days) | 3.1 (3.0–3.2) | 3.4 (3.2–3.5) | 3.0 (2.9–3.1) | 3.1 (2.9–3.3) | 0.0007 |
LOS in hospital (median days) | 10.8 (10.2–11.3) | 12.1 (11.1–13.1) | 10.8 (10.0–11.7) | 11.5 (10.2–12.9) | 0.0359 |
Cost analysis
Results were similar when controlling for NIHSS score on arrival at hospital, instead of level of consciousness, on the smaller sample of patients with non-missing NIHSS data: results with p-values < 0.05 and trends across the week were unchanged (see Report Supplementary Material 2, Figures S1–S6 and Table S3).
Variations in the non-time-dependent quality-of-care measures by the four time periods are shown in Figure 10).
FIGURE 10.
Non-time-dependent quality-of-care measures across four periods in the week in London HASUs. Figures are average predicted probabilities of each measure in each time period controlling for the covariates. We tested for statistically significant variations across the four time periods using Wald tests and reported the results as joint p-values under the null hypothesis that the regression coefficients for every time period relative to the omitted time period were zero (p-values in parentheses). All patients received a brain scan in every time period. n/a, not applicable.
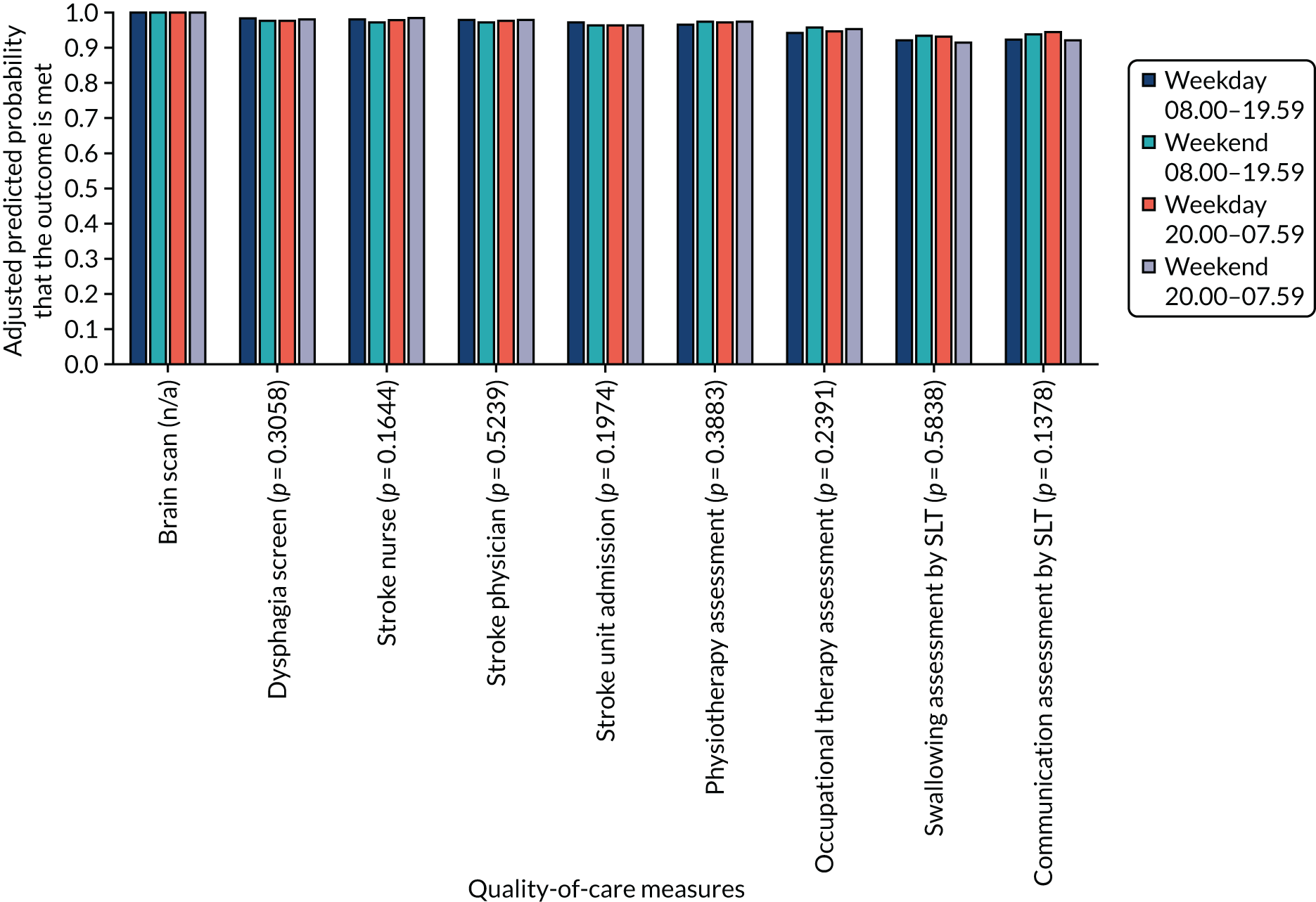
In contrast to the findings for the time-dependent versions of these measures in Table 6, Figure 10 shows that > 90% of patients receive every measure and that there is little variation across the four time periods. We also note from Table 6 that there is no variation in administration of intravenous thrombolysis across the four time periods. We conclude from these data that the costs of acute care in London HASUs, with respect to these measures, is unlikely to vary on different days and at different times of admission. Table 6 shows significant variation in median LOS for London HASUs, with longer HASU and total LOS among patients admitted at the weekend. Table 7 shows a similar trend for mean LOS (note that the mean values in Table 7 are higher than the corresponding median values in Table 6, indicating skewed data).
LOS | 08.00–19.59 | 20.00–07.59 | p-value | ||
---|---|---|---|---|---|
Weekday | Weekend | Weekday | Weekend | ||
LOS in HASU (mean days) | 4.1 (4.0 to 4.2) | 4.5 (4.3 to 4.7) | 4.0 (3.9 to 4.2) | 4.1 (3.9 to 4.4) | 0.0011 |
LOS in hospital (mean days) | 23.8 (22.4 to 25.3) | 26.9 (24.6 to 29.2) | 24.0 (22.1 to 26.0) | 25.6 (22.5 to 28.7) | 0.0359 |
In Table 8 we compute the cost implications of the difference in LOS. Focusing on differences in mean LOS, patients who were admitted between 08.00 and 19.59 at the weekend were, on average, £166 more costly to treat in the HASU (£668 when including total LOS) than those admitted between 08.00 and 19.59 on a weekday. Those who were admitted between 20.00 and 07.59 at the weekend were on average no more costly than those admitted between 08.00 and 19.59 on Monday to Friday (£445 more costly when including total LOS). For those admitted between 20.00 and 07.59 on a weekday, the cost difference per patient was small compared with those admitted between 08.00 and 19.59 on a weekday.
Differences in LOS (days) | Unit cost per day (£) | Differences in costs (£) | |||||||
---|---|---|---|---|---|---|---|---|---|
08.00–19.59 | 20.00–07.59 | 08.00–19.59 | 20.00–07.59 | ||||||
Weekday | Weekend | Weekday | Weekend | Weekday | Weekend | Weekday | Weekend | ||
Based on median LOS | |||||||||
HASU | 0.3 | –0.1 | 0 | 415 | 124 | –41 | 0 | ||
Total hospital minus HASU | 1 | 0.1 | 0.7 | 247 | 247 | 25 | 173 | ||
Sum (total LOS) | 1.3 | 0 | 0.7 | 372 | –17 | 173 | |||
Based on mean LOS | |||||||||
HASU | 0.4 | –0.1 | 0 | 415 | 166 | –41 | 0 | ||
Total hospital minus HASU | 2.7 | 0.3 | 1.8 | 247 | 668 | 74 | 445 | ||
Sum (total LOS) | 3.1 | 0.2 | 1.8 | 834 | 33 | 445 |
Discussion
This section is based on a paper by Melnychuk et al. 65 This is an Open Access article distributed in accordance with the terms of the Creative Commons Attribution (CC BY 4.0) license, which permits others to distribute, remix, adapt and build upon this work, for commercial use, provided the original work is properly cited. See: http://creativecommons.org/licenses/by/4.0/. The text below includes minor additions and formatting changes to the original text.
Principal findings
In our study, we found no evidence for an admission effect across the week on early outcomes in acute stroke patients admitted to a London HASU: 3-day mortality and mRS score at hospital discharge did not vary by day and time of admission in London HASUs. This is consistent with a recent study based on administrative data in the UK. 34 The study34 found a steady reduction in in-hospital mortality difference between weekday and weekend stroke admissions in 2008–14 across England, and that this difference is no longer statistically significant in 2014.
There was also no variation by day and time of admission across the week in terms of rapid access to brain scanning, stroke nursing care and thrombolysis in London HASUs. Other quality-of-care measures did significantly vary across the week in London HASUs and three patterns of variation were detected: (1) by time of day, but not day of the week; (2) by day of the week, but not time of day; and (3) by time of day and day of the week. LOS was longer among patients admitted to London HASUs at the weekend. We hypothesised that there would be non-significant variation across the week in care quality measures in London HASUs and that this would translate into non-significant variations in outcomes. The lower variation in care quality measures across the week in London HASUs was confirmed, but only with respect to ‘front-door’ measures of acute stroke care. With respect to the health outcomes, there was no variation in mortality at 3 days and disability at hospital discharge by day and time of admission across the week in London HASUs. This is consistent with previous studies showing that timely access to thrombolysis is associated with good stroke outcomes. 70
We found that patients who were admitted at the weekend were on average more costly than those who were admitted during the week because of their longer LOS. When incorporating total LOS, the additional mean cost per patient was £445–668, depending on when the patient was admitted at the weekend. Those who were admitted between 20.00 and 07.59 on a weekday had similar costs to those who were admitted between 08.00 and 19.59 on a weekday.
Strengths and weaknesses
The main strength of our study is the rich data set we have used, containing detailed information on quality of care, outcomes and patient characteristics. We have examined whether or not time of admission was related to quality of care using a comprehensive set of indicators from across the acute stroke care pathway. Most of the measures were from a pre-existing set of national acute stroke care indicators, and those that were added had more stringent time constraints to reflect the time-critical nature of acute stroke care. Our outcomes were stroke mortality and disability, whereas previous studies have focused on mortality. 6,25,34,71–73 The rich set of patient characteristics in the data set meant that we could control for patient factors likely to affect quality of care and outcomes that vary by day and time of admission across the week. There are several weaknesses. First, although case ascertainment in the SSNAP was 90% during the time period of our study, these data might not be representative of all stroke patients. Second, although analyses of hospital administrative data to investigate weekend effects in stroke may have been undermined by evidence of variations in inaccurate coding across the week,58 in the SSNAP data are inputted voluntarily by hospitals and we cannot exclude the possibility of inaccurate or selective reporting. Particularly problematic for our study would be if this bias was more likely to vary by time of admission. Third, we were unable to measure long-term outcomes, as these were not available in the SSNAP. Mortality data in the SSNAP are currently available only for patients who are in hospital and, therefore, to reduce the risk of bias, we measured mortality at 3 days after admission, when most patients will still be in hospital. Three-day mortality has been used in previous studies to evaluate the centralisation of acute stroke services in London,35 but the focus in our study on in-hospital mortality only is a further limitation. Similarly, long-term disability data are not reliably collected in the SSNAP, and so disability was measured by the mRS at the end of the inpatient spell. Fourth, although the richness of our data set means that we have been able to control for confounding factors, we cannot exclude the possibility of confounding due to unobserved patient characteristics or staffing levels. Fifth, although the sample size of our study is relatively large (n = 7094), when evaluating quality of care and outcomes across the week the number of observations in each of the 42 time periods was considerably smaller (mean of 169). We cannot exclude the possibility that this number of patients resulted in wide confidence intervals (CIs) around the adjusted predicted probabilities in each time period, making it less likely to show significant variation in the measures evaluated. It is difficult to provide sample size calculations for statistical analyses between 42 groups (time periods). However, when we re-ran our analyses of quality of care and outcomes across the four pooled time periods in the week in London HASUs, the results were broadly similar, and this provides some reassurance about the statistical power of our analyses. Sixth, we acknowledge that previous studies have identified that improvements in outcomes following acute stroke in England have been shown to be statistically unrelated to the centralisation of stroke services in London, but are consistent with an overall national focus on improving stroke services. 34 Further research examining whether or not the findings seen in the present study for London HASUs were also achieved in the rest of England would be beneficial.
Comparison with other studies
There is a large literature examining weekend effects in health care across a range of clinical areas. 74 In acute stroke, there is conflicting evidence on whether patients admitted at weekends have higher or lower quality of care and better or worse outcomes. 6,9,40,71,72,75 However, recent analyses have shown that care quality and outcomes in acute stroke vary across the week, and that comparing weekend with weekday or in-hours effects with out-of-hours effects is flawed, as it does not take into account variations by day of the week and time of day. 22 This study,22 using the same data set as ours, but from an earlier time period and analysing the whole of England and Wales, found that quality of care varied across the entire week, not only between weekends and weekdays, with a number quality-of-care measures showing different patterns of variation over the week. What our study adds is analyses of variation in quality of care and outcomes in London HASUs by separately following the centralisation of acute stroke services in London in 2010, which has been shown to increase the quality of care and outcomes overall. 35,38,41
Implications
There are several implications of our study. The first is that London HASUs appear to operate a uniform service across the week with respect to some, but not all, aspects of acute stroke care. Performance standards originally set by Healthcare for London stipulated that London HASUs should operate a 24/7 service with respect to first assessment by a stroke nurse, rapid access to brain scans and administration of thrombolysis to eligible patients; our findings show that London HASUs do operate a 24/7 service with respect to these measures. However, for other less time-critical measures, such as senior stroke physician assessment within 24 hours and therapist assessments within 72 hours, we found significant variation by day and time of admission in London HASUs. This suggests that some performance standards, such as ‘front-door’ interventions, may be emphasised more than others. The second implication is that our study focuses only on patients admitted to London HASUs and not other hospitals in London; our data suggest that 6% of acute stroke patients in London are not treated in a HASU. However, some of these patients will not have been eligible for HASU care because of greatly delayed presentation or identification of stroke, and others will have had a stroke after surgical procedures or in another context which precluded their admission to a HASU. Our focus on London HASUs was deliberate, as the aim of our study was to evaluate the HASU model. However, it means that our findings for London HASUs should not be generalised to all patients in London. Indeed, there is evidence that quality of care is lower for acute stroke patients in London not treated in a HASU than for those who are. 38 Second, the London model may not apply to services operating in other areas, in particular rural settings, where the greater travel times make centralisation of this kind challenging. 76 This means that potential benefits of the London model, in terms of 24/7 care, are unlikely to be achieved nationwide. Third, the centralisation of acute stroke services in London was estimated to occur at an additional cost of £20M, allocated to cover the increased cost per bed-day in a HASU. 77 With this additional level of funding it might be expected that the quality of care in London should improve, although whether or not it should produce less variation in quality of care and outcomes across the week in London is unclear. There is some evidence that the reorganisation in London was cost-effective,67,68 but further analyses accounting for the size of the upfront investment, the relatively high costs per day of hyperacute stroke care, the impact on mortality and disability, and the lifetime costs incurred by the NHS, social services and families caring for stroke survivors at different levels of disability, would be helpful.
We evaluated the costs of acute stroke care in London HASUs on different days and at different times of admission. We found that patients who were admitted at the weekend were on average more costly than those who were admitted during the week, due to their longer LOS. Those who were admitted between 20.00 and 07.59 on a weekday had similar costs to those who were admitted between 08.00 and 19.59 on a weekday.
Chapter 4 What does it take to provide clinical interventions with temporal consistency? A qualitative study of London hyperacute stroke units
What is known about this topic?
-
Hyperacute stroke units display no variation by day or time of admission in 3-day mortality or disability at hospital discharge, and no variation by day or time of admission in delivery of ‘front-door’ interventions, such as stroke nursing assessment, brain scanning and thrombolysis measures.
-
Hyperacute stroke units show significant variations by day and time of admission in other interventions (including timely consultations and assessments with stroke specialists and therapies, and length of hospital stay).
What this analysis adds
-
Consistency is achieved by adapting and extending roles, creating continuities between different times of day, building relationships and trust, and prioritisation of ‘front-door’ interventions. However, these adaptations have unintended consequences.
-
Variation persists due to inconsistencies in medical, managerial and allied health professional staffing by time of day, delivery of therapist assessments and barriers in repatriation processes.
Introduction
Seven-day provision of consistent, high-quality, urgent and emergency care settings is an international research and policy priority. 9,11,29,61,78,79 This has motivated 15 years of attention on the ‘weekend effect’ and other temporal variations,22 scrutinising mortality and other outcomes for those admitted at the weekend compared with those admitted during the week. 6,7,9,31,80–83 Varying features that may explain differential outcomes include staffing levels31 and patient mix,25 leading to widespread interest in studying weekend organisational features. 22,84
In Chapter 3 we showed that HASU patients’ mortality and disability outcomes are consistent according to the time of their admission. However, there were some variations in the delivery of care. In this chapter, we set out to explore how acute care interventions are delivered with temporal consistency, and why some care interventions are resistant to temporal consistency, by examining the organisation of services at different times of day and week.
Methods
This section is based on a paper by Black and Ramsay et al. 85 This is an Open Access article distributed in accordance with the terms of the Creative Commons Attribution (CC BY 4.0) license, which permits others to distribute, remix, adapt and build upon this work, for commercial use, provided the original work is properly cited. See: http://creativecommons.org/licenses/by/4.0/. The text below includes minor additions and formatting changes to the original text.
Our methods are reported in accordance with COnsolidated criteria for REporting Qualitative studies (COREQ) reporting guidelines. 49
Recruitment and sample
Our recruitment procedures are detailed in Chapter 2, Recruitment and consent. The particular data sample relating to this chapter is described in Tables 2 and 3.
Non-participant observations
We conducted non-participant observations at least four times at each HASU site, two visits on weekdays, one in the evening during the week and one at the weekend, with additional visits to confirm or add to our findings. We collected data on various aspects of HASU activity likely to influence care provision, guided by clinical interventions in our quantitative analysis and our initial observations (Table 9).
Activities observed | Total (of eight sites) |
---|---|
‘Front-door’ activity | 7/8 |
Ward round | 6/8 |
MDT meeting | 7/8 |
16.00 catch-up meeting | 3/4a |
Nurse handover | 8/8 |
Bed meeting | 6/8 |
Discharge | 4/8 |
Total conducted | 41 |
Purposive sampling of observations is described in Methods.
We initially structured observations so that availability of staff and key processes (e.g. handover and ward rounds) were covered. Subsequently, we targeted our observations towards our emerging analysis, such as following patients’ journeys from the ED to the HASU ward, and shadowing the nurse in charge and consultants to understand their roles.
We obtained global written consent for observations from service leads and from a selection of staff at team meetings. Thereafter, verbal consent was given by staff members for researchers to make observations of staff meetings and of care provision.
Interviews
Staff were sampled purposively to ensure coverage of a range of professional roles within all eight HASUs, with perspectives on the clinical interventions65 (e.g. interpreting brain scans, thrombolysis, therapy assessments), including medical, nursing, therapy, and administrative or managerial staff (see Chapter 2, Sampling).
Researchers approached staff during the observation work and through existing contacts with study information, stating that participation would be confidential and anonymously presented in publications. Interviews were conducted with fully informed, written consent and in private settings, according to a semistructured topic guide (see Report Supplementary Material 1), and with the aim of understanding temporal variations from the staff perspective, including typical daily activities and attitudes towards working in and out of hours. Interviews lasted between 20 minutes and 1 hour. No field notes were taken, but each interview was discussed with the other team members. Interviews were audio-recorded and transcribed verbatim.
Analysis
Quality and trustworthiness of the analysis were maintained through a select number of joint observations and interviews, to sensitise ourselves to pertinent contextual issues, and regular reflexive discussions between the researchers. Our five-step analysis process is outlined in Chapter 2, Qualitative analysis. For this chapter, we mapped the results against the variations in practice across the 7-day week found in our analysis of the performance data for care provision in London HASUs set out in Chapter 3. In particular, our analysis focused on the distinction between ‘front-door’ clinical interventions (which were provided consistently) and other interventions (which were not).
Results
This section is based on a paper by Black and Ramsay et al. 85 This is an Open Access article distributed in accordance with the terms of the Creative Commons Attribution (CC BY 4.0) license, which permits others to distribute, remix, adapt and build upon this work, for commercial use, provided the original work is properly cited. See: http://creativecommons.org/licenses/by/4.0/. The text below includes minor additions and formatting changes to the original text.
London HASUs deliver ‘front-door’ clinical interventions, including brain scans, thrombolysis and dysphagia assessment, without significant temporal variation. 65 LOS showed significant variation without a clear temporal trend, assessment by a stroke physician within 12 or 24 hours varied by time of day and assessments by therapists varied by the day of the week. Care quality was worse for patients admitted on a Friday.
In the following sections, we present (1) factors that may explain why ‘front-door’ interventions showed temporal consistency, as well as some unintended consequences of the strategies London HASUs employed; and (2) factors that may explain why other interventions and outcomes showed significant temporal variations (Table 10).
Theme | Impact | |
---|---|---|
Factors influencing temporally consistent care:
|
Adapting and extending roles |
|
Creating continuities between different times of day |
|
|
Building relationships and trust |
|
|
Prioritisation of ‘front-door’ interventions |
|
|
Unintended consequences of adaptations |
|
|
Factors influencing temporally inconsistent care:
|
Variations in medical, managerial and allied health professional staffing by time of day |
|
Variations in delivering therapist assessments |
|
|
Variations in repatriation processes |
|
Factors influencing temporally consistent care
We found that there was no variation between the HASUs in terms of adherence to the London stroke service standards. The London stroke service standards required that HASUs have 24/7 availability of staff who are trained to assess eligibility for and deliver thrombolysis; this was normally completed by a team of medical and nursing staff who were on standby to immediately attend patients at the ED when alerted to new potential thrombolysis patients by the ED team (Figure 11).
FIGURE 11.
Thrombolysis pathway. This figure is adapted from Black and Ramsay et al. 85 This is an Open Access article distributed in accordance with the terms of the Creative Commons Attribution (CC BY 4.0) license, which permits others to distribute, remix, adapt and build upon this work, for commercial use, provided the original work is properly cited. See: http://creativecommons.org/licenses/by/4.0/. The figure includes minor additions and formatting changes to the original figure.
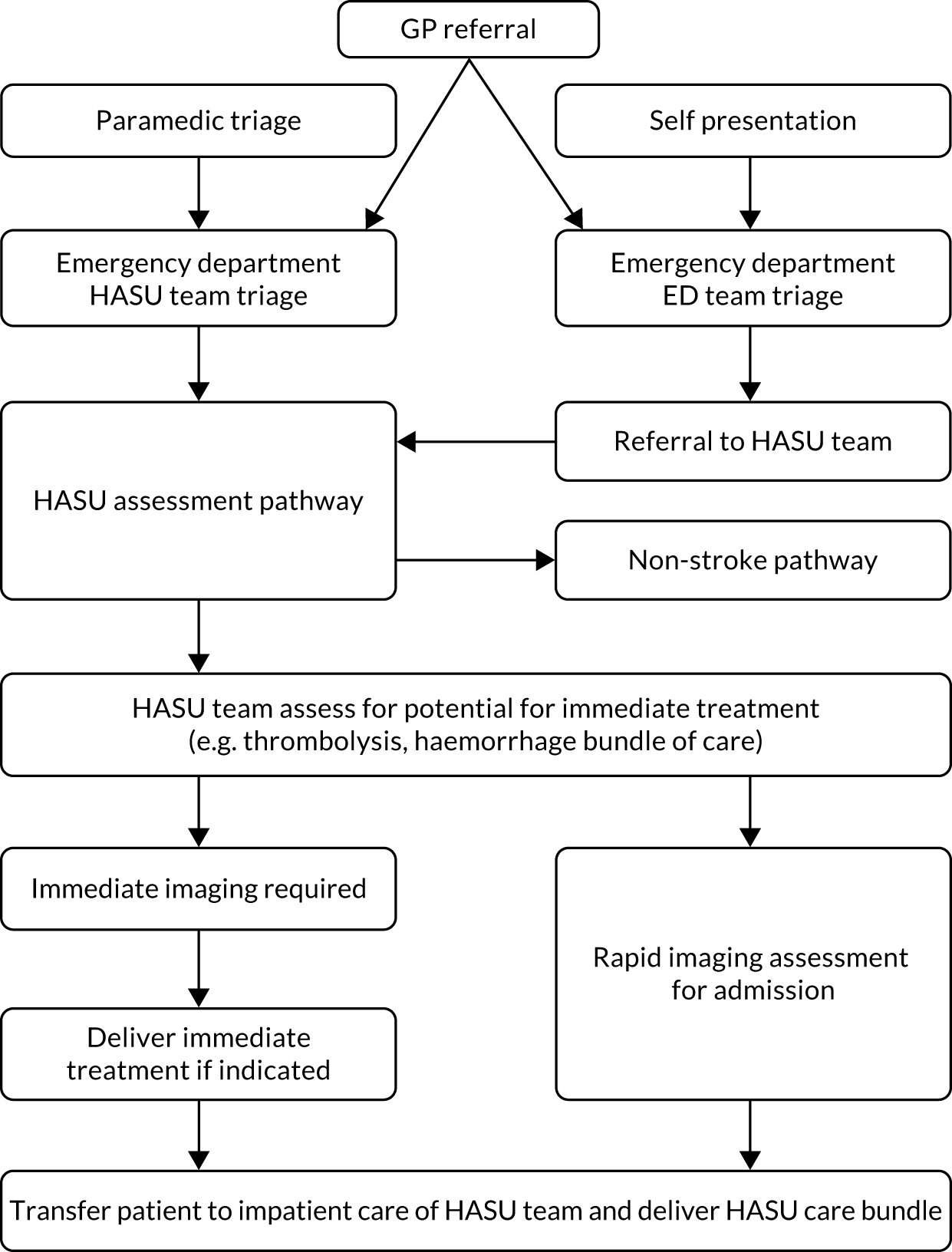
Thrombolysis was administered by the stroke team when appropriate, after a battery of clinical and imaging tests had been conducted. The time taken to complete this process is one of the core performance metrics by which each treating unit is measured. Each HASU attempted to make this pathway as efficient as possible, by having a designated ‘thrombolysis team’ irrespective of clinical activity elsewhere in the stroke service and through local arrangements with radiology, to guarantee that 100% of stroke patients potentially eligible for thrombolysis were scanned within the next CT scan slot. However, as detailed in Table 11, the staff supporting ‘front-door’ activity reduced both in number and in seniority in the evenings and at the weekends. As a result, the HASU teams made significant adaptations out of hours, including extending roles and responsibilities, and introducing processes to ensure continuity between different times of day.
HASU site ID | Weekday | Weekend | Weekday or weekend | |||||||||
---|---|---|---|---|---|---|---|---|---|---|---|---|
09.00–17.00 | 09.00–17.00 | 17.00–21.00 | 21.00–09.00 | |||||||||
Medical | Nursing | Scans | Medical | Nursing | Scans | Medical | Nursing | Scans | Medical | Nursing | Scans | |
H1 | C; SpR | CNS | MRI; Doppler | SpR; SHO | B6 | MRI; CTA | SpR | NIC | MRI; Doppler | SHO | B6 | |
H2 | C; SHO | NIC | MRI; Doppler | SHO | B6 | SpR/SHO | NIC | MRI; Doppler | SHO | B6 | ||
H3 | C; sR; SHO | CNS | MRI; Doppler; echocardiogram | sR; SHO; L | NIC | MRIa | SpR; SHO | CNS | MRI; Doppler; echocardiography | nR; SHO/L | NIC | MRIb |
H4 | SpR | CNS/B6 | MRI; Doppler | SpR; SHO | B6 | Doppler | nR/sR; SHO | B6 | MRI; Doppler | nR/sR; SHO | B6 | |
H5 | C; SHO | CNS/NIC | MRI; Doppler | SHO | CNS/NIC | MRI | SHO | B6 | MRI; Doppler | SHO | NIC | CTA |
H6 | C; fellow; SHO | B6 | MRI; Doppler; echocardiogram | SpR | CNS | CTA | SpR; SHO | NIC | MRI; Doppler; echocardiography | SpR | NIC | |
H7 | SpR; SHO | B5/B6/B7 | MRI;a CTA | SpR; SHO | B5/B6/B7 | MRIa | SpR; SHO | B5/B6/B7 | MRI;a CTA | SpR; SHO | B5/B6/B7 | |
H8 | SpR | B6 | MRI;a Doppler | SpR | B6 | MRI | SpR | B6 | MRI;a Doppler | SpR | B6 |
Adapting and extending roles
At all times of day, stroke specialist registrars and senior house officers (junior doctors) made decisions about thrombolysis with consultant telephone support (for additional data see Report Supplementary Material 2, Theme 1a). However, observations and interviews confirmed that, in some HASUs, at night, the consultant was called for positive confirmation only if the registrar thought a patient eligible for thrombolysis. This was seen as a positive educational opportunity for junior doctors, but it placed greater responsibility on senior nurses to decide whether or not to admit the patient to the HASU in cases when the stroke diagnosis was unclear.
Creating continuities between different times of day
Rapid movement of patients through the hyperacute stroke pathway required quick resolution of problems, and transfer of detailed information between team members who work at different times of the day and week (see Report Supplementary Material 2, Theme 1b). Key mechanisms took the form of handover meetings, MDT meetings and ward rounds:
Bay nurse leads on his patients, going through the discharge sheet that everyone has and focuses on any particular issues that have arisen, or that the next nurse needs to be aware of [. . .]
Evening observation, H4
Other ways of creating continuity included shifting or staggering staff rotas (to bridge gaps between shifts) and extending therapists’ hours into the early evening.
Building relationships and trust
Delivering ‘front-door’ interventions consistently depended on rapid decision-making, an important facilitator of which was the development of strong and trusting relationships with allied disciplines, in particular with the ED and neuroradiology (see Report Supplementary Material 2, Theme 1c).
Hyperacute stroke unit staff worked alongside ED staff to assess the patient and there were often overlaps when a patient’s diagnosis was unclear or when multiple patients presented at once. HASU staff felt that ED clinicians also valued this close relationship:
We are popular with the ED team [. . .] we are one of the few teams where you’ve got a consultant down there sweating away with them.
Consultant physician, H2
Hyperacute stroke unit staff also had an important relationship with radiology, with prioritised access to CT scans as outlined in the London stroke service standards. The HASU team often conducted initial interpretation of CT scans, which reduced decision-making delays. HASU staff felt that this self-sufficiency strengthened their relationship with ED and radiology staff.
Prioritisation of ‘front-door’ interventions by staff
Hyperacute stroke unit staff’s enthusiasm was an important facilitator of sustained performance in delivering ‘front-door’ interventions (see Report Supplementary Material 2, Theme 1d). Interviews and observations (e.g. of the urgency with which staff responded to calls to attend the ED) suggested that HASU staff relished the early stages of acute stroke care and the potential to see rapid positive outcomes:
When the stroke happens we have to work fast: run to ED, do everything within four hours to ensure that the patient can be thrombolysed, and I’ve seen the patient like almost dead [. . .] we were able to save the patient’s life because immediately we were able to assess, go for CT, thrombolyse the patient [. . .] to me it’s great work.
Stroke nurse, H2
Unintended consequences of adaptations
Although extending roles out of hours was seen as an opportunity for staff development, some interviewees felt that the threshold for admission to the HASU was weakened (see Report Supplementary Material 2, Theme 1e). Junior doctors were seen as more risk averse than consultants, thus admitting more patients unnecessarily out of hours and in turn placing greater strain on the service at a time when it is particularly difficult to move patients from the HASU:
Some doctors, they will send them in to you, put the pressure on you to take that patient [. . .] in the morning when the consultant sees the patient, this patient has not had a stroke [. . .] once the patient gets in here, it’s difficult to send the patient back to the wards, that becomes a big problem, they’re here one week, they’re still waiting for medics to take the patient over.
Ward manager, H1
Factors influencing temporally inconsistent care
Variations in outcomes and delivery of clinical interventions in London HASUs are summarised in Table 12. We explain these findings in terms of reductions in both the number and the seniority of medical, managerial and allied health professionals out of hours, and reductions in repatriation options out of hours.
Type of variation | Examples |
---|---|
Time of day, but not day of the week | Admission to HASU within 4 hours
|
Day of the week, but not time of day | Therapist (physiotherapist, occupational therapist, SLT) assessments within 72 hours
|
Time of day and day of the week | Therapist assessments within 24 hours
|
Outcome | LOS
|
Variations in medical, managerial and allied health professionals by time of day
Variations in delivery of interventions by time of day but not by day of week (see Table 12) are likely to have been influenced by variations in HASU activity and staffing variations that derived from the London stroke service standards (see Report Supplementary Material 2, Theme 2a).
Although some staffing levels were lower at night, the number of patients arriving also reduced, giving patients a better chance of being admitted to the HASU within 4 hours:
We know less people have strokes overnight [. . .] so we know it’s like going to be quieter, but from a staffing perspective, that’s why we’ve done the 24-hour thing, so there is always those amount of staff on.
Senior nurse, H4
Patients were assessed during the consultant-led ward round, which the standards required to take place daily and which commonly occurred between 09.00 and 12.00. Undergoing consultant assessment within 12 and 24 hours therefore depended on patients reaching the ward during this period. For example, if a patient arrived at 03.00, their first consultant assessment would be likely to take place during that morning’s ward round (≈6-hour wait). If they arrived at 15.00, it would be likely to occur the following morning (≈18-hour wait):
After the ward round has finished and we’ve tidied up a bit, yes, you’re less likely to come back and see a case, unless it was very urgent or some unusual type thing.
Lead consultant, H4
Variations in delivering therapist assessments
Therapists generally worked ‘in-hours’ shifts, so patients arriving at hospital in the morning were unlikely to be assessed until the following morning (see Table 11) after a board round, when the team make daily decisions about individual patients’ care. This meant that patients arriving at hospital between 08.00 and 09.00 were the least likely to be assessed within 24 hours.
The London stroke service standards specified therapy staffing levels that fully covered only 5 days per week and, therefore, HASUs faced a decision on how best to use these limited resources: some chose Monday to Friday because of traditional working patterns on these days (see Report Supplementary Material 2, Theme 2b). The resultant gap in therapist coverage at weekends explained why patients admitted on a Friday were less likely to undergo therapy interventions within 72 hours of arrival (whereas patients admitted at the weekend were more likely to be assessed on the following Monday or Tuesday). Therapists described feeling rushed on a Friday, as they struggled to get through their workload before the weekend:
Yeah, I think it can be very stressful on a Friday, just if patients are going home when there’ve been a few discharges at the same time it can get quite complicated trying to co-ordinate a lot of family members, patients, staff to fill in documentation, social work, making sure a care package has gone in and completing lots of referral forms.
SLT, H2
Other HASUs spread their limited therapy resources into the weekend, which was reported to have a beneficial outcome on discharge figures; however, participants reported a change in priorities. Assessment of new patients dominated, and therapeutic work or talking to families was diminished. Furthermore, by spreading therapist resources into the weekend, HASUs reduced therapist capacity during the week. Therapists in almost all HASUs suggested that existing attempts to cover weekend working resulted in reduced prioritisation of therapeutic activity, suggesting that no current staffing model observed permitted consistently sufficient therapy provision.
Factors influencing length of stay
Patients admitted at the weekend in London had a longer hospital stay. This related to a number of factors (see Report Supplementary Material 2, Theme 2c). As patients admitted at weekends were less likely to be seen by therapists, patients could potentially fail to have their rehabilitation and nutritional needs assessed for 3 days in a row:
If you’re nil by mouth on the Friday when you come in, say at half past four [. . .] you could technically be nil by mouth until Monday.
Occupational therapist, H1
Reduced therapeutic capacity could also delay discharge and, therefore, extend LOS:
[. . .] you think, ‘Well, this person can’t go because they need a physio, and we could have discharged them on the Saturday but they have to wait till Sunday or even Monday, which [. . .] can cause a problem sometimes if we need beds [. . .] and that person’s then spent another day potentially in hospital that they potentially don’t need to.
Occupational therapist, H8
Hyperacute stroke unit staff suggested that social services, care homes, stroke units (acute rehabilitation units) and community rehabilitation units were significantly less likely to accept new cases at weekends. This restricted the timing of discharges from the HASU, often leading to longer stays. Input from social services and early supported discharge (a service designed to accelerate the discharge home of patients in hospital86) was important in ensuring that patients returned home or to care homes quickly once sufficiently recovered. However, social services had extremely limited weekend operation, which prevented liaison during the weekends to prepare packages of care or transfer patients.
Discussion
This section is based on a paper by Black and Ramsay et al. 85 This is an Open Access article distributed in accordance with the terms of the Creative Commons Attribution (CC BY 4.0) license, which permits others to distribute, remix, adapt and build upon this work, for commercial use, provided the original work is properly cited. See: http://creativecommons.org/licenses/by/4.0/. The text below includes minor additions and formatting changes to the original text.
To our knowledge, this is the first qualitative study about the organisation of stroke care with respect to temporal variation. This study reports qualitative data that help explain the findings presented in Chapter 3. Consistent provision of clinical interventions was underpinned by (1) junior nursing and medical staff extending their in-hours responsibilities to cover key decision-making roles, such as that of the thrombolysis nurse; (2) intervening to bridge potential gaps caused by shift-working (staggering rotas, holding meetings to share information); and (3) HASU leadership building trust and respect across staff both within HASUs and with key specialties elsewhere in the hospital (such as the ED and neuroradiology). Key issues leading to temporal variation in care provision included reductions in medical, managerial and allied health professions, and significantly reduced options for repatriation to other acute services and community services, at night and at the weekend. Variations resulted in greater pressure on the ward from low thresholds for admission at night, dilution of staff capacity and bottlenecks in repatriation pathways. Some of these effects were mitigated by strategies to create ward space and expedite discharges on a Friday, but these strategies had a number of unintended negative consequences in terms of patient outcomes.
The London stroke service standards were an important influence on the delivery of clinical interventions, whether consistent or inconsistent. When standards required 24/7 availability of staff (e.g. nurses), aspects of care associated with these staff groups tended to be delivered consistently, regardless of time of day or day of week. When standards required that a key activity was conducted on a daily basis (such as the consultant-led ward round), the likelihood of patients undergoing the associated intervention varied significantly according to when they were admitted. Finally, when standards specified staffing levels to cover only 5 days (as with therapies), it was not possible to provide interventions consistently over 7 days, regardless of local adaptations employed.
The strengths of our study are founded on detailed data collection in each London HASU at different times of day, both during the week and at weekends, providing a rich picture of the realities of organising and providing a high-performing acute care system. Observing and comparing how eight sites organised themselves in different ways to meet the same standards affords generalisability to our results. There were several limitations to the study. First, we did not study any hyperacute stroke services operating within a different service model (whether centralised or non-centralised). The lack of a comparator limited our confidence that our findings explain 24/7 care per se (as compared with a centralised model of care). Second, we did not interview all potentially relevant professions within the studied organisations (e.g. pharmacy, emergency medical practitioners). Therefore, our perspective on important working relationships beyond the HASU was based on HASU staff perceptions, although they were supported by our own observations, particularly of ED co-ordination. Finally, these services develop constantly and some aspects of provision, such as staffing levels both within and beyond the HASUs, are likely to have changed.
Chapter 5 What aspects of nurse staffing influence care delivery and outcomes on a hyperacute stroke unit? Mixed-methods study
What is known about this topic?
-
There is temporal variation in some clinical processes in HASU (see Chapter 3), and no measurable variation in ‘front-door’ processes and patient outcomes.
-
Consistency in ‘front-door’ processes is partly achieved by extending nursing roles at night and at the weekend (see Chapter 4).
-
Senior nursing is perceived to be an important component of achieving high standards of HASU care (see Chapter 4).
What this analysis adds
-
There is variation in the nurse staffing by time of the week, particularly in terms of the seniority of key roles, rather than the number of nurses overall.
-
Senior nursing roles are more differentiated than junior roles, which can be filled by bank nurses and other nursing staff.
-
When senior nurses take on the nurse-in-charge role during the day and during the week, it has an impact on the delivery of clinical interventions.
Introduction
London HASUs reduced variations in the delivery of ‘front-door’ clinical interventions overall41 and at different times of the day and week (see Chapter 3). 34 The London stroke service standards recognise the pivotal role played by stroke nurses in delivering high-quality care and patient outcomes, and, therefore, in reducing this variation. An A1 standard (i.e. a standard that must be met if a service is to be classified and funded as a HASU36,39) requires consistent nurse staffing levels at all times:
Provision of 24/7 nursing workforce to provide: 2.9 WTE [whole-time equivalent] nurses per bed; 80 : 20 trained to untrained skill mix.
NHS England36
However, this standard leaves a degree of flexibility in terms of how nurses are organised in a HASU. For instance, it does not specify nurse leadership roles, the proportion of senior nurses, or the roles and responsibilities of the nurse in charge.
Our analysis in Chapter 3 showed that London HASUs operate a 24/7 service with respect to nursing-related care, rapid access to brain scans and administration of thrombolysis to eligible patients, but not other aspects of care. It also showed that there was no variation among patients treated at London HASUs by day and time of admission across the week in terms of 3-day mortality and mRS score at hospital discharge. It has been suggested that staffing levels, in particular nurse-to-bed ratios, can influence variations in quality of care and outcomes by day and time of admission across the week. 60 Recently, several studies have found that stroke units with higher ratios of nurses trained in dysphagia screening are associated with lower risk of death87 and lower risk of stroke-related pneumonia. 88,89 The measures used in these studies were derived from the SSNAP Organisational Audit, which is based on a one-off snapshot of staffing, carried out once every 2 years, and does not account for day-to-day variation. We used a novel data set of London HASU nurse staffing based on detailed information from nursing rotas for every time of the day in all HASUs in London, applied to the SSNAP data, to examine the relationship between nurse staffing in the HASU and processes of care and outcomes. This was informed and supported by a qualitative analysis of temporal variation of the organisation of stroke care and nursing roles on the HASU. The results from this analysis indicate that nurse staff profile – seniority and separation of roles at the HASU – contributes to the quality of processes management within the ward and there are variations in nurse staffing by day and night and across the week. Subsequently, the aims of the analysis were to investigate (1) how nurse roles on the HASU are organised, (2) whether or not nurse staffing affected outcomes and quality of acute stroke care in London HASUs and (3) whether or not there is variation in those effects by day and time of admission across the week.
Methods
This was a mixed-methods analysis. Data collection and analysis are described in Chapter 2. In Chapter 4, we identified aspects of organisation that are important for care delivery and outcomes, especially the roles of nursing staff on the HASU, including the seniority and specialisation of nursing staff. For every patient who was admitted to a London HASU during the study period, we identified the characteristics of the nurse staffing on the HASU at the time they were admitted to the hospital and tested whether or not these factors were associated with processes and outcomes. The quantitative results were discussed in the light of the qualitative findings, and were then used to explain the variation of acute stroke care and outcomes.
Data and measures
Interview and observational data collection are explained in Chapter 2, Qualitative data collection, as are quantitative nurse staffing data collection and linkage. Each day was divided into 48 30-minute shifts. Every patient admitted within each of these shifts was assigned a corresponding nurse staffing measure during the same time period.
For this analysis, the following quality of care indicators were used, as nurses’ input was essential to meet the standards of care: brain scan within 1 hour, dysphagia screen within 4 hours, admission to a stroke unit within 4 hours, administration of thrombolysis to eligible patients and door-to-needle time. Outcomes were measured as whether or not the patient died within 3 days and disability using the mRS score (0–2 vs. 3–6; moderate, moderately severe, severe disability or death) at the end of the inpatient stay. We also analysed the effect of nurse staffing on LOS in HASU and total LOS in the hospital.
We matched the nurse staffing data set with the SSNAP data set based on the time of admission to the hospital, not the HASU, in line with the way in which clinicians describe the ‘clock start’ of other process measures in the SSNAP audit. As reflected in Chapter 5, many of the processes of care analysed are likely to be delivered in the ED on arrival at the hospital. Note that data were not collected on medical staffing, so we were unable to control for this in our analyses.
To examine trends across the week we used four time periods of time of admission to the hospital, similar to broad trends used in Chapter 3, Data and measures: Monday to Friday, 08.00 to 19.59; Monday to Friday, 20.00 to 07.59; Saturday and Sunday, 08.00 to 19.59; and Saturday and Sunday, 20.00 to 07.59. This is different from previous research in which the only comparison was between weekdays and weekends. 60
Sequential mixed-methods analysis
Qualitative analysis
Interview and observational data analysis methods are summarised in Chapter 4, Analysis. Findings related to nursing seniority, highlighted in Chapter 4, Results, informed the quantitative analysis.
Quantitative analysis
We examined whether or not nurse staffing varied across the week. We tested for statistically significant variations across the four time periods using chi-squared tests.
To evaluate whether or not nurse staffing affected 24/7 access to high-quality acute stroke care, we tested the impact of nurse staffing on the variation in the quality of acute stroke care and outcomes by day and time of admission in London HASUs. We ran three sets of patient-level regression models following the results from qualitative observations. First, we ran exactly the same logistic model for the selected outcomes and quality-of-care measures used in the analysis reported in Chapter 3: Statistical analysis for only London HASUs, including the four time periods and the same baseline patient and case mix covariates. In addition, these models included the nurse staffing variables (band of the nurse in charge, whether or not there was a dedicated thrombolysis nurse who was different from the nurse in charge, total number of nurses on duty in the HASU) plus the number of beds in each HASU, and whether or not the patient was admitted to the HASU during the nursing shift handover period. Handover is the half-hour time period between the day and the night shifts (e.g. 07.30–20.00 and 19.00–08.00) to transfer responsibility and accountability for some or all aspects of patient care. Second, we re-ran these models for each of the four time periods separately. Third, based on input from the qualitative findings showing that in the delivery of ‘front-door’ services patients eligible for tissue plasminogen activator (tPA) are prioritised, we re-ran the models stratified by subgroups of patients eligible and not eligible for thrombolysis at the time of arrival to the hospital.
For each outcome and quality-of-care measure we tested whether or not the nurse staffing variables were significantly different from zero (individually significant in the case of whether or not there was a dedicated thrombolysis nurse who was different from the nurse in charge and the number of nurses on duty in the HASU, and jointly significant for the categories describing the band of the nurse in charge). For the categorical variables (band of the nurse in charge and whether or not there was a dedicated thrombolysis nurse who was different from the nurse in charge) we computed average predicted probabilities for each outcome, controlling for the covariates. For variables included as linear terms (number of nurses on duty in the HASU) we computed average marginal effects showing the increased probability that each outcome would be achieved if the number of nurses on duty increased by one unit.
Using the second and third sets of models, for every measure we also tested whether or not the coefficients on the nursing variables were significantly different between the four time periods and two subgroups, using likelihood ratio tests. This provides an indicator of whether or not nurse staffing affects variations in quality of care and outcomes by time of admission and by subgroups in London HASUs.
Results
Our results present a multifaceted analysis of how senior nurses contribute to HASU processes and patient outcomes. First, we outline the difference between junior and senior nurses in terms of tasks on the ward, and give contextual information about where they conducted these tasks.
Nursing roles on the hyperacute stroke unit
Junior nursing roles on the hyperacute stroke unit
In our data, the average daily number of patients arriving Monday to Friday was 2.2 in hours and 1.4 out of hours. Arriving Saturday to Sunday, the average daily number of patients was 2 in hours and 1.4 out of hours. The London stroke service standards specified a certain number of nurses per HASU bed and specified that these were to be provided on a consistent (24/7) basis. This created a sense of continuity and a consistency in the level of bedside care. The level of seniority was not specified. In some HASUs the nurses themselves were assigned to a specific room or number of rooms, making the link between beds and nurses concrete. These nurses were broadly known as ‘junior nurses’.
Junior nurses engage in activities on the HASU that mainly involve direct patient care with some administrative duties. A list of junior nurse roles in the HASU, as evidenced by our interview and observation data, is provided in Table 13.
Role | Task |
---|---|
Administration |
|
Patient assessment and care planning |
|
Stroke nursing |
|
Supervision |
|
Therapy activities |
|
Traditional nursing |
|
Senior nursing roles interacting with the hyperacute stroke unit
There were other nursing roles, however, that are not tied to bed numbers, and these have specific functions (Figure 12).
FIGURE 12.
Senior nursing roles and working sites.
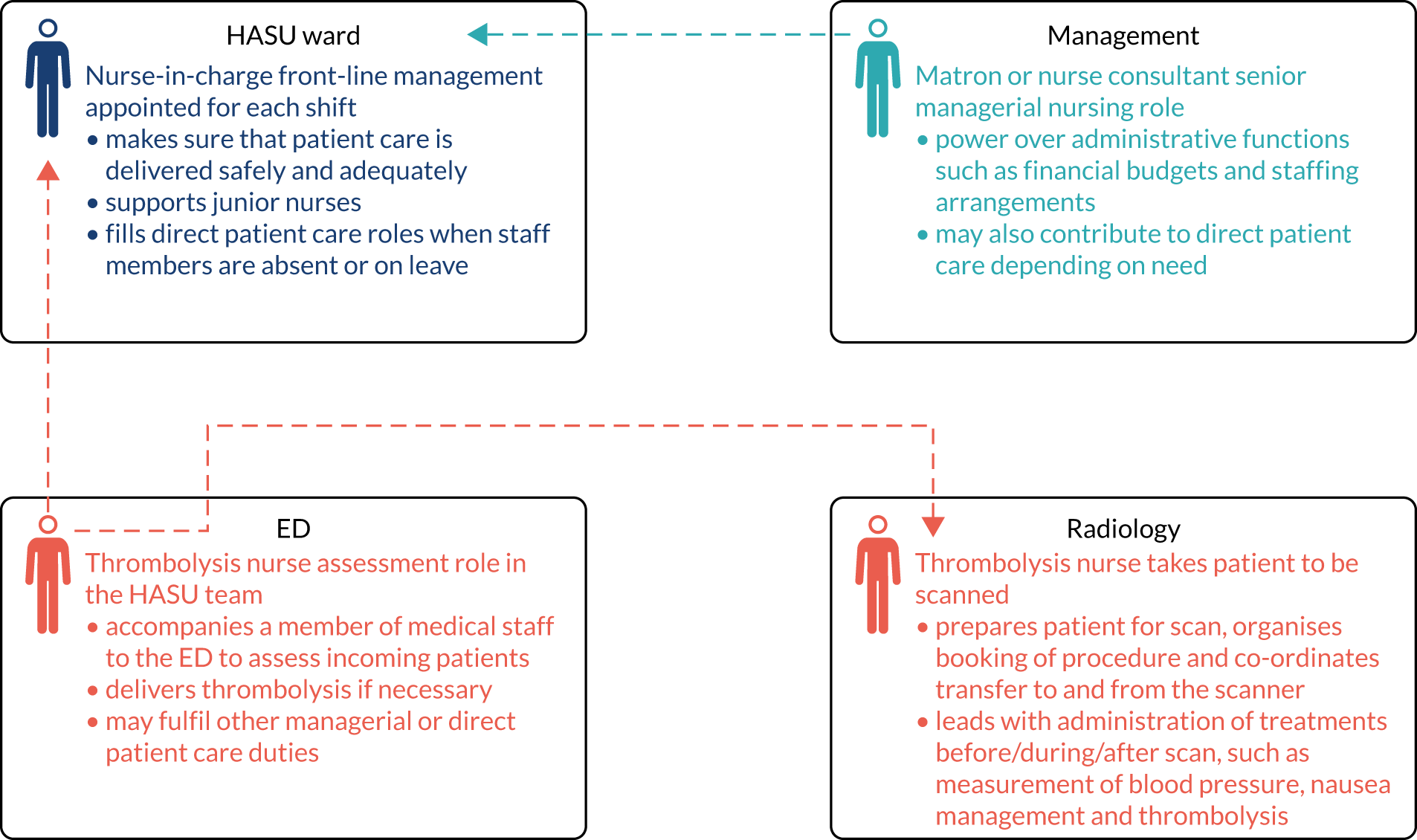
Senior nurses had very different roles (Table 14) from junior nurses (see Table 13), although we did find that some HASUs had trained their junior nurses to fulfil specific tasks, such as thrombolysis and dysphagia screening. Senior nurses were always present in the daytime during the week, but were not always present during the nights or at weekends. Someone in the ward will always fulfil the role of nurse in charge and thrombolysis nurse, but at night and at weekends this may be a lower band nurse or the same person may complete both roles. However, the actual tasks performed by these roles are different in weekday hours (Tables 14 and 15). It is worth noting that when a senior nurse is actively involved in patients’ care, their ward management and oversight activities are reduced.
Role | Task |
---|---|
Communication roles |
|
Patient care roles |
|
Support roles |
|
Co-ordination and oversight |
|
Role | Task |
---|---|
Education |
|
Patient assessment |
|
Service management |
|
How senior is the nurse in charge of the ward at different times of day and days of the week?
Across all HASUs, for the majority of patients admitted to a hospital, the nurse in charge was a band 6 nurse (for 68–90% of patients in each time period across the week) (Figure 13). The nurse in charge was band 5 for 7–29% of patients across the week, with higher proportions of band 5 nurses in charge during the night. The nurse in charge was band 7 for 0–13% of patients across the week, mainly during the day on weekdays. The nurse in charge was rarely a band 8 nurse or a bank or agency nurse.
FIGURE 13.
Band of nurse in charge, by day and time of admission. Variation across the week (p < 0.001).
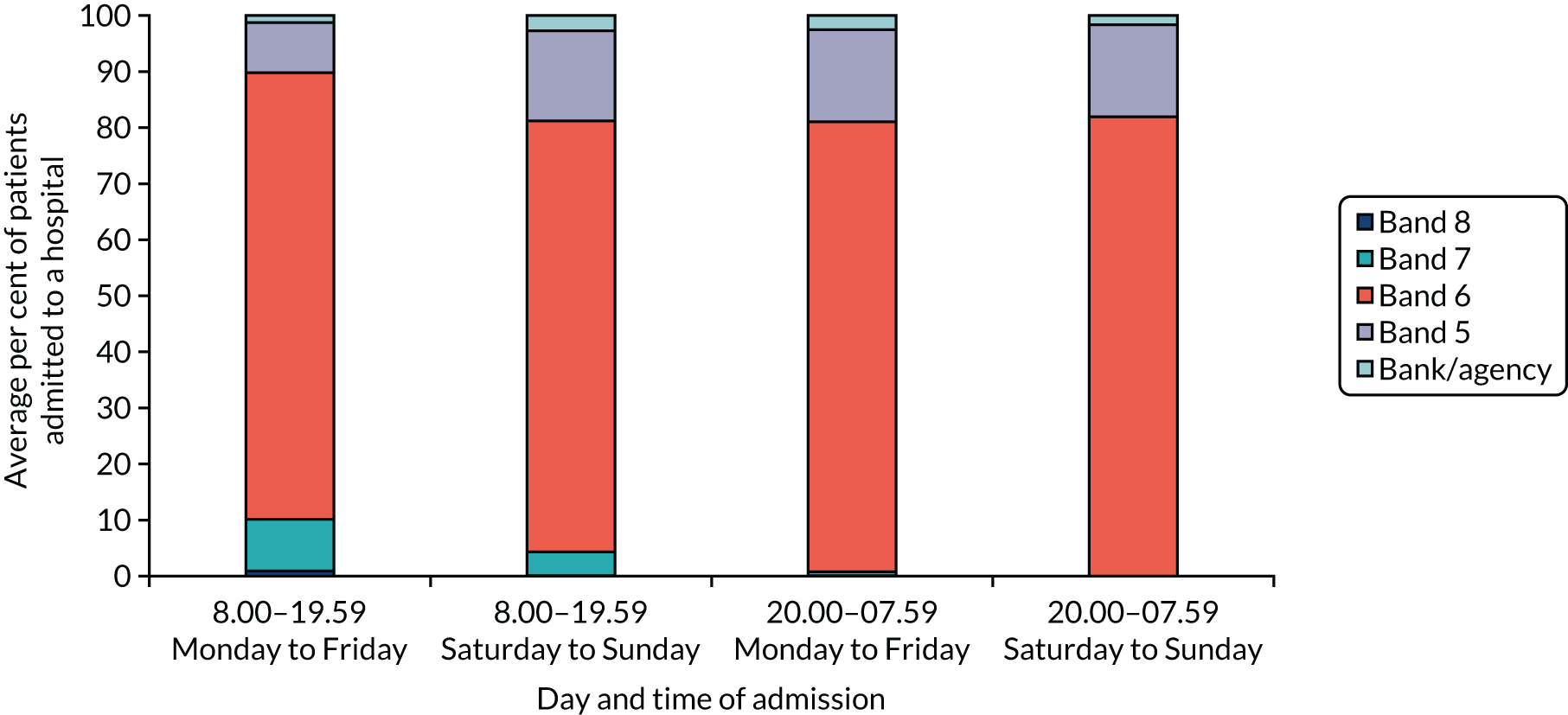
For 61% of patients there was a dedicated thrombolysis nurse who was separate from the nurse in charge on arrival (Figure 14), varying from 36% to 80% across the week. For patients admitted at night, there was a greater likelihood that the thrombolysis nurse and nurse in charge were roles held by one person.
FIGURE 14.
Whether or not there were separate nurses managing thrombolysis and the ward, by day and time of admission. Variation across the week (p < 0.001).
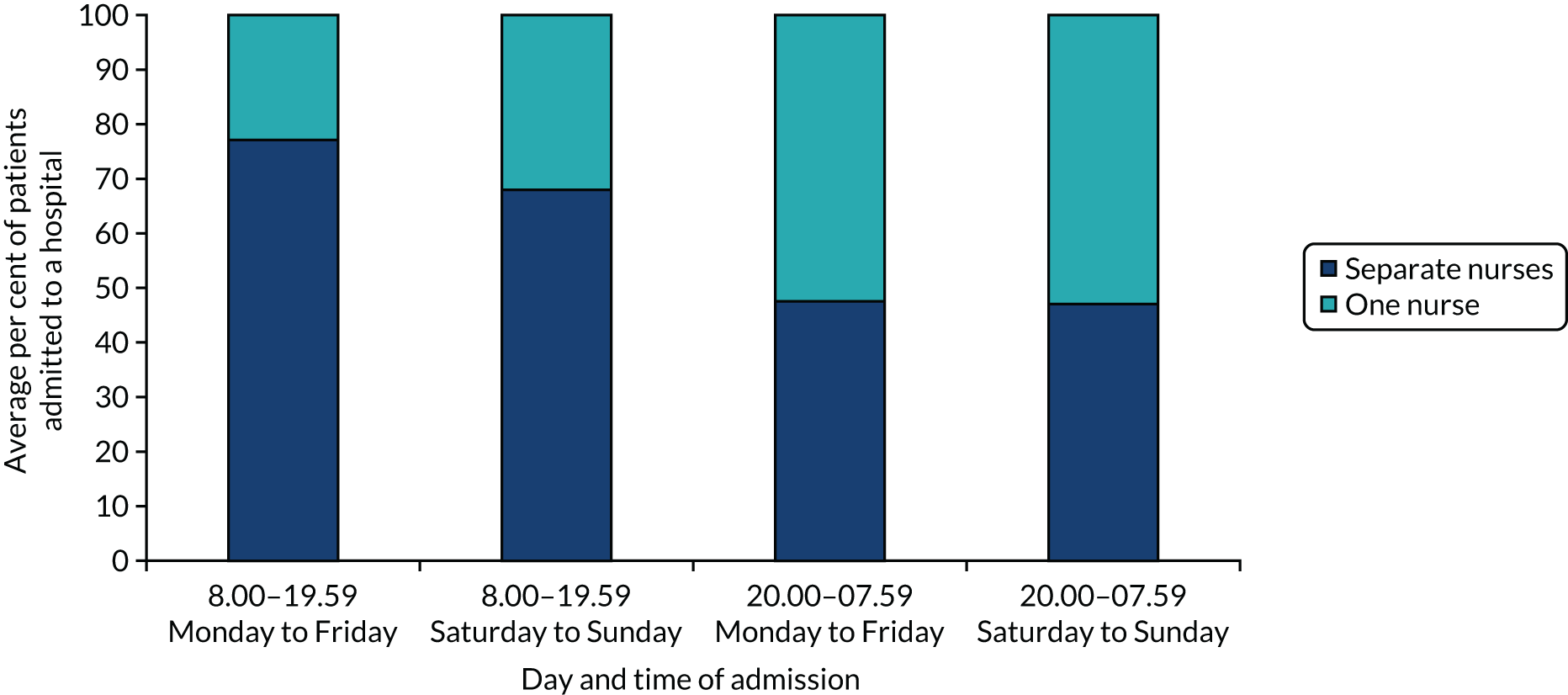
The mean number of HASU nurses varied between 8 and 11 across the week, with higher numbers working during the day (Figure 15). The most common band of nurse in each time period was band 5, with, on average, three to five band 5 nurses working across the week, followed by health-care assistants (two or three nurses working across the week). The next most common were band 6 nurses (one or two nurses) and bank or agency nurses (one or two nurses).
FIGURE 15.
Number of nurses on duty involved in patient care by band, by day and time of admission. Variation in all but band 8 across the week (p < 0.001). HCA, health-care assistant.
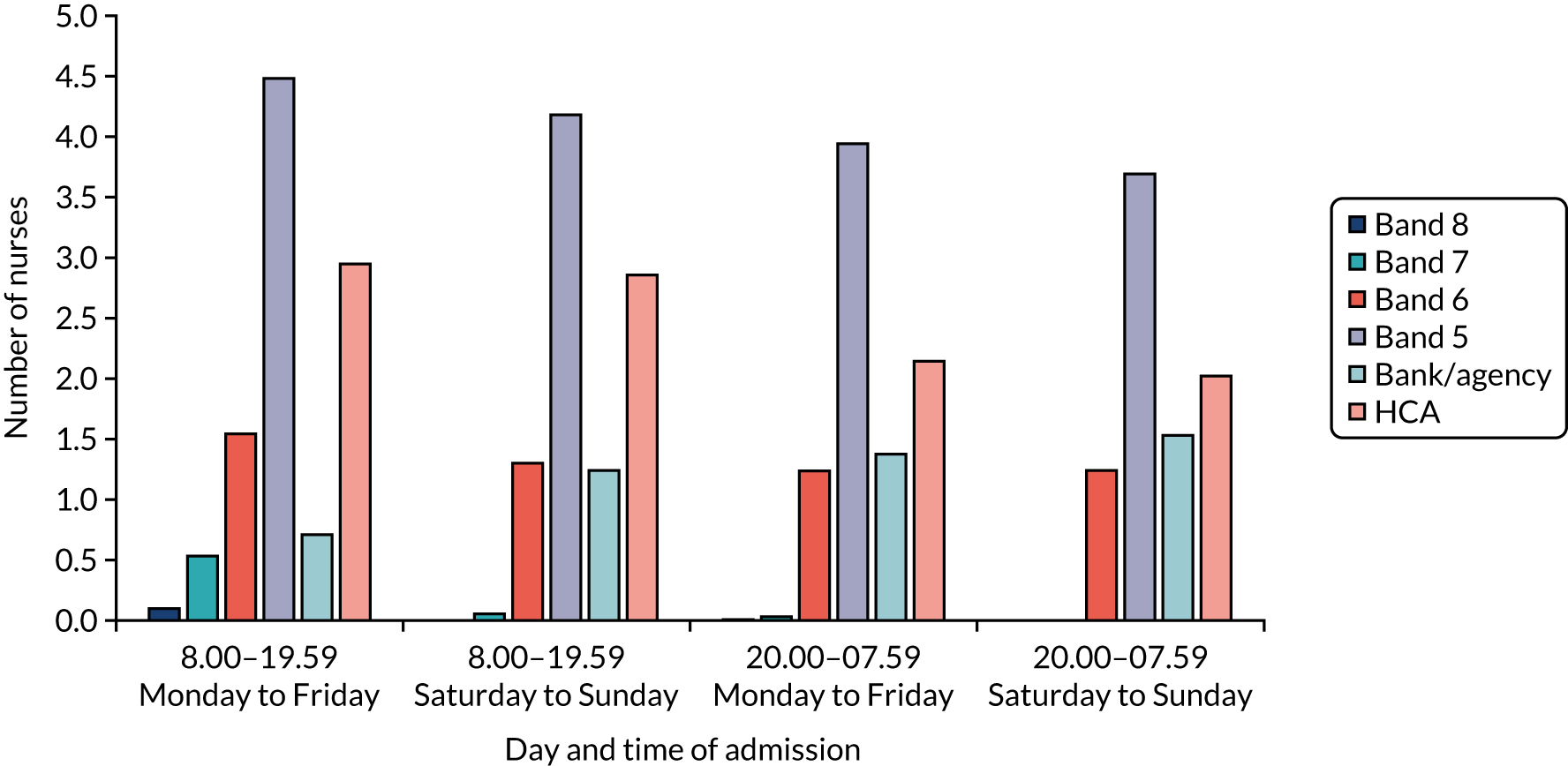
Although we have granular data describing nurse staffing for every half-hour of the day over the study period, nurse staffing varies largely by shift. On average, the number of band 7 and 8 nurses was small (< 1), seen mainly during the day on weekdays. Band 7 and 8 ward managers in most cases are not included in rotas, as they do not provide patient care. There was greatest variation across the week in band 5 nurses, health-care assistants and bank and agency nurses, with higher numbers of band 5 nurses and health-care assistants during the day, and higher numbers of bank and agency nurses at night.
How does nursing seniority impact on clinical processes and patient outcomes?
Results we report are for patients admitted during all time periods combined and in each of four time periods separately. In Report Supplementary Material 2, Table S4, we report analogous results but only for patients eligible for thrombolysis on admission to the hospital (according to the Royal College of Physicians’ definition of eligibility). In Report Supplementary Material 2, Table S5, we report analogous results for patients not eligible for thrombolysis.
Nurse staffing had a statistically significant impact on having a brain scan within 1 hour, dysphagia screening within 4 hours and the probability of being discharged with at least moderate disability (Table 16). It did not have a significant impact on administration of thrombolysis, admission to the HASU within 4 hours, inpatient mortality within 3 days or LOS on HASU and in the hospital.
Components of LOS/costs | All | 08.00–19.59 | 20.00–07.59 | ||
---|---|---|---|---|---|
Weekday | Weekend | Weekday | Weekend | ||
Brain scan within 1 hour | |||||
Band 8 | 0.80 (0.67–0.92) | 0.82 (0.70–0.94) | |||
Band 7 | 0.63 (0.58–0.67) | 0.61 (0.56–0.66) | 0.59 (0.46–0.73) | 0.70 (0.42–0.97) | |
Band 6 | 0.61 (0.60–0.63) | 0.59 (0.58–0.61) | 0.64 (0.61–0.67) | 0.64 (0.61–0.66) | 0.65 (0.61–0.70) |
Band 5 | 0.56 (0.53–0.60) | 0.55 (0.49–0.60) | 0.55 (0.48–0.62) | 0.55 (0.48–0.62) | 0.61 (0.50–0.71) |
Band/agency | 0.62 (0.54–0.70) | 0.61 (0.47–0.74) | 0.63 (0.47–0.79) | 0.66 (0.51–0.80) | 0.78 (0.51–1.04) |
p-value | 0.01 | 0.02 | 0.15 | 0.11 | 0.49 |
Separate nurses | 0.59 (0.58–0.61) | 0.58 (0.56–0.60) | 0.60 (0.57–0.64) | 0.62 (0.58–0.66) | 0.63 (0.56–0.69) |
One nurse | 0.64 (0.62–0.66) | 0.64 (0.60–0.67) | 0.67 (0.62–0.72) | 0.63 (0.59–0.67) | 0.66 (0.61–0.72) |
p-value | 0.00 | 0.01 | 0.04 | 0.63 | 0.46 |
RN, n | 0.01 (0.13) | 0.02 (0.00) | 0.02 (0.02) | –0.03 (0.00) | –0.01 (0.45) |
HCA, n | 0.00 (0.29) | 0.00 (0.82) | –0.01 (0.30) | 0.03 (0.01) | 0.02 (0.40) |
Dysphagia screening within 4 hours | |||||
Band 8 | |||||
Band 7 | 0.76 (0.72–0.80) | 0.72 (0.67–0.77) | 0.79 (0.67–0.92) | 0.85 (0.58–1.12) | |
Band 6 | 0.76 (0.74–0.77) | 0.74 (0.72–0.75) | 0.78 (0.76–0.81) | 0.78 (0.75–0.80) | 0.79 (0.75–0.82) |
Band 5 | 0.70 (0.67–0.73) | 0.71 (0.66–0.76) | 0.65 (0.59–0.72) | 0.70 (0.64–0.77) | 0.77 (0.67–0.86) |
Band/agency | 0.74 (0.67–0.81) | 0.72 (0.60–0.84) | 0.77 (0.63–0.92) | 0.82 (0.71–0.92) | 0.69 (0.40–0.98) |
p-value | 0.01 | 0.61 | < 0.01 | 0.13 | 0.74 |
Separate nurses | 0.79 (0.78–0.80) | 0.77 (0.76–0.79) | 0.80 (0.78–0.83) | 0.81 (0.77–0.84) | 0.86 (0.82–0.90) |
One nurse | 0.66 (0.64–0.68) | 0.60 (0.57–0.64) | 0.65 (0.60–0.70) | 0.73 (0.69–0.77) | 0.71 (0.65–0.77) |
p-value | 0.00 | 0.00 | 0.00 | 0.01 | 0.00 |
RN, n | 0.00 (0.72) | 0.02 (0.00) | 0.00 (0.97) | –0.03 (0.00) | –0.03 (0.02) |
HCA, n | 0.05 (0.00) | 0.05 (0.00) | 0.04 (0.00) | 0.08 (0.00) | 0.03 (0.18) |
Admission to the HASU within 4 hours | |||||
Band 8 | 0.64 (0.49–0.79) | 0.66 (0.51–0.81) | |||
Band 7 | 0.63 (0.59–0.68) | 0.61 (0.56–0.66) | 0.61 (0.47–0.75) | 0.60 (0.28–0.91) | |
Band 6 | 0.65 (0.63–0.66) | 0.62 (0.60–0.64) | 0.68 (0.65–0.71) | 0.68 (0.66–0.71) | 0.70 (0.66–0.74) |
Band 5 | 0.61 (0.57–0.64) | 0.60 (0.55–0.66) | 0.51 (0.44–0.59) | 0.62 (0.56–0.69) | 0.75 (0.66–0.84) |
Band/agency | 0.67 (0.60–0.75) | 0.67 (0.54–0.79) | 0.61 (0.45–0.77) | 0.78 (0.66–0.90) | 0.56 (0.26–0.86) |
p-value | 0.22 | 0.87 | 0.00 | 0.14 | 0.38 |
Separate nurses | 0.65 (0.63–0.66) | 0.62 (0.60–0.63) | 0.67 (0.63–0.70) | 0.69 (0.65–0.73) | 0.73 (0.67–0.79) |
One nurse | 0.63 (0.61–0.65) | 0.62 (0.59–0.65) | 0.61 (0.56–0.66) | 0.66 (0.63–0.70) | 0.68 (0.63–0.74) |
p-value | 0.19 | 0.94 | 0.06 | 0.40 | 0.29 |
RN, n | 0.02 (0.00) | 0.03 (0.00) | 0.02 (0.06) | 0.00 (0.89) | 0.02 (0.16) |
HCA, n | 0.02 (0.00) | 0.03 (0.00) | 0.01 (0.37) | 0.03 (0.01) | –0.01 (0.52) |
Thrombolysis rate | |||||
Band 7 | 0.85 (0.75–0.94) | 0.87 (0.77–0.96) | |||
Band 6 | 0.89 (0.87–0.91) | 0.89 (0.86–0.91) | 0.88 (0.84–0.91) | ||
Band 5 | 0.84 (0.78–0.90) | 0.81 (0.70–0.92) | 0.81 (0.66–0.96) | ||
Band/agency | 0.82 (0.66–0.99) | 0.87 (0.65–1.09) | 0.41 (0.06–0.76) | ||
p-value | 0.37 | 0.52 | 0.05 | ||
Separate nurses | 0.88 (0.86–0.90) | 0.87 (0.84–0.90) | 0.88 (0.82–0.94) | ||
One nurse | 0.86 (0.82–0.90) | 0.90 (0.85–0.95) | 0.84 (0.76–0.92) | ||
p-value | 0.35 | 0.36 | 0.50 | ||
RN, n | 0.00 (0.71) | 0.00 (0.67) | 0.02 (0.35) | ||
HCA, n | 0.01 (0.12) | 0.00 (0.61) | 0.02 (0.50) | ||
Door-to-needle time < 1 hour | |||||
Band 8 | 0.84 (0.55–1.13) | 0.86 (0.60–1.12) | |||
Band 7 | 0.76 (0.65–0.87) | 0.72 (0.60–0.85) | 0.73 (0.35–1.10) | ||
Band 6 | 0.85 (0.83–0.87) | 0.85 (0.82–0.88) | 0.90 (0.86–0.94) | 0.80 (0.75–0.86) | |
Band 5 | 0.81 (0.74–0.88) | 0.85 (0.76–0.94) | 0.66 (0.45–0.87) | 0.81 (0.66–0.95) | |
Band/agency | 0.80 (0.63–0.96) | 0.82 (0.58–1.05) | 0.95 (0.80–1.09) | 0.76 (0.43–1.08) | |
p-value | 0.37 | 0.31 | 0.07 | 0.96 | |
Separate nurses | 0.84 (0.81–0.86) | 0.83 (0.80–0.86) | 0.91 (0.87–0.95) | 0.78 (0.70–0.86) | |
One nurse | 0.85 (0.81–0.89) | 0.87 (0.81–0.92) | 0.75 (0.62–0.87) | 0.82 (0.74–0.90) | |
p-value | 0.58 | 0.28 | 0.01 | 0.56 | |
RN, n | 0.01 (0.27) | 0.02 (0.02) | –0.01 (0.40) | –0.02 (0.18) | |
HCA, n | 0.01 (0.20) | 0.01 (0.45) | 0.02 (0.22) | 0.01 (0.78) | |
mRS score of 3–6 at HASU discharge | |||||
Band 8 | 0.54 (0.40–0.68) | 0.58 (0.44–0.72) | |||
Band 7 | 0.56 (0.52–0.61) | 0.55 (0.51–0.60) | 0.62 (0.51–0.74) | 0.27 (0.02–0.53) | |
Band 6 | 0.60 (0.59–0.61) | 0.59 (0.58–0.61) | 0.61 (0.58–0.64) | 0.60 (0.58–0.63) | 0.58 (0.54–0.62) |
Band 5 | 0.57 (0.54–0.60) | 0.60 (0.56–0.65) | 0.64 (0.58–0.70) | 0.51 (0.46–0.57) | 0.42 (0.33–0.51) |
Band/agency | 0.65 (0.59–0.72) | 0.68 (0.57–0.80) | 0.70 (0.58–0.82) | 0.60 (0.46–0.73) | 0.66 (0.39–0.92) |
p-value | 0.05 | 0.21 | 0.52 | 0.02 | 0.01 |
Separate nurses | 0.58 (0.57–0.60) | 0.58 (0.56–0.59) | 0.61 (0.59–0.64) | 0.55 (0.52–0.59) | 0.57 (0.51–0.64) |
One nurse | 0.62 (0.60–0.64) | 0.63 (0.60–0.66) | 0.63 (0.59–0.67) | 0.61 (0.58–0.65) | 0.54 (0.49–0.59) |
p-value | 0.00 | 0.00 | 0.55 | 0.05 | 0.46 |
RN, n | 0.00 (0.92) | 0.01 (0.21) | –0.01 (0.08) | 0.01 (0.30) | –0.03 (0.07) |
HCA, n | 0.03 (0.00) | 0.02 (0.00) | 0.05 (0.00) | 0.05 (0.00) | 0.00 (0.84) |
In-hospital mortality at 3 days | |||||
Band 7 | 0.03 (0.01–0.04) | 0.03 (0.02–0.05) | |||
Band 6 | 0.03 (0.02–0.03) | 0.03 (0.02–0.03) | 0.04 (0.03–0.06) | 0.03 (0.02–0.04) | |
Band 5 | 0.02 (0.01–0.03) | 0.04 (0.02–0.07) | 0.01 (0.00–0.02) | 0.01 (0.00–0.03) | |
Band/agency | 0.02 (0.00–0.05) | 0.03 (–0.02–0.08) | 0.07 (–0.04–0.18) | ||
p-value | 0.92 | 0.61 | 0.02 | 0.23 | |
Separate nurses | 0.03 (0.02–0.03) | 0.03 (0.02–0.03) | 0.05 (0.03–0.06) | 0.03 (0.02–0.04) | |
One nurse | 0.03 (0.02–0.03) | 0.04 (0.02–0.05) | 0.03 (0.01–0.04) | 0.03 (0.01–0.04) | |
p-value | 1.00 | 0.35 | 0.10 | 0.97 | |
RN, n | 0.00 (0.89) | 0.00 (0.87) | 0.00 (0.94) | 0.00 (0.57) | |
HCA, n | 0.00 (0.24) | 0.00 (0.10) | 0.00 (0.98) | 0.00 (0.64) | |
HASU LOS | |||||
Band 8 | 3.19 (2.34–4.04) | 3.17 (2.30–4.04) | 1.92 (–1.26–5.10) | ||
Band 7 | 2.93 (2.70–3.17) | 2.79 (2.55–3.04) | 3.44 (2.79–2.79) | 4.03 (1.41–6.65) | |
Band 6 | 3.13 (3.06–3.20) | 3.13 (3.04–3.22) | 3.35 (3.20–3.20) | 3.00 (2.85–3.16) | 3.17 (2.93–3.41) |
Band 5 | 3.34 (3.15–3.53) | 3.21 (2.92–3.50) | 3.79 (3.38–3.38) | 3.27 (2.87–3.67) | 3.39 (2.81–3.98) |
Band/agency | 3.11 (2.67–3.56) | 2.94 (2.26–3.63) | 3.74 (2.80–2.80) | 2.72 (1.92–3.51) | 5.07 (2.64–7.49) |
p-value | 0.11 | 0.16 | 0.22 | 0.53 | 0.14 |
Separate nurses | 3.18 (3.10–3.26) | 3.16 (3.07–3.26) | 3.46 (3.29–3.62) | 2.95 (2.73–3.18) | 3.30 (2.94–3.66) |
One nurse | 3.07 (2.96–3.18) | 2.91 (2.75–3.08) | 3.38 (3.14–3.62) | 3.13 (2.91–3.36) | 3.18 (2.87–3.49) |
p-value | 0.13 | 0.02 | 0.63 | 0.31 | 0.64 |
RN, n | –0.07 (0.00) | –0.06 (0.06) | –0.18 (0.00) | 0.02 (0.75) | –0.11 (0.15) |
HCA, n | –0.08 (0.00) | –0.09 (0.00) | 0.04 (0.50) | –0.09 (0.24) | –0.20 (0.12) |
Hospital LOS | |||||
Band 8 | 7.84 (4.33–11.34) | 8.34 (4.45–12.23) | 12.36 (–16.79–41.50) | 3.09 (–4.98–11.16) | |
Band 7 | 10.20 (8.76–11.64) | 9.30 (7.86–10.74) | 14.52 (9.24–19.80) | 7.84 (0.68–15.01) | |
Band 6 | 11.13 (10.58–11.68) | 10.76 (10.03–11.49) | 13.72 (12.04–15.40) | 10.67 (9.55–11.79) | 12.84 (10.27–15.41) |
Band 5 | 10.90 (9.81–12.00) | 11.68 (9.86–13.51) | 13.61 (10.71–16.52) | 9.40 (7.49–11.31) | 10.82 (7.13–14.51) |
Band/agency | 11.20 (8.53–13.86) | 11.44 (7.03–15.85) | 16.96 (9.16–24.76) | 9.24 (5.02–13.45) | 15.37 (1.91–28.83) |
p-value | 0.45 | 0.18 | 0.92 | 0.60 | 0.54 |
Separate nurses | 11.04 (10.47–11.61) | 10.76 (10.03–1.49) | 14.20 (12.42–15.97) | 9.60 (8.27–10.92) | 14.27 (10.77–17.77) |
One nurse | 11.03 (10.26–11.80) | 10.44 (9.31–11.57) | 12.89 (10.80–14.97) | 11.20 (9.72–12.67) | 11.14 (8.58–13.71) |
p-value | 0.98 | 0.60 | 0.24 | 0.10 | |
RN, n | –0.25 (0.05) | –0.10 (0.23) | –0.68 (0.05) | –0.03 (0.91) | –0.89 (0.12) |
HCA, n | –0.10 (0.47) | –0.18 (0.16) | 0.69 (0.11) | –0.15 (0.72) | –1.59 (0.08) |
Impact of the seniority of the nurse in charge on clinical processes
After controlling for time of admission across the week, number of staff, and separation of ward management and thrombolysis roles, a higher band of the nurse in charge would result in a higher probability that a patient would undergo a brain scan within 1 hour (a probability of 0.56 if the nurse-in-charge was band 5, rising to a probability of 0.80 if the nurse in charge was band 8). Dysphagia screening within 4 hours was also affected by the band of the nurse in charge (see Table 16), with a probability of 0.70 if the nurse in charge was band 5 and rising to a probability of 0.76 if the nurse-in-charge was band 7.
There was a significant variation in the effect of nurse staffing measures during the four time periods across the week; this reflects the importance of the contribution of senior nurses to co-ordination within (improving the likelihood of dysphagia screening on time) and outside (improving the likelihood of brain scanning on time) the HASU. The effect of nurse-in-charge seniority was significant in hours, Monday to Friday, for brain scanning; in hours, Saturday to Sunday, for dysphagia assessment; and out of hours, Monday to Sunday, for the mRS measure. The probability of admission to the HASU within 4 hours did not vary significantly with nurse staffing, except for a higher probability of patients being admitted in hours over the weekend if a more senior nurse was in charge of the ward.
Impact of separating the nurse in charge and thrombolysis nurse roles on clinical processes
The availability of a dedicated thrombolysis nurse who was not the nurse in charge was associated with a higher probability of completing the dysphagia assessment within 4 hours by 13 percentage points (from 66% to 79%). The probability of completing the brain scan within 1 hour was higher when the nurse in charge was also the thrombolysis nurse. This result was particularly significant for patients not eligible for thrombolysis, admitted in hours, Monday to Friday.
The effect of having a separate tPA nurse and nurse in charge was significant in hours, Monday to Sunday, for brain scanning, in hours and out of hours, Monday to Sunday, for dysphagia assessment, and in hours, Monday to Friday, for the mRS measure. Better outcomes for dysphagia assessment and disability measures were associated with having separate nurses for these roles, whereas for brain scanning better outcomes were achieved when there was one nurse managing the ward and responsible for tPA. This result is reflecting the fact that a more senior nurse on duty is usually taking the role of the nurse in charge, and when they are busy with a new patient (e.g. arranging the brain scan) there is no one on the ward to provide the leadership that supports rapid dysphagia screening.
For example, we observed a new patient arriving in the evening at site H8. The nurse in charge was asked to supervise a dysphagia screen by one of the junior nurses who was being trained, but the tPA nurse was still down in the ED. She had the time to contribute to training and make sure the patient was screened properly. By contrast, in an in-hours observation in H1, the nurse in charge was a band 5 nurse who had stepped in for the day and was also the tPA nurse. She was struggling to cover all the patients as there were some sickness absences and was struggling, in particular, with some ‘confused’ patients [in-hours observation 1 (IH01) H1]. It is notable that these effects were observed for patients not eligible for tPA, mostly.
Impact of nursing on health outcomes
For the health outcomes measures, the availability of a dedicated thrombolysis nurse, different from the nurse in charge, and a more senior nurse in charge were associated with a lower probability of being discharged with at least moderate disability (mRS score of ≥ 3). Inpatient mortality was not significantly related to the nurse staffing, in hours or out of hours.
The number of nurses had a statistically significant impact on some processes and outcomes, but the size of the effect was very small (e.g. ± 5 percentage points when one extra nurse was working), suggesting a limited effect of changes in the number of nurses, once controlling for the roles and seniority. We noticed that an increase in the number of regular nurses was associated with a lower HASU LOS in hours, Saturday to Sunday: having one additional nurse decreases the LOS by 0.18 days (p < 0.01). On the other hand, one result from Chapter 3 suggested that patients admitted within the same time window were staying 0.4 days longer on the HASU. Combined, these findings indicate that employing two more regular nurses might bring down the LOS on HASU, but further analysis on cost and effects needs to be carried out to check whether or not employing extra staff compensates for the reduction of cost due to shorter LOS.
Discussion
There is variation in nurse staffing by time of the week, particularly in terms of the seniority of key roles, rather than the number of nurses overall. This is an important finding, as senior nursing roles are more differentiated than junior roles, in which bank nurses and other nursing staff can more easily substitute for missing personnel.
Qualitative analysis indicates that senior nurses tended to be more able to manage processes within the ward. Many HASUs timetable nurses at higher bands (e.g. band 7) to take on senior nursing tasks, such as the nurse-in-charge role, during the day in the week. This has an impact on the delivery of clinical interventions: nurse staffing had a direct effect on quality-of-care measures soon after arrival at the hospital (brain scan within 1 hour and dysphagia screen within 4 hours). In these cases, quality of care was better if the nurse in charge was a higher band and/or there were more nurses on duty. We explain why nurses with more experience at a higher banding would be more able to fulfil the many tasks expected of them within the time frame expected by the stroke standards. Although some HASUs provide training in particular tasks for more junior nurses, this does not enable them to juggle the many demands of the senior role that are so different from junior tasks.
Meeting the targets set by a financially incentivised set of standards has positive consequences for patient care. Variations in nurse staffing numbers across London HASUs have very limited effects on the likelihood of achievement of HASU standards. This may be because minimum nursing levels in London HASUs, which are stipulated in performance standards, are sufficient to address variations in quality of care and, therefore, variations in nurse staffing over and above this minimum have little additional impact.
We observed limited variation in the number of nurses but greater average seniority of nurses working regular working-week hours (Monday–Friday, 08.00–19.59) than out of hours (Monday–Friday, 20.00–07.59) and at weekends, yet the actual number of patients arriving is not that different. It is partially attributed to the fact that the regular work shifts of senior nurses (Monday–Friday, 08.00–16.00 or 09.00–17.00) do not completely overlap with regular ward working shifts (Monday–Friday, 08.00–19.59).
In the quantitative analysis we did not find variation by nurse staffing in the performance of care processes related to thrombolysis. This finding is in line with our qualitative observations suggesting a certain hierarchy favouring tPA patients, despite London stroke service standards that specify that most patients should be undergoing rapid ‘front-door’ services.
On the other hand, the achievement of key processes for patients not eligible for thrombolysis might differ if there is not enough senior nurse leadership, and separate responsibilities of the nurse in charge and thrombolysis nurse. The greater leadership capacity of senior nurses increased the likelihood of adherence to the system: senior nurses know that the London system is meant to benefit all stroke patients, whereas less senior staff might bend in the face of staff who want to prioritise only the tPA subsample.
Our findings suggest that one nurse managing thrombolysis and the ward is sufficient for rapid access to brain scanning, but separate nurses for these roles would be more efficient for dysphasia screening within 4 hours. We noted, from observations in our qualitative study, that brain scans were more likely to be undertaken soon after arrival at the ED, whereas dysphagia screening was more likely to occur on the HASU. We also observed that the tPA nurse was more likely to spend time at the ED (i.e. seeing patients soon after they arrived at the hospital). If the tPA nurse was also the nurse in charge, then this suggests that the nurse in the ED would be relatively senior, which might explain why brain scans within 1 hour were more likely to be achieved. Conversely, if the nurse in charge is not the tPA nurse, then the nurse in charge is more likely to be located on the HASU, which is where dysphagia assessments typically occur. The senior nurse being located on the HASU might therefore explain why dysphagia screening is more likely to be completed within 4 hours if the nurse in charge and tPA nurse roles are separate.
Improved quality-of-care measures and their contribution towards health outcomes might be expected by ensuring better senior nurse coverage out of hours and during the weekend, especially for patients not eligible for thrombolysis. There are advantages and disadvantages when the nurse in charge is the thrombolysis nurse.
Limitations
There are several weaknesses in our analysis. First, nurse staffing shifts were not the same in all eight HASUs. We chose 12-hour time periods, starting at 08.00, to be consistent with the in-hours and out-of-hours definition adopted in Chapter 3. However, in several HASUs, the first shift started at 07.00 or 07.30, and in one HASU there were three daily shifts instead of two. This was taken into account in the handover control variable. Second, mRS score at discharge from a HASU is a complex measure that accounts for many aspects of patient care and, as uncovered in our qualitative observations, is also affected by the organisation of repatriation processes, availability of local services and HASU demands, which might not always reflect an outcome of quality of care. Third, our data set did not include medical staffing and, therefore, we were unable to control for this in our analyses, which could confound the results. Future research exploring the interaction between medical and nursing staffing in this context would be beneficial. Fourth, similar to what is discussed in Chapter 3, Discussion, there was limited follow-up of patients, including mortality and disability indicators not available in the SSNAP.
Summary
-
There was variation in nurse staffing by time of the week, particularly in terms of the seniority of key roles, rather than the number of nurses overall.
-
Senior nursing roles were more differentiated than junior roles, which can be substituted by bank nurses and other nursing staff.
-
When senior nurses took on the nurse-in-charge role during the day in the week, it had an impact on the delivery of clinical interventions.
Chapter 6 The temporal structuring of hyperacute stroke wards: what happens when and why?
What is known about this topic?
-
Interest in 7-day working has hitherto taken two approaches: (1) a binary in-hours/out-of-hours approach or (2) a temporal variation approach, looking at blocks of time across the day and week.
-
Measurement of temporal variation relies on routinely collected hospital and clinical audit data.
-
There was a lack of clarity about how temporal variation arises.
What this analysis adds
-
The SSNAP and London stroke service standards dominate the timing of clinical events in the ‘front-door’ part of the patient pathway, through setting of micro time limits that are evidence linked to clinical outcomes (1 hour, 4 hours, etc.).
-
The SSNAP metrics later in the patient pathway have longer time limits (24 hours, 72 hours) that are not indicative of clinical benefit, but reflect cultural norms about rotas and activities, such as ward rounds, which have financial implications.
-
Assessing temporal variation for events that are more than 1 hour after arrival in terms of in-hours and out-of-hours arrival periods was meaningless, as the punctuation of temporal structures, such as therapy rotas and ward rounds, was what determined clinical contacts.
-
Repatriation from the HASU was temporally structured by the cultural norms and working patterns of other departments and organisations.
-
Measuring temporal concepts, such as clustering, pressure and bottlenecks, is as important as consistency.
Introduction
A number of different approaches have been taken to try to understand temporal variation in stroke: the weekend in combination with night-time as a unitary out-of-hours block (e.g. Campbell et al. 26); everything outside Monday–Friday, 08.00–18.00; 08.00–20.00 on Sunday and Wednesday as a comparison;31 and weekend admissions compared with weekday admissions. 73
Latterly, debates about poorer outcomes for patients in secondary health-care systems outside the 5-day working week, or the ‘weekend effect’, have moved towards acknowledging patterns of temporal variation. 22–25 Recently, this movement was characterised by the idea that certain times of the day, or days of the week, are associated with poorer outcomes for the patient, but that this is not limited to the weekend or night-time. 22 As a result, the concept of out-of-hours working, which once dominated the discourse of the literature on temporal variation,22,26–28 has been re-evaluated.
Therefore, with a lack of compelling explanations for temporal variation, NHS England continues to implement its policy for a 7-day NHS by the year 2020. 17 The NHS Long Term Plan18 states that all stroke units will, over the next 5 years, meet the NHS 7-day standards for stroke care and the national clinical guidelines for stroke over the next 5 years.
Our aim in this chapter is to develop the debate around 7-day working by describing the factors that influence the timing of clinical interventions in a particular acute setting, on a hyperacute stroke ward, and explaining how these factors influence studies of temporal variation. This will have a novel impact on (1) providing and organising construct for more accurate quantitative analysis of temporal variation in stroke and other acute diseases; and (2) using this to design health service provision, which takes temporal variation into account and returns health outcomes that are better linked to the care timeline.
Our starting position is that a binary conception of in hours and out of hours is inaccurate, and that there are many different characteristic time periods and patterns in activity on stroke wards, which we evidence in this chapter. Therefore, we use the term ‘temporal structuring’ to describe regular or changing temporal patterns that orient staff activities. 90 Each ward creates its own temporal structures by its everyday practices and ideas about when to complete different tasks. 90 Work in organisations such as hospitals is reliant on co-operation and connection between groups of people with different conceptions of temporality. 91 This analysis was important in helping to untangle the conflicting and confusing evidence for temporal inconsistency, and clarifying controversy and providing a new direction for tackling this knotty problem. Ultimately, this contributes to the reduction of avoidable, systematic inequalities in stroke care and better outcomes for patients.
In Chapter 3, we reported the finding that certain aspects of evidence-based care are indeed provided with no discernible temporal variation, even when temporal variation was considered in very granular time periods. In Chapter 4, we reported that this was due to a number of factors, including adapting and extending roles, creating continuities between different times of day, building relationships and trust, prioritisation of ‘front-door’ interventions and unintended consequences of adaptations.
Research suggests that London is not unique in showing no significant ‘weekend effect’ in stroke patients in terms of such outcomes as LOS, mortality and functional outcome (both in hospital and at 90 days), both in similarly specialised comprehensive stroke centres62 and in unspecialised settings. 14 Variation persists due to inconsistencies in medical, managerial and allied health professional staffing by time of day, delivery of therapist assessments and barriers to repatriation processes.
Figure 16 summarises our findings about temporal consistency in the HASUs, reported in our previous three chapters (see Chapters 3–5).
FIGURE 16.
Summary of findings from Chapters 3–4 about HASU temporal consistency. 24/7, 24 hours a day, 7 days a week.
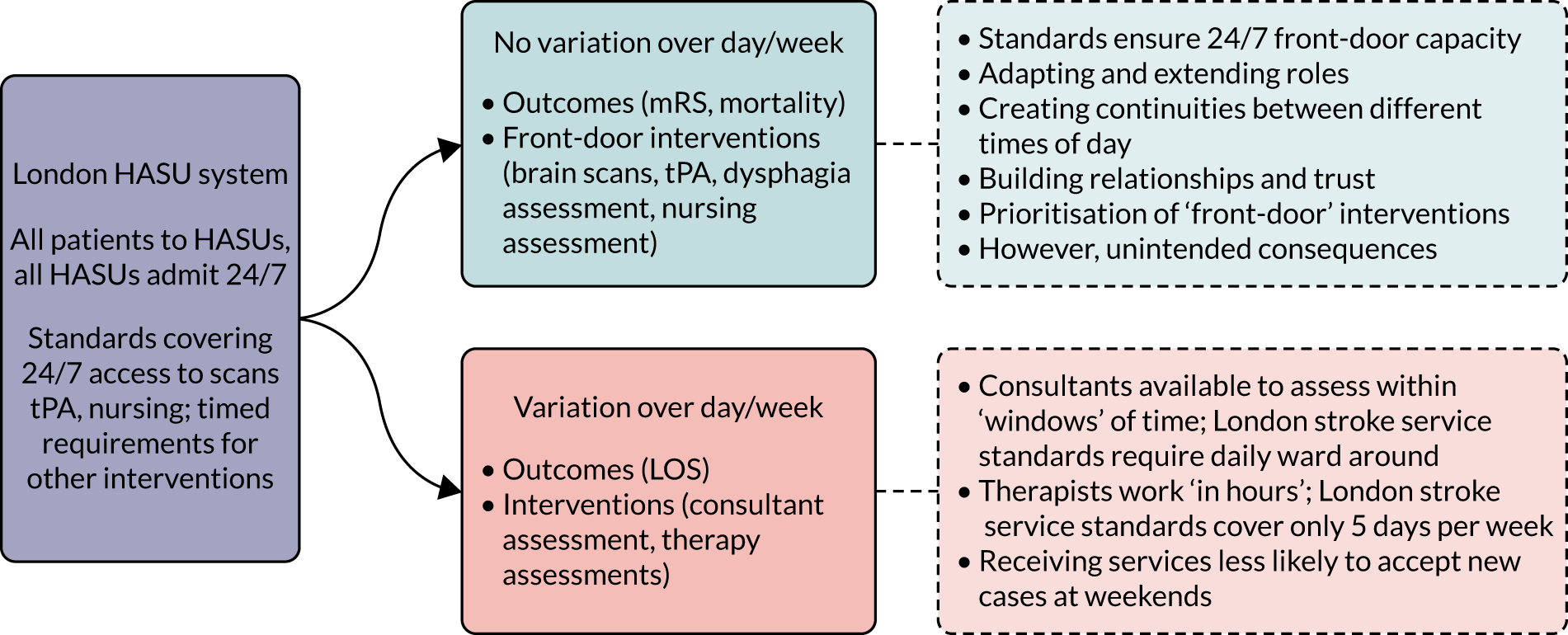
Methods
Full methods are outlined in Chapter 2. The basis of these findings depends on thematic analysis and mixed-methods synthesis of:
-
staff interviews
-
observations
-
quantitative data analysis of the SSNAP database 1 January to 31 December 2014
-
documentary analysis of the SSNAP guidelines and London stroke service standards guidance.
Analysis
An inductive coding process initiated the analysis, for which authors GB, AIGR, ABL and JE derived a group of codes relating to definitions of ‘in hours’ and ‘out of hours’. We developed this with a set of working ideas about how these different definitions had arisen. This was incorporated into iterative processes of discussion with the whole authorship team, in which we developed different ideas about how best to use our quantitative data to truly measure temporal variation. Many of these discussions involved ‘dialogues’ between the qualitative (particularly observational) and the quantitative data. For example, when we felt that we had witnessed temporal variation, we then tried to find a measure that might reflect the dynamic we had identified. This exposed difficulties with the SSNAP data set and provoked new measures (such as looking at patients who had not been eligible for thrombolysis) to expose procedural elements that might be prone to greater temporal variation. The resultant analysis was the result of these processes, presented partly in a thematic form around the concept of temporal structuring, with graphically illustrated examples.
Results
Our results reconsider temporal variation measurement and meaning, by exploring the patients’ pathways through the HASU. We contrast this with the temporal structures of the HASU, of which the patients may have limited awareness, and how these have an impact on the timing of patient care. We finally present a critique of the current methods of measuring temporal variation based on routinely collected data, highlighting the relationship between patient need, quality of care and how these are measured..
Understanding the patient pathway
The first part of this analysis was a schematic or generalised account of the patient’s pathway through the HASU and how patients’ clinical outcomes are influenced by the temporal structures of the HASU. The second part describes in more detail what the temporal structures operating in the HASUs are and how they came to exist. The final section describes how the patient pathway interacts with temporal structures to create measured temporal variation.
Consistent with patient-focused studies,92 our observations and interviews characterised the HASU patient pathway in three main temporal intervals: (1) acute care, (2) assessment and stabilisation, and (3) repatriation (Figure 17).
‘Front-door’ activities
Front-door activities begin when a patient arrives via ambulance or other transport to the ED of a hospital. Ambulances are instructed to take patients suspected of stroke to a hospital with a HASU. Patients arrive at hospitals with a suspected stroke at all hours of the day, although the numbers of admissions are higher during the day than at night, and during the week than at the weekend. From there, patients receive a number of neurological and medical tests and a brain scan, before immediate treatment decisions are made, such as tPA. Those who are still suspected to have had a stroke are admitted to the ward when a bed becomes available.
Clinical assessment, investigation and provision of time-critical treatments
During their stay on the ward, patients are given a range of clinical input, including medical, nursing and allied health professionals (e.g. occupational therapy, speech and language therapy, physiotherapy). Therapists assess the patient for independence, nutrition, continence, mood, etc., and repatriation is contingent on these findings. Patient-centred temporal structures are created by patients’ needs and biorhythms, such as needing to eat, move around and connect with their families. Clinical input was therefore broadly temporally structured around these needs, with therapeutic input, such as assessments and curative therapy, and non-emergency nursing care, such as washing, feeding and liaising with family members, given during the day. Similarly, medical assessments, scans and reviews take place during the day, although emergency medical attention can be provided at any time.
Repatriation
Hyperacute stroke units are designed to provide hyperacute intervention, with an expected LOS for the patient of around 3 days. The price was calculated on a bed-day basis. There was a different price for day 1 compared with days 2–4, which have the same price, and post day 4, which will be priced at the standard excess bed-day rate. 36 This means that repatriation from the HASU to a stroke unit (ASU), a patient’s home or a care home is an important part of HASU staff work. Preparing a patient for discharge involves co-ordinated assessments and paperwork from a variety of staff, including nursing, allied health professionals and the repatriation co-ordinator:
So we, we certainly don’t rush patients home, um, but there is a continuous pressure to rush patients out to create beds, [. . .], so, some patients, we can get into the hospital much quicker than other patients, just by knowing what access they have to necessary services in the community. In terms of repatriation, there are ongoing huge delays, there are patients unnecessarily in the HASU who have been ready for 7 days, up to 2 weeks sometimes, waiting for transfers, and they’ve suffered, because they’re not always prioritised by the therapists, has been a SU [stroke unit] patient, by the fact that they’re geographically still on the HASU patient, even though they should have moved into SU mode.
Consultant, H3
The temporal expectation of the HASU to repatriate patients within a short time frame was frustrated by how the pathway can be inhibited by the availability of beds at the destination of the patient:
As long as they’re not um needing an investigation for the Monday then they will go home, um, we will make referrals but obviously we can’t follow them up um until the Monday, because obviously with the community teams aren’t working, if anyone needs a package of care restart or anything, then they’ll have to stay until Monday as well.
Physiotherapist, H7
Temporal structures acting on the patient pathway
Temporal imperatives: quality assurance metrics
London HASUs are subject to two sets of quality assurance metrics: (1) the SSNAP, which applies to all stroke services in England, Wales and Northern Ireland; and (2) the London stroke service standards. The SSNAP pro forma demands detailed information about patients and their care, including temporal information about the timing of certain procedures. The London stroke service standards (which influence funding for the HASUs) demand temporal indicators measured from ‘clock start’ (entrance to the hospital for patients outside hospital or the time stroke symptoms are spotted for inpatients), including:
-
a ‘door to needle’ (thrombolysis) time of 45 minutes for eligible patients
-
dysphagia screening within 4 hours
-
patients scanned within 12 hours (although given the standard for thrombolysis, most patients will be scanned within 30 minutes to ascertain eligibility)
-
assessment by a stroke specialist physician within 24 hours
-
therapist assessment within 72 hours.
Therefore, the items with the shortest timelines in these standards are focused around prolonging life and improving assessments and interventions in the diagnostic assessment phase of the patient pathway. The London stroke service standards additionally prescribe the level of staffing for each HASU according to the size of the unit, expectations with regard to delays, the proportion of time spent on the HASU (as opposed to other wards), etc., with financial implications for compliance.
London stroke service standards relating to contact with stroke staff (e.g. consultant and therapists) are much broader, with expected timelines of up to 72 hours post admission and 45 minutes of therapeutic daily contact. Considering quality of care and the real impact of these interventions on patient outcomes, the difference between seeing a therapist 10 hours after admission and 20 hours after admission is unknown. The 72-hour limit imposed by the SSNAP quality assessment was deliberately judged in the context that stroke therapists do not routinely work at weekends and, at the time the standards were set, were unlikely to transfer to a 7-day working pattern in the foreseeable future.
Temporal peaks: staff rotas
Nursing, medical and therapy rotas followed very different patterns and varied in each HASU. Our interviews suggested that there was awareness that bedside nursing was temporally structured to be a constant, with overlapping shifts covering the whole 24-hour period. For example, there was one HASU that had an early shift (07.30–15.30), a late shift (12.30–20.30) and a night shift (20.00–08.00) (Table 17).
HASU site ID | Profession | ||
---|---|---|---|
Nursing | Medical | Therapy | |
H1 | 07.30–20.00; 19.30–08.00 | 09.00–17.00; 17.00–21.00; 21.00–09.00 | 09.00–17.00 |
H2 | 08.00–20.30; 20.00–08.30 | 09.00–17.00; 17.00–21.00; 21.00–09.00 | 09.00–18.00 |
H3 | 07.30–20.00; 19.30–08.00 | 09.00–17.00; 17.00–21.00; 21.00–09.00 | 09.00–18.00 |
H4 | 07.30–15.30; 12.30–20.30; 20.00–08.00 | 09.00–17.00; 17.00–21.00; 21.00–09.00 | 09.00–17.00 |
H5 | 07.00–19.30; 19.00–07.30 | 09.00–17.00; 17.00–21.00; 21.00–09.00 | 09.00–17.00 |
H6 | 07.30–20.00; 19.30–08.00 | 09.00–17.00; 17.00–21.00; 21.00–09.00 | 09.00–17.00 |
H7 | 07.30–20.00; 19.30–08.00 | 09.00–15.30; 14.00–20.00; 20.00–09.00 | 09.00–17.00 |
H8 | 08.00–20.30; 20.00–08.30 | 09.00–17.00; 17.00–21.00; 21.00–09.00 | 09.00–17.00 |
The aim of this structure was to have the maximum staffing from 12.30 to 15.30, which was deemed to be an especially busy period. Other HASUs had a day shift (e.g. 07.00–19.30) and a night shift (19.00–07.30). The structure of nurse rotas was partly defined by the London stroke service standards, which in themselves concretise beliefs about the value of certain professional roles. For example, in some hospitals, nurses take on organisational roles, such as patient repatriation and bed management, in the evenings and at weekends. They may also have to occupy two daytime roles, such as nurse in charge and responding to patients arriving in the ED (see Chapter 4, Adapting and extending roles, and Chapter 5, Impact of separating the nurse in charge and thrombolysis nurse roles on clinical processes).
Therapists were mostly employed in standard 09.00–17.00 shifts on weekdays, but some HASUs experimented with extended shifts to 18.00 and short shifts at weekends. This was in recognition of the backlog that would be created without any therapy presence (see Chapter 4, Variations in delivering therapist assessments). However, this was seen as a ‘skeleton service’ rather than full cover.
Patients confirmed that physiotherapeutic activity was restricted to assessments on the weekend, leading to a feeling of disappointment due to lack of progress:
I was a bit disappointed actually because the Thursday was the first day I actually went to physio [physiotherapy]. And I just thought they were so [. . .] they’d got me on the parallel bars, you know see if we can [. . .] you can walk and put [. . .] and I thought this is brilliant, you know, this is what I want, you know, a step forward. And then, of course, it I didn’t have it for 4 days [due to a Bank Holiday]. So that was disappointing.
Patient 15, H7
Medical rotas were characterised by the presence and leadership of consultants during normative working hours (e.g. 09.00–18.00), and junior doctors on day and night shifts. Consultants were present at the weekend and available by telephone at night. However, the correspondence between shifts and the times that medical staff would actually be on the HASU ward was less clear. Patients and carers reported that consultants were hard to access in the late afternoon on a Friday, a time when carers might particularly want advice and discussion:
And they’ll even say it, when you ask if there’s a consultant around, they’ll look at their watches and go, ‘Oh, well, it’s 4 o’clock on a Friday, there’s not going to be anyone here now’ [. . .] So you know, everyone knows that.
It doesn’t matter how sick you are.
Yeah. There’s not, unless they’re on call, there is no-one other than the sisters and the nurses on the ward, there is no-one after 4 o’clock on a Friday.
Our interviews showed broad acceptance of the lowered staffing and testing provision at night. Doctors, in particular, felt that they were not restricted in their management of a patient, ‘There is nothing I would want to have done that would be restricted based on time’ (SHO, H6), despite the limited availability of tests (such as MRI scans and Doppler ultrasound studies) at night (see Table 11 for individual scan availability for each HASU at different times). In particular, it was felt that therapists did not need to be on the ward at night:
There’s always going to be a different need in the day and the nights, I think. You know, the therapists aren’t going to be doing physiotherapy and occupational therapy assessments at 3 o’clock in the morning.
Junior doctor, H8
Despite this certainty, participants expressed uncertainty about when peak activity ended, with some rotas ending too early in the day and potential value in extending therapy hours into the early evening:
I’m not sure that doctor numbers at night need to be the same as during the day because it is normally less busy especially, you know after midnight, things usually quieten down not every night obviously, maybe what a compromise would be would be to have a 7-day service but also the daytime hours to be extended either with consultants or junior doctors later into the evening because actually you know staff start leaving at 5 or 6 and actually that’s when it tends to get very busy. It’s the early part of the evening that is the time that maybe and even for therapists, they often don’t have time to finish seeing all their patients by 4 o’clock which is I believe when they normally leave or 5 o’clock so maybe a slightly longer therapy day.
Consultant, H8
This quotation highlights that patients need therapy to continue beyond a traditional 17.00 or 18.00 shift end. We observed that this was often related to the presence of family members on the ward and patients waiting to be discharged. This may account for the diurnal pattern observed by Bray et al. :22 that delivery of clinical processes can become slower as the day progresses. Participants also debated whether or not medical testing was available, with some seeing availability in relation to necessity:
We can get everything at any time. We don’t always get everything at any time, because there is, of course, out of hours, there is a restriction to saying, is it really necessary, or we would rather do it tomorrow [. . .] So you have to be a little bit more selective in terms of indication for a certain procedure, such as CT angiography or so on. It just has to do with someone has to read those scans, and there are not that many people there at night. However, in general, we can get an MRI, we can get a CTA advanced imaging, for example, any time, and the same with neurosurgical procedures.
Consultant, H3
Other participants saw the differences in availability in relation to the ease with which scans would be approved:
I would say no much different unless, let’s say in anyone has an MRI scan during the daytime it might happen, we can very quickly but at night mostly it will not happen [. . .] because I mean for [. . .] there’s no CD [carotid Dopplers] we can request out of hours if we have a problem. [It’s] a big problem because we need to get radiological approval so we have to go through all the processes.
Junior doctor, H1
In reality, only one HASU was able to carry out MRI at night, and that was through consultant–consultant referral rather than open access (see Table 11). At the weekend, five of the eight HASUs could access MRI, but some were restricted to a limited number of slots per day. Weekends were also characterised by the use of CT angiography rather than carotid Doppler ultrasound studies to examine for carotid disease, driven by the unavailability of ultrasound technicians at weekends to perform the Doppler studies. This quote does reveal, however, that part of the decision-making about what was natural for night-time was also based on whether or not something was ‘necessary’. Necessity was in itself temporally mediated, as the naturalness of daytime work creates an expectation that night-times are for selective actions only.
Temporal rhythms: board rounds, handovers and ward rounds
Clinical meetings and visits, such as staff handovers, the ward round and visiting hours, pattern the day and constitute the framework on which clinical interventions are organised. For a patient to receive evidence-based care, these moments of communication between all the staff members, the patients and their families are pivotal. Almost every HASU we observed had both a board round (sometimes called a MDT meeting) and a ward round every weekday, and a variety of arrangements at the weekend (Table 18). For example, we observed that site H2 had no board round at the weekend, but therapists liaised directly with the nurse in charge about which patients to prioritise. The consultant also made decisions about which patients were ready to be repatriated and communicated this directly to therapists (out-of-hours observation, H2). Although site H3 did have a board round at the weekend, a consultant reported that it was a much smaller group, comprising only one physiotherapist, one occupational therapist and no SLT. A nurse in site H8 reported that a lack of weekend board round made it easier to do other tasks, such as teaching and administration (see Table 18).
HASU site ID | ||||||||
---|---|---|---|---|---|---|---|---|
H1 | H2 | H3 | H4 | H5 | H6 | H7 | H8 | |
Number of board rounds on weekday | Once daily (09.00) | Twice daily (09.00, 16.00) | Three times daily (09.00, 12.00, 16.00) | Once daily (Monday and Tuesday 11.30; Wednesday–Friday 14.30) | Once daily (09.00) | Once daily ‘handover’, twice weekly MDT meeting | Twice daily (08.45, 15.30) | Twice daily (10.00, 16.00) |
Weekend | Same as weekday | None | Same as weekday | Same as weekday | None | Same as weekday | Same as weekday | None |
Discipline leading board round | Medical | Therapies | Medical | Nursing | Medical | Medical | Medical | Nursing |
Individual disciplines often had handovers between ending and starting shifts (nurses, junior doctors) or a team meeting to collate information in advance of the ward round (therapists). These events had to occur before the whole ward board round to contribute to decision-making:
It’s brilliant, on a daily basis, the day staff come in, 7.30, and the shift starts immediately, maybe it takes 30 to 45 minutes, we hand over and we do it thoroughly, we go through every piece of the patients notes, do it thoroughly, from medication, to mobility, whether they’re eating and drinking, their breathing score, their speak and everything, we go through everything by the bedside, their skin and everything that’s going on with them, so we do that for each and every patient, and when you talk to us, come in, I do the ward round, with the therapists and the doctors, so we go through all the patient with the doctors and the therapists before we start the ward round.
Ward manager, H1
The ward round was a single daily event in most HASUs, starting in the morning and lasting 1–2 hours. Some HASUs had a second ward round in the afternoon. Given the short intended duration of patient stay at the HASU, much of the conversation in the board round revolved around new admissions and potential for discharge:
[. . .] so the multidisciplinary team meetings, we have Monday to Friday, twice a day, so at 10 o’clock in the morning, so that we can talk about with the therapists, patients that have come in overnight, and prioritise patients who might be able to be discharged, and let the therapist know, who’s stroke and who’s not a stroke.
Specialist registrar, H8
In HASUs without a weekend board round (sites H2, H5 and H8), our interviews revealed that patients were disadvantaged by needing to wait until Monday morning to see the MDT:
On the weekend, there’s one therapist on a Saturday for 4 hours, and one therapist on a Sunday for 4 hours. Um and so we don’t then have the sort of formal MDT meeting, and those therapists come in, I think, I think it’s, 1 day it’s an occupational therapist, 1 day it’s a physiotherapist, and they come in to try and prioritise, patients that might be able to be discharged over the weekend. Um, so, so we don’t have the MDT meetings on a Saturday or a Sunday. And sometimes patients do build up over the weekend, because they need to be assessed by, by therapists.
Junior doctor, H8
In our interviews, junior doctors, therapists and nurses described how the ward round acted as a stimulus for new work activities, which filled the rest of the afternoon. Every ward also had restricted visiting hours for families, normally starting in the mid-afternoon. The arrival of families and friends while staff were trying to carry out these activities created an intense working phase, with conflicting priorities between the needs of both parties. Families want information and updates on their relatives from doctors and nurses, yet this was a crucial time of day for carrying out diagnostic tests, therapeutic treatments and making discharge arrangements:
I think during the day we do [. . .] I’d say the busiest time of the day really is just [. . .] is maybe [. . .] comes about just after lunch time, really. The patients have been reviewed on the ward round by the medical staff, so some new plans are written up [. . .] so I’d say that’s the most [. . .] that’s probably the busiest part of the day erm, and it’s really the part of the day where we are open, really starting to open to visiting as well, just after lunch, so at that stage we’re going to be having a chat with some of the families and things as well, and trying to update them [. . .]
M01, H4
In some HASUs, the nurse rotas were adjusted to account for this, with overlapping shifts in the early afternoon, to tackle the workload, and shifts extending longer into the afternoon. Consultants do not have this arrangement, and must still engage in assessing new potential stroke patients in the ED or in other wards of the hospital. Patients’ families often wish to speak only to a consultant, which creates a conflict:
We’ve had feedback from the patients and families about access to the consultant. One of our problems is that you know, it’s very busy our HASU, so the consultant will do ward round in the morning, it takes them ’til 11 or 12 every day to finish the ward round. They’ve then got [ED] all through the afternoon. So they may just [. . .] and then they’ve got to do a follow-up ward round late afternoon to deal with all the new things that have happened during the day. So there’s a lot of pressure on them that takes them away from sitting down with the families and talking with them. So I think, and visiting hours is from 3 o’clock onwards. And we used to have open visiting, and it was chaos. I mean, incredibly noisy environment, very, very difficult for rehab, very unrestful. And so on. So we’ve, personally I think that, we’ll try and do this, is that everybody gets a meeting with the consultant to discuss things, but the feedback is it’s not happening all the time.
Consultant, H2
The natural progression of patient pathways also has an impact on the peaks of daily activity on the ward. For example, discharges that are planned early in the day often extend into the evening. This may be caused by waiting for sign-off from a range of professionals (e.g. consultant, therapist), for hospital transport or for a family member to finish work. There are also late admissions to the ward, which are prioritised, delaying consultant approval for discharge.
In one early evening observation, hospital transport to take a patient home was booked early in the morning and was meant to arrive within a 90-minute window around 16.00. The nurses tried to co-ordinate transport with the patient’s care package and, as the transport ran later and later, the nurses became increasingly anxious about the possibility of the patient going home. Many telephone calls were made to the transport provider and to the patient’s social worker. Despite the early-morning booking, the patient could not be guaranteed a transport time because he was low priority compared with patients with higher needs (such as dialysis). The transport eventually arrived at 19.00, but without the wheelchair that was ordered by the nurse. The social worker was still not contactable by telephone and the discharging nurse was concerned about the patient’s medication. They decided to give him the medication on the ward and confirmed that his wife and son were at home to receive him. In another evening observation, we saw a patient being transferred to a local stroke unit by ambulance as late as 21.00. There were concerns that the receiving stroke unit would not have enough staff to accept the patient if the ambulance could not get there on time. This example demonstrates the complexity of intersecting temporal work needed to discharge a patient and how this intense activity can continue into the evening.
At night, all therapeutic activity is suspended and decisions to discharge cease. Some patients will still be admitted and the lack of consultant presence in the hospital was often raised by interview participants as something that affects their workload. The decision to admit a patient at night was made usually by a junior stroke doctor, or sometimes an ED doctor, in most HASUs with the help of a band 5 or 6 stroke nurse.
This creates an extra workload early in the morning for HASU staff, who must assess and try to transfer the non-stroke patients out of the ward. One participant suggested that a registrar could be competent to make the admission decisions at night, but it should be someone with a substantial experience of stroke patients. Many registrars on the ward at night are either covering neurology doctors (with less experience of acute medicine and the stroke care pathways and protocols than the regular day team) or acute medicine- or geriatric medicine-trained doctors (with less experience of neurological conditions that mimic stroke):
Some of the registrars are excellent and have done lots of strokes and you may get a very junior registrar who I think, I think they’ll always going to be safe [. . .] and provide safe care but in terms of efficiency [. . .] and there is something about experience that a consultant would add something in that respect.
Consultant, H8
It is likely that a ward with extra demand from non-stroke patients will have less workforce capacity to conduct timely tests and treatment protocols in line with national guidance. It is important to note that the use at night of doctors who are less familiar with stroke patterns or the stroke service routines than the day team is based on an assumption about the temporal workload during this time. Nevertheless, this has an impact on the processes of care around ward capacity and pathway management the next day, exemplifying the contingent and deterministic nature of the concept of ‘out of hours’: working activities at night affect activities during the day and vice versa.
Temporal norms: acceptance of differences in night and weekend activity
Despite the organisation of the HASU to provide certain aspects of the service on a 24/7 basis, the cultural expectation of some HASU staff and more widely in the hospital was that provision of certain types of care at night and at weekends is just for emergencies. For example, although it was deemed routine to gain additional information about the diagnosis using a MRI in addition to a CT head scan during the week, this was often not considered a priority at weekends, despite the presence of radiology teams:
So I think there’s a bit of a culture change about, well, you know, it may not be a life-saving investigation but, you know, this allows the patient to be processed much more efficiently and quickly if it’s done now, rather than Monday, so it does seem a culture that you know, that can wait ‘til Monday because it’s not urgent or emergency. [. . .] sometimes to get an opinion, a radiology [. . .] a consultant opinion, is quite difficult out of hours. [. . .] It’s difficult enough in the week, actually, for us, but doing Saturday and Sunday, that just doesn’t happen.
Consultant, H4
It is important to note that that during the day the HASU consultant usually determines the treatment plan and the investigation pathway, and will control resources to prioritise some patients over others for tests, such as MRI, when there is finite capacity. In contrast, at night, the decision-making about treatment and investigations is, to a large extent, the responsibility of the onsite team without involvement of the consultant. The contrast at night and at the weekend contributes to a sense of having to work hard to ‘get things done’, leading to avoidance or deferral, or laborious referral procedures, such as arranging tests by direct communication from consultant to consultant:
There’s probably one extra step on the phone call trying to organise the CT scan because you call the radiology registrar and explain that you want a scan rather than calling CT directly whereas during the day you tend to just order CT and they will get a radiologist to report your scan or they automatically get done during 9–5, you want a quicker result then you call them. But it’s more like a permission in a way because you know CT won’t do it without a radiology registrar calling them and saying you can do a scan.
Junior doctor, H4
This deferral could have knock-on effects for patients who were waiting for specific tests to be discharged, leading to frustration:
I was up on there for 5 days just for an echocardiograph. Er [. . .] my wife came in on the, er, Monday or, Monday, yeah, because I’d already been up, taking up a bed for 2 days where I couldn’t do anything. [. . .] why I wasn’t the first one down there on the Monday to get me out of the place. I was just surprised. And then when they say, ‘Are you still here?’ I’m going, ‘Can you found out?’.
Patient 28, H3
How temporal structures have an impact on measurement of temporal variation
The third section of this chapter describes how the temporal structures outlined above result in measured temporal variation. Temporal variation in stroke has mainly been assessed using the SSNAP data set. This has the advantage that it was a stroke-specific database relating to nationally agreed standards of care, but carries the risk that the metrics imposed as a measure of quality in this database could become concretised as definitive of care quality more widely.
Temporal imperatives relate to outcomes and not quality
The consensus measures in the SSNAP are taken from national guidelines for stroke93 and mostly concern the timing, rather than the nature, of interaction. For example, therapists who did work at the weekend described being more likely to do assessment work to meet SSNAP targets than to deliver therapy:
At the weekend you have either an OT [occupational therapist] or a physio[therapist] and it’s reduced hours. So hardly, not much at all. Em, so it becomes a totally different role, so you’re just, er, doing a quick assessment of people and making sure that basic therapy needs are met. But it’s a different role at the weekend anyway because again those, the office people aren’t working, ESDs [early supported discharges] aren’t there and discharge planning can’t really be done.
G01, H8
This was echoed by patients, who reported being stationary in bed over the weekend while there was limited therapist presence:
But after that day, I got quite distressed about being in bed for so long. [. . .] by the evening, I said, ‘I just need to get up, please, put me in a chair anywhere’. You know, ‘My back has been on this mattress now for so long, I just can’t take it, I need to move’. [. . .] Yes, [the nurse], she said I had a red mark on my back. I said, ‘Have I?’ I said, ‘Yes’ [laughs] it was a red mark on your back. You’re getting on the mattress so that was alright.
Patient 18, H4
We suggest, therefore, that many quality indicators are not included in the temporal structures of quality metrics. These could include more information on the actual therapeutic processes and activities taking place, and how these were experienced by the patient and their family. It could also include ‘softer’ outcomes, such as bed sores, muscle strength, mood, etc.
Rotas and temporal rhythms are more deterministic than time of admission on longer time-related indicators
Looking further at the limitation of the longer binary temporal variables (e.g. whether or not a patient was seen by a consultant within 24 hours, whether or not the patient saw a therapist within 72 hours), the time of admission is no longer fully relevant. Figure 18 illustrates this problem: the lines shown are based on the median time intervals for patients arriving in the time slots indicated from our SSNAP data set. Patients A and B both arrive on a weekday in hours. However, patient A sees a therapist after 24 hours and patient B sees a therapist after 60 hours because there are no therapists on the HASU over the weekend. Patient C arrives on a Sunday (out of hours), but sees a therapist relatively quickly when they return to work on Monday.
FIGURE 18.
Exemplars of patient trajectories and temporal variation in relation to the time and day of admission based on median SSNAP data for the time periods indicated.
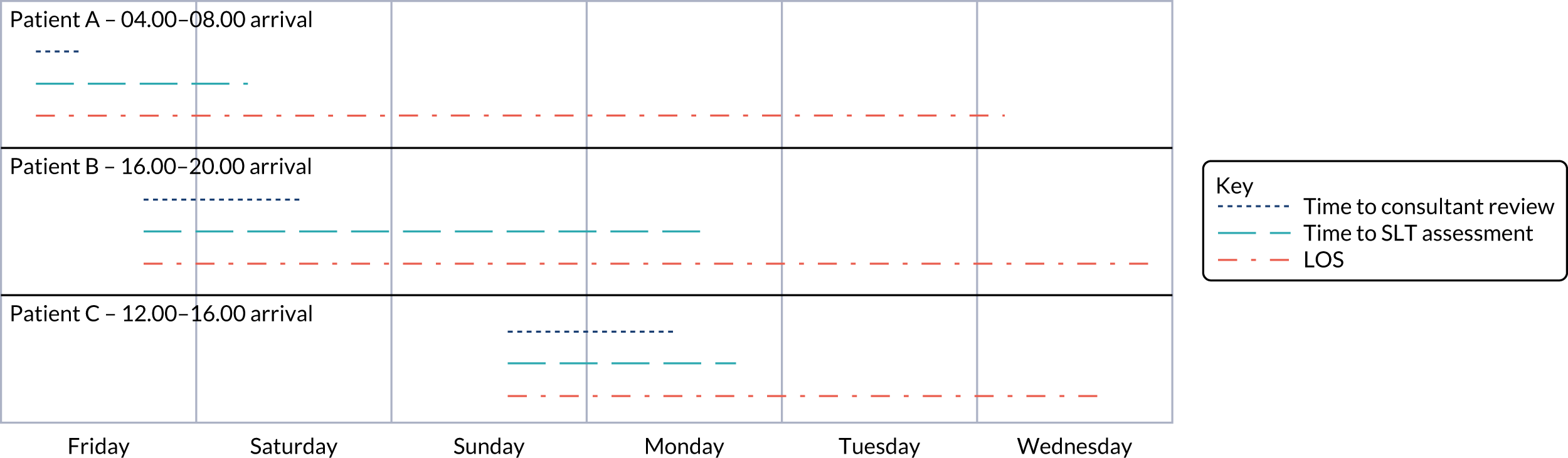
Therefore, looking at outcomes such as therapist contact as a binary variable in relation to the time or day of admission was meaningless, for two reasons: (1) the SSNAP and London stroke standards accept that therapists do not work over the weekend, showing a cultural expectation that this state of affairs was normative and did not affect patient outcomes; and (2) the trajectory of the patient pathway in relation to therapist ward rotas dictates the length of time taken to see a therapist, not the time of admission. Similarly, the time taken to see the consultant was directly related to the patient pathway in relation to the morning ward round, not whether their time of admission was in hours or out of hours.
Temporal consistency at the patient level does not capture bottlenecks or other ‘knock-on’ effects
Patient-level data cannot capture the important peaks and troughs in activity at a ward level, or the relationship between ward activity and individual patient-level care. In Chapter 4 we discussed how temporally inconsistent care created delays in repatriation (see Chapter 4, Factors influencing length of stay). Backlogs and bottlenecks were created by inconsistent staffing, such as the junior doctors’ risk-averse admission behaviours at night (when more stroke mimics are admitted) (see Chapter 4, Unintended consequences of adaptations) or the reduced therapy presence on the weekend.
This phenomenon reveals the limitation of patient-level data: it is not the patient being admitted at the weekend who is affected, but the one who arrives on Monday to a HASU with highly pressured therapists who are struggling to resolve the backlog of unseen patients. Separately, temporal variation in the admission of stroke mimics may also cause periods of high staff pressure and workload. This is due to stroke patient displacement into wards away from the HASU and the transfer of resources from patients on the stroke pathway to those without stroke but occupying stroke beds, which in turn could lead to adverse outcomes for stroke patients.
Inconsistencies in staff pressure could affect risk levels and lead to staff ill health and suboptimal patient care. This was exemplified in one Wednesday observation (site H3), when we witnessed ‘knock-on’ effects late in the day due to discharge delays. We arrived at 16.30 to find that the ward was already over capacity and there were patients in the ED waiting to be admitted. The atmosphere was hectic, as the consultant and junior doctors worked into overtime to try and empty the beds. Owing to the pressures on the ward, they had not had their second board round of the day. In this case, the consistency with which the staff were able to deliver interventions was directly affected by the delays incurred by fact that care needed to be delivered to patients who were about to be discharged. Conversely, the patients being discharged may have been at higher risk of poor care, given the pressure to move them out of the ward quickly.
Discussion
The SSNAP and London stroke service standards dominate the timing of clinical events in the ‘front-door’ part of the patient pathway through setting of micro time limits that are evidence linked to clinical outcomes (1 hour, 4 hours, etc.). The SSNAP metrics later in the patient pathway have longer time limits (24 hours, 72 hours) that are not indicative of clinical benefit but reflect cultural norms about rotas and activities, such as ward rounds, which have financial implications. Temporal variation in events that occur > 1 hour after arrival in terms of ‘in-hours’ and ‘out-of-hours’ arrival periods is not necessarily a good measure of consistency, as the punctuation of temporal structures, such as therapy rotas and ward rounds, is what determines clinical contacts. Repatriation from the HASU was temporally structured by the working patterns of other departments and organisations. Measuring temporal concepts, such as clustering, pressure and bottlenecks, is as important as consistency.
Summary
-
Assessment of temporal variation requires a new paradigm, in which markers of quality reflect the whole pathway and incorporate better information about risk, safety, temporal delay and patient-centred outcomes (e.g. management of fluid balance and impaired swallow function).
-
This could include routinely collected markers of ward complications, such as chest infections, thrombolysis haemorrhages, falls (i.e. safety in general).
-
Temporal structures should be considered with respect to variation (e.g. different patterns of ward rounds or rotas and how these can have an impact on pressure, safety and patient experience).
-
Outcomes that are measured only in immediate proximity to time of arrival should be measured in relation to this point in time (e.g. within 4 hours). Other outcomes could be measured relative to agreed standards, such as daily physiotherapy, aspirational rates of infection or falls and psychological well-being.
Chapter 7 Discussion
Overview
This study used mixed methods to identify the extent to which London stroke services were providing 24/7 care and, if they were, how it was being achieved. The research presented a range of organisational factors that influence 24/7 provision of stroke care, such as staffing rotas and availability of scans, assessing the extent to which temporal patterns of provision are reflected in patient outcomes, including mortality, LOS and functional independence. Our five research objectives were to investigate:
-
the extent to which evidence-based care received by stroke patients differs between in-hours and out-of-hours services in London HASUs
-
the extent to which clinical outcomes differ between in-hours and out-of-hours services in London HASUs
-
how in-hours and out-of-hours services are organised and provided in London HASUs
-
how these services are perceived by stakeholders (including stroke clinicians, patients and carers)
-
the costs of acute stroke care in London HASUs on different days and at different times of admission.
In this chapter, we present a summary of our principal findings, linked to our research objectives. We then discuss the implications of these findings, as well as the strengths and limitations of our study, and propose a future research agenda.
Summary of main findings
Our analysis of the extent to which evidence-based care (objective 1), clinical outcomes (objective 2) and costs (objective 5) differ between in-hours and out-of-hours services in London HASUs found the following:
-
London HASUs do not operate a 24/7 service in all aspects of acute stroke care. However, nor are they designed to.
-
There was no variation in 3-day mortality and mRS score at hospital discharge by day of admission.
-
In accordance with national and local stroke standards, London HASUs do not vary temporally in quality of care by day and time of admission across the week in terms of timely access to:
-
brain scanning
-
stroke nursing care
-
thrombolysis.
-
-
Other time-dependent quality-of-care measures did significantly vary across the week in London HASUs.
-
Three patterns of variation were detected: (1) by time of day, but not by day of the week; (2) by day of the week, but not by time of day; and (3) by time of day and by day of the week.
-
We found that there was little variation in the cost of care processes, including receipt of thrombolysis across the week, although LOS on the HASU and total LOS were longer for patients admitted during the weekend.
-
When incorporating total LOS, the additional mean cost per patient was £445–668, depending on when the patient was admitted during the weekend.
-
Those who were admitted 20.00–07.59 on a weekday had similar costs to those who were admitted 08.00–19.59 on a weekday.
Our analysis of temporal variation in nurse staffing and the effect on quality-of-care measures (objectives 1–3) found the following:
-
Many HASUs timetable nurses at higher bands (e.g. band 7) to take on senior nursing tasks, such as the nurse-in-charge role, during the day on weekdays.
-
Quality of care was better if the nurse in charge was a higher band and/or there were more nurses on duty.
-
The more senior the nurse in charge, the less likely it was for a patient to see a stroke physician within the first 12 or 24 hours.
-
Nurse staffing had a direct effect on quality-of-care measures soon after arrival at the hospital (brain scan within 1 hour, dysphagia screen within 4 hours, admission to a stroke unit within 4 hours).
-
Wards are organised and made efficient by senior nurse care.
-
In both the acute admission area and the ward area of hyperacute stroke units there are some specific tasks that require the attendance of a senior nurse.
-
Senior nurse care was demonstrated to make a difference to some outcomes, but this may be compromised if the senior nurse has to manage two areas.
-
Junior nurses in some HASUs are trained to perform certain tasks so that they can ‘act up’ to senior roles; however, this does not enable them to juggle the many demands of the senior role that are so different from junior tasks.
Our analysis of how in-hours and out-of-hours services are organised and provided in London HASUs (objective 3) and how this was perceived by stakeholders (including stroke clinicians, patients and carers) (objective 4) found the following:
-
24/7 services were built out of ‘old-fashioned’ models of team practice.
-
This was minimally disruptive to previous practices: ward rounds and therapy rota models stayed the same.
-
Consistency was achieved by:
-
adapting and extending roles
-
creating continuities between different times of day
-
building relationships and trust
-
prioritisation of ‘front-door’ interventions.
-
-
There are unintended consequences of adaptations, however, such as delays in repatriation, higher levels of pressure for staff and increased admissions to the HASU at night.
-
Variation persists due to inconsistencies in:
-
medical, managerial and allied health professional staffing by time of day
-
delivery of therapist assessments
-
barriers in repatriation processes.
-
Our study has revealed a new way of thinking about temporal consistency, characterised by the following:
-
The SSNAP and London stroke service standards dominate the timing of clinical events in the ‘front-door’ part of the patient pathway through setting of micro time limits that are evidence linked to clinical outcomes (1 hour, 4 hours, etc.).
-
The SSNAP metrics later in the patient pathway have longer time limits (24 hours, 72 hours) that are not indicative of clinical benefit but reflect cultural norms about rotas and activities, such as ward rounds, which have financial implications.
-
Assessing temporal variation for events that occur > 1 hour after arrival in terms of ‘in-hours’ and ‘out-of-hours’ arrival periods was meaningless, as the punctuation of temporal structures, such as therapy rotas and ward rounds, is what determines clinical contacts.
-
Repatriation from the HASU was temporally structured by the cultural norms and working patterns of other departments and organisations.
-
Measuring temporal concepts, such as clustering, pressure and bottlenecks, is as important as consistency.
Implications of these findings
Part of the value of this study has been the chance to evaluate the study of temporal consistency as a whole. As such, we have developed new ways of analysing routine data (see Chapter 3) and have demonstrated the benefits of gathering other forms of data (e.g. nursing rotas, see Chapter 5) and qualitative data (e.g. interviews and observations to understand variation from a patient and professional perspective, see Chapters 4–6). This enabled us to move from an ‘in-hours’ and ‘out-of-hours’ comparison to granular analysis of processes and outcomes over time (see Table 6).
This also builds on previous studies40 of the London HASU structural reorganisations by showing, for example, the downstream effect of strong designated leadership on HASU culture and ethos.
Implications for whether or not acute stroke services can be temporally consistent (24/7)
London HASUs were designed to be 24/7 services, but were built out of ‘old-fashioned’ models of team practice in a way that was minimally disruptive to previous practices. This can be likened to ‘sedimentation’, whereby elements of the new culture merely sediment over the values and structures of the old culture. 94 This has been recognised as a limitation to aspirations for ‘transformational’ change in health care. 95
Hyperacute stroke units have been able to produce temporal consistency in provision of some crucial interventions and clinical outcomes; however, we found deep-rooted patterns of variation on HASUs in a number of key areas:
-
Medical and nursing staff were reduced in number and seniority at night, and junior staff had to ‘act up’ to fulfil senior responsibilities.
-
Therapy provision was a contentious issue, with some HASUs attempting 7-day provision and others not (see Chapter 4, Variations in delivering therapist assessments).
-
Those that did attempt 7-day provision found that their staff were stretched thin and only basic assessments were completed over the weekend.
-
Many patterns of working on the HASU still have observable variation in the way that rotas are constructed in different professional groups. For example, the rotas of all key staffing groups (doctors, nurses and therapists) remain based around conventional working-week models.
-
This is seen most strongly with the therapy teams, who work only conventional working hours 5 days per week, and least seen with the nursing teams, in which staffing is more often timetabled to meet peaks in activity.
-
Many hospital departments had no access to testing at night or only with consultant-to-consultant request, in a stark contrast to the facilitated pathways during the week.
-
Repatriation to social services and rehabilitation centres is bottlenecked by entrenched 5-day working patterns.
For stroke and other services to achieve greater gains in temporal consistency, they will need to overcome cultural acceptance of ‘difference’ at weekends and at night. Otherwise quality improvement will continue to be hampered and lead to frustration among patients who cannot progress to repatriation or make therapeutic advances.
We gathered data in each London HASU at different times of day, both during the week and at weekends, providing a rich picture of the realities of organising and providing a high-performing acute care system. Our study has provided detailed information about the organisation and management of the HASU, particularly in terms of nursing (see Chapter 5). Our study suggests the following:
-
Senior nursing management is crucial to a well-managed ward and high-quality patient care.
-
Both HASUs and EDs are dynamic and, if the clinician responsible for organising an interaction between the CT scanner and these two environments is in charge of both the patient in the ED and the preparation of the potential bed-space in HASU after brain scanning, this task may well be performed more efficiently.
-
In addition to the urgent patient assessments, our observations identify that those patients presenting to the ED who do not need an immediate input could be deprioritised, either by junior nurses in the tPA role or by the team in the radiology department.
-
Senior stroke nurses working in the ED (and controlling the HASU bed space) may be more aware of the potential for this deprioritisation to result in poorer and slower care, and so manage both the urgent and the non-urgent pathways more effectively than junior nurses with less awareness.
-
Unsurprisingly, a nurse who is acting as both ward manager and tPA nurse is able to manage transitions well, but they neglect the management of essential interventions, such as dysphagia screening.
-
Patients who are not eligible for thrombolysis could be perceived as less urgent, either by junior nurses in a tPA role or by, for example, radiology: we showed that a senior nurse manages this process better for non-thrombolysis patients.
Our finding that managerial nursing seniority varies between weekdays and weekends and between day and night is novel, and has broad implications. We did not find that nurse-in-charge seniority had an effect on 3-day and discharge mortality in consort with studies looking at medical specialist intensity,31 which could be interpreted as consistent quality. A contrasting interpretation of these findings, however, is that staff are able to ‘step up’ in junior roles or ‘rescue’ patients in particularly serious situations. However, some authors, such as Dixon-Woods,96 have cautioned that normalisation of these workarounds as a way of adapting to staff shortages and bed pressures can be an inadequate defence against patient safety threat when staff are inexperienced or undermanaged. Regular use of stretched junior staff may also lead to unforeseen consequences, such as that being constantly under strain is normal and valuable, rather than a sign of mismanagement. 96 Therefore, stroke services should not interpret our results as evidence that wards can be equally well managed by junior nurses, but that infrequent use of junior staff as subtitutes can be safe, but should not be normalised.
An example of this is to look at processes rather than outcomes. Indeed, we did find that senior nurses in charge of the ward were more likely to ensure dysphagia screen assessment unless also working as thrombolysis nurses (see Table 16) than junior colleagues. Other studies have also shown the important relationship between well-trained nursing staff, dysphagia and mortality. 87 Without long-term mortality data, our ability to perceive an impact on patient outcomes is limited. However, other studies have found that missed dysphagia screening predicts higher odds of pneumonia, severe disability, discharge to long-term care and 1-year mortality. 97 Furthermore, considered in conjunction with our findings about reduced therapist provision over the weekend, the risk of pneumonia and, therefore, long-term poor outcomes, may be further increased: a study of stroke patients found that delays in dysphagia screening and speech and language therapy dysphagia assessment were associated with a higher risk of pneumonia, with an absolute increase in the incidence of pneumonia of 1% per day of delay. 89
Our novel findings about this inpatient setting may have generalisable implications for 7-day working and bed management more widely. For example, the observed extension of nursing roles to create continuity could have widespread implications for NHS recruitment and training in the transition to 7-day services. Strategies that we found to be important included creating ward capacity, staff acting up to other roles and achieving a degree of self-sufficiency in making thrombolysis decisions. The flexible use of adjacent ward space to create free beds, and the staff prioritisation of new patients and those ready for discharge, is consistent with existing literature, suggesting that emergency room staff anchor working practice around admission and discharge possibilities. 98
The second implication is that we found evidence of no time-of-admission effect across the week on early outcomes in acute stroke patients admitted to a London HASU. We also found no evidence of an effect in terms of rapid access to brain scanning, stroke nursing care and thrombolysis in London HASUs. Other quality-of-care measures did significantly vary across the week in London HASUs. Therefore, in terms of the outcomes considered here, and for ‘front-door’ quality-of-care measures, the centralised model in London would seem to be more effective at providing 24/7 care. Several issues are worth bearing in mind. First, our study focuses on patients admitted to London HASUs only, not other hospitals in London; our data suggest that 6% of acute stroke patients in London are not treated in a HASU. Our focus on London HASUs was deliberate, as the aim of our study was to evaluate if they operate 24/7 care, but it means that our findings for London HASUs should not be generalised to all stroke patients in London. Indeed, there is evidence that quality of care is lower for the 6% of acute stroke patients in London not treated in a HASU than for those who are treated in a HASU. 41,99 Hence, better adherence to HASU referral protocols in these patients is likely to mean that the quality of care that they receive will increase. Another issue is that the centralisation of acute stroke services in London was not without cost; the additional cost required as a result of the higher cost per bed-day in a HASU was estimated at £20M. With this additional level of funding, it might be expected that there should be little or no variation in quality of care and outcomes across the week in London. There is some evidence that the reorganisation in London was cost-effective, but further analyses accounting for the size of the up-front investment, the relatively high costs per day of hyperacute stroke care, the impact on mortality and functional outcome, and the lifetime costs incurred by the NHS, social services and families caring for stroke survivors at different levels of functional outcome would be helpful.
Implications for the study of temporal variation in acute stroke services
There is a large literature examining weekend effects in health care across a range of clinical areas (see Chapter 1). In agreement with more recent studies22 we found that (1) there is no significant temporal variation in HASU care in London in all but a few clinical variables; (2) mortality is not affected by time and day of admission when controlling for baseline patient characteristics and case mix; and (3) temporal variation in a wider range of variables should be measured. 22 The study of temporal variation is restricted by reliance on routinely collected data sets, such as the SSNAP, that themselves enshrine some of the cultural norms of daytime, 5-day working in the metrics relating to consultant and therapist contact. In the case of stroke, the SSNAP data set provides a more accurate record of stroke patients’ clinical interventions and outcomes than other hospital data, but has not shown more severe variations in outcome than other hospital data sets. 100 We suggest that:
-
other indexes of quality should be derived from patient-centred principles, and assessed for temporal variation
-
staff in a successful system work to fulfil the commitments of their audited service standards, and this has driven temporal consistency in London’s HASUs in the metrics relating to ‘front-door’ provision
-
temporal variation remains further along the patient pathway and may influence long-term patient outcomes
-
assessment of temporal variation for improvement is limited when success or quality are being assessed by what happened, rather than assessing whether or not potential risks were taken, or whether or not the service was optimal.
This has been highlighted in cases of grave misconduct, such as the Morecambe Bay investigation, where the reliance on ‘high-level data may not reveal underlying risks’ (Reproduced with permission from Morecambe Bay Investigation copyright 2015. The Report of the Morecambe Bay Investigation101). Another way to characterise this is the over-reliance on ‘hard metrics’, such as incidents, and not enough attention to ‘soft metrics’, such as cultural impediments and social practices, as identified by senior leaders of health systems. 102 We hope that the results of this report contribute to soft intelligence in striving to meet the goals for 7-day working.
Strengths and weaknesses
We gathered data in each London HASU at different times of day, both during the week and at weekends, providing a rich picture of the realities of organising and providing a high-performing acute care system. In terms of our analyses of how temporal consistency was and was not achieved, our main strength was observing and comparing how eight sites organised themselves in different ways to meet the same standards. Furthermore, we collected in-depth qualitative data collected at all times of the day and week, to analyse a large centralised acute stroke system that has been demonstrated to deliver better care and outcomes. This affords generalisability to our results.
In our analyses of whether or not London HASUs generate temporal consistency in terms of clinical outcomes and processes, our main strength is the large number of data used, which contains detailed audit data, including patient characteristics, clinical processes and outcomes, as well as a unique nursing rota data set.
Unlike previous studies (see our literature review in Chapter 1, The ‘weekend effect’ and 7-day working policies), we have taken a critical approach to temporal variation and its measurement, developing our own variables, such as whether or not the roles of nurse in charge and thrombolysis nurse were fulfilled by the same person. Our outcomes were stroke mortality and disability, whereas previous studies have focused on mortality. The rich set of patient characteristics in the data set meant that we could control for patient factors likely to affect quality of care and outcomes that vary by day and time of admission across the week.
There were several limitations to the study. First, we were unable to measure long-term outcomes, as our request for these was not granted. Mortality data in the SSNAP are currently available only for patients who are in hospital and, therefore, to reduce the risk of bias, we measured mortality at 3 days after admission, when most patients will still be in hospital. Three-day mortality has been used in previous studies to evaluate the centralisation of acute stroke services in London. Similarly, long-term functional outcome data are not available in the SSNAP, and so the long-term functional outcome was measured by mRS score at the end of the inpatient spell. This will be improved with the recent addition of a Commissioning for Quality and Innovation payment requiring completion of 6-month reviews. This is measured at different points in time for different patients, so to control for this we included an indicator for number of days after admission at which this outcome was measured.
We did not gather any (qualitative) data in hyperacute stroke services operating within a different service model (whether centralised or non-centralised). The lack of a comparator (e.g. a centralised model of care) limited our confidence that our findings explain 24/7 care per se with respect to our quantitative analysis.
Second, we did not interview all relevant professions within the studied organisations (e.g. pharmacy, emergency medical practitioners). Therefore, our perspective on important working relationships beyond the HASU was based on HASU staff perceptions, although they were supported by our own observations, particularly of the ED co-ordination. Although we had an interdisciplinary research team, we could have gained from training a patient researcher to conduct some of our interviews and observations. Finally, these services develop constantly, and some aspects of provision, such as staffing levels, are likely to have changed.
Furthermore, there are specific limitations relating to the SSNAP data set. Although case ascertainment (the selection of patients for the data set) in the SSNAP was 90% during the time period of our study, these data might not be representative of all stroke patients. Although analyses of hospital administrative data to investigate weekend effects in stroke have been undermined by evidence of variations in inaccurate coding across the week, SSNAP data were collected on a voluntary basis by hospitals and we cannot exclude the possibility of inaccurate or selective reporting. Particularly problematic for our study would be if this bias was more likely to vary by time of admission.
Although the richness of our data set means that we have been able to control for confounding factors, we cannot exclude the possibility of confounding due to unobserved patient characteristics. Although the sample size of our study is large, when evaluating quality of care and outcomes across the week the number of observations in each of the 42 time periods was relatively small. We cannot exclude the possibility that this resulted in wide CIs around the adjusted predicted probabilities in each time period, making the analysis less likely to show significant variation.
Recommendations for research
The London HASUs were an attempt to build a 24/7 service, and we have shown that these were built onto 9-to-5, 5-day models of delivery, creating a hybrid that is demonstrated in the temporal consistencies and inconsistencies of its processes and outcomes. There is now an opportunity to refine this model to achieve greater levels of consistency. Research is needed to discover the best patterns for therapeutic coverage, rota lengths, ward rounds, etc. The Stroke Sentinel National Audit Programme suggests that the organisational factors we have identified as being important in achieving temporally consistent outcomes, such as daily consultant ward rounds and differentiated junior and senior nursing roles, are not replicated elsewhere in the UK. 103 Therefore, further research would help to further inform how 24/7 acute stroke services ought to be designed in the future to maximise patient outcomes in a cost-effective manner. Researchers also need to examine other efforts to deliver clinical interventions 24/7 in stroke and other clinical settings. In-depth analysis of the interdependencies that influence 24/7 delivery of care, both within and beyond the host hospital, would be of value.
Future research into temporal variation will continue to be based, at least in part, on clinical audit data such as SSNAP data. Although we have outlined the limitations of this resource, its accuracy and coverage make it a unique and invaluable source of data. Researchers using these data should consider absolute time intervals rather than binary fulfilment of the SSNAP criteria, as well as patterns of timing (e.g. clustering and limit-stretching periods of higher clinical risk), to accurately visualise temporal variation by times of admission. In addition, researchers should collect variables in accordance with their own values and patient-centred indicators of quality, such as sedentary periods for patients, management of fluid balance, impaired swallow function and avoidable delays in repatriation, and assess those for temporal variation. Outcomes other than mortality and mRS score that have meaning to patients should be linked and incorporated.
We suggest that new types of temporal variation questions should be asked of stroke care, based on patients’ needs, taking a wider approach to quality. The key targets for clinical improvement lie in looking at cultural norms and practices in the hospital outside the HASU as well as stroke units, rehabilitation centres and other repatriation routes for patients. More research into optimal time frames for therapies and other interventions rely on the availability of longer-term morbidity data and the capturing of information about what type of intervention is being made by therapists at each contact with patients. Discussions about staff rotas should be had on an interprofessional basis, so that ideas about when peaks and troughs of activity will fall will be shared.
The methods we have employed could be usefully used to understand performance in more focused analyses of different service models in different locations. Further research would be beneficial to evaluate the impact of stroke admission at different times of the week on longer-term mortality and disability outcomes, and to investigate the relationship between quality of care and outcomes and if this relationship varies by time of admission. Further research would also be useful to investigate the reasons why for some standards, care in London HASUs was constant across the week, irrespective of day and time of admission, but for others it was not. In addition, accounting for the organisational factors at the stroke unit level could explain an important part of the variation in quality of acute stroke care and outcomes by day and the time of admission in London HASUs. This research would help to further inform how acute stroke services ought to be designed in the future to maximise patient outcomes in a cost-effective manner.
Conclusions
Movement towards temporal consistency in health care is part of UK policy and the research basis for organising this movement is developing. Part of creating temporal consistency will be in the design of services, including staffing, targets and patient pathway design. Our study has shown that NHS staff are adept in learning how to meet targets and can achieve new standards that save lives. The attention to tight, ambitious targets is what enables meaningful change. Enshrining cultural values that are discordant with temporal consistency within targets are not and have financial implications (improving therapy services at weekends will take a financial investment and not just changes to rotas). Mixed-methods studies such as this show that temporal consistency is possible and how it can be achieved. The measurement of temporal consistency should acknowledge how services are organised and not just rely on routinely collected or audit data, as this is focused on outcomes.
Acknowledgements
The authors would like to thank the Study Steering Group, in particular Professor Pippa Tyrell, the patient representatives Gareth Jones and Stan Spinks, all the HASU staff who facilitated our qualitative data collection and were so kind and helpful, and the patients and their families who contributed to the study.
Contributions of authors
Robert Simister (https://orcid.org/0000-0003-0578-6711) (Lead Consultant) was the principal investigator and led the study, provided oversight for all the qualitative and quantitative analyses, and led the discussion (see Chapter 7). He contributed to the design and analysis of all aspects of the research, and is lead author of the final report.
Georgia B Black (https://orcid.org/0000-0003-2676-5071) (Senior Research Associate) co-led the analysis of how temporal consistency is achieved (see Chapter 4), led the analysis of temporal structuring of HASU wards (see Chapter 6) and contributed to the study of nurse staffing (see Chapter 5). She contributed to study design, to qualitative data collection, to all qualitative, quantitative and mixed-methods analyses, and to the writing of the report.
Mariya Melnychuk (https://orcid.org/0000-0003-4992-1612) (Research Associate) led the analyses of temporal variation in stroke care (see Chapter 3) and nurse staffing (see Chapter 5). She led the quantitative data requests, contributed to all quantitative and mixed-methods analyses, and to the writing of the report.
Angus IG Ramsay (https://orcid.org/0000-0002-4446-6916) (Senior Research Fellow) contributed to the conception and design of the work. He co-led the analysis of how temporal consistency is achieved (see Chapter 4), and contributed to all qualitative, quantitative and mixed-methods analyses.
Abigail Baim-Lance (https://orcid.org/0000-0001-8602-6022) (Research Assistant Professor) contributed to the qualitative study design, to qualitative data collection and to all qualitative analyses.
David L Cohen (https://orcid.org/0000-0001-7318-8567) (Consultant Stroke Physician) contributed expert knowledge on the organisation and provision of acute stroke services in general, and the planning of 24/7 services in London. He contributed to the study design and all analyses.
Jeannie Eng (https://orcid.org/0000-0002-7592-4246) (Clinical Research Practitioner) led the qualitative data collection and managed the project. She contributed to all qualitative and quantitative analyses.
Penny D Xanthopoulou (https://orcid.org/0000-0002-1510-3382) (Research Fellow) contributed to the qualitative study design, to qualitative data collection and to all qualitative analyses.
Martin M Brown (https://orcid.org/0000-0002-3273-1356) (Professor of Stroke Medicine) contributed to the conception and design of the work, and contributed expert knowledge on the organisation and provision of acute stroke services in general. He contributed to all quantitative and qualitative analyses.
Anthony G Rudd (https://orcid.org/0000-0002-5738-2703) (Professor of Stroke Medicine) contributed to the conception and design of the work, and contributed expert knowledge on the organisation and provision of acute stroke services in general. He contributed to all quantitative and qualitative analyses, and to the Study Steering Group management.
Steve Morris (https://orcid.org/0000-0002-5828-3563) (Professor of Health Economics) provided oversight for all the quantitative analyses. He contributed to the study design, quantitative data requests and all qualitative and quantitative analyses.
Naomi J Fulop (https://orcid.org/0000-0001-5306-6140) (Professor of Health Care Organisation and Management) provided oversight for all the qualitative analyses and led the analysis. She contributed to the design and analysis of all aspects of the research.
Publications
Melnychuk M, Morris S, Black G, Ramsay AI, Eng J, Rudd A, et al. Variation in quality of acute stroke care by day and time of admission: prospective cohort study of weekday and weekend centralised hyperacute stroke unit care and non-centralised services. BMJ Open 2019;9:e025366.
Black GB, Ramsay AI, Baim-Lance A, Eng J, Melnychuk M, Xanthopoulou P, et al. What does it take to provide clinical interventions with temporal consistency? A qualitative study of London hyperacute stroke units. BMJ Open 2019;9:e025367.
Data-sharing statement
The quantitative data used in this study were obtained by formal application to the SSNAP. The agreements in place for these data do not permit further distribution or sharing. Requests for the relevant data sets must be made directly to the SSNAP. All qualitative data generated that can be shared are contained within the report. The nature of the data means that nothing else can be provided. Further information can be obtained from the corresponding author.
Patient data
This work uses data provided by patients and collected by the NHS as part of their care and support. Using patient data is vital to improve health and care for everyone. There is huge potential to make better use of information from people’s patient records, to understand more about disease, develop new treatments, monitor safety, and plan NHS services. Patient data should be kept safe and secure, to protect everyone’s privacy, and it’s important that there are safeguards to make sure that it is stored and used responsibly. Everyone should be able to find out about how patient data are used. #datasaveslives You can find out more about the background to this citation here: https://understandingpatientdata.org.uk/data-citation.
Disclaimers
This report presents independent research funded by the National Institute for Health Research (NIHR). The views and opinions expressed by authors in this publication are those of the authors and do not necessarily reflect those of the NHS, the NIHR, NETSCC, the HS&DR programme or the Department of Health and Social Care. If there are verbatim quotations included in this publication the views and opinions expressed by the interviewees are those of the interviewees and do not necessarily reflect those of the authors, those of the NHS, the NIHR, NETSCC, the HS&DR programme or the Department of Health and Social Care.
References
- Bell CM, Redelmeier DA. Mortality among patients admitted to hospitals on weekends as compared with weekdays. N Engl J Med 2001;345:663-8. https://doi.org/10.1056/NEJMsa003376.
- Freemantle N, Richardson M, Wood J, Ray D, Khosla S, Shahian D, et al. Weekend hospitalization and additional risk of death: an analysis of inpatient data. J R Soc Med 2012;105:74-8. https://doi.org/10.1258/jrsm.2012.120009.
- Lilford RJ, Chen YF. The ubiquitous weekend effect: moving past proving it exists to clarifying what causes it. BMJ Qual Saf 2015;24:480-2. https://doi.org/10.1136/bmjqs-2015-004360.
- Kostis WJ, Demissie K, Marcella SW, Shao YH, Wilson AC, Moreyra AE. Myocardial Infarction Data Acquisition System (MIDAS 10) Study Group . Weekend versus weekday admission and mortality from myocardial infarction. N Engl J Med 2007;356:1099-109. https://doi.org/10.1056/NEJMoa063355.
- Janszky I, Ahnve S, Ljung R. Weekend versus weekday admission and stroke outcome in Sweden from 1968 to 2005. Stroke 2007;38. https://doi.org/10.1161/STROKEAHA.107.491449.
- Palmer WL, Bottle A, Davie C, Vincent CA, Aylin P. Dying for the weekend: a retrospective cohort study on the association between day of hospital presentation and the quality and safety of stroke care. Arch Neurol 2012;69:1296-302. https://doi.org/10.1001/archneurol.2012.1030.
- Roberts SE, Thorne K, Akbari A, Samuel DG, Williams JG. Mortality following stroke, the weekend effect and related factors: record linkage study. PLOS ONE 2015;10. https://doi.org/10.1371/journal.pone.0131836.
- Rudd AG, Hoffman A, Down C, Pearson M, Lowe D. Access to stroke care in England, Wales and Northern Ireland: the effect of age, gender and weekend admission. Age Ageing 2007;36:247-55. https://doi.org/10.1093/ageing/afm007.
- Saposnik G, Baibergenova A, Bayer N, Hachinski V. Weekends: a dangerous time for having a stroke?. Stroke 2007;38:1211-15. https://doi.org/10.1161/01.STR.0000259622.78616.ea.
- Aylin P, Alexandrescu R, Jen MH, Mayer EK, Bottle A. Day of week of procedure and 30 day mortality for elective surgery: retrospective analysis of hospital episode statistics. BMJ 2013;346. https://doi.org/10.1136/bmj.f2424.
- Sharp AL, Choi H, Hayward RA. Don’t get sick on the weekend: an evaluation of the weekend effect on mortality for patients visiting US EDs. Am J Emerg Med 2013;31:835-7. https://doi.org/10.1016/j.ajem.2013.01.006.
- Luyt CE, Combes A, Aegerter P, Guidet B, Trouillet JL, Gibert C, et al. Mortality among patients admitted to intensive care units during weekday day shifts compared with ‘off’ hours. Crit Care Med 2007;35:3-11. https://doi.org/10.1097/01.CCM.0000249832.36518.11.
- Concha OP, Gallego B, Hillman K, Delaney GP, Coiera E. Do variations in hospital mortality patterns after weekend admission reflect reduced quality of care or different patient cohorts? A population-based study. BMJ 2013;23:215-22. https://doi.org/10.1136/bmjqs-2013-002218.
- Streifler J, Benderly M, Molshatzki N, Bornstein N, Tanne D. Off-hours admission for acute stroke is not associated with worse outcome – a nationwide Israeli stroke project. Eur J Neurol 2012;19:643-7. https://doi.org/10.1111/j.1468-1331.2011.03603.x.
- House of Commons . Oral Answers to Questions – Health. Col 147 2015. https://publications.parliament.uk/pa/cm201516/cmhansrd/cm151013/debtext/151013-0001.htm (accessed 28 March 2019).
- Goddard AF. Lessons to be learned from the UK junior doctors’ strike. JAMA 2016;316:1445-6. https://doi.org/10.1001/jama.2016.12029.
- NHS England . Seven Day Hospital Services – Our Ambition 2016. www.england.nhs.uk/ourwork/qual-clin-lead/seven-day-hospital-services/our-ambition/ (accessed 28 March 2019).
- NHS England . The NHS Long Term Plan 2019. https://www.longtermplan.nhs.uk/ (accessed 22 June 2020).
- Meacock R, Anselmi L, Kristensen SR, Doran T, Sutton M. Higher mortality rates amongst emergency patients admitted to hospital at weekends reflect a lower probability of admission. J Health Serv Res Policy 2017;22:12-9. https://doi.org/10.1177/1355819616649630.
- Needleman J, Buerhaus P, Pankratz VS, Leibson CL, Stevens SR, Harris M. Nurse staffing and inpatient hospital mortality. N Engl J Med 2011;364:1037-45. https://doi.org/10.1056/NEJMsa1001025.
- Hartley J, Bennington J. Leadership for Healthcare. Portland, OR: The Policy Press; 2010.
- Bray BD, Cloud GC, James MA, Hemingway H, Paley L, Stewart K, et al. Weekly variation in health-care quality by day and time of admission: a nationwide, registry-based, prospective cohort study of acute stroke care. Lancet 2016;388:170-7. https://doi.org/10.1016/S0140-6736(16)30443-3.
- Bray BD, Steventon A. Data linkage studies can help to explain the weekend effect. Lancet 2017;390:8-9. https://doi.org/10.1016/S0140-6736(17)31195-9.
- Kostis WJ, Moreyra AE. Severity of illness and the weekend effect. Lancet 2017;390:1734-5. https://doi.org/10.1016/S0140-6736(17)32642-9.
- Walker AS, Mason A, Quan TP, Fawcett NJ, Watkinson P, Llewelyn M, et al. Mortality risks associated with emergency admissions during weekends and public holidays: an analysis of electronic health records. Lancet 2017;390:62-7. https://doi.org/10.1016/S0140-6736(17)30782-1.
- Campbell JT, Bray BD, Hoffman AM, Kavanagh SJ, Rudd AG, Tyrrell PJ. Intercollegiate Stroke Working Party . The effect of out of hours presentation with acute stroke on processes of care and outcomes: analysis of data from the Stroke Improvement National Audit Programme (SINAP). PLOS ONE 2014;9. https://doi.org/10.1371/journal.pone.0087946.
- Perez I, Brown M, Pinchin J, Martindale S, Sharples S, Shaw D, et al. Out of hours workload management: Bayesian inference for decision support in secondary care. Artif Intell Med 2016;73:34-4. https://doi.org/10.1016/j.artmed.2016.09.005.
- Rogers AE, Hwang WT, Scott LD, Aiken LH, Dinges DF. The working hours of hospital staff nurses and patient safety. Health Aff 2004;23:202-12. https://doi.org/10.1377/hlthaff.23.4.202.
- Bell A, McDonald F, Hobson T. The ethical imperative to move to a seven-day care model. J Bioeth Inq 2016;13:251-60. https://doi.org/10.1007/s11673-016-9708-2.
- Sutton E, Bion J, Aldridge C, Boyal A, Willars J, Tarrant C. Quality and safety of in-hospital care for acute medical patients at weekends: a qualitative study. BMC Health Serv Res 2018;18. https://doi.org/10.1186/s12913-018-3833-z.
- Aldridge C, Bion J, Boyal A, Chen YF, Clancy M, Evans T, et al. Weekend specialist intensity and admission mortality in acute hospital trusts in England: a cross-sectional study. Lancet 2016;388:178-86. https://doi.org/10.1016/S0140-6736(16)30442-1.
- Conway R, Cournane S, Byrne D, O’Riordan D, Silke B. Improved mortality outcomes over time for weekend emergency medical admissions. Ir J Med Sci 2018;187:5-11. https://doi.org/10.1007/s11845-017-1627-7.
- Khoshchehreh M, Groves EM, Tehrani D, Amin A, Patel PM, Malik S. Changes in mortality on weekend versus weekday admissions for acute coronary syndrome in the United States over the past decade. Int J Cardiol 2016;210:164-72. https://doi.org/10.1016/j.ijcard.2016.02.087.
- Balinskaite V, Bottle A, Shaw LJ, Majeed A, Aylin P. Reorganisation of stroke care and impact on mortality in patients admitted during weekends: a national descriptive study based on administrative data. BMJ Qual Saf 2017;27:611-18. https://doi.org/10.1136/bmjqs-2017-006681.
- Morris S, Hunter RM, Ramsay AI, Boaden R, McKevitt C, Perry C, et al. Impact of centralising acute stroke services in English metropolitan areas on mortality and length of hospital stay: difference-in-differences analysis. BMJ 2014;349. https://doi.org/10.1136/bmj.g4757.
- London Strategic Clinical Networks . Stroke Acute Commissioning and Tariff Guidance 2014. www.londonscn.nhs.uk/wp-content/uploads/2015/01/Stroke-acutecommissioning-and-tariff-guidance-2014.pdf (accessed 22 June 2020).
- Fulop N, Boaden R, Hunter R, McKevitt C, Morris S, Pursani N, et al. Innovations in major system reconfiguration in England: a study of the effectiveness, acceptability and processes of implementation of two models of stroke care. Implement Sci 2013;8. https://doi.org/10.1186/1748-5908-8-5.
- Ramsay AI, Morris S, Hoffman A, Hunter RM, Boaden R, McKevitt C, et al. Effects of centralizing acute stroke services on stroke care provision in two large metropolitan areas in England. Stroke 2015;46:2244-51. https://doi.org/10.1161/STROKEAHA.115.009723.
- Healthcare for London . Stroke Acute Commissioning and Tariff Guidance 2009.
- Turner S, Ramsay A, Perry C, Boaden R, McKevitt C, Morris S, et al. Lessons for major system change: centralization of stroke services in two metropolitan areas of England. J Health Serv Res Policy 2016;21:156-65. https://doi.org/10.1177/1355819615626189.
- Morris S, Ramsay AIG, Boaden RJ, Hunter RM, McKevitt C, Paley L, et al. Impact and sustainability of centralising acute stroke services in English metropolitan areas: retrospective analysis of hospital episode statistics and stroke national audit data. BMJ 2019;364. https://doi.org/10.1136/bmj.l1.
- Fulop NJ, Ramsay AI, Hunter RM, McKevitt C, Perry C, Turner SJ, et al. Evaluation of reconfigurations of acute stroke services in different regions of England and lessons for implementation: a mixed-methods study. Health Serv Deliv Res 2019;7. https://doi.org/10.3310/hsdr07070.
- Lintern S. Analysed: Review Aims to Improve Stroke Services in the Midlands and East 2012. www.hsj.co.uk/hsj-local/briefing/midlands-and-east/analysed-review-aims-to-improve-stroke-services-in-the-midlands-and-east/5047149.article (accessed 10 January 2020).
- Williams D. Major Reconfiguration Plans Emerging Across England 2011. www.hsj.co.uk/acute-care/major-reconfiguration-plans-emerging-across-england/5037539.article (accessed 10 January 2020).
- Östlund U, Kidd L, Wengström Y, Rowa-Dewar N. Combining qualitative and quantitative research within mixed method research designs: a methodological review. Int J Nurs Stud 2011;48:369-83. https://doi.org/10.1016/j.ijnurstu.2010.10.005.
- Yin RK. Case Study Research: Design and Methods. London: SAGE Publications Ltd; 2009.
- Yin RK. Enhancing the quality of case studies in health services research. Health Serv Res 1999;34.
- Baker GR. The contribution of case study research to knowledge of how to improve quality of care. BMJ Qual Saf 2011;20:i30-5. https://doi.org/10.1136/bmjqs.2010.046490.
- Tong A, Sainsbury P, Craig J. Consolidated criteria for reporting qualitative research (COREQ): a 32-item checklist for interviews and focus groups. Int J Qual Health Care 2007;19:349-57. https://doi.org/10.1093/intqhc/mzm042.
- Marcus GE. Ethnography in/of the world system: the emergence of multi-sited ethnography. Annu Rev Anthropol 1995;24:95-117. https://doi.org/10.1146/annurev.an.24.100195.000523.
- Savage J. Ethnography and health care. BMJ 2000;321:1400-2. https://doi.org/10.1136/bmj.321.7273.1400.
- Royal College of Physicians . Sentinel Stroke National Audit Programme (SSNAP): Clinical Audit April–June 2015 Public Report 2015.
- Bath PM, Lees KR, Schellinger PD, Altman H, Bland M, Hogg C, et al. Statistical analysis of the primary outcome in acute stroke trials. Stroke 2012;43:1171-8. https://doi.org/10.1161/STROKEAHA.111.641456.
- Sentinel Stroke National Audit Programme (SSNAP) . SSNAP Dataset and Helpnotes 2017.
- Braun V, Clarke V. Using thematic analysis in psychology. Qual Res Psychol 2006;3:77-101. https://doi.org/10.1191/1478088706qp063oa.
- Turner M, Barber M, Dodds H, Dennis M, Langhorne P, Macleod MJ. Scottish Stroke Care Audit . Stroke patients admitted within normal working hours are more likely to achieve process standards and to have better outcomes. J Neurol Neurosurg Psychiatry 2016;87:138-43. https://doi.org/10.1136/jnnp-2015-311273.
- Brunot V, Landreau L, Corne P, Platon L, Besnard N, Buzançais A, et al. Mortality associated with night and weekend admissions to ICU with on-site intensivist coverage: results of a nine-year cohort study (2006–2014). PLOS ONE 2016;11. https://doi.org/10.1371/journal.pone.0168548.
- Li L, Rothwell PM. Oxford Vascular Study . Biases in detection of apparent ‘weekend effect’ on outcome with administrative coding data: population based study of stroke. BMJ 2016;353. https://doi.org/10.1136/bmj.i2648.
- Goddard AF, Lees P. Higher senior staffing levels at weekends and reduced mortality. BMJ 2012;344. https://doi.org/10.1136/bmj.e67.
- Bray BD, Ayis S, Campbell J, Cloud GC, James M, Hoffman A, et al. Associations between stroke mortality and weekend working by stroke specialist physicians and registered nurses: prospective multicentre cohort study. PLOS Med 2014;11. https://doi.org/10.1371/journal.pmed.1001705.
- Albright KC, Raman R, Ernstrom K, Hallevi H, Martin-Schild S, Meyer BC, et al. Can comprehensive stroke centers erase the ‘weekend effect’?. Cerebrovasc Dis 2008;27:107-13. https://doi.org/10.1159/000177916.
- Albright KC, Savitz SI, Raman R, Martin-Schild S, Broderick J, Ernstrom K, et al. Comprehensive stroke centers and the ‘weekend effect’: the SPOTRIAS experience. Cerebrovasc Dis 2012;34:424-9. https://doi.org/10.1159/000345077.
- McKinney JS, Deng Y, Kasner SE, Kostis JB. Myocardial Infarction Data Acquisition System (MIDAS 15) Study Group . Comprehensive stroke centers overcome the weekend versus weekday gap in stroke treatment and mortality. Stroke 2011;42:2403-9. https://doi.org/10.1161/STROKEAHA.110.612317.
- Royal College of Physicians . Sentinel Stroke National Audit Programme (SSNAP): Clinical Audit First Pilot Public Report 2013.
- Melnychuk M, Morris S, Black G, Ramsay AIG, Eng J, Rudd A, et al. Variation in quality of acute stroke care by day and time of admission: prospective cohort study of weekday and weekend centralised hyperacute stroke unit care and non-centralised services. BMJ Open 2019;9. https://doi.org/10.1136/bmjopen-2018-025366.
- Thompson SG, Barber JA. How should cost data in pragmatic randomised trials be analysed?. BMJ 2000;320:1197-200. https://doi.org/10.1136/bmj.320.7243.1197.
- Hunter RM, Davie C, Rudd A, Thompson A, Walker H, Thomson N, et al. Impact on clinical and cost outcomes of a centralized approach to acute stroke care in London: a comparative effectiveness before and after model. PLOS ONE 2013;8. https://doi.org/10.1371/journal.pone.0070420.
- Hunter RM, Fulop NJ, Boaden RJ, McKevitt C, Perry C, Ramsay AIG, et al. The potential role of cost-utility analysis in the decision to implement major system change in acute stroke services in metropolitan areas in England. Health Res Policy Syst 2018;16. https://doi.org/10.1186/s12961-018-0301-5.
- Curtis LA, Burns A. Unit Costs of Health and Social Care 2015. Canterbury: PSSRU, University of Kent; 2015.
- Emberson J, Lees KR, Lyden P, Blackwell L, Albers G, Bluhmki E, et al. Effect of treatment delay, age, and stroke severity on the effects of intravenous thrombolysis with alteplase for acute ischaemic stroke: a meta-analysis of individual patient data from randomised trials. Lancet 2014;384:1929-35. https://doi.org/10.1016/S0140-6736(14)60584-5.
- Fang J, Saposnik G, Silver FL, Kapral MK. Investigators of the Registry of the Canadian Stroke Network . Association between weekend hospital presentation and stroke fatality. Neurology 2010;75:1589-96. https://doi.org/10.1212/WNL.0b013e3181fb84bc.
- Reeves MJ, Smith E, Fonarow G, Hernandez A, Pan W, Schwamm LH. GWTG – Stroke Steering Committee and Investigators . Off-hour admission and in-hospital stroke case fatality in the Get with the Guidelines – stroke program. Stroke 2009;40:569-76. https://doi.org/10.1161/STROKEAHA.108.519355.
- Crowley RW, Yeoh HK, Stukenborg GJ, Medel R, Kassell NF, Dumont AS. Influence of weekend hospital admission on short-term mortality after intracerebral hemorrhage. Stroke 2009;40:2387-92. https://doi.org/10.1161/STROKEAHA.108.546572.
- Godlee F. What to do about the ‘weekend effect’. BMJ 2015;351. https://doi.org/10.1136/bmj.h4840.
- Patel AA, Mahajan A, Benjo A, Pathak A, Kar J, Jani VB, et al. A nationwide analysis of outcomes of weekend admissions for intracerebral hemorrhage shows disparities based on hospital teaching status. Neurohospitalist 2016;6:51-8. https://doi.org/10.1177/1941874415601164.
- Allen M, Pearn K, Villeneuve E, Monks T, Stein K, James M. Feasibility of a hyper-acute stroke unit model of care across England: a modelling analysis. BMJ Open 2017;7. https://doi.org/10.1136/bmjopen-2017-018143.
- Healthcare for London . The Shape of Things to Come: Appendix 7d (Finance Commissioning Assurance) 2009.
- NHS England . Five Year Forward View 2014. www.england.nhs.uk/wp-content/uploads/2014/10/5yfv-web.pdf (accessed 10 January 2020).
- NHS England . Next Steps on the NHS Five Year Forward View 2017. www.england.nhs.uk/wp-content/uploads/2017/03/NEXT-STEPS-ON-THE-NHS-FIVE-YEAR-FORWARD-VIEW.pdf (accessed 14 September 2017).
- Freemantle N, Ray D, McNulty D, Rosser D, Bennett S, Keogh BE, et al. Increased mortality associated with weekend hospital admission: a case for expanded seven day services?. BMJ 2015;351. https://doi.org/10.1136/bmj.h4596.
- Black N. Is hospital mortality higher at weekends? If so, why?. Lancet 2016;388:108-11. https://doi.org/10.1016/S0140-6736(16)30505-0.
- McKee M. The weekend effect: now you see it, now you don’t. BMJ 2016;353. https://doi.org/10.1136/bmj.i2750.
- Bray BD, Steventon A. What have we learnt after 15 years of research into the ‘weekend effect’?. BMJ Qual Saf 2017;26:607-10. https://doi.org/10.1136/bmjqs-2016-005793.
- Mathew A, Fyyaz SA, Carter PR, Potluri R. The enigma of the weekend effect. J Thorac Dis 2018;10:102-5. https://doi.org/10.21037/jtd.2017.12.115.
- Black GB, Ramsay AIG, Baim-Lance A, Eng J, Melnychuk M, Xanthopoulou P, et al. What does it take to provide clinical interventions with temporal consistency? A qualitative study of London hyperacute stroke units. BMJ Open 2019;9. https://doi.org/10.1136/bmjopen-2018-025367.
- Early Supported Discharge Trialists . Services for reducing duration of hospital care for acute stroke patients. Cochrane Database Syst Rev 2005;2. https://doi.org/10.1002/14651858.CD000443.
- Paley L, Williamson E, Bray BD, Hoffman A, James MA, Rudd AG. SSNAP Collaboration . Associations between 30-day mortality, specialist nursing, and daily physician ward rounds in a national stroke registry. Stroke 2018;49:2155-62. https://doi.org/10.1161/STROKEAHA.118.021518.
- Eltringham SA, Kilner K, Gee M, Sage K, Bray BD, Pownall S, et al. Impact of dysphagia assessment and management on risk of stroke-associated pneumonia: a systematic review. Cerebrovasc Dis 2018;46:99-107. https://doi.org/10.1159/000492730.
- Bray BD, Smith CJ, Cloud GC, Enderby P, James M, Paley L, et al. The association between delays in screening for and assessing dysphagia after acute stroke, and the risk of stroke-associated pneumonia. J Neurol Neurosurg Psychiatry 2017;88:25-30. https://doi.org/10.1136/jnnp-2016-313356.
- Orlikowski WJ, Yates J. It’s about time: temporal structuring in organizations. Organ Sci 2002;13:684-700. https://doi.org/10.1287/orsc.13.6.684.501.
- McGivern G, Dopson S, Ferlie E, Fischer M, Fitzgerald L, Ledger J, et al. The silent politics of temporal work: a case study of a management consultancy project to redesign public health care. Organ Stud 2017;39:1007-30. https://doi.org/10.1177/0170840617708004.
- Lutz BJ, Young ME, Cox KJ, Martz C, Creasy KR. The crisis of stroke: experiences of patients and their family caregivers. Top Stroke Rehabil 2011;18:786-97. https://doi.org/10.1310/tsr1806-786.
- Intercollegiate Stroke Working Party . National Clinical Guideline for Stroke 2012.
- Cooper DJ, Hinings B, Greenwood R, Brown JL, Cooper DJ, Hinings B, et al. Sedimentation and transformation in organizational change: the case of Canadian law firms. Organ Stud 1996;17:623-47. https://doi.org/10.1177/017084069601700404.
- McNulty T, Ferlie E. Process transformation: limitations to radical organizational change within public service organizations. Organ Stud 2004;25:1389-412. https://doi.org/10.1177/0170840604046349.
- Dixon-Woods M. Why is patient safety so hard? A selective review of ethnographic studies. J Health Serv Res Policy 2010;15:11-6. https://doi.org/10.1258/jhsrp.2009.009041.
- Joundi RA, Martino R, Saposnik G, Giannakeas V, Fang J, Kapral MK. Predictors and outcomes of dysphagia screening after acute ischemic stroke. Stroke 2017;48:900-6. https://doi.org/10.1161/STROKEAHA.116.015332.
- Nugus P, Holdgate A, Fry M, Forero R, McCarthy S, Braithwaite J. Work pressure and patient flow management in the emergency department: findings from an ethnographic study. Acad Emerg Med 2011;18:1045-52. https://doi.org/10.1111/j.1553-2712.2011.01171.x.
- Friebel R, Hauck K, Aylin P. Centralisation of acute stroke services in London: impact evaluation using two treatment groups. Health Econ 2018;27:722-32. https://doi.org/10.1002/hec.3630.
- Honeyford K, Cecil E, Lo M, Bottle A, Aylin P. The weekend effect: does hospital mortality differ by day of the week? A systematic review and meta-analysis. BMC Health Serv Res 2018;18. https://doi.org/10.1186/s12913-018-3688-3.
- Kirkup B. The Report of the Morecambe Bay Investigation: An Independent Investigation into the Management, Delivery and Outcomes of Care Provided by the Maternity and Neonatal Services at the University Hospitals of Morecambe Bay NHS Foundation Trust from January 2004 to June 2013 2015.
- Martin GP, McKee L, Dixon-Woods M. Beyond metrics? Utilizing ‘soft intelligence’ for healthcare quality and safety. Soc Sci Med 2015;142:19-26. https://doi.org/10.1016/j.socscimed.2015.07.027.
- Royal College of Physicians . Sentinel Stroke National Audit Programme (SSNAP): Acute Organisational Audit Report 2014 2014.
Appendix 1 COnsolidated criteria for REporting Qualitative studies (COREQ): 32-item checklist
Developed from Tong A, et al. 49
Item number | Guide questions/description | Reported on page number |
---|---|---|
Domain 1: research team and reflexivity | ||
Personal characteristics | ||
1. Interviewer/facilitator | Which author/s conducted the interview or focus group? | 14 |
2. Credentials | What were the researcher’s credentials (e.g. PhD, MD)? | 14 |
3. Occupation | What was their occupation at the time of the study? | 1 |
4. Gender | Was the researcher male or female? | 1 |
5. Experience and training | What experience or training did the researcher have? | 14 |
Relationship with participants | ||
6. Relationship established | Was a relationship established prior to study commencement? | 14 |
7. Participant knowledge of the interviewer | What did the participants know about the researcher (e.g. personal goals, reasons for doing the research)? | 14 |
8. Interviewer characteristics | What characteristics were reported about the interviewer/facilitator (e.g. bias, assumptions, reasons and interests in the research topic)? | 14 |
Domain 2: study design | ||
Theoretical framework | ||
9. Methodological orientation and Theory | What methodological orientation was stated to underpin the study (e.g. grounded theory, discourse analysis, ethnography, phenomenology, content analysis)? | 15 |
Participant selection | ||
10. Sampling | How were participants selected (e.g. purposive, convenience, consecutive, snowball)? | 14 |
11. Method of approach | How were participants approached (e.g. face to face, telephone, mail, e-mail)? | 14 |
12. Sample size | How many participants were in the study? | Table 3 (page 13) |
13. Non-participation | How many people refused to participate or dropped out? Reasons? | n/a, informal recruitment process |
Setting | ||
14. Setting of data collection | Where was the data collected (e.g. home, clinic, workplace)? | 14 |
15. Presence of non-participants | Was anyone else present besides the participants and researchers? | 14 |
16. Description of sample | What are the important characteristics of the sample (e.g. demographic data, date)? | Table 3 (page 13) |
Data collection | ||
17. Interview guide | Were questions, prompts, guides provided by the authors? Was it pilot tested? | 14, not pilot tested |
18. Repeat interviews | Were repeat interviews carried out? If yes, how many? | n/a, no repeat interviews |
19. Audio-/visual-recording | Did the research use audio or visual recording to collect the data? | 14 |
20. Field notes | Were field notes made during and/or after the interview or focus group? | 14 |
21. Duration | What was the duration of the interviews or focus group? | 14 |
22. Data saturation | Was data saturation discussed? | n/a, not relevant to this analytical method |
23. Transcripts returned | Were transcripts returned to participants for comment and/or correction? | n/a, not relevant to this analytical method |
Domain 3: analysis and findings | ||
Data analysis | ||
24. Number of data coders | How many data coders coded the data? | 15–16 |
25. Description of the coding tree | Did authors provide a description of the coding tree? | 15–16, 41 |
26. Derivation of themes | Were themes identified in advance or derived from the data? | 15–16, 41 |
27. Software | What software, if applicable, was used to manage the data? | 15–16 |
28. Participant checking | Did participants provide feedback on the findings? | n/a, not relevant to this method |
Reporting | ||
29. Quotations presented | Were participant quotations presented to illustrate the themes/findings? Was each quotation identified (e.g. participant number)? | Yes (e.g. page 43 and throughout) |
30. Data and findings consistent | Was there consistency between the data presented and the findings? | Yes, see Chapters 4 and 6 |
31. Clarity of major themes | Were major themes clearly presented in the findings? | Yes, see Chapters 4 and 6 |
32. Clarity of minor themes | Is there a description of diverse cases or discussion of minor themes? | Yes (e.g. page 47 last paragraph) |
List of abbreviations
- 24/7
- 24 hours a day, 7 days a week
- CI
- confidence interval
- CT
- computerised tomography
- ED
- emergency department
- HASU
- hyperacute stroke unit
- LOS
- length of stay
- MDT
- multidisciplinary team
- mRS
- modified Rankin Scale
- NIHSS
- National Institutes of Health Stroke Scale
- ONS
- Office for National Statistics
- SLT
- speech and language therapist
- SSNAP
- Sentinel Stroke National Audit Programme
- tPA
- tissue plasminogen activator
Notes
Supplementary material can be found on the NIHR Journals Library report page (https://doi.org/10.3310/hsdr08340).
Supplementary material has been provided by the authors to support the report and any files provided at submission will have been seen by peer reviewers, but not extensively reviewed. Any supplementary material provided at a later stage in the process may not have been peer reviewed.